- 1Finnish Red Cross Blood Service, Helsinki, Finland
- 2Stem Cell Transplantation Unit, Department of Hematology, Comprehensive Cancer Center, Helsinki University Hospital, Helsinki, Finland
- 3Stem Cell Transplantation Unit, Division of Medicine, Department of Hematology, Turku University Hospital, Turku, Finland
- 4Department of Human Genetics, McGill University and Genome Quebec Innovation Centre, McGill University, Montreal, QC, Canada
- 5Center for Pediatric Genomic Medicine, Children's Mercy, Kansas City, MO, United States
Genetic mismatches in protein coding genes between allogeneic hematopoietic stem cell transplantation (allo-HSCT) recipient and donor can elicit an alloimmunity response via peptides presented by the recipient HLA receptors as minor histocompatibility antigens (mHAs). While the impact of individual mHAs on allo-HSCT outcome such as graft-vs.-host and graft-vs.-leukemia effects has been demonstrated, it is likely that established mHAs constitute only a small fraction of all immunogenic non-synonymous variants. In the present study, we have analyzed the genetic mismatching in 157 exome-sequenced sibling allo-HSCT pairs to evaluate the significance of polymorphic HLA class I associated peptides on clinical outcome. We applied computational mismatch estimation approaches based on experimentally verified HLA ligands available in public repositories, published mHAs, and predicted HLA-peptide affinites, and analyzed their associations with chronic graft-vs.-host disease (cGvHD) grades. We found that higher estimated recipient mismatching consistently increased the risk of severe cGvHD, suggesting that HLA-presented mismatching influences the likelihood of long-term complications in the patient. Furthermore, computational approaches focusing on estimation of HLA-presentation instead of all non-synonymous mismatches indiscriminately may be beneficial for analysis sensitivity and could help identify novel mHAs.
Introduction
Allogeneic hematopoietic stem cell transplantation (allo-HSCT) presents a potentially curative treatment for a variety of malignant diseases and other serious disorders of the blood and hematopoietic system. The human leukocyte antigen (HLA) allele matching across several loci between the donor and recipient is a prerequisite for allo-HSCT to avoid lethal alloimmunity complications where the grafted T-cells mount an immune response against healthy recipient tissues (1). However, despite comprehensive HLA identity and advances in immunosuppressive medication, the graft-vs.-host disease (GvHD) remains a major cause of morbidity and mortality (2). On the other hand, alloreactivity directed against leukemic cells via the graft-vs.-leukemia (GvL) effect (3) is required for elimination of residual malignancy and curing the primary disease.
Following a HLA-matched allo-HSCT, the T-cell mediated alloimmunity is initiated mainly by non-HLA genetic differences encoding protein-level polymorphisms known as minor histocompatibility antigens (mHAs) (4). Common coding region genetic variability such as splicing variants (5), gene deletions (6), and non-synonymous single nucleotide variants (7) as well as de novo somatic mutations (8) can produce peptides that are presented by the HLA receptors on the cell surface and recognized by specific donor-derived T-cells as “non-self” epitopes. mHAs limited to the hematopoietic tissue are able elicit a highly specific GvL effect in allo-HSCT setting (9), while mHAs with broader and more varying expression profiles typically contribute to both GvL and GvHD (10). Since GvL can occur independent of GvHD, mHAs hold significant therapeutic potential for manipulating the alloimmunity (11). However, even though in vitro experimental analyses have identified several ligands for various alleles of different types of HLA receptors (12), only about 50 actual mHAs relevant for GvHD or GvL are currently known (13). Thus, extensive characterization of the mHA repertoire arising from germline or somatic genetic variability is expected to bring mHA targeted cell therapies closer to clinical application.
Due to the limited number of known mHAs, the extent of overall genome-wide non-synonymous mismatching has been proposed as a measure of alloreactivity potential in allo-HSCT recipients (14–16). In principle, separating the protein-coding mismatches by expression patterns could help assess the magnitude in and balance between GvHD and GvL. However, analysis of protein coding differences in related and unrelated allo-HSCTs has shown only weak association of estimated mismatching to the risk of severe acute GvHD (14). Further, in an alternative approach, computational prediction of HLA binding affinities of amino-acid altering genetic differences has shown a difference between related and unrelated allo-HSCTs (16), and has been reported to associate with GvHD risk (17). Studies analyzing the presence of known mismatched mHAs in allo-HSCT recipients have likewise identified small effects on GvHD risk and relapse-free survival (18–22). Given the various methodological approaches and that few of these associations remain statistically significant after multiple testing adjustment, further studies are warranted to investigate the graft alloimmunity capacity.
Here, we have analyzed genomic mismatching in 157 sibling allo-HSCT pairs to study the effects of HLA-presentation of non-synonymous variants on chronic GvHD. Based on previous reports, our hypothesis was that patients with severe cGvHD are more likely to harbor a higher number of mismatched HLA class I ligands than patients without cGvHD. Owing to the relatively small set of donor-recipient pairs in our study cohort, we have not considered acute GvHD since the number patients with severe form of this condition may not be adequate. Similarly, as the number of bone marrow expressed peptides is significantly smaller than peptides expressed in epithelial tissues, we have chosen not to focus on relapse or GvL. While the impact of predicted and experimental mHAs on GvHD have been studied previously by computational techniques, to our knowledge, existing databases of in vitro verified HLA ligands have not been employed in genomic studies of GvHD before. In contrast to mHAs, the relatively large amount of available experimental HLA ligands allows both statistical estimates with higher confidence and analyses in conjunction with other external data sets. Thus, comparison of this approach with established methods is needed to better understand alloreactivity and its computational modeling. To this end, we have carried out a computational analysis of class I HLA peptide binding affinity and immunogenicity potential, included epithelial protein expression data and enumerated the presence of experimentally verified HLA ligands and mHAs available in the public domain to evaluate the capability of these different approaches to estimating long-term alloreactivity capacity.
Methods
HSCT Pairs
The study cohort consisted of 157 Finnish HLA-matched sibling HSCT donors and recipients undergoing HSCT during the years 2001–2015 in two transplantation centers in Finland. The cohort and definitions of clinical outcomes have been described previously in detail (23). General characteristics of the cohort are given in Table 1. The study was approved by the Ethics Committees of Helsinki University Central Hospital and Turku University Central Hospital and the Finnish National Supervisory Authority for Welfare and Health (Valvira; https://www.valvira.fi/en/web/en). Informed and written consent was taken when possible, and in cases when it could not be asked (e.g., if the patient had deceased), a retrospective consent was given by the supervisory authority.
HLA-Typing and Matching
The extent of HLA matching between the sibling donor-recipient pairs has varied over time during the 1990s and the 2000s. Depending on a given time period, HLA-C, and/or HLA-DQB1 genes were genotyped only in the pairs with an HLA mismatch in any of the HLA-A, -B, -DRB1 genes, conforming with the European Federation of Immunogenetics (EBI) guidelines at the time. Thus, not all pairs were initially guaranteed to match over the six HLA genes (HLA-A, -B, -C, -DRB1, -DQB1, DPB1). Hence, all pairs were re-typed with NGS technology in this study.
HLA typing was performed as described previously (24). Briefly, the alleles of HLA-A, -B, -C, -DQB1, and -DRB1 genes were identified at the resolution level of the first field (i.e., allele group) in the HLA Laboratory of the Finnish Red Cross Blood Service using rSSO-Luminex technology (Labtype, One Lambda, Inc., CA, USA) and PCR-SSP (Micro SSP™ Generic HLA Class I/II DNA Typing Trays, One Lambda, Inc.; Olerup SSP® genotyping, Olerup SSP AB, Stockholm, Sweden). Two pairs with 5/6 HLA-match were confirmed by sequencing at the second field resolution level (i.e., amino acid level) (AlleleSEQR PCR/Sequencing kits, Atria Genetics, Hayward, CA, USA) using ABI 3130xl genetic analyzer (Applied Biosystems, Thermo Fisher Scientific, MA, USA). The results were analyzed with Assign 3.5+ software (Conexio Genomics Pty Ltd, Fremantle, Australia). The FASTQ read data of all pairs were analyzed using the Omixon Explore program version 1.2.0 (Omixon, Budapest, Hungary) to assign alleles at third field resolution level (i.e., allelic level) for HLA-A, -B, -C, -DRB1, -DQB1, -DPB1.
Exome Sequencing
Exome sequencing and quality control was performed as described previously (23). Briefly, a custom sequencing panel targeting the whole exome, full MHC region and active immunoregulatory regions was applied (25). The sequencing was performed with Illumina HiSeq 2000 instrument at the McGill Genome Center (McGill University, Montreal, Canada). The resulting reads were aligned against the human reference genome GRCh37/hg19 (25). Variant calling and quality filtering steps were carried out using the Genotype Analysis Tool Kit (GATK) v3.2–2 (26, 27), and further quality filtering was implemented by applying a hard cutoff on approximate read depth (DP) and genotype quality (GQ) parameter values obtained through comparing genotype similarities between technical replicates.
Experimental HLA Ligand Data
HLA ligand assay data was downloaded from the Immune Epitope Database (IEDB) website (www.iedb.org) (12) in October 2016. Assay results were filtered to include only ligands that originated from H. sapiens, had qualitative measure of “positive” or “positive-high” and had HLA allele information available. Duplicated peptides were removed. Minor histocompatibility antigen data set was obtained from published literature (13). Peptides longer than 9 amino acids were transformed into 9-mer format by extracting all 9-mer frames. Duplicated entries were removed.
Peptide Data Analyses
The analysis pipeline is summarized in Figure 1. Variant positions annotated as locating in a protein coding region and having a missense, insertion, deletion, frameshift, or stop relative to the GRCh37 reference genome were extracted from the sample VCF files. The GRCh37.75 fasta-formatted coding sequences were downloaded from the Ensembl FTP server (ftp://ftp.ensembl.org/pub/release-75/fasta/homo_sapiens/cds/Homo_sapiens.GRCh37.cds.all.fa.gz) (28, 29) and filtered by including only entries starting with the canonical ATG codon and having a length of at least 50 nucleotides. The non-synonymous variants in each sample were mapped to the filtered GRCh37 reference coding sequences followed by translation of the generated individual transcriptome of each subject into protein sequences. The in silico proteomes between each HSCT donor-recipient pair were compared to exclude identical protein sequences. Proteins with at least one amino acid difference were split into 9-mer peptides from all frames, and peptides unique to the recipient were extracted and included in further analyses.
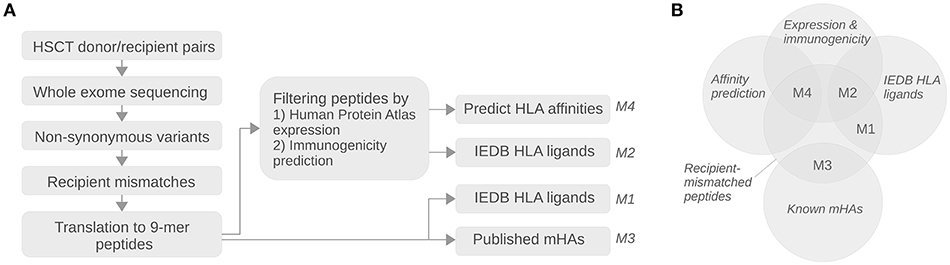
Figure 1. Schematic diagram of the analysis pipeline. (A) The vertical arrows in top-down direction in the diagram show the processing steps for translating whole exome sequencing data into recipient-mismatched peptide sets. The horizontal arrows in left-to-right direction show the analysis steps involved in sub-setting the mismatched peptides into sets relevant for alloreactivity; in the first step the peptides are filtered for epithelial expression and immunogenicity, and in the second step they are intersected with experimental HLA ligand databases or HLA affinity predictions according to each pair's HLA class I type. Finally, the obtained HLA-presented peptide count estimates from the four analysis approaches (labeled as M1–M4; Table 2) are examined for possible association with chronic GvHD. (B) Euler diagram showing the analysis methods M1–M4 as intersections between peptide sets.
HLA class I immunogenicity prediction on the peptides was performed with the IEDB (12) Immunogenicity-1.1 tool (30) without HLA type specification. Peptides with immunogenicity score >0.2 were selected for further analysis.
Expression levels for the filtered peptides were acquired from the Human Protein Atlas (HPA) database (31) v18/Ensembl v88.38. The data were filtered to include only proteins for which reliability was classified as supportive in skin, intestine, lung, liver or bone marrow tissue. The HPA data were mapped to the peptide sequence data based on Ensembl transcript and gene IDs.
The HLA-A and -B receptor binding affinity predictions to the unique peptides of the HSCT recipients were performed with the IEDB tool predict_binding.py v2.17 using the IEDB_recommended option which combines the results of multiple prediction algorithms (32–37). The consensus percentile rank with cutoff values of ≤ 4 or ≤ 6 were used in further analyses as a measure of number of high-affinity peptides in each recipient.
The sets of recipient-specific peptides obtained as described above were intersected with the filtered IEDB HLA ligand peptide sequences and published mHA peptide sequences to calculate the numbers of these HLA ligands in each recipient. Table 2 summarizes the four methods for estimating alloreactivity based on the above analyses of the peptide sets.
Statistical Methods
Statistical tests were carried out in R v3.4.4 (38) with custom scripts. Association between estimated ligand count measures and the chronic GvHD grades “none” vs. “extensive” was tested with logistic regression using the R function glm. P-values were calculated using the default Wald test. Three variables that could confound the estimates of the number of HLA-presented peptides were included as covariates in the regression analyses. The rationale was that rare HLA types may not be as well-covered as more common ones in the HLA ligand databases or HLA binding affinity training data, and can thus lead to under-representation of ligands in these types. Similarly, the total number of mismatches could mask the possible effect of HLA-presented peptides. Thus, the used covariates were: the sum of cohort frequencies of unique HLA types present in each recipient, the number of unique HLA types in each recipient, and the total number of mismatched peptides in each recipient. Furthermore, transplantation year, donor age and transplant direction (female-to-male vs. others) and the extent of matching over six HLA genes (HLA-A, -B, -C, -DRB1, -DQB1, -DPB1) for each HSCT pair were also included as covariates as these can be relevant for allo-HSCT outcome (22, 39). Each numerical predictor variable was centered and scaled. Benjamini–Hochberg adjusted p-value of <0.05 was considered significant. Data management and plotting were carried out using the R libraries tidyverse v1.2.1 (40), ggpubr v0.1.6 (41), seqminer v6.0 (42), data.table (43), and Biostrings v2.42.1 (44).
Code Availability
The analysis code is available in GitHub (https://github.com/FRCBS/HSCT-peptide).
Results
The results of the exome sequencing genotype calling and quality filtering steps have been described previously (23). To summarize, the obtained mean depth of read mapping coverage was 32.8 with standard deviation of 7.0 over all the samples. The donors had in total 468,426 quality filtered variants and the recipients had 470,135. The numbers of all 9-mer peptides generated from the recipient-mismatched non-synonymous variants had a mean of 872,895, standard deviation of 179,627, minimum of 281,009, and maximum of 1,583,366. Numbers of 9-mer peptides filtered for immunogenicity and HPA data had a mean of 28,146, standard deviation of 6,205, minimum of 8,523, and maximum of 51,425. On average, the number of unique peptides per sample with predicted high binding affinity was 39.5, ranging between 0 and 302 with a standard deviation of 46.9. Further details are given in Table 1.
HLA matching measured as the number of identical alleles between the donor-recipient pairs over the six genes (HLA-A, -B, -C, -DRB1, -DQB1, -DPB1) had a mean of 11.73 and a standard deviation of 1.31. 147, 3 2, and 5 patients had 12, 11, 10, and 9 or less matched alleles, respectively. To evaluate the HLA allele overlap between our data and the utilized external data sources, the HLA class I allele representation in the IEDB and mHA data sets was explored. For HLA-A, 96.8% of alleles in our cohort were found in the IEDB data and 78.7% in the mHA data. For HLA-B, the figures were 99.8 and 31.8%, respectively. 89.5% of HLA-C alleles were found in the IEDB data, and HLA-C was not represented in the mHA data.
Based on the sets of recipient-mismatched peptides, four approaches to estimating cGvHD related alloreactivity in sibling HSCT were evaluated as given in Table 2. The estimated ligand counts as given by the four approaches (termed M1–M4) are shown by the boxplots in Figure 2A. The results of logistic regression analysis between the estimates and cGvHD grades “no” vs. “extensive” (n = 77 and n = 51, respectively) are summarized by Figure 2B. The logistic regression model probabilities for severe cGvHD vs. recipient-mismatched ligand counts are shown by Figure 2C. Full logistic regression results are given in Table 3. The obtained nominal p-values for methods M1–M4 were 0.00976, 0.0234, 0.0366, and 0.36, respectively. After Benjamini–Hochberg FDR adjustment the values were 0.04, 0.046, 0.0493, and 0.36, respectively. Thus, M1–M3 remained significant (FDR <0.05). The obtained regression coefficients (log odds) and their 95% confidence intervals, respectively, were 0.639 (0.392–0.886), 0.507 (0.283–0.731), 0.457 (0.238–0.676), and 0.206 (−0.019–0.431), Thus, the methods M1–M4 were all consistent in their positive effect direction (Figure 2B). To evaluate the robustness of the M4 approach to parameter value selection, a less stringent cutoff value of ≤ 6 was also used. This did not significantly change the result as the obtained regression coefficient was 0.196.
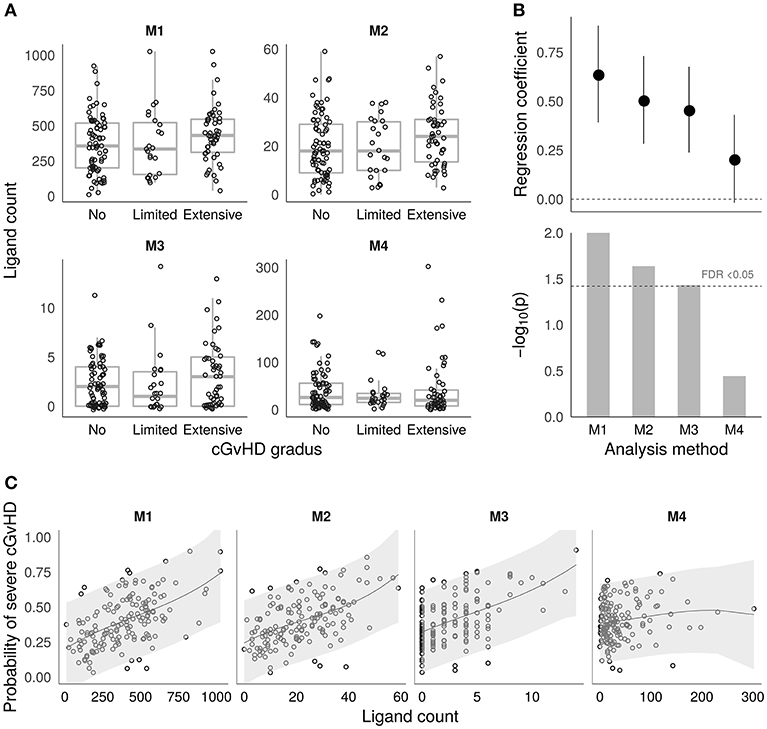
Figure 2. Alloimmunity estimates and associations with chronic GvHD. (A) Boxplots showing the distributions of the four different HLA ligand estimates (M1–M4) based on recipient-mismatched peptides (see the Methods section and Table 2 for a detailed description). The y-axis shows the estimated ligand count, and the x-axis shows the cGvHD grades. (B) Logistic regression coefficient estimates with ± 1 S.E. (top) and p-values (bottom) from the models testing the associations for cGvHD grades “no” (n = 77) vs. “extensive” (n = 51) in the four methods M1–M4. The coefficients are calculated based on scaled and centered ligand count values to make them comparable between M1–M4. The FDR <0.05 threshold is shown by the dashed horizontal line in the lower panel. (C) Estimated probabilities for severe chronic GvHD vs. the number of mismatched ligands as given by the fitted logistic regression models for M1–M4. The shaded areas visualize the 95% confidence intervals for prediction.
Discussion
Collectively, the results of the present study assessing the alloimmunity capacity in a sibling allo-HSCT setting suggest that the risk of extensive chronic GvHD is increased by a higher degree of recipient mismatching. Even though the observed effect was relatively weak, all four of the approaches applied to estimating the alloimmunity capacity were in agreement with regard to their effect direction, supporting the authenticity of the association with chronic GvHD and suggesting that these methods are able to measure relevant properties of recipient-mismatching peptides. This result is also consistent with studies analyzing the presence of sets of known recipient-mismatched mHAs in chronic GvHD (18, 21). However, a study by Martin et al. using the total sum of mismatches in coding variants as a measure of alloimmunity potential (14) reported an association for acute GvHD in sibling allo-HSCT, but found no association for chronic GvHD. This discrepancy could be due to differences in methodology, as in our study we focused solely on HLA-presentation of mismatching peptides rather than all non-synonymous mismatches. Synonymous variants in general have been recognized to affect gene expression through codon usage bias (45) and in this way may contribute to mismatching in allo-HSCT as well. In terms of disease mechanisms, it is established that the acute form of GvHD is triggered by strong cytokine storm as a result of leakage of lipopolysaccharides of commensal microbes from the intestinal lumen (46). Moreover, autosomal mHAs were not found to associate with acute GvHD, except for Y-chromosomal mHAs in female-to-male HSCT (22). Hence, the role of alloimmune differences between the donor and recipient may be secondary for acute GvHD, but could influence the risk for autoimmune-like chronic GvHD.
Out of the four methods applied for assessing the alloimmunity capacity, the in silico prediction of HLA peptide binding affinity and immunogenicity showed the weakest association. Although prediction of HLA binding for peptides originating from within known mHA-producing genes has been shown to allow discovering more potential mHAs in the same genes and correlate with GvHD risk (20), applied genome-wide, the computational prediction method did not significantly associate with clinical outcome. This could be due to the fact that the process of peptide cleavage and the formation of HLA-peptide complex are still incompletely understood and cannot be reliably modeled from sequence alone (47, 48). However, since in our analysis we did not model proteasomal cleavage or TAP transport independently of HLA affinity, their contribution to the overall prediction capacity of HLA presentation of peptides could not be assessed. In a genome-wide application, this approach could lead to a relatively high number of false positives or low sensitivity, and thus may not be ideal for assessing total alloimmunity capacity. Also, the choice of an inclusion threshold value for predicted high-affinity peptides could affect the analysis result, albeit our analysis with lower stringency threshold suggests that the approach is relatively robust for the choice of threshold.
Moreover, we were not able to find advantage in limiting the pool of analyzed recipient-mismatched and IEDB matched peptides to computationally predicted immunogenic and hematopoietic and epithelial tissue expressed molecules. Similarly to current methods of HLA affinity prediction, the immunogenicity algorithms may not be accurate enough for genome-wide application despite that these computational tools have proven to be useful when combined with an experimental proteogenomics approach that specifically targets HLA-associated peptides (47). On the other hand, the accuracy of immunogenicity estimate may benefit from masking of certain amino acid positions in particular alleles which was not implemented in our filtering step. Furthermore, the observation that utilizing all available IEDB ligands together with total recipient-mismatched peptides provided the best estimate suggests that there may be numerous, presently unknown mHAs relevant for alloimmunity (49).
While the method employing known mHAs showed an effect to the same direction as the full IEDB HLA ligand based approach, the effect was clearly smaller, which could be due to low numbers of mHA peptides in the set and limited to a few HLA alleles, reducing the signal-to-noise ratio and leading to lower statistical power. Our study is also limited by restricting the analysis exclusively to 9-mer peptides whereby it cannot address the effect of ligands presented by HLA class II receptors such as HLA-DR, -DB, and -DP (13). Thus, expanding the peptide length repertoire could improve particularly the results of the mHA based analysis, provided that sufficient HLA class II mHA data are available. Furthermore, the results we obtained for chronic GvHD may not be directly comparable with non-European populations owing both to our study cohort and to the IEDB and mHA data collections' focus on ligands from European subjects. Allele frequencies differing between populations (50) may impact the likelihood of finding matches in HLA ligand data sets.
In summary, the effects observed by us and others in similar studies emphasize the need for a comprehensive meta-analysis and large cohorts to gain further insight into the impact of non-HLA mismatches in allo-HSCT. In particular, it is essential to expand the knowledge of the mHA repertoire for the design of novel treatments and diagnostic tools for allo-HSCT complications. In this respect, it may be beneficial to employ computational approaches making use of available experimental HLA ligand data.
Data Availability
The datasets for this manuscript are not publicly available because: they contain unique identifying information of the patients. Requests to access the datasets should be directed to JP, anVra2EucGFydGFuZW5AYmxvb2RzZXJ2aWNlLmZp.
Ethics Statement
The study was approved by the Ethics Committees of Helsinki University Central Hospital and Turku University Central Hospital and the Finnish National Supervisory Authority for Welfare and Health (https://www.valvira.fi/en/web/en).
Author Contributions
JP and JR designed the study. SK managed the patient DNA samples and provided expertise on HLA typing. MP, AN, RN, MI-R, US, and LV managed and collected the clinical data on patients and provided clinical expertise. TK and TP performed the sequencing. KH, JR, and TK preprocessed the data. JR analyzed the data and wrote the manuscript.
Funding
This study was supported by the Academy of Finland grant 288393 and by the Finnish Funding Agency for Technology and Innovation (Tekes) to the Salwe GID (Personalized Diagnostics and Care) program (ID 3982/31/2013).
Conflict of Interest Statement
The authors declare that the research was conducted in the absence of any commercial or financial relationships that could be construed as a potential conflict of interest.
Acknowledgments
The authors thank CSC—IT Center for Science, Finland, for computational resources.
References
1. Furst D, Muller C, Vucinic V, Bunjes D, Herr W, Gramatzki M, et al. High-resolution HLA matching in hematopoietic stem cell transplantation: a retrospective collaborative analysis. Blood. (2013) 122:3220–9. doi: 10.1182/blood-2013-02-482547
3. Falkenburg JH, Warren EH. Graft versus leukemia reactivity after allogeneic stem cell transplantation. Biol Blood Marrow Transplant. (2011) 17:S33–8. doi: 10.1016/j.bbmt.2010.11.009
4. Robertson NJ, Chai JG, Millrain M, Scott D, Hashim F, Manktelow E, et al. Natural regulation of immunity to minor histocompatibility antigens. J Immunol. (2007) 178:3558–65. doi: 10.4049/jimmunol.178.6.3558
5. Broen K, Levenga H, Vos J, van Bergen K, Fredrix H, Greupink-Draaisma A, et al. A polymorphism in the splice donor site of ZNF419 results in the novel renal cell carcinoma-associated minor histocompatibility antigen ZAPHIR. PLoS ONE. (2011) 6:e21699. doi: 10.1371/journal.pone.0021699
6. McCarroll SA, Bradner JE, Turpeinen H, Volin L, Martin PJ, Chilewski SD, et al. Donor-recipient mismatch for common gene deletion polymorphisms in graft-versus-host disease. Nat Genet. (2009) 41:1341–4. doi: 10.1038/ng.490
7. Van Bergen CA, Rutten CE, Van Der Meijden ED, Van Luxemburg-Heijs SA, Lurvink EG, Houwing-Duistermaat JJ, et al. High-throughput characterization of 10 new minor histocompatibility antigens by whole genome association scanning. Cancer Res. (2010) 70:9073–83. doi: 10.1158/0008-5472.CAN-10-1832
8. Schumacher TN, Schreiber RD. Neoantigens in cancer immunotherapy. Science. (2015) 348:69–74. doi: 10.1126/science.aaa4971
9. Fontaine P, Roy-Proulx G, Knafo L, Baron C, Roy DC, Perreault C. Adoptive transfer of minor histocompatibility antigen-specific T lymphocytes eradicates leukemia cells without causing graft-versus-host disease. Nat Med. (2001) 7:789–94. doi: 10.1038/89907
10. de Bueger M, Bakker A, Van Rood JJ, Van der Woude F, Goulmy E. Tissue distribution of human minor histocompatibility antigens. ubiquitous versus restricted tissue distribution indicates heterogeneity among human cytotoxic T lymphocyte-defined non-MHC antigens. J Immunol. (1992) 149:1788–94.
11. Marijt WA, Heemskerk MH, Kloosterboer FM, Goulmy E, Kester MG, van der Hoorn MA, et al. Hematopoiesis-restricted minor histocompatibility antigens HA-1- or HA-2-specific T cells can induce complete remissions of relapsed leukemia. Proc Natl Acad Sci USA. (2003) 100:2742–7. doi: 10.1073/pnas.0530192100
12. Vita R, Overton JA, Greenbaum JA, Ponomarenko J, Clark JD, Cantrell JR, et al. The immune epitope database. (IEDB) 3.0. Nucleic Acids Res. (2015) 43:D405–12. doi: 10.1093/nar/gku938
13. Griffioen M, van Bergen CA, Falkenburg JH. Autosomal minor histocompatibility antigens: how genetic variants create diversity in immune targets. Front Immunol. (2016) 7:100. doi: 10.3389/fimmu.2016.00100
14. Martin PJ, Levine DM, Storer BE, Warren EH, Zheng X, Nelson SC, et al. Genome-wide minor histocompatibility matching as related to the risk of graft-versus-host disease. Blood. (2017) 129:791–8. doi: 10.1182/blood-2016-09-737700
15. Sampson JK, Sheth NU, Koparde VN, Scalora AF, Serrano MG, Lee V, et al. Whole exome sequencing to estimate alloreactivity potential between donors and recipients in stem cell transplantation. Br J Haematol. (2014) 166:566–70. doi: 10.1111/bjh.12898
16. Jameson-Lee M, Koparde V, Griffith P, Scalora AF, Sampson JK, Khalid H, et al. In silico derivation of HLA-specific alloreactivity potential from whole exome sequencing of stem-cell transplant donors and recipients: understanding the quantitative immunobiology of allogeneic transplantation. Front Immunol. (2014) 5:529. doi: 10.3389/fimmu.2014.00529
17. Koparde V, Abdul Razzaq B, Suntum T, Sabo R, Scalora A, Serrano M, et al. Dynamical system modeling to simulate donor T cell response to whole exome sequencing-derived recipient peptides: understanding randomness in alloreactivity incidence following stem cell transplantation. PLoS ONE. (2017) 12:e0187771. doi: 10.1371/journal.pone.0187771
18. Turpeinen H, Ojala PJ, Ojala K, Miettinen M, Volin L, Partanen J. Minor histocompatibility antigens as determinants for graft-versus-host disease after allogeneic haematopoietic stem cell transplantation. Int J Immunogenet. (2013) 40:495–501. doi: 10.1111/iji.12051
19. Hobo W, Broen K, van der Velden WJ, Greupink-Draaisma A, Adisty N, Wouters Y, et al. Association of disparities in known minor histocompatibility antigens with relapse-free survival and graft-versus-host disease after allogeneic stem cell transplantation. Biol Blood Marrow Transplant. (2013) 19:274–82. doi: 10.1016/j.bbmt.2012.09.008
20. Larsen ME, Kornblit B, Larsen MV, Masmas TN, Nielsen M, Thiim M, et al. Degree of predicted minor histocompatibility antigen mismatch correlates with poorer clinical outcomes in nonmyeloablative allogeneic hematopoietic cell transplantation. Biol Blood Marrow Transplant. (2010) 16:1370–81. doi: 10.1016/j.bbmt.2010.03.022
21. Spierings E, Kim YH, Hendriks M, Borst E, Sergeant R, Canossi A, et al. Multicenter analyses demonstrate significant clinical effects of minor histocompatibility antigens on GvHD and GvL after HLA-matched related and unrelated hematopoietic stem cell transplantation. Biol Blood Marrow Transplant. (2013) 19:1244–53. doi: 10.1016/j.bbmt.2013.06.001
22. Wang W, Huang H, Halagan M, Vierra-Green C, Heuer M, Brelsford JE, et al. Chromosome Y-encoded antigens associate with acute graft-versus-host disease in sex-mismatched stem cell transplant. Blood Adv. (2018) 2:2419–29. doi: 10.1182/bloodadvances.2018019513
23. Ritari J, Hyvarinen K, Koskela S, Itala-Remes M, Niittyvuopio R, Nihtinen A, et al. Genomic prediction of relapse in recipients of allogeneic haematopoietic stem cell transplantation. Leukemia. (2018) 33:240–8. doi: 10.1038/s41375-018-0229-3
24. Koskela S, Ritari J, Hyvarinen K, Kwan T, Niittyvuopio R, Itala-Remes M, et al. Hidden genomic MHC disparity between HLA-matched sibling pairs in hematopoietic stem cell transplantation. Sci Rep. (2018) 8:5396. doi: 10.1038/s41598-018-23682-y
25. Morin A, Kwan T, Ge B, Letourneau L, Ban M, Tandre K, et al. Immunoseq: the identification of functionally relevant variants through targeted capture and sequencing of active regulatory regions in human immune cells. BMC Med Genomics. (2016) 9:59. doi: 10.1186/s12920-016-0220-7
26. Van der Auwera GA, Carneiro MO, Hartl C, Poplin R, Del Angel G, Levy-Moonshine A, et al. From FastQ data to high confidence variant calls: the genome analysis toolkit best practices pipeline. Curr Protoc Bioinformatics. (2013) 43:11. doi: 10.1002/0471250953.bi1110s43
27. McKenna A, Hanna M, Banks E, Sivachenko A, Cibulskis K, Kernytsky A, et al. The genome analysis toolkit: a MapReduce framework for analyzing next-generation DNA sequencing data. Genome Res. (2010) 20:1297–303. doi: 10.1101/gr.107524.110
28. Flicek P, Amode MR, Barrell D, Beal K, Billis K, Brent S, et al. Ensembl 2014. Nucleic Acids Res. (2014) 42:D749–55. doi: 10.1093/nar/gkt1196
29. Zerbino DR, Achuthan P, Akanni W, Amode MR, Barrell D, Bhai J, et al. Ensembl 2018. Nucleic Acids Res. (2018) 46:D754–61. doi: 10.1093/nar/gkx1098
30. Calis JJ, Maybeno M, Greenbaum JA, Weiskopf D, De Silva AD, Sette A, et al. Properties of MHC class I presented peptides that enhance immunogenicity. PLoS Comput Biol. (2013) 9:e1003266. doi: 10.1371/journal.pcbi.1003266
31. Uhlen M, Fagerberg L, Hallstrom BM, Lindskog C, Oksvold P, Mardinoglu A, et al. Proteomics. tissue-based map of the human proteome. Science. (2015) 347:1260419. doi: 10.1126/science.1260419
32. Bui HH, Sidney J, Peters B, Sathiamurthy M, Sinichi A, Purton KA, et al. Automated generation and evaluation of specific MHC binding predictive tools: ARB matrix applications. Immunogenetics. (2005) 57:304–14. doi: 10.1007/s00251-005-0798-y
33. Sidney J, Assarsson E, Moore C, Ngo S, Pinilla C, Sette A, et al. Quantitative peptide binding motifs for 19 human and mouse MHC class I molecules derived using positional scanning combinatorial peptide libraries. Immunome Res. (2008) 4:2. doi: 10.1186/1745-7580-4-2
34. Stranzl T, Larsen MV, Lundegaard C, Nielsen M. NetCTLpan: pan-specific MHC class I pathway epitope predictions. Immunogenetics. (2010) 62:357–68. doi: 10.1007/s00251-010-0441-4
35. Peters B, Sette A. Generating quantitative models describing the sequence specificity of biological processes with the stabilized matrix method. BMC Bioinformatics. (2005) 6:132. doi: 10.1186/1471-2105-6-132
36. Karosiene E, Lundegaard C, Lund O, Nielsen M. NetMHCcons: a consensus method for the major histocompatibility complex class I predictions. Immunogenetics. (2012) 64:177–86. doi: 10.1007/s00251-011-0579-8
37. Nielsen M, Lundegaard C, Worning P, Lauemoller SL, Lamberth K, Buus S, et al. Reliable prediction of T-cell epitopes using neural networks with novel sequence representations. Protein Sci. (2003) 12:1007–17. doi: 10.1110/ps.0239403
38. R Core Team. R: A Language and Environment for Statistical Computing. R package version 3.3.3. Vienna (2017).
39. Nakasone H, Tian L, Sahaf B, Kawase T, Schoenrock K, Perloff S, et al. Allogeneic HY antibodies detected 3 months after female-to-male HCT predict chronic GVHD and nonrelapse mortality in humans. Blood. (2015) 125:3193–201. doi: 10.1182/blood-2014-11-613323
40. Wickham H. Tidyverse: Easily Install and Load the ‘Tidyverse'. R package version 1.2.1. Houston, TX (2017).
41. Kassambara A. ggpubr: ‘ggplot2' Based Publication Ready Plots. R package version 0.1.6. Marseille (2017).
42. Zhan X, Liu DJ. SEQMINER: an R-package to facilitate the functional interpretation of sequence-based associations. Genet Epidemiol. (2015) 39:619–23. doi: 10.1002/gepi.21918
44. Pages H, Aboyoun P, Gentleman R, DebRoy S. Biostrings: String Objects Representing Biological Sequences, and Matching Algorithms. R package version 2.42.1. Seattle, WA (2017).
45. Plotkin JB, Kudla G. Synonymous but not the same: the causes and consequences of codon bias. Nat Rev Genet. (2011) 12:32–42. doi: 10.1038/nrg2899
46. Ferrara JL, Levine JE, Reddy P, Holler E. Graft-versus-host disease. Lancet. (2009) 373:1550–61. doi: 10.1016/S0140-6736(09)60237-3
47. Granados DP, Sriranganadane D, Daouda T, Zieger A, Laumont CM, Caron-Lizotte O, et al. Impact of genomic polymorphisms on the repertoire of human MHC class I-associated peptides. Nat Commun. (2014) 5:3600. doi: 10.1038/ncomms4600
48. Nielsen M, Lund O, Buus S, Lundegaard C. MHC class II epitope predictive algorithms. Immunology. (2010) 130:319–28. doi: 10.1111/j.1365-2567.2010.03268.x
49. Granados DP, Rodenbrock A, Laverdure JP, Cote C, Caron-Lizotte O, Carli C, et al. Proteogenomic-based discovery of minor histocompatibility antigens with suitable features for immunotherapy of hematologic cancers. Leukemia. (2016) 30:1344–54. doi: 10.1038/leu.2016.22
Keywords: whole-exome sequencing, HSCT, HLA, minor histocompatibility antigen, genomics, graft-vs.-host
Citation: Ritari J, Hyvärinen K, Koskela S, Niittyvuopio R, Nihtinen A, Salmenniemi U, Putkonen M, Volin L, Kwan T, Pastinen T, Itälä-Remes M and Partanen J (2019) Computational Analysis of HLA-presentation of Non-synonymous Recipient Mismatches Indicates Effect on the Risk of Chronic Graft-vs.-Host Disease After Allogeneic HSCT. Front. Immunol. 10:1625. doi: 10.3389/fimmu.2019.01625
Received: 20 March 2019; Accepted: 01 July 2019;
Published: 16 July 2019.
Edited by:
Katharina Fleischhauer, Essen University Hospital, GermanyReviewed by:
Paul Joseph Martin, Fred Hutchinson Cancer Research Center, United StatesAmir Ahmed Toor, Virginia Commonwealth University, United States
Copyright © 2019 Ritari, Hyvärinen, Koskela, Niittyvuopio, Nihtinen, Salmenniemi, Putkonen, Volin, Kwan, Pastinen, Itälä-Remes and Partanen. This is an open-access article distributed under the terms of the Creative Commons Attribution License (CC BY). The use, distribution or reproduction in other forums is permitted, provided the original author(s) and the copyright owner(s) are credited and that the original publication in this journal is cited, in accordance with accepted academic practice. No use, distribution or reproduction is permitted which does not comply with these terms.
*Correspondence: Jarmo Ritari, amFybW8ucml0YXJpQGJsb29kc2VydmljZS5maQ==