- 1Molecular and Experimental Mycobacteriology, Research Center Borstel, Borstel, Germany
- 2German Center for Infection Research (DZIF), Partner Site Borstel-Hamburg-Lübeck-Riems, Hamburg, Germany
- 3Cluster of Excellence Precision Medicine in Chronic Inflammation, Kiel, Germany
- 4Evolutionary Ecology and Genetics, Zoological Institute, Christian-Albrechts-Universität, Kiel, Germany
- 5Section of Evolutionary Medicine, Institute for Experimental Medicine, Kiel University and Max Planck Institute for Evolutionary Biology, Plön, Germany
- 6Department of Internal Medicine I, University Hospital Schleswig-Holstein, Kiel, Germany
The rise of antimicrobial resistance (AMR) in bacterial pathogens is acknowledged by the WHO as a major global health crisis. It is estimated that in 2050 annually up to 10 million people will die from infections with drug resistant pathogens if no efficient countermeasures are implemented. Evolution of pathogens lies at the core of this crisis, which enables rapid adaptation to the selective pressures imposed by antimicrobial usage in both medical treatment and agriculture, consequently promoting the spread of resistance genes or alleles in bacterial populations. Approaches developed in the field of Evolutionary Medicine attempt to exploit evolutionary insight into these adaptive processes, with the aim to improve diagnostics and the sustainability of antimicrobial therapy. Here, we review the concept of evolutionary trade-offs in the development of AMR as well as new therapeutic approaches and their impact on host-microbiome-pathogen interactions. We further discuss the possible translation of evolution-informed treatments into clinical practice, considering both the rapid cure of the individual patients and the prevention of AMR.
Introduction
The evolution of antimicrobial resistance (AMR) in bacteria by mutation of the chromosome or horizontal gene transfer is a naturally occurring phenomenon that can be observed even in the absence of human interventions (1, 2). However, it is the widespread use of antibiotics for almost a century that led in the actual public health crisis. Indeed, numerous pathogens show an increase in their antimicrobial resistance (AMR) levels, often directed at multiple antibiotic drugs (i.e., multidrug resistance, MDR). Infections caused by MDR pathogens are difficult, if not impossible to treat with the most commonly used drugs, thereby requiring the application of less effective and/or more toxic regimens. By the year 2050, more patients are expected to die from infections with MDR pathogens (10 million/year) than from cancer today (8.2 million/year) (3). Current treatment practices, exemplified by proverbs such as “One size fits all” and “Hit hard and hit early” at least partially contributed to this scenario, and are unlikely to suffice for the challenges that medicine will face in the future (4).
The field of Evolutionary Medicine strives to combat increasing drug resistance rates by exploiting evolutionary principles of resistance emergence and spread to design new treatment concepts (5, 6). The aims are 3-fold: (i) reducing intra-patient resistance selection that can lead to treatment failure, (ii) providing more rapid and less toxic cures to individual patients, and (iii) reducing the likelihood of AMR evolution and transmission at the population level. Achieving these aims is particularly challenging, because individualized patient care and public health considerations do not necessarily align. In particular, treatment regimens that were conventionally optimized to eliminate an infection as fast as possible impose a strong, often monotonic selective pressure on causal pathogens. Depending on bacterial adaptive capabilities, this can potentiate the risk for both short- and long-term resistance development (7). Furthermore, a broad activity of antimicrobials, which is often required for effective empirical anti-infective treatment, can compromise the healthy microbiome and thereby bears a risk for the development or aggravation of secondary diseases (8). Thus, we need to carefully consider evolutionary trajectories of pathogens in response to drug therapy, host immune system, as well as within a complex ecosystem of commensal microbial communities (Figure 1). In this context, Evolutionary Medicine is inevitably connected to applied Precision Medicine, where patients are stratified based on host, microbiome and pathogen characteristics, in order to deliver the most effective and sustainable treatment for a particular patient or group (9).
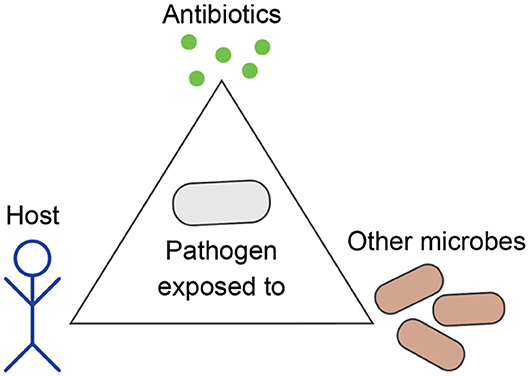
Figure 1. Evolution of bacterial pathogens during infection is influenced by the host's characteristics, other microbes, and antibiotics.
Here, we review (1) principles of bacterial resistance evolution and currently proposed concepts of evolution-informed therapies, (2) related challenges and opportunities, particularly with regard to host-microbiome-pathogen interactions, and (3) their relevance for clinical practice and Precision Medicine in the future.
Principles of Drug Resistance and Evolution Informed Therapies
Drug Resistance Mechanisms and Possible Fitness Costs
Throughout the course of evolution, molecules with antimicrobial activity were naturally selected in different organisms in order to outcompete bacteria in their environment by targeting essential functions such as DNA replication, transcription, translation, or metabolism (10). Although drug resistance evolution represents a paramount example of evolution observable in real-time, the design of antibiotic treatment regimens most often ignores the obtained insights into the involved selective processes (11). In fact, resistance evolving under antibiotic treatment in humans was traditionally seen as a phenomenon in individual patients (12, 13). Even if resistance-mediating adaptations arise in this context, their overall effect is assumed to be detrimental and changes may lead to lower fitness, so that resistant strains would usually not outcompete their susceptible counterparts (13). Thus, treatment designs over the past decades mostly focused on the principle of “Hit hard and hit early,” which goes back to the pioneering work of Paul Ehrlich from over 100 years ago (“frapper fort et frapper vite”) (14). Interestingly, Ehrlich already recognized the “great power of adaptation” and the risk for relapse and resistance evolution if a small number of pathogenic bacteria survives treatment (15). In fact, within the last decades, several pathogens acquired MDR, which prevailed under constant antibiotic selective pressure in hospitals and maintained transmissibility in the population (9).
One explanation for the success of AMR pathogens is the selection of low-cost or even no-cost resistance mediating mutations as shown for pathogens of the Mycobacterium tuberculosis complex (Mtbc), the causative agent of tuberculosis. The most prevalent mutations that mediate MDR, i.e., resistance against the widely used anti-tuberculous drugs isoniazid (katG p.S315T) and rifampicin (rpoB p.S450L), confer almost no fitness cost compared to wildtype strains (16, 17). Thus, even in the absence of the selective pressure of antibiotic therapy, MDR Mtbc strains persist and are associated with MDR tuberculosis outbreaks mainly in Eastern Europe, Central Asia, and South Africa (18, 19). Some resistance mechanisms, especially efflux pumps, are under extensive transcriptional control and can be amplified while antibiotics are present, so that any associated fitness costs are removed once resistance can be scaled back (20–22). Unlike Mtbc strains that evolve purely clonally, other bacteria such as Pseudomonas aeruginosa, an opportunistic pathogen in chronic lung infections, can acquire drug resistance not only by mutation, but also via horizontal gene transfer (HGT), e.g., involving plasmids (23). Such mobile resistance elements allow for the loss of AMR-mediating genes in the absence of antibiotic exposure, possibly attenuating AMR-associated fitness costs (24).
Another important evolutionary phenomenon observed in a number of AMR bacteria is compensatory evolution. Compensatory mutations are secondary mutations that reduce or ameliorate the fitness costs by the resistance mutation itself (25, 26) and have been described in a number of bacterial species in vitro (27, 28), and in vivo (28, 29), and have been associated with enhanced transmissibility (18, 19). Compensatory mutations can be clinically relevant, as they maintain resistance alleles and/or resistant clones in bacterial populations.
Beyond genetic mechanisms that allow bacteria to resist antibiotics or compensate for associated fitness costs, several species are able to express specific traits that protect them against antibiotics. P. aeruginosa, for example, forms biofilms and drug tolerant persister cells, leading to phenotypic resistance, often associated with chronic infection and relapse in patients with cystic fibrosis (30). M. tuberculosis has a unique thick cell wall and evolved a life cycle that mostly takes place in a granuloma (i.e., a concise structure of immune cells), which maintains the pathogen locally at the site of infection (e.g., the lung tissue) and shields from antibiotic penetration (31). Mtbc strains can also shift to a dormant state that reduces metabolic activity and increases the resistance to host/environmental stresses, including antibiotics (32).
These examples illustrate that drug resistance is a multifaceted evolutionary process, and that different pathogens have evolved diverse strategies which cause the traditional assumptions of antimicrobial therapy to fail. An in-depth understanding of these mechanisms may help to refine anti-infective therapies with currently available drugs, for example by focusing on antimicrobials, for which resistance comes at high costs (9).
From a public health perspective, resistance, and related bacterial fitness have to be extended to a broader context in which the (environmental) transmissibility of human pathogens and man-made selective pressures due to population-level antibiotic usage and its environmental distribution are considered (33). Moreover, the optimization of treatment strategies always has to be aligned with public health programs, in order to take account of insufficient disease control and surveillance measures as well as poor pharmaceutical control (e.g., availability of antibiotics in supermarkets), as observed in some structurally developing regions (34).
Synergistic and Antagonistic Combination Treatments
MDR infections are often treated with drug combinations. Such combination therapy follows the rationale that the simultaneous emergence of resistance against employed drugs is less likely than that against single drugs. In detail, resistance against a hypothetical drug A (conferred by a mutation) may arise rapidly by chance, depending on the mutation frequency and the population size of the infecting pathogen. In contrast, the combined probability of the simultaneous occurrence of mutations conferring resistance against two different drugs A and B in any one cell is very low, not considering cross-resistance.
Combination therapy is often designed to enhance treatment efficacy by combining drugs that mutually enhance each other. A hypothetical drug pair A and B usually interact additively. Each drug individually may inhibit bacterial growth by 50% at a given concentration, in combination a theoretical growth reduction of 75% (residual growth = 0.5 × 0.5) is expected. The effect on growth can further be influenced by physiological interactions, so that antibiotic combinations become antagonistic (combination causes less growth reduction than expected based on the additive effect) or synergistic (combination leads to a higher growth reduction than expected) (35). Synergistic drug combinations that mutually improve their therapeutic effects should enhance treatment efficacy. Counterintuitively, however, several studies suggested that in the long-term they may rather promote the spread of resistance, possibly as a consequence of competitive release (i.e., the stronger effect eliminates competitors so that the surviving resistant variants obtain enhanced access to resources) (36–39).
Combination treatment may also select for mutations that confer resistance to both drugs simultaneously, instead of a sequence of individual drug resistances. De-repression of efflux pump expression is one example (40). While efflux pumps are generally upregulated under cellular stress, leading to temporary drug resistance, this upregulation can be made permanent through loss-of-function variants of repressor genes, as repeatedly observed in clinical isolates (41).
A recent systematic analysis of combination therapy in P. aeruginosa suggests that the picture is more complex. A comprehensive set of more than 1,600 evolution experiments and 38 distinct combination treatments demonstrates that synergistic antibiotic combinations increase the extinction of bacterial populations, even if drugs are used at sub-lethal level, while no clear effect on adaptation rates was observed in the surviving populations (42). Instead, evolutionary trade-offs appear to be the prime determinant of combination efficacy, in particular when these trade-offs take the form of evolved collateral sensitivities (see below), leading to significant reductions in adaptation rates, at least under the experimental conditions (42).
Sequential Treatments
Sequential antibiotic treatment refers to the administration of different drugs in a chronological sequence to an individual patient, rather than their simultaneous combination, and in distinction to hospital drug rotation protocols (6).
Fundamentally, antibiotic monotherapy and sequential treatment both expose the patient and the infectious agent to one drug at a time. Evolutionary theory and in vitro experiments predict that bacteria are often able to adapt easily to a singular, continuous selective pressure (6, 43, 44). Consequently, in vivo observations confirm that resistance evolution indeed can happen within few days within patients (45). Antibiotic resistance phenotypes may be present at low frequencies in the infecting population or arise through spontaneous mutation, and subsequent selection by the antibiotic pressure (46). In a sequential treatment, however, selection of resistant subpopulations is repeatedly interrupted by changing the selective pressure. This strategy alone is able to slow the evolution of resistance down (47), and can be further amplified by limiting bacterial resistance evolution itself, through collateral sensitivity and negative hysteresis.
Collateral sensitivity (CS) was first described in the early antibiotic era (48), and has recently resurfaced as a promising strategy to limit bacterial resistance evolution (49). The effect results from an evolutionary trade-off, in which a mutation conferring resistance to one drug simultaneously causes increased sensitivity to another drug. The phenomenon of CS has now been described for a variety of bacterial taxa, such as Acinetobacter baumannii, Eschericia coli, Enterococcus faecalis, P. aeruginosa, or Staphylococcus aureus. It's strength and prevalence might depend however on the drug sequence, the bacterial species, and the evolutionary lineage within a bacterial species (44, 49–56). Overall, CS shows high potential for precision medicine to tailor treatments specific to a pathogen or even a specific strain (49, 57).
Whilst CS is based on changes in molecular structure (genetic mutations) occurring during resistance evolution, the concept of negative hysteresis (NH) results from a physiological response. In particular, NH occurs when the application of one antibiotic increases the efficacy of a second, subsequently applied antibiotic (58). Thus, increased susceptibility is not based on mutation and selection, but caused by physiological changes within a bacterial generation, even at sub-lethal antibiotic concentrations. NH may enhance the efficacy of sequential treatments, even at locations of poor drug penetration (e.g., biofilms) or with elevated levels of resistance (58).
Host-Microbiome-Pathogen Interaction
In medical practice and research, pathogens are often studied as stand-alone entities that grow independently from other microbes and evolve antibiotic resistance as an adaptation to anti-infective therapy. However, pathogens are part of a complex ecosystem within the host, which can greatly influence the outcome of anti-infective treatment and the occurrence of AMR. During infection, pathogens evolve not only to resist antibiotics, but also to increase their fitness within the particular host in competition to the commensal microbiota, which needs to be taken into account when applying evolutionary approaches to medicine (Figure 1).
The spectrum of colonizing bacteria can be influenced by a variety of host mechanisms. For example, gastrointestinal glycans such as those generated by the enzyme encoded by the blood-group related gene B4galnt2 were shown to be involved in shaping the resident microbiota (59) and, importantly, play a role in susceptibility to infection, e.g., by Salmonella (60).
Moreover, bacteria are forced to interact with other microbes upon colonization or infection of the host. In the process, commensal microbes impact the eco-evolutionary dynamics by affecting traits under selection (61). For example, mono-colonization of the mouse gut by E. coli can lead to predictable bacterial metabolic adaptations through the selection of mutations in genes related to amino acid metabolism, a process that is, however, altered by co-colonization with other microbes (61). Another example is colonization resistance, where presence of a complex residing microbial community can protect against pathogen infections (62).
Importantly, host-microbe and microbe-microbe interactions translate to ecological and evolutionary pressures that exhibit clinical relevance. These eco-evolutionary mechanisms affect both symbiotic organisms and pathogens, making their understanding key to sustainable pathogen control.
Furthermore, several known forms of bacterial interaction are directly linked to antimicrobial resistance.
Bacterial Co-operation
During infection, pathogens can engage in cooperative behavior. For example, P. aeruginosa in the lungs of cystic fibrosis patients acquires iron via the siderophore pyoverdin, which is costly to produce. Pyoverdin is secreted into the bacterial environment where it represents a public good: Either it gets taken back up in its iron-loaded form by the secreting cell, thereby offsetting the cost of its production, or by a non-pyoverdin-producing bacterium, which thereby benefits from iron uptake without paying the cost of siderophore production (63–65). Resistance to β-lactam antibiotics can be mediated in a similar cooperative manner: secreted β-lactamases act as a public good by simultaneously protecting bacteria that do not produce the enzyme (66–68).
Bacterial Competition
Bacteria-bacteria killing is a form of bacterial competition and can be mediated by the type VI secretion system (T6SS). In patients with A. baumanii infection, however, some bacterial isolates display an inactivated bacterial killing machinery whilst carrying several antimicrobial resistance genes (69). In these isolates, a large, self-transmissible plasmid encoded genes for resistance toward antibiotics ranging from beta-lactams to chloramphenicol, whilst carrying a negative regulator of T6SS. Only bacteria that could suppress their T6SS (thereby reducing elimination of surrounding bacteria) were able to successfully transmit the AMR-encoding plasmid containing the T6SS suppressor to other microbes, additionally facilitating the transmission of other AMR-encoding plasmids (70). Thus, a mechanistic linkage to bacteria-bacteria killing mechanisms can directly affect the spread of AMR.
Bacterial Communities
Within an infected host, individual bacteria usually grow as part of communities with specific composition and spatial organization. In the example of P. aeruginosa in cystic fibrosis lungs, isolates from one particular site of a patient's lung can show resistance to a particular antibiotic, whereas bacteria from other sites of the same lung do not (71). Laboratory studies supported by computational modeling previously detected different levels of AMR depending on the density of the community and the levels of bacterial mixing (72, 73). As another example, E. coli may either tolerate higher concentrations of antibiotics (74) or, alternatively, be prevented to evolve resistance when embedded in a diverse bacterial community, highlighting the importance of bacterial communities for AMR of pathogens (75).
In a nutshell, bacterial pathogens are part of complex ecological environments, where their evolutionary dynamics are heavily affected by host-microbe and bacteria-bacteria interactions with cooperation, competition and community organization directly affecting resistance to antimicrobials. Looking ahead, the challenge will be to incorporate these aspects of a host's ecology and evolutionary history, and bacterial life into our understanding of AMR to inform therapy design.
Relevance for Clinical Practice and Precision Medicine
Currently favored policies to counter AMR foremost focus on limiting the use of antibiotics to necessary medical treatments and encouraging the development of new antimicrobial substances (76). Whilst these measures are self-evident, they are not sufficient to provide a sustainable solution to the problem on their own. Evolutionary adaptation is at the very core of the bacterial AMR crisis. Therefore, the development of future treatment strategies against bacterial infections should necessarily follow evolutionary principles. Newly developed antimicrobial substances will at most provide a temporary relief, as evolution is a continuously ongoing process and almost certainly, resistance will eventually emerge against any new substance. Approaches following the “hit hard and hit early” principle, present since the beginning of the antibiotic era, need to be refined, taking into account individual characteristics of the infecting pathogen lineages, the affected patient, and the associated microbiota. Especially chronic recurring infections (such as P. aeruginosa in cystic fibrosis) or long-term antibiotic use (such as tuberculosis therapy) highlight that in numerous cases, due consideration should be given to avoid promoting resistance unintentionally by merely focusing on the simplicity of therapy. Even though evolution informed treatment strategies such as those outlined above might come with more complicated and time intensive protocols than those currently applied, they have the likely advantage to reduce the rate of resistance evolution and thereby improve therapy outcome, not only in individual patients, but also on the population level.
The complex interactions of pathogens with the host, local environments and residential microbiota render their evolutionary responses to antimicrobial substances when applied in vivo just as complex. This is where Precision Medicine comes into play. There is an urgent need to characterize bacterial infections, including identification of the pathogen taxa, the site of infection(s), phenotypic and genotypic drug resistance profiles, but also individual host and microbiome characteristics for better and more sustainable treatments. Developing and applying tailored treatments, however, comes with challenges inherent to Precision Medicine, requiring thoughtful balancing of individual and public interests: Deep sampling of pathogens in an infection for current explorative research as well as for future diagnostics or therapy surveillance, in conjunction with comprehensive characterization of patients in a system medicine approach comes with a high demand of personal and material resources. If patients are prone to undergo more intensive and invasive procedures than currently required when adhering to established treatment concepts, physicians may run into an ethical dilemma when weighing the benefit for the individual patient against the benefit for the public. However, evolution-informed therapies may offer an opportunity to meet both of these requirements by ensuring pathogen elimination and minimizing resistance spread, thereby sustaining treatment efficacy.
Author Contributions
MM, LT, MV, EG, LS, and DU drafted the manuscript. MM, SN, and HS conceived the manuscript. All authors made intellectual contributions, critically revised the manuscript, and gave final approval.
Funding
This work was supported by the Cluster of Excellence in Precision Medicine in chronic Inflammation (EXC 2167-390884018), the German Center for Infection Research (DZIF) and the Leibniz Science Campus Evolutionary Medicine of the Lung (EvoLUNG).
Conflict of Interest
The authors declare that the research was conducted in the absence of any commercial or financial relationships that could be construed as a potential conflict of interest.
References
1. D'Costa VM, King CE, Kalan L, Morar M, Sung WWL, Schwarz C, et al. Antibiotic resistance is ancient. Nature. (2011) 477:457–61. doi: 10.1038/nature10388
2. Wellington EMH, Boxall AB, Cross P, Feil EJ, Gaze WH, Hawkey PM, et al. The role of the natural environment in the emergence of antibiotic resistance in gram-negative bacteria. Lancet Infect Dis. (2013) 13:155–65. doi: 10.1016/S1473-3099(12)70317-1
3. Home. AMR Review. Available online at: https://amr-review.org/ (accessed May 7, 2020).
4. G7 Science Academies' Statement 2015: Infectious Diseases and Antimicrobial Resistance: Threats and Necessary Actions. (2015). Available online at: https://www.academie-sciences.fr/pdf/rapport/2015-G7-Infectious-Diseases.pdf (accessed May 28, 2020).
5. Nesse RM, Ganten D, Gregory TR, Omenn GS. Evolutionary molecular medicine. J Mol Med Berl Ger. (2012) 90:509–22. doi: 10.1007/s00109-012-0889-9
6. Roemhild R, Schulenburg H. Evolutionary ecology meets the antibiotic crisis: can we control pathogen adaptation through sequential therapy? Evol Med Public Health. (2019) 2019:37–45. doi: 10.1093/emph/eoz008
7. Day T, Huijben S, Read AF. Is selection relevant in the evolutionary emergence of drug resistance? Trends Microbiol. (2015) 23:126–33. doi: 10.1016/j.tim.2015.01.005
8. Weersma RK, Zhernakova A, Fu J. Interaction between drugs and the gut microbiome. Gut. (2020) 69:1510–19. doi: 10.1136/gutjnl-2019-320204
9. Andersson DI, Balaban NQ, Baquero F, Courvalin P, Glaser P, Gophna U, et al. Antibiotic resistance: turning evolutionary principles into clinical reality. FEMS Microbiol Rev. (2020) 44:171–88. doi: 10.1093/femsre/fuaa001
10. Martínez JL. Antibiotics and antibiotic resistance genes in natural environments. Science. (2008) 321:365–7. doi: 10.1126/science.1159483
11. Read AF, Huijben S. Evolutionary biology and the avoidance of antimicrobial resistance. Evol Appl. (2009) 2:40–51. doi: 10.1111/j.1752-4571.2008.00066.x
12. Melnyk AH, Wong A, Kassen R. The fitness costs of antibiotic resistance mutations. Evol Appl. (2015) 8:273–83. doi: 10.1111/eva.12196
13. Andersson DI, Hughes D. Antibiotic resistance and its cost: is it possible to reverse resistance? Nat Rev Microbiol. (2010) 8:260–71. doi: 10.1038/nrmicro2319
15. Ehrlich P. Address in pathology on chemotherapeutics:: scientific principles, methods, and results. Lancet. (1913) 182:445–51.
16. Pym AS, Saint-Joanis B, Cole ST. Effect of katG mutations on the virulence of Mycobacterium tuberculosis and the implication for transmission in humans. Infect Immun. (2002) 70:4955–60. doi: 10.1128/IAI.70.9.4955-4960.2002
17. Gagneux S, Long CD, Small PM, Van T, Schoolnik GK, Bohannan BJM. The competitive cost of antibiotic resistance in Mycobacterium tuberculosis. Science. (2006) 312:1944–6. doi: 10.1126/science.1124410
18. Merker M, Barbier M, Cox H, Rasigade J-P, Feuerriegel S, Kohl TA, et al. Compensatory evolution drives multidrug-resistant tuberculosis in Central Asia. eLife. (2018) 7:e38200. doi: 10.7554/eLife.38200
19. de Vos M, Müller B, Borrell S, Black PA, van Helden PD, Warren RM, et al. Putative compensatory mutations in the rpoC gene of rifampin-resistant Mycobacterium tuberculosis are associated with ongoing transmission. Antimicrob Agents Chemother. (2013) 57:827–32. doi: 10.1128/AAC.01541-12
20. Blanco P, Hernando-Amado S, Reales-Calderon JA, Corona F, Lira F, Alcalde-Rico M, et al. Bacterial multidrug efflux pumps: much more than antibiotic resistance determinants. Microorganisms. (2016) 4:14. doi: 10.3390/microorganisms4010014
21. Hay T, Fraud S, Lau CH-F, Gilmour C, Poole K. Antibiotic inducibility of the mexXY multidrug efflux operon of Pseudomonas aeruginosa: involvement of the MexZ anti-repressor ArmZ. PLoS ONE. (2013) 8:e56858. doi: 10.1371/journal.pone.0056858
22. Laehnemann D, Peña-Miller R, Rosenstiel P, Beardmore R, Jansen G, Schulenburg H. Genomics of rapid adaptation to antibiotics: convergent evolution and scalable sequence amplification. Genome Biol Evol. (2014) 6:1287–301. doi: 10.1093/gbe/evu106
23. Botelho J, Grosso F, Peixe L. Antibiotic resistance in Pseudomonas aeruginosa- mechanisms, epidemiology and evolution. Drug Resist Updat Rev Comment Antimicrob Anticancer Chemother. (2019) 44:100640. doi: 10.1016/j.drup.2019.07.002
24. Munita JM, Arias CA. Mechanisms of antibiotic resistance. Microbiol Spectr. (2016) 4:VMBF-0016-2015. doi: 10.1128/microbiolspec.VMBF-0016-2015
25. Song T, Park Y, Shamputa IC, Seo S, Lee SY, Jeon H-S, et al. Fitness costs of rifampicin resistance in Mycobacterium tuberculosis are amplified under conditions of nutrient starvation and compensated by mutation in the β' subunit of RNA polymerase. Mol Microbiol. (2014) 91:1106–119. doi: 10.1111/mmi.12520
26. Maisnier-Patin S, Andersson DI. Adaptation to the deleterious effects of antimicrobial drug resistance mutations by compensatory evolution. Res Microbiol. (2004) 155:360–9. doi: 10.1016/j.resmic.2004.01.019
27. Maisnier-Patin S, Berg OG, Liljas L, Andersson DI. Compensatory adaptation to the deleterious effect of antibiotic resistance in Salmonella typhimurium. Mol Microbiol. (2002) 46:355–66. doi: 10.1046/j.1365-2958.2002.03173.x
28. Comas I, Borrell S, Roetzer A, Rose G, Malla B, Kato-Maeda M, et al. Whole-genome sequencing of rifampicin-resistant Mycobacterium tuberculosis strains identifies compensatory mutations in RNA polymerase genes. Nat Genet. (2011) 44:106–10. doi: 10.1038/ng.1038
29. Björkholm B, Sjölund M, Falk PG, Berg OG, Engstrand L, Andersson DI. Mutation frequency and biological cost of antibiotic resistance in Helicobacter pylori. Proc Natl Acad Sci USA. (2001) 98:14607–12. doi: 10.1073/pnas.241517298
30. Pang Z, Raudonis R, Glick BR, Lin T-J, Cheng Z. Antibiotic resistance in Pseudomonas aeruginosa: mechanisms and alternative therapeutic strategies. Biotechnol Adv. (2019) 37:177–92. doi: 10.1016/j.biotechadv.2018.11.013
31. Ramakrishnan L. Revisiting the role of the granuloma in tuberculosis. Nat Rev Immunol. (2012) 12:352–66. doi: 10.1038/nri3211
32. Caño-Muñiz S, Anthony R, Niemann S, Alffenaar J-WC. New approaches and therapeutic options for Mycobacterium tuberculosis in a dormant state. Clin Microbiol Rev. (2018) 31:e00060-17. doi: 10.1128/CMR.00060-17
33. Olesen SW, Barnett ML, MacFadden DR, Brownstein JS, Hernández-Díaz S, Lipsitch M, et al. The distribution of antibiotic use and its association with antibiotic resistance. eLife. (2018) 7:e39435. doi: 10.7554/eLife.39435
34. Manyi-Loh C, Mamphweli S, Meyer E, Okoh A. Antibiotic use in agriculture and its consequential resistance in environmental sources: potential public health implications. Mol J Synth Chem Nat Prod Chem. (2018) 23:795. doi: 10.3390/molecules23040795
35. Bollenbach T. Antimicrobial interactions: mechanisms and implications for drug discovery and resistance evolution. Curr Opin Microbiol. (2015) 27:1–9. doi: 10.1016/j.mib.2015.05.008
36. Hegreness M, Shoresh N, Damian D, Hartl D, Kishony R. Accelerated evolution of resistance in multidrug environments. Proc Natl Acad Sci USA. (2008) 105:13977–81. doi: 10.1073/pnas.0805965105
37. Yeh PJ, Hegreness MJ, Aiden AP, Kishony R. Drug interactions and the evolution of antibiotic resistance. Nat Rev Microbiol. (2009) 7:460–6. doi: 10.1038/nrmicro2133
38. Pena-Miller R, Laehnemann D, Jansen G, Fuentes-Hernandez A, Rosenstiel P, Schulenburg H, et al. When the most potent combination of antibiotics selects for the greatest bacterial load: the smile-frown transition. PLoS Biol. (2013) 11:e1001540. doi: 10.1371/journal.pbio.1001540
39. Saputra EC, Huang L, Chen Y, Tucker-Kellogg L. Combination therapy and the evolution of resistance: the theoretical merits of synergism and antagonism in cancer. Cancer Res. (2018) 78:2419–31. doi: 10.1158/0008-5472.CAN-17-1201
40. Vestergaard M, Paulander W, Marvig RL, Clasen J, Jochumsen N, Molin S, et al. Antibiotic combination therapy can select for broad-spectrum multidrug resistance in Pseudomonas aeruginosa. Int J Antimicrob Agents. (2016) 47:48–55. doi: 10.1016/j.ijantimicag.2015.09.014
41. Llanes C, Hocquet D, Vogne C, Benali-Baitich D, Neuwirth C, Plésiat P. Clinical strains of Pseudomonas aeruginosa overproducing MexAB-OprM and MexXY efflux pumps simultaneously. Antimicrob Agents Chemother. (2004) 48:1797–802. doi: 10.1128/AAC.48.5.1797-1802.2004
42. Barbosa C, Beardmore R, Schulenburg H, Jansen G. Antibiotic combination efficacy (ACE) networks for a Pseudomonas aeruginosa model. PLoS Biol. (2018) 16:e2004356. doi: 10.1371/journal.pbio.2004356
43. Lázár V, Pal Singh G, Spohn R, Nagy I, Horváth B, Hrtyan M, et al. Bacterial evolution of antibiotic hypersensitivity. Mol Syst Biol. (2013) 9:700. doi: 10.1038/msb.2013.57
44. Imamovic L, Sommer MOA. Use of collateral sensitivity networks to design drug cycling protocols that avoid resistance development. Sci Transl Med. (2013) 5:204ra132. doi: 10.1126/scitranslmed.3006609
45. Tueffers L, Barbosa C, Bobis I, Schubert S, Höppner M, Rühlemann M, et al. Pseudomonas aeruginosa populations in the cystic fibrosis lung lose susceptibility to newly applied β-lactams within 3 days. J Antimicrob Chemother. (2019) 74:2916–25. doi: 10.1093/jac/dkz297
46. Bloemberg GV, Keller PM, Stucki D, Stuckia D, Trauner A, Borrell S, et al. Acquired resistance to bedaquiline and delamanid in therapy for tuberculosis. N Engl J Med. (2015) 373:1986–8. doi: 10.1056/NEJMc1505196
47. Roemhild R, Barbosa C, Beardmore RE, Jansen G, Schulenburg H. Temporal variation in antibiotic environments slows down resistance evolution in pathogenic Pseudomonas aeruginosa. Evol Appl. (2015) 8:945–55. doi: 10.1111/eva.12330
48. Szybalski W, Bryson V. Genetic studies on microbial cross resistance to toxic agents. I. Cross resistance of Escherichia coli to fifteen antibiotics. J Bacteriol. (1952) 64:489–99. doi: 10.1128/JB.64.4.489-499.1952
49. Imamovic L, Ellabaan MMH, Dantas Machado AM, Citterio L, Wulff T, Molin S, et al. Drug-driven phenotypic convergence supports rational treatment strategies of chronic infections. Cell. (2018) 172:121–34.e14. doi: 10.1016/j.cell.2017.12.012
50. Barbosa C, Trebosc V, Kemmer C, Rosenstiel P, Beardmore R, Schulenburg H, et al. Alternative evolutionary paths to bacterial antibiotic resistance cause distinct collateral effects. Mol Biol Evol. (2017) 34:2229–44. doi: 10.1093/molbev/msx158
51. Maltas J, Wood KB. Pervasive and diverse collateral sensitivity profiles inform optimal strategies to limit antibiotic resistance. PLoS Biol. (2019) 17:e3000515. doi: 10.1371/journal.pbio.3000515
52. Rodriguez de Evgrafov M, Gumpert H, Munck C, Thomsen TT, Sommer MOA. Collateral resistance and sensitivity modulate evolution of high-level resistance to drug combination treatment in Staphylococcus aureus. Mol Biol Evol. (2015) 32:1175–85. doi: 10.1093/molbev/msv006
53. Oz T, Guvenek A, Yildiz S, Karaboga E, Tamer YT, Mumcuyan N, et al. Strength of selection pressure is an important parameter contributing to the complexity of antibiotic resistance evolution. Mol Biol Evol. (2014) 31:2387–401. doi: 10.1093/molbev/msu191
54. Nichol D, Rutter J, Bryant C, Hujer AM, Lek S, Adams MD, et al. Antibiotic collateral sensitivity is contingent on the repeatability of evolution. Nat Commun. (2019) 10:334. doi: 10.1038/s41467-018-08098-6
55. Podnecky NL, Fredheim EGA, Kloos J, Sørum V, Primicerio R, Roberts AP, et al. Conserved collateral antibiotic susceptibility networks in diverse clinical strains of Escherichia coli. Nat Commun. (2018) 9:3673. doi: 10.1038/s41467-018-06143-y
56. Santos-Lopez A, Marshall CW, Scribner MR, Snyder DJ, Cooper VS. Evolutionary pathways to antibiotic resistance are dependent upon environmental structure and bacterial lifestyle. eLife. (2019) 8:e47612. doi: 10.7554/eLife.47612
57. Barbosa C, Römhild R, Rosenstiel P, Schulenburg H. Evolutionary stability of collateral sensitivity to antibiotics in the model pathogen Pseudomonas aeruginosa. eLife. (2019) 8:e51481. doi: 10.7554/eLife.51481
58. Roemhild R, Gokhale CS, Dirksen P, Blake C, Rosenstiel P, Traulsen A, et al. Cellular hysteresis as a principle to maximize the efficacy of antibiotic therapy. Proc Natl Acad Sci USA. (2018) 115:9767–72. doi: 10.1073/pnas.1810004115
59. Staubach F, Künzel S, Baines AC, Yee A, McGee BM, Bäckhed F, et al. Expression of the blood-group-related glycosyltransferase B4galnt2 influences the intestinal microbiota in mice. ISME J. (2012) 6:1345–55. doi: 10.1038/ismej.2011.204
60. Rausch P, Steck N, Suwandi A, Seidel JA, Künzel S, Bhullar K, et al. Expression of the blood-group-related gene B4galnt2 alters susceptibility to Salmonella infection. PLoS Pathog. (2015) 11:e1005008. doi: 10.1371/journal.ppat.1005008
61. Barroso-Batista J, Pedro MF, Sales-Dias J, Pinto CJG, Thompson JA, Pereira H, et al. Specific eco-evolutionary contexts in the mouse gut reveal Escherichia coli metabolic versatility. Curr Biol. (2020) 30:1049–62.e7. doi: 10.1016/j.cub.2020.01.050
62. Sorbara MT, Pamer EG. Interbacterial mechanisms of colonization resistance and the strategies pathogens use to overcome them. Mucosal Immunol. (2019) 12:1–9. doi: 10.1038/s41385-018-0053-0
63. Griffin AS, West SA, Buckling A. Cooperation and competition in pathogenic bacteria. Nature. (2004) 430:1024–7. doi: 10.1038/nature02744
64. Andersen SB, Marvig RL, Molin S, Krogh Johansen H, Griffin AS. Long-term social dynamics drive loss of function in pathogenic bacteria. Proc Natl Acad Sci USA. (2015) 112:10756–61. doi: 10.1073/pnas.1508324112
65. Andersen SB, Ghoul M, Marvig RL, Lee Z-B, Molin S, Johansen HK, et al. Privatisation rescues function following loss of cooperation. eLife. (2018) 7:e38594. doi: 10.7554/eLife.38594
66. Brook I. The role of beta-lactamase-producing-bacteria in mixed infections. BMC Infect Dis. (2009) 9:202. doi: 10.1186/1471-2334-9-202
67. Yurtsev EA, Chao HX, Datta MS, Artemova T, Gore J. Bacterial cheating drives the population dynamics of cooperative antibiotic resistance plasmids. Mol Syst Biol. (2013) 9:683. doi: 10.1038/msb.2013.39
68. Ciofu O, Beveridge TJ, Kadurugamuwa J, Walther-Rasmussen J, Høiby N. Chromosomal beta-lactamase is packaged into membrane vesicles and secreted from Pseudomonas aeruginosa. J Antimicrob Chemother. (2000) 45:9–13. doi: 10.1093/jac/45.1.9
69. Weber BS, Ly PM, Irwin JN, Pukatzki S, Feldman MF. A multidrug resistance plasmid contains the molecular switch for type VI secretion in Acinetobacter baumannii. Proc Natl Acad Sci USA. (2015) 112:9442–47. doi: 10.1073/pnas.1502966112
70. Di Venanzio G, Moon KH, Weber BS, Lopez J, Ly PM, Potter RF, et al. Multidrug-resistant plasmids repress chromosomally encoded T6SS to enable their dissemination. Proc Natl Acad Sci USA. (2019) 116:1378–83. doi: 10.1073/pnas.1812557116
71. Jorth P, Staudinger BJ, Wu X, Hisert KB, Hayden H, Garudathri J, et al. Regional isolation drives bacterial diversification within cystic fibrosis lungs. Cell Host Microbe. (2015) 18:307–19. doi: 10.1016/j.chom.2015.07.006
72. Estrela S, Brown SP. Community interactions and spatial structure shape selection on antibiotic resistant lineages. PLoS Comput Biol. (2018) 14:e1006179. doi: 10.1371/journal.pcbi.1006179
73. Frost I, Smith WPJ, Mitri S, Millan AS, Davit Y, Osborne JM, et al. Cooperation, competition and antibiotic resistance in bacterial colonies. ISME J. (2018) 12:1582–593. doi: 10.1038/s41396-018-0090-4
74. Klümper U, Recker M, Zhang L, Yin X, Zhang T, Buckling A, et al. Selection for antimicrobial resistance is reduced when embedded in a natural microbial community. ISME J. (2019) 13:2927–37. doi: 10.1038/s41396-019-0483-z
75. Baumgartner M, Bayer F, Pfrunder-Cardozo KR, Buckling A, Hall AR. Resident microbial communities inhibit growth and antibiotic-resistance evolution of Escherichia coli in human gut microbiome samples. PLOS Biol. (2020) 18:e3000465. doi: 10.1371/journal.pbio.3000465
76. Antibiotic Resistance. Available online at: https://www.who.int/news-room/fact-sheets/detail/antibiotic-resistance (accessed May 12, 2020).
Keywords: AMR, drug resistance, evolution, precision medicine, evolutionary medicine
Citation: Merker M, Tueffers L, Vallier M, Groth EE, Sonnenkalb L, Unterweger D, Baines JF, Niemann S and Schulenburg H (2020) Evolutionary Approaches to Combat Antibiotic Resistance: Opportunities and Challenges for Precision Medicine. Front. Immunol. 11:1938. doi: 10.3389/fimmu.2020.01938
Received: 01 June 2020; Accepted: 17 July 2020;
Published: 27 August 2020.
Edited by:
Ralf J. Ludwig, University of Lübeck, GermanyReviewed by:
Antoine Abou Fayad, American University of Beirut, LebanonElsa Anes, University of Lisbon, Portugal
Copyright © 2020 Merker, Tueffers, Vallier, Groth, Sonnenkalb, Unterweger, Baines, Niemann and Schulenburg. This is an open-access article distributed under the terms of the Creative Commons Attribution License (CC BY). The use, distribution or reproduction in other forums is permitted, provided the original author(s) and the copyright owner(s) are credited and that the original publication in this journal is cited, in accordance with accepted academic practice. No use, distribution or reproduction is permitted which does not comply with these terms.
*Correspondence: Matthias Merker, bW1lcmtlckBmei1ib3JzdGVsLmRl
†These authors have contributed equally to this work