- Department of Molecular Pathobiology and Cell Adhesion Biology, Mie University Graduate School of Medicine, Mie, Japan
Sepsis remains a major problem for human health worldwide, thereby manifesting high rates of morbidity and mortality. Sepsis, once understood as a monophasic sustained hyperinflammation, is currently recognized as a dysregulated host response to infection, with both hyperinflammation and immunoparalysis occurring simultaneously from the earliest stages of sepsis, involving multiple organ dysfunctions. Despite the recent progress in the understanding of the pathophysiology underlying sepsis, no specific treatment to restore immune dysregulation in sepsis has been validated in clinical trials. In recent years, treatment for immune checkpoints such as the programmed cell death protein 1/programmed death ligand (PD-1/PD-L) pathway in tumor-infiltrating T-lymphocytes has been successful in the field of cancer immune therapy. As immune-paralysis in sepsis involves exhausted T-lymphocytes, future clinical applications of checkpoint inhibitors for sepsis are expected. In addition, the functions of PD-1/PD-L on innate lymphoid cells and the role of exosomal forms of PD-L1 warrant further research. Looking back on the history of repeatedly failed clinical trials of immune modulatory therapies for sepsis, sepsis must be recognized as a difficult disease entity for performing clinical trials. A major obstacle that could prevent effective clinical trials of drug candidates is the disease complexity and heterogeneities; clinically diagnosed sepsis could contain multiple sepsis subgroups that suffer different levels of hyper-inflammation and immune-suppression in distinct organs. Thus, the selection of appropriate more homogenous sepsis subgroup is the key for testing the clinical efficacy of experimental therapies targeting specific pathways in either hyperinflammation and/or immunoparalysis. An emerging technology such as artificial intelligence (AI) may help to identify an immune paralysis subgroup who would best be treated by PD-1/PD-L1 pathway inhibitors.
Introduction
Sepsis remains an ongoing threat to human health worldwide. Sepsis is one of the leading causes of death in intensive care units (1, 2). The World Health Organization (WHO) has recommended that sepsis be recognized as a Global Health Priority (3). Although there has been an overall improvement in clinical outcomes globally, which seems due to improved treatment practices by disseminating and complying with the Surviving Sepsis Campaign guidelines (4) during the past few decades (5), mortality rates remains unbearably high, reaching 25%–30% for sepsis, and up to 40%–50% in cases of septic shock, though there are some differences depending on the country (6, 7). To put it simply, the central pathophysiology of sepsis is dysregulated host response to infection (8). The concept of dysregulation includes not only excessive inflammation, but also immunosuppression. Moreover, hyper-inflammation and immunoparalysis can exist concomitantly from the very onset of sepsis. A previous biphasic concept that the immunosuppressive late phase follows the hyperinflammatory early phase has now been outdated (9). The purpose of this review is to describe the underlying mechanisms of the immune deregulation in sepsis, to summarize the failed history of clinical trials testing therapeutic agents for host immune responses in sepsis, and to specifically address treatments aimed to reverse the immunosuppression, focusing in particular on the programmed cell death protein 1/programmed death ligand (PD-1/PD-L) pathway on T cells. In addition, the functions of the PD-1/PD-L pathway on innate lymphoid cells and PD-L1 present on exosomes are discussed as characteristic aspects of sepsis in this review. In the last section, we discuss the application of artificial intelligence (AI) for subgrouping of septic patients and selecting the appropriate patients as the most promising approach for achieving a breakthrough in new treatments for sepsis. If a revolution in sepsis treatment is to be realized, new concepts and new methods are absolutely essential. This means immunomodulation and AI.
The Clinical Definition of Sepsis
Although the breadth and depth of our knowledge about the pathophysiology underlying sepsis has increased dramatically in recent years thanks to the expansion of biomedical research publications, there has been only limited progress in treatment in terms of interventions for the essential host response rather than supportive care such as mechanical ventilation strategies, nutrition, and PADIS (Pain, Agitation, Delirium, Immobility, and Sleep) management. In addition, chronic persistent inflammatory-immunosuppressive syndrome has emerged as a new topic regarding survival in wake of acute phases of sepsis. Quality of life after surviving initial septic insult and discharge remains poor. Thus, sepsis ranks among today’s leading medical, economic, and social issues.
Historically, the term “sepsis” has been used extensively, although until about 1990 there was no clear definition. In 1991, the ACCP/SCCM (American College of Chest Physicians/Society of Critical Care Medicine) Consensus Conference defined sepsis as Systemic Inflammatory Response Syndrome (SIRS), which was caused by microbial infections (10). The SIRS case must meet two or more of the following four criteria; tachypnoea, tachycardia, abnormal body temperature and/or abnormal white blood cell count or >10% presence of immature white blood cell forms. This definition emphasizes the concept that systemic inflammation is the key to sepsis, and therefore mandates physical examinations or laboratory parameters that address hyper inflammation. Until the advent of the Third International Consensus Definitions for Sepsis and Septic Shock (Sepsis-3), sepsis was largely understood as a systemic inflammatory syndrome. In 2016, Sepsis-3 defined sepsis as life-threatening organ dysfunction caused by a dysregulated host response to infection (11). This new definition showed that the past characterizations were excessively focused on inflammation. Septic shock was defined as a condition requiring continuous administration of vasopressors to maintain mean arterial blood pressure, despite initial fluid resuscitation. In Sepsis 3, the term “severe sepsis” was eliminated. This meant that the pathological condition of sepsis, which causes organ damage as a result of infection, was in itself a very serious pathology.
Immune Deregulation; The Central Pathophysiology of Sepsis/Septic Shock
Sepsis constitutes a dysregulated host response to infection. Hyper-inflammation and the immunoparalysis can exist concomitantly from the onset of sepsis. In most cases, infections are contained and eventually cured by the cooperations by the immune system, antibiotics, and source control/drainage, thereby restoring to normal homeostasis. However, infection can progress to sepsis when a dysregulated host response persists. The time course of sepsis was previously thought to consist of an initial hyperinflammatory state that transitioned to a hypoinflammatory state, eventually leading to prolonged and significant immunosuppression. In terms of paired words, systemic inflammatory response syndrome (SIRS) and compensatory anti-inflammatory response syndrome (CARS) symbolize this paradigm (12).
Recent studies have shown that inflammation and immunosuppression occur simultaneously but not sequentially. Both pro-inflammatory and anti-inflammatory cytokine storms occur during the earliest stages of infection, and the balance between the two determines whether clinically over-inflammation or immunosuppression then occurs (13). (Figure 1) Analyses of leukocyte gene expression in patients with severe sepsis have revealed that both the inflammatory response and the expression of immunosuppression-related genes occur at the same time, immediately after sepsis onset. In fact, the more severe a patient’s case is, the higher the immunosuppression-related gene expression level (14). Excessive inflammation is not the only cause of death in sepsis. Similarly, immunosuppression is not the only cause of sepsis-related death. A mixture of the two is responsible for the difficulty of treating sepsis and its overall poor prognosis.
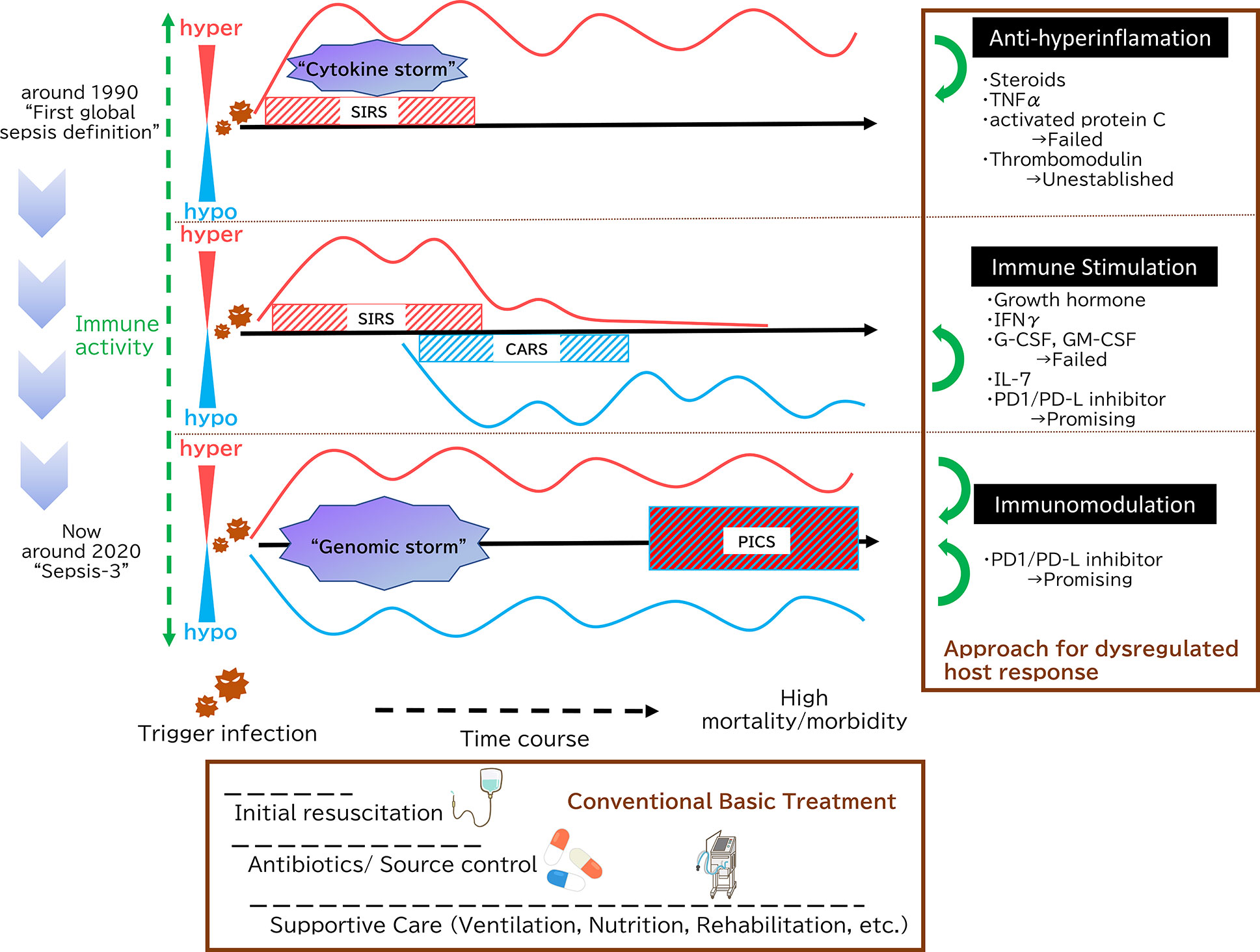
Figure 1 Paradigm shift in sepsis. In 1991, sepsis was defined as Systemic Inflammatory Response Syndrome (SIRS), which was caused by microbial infections. This definition emphasizes the concept that systemic inflammation is the key to sepsis. During the initial phases of sepsis, inflammation originating in the innate immune system is enhanced by multiple pathways as “cytokine storm”. Then, a new theory has since emerged positing that immunosuppression following initial hyperinflammation, eventually leading to prolonged and significant immunosuppression is the key pathophysiology. In terms of paired words, SIRS and compensatory anti-inflammatory response syndrome (CARS) symbolize this paradigm. In 2016, The Third International Consensus Definitions for Sepsis and Septic Shock (Sepsis-3) defined sepsis as life-threatening organ dysfunction caused by a dysregulated host response to infection. Both pro-inflammatory and anti-inflammatory genomic storms occur beginning in the earliest stages of infection, and the balance between the two determines whether clinically over-inflammation or immunosuppression then occurs. Persistent Inflammation, Immunosuppression, and Catabolism Syndrome (PICS) is an intriguing concept from the integrated point of view, which contends that SIRS and its counterpart CARS do not exist independently; rather, both are occurring simultaneously. As shown in the Surviving Sepsis Campaign guidelines, initial fluid resuscitation, earlier antibiotic administration and supportive care such as mechanical ventilation strategies, nutrition and PADIS (Pain, Agitation, Delirium, Immobility, and Sleep) management are key of sepsis management. With the development of these conventional basic treatment, there is an overall clinical outcome improvement, but mortality of sepsis still reaches high. Therefore, additional treatment method that targets the underlying essence of sepsis has been expected. At first, as sepsis was essentially understood as hyperinflammation, many anti-inflammation approaches were tried. However, none have demonstrated that those sepsis treatment strategies are effective. Then, focusing on the immunoparalytic aspect of sepsis, immune stimulation represents a new strategy for targeting sepsis. There are some promising molecules, among them PD-1/PD-L inhibitors, which can not only reverse immuostimulation but act as immunomodulation, are highly expected.
There is also a concept that sepsis constitutes what has been termed Persistent Inflammation, Immunosuppression and Catabolism Syndrome (PICS) (15). This is an intriguing concept from the integrated point of view, which contends that SIRS and its counterpart CARS do not exist independently; rather, both are occurring simultaneously. To summarize, in sepsis, hyper-inflammation and immunoparalysis coexist from the early to the late stages of the pathological condition, which is evident from recent basic researches and clinical recognitions.
Sepsis Induced Hyper-Inflammation
Knowing that inflammation and immunosuppression occur simultaneously from the earliest stages of infection, hyperinflammation remains a hallmark of sepsis. During the initial phases of sepsis, acute inflammation originating from the activation of the innate immune system is triggered and enhanced by multiple pathways. Key pro-inflammatory responses contain various biological systems including complement system, coagulation system, platelet, vascular endothelium, neutrophil extracellular traps (NETs), and many kinds of immunity-oriented cells. These pro-inflammatory systems interact in a highly intricate manner. The word “cytokine storm” is a suitable description and well worth underscoring.
After infection, invading pathogens are immediately recognized by the host innate immune system. Innate immune cells such as neutrophils and macrophages, whose primary function is immune surveillance, recognize pathogen-associated molecular patterns (PAMPs) derived from microorganisms via pattern recognition receptors (PRRs) (16). PRRs include Toll-like receptors (TLRs), nucleotide-binding oligomerization domain-like receptors (NLRs), retinoic acid-inducible gene-like receptors (RLRs) and C-type lectin receptors (CLRs) (8, 16). PRRs recognize not only PAMPs derived from microorganisms, but also damage-associated molecular pattern molecules (DAMPs or Alarmin) (17). The latter are derived from the host’s own cells. The fact that PRRs recognize various PAMPs and DAMPs explains similar clinical appearances presented by critically ill patients, regardless of the trigger infections caused by various pathogens originating at various organs (18).
NF-κB and interferons (IFNs) pathways are two major signaling cascades to activate innate immune systems. These intracellular signaling cascades generate pro-inflammatory mediators by promoting gene transcription beginning within a few minutes of PRRs recognizing PAMPs or DAMPs. In the NF-κB pathway, the binding of PAMPs to TLR4 triggers the recruitment of the myeloid differentiation primary response gene 88 (MyD88), which acts as an adopter protein, and interleukin-1 receptor-associated kinases 1 and 4 (IRAK1, 4), act as signaling molecules. Then these signaling molecules form a complex with tumor necrosis factor (TNF) receptor-associated factor 6 (TRAF6). This cascade further activates the downstream TGF-β-activated kinase and inhibitor of nuclear factor kappa-B kinase (IKK), which then regulate NF-κB. NF-κβ, which is usually present in the cytoplasm, migrates to the nucleus where it activates targeted genes coding pro-inflammatory cytokines like TNF and IL-1. These cytokines then re-initiate signaling cascades to activate or produce other inflammatory cytokines and chemokines inter- or intra-cells in the innate immune response (19). IFNs are a group of signaling proteins that have diverse effects on the innate immune system (20). By binding its receptor, IFN starts the signaling cascade, leading to the expression of interferon-stimulated genes (ISGs) and IFN gamma-activated site (GAS) genes. In these inflammation cascades, small ubiquitin-like modifier (SUMO) molecules play an important role by binding to target proteins and modulating their function. In the NF-kB pathway, SUMO proteins play a dual role of promoting and limiting excessive activation of them. That is, SUMO-1 binds to the TRAF family member-associated NF-kappa-B activator while SUMO-3 binds to IKK-γ, which is one of the NF-κB essential modulators accelerating the NF-kB pathway. On the other hand, sumoylation of IκBα by SUMO-1 limits excessive activation (21). This bidirectional effect of SUMO can be observed in the IFN pathway, as well. For example, SUMO has both promoting and inhibiting effects on antiviral interferon regulatory transcription factor-3 (IRF-3) activity, through sumoylation and desumoylation, via peptidyl-prolyl cis/trans isomerase (Pin1) (21, 22).
Failures of Anti-Inflammatory Therapies in Clinical Trials
Most basic and clinical research efforts on sepsis has focused on suppressing hyperinflammation. Numerous experiments involving animal sepsis models have shown that the suppression of specific inflammatory cascades improved outcomes (23–25). Over the last few decades more than one hundred clinical studies have sought to suppress excessive inflammation in sepsis by targeting PRRs, PAMPs, cytokines and various mediators, however, none have convincingly proved the clinical effectiveness for the treatment of sepsis in clinical settings. Here, we show representative clinical trials focusing on adrenal insufficiency, pro-inflammatory cytokines and immunothrombosis.
Relative adrenal insufficiency, also known as critical illness-related corticosteroid insufficiency, occurs in critically ill patients (26). Corticosteroids have been used for the purpose of early recovery from shock, rather than for reducing mortality, as indicated in the guideline published by the Society of Critical Care Medicine (SCCM) and the European Society of Intensive Care Medicine (ESICM) in 2017 (27). Glucocorticoids affect both innate and adaptive immunity; they inhibit the maturation, differentiation, and proliferation of leukocytes, including myeloid cells and lymphocytes. Glucocorticoids have an anti-inflammatory effect by producing anti-inflammatory proteins and inhibiting pro-inflammatory proteins. Glucocorticoids bind to glucocorticoid receptor (GR), which is a transcription factors belonging to the nuclear receptor superfamily, and GR translocates to the nucleus. The GR-glucocorticoid complex inhibits the production of pro-inflammatory proteins by sequestering NF-κB into the cytoplasm. It also promotes the production of annexin 1, which inhibits the expression of phospholipase A2. The functional differences between mineralocorticoid and glucocorticoid are still under investigation, although there are many overlapping aspects. It is worth noting that the expression of GR is higher in the immune system than that of mineralocorticoid receptor (MR), but MR and GR are similarly expressed in the cardiovascular system (28). Corticosteroids also have the disadvantages of elevating blood glucose levels and increasing catabolism. Therefore, an investigation as to whether or not corticosteroids can improve patient prognosis has long been needed. However, two large randomized controlled trials (RCTs) published in 2018 failed to produce consistent results. The APROCCHS (Activated Protein C and Corticosteroid for Human Septic Shock) trail, which compared activated protein C, low does corticosteroid, their combination, and placebo, demonstrated that sepsis patients treated with corticosteroid showed a significantly improved 90-day mortality rate (29), while the ADRENAL (Adjunctive corticosteroid treatment iN critically ilL patients with septic shock) trial reported no significant benefits at all (30). There are many points worth discussing regarding these two trials; e.g., the differences in sepsis severity between them and the different medication regimens using fludrocortisone, in addition to hydrocortisone, or not. In the end, however, the role of steroids in improving the prognosis of sepsis patients remains inconclusive.
Another approach involves suppressing inflammatory cell signaling proteins, which seems to have a direct effect on the hyper-inflammatory states. Among inflammatory proteins, TNF-α is a pro-inflammatory cytokine that has been shown to increase in the blood after endotoxin administration (31). Moreover, administration of TNF-α induces a biological reaction similar to those of sepsis (32, 33). Therefore, it is reasonable to hypothesize that inhibition of TNF-α represents a potential treatment option for sepsis in patients. Preclinical studies using animal model experiments have shown favorable results: a protective effect against sepsis (34, 35). However, a single dose of tumor necrosis factor receptor-Fc (TNFR-Fc), which is a fusion protein comprised of the TNFR extracellular domain and the Fc region of the human immunoglobulin heavy chain, injected into 141 patients with septic shock generated no improvements in patient prognosis. On the contrary, it unexpectedly increased mortality in a dose-dependent manner (36). Thus, this potential therapeutic agent can actually worsen the prognosis. The usefulness of TNFR-Fc was demonstrated later in rheumatoid arthritis (37). In both sepsis and rheumatoid arthritis, the central role of TNF in dysregulation of the immune system leading to hyperinflammation were assumed to be the essence of the pathology. However, insights gained from this clinical trial on TNF-α inhibition does not support a simple concept of hyper-inflammation induced by TNF driving the pathogenesis of sepsis.
Immunothrombosis refers to the crosstalk that occurs between coagulation and the immune system (38, 39). Thrombus formation may play an important role in preventing the spread of infection from local sites to the entire body. However, excessive coagulation and platelet activation can induce immune cell recruitment and inflammation. Overactivated platelets cause not only vascular occlusion in micro vessels, but also tissue injury due to the release of platelet-derived microparticles (40). Moreover, these platelets also cause excessive consumption of coagulation factors, resulting in so-called disseminated intravascular coagulation (DIC). Furthermore, inflammation induced the expression of tissue factor on monocyte, thereby triggering the coagulation cascades that lead to the platelet activation (41). With this theoretical background, the suppression of excessive coagulation related to sustained inflammation can be an effective treatment. Activated protein C, which is converted from protein C via a complex of thrombin and thrombomodulin, promotes fibrinolysis and suppresses thrombus and inflammation during inflammatory activation in sepsis (42). In addition, activated protein C has been shown to suppress inflammation by directly acting on neutrophils to inhibit integrin activation and NETs formation (43, 44). Administration of activated protein C might be useful for maintaining an appropriate degree of immunothrombosis and preventing DIC. This is due to the fact that in sepsis, after thrombomodulin has been down-regulated by inflammatory cytokines, the conversion of protein C to activated protein C is suppressed (45).
In the clinical trial lead by the Recombinant Human Activated Protein C Woldwide Evaluation in Severe Sepsis (PROWESS) Study group, recombinant human activated protein C (rhAPC) was shown to reduce patient mortality from sepsis (46). However, a subgroup analysis also revealed that the administration of rhAPC was most effective in the severe group (those with an APACHE (acute physiology and chronic health evaluation) II score of 25 or higher). In fact, no reduction in mortality was observed in patients with an APACHE II score of less than 25, and the risk of serious bleeding increased in the patient group with APACHE II scores less than 20. In a metanalysis of the four RCTs that compared activated protein C versus placebo, the effectiveness of APC was not evident when a 28-day mortality was set as primary endpoint. Moreover, this metanalysis revealed the increased risk of bleeding associated with rhAPC (47). A ROWESS-SHOCK trial that focused on patient with septic shock found no significant beneficial effects on either mortality or bleeding (48). Ultimately, rhAPC was withdrawn from the market.
Thrombomodulin acts as dual regulator in coagulation and inflammation, making it a promising target for immunothrombosis in sepsis (49). In addition to generating an anticoagulant effect by converting protein C to activated protein C via the formation of a complex with thrombin, thrombomodulin exerts an anti-inflammatory effect by absorbing high-mobility group box 1 (HMGB-1) via the lectin-like region (D1) located at the N-terminus and by inhibiting leukocyte integrins via the serine/threonine domain (50, 51). Moreover, thrombomodulin binds to C3b and factor H, thereby negatively regulating the activation of C3b (52). Complement, which recruits and activates leukocytes, endothelial cells and platelets, is important to the innate immune system. However, its uncontrolled activation during sepsis can injure organs (53). Recombinant human soluble thrombomodulin (rhsTM) is an active extracellular domain comprised of thrombomodulin. To date, there have been many discussions regarding the clinical use of thrombomodulin for the treatment of septic patients. The SCARLET (Sepsis Coagulopathy Asahi Recombinant LE Thrombomodulin) trial that utilized rhsTM to treat sepsis failed to improve the 28-day all-cause mortality rate (54). At the moment, there is little clinical evidence to support the use of thrombomodulin to treat sepsis.
As discussed herein, many anti-inflammatory treatments targeting various pathways that have proven effective in animal studies have not yielded convincingly positive results in human sepsis clinical trials. Meanwhile, acknowledging that hyperinflammation is not the only key therapeutic target, the focus of sepsis research is shifting from only suppressing hyper-activated immune system to restoring paralyzed immune system.
Paradoxical Immunoparalysis
Although sepsis has traditionally been characterized as constituting an aberrantly augmented immune response, the increasing prevalence of nosocomial infections in sepsis patients suggests the presence of sustained immunosuppression (55). The deregulations of the innate and adaptive immune systems are linked to the pathogenesis of the immunosuppression in sepsis (56).
Aberrantly induced cell death of immune cells represents a primary cause of immunosuppression in sepsis. A previous in vivo study utilizing Caspase-7 knockout mice have shown that lymphocyte apoptosis in sepsis was suppressed, which lead to the improved survival (57). Apoptosis is a highly regulated form of programmed cell death without eliciting an inflammatory response. Programed cell death and apoptosis are different in their details; for example, the caspase-dependent form constitutes apoptosis (58). There are classically at least two major signaling pathways that lead to apoptosis: the extrinsic pathway and the intrinsic pathway. The extrinsic pathway starts from binding ligands to those receptors anchored to cell membrane. Ligands are comprised of TNF, Fas ligand, and TRAIL (TNF-related apoptosis-inducing ligand). Receptors are comprised of TNFR-1, TNFR-2, Fas receptors (CD95), and TRAIL receptors. The triggering of these receptors by ligands results in the formation of a death-inducing signaling complex (DISC). Caspase 8 dissociates from the DISC to start the caspase activation cascade that lead to apoptosis (59). On the other hand, the intrinsic pathway is non-receptor-mediated; rather, it is driven by mitochondrias. Bax/Bak insertion into mitochondrial membrane causes cytochrome c release from the mitochondrial intermembrane space into the cytosol (60). Cytochrome c forms an apoptosome with Apaf–1 and procaspase-9. Apoptosome triggers caspase 9, which starts the caspase-3 signaling cascade toward apoptosis (61). Both the extrinsic and intrinsic pathways are activated and apoptosis-induced lymphopenia is observed in sepsis (62). Patients with sepsis exhibit apoptosis and/or suppressed functions of immune cells such as CD4+ and CD8+ T cells, B cells and dendritic cells (63) (64, 65). Among them, CD4+ helper T cells, which are crucial for directing appropriate immune responses, are divided into multiple subtypes: not only classically recognized Th1, Th2, and Th17 effector T cells, but also unique sub-populations such as regulatory T cells (Tregs) and Th9 (66). Th9 cells are an abundant source of IL-9, which is a pleiotropic cytokine that acts on many cell types. One immunological role played by IL-9 is the production of IL-4-mediated IgE and IgG from B cells (67). The Th1/Th2 paradigm cannot completely explain the role of current known helper T cells (68). Immune function is intricately suppressed by the apoptosis of various lymphocytes.
Unresponsive adaptive immunity caused by lymphocyte exhaustion is another mechanism of immuno-suppression in sepsis. In chronic viral infections and cancers, T cells are exposed to persistent antigens and inflammatory signals. Continued stimulation of T cells leads to gradual exhaustion. One of the mechanisms of exhaustion is the expression of PD-1 on the surface of T cells that function as immune checkpoints. Exhausted T cells lose their effector function. Specifically, they first lose IL-2 production and their high proliferative capacity, followed by a decrease in the production of IFNγ, TNF, and chemokines (69). The immune system is unable to mount an appropriate immune response against virus-infected cells or cancer cells. T-cell exhaustion is involved in the immune-paralysis in sepsis. T cells harvested from the spleen of patients who died of sepsis have only a low capacity to produce IFNγ and TNF, suggesting a state of T-cell exhaustion. This study also showed the increased expression of PD-1 on T cells and increased expression of PD-L1 on macrophages and endothelial cells (63). These results indicate the importance of the PD-1/PD-L1 pathway for lymphocyte exhaustion, eventually leading to poor outcomes in patients with sepsis. However, it is important to note that the activity of exhausted T cells can be restored by interfering with the PD-1/PD-L1 pathway, as described later.
Suppressed activities of antigen presentation is also responsible for immune suppression in sepsis. In sepsis, the expression of major histocompatibility complex (MHC) class II molecules and human leukocyte antigen DR isotype (HLADR) on antigen-presenting cells (APCs), including dendritic cells and macrophages, is reduced. In addition, the number of dendritic cells that undergo apoptosis increases in patients with sepsis (70). Decreased HLA-DR expression in monocytes is known to correlate with poor outcomes in sepsis (71). In animal studies, the impact of sepsis on tissue-resident dendritic cells differ in the systemic and mucosal organs (72) and the prevention of dendritic cell apoptosis improved the survival rate of sepsis (73). These facts support the contention that the reprogramming of antigen-presenting cells causes immunoparalysis in patients with sepsis.
The expansion of regulatory T cell and myeloid-derived suppressor cell (MDSC) populations constitutes an important mechanism to induce immunoparalysis in sepsis. Regulatory T cells usually maintain self-tolerance by suppressing the activation of auto-reactive effector T cells, thereby preventing auto-immune diseases under physiologic condition. Regulatory T cells increase during sepsis and generate pathologic immunosuppression by suppressing not only effector T cells, but also monocytes and neutrophil functionality (74). Inhibition of regulatory T cells has been reported to improve immunity and mortality rates in septic animal models (75). MDSCs are heterogeneous immature myeloid cells that are not normally detected, but which reach increased levels in cancers and sepsis (76). MDSCs suppress antigen-specific CD4+ and CD8+ T-cell activation (77). In addition, increased MDSCs are associated with greater numbers of regulatory T cells (78). Clinically, increased blood MDSCs have been linked to the increased prevalence of nosocomial infections in patients with sepsis (79).
Immunomodulation; Past Failures and the Future Attempts
Recent progress in the sepsis research has revealed that immunosuppression occurs from the onset, but not in the late phase, of sepsis. The immune-suppression in sepsis results from the paralyzed immune system. The key to the treatment of sepsis is to restore the functions of the paralyzed immune system while avoiding the exacerbation of the immune system, which could induce unwanted hyperinflammation. In 1996, the restoration of the anti-tumor activity of T cells by CTLA-4 blockade was reported in a mouse model (80). A human lgG 1 kappa monoclonal antibody, ipilimumab, has been developed that binds to CTLA-4 (81). Therapeutic efficacy of Ipilimumab has been reported to correlate with the ability of receptors binding to Ipilimumab, indicating the importance of interaction between ligand and receptor of immune check point pathway (82). The clinical application of immunotherapy for cancer using checkpoint inhibitors targeting CTLA-4 and PD-1/PD-L1 pathway has been expanding. Many reports have been published on the safety of utilizing immunomodulation aiming to restore chronically suppressed immune functions in patients with malignant tumors (83). However, the immune suppression in patients with sepsis often occurs more acutely than in those with cancer. Moreover, patients with sepsis are severely ill requiring intensive care management compared with cancer patients. Thus, one should be cautious in extrapolating from the findings of the immune suppression in cancer patients to the application for sepsis patients.
A few growth factors have been studied to restore the impaired metabolism of the critical organ systems including the immune system in severely ill patients. Growth hormone administration has been reported to improve the balance of nitrogen in patients with sepsis (84). In surgical patients, the administration of growth hormones enhanced immunity by maintaining immunoglobulin levels, which led to the reduction of the postoperative surgical site infections (85). Growth hormone may restore the paralyzed immune system by maintaining protein synthesis in critically ill patients. However, in contrast to the expectation, two RCTs comparing high doses of growth hormone or placebo in critically ill patients showed increased rates of mortality. Moreover, growth hormone treatment was associated with prolonged mechanical ventilation, ICU stays and in-hospital admission days (86). These results fail to support the strategy of treating critically ill patients by improving protein synthesis with growth hormone. Similarly, Keratinocyte growth factor (KGF) aiming to restore the alveolar epithelial damages in the lung injury was studied in a Phase2 clinical trial for Acute Respiratory Distress Syndrome (ARDS) patients, thereby failing to improve their clinical outcomes. Moreover, KGF therapy was associated with the adverse events stemming from pyrexia (87).
Administrations of cytokines have been studied to restore the paralyzed immune system in sepsis. IFNγ has been shown to improve phagocytic capacity and HLA-DR expression on monocytes in human sepsis and related to earlier recovery from sepsis (88). Granulocyte-colony stimulating factor (G-CSF) and granulocyte-macrophage colony stimulating factor (GM-CSF) are also potential therapeutic agents for restoring the paralyzed innate immune system in sepsis. The meta-analysis performed in 2011 encompassing 12 RCTs revealed that G-CSF and GM-CSF failed to improve the mortality of sepsis patients (89). On the other hand, G-CSF and GM-CSF did not increase the frequency of complications related to hyperinflammation which was defined as life threatening organ dysfunctions like acute respiratory distress syndrome.
IL-7 plays a major role in the proliferation of CD4 + and CD8 + T cells, and also possesses antiapoptotic properties (90). Significant improvements in lymphocyte function by IL-7 have been observed in experiments involving an animal model of sepsis and using the blood of septic patients (91). The safety of IL-7 has been confirmed in clinical practice in patients with malignant tumors and HIV. In 2018, the phase 2b clinical trial studying the ability of recombinant human IL-7 to reverse the immunosuppression in sepsis demonstrated that the IL-7 treatment increased the number of lymphocytes without exacerbating inflammation (92). Future large-scale RCTs are expected to address clinical effectiveness of IL-7 for the treatment of sepsis.
PD-1/PD-L as a Novel Therapeutic Target to Reverse Immune Paralysis
PD-1 represents a pivotal inhibitory checkpoint regulator that acts to dampen the activation signals elicited by T-cell receptor (93). PD-1 is a transmembrane protein widely expressed on immune cells including dendritic cells, NK cells and monocytes, which function as a costimulatory molecule (94). PD-L1 and PD-L2 are the ligands to which PD-1 binds and are expressed on the surface of various cells including tumor cells. PD-L2 binds to PD-1 with a higher affinity than does PD-L1 (95). The binding of PD-1 to PD-L suppresses T cell activation and cytokine production due to T-effector cell exhaustion and conversion of T-effector cells to regulatory T cells (96). By expressing the ligand of PD-1, tumor cells suppress the immune activity towards cancer cells, thereby escaping the elimination by immune cells. Several inhibitors to perturb the interactions between PD-1 and PD-L, thereby aiming to restore the paralyzed immunity against cancer, have proved clinically effective and been approved for the treatment of cancer (97). For example, Nivolumab, a human monoclonal antibody to PD-1, is used for the treatment of advanced melanoma (98). As a part of the mechanisms underlying immunosuppression in sepsis and malignancies are similar (99), the inhibition of the PD-1/PD-L1 interaction has been studied for theoretically restoring the immune suppressive states in sepsis.
PD-1/PD-L in Sepsis
The enhanced expression of PD-L1 on various types of cells in sepsis has been documented. The expression of PD-L1 on both stromal cells and dendritic cells increased during sepsis. Splenic capillary endothelial cells from patients who died of sepsis expressed more PD-L1 than spleens from patients with brain death or trauma requiring emergent splenectomy (63). PD-1 is upregulated on T lymphocytes whereas PD-L1 is upregulated on monocytes in septic shock patients (100). The levels of PD-L1 expression on monocytes correlates with 28-day mortality rates in patients with sepsis (101). These findings support the idea that the aberrant activation of the PD-1/PD-L1 pathway constitutes a major cause of the immuneparalysis in septic patients.
Clinical Trials Approaching for PD-1/PD-L
The significance of the PD-1/PD-L1 pathway in the pathogenesis of sepsis-induced immune paralysis has been substantiated in mouse sepsis models. Huang et al. have demonstrated that the survival rates of PD-1 knockout mice improved in a sepsis mouse model induced by the cecal ligation-and-puncture procedure, which caused bacterial pan-peritonitis leading to sepsis (102). Following up on this study, sepsis animal models induced by both bacteria and fungi have been treated with PD-1 or PD-L antibodies, improving overall survival rates (103–105), which support further evaluation in clinical trials. Nivolumab is the anti-PD-1 antibody approved for the treatment of cancer patients. The 2019 phase 1b clinical trial has studied the safety and tolerability of nivolumab given to septic patients, thereby showing that no adverse incidences including the aforementioned “cytokine storm”, were found (106). Another 2019 phase 1b clinical trial studying the safety of the anti-PD-L1 antibody BMS-936559 has also confirmed the safety in sepsis patients, thereby showing no adverse incidence of hypercytokinemia (107). Phase 2/3 clinical trials in the future are required to validate the PD-1/PD-L1 pathway as the therapeutic target for reversing the immune paralysis in sepsis.
PD-1/PD-L and Innate Lymphoid Cells; Not Causing Immune-Paralysis But Rather Hyperinflammation
PD-1 on T cells has been well characterized and validated for the therapeutic target for cancer immunotherapy. A few recent investigations have studied the roles of PD-1 expressed on innate lymphoid cells (ILCs), emerging types of non-T, non-B lymphocytes lacking the expression of antigen receptors (108). ILCs play an important role both in homeostasis and in the inflammatory response in the immune system (109). ILCs are classified into at least three major subsets: type 1, type 2, and type 3 ILCs. ILC1, ILC2, and ILC3 execute the important immune regulatory roles to counterpart with Th (CD4+ T helper) 1, Th2, and Th17 T lymphocyte effector subsets, respectively. The roles of ILC2s in the lung inflammation induced by sepsis have been reported. ILC2, the most abundant ILC subset in the lungs, secretes type 2 cytokines such as IL-5 and IL-13, thereby playing the important roles in the regulation of type 2 immune responses, which is required for resolving inflammation and remodeling tissues (110). Insufficient resolution of inflammation and aberrant regulation of tissue repair and remodeling causes acute lung injury (ALI), and lung fibrosis, leading to the irreversible destruction of pulmonary functions. Thus, balanced regulation of ILC2 activation is critical for the treatment of sepsis, especially sepsis-induced pulmonary inflammation and ARDS.
Akama et al. investigated temporal changes in ILC2 functionality in the lungs of septic mice over time by examining the relationship of the ILC2 functions and the levels of lung injury in a cecum ligation and puncture (CLP) mouse sepsis model (111). The authors studied how the activities of ILC2s to produce the type 2 cytokine IL-13 correlated with the expressions of the stimulatory receptor ST2 and inhibitory receptor PD-1. IL-13 could exert a protective effect against sepsis by suppressing local inflammation, as shown by the previous study that antibody-mediated inhibition of IL-13 in the sepsis model increased macrophage inflammatory protein-2, macrophage inflammatory protein-1α and TNF-α levels (112). IL-33 is known to activate ILC-2 via ST2 receptor, thereby inducing the secretion of IL-13 that would promote the differentiation of macrophages to the anti-inflammatory M2 phenotype (113). The study has revealed that down-regulation of IL-13 in ILC2s correlated with the elevated expression of PD-1 on ILC2 in septic lungs, thereby suggesting that the PD-1/PD-L1 pathway in ILC2s functions as the inhibitory circuit to blunt type 2 immune response. IL-33 released from the injured pulmonary epithelial cells is necessary for the activation of ILC2 via the ST2 receptor. The induction of the type 2 immune response in a timely manner is critical for the resolution of inflammation, as shown by the experiment using IL-33 KO mice that lack the ILC2 activation (111). IL-33 KO mice showed delayed recovery from sepsis-induced systemic inflammation and wasting condition. Taken together, the perturbation of the PD-1/PD-L pathway in ILC2 could inhibit the induction of type 2 immune response in sepsis, thereby potentially compromising the resolution of inflammation.
PD-1 and PD-L1 on Exosomes
The inhibitory signals through PD-1 on T cells are usually elicited by the binding to PD-L1 present on the opposing cells. Recent investigations have revealed that exosomes function not only as an alternative vehicle of PD-L1 to induce signals through PD-1, but also as a specialized platform to induce more robust inhibitory signals. EVs are lipid bilayered nanoparticles that contain RNA, DNA and proteins, thereby playing a role in intracellular communication. It has been reported that the depletion of exosomal PD-L1 by a genetic manipulation reversed cancer-associated immune suppression despite the intact presence of PD-L1 on the cell surface (114). The depletion of exosomal PD-L1 also inhibits tumor growth and achieve survival in a mouse model, supporting the idea that it is exosomal but not cell surface PD-L1 that is responsible for PD-1-mediated immune suppression. Of note, exosomal PD-L1 appears to be resistant to anti-PD-L1 antibody blockades aimed to interfere with the interaction of PD-1 on T-cells with exosomal PD-L1 (114). Although underlying mechanisms are unclear, exosomes present deep in the core of the tumor microenvironment might be sequestered and unreachable from systemically administered antibodies.
The roles of exosomes in the pathogenesis of sepsis have attracted much attention (115). PD-L1 and PD-L2 on exosomes circulating in the plasma of sepsis patients have been investigated by Kawamoto et al. who revealed that the beta2 integrin and PD-L2 on exosomes increased levels during sepsis compared to non-septic SIRS and health volunteers (116). Whereas the levels of PD-L1 on exosomes did not change in sepsis, the amount of circulating soluble PD-L1 including exosomal PD-L1 increased in sepsis. The levels of soluble PD-L1 and the leukocytic beta2 integrin showed significant correlation to clinically defined organ dysfunctions such as kidney injuries. The pathological roles of exosomal PD-L1 and PD-L2 in sepsis-induced immune suppression warrant further investigations in the future.
Heterogeneity of Sepsis Syndrome: How the Main Obstacle for Clinical Trials Could Be Solved by AI
As a deeper understanding of the pathology underlying sepsis is being gained in the laboratories, many experimental therapeutic (but not prophylactic) treatments for inflammation suppression and immunomodulation have been successful in animal sepsis models (23–25). However, none of such treatments have proven effective in controlled clinical trials. The reason why they have not been successful in clinical trials could be animal models not recapitulating human sepsis pathophysiology as well as the diverse pathophysiology of human sepsis resulting in the heterogenous patient populations. Most animal sepsis models are developed in genetically homogenous laboratory animal strains using relatively unified “inducers”, such as administration of an endotoxin or bacterial peritonitis by CLP. In clinical practice, patients’ genetic and social backgrounds as well as types of sepsis inducers vary vastly; individual medical histories, the triggering infection, and the clinical course vary greatly among patients. Nonetheless, because sepsis is in fact a syndrome defined as a life-threatening organ dysfunction caused by a dysregulated host response to infection, it is diagnosed based on clinical symptoms and medical history, and not on the underlying molecular mechanisms, which can involve a wide variety of pathologies. At present, although sepsis has not been formally classified based on the underlying type of pathology, the identification of specific sepsis subgroups that could respond to a certain treatment is under intense investigations using a new enabling technology, AI. The drawback for classification is that sepsis has a clinically rapid time course compared to malignant tumors and chronic diseases. As the “Hour-1 Bundle” (117) proposal sets forth, medical professionals need to formulate a treatment policy as soon as a patient is diagnosed with sepsis. If lifesaving is to be successful, it is particularly important to detect sepsis using broad diagnostic criteria and not to miss those patients who need intensive care resources. It is extremely difficult for even a skilled clinician to classify sepsis based on the limited information typically available at the beginning of treatment. AI may make this possible.
Artificial Intelligence and Sepsis
Machine learning in big data analysis is a fast-growing area in recent years (118). Unlike traditional statistical analysis, the process of the optimized classification derived by machine learning, in particular that by deep learning, is not transparent at first glance and is difficult for humans to understand and interpret intuitively (119). Furthermore, deep learning models, which form a part of machine learning, use a deep neural network to create an optimal model from raw data and require less human guidance. For example, both the hypothesis and verification that low oxygen saturation is related to the over-expression of PD-L1 on monocytes, resulting in an impaired immune response during sepsis, is easy to understand from our clinical standpoint (120). It is assumed by many of us that these kinds of reasonable hypothesis-based research paradigms are representing the mainstream science and will continue to play some roles in the future. However, AI has already begun to change the framework of research on sepsis in various ways such as the realization of very early diagnosis and disease subgroup classification.
A very early diagnosis based on the unbiased prediction is where AI can surpass human physicians, thereby potentially innovating the management of sepsis. As mentioned above, in clinical practice, the injection of antibiotics cannot be delayed in patients with suspected sepsis. Patients with suspected sepsis must be given antibiotics that can adequately combat the causative organism and be collected blood cultures within 1 h (117). On the other hand, excessive administration of broad-spectrum antimicrobials can generate a hotbed for drug-resistant bacteria, which should be avoided from a public health perspective. A rapid, sensitive and specific diagnosis is desirable. It has been shown that an algorithm constructed by machine learning can recognize sepsis hours earlier than can be done by humans in clinical practice (121). In fact, an algorithm created by AI that can predict sepsis up to 48 h in advance has been reported (122). Achieving early diagnoses using multiple parameters is a capability unique to the innumerable calculations made possible by machines.
Predicting the deterioration of specific organ functions at an early stage is similarly a specialty of AI. Although early detection of acute kidney injury is difficult to detect as a clinical symptom, early detection of it reportedly leads to improved prognosis (123). In 2019, with the growing demand for early diagnosis of acute kidney injury, an AI was developed based on the data from more than 700,000 cases that could predict the onset of acute kidney injury requiring hemodialysis. Its reliability measured 90% or more (124). The AI algorithm developed by DeepMind, a subsidiary of Google, known for its successful development of AI capabilities AlphaGo and AlphaStar that exceed the human world champion level of a strategy board game Go. With the advent of deep learning, not only medical professionals and medical researchers but also information technology specialists and engineers/programmers/data scientists are becoming more involved in the advancement of medicine.
As with kidney injuries, AI can also be used to predict the onset of ARDS, which is a serious condition of the lungs (125, 126). Ventilation management is essential in severe ARDS and requires significant medical resources. As this has emerged as a major social problem with the COVID-19 (coronavirus disease 2019) pandemic, predicting the onset of ARDS is crucial to determining policies aiming to properly allocate the limited medical resources such as ventilators and ICU beds. In addition, deep learning, which has led to treatment strategies that can have made direct contributions to clinical practice, continues to advance (127). This study showed that AI could offer more reliable treatment strategies than human doctors. In one validation cohort, the mortality rate was lowest when the treatment strategy of the human clinician matched that proposed by AI.
In addition to the very early unbiased diagnosis of sepsis and organ failures, AI has made significant contributions to the clinically significant sub-grouping of sepsis. This could lead to the solution of the heterogeneity problem in sepsis, which underlies the difficulty of achieving translational success in the treatment of sepsis. In 2019, an innovative report was published in JAMA that sorted sepsis into four clinical phenotypes based on machine learning data analysis of 20,189 patients with sepsis (128). The raw data incorporated into this machine learning study were simply the parameters commonly collected through usual clinical practice: demographic information, laboratory abnormalities and organ dysfunctions. AI has achieved the unprecedented classification consisting of 4 sepsis subgroups: α, β, γ and δ that physicians have been unable to formulate on their own. The α phenotype shoewed neither abnormal blood results nor organ damage in α phenotype, thereby indicating the lowest in-hospital mortality rate. The β phenotype was older, had more chronic diseases, and suffered from renal dysfunction. The γ phenotype was marked by fever and elevated blood collection markers associated with inflammation and vascular endothelial damage. The δ phenotype showed the highest 28-day and 1-year mortality and hypotension and elevated serum lactate levels. Coagulation-related laboratory values were also notably elevated in this δ phenotype group. The four phenotypes of sepsis reported in the JAMA paper were different from the conventional classification system, which is based on the types of primary disease, organ damage, and severity of systematic conditions that are consistent with clinical impressions. (Figure 2)
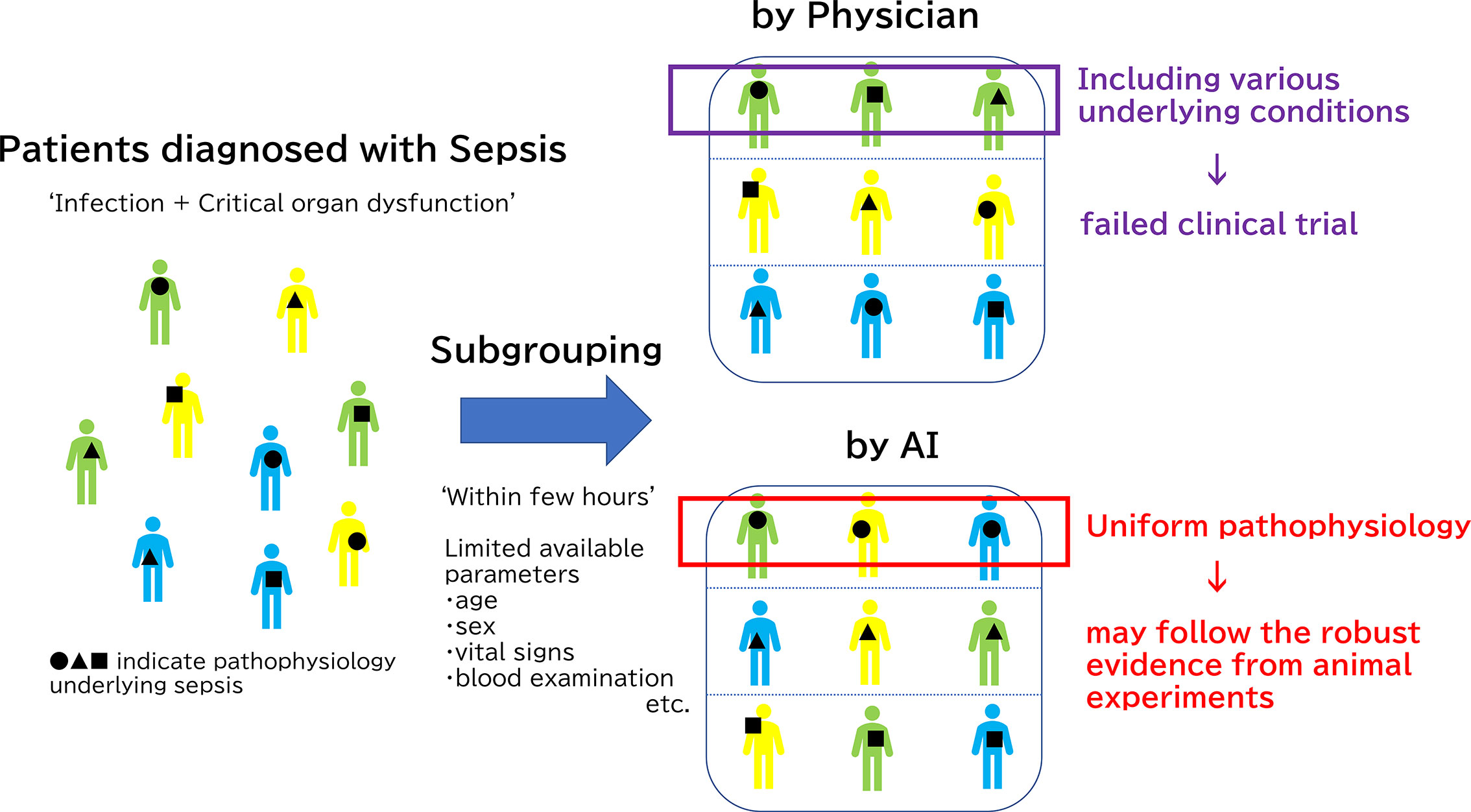
Figure 2 Subgrouping difference between human and Artificial Intelligence (AI). The reason why clinical trials have not been successful regardless of a deeper understanding of the pathology underlying sepsis is the diverse pathophysiology of sepsis in humans compared to animal sepsis models which are developed using relatively unified methods. Physicians usually classify septic patients by based on the types of primary disease, organ damage, and severity of systematic conditions that are consistent with clinical impressions. However, it is extremely difficult for even a skilled clinician to classify sepsis based on the limited information typically available at the beginning of treatment: medical history, vital signs, and few blood examinations. Thus, clinical trials could not have targeted specific patients. On the other hands, AI may achieve new classification, which is not transparent at first glance and is difficult for humans to understand, by machine learning. Only patients with immunoparalysis detected by AI should be treated with immune checkpoints inhibitors for successful clinical trials, for example.
As described earlier in this article, therapeutic agents used for immunothrombosis, such as activated protein C and thrombomodulin, failed to prove effective for sepsis in clinical trials (47, 48, 54). However, in the light of the AI-based new sub-group classification of sepsis, [e.g., α, β, γ, and δ phenotypes (128)], we may have to re-visit the experimental therapies. The formation of NETs is known to be involved in septic DIC, and the mechanism underlying neutrophil activation has been divided, in terms of molecular pathology, into two types; that by pathogen invasion and that by the indirect formation of suicidal NETs as a result of cytokine overproduction (129). The generation of NETs by the former mechanism can be suppressed by rhsTM (130). Even when the mechanism is examined at the molecular level, it remains impossible to recommend therapeutic agents based on the idea that sepsis is a singular condition. In other words, if the pathogenesis of NET can be inferred from clinical findings, clinical trials can be conducted only in those patients for whom rhsTM is effective (131). In this manner, results that might significantly improve patient prognosis could be obtained. It is reasonable to expect that AI will become capable of detecting cases of sepsis in which immunoparalysis is the main pathological condition, too. Targeting only the appropriate cases increases the likelihood that clinical trials will succeed.
AI has proven a powerful enabling technology in the management of sepsis, it is important to note that AI is not a panacea. The black-box problem and the frame problem are two major caveats that could potentially prevent the successful applications of AI in the field of medicine. The black box problem is that AI automatically makes an “optimized” decision for us without showing the process of optimization in such a way as human can understand intuitively. In the black-box model, we don’t usually have a clue as to whether the optimized decision made by AI is likely to be right or not. In an extreme scenario, we may not even know whether AI malfunctions as did HAL9000, a fictional AI in Arthur C Clarke’s Space Odyssey. In fact, malfunctioning of AI is not unreal in the face of the emergence of generative adversarial networks. While it is a merit of AI to be able to perform calculations that are not bound by human capacity and biases, applications of AI in medicine would demand a white-box aspect, in which the process of optimization is monitored and interpreted by human. Balancing interpretability and accuracy is an unresolved issue contemporarily. Further, the frame problem is an important one that has been pointed out for several decades (132). A myriad of events can happen in the real world, most of which have nothing to do with the immediate problem. A machine with only a finite amount of information processing power is not capable of dealing with all the possible real-world problems. AI related to sepsis has the limitation that a human must intervene, as it is necessary to specify which parameters should be used for learning. Fortunately, in the clinical setting of sepsis, the parameters available to the physician are limited to some demographic data, laboratory results, and vital signs due to time constraints. Therefore, realistically, almost all of these will be invested in the machine learning. However, creativity of adding more parameters, such as the addition of new blood tests, will still depend on humans.
Discussion and Summary
Numerous clinical trials, including translational research based on animal experiments for sepsis, have been conducted. Nonetheless, there is still no clinically established treatment that targets the underlying essence of sepsis; i.e., the deregulated immune response leading to the simultaneous co-existence of hyperinflammation and immunosuppression. Reversing sepsis-induced immune suppression by inhibiting the PD-1/PD-L pathway could represent a promising therapeutic approach, awaiting a validation in clinical trials. A new consensus has also emerged that PD-1/PD-L is not merely the cause of T-cell exhaustion, but also affects ILCs. Moreover, rather than focusing on the expression of PD-L1 on immune cells, tackling exosomal forms of PD-L1 and soluble PD-L1 could lead to a breakthrough. The essential treatment for sepsis in the future will likely involve immunomodulation by inhibiting the PD-1/PD-L1 pathway in immunoparalyzed patients. Even though the immunoparalyzed subgroup of sepsis was not extracted in the previous machine learning study (128), it is important to identify such subgroups of immunoparalyzed. In the future, data-driven, AI-assisted personalized sepsis treatment might become a reality.
Author Contributions
YN and MS contributed to the conceptualization, scope, and outline of this review. YN, EP, and MS analyzed the referenced manuscripts in this manuscript and participated in preparing the manuscript. All authors contributed to the article and approved the submitted version.
Funding
This work was supported by the Japan Society for the Promotion of Science KAKENHI Grants (YN, 20K17835).
Conflict of Interest
The authors declare that the research was conducted in the absence of any commercial or financial relationships that could be construed as a potential conflict of interest.
References
1. Mayr FB, Yende S, Angus DC. Epidemiology of severe sepsis. Virulence (2014) 5(1):4–11. doi: 10.4161/viru.27372
2. Martin GS, Mannino DM, Eaton S, Moss M. The epidemiology of sepsis in the United States from 1979 through 2000. New Engl J Med (2003) 348(16):1546–54. doi: 10.1056/NEJMoa022139
3. Reinhart K, Daniels R, Kissoon N, Machado FR, Schachter RD, Finfer S. Recognizing Sepsis as a Global Health Priority - A WHO Resolution. New Engl J Med (2017) 377(5):414–7. doi: 10.1056/NEJMp1707170
4. Dellinger RP, Carlet JM, Masur H, Gerlach H, Calandra T, Cohen J, et al. Surviving Sepsis Campaign guidelines for management of severe sepsis and septic shock. Intensive Care Med (2004) 30(4):536–55. doi: 10.1007/s00134-004-2210-z
5. Kaukonen KM, Bailey M, Suzuki S, Pilcher D, Bellomo R. Mortality related to severe sepsis and septic shock among critically ill patients in Australia and New Zealand, 2000-2012. Jama (2014) 311(13):1308–16. doi: 10.1001/jama.2014.2637
6. Vincent JL, Marshall JC, Namendys-Silva SA, François B, Martin-Loeches I, Lipman J, et al. Assessment of the worldwide burden of critical illness: the intensive care over nations (ICON) audit. Lancet Respir Med (2014) 2(5):380–6. doi: 10.1016/s2213-2600(14)70061-x
7. Vincent JL, Sakr Y, Sprung CL, Ranieri VM, Reinhart K, Gerlach H, et al. Sepsis in European intensive care units: results of the SOAP study. Crit Care Med (2006) 34(2):344–53. doi: 10.1097/01.ccm.0000194725.48928.3a
8. van der Poll T, van de Veerdonk FL, Scicluna BP, Netea MG. The immunopathology of sepsis and potential therapeutic targets. Nat Rev Immunol (2017) 17(7):407–20. doi: 10.1038/nri.2017.36
9. Venet F, Monneret G. Advances in the understanding and treatment of sepsis-induced immunosuppression. Nat Rev Nephrol (2018) 14(2):121–37. doi: 10.1038/nrneph.2017.165
10. Bone RC, Sibbald WJ, Sprung CL. The ACCP-SCCM consensus conference on sepsis and organ failure. Chest (1992) 101(6):1481–3. doi: 10.1378/chest.101.6.1481
11. Singer M, Deutschman CS, Seymour CW, Shankar-Hari M, Annane D, Bauer M, et al. The Third International Consensus Definitions for Sepsis and Septic Shock (Sepsis-3). Jama (2016) 315(8):801–10. doi: 10.1001/jama.2016.0287
12. Bone RC. Sir Isaac Newton, sepsis, SIRS, and CARS. Crit Care Med (1996) 24(7):1125–8. doi: 10.1097/00003246-199607000-00010
13. Xiao W, Mindrinos MN, Seok J, Cuschieri J, Cuenca AG, Gao H, et al. A genomic storm in critically injured humans. The Journal of experimental medicine. Experiment Med (2011) 208: (13):2581–90. doi: 10.1084/jem.20111354
14. Xiao W, Mindrinos MN, Seok J, Cuschieri J, Cuenca AG, Gao H, et al. A genomic storm in critically injured humans. J Exp Med (2011) 208(13):2581–90. doi: 10.1084/jem.20111354
15. Gentile LF, Cuenca AG, Efron PA, Ang D, Bihorac A, McKinley BA, et al. Persistent inflammation and immunosuppression: a common syndrome and new horizon for surgical intensive care. J Trauma Acute Care Surg (2012) 72(6):1491–501. doi: 10.1097/TA.0b013e318256e000
16. Takeuchi O, Akira S. Pattern recognition receptors and inflammation. Cell (2010) 140(6):805–20. doi: 10.1016/j.cell.2010.01.022
17. Tang D, Kang R, Coyne CB, Zeh HJ, Lotze MT. PAMPs and DAMPs: signal 0s that spur autophagy and immunity. Immunol Rev (2012) 249(1):158–75. doi: 10.1111/j.1600-065X.2012.01146.x
18. Chan JK, Roth J, Oppenheim JJ, Tracey KJ, Vogl T, Feldmann M, et al. Alarmins: awaiting a clinical response. J Clin Invest (2012) 122(8):2711–9. doi: 10.1172/jci62423
19. Liu X, Wang Q, Chen W, Wang C. Dynamic regulation of innate immunity by ubiquitin and ubiquitin-like proteins. Cytokine Growth Factor Rev (2013) 24(6):559–70. doi: 10.1016/j.cytogfr.2013.07.002
20. McNab F, Mayer-Barber K, Sher A, Wack A, O’Garra A. Type I interferons in infectious disease. Nat Rev Immunol (2015) 15(2):87–103. doi: 10.1038/nri3787
21. Adorisio S, Fierabracci A, Muscari I, Liberati AM, Ayroldi E, Migliorati G, et al. SUMO proteins: Guardians of immune system. J Autoimmun (2017) 84:21–8. doi: 10.1016/j.jaut.2017.09.001
22. Chang TH, Xu S, Tailor P, Kanno T, Ozato K. The small ubiquitin-like modifier-deconjugating enzyme sentrin-specific peptidase 1 switches IFN regulatory factor 8 from a repressor to an activator during macrophage activation. J Immunol (Baltimore Md 1950) (2012) 189(7):3548–56. doi: 10.4049/jimmunol.1201104
23. Benjamim CF, Lundy SK, Lukacs NW, Hogaboam CM, Kunkel SL. Reversal of long-term sepsis-induced immunosuppression by dendritic cells. Blood (2005) 105(9):3588–95. doi: 10.1182/blood-2004-08-3251
24. Unsinger J, Burnham CA, McDonough J, Morre M, Prakash PS, Caldwell CC, et al. Interleukin-7 ameliorates immune dysfunction and improves survival in a 2-hit model of fungal sepsis. J Infect Dis (2012) 206(4):606–16. doi: 10.1093/infdis/jis383
25. Shindo Y, Fuchs AG, Davis CG, Eitas T, Unsinger J, Burnham CD, et al. Interleukin 7 immunotherapy improves host immunity and survival in a two-hit model of Pseudomonas aeruginosa pneumonia. J Leukocyte Biol (2017) 101(2):543–54. doi: 10.1189/jlb.4A1215-581R
26. Cooper MS, Stewart PM. Corticosteroid insufficiency in acutely ill patients. New Engl J Med (2003) 348(8):727–34. doi: 10.1056/NEJMra020529
27. Annane D, Pastores SM, Rochwerg B, Arlt W, Balk RA, Beishuizen A, et al. Guidelines for the diagnosis and management of critical illness-related corticosteroid insufficiency (CIRCI) in critically ill patients (Part I): Society of Critical Care Medicine (SCCM) and European Society of Intensive Care Medicine (ESICM) 2017. Intensive Care Med (2017) 43(12):1751–63. doi: 10.1007/s00134-017-4919-5
28. Heming N, Sivanandamoorthy S, Meng P, Bounab R, Annane D. Immune Effects of Corticosteroids in Sepsis. Front Immunol (2018) 9:1736. doi: 10.3389/fimmu.2018.01736
29. Annane D, Renault A, Brun-Buisson C, Megarbane B, Quenot JP, Siami S, et al. Hydrocortisone plus Fludrocortisone for Adults with Septic Shock. New Engl J Med (2018) 378(9):809–18. doi: 10.1056/NEJMoa1705716
30. Venkatesh B, Finfer S, Cohen J, Rajbhandari D, Arabi Y, Bellomo R, et al. Adjunctive Glucocorticoid Therapy in Patients with Septic Shock. New Engl J Med (2018) 378(9):797–808. doi: 10.1056/NEJMoa1705835
31. Michie HR, Manogue KR, Spriggs DR, Revhaug A, O’Dwyer S, Dinarello CA, et al. Detection of circulating tumor necrosis factor after endotoxin administration. New Engl J Med (1988) 318(23):1481–6. doi: 10.1056/nejm198806093182301
32. Bauss F, Dröge W, Männel DN. Tumor necrosis factor mediates endotoxic effects in mice. Infect Immun (1987) 55(7):1622–5. doi: 10.1128/iai.55.7.1622-1625.1987
33. van der Poll T, Büller HR, ten Cate H, Wortel CH, Bauer KA, van Deventer SJ, et al. Activation of coagulation after administration of tumor necrosis factor to normal subjects. New Engl J Med (1990) 322(23):1622–7. doi: 10.1056/nejm199006073222302
34. Beutler B, Milsark IW, Cerami AC. Passive immunization against cachectin/tumor necrosis factor protects mice from lethal effect of endotoxin. Sci (N Y NY) (1985) 229(4716):869–71. doi: 10.1126/science.3895437
35. Tracey KJ, Fong Y, Hesse DG, Manogue KR, Lee AT, Kuo GC, et al. Anti-cachectin/TNF monoclonal antibodies prevent septic shock during lethal bacteraemia. Nature (1987) 330(6149):662–4. doi: 10.1038/330662a0
36. Fisher CJ Jr., Agosti JM, Opal SM, Lowry SF, Balk RA, Sadoff JC, et al. Treatment of septic shock with the tumor necrosis factor receptor:Fc fusion protein. The Soluble TNF Receptor Sepsis Study Group. New Engl J Med (1996) 334(26):1697–702. doi: 10.1056/nejm199606273342603
37. Moreland LW, Baumgartner SW, Schiff MH, Tindall EA, Fleischmann RM, Weaver AL, et al. Treatment of rheumatoid arthritis with a recombinant human tumor necrosis factor receptor (p75)-Fc fusion protein. New Engl J Med (1997) 337(3):141–7. doi: 10.1056/nejm199707173370301
38. Aird WC. The role of the endothelium in severe sepsis and multiple organ dysfunction syndrome. Blood (2003) 101(10):3765–77. doi: 10.1182/blood-2002-06-1887
39. Engelmann B, Massberg S. Thrombosis as an intravascular effector of innate immunity. Nat Rev Immunol (2013) 13(1):34–45. doi: 10.1038/nri3345
40. de Stoppelaar SF, van ‘t Veer C, van der Poll T. The role of platelets in sepsis. Thromb Haemostasis (2014) 112(4):666–77. doi: 10.1160/th14-02-0126
41. Gando S, Kameue T, Morimoto Y, Matsuda N, Hayakawa M, Kemmotsu O. Tissue factor production not balanced by tissue factor pathway inhibitor in sepsis promotes poor prognosis. Crit Care Med (2002) 30(8):1729–34. doi: 10.1097/00003246-200208000-00009
42. Esmon CT. The protein C anticoagulant pathway. Arterioscler Thromb J Vasc Biol (1992) 12(2):135–45. doi: 10.1161/01.atv.12.2.135
43. Sarangi PP, Lee HW, Lerman YV, Trzeciak A, Harrower EJ, Rezaie AR, et al. Activated Protein C Attenuates Severe Inflammation by Targeting VLA-3(high) Neutrophil Subpopulation in Mice. J Immunol (Baltimore Md 1950) (2017) 199(8):2930–6. doi: 10.4049/jimmunol.1700541
44. Healy LD, Puy C, Fernández JA, Mitrugno A, Keshari RS, Taku NA, et al. Activated protein C inhibits neutrophil extracellular trap formation in vitro and activation in vivo. J Biol Chem (2017) 292(21):8616–29. doi: 10.1074/jbc.M116.768309
45. Boehme MW, Deng Y, Raeth U, Bierhaus A, Ziegler R, Stremmel W, et al. Release of thrombomodulin from endothelial cells by concerted action of TNF-alpha and neutrophils: in vivo and in vitro studies. Immunology (1996) 87(1):134–40.
46. Bernard GR, Vincent JL, Laterre PF, LaRosa SP, Dhainaut JF, Lopez-Rodriguez A, et al. Efficacy and safety of recombinant human activated protein C for severe sepsis. New Engl J Med (2001) 344(10):699–709. doi: 10.1056/nejm200103083441001
47. Martí-Carvajal A, Salanti G, Cardona AF. Human recombinant activated protein C for severe sepsis. Cochrane Database Syst Rev (2008) 1):Cd004388. doi: 10.1002/14651858.CD004388.pub3
48. Ranieri VM, Thompson BT, Barie PS, Dhainaut JF, Douglas IS, Finfer S, et al. Drotrecogin alfa (activated) in adults with septic shock. New Engl J Med (2012) 366(22):2055–64. doi: 10.1056/NEJMoa1202290
49. Okamoto T, Tanigami H, Suzuki K, Shimaoka M. Thrombomodulin: a bifunctional modulator of inflammation and coagulation in sepsis. Crit Care Res Pract (2012) 2012:614545. doi: 10.1155/2012/614545
50. Abeyama K, Stern DM, Ito Y, Kawahara K, Yoshimoto Y, Tanaka M, et al. The N-terminal domain of thrombomodulin sequesters high-mobility group-B1 protein, a novel antiinflammatory mechanism. J Clin Invest (2005) 115(5):1267–74. doi: 10.1172/jci22782
51. Kawamoto E, Okamoto T, Takagi Y, Honda G, Suzuki K, Imai H, et al. LFA-1 and Mac-1 integrins bind to the serine/threonine-rich domain of thrombomodulin. Biochem Biophys Res Commun (2016) 473(4):1005–12. doi: 10.1016/j.bbrc.2016.04.007
52. Delvaeye M, Noris M, De Vriese A, Esmon CT, Esmon NL, Ferrell G, et al. Thrombomodulin mutations in atypical hemolytic-uremic syndrome. New Engl J Med (2009) 361(4):345–57. doi: 10.1056/NEJMoa0810739
53. Merle NS, Noe R, Halbwachs-Mecarelli L, Fremeaux-Bacchi V, Roumenina LT. Complement System Part II: Role in Immunity. Front Immunol (2015) 6:257:257. doi: 10.3389/fimmu.2015.00257
54. Vincent JL, Francois B, Zabolotskikh I, Daga MK, Lascarrou JB, Kirov MY, et al. Effect of a Recombinant Human Soluble Thrombomodulin on Mortality in Patients With Sepsis-Associated Coagulopathy: The SCARLET Randomized Clinical Trial. Jama (2019) 321(20):1993–2002. doi: 10.1001/jama.2019.5358
55. Otto GP, Sossdorf M, Claus RA, Rödel J, Menge K, Reinhart K, et al. The late phase of sepsis is characterized by an increased microbiological burden and death rate. Crit Care (2011) 15(4):R183–R. doi: 10.1186/cc10332
56. Hoogendijk AJ, Garcia-Laorden MI, van Vught LA, Wiewel MA, Belkasim-Bohoudi H, Duitman J, et al. Sepsis Patients Display a Reduced Capacity to Activate Nuclear Factor-κB in Multiple Cell Types. Crit Care Med (2017) 45(5):e524–e31. doi: 10.1097/ccm.0000000000002294
57. Lamkanfi M, Moreira LO, Makena P, Spierings DC, Boyd K, Murray PJ, et al. Caspase-7 deficiency protects from endotoxin-induced lymphocyte apoptosis and improves survival. Blood (2009) 113(12):2742–5. doi: 10.1182/blood-2008-09-178038
58. Jan R, Chaudhry GE. Understanding Apoptosis and Apoptotic Pathways Targeted Cancer Therapeutics. Advanced Pharm Bull (2019) 9(2):205–18. doi: 10.15171/apb.2019.024
59. Bredesen DE, Rao RV, Mehlen P. Cell death in the nervous system. Nature (2006) 443(7113):796–802. doi: 10.1038/nature05293
60. Kim R. Recent advances in understanding the cell death pathways activated by anticancer therapy. Cancer (2005) 103(8):1551–60. doi: 10.1002/cncr.20947
61. Yuan S, Akey CW. Apoptosome structure, assembly, and procaspase activation. Struct (London Engl 1993) (2013) 21(4):501–15. doi: 10.1016/j.str.2013.02.024
62. Hotchkiss RS, Nicholson DW. Apoptosis and caspases regulate death and inflammation in sepsis. Nat Rev Immunol (2006) 6(11):813–22. doi: 10.1038/nri1943
63. Boomer JS, To K, Chang KC, Takasu O, Osborne DF, Walton AH, et al. Immunosuppression in patients who die of sepsis and multiple organ failure. JAMA (2011) 306(23):2594–605. doi: 10.1001/jama.2011.1829
64. Byrne A, Reen DJ. Lipopolysaccharide induces rapid production of IL-10 by monocytes in the presence of apoptotic neutrophils. J Immunol (Baltimore Md 1950) (2002) 168(4):1968–77. doi: 10.4049/jimmunol.168.4.1968
65. Hotchkiss RS, Swanson PE, Freeman BD, Tinsley KW, Cobb JP, Matuschak GM, et al. Apoptotic cell death in patients with sepsis, shock, and multiple organ dysfunction. Crit Care Med (1999) 27(7):1230–51. doi: 10.1097/00003246-199907000-00002
66. Pelaia G, Vatrella A, Maselli R. The potential of biologics for the treatment of asthma. Nat Rev Drug Discovery (2012) 11(12):958–72. doi: 10.1038/nrd3792
67. Goswami R. Th9 Cells: New Member of T Helper Cell Family. Methods Mol Biol (Clifton NJ) (2017) 1585:1–19. doi: 10.1007/978-1-4939-6877-0_1
68. Hirahara K, Nakayama T. CD4+ T-cell subsets in inflammatory diseases: beyond the Th1/Th2 paradigm. Int Immunol (2016) 28(4):163–71. doi: 10.1093/intimm/dxw006
69. Wherry EJ, Kurachi M. Molecular and cellular insights into T cell exhaustion. Nat Rev Immunol (2015) 15(8):486–99. doi: 10.1038/nri3862
70. Hotchkiss RS, Tinsley KW, Swanson PE, Grayson MH, Osborne DF, Wagner TH, et al. Depletion of dendritic cells, but not macrophages, in patients with sepsis. J Immunol (Baltimore Md 1950) (2002) 168(5):2493–500. doi: 10.4049/jimmunol.168.5.2493
71. Hynninen M, Pettilä V, Takkunen O, Orko R, Jansson SE, Kuusela P, et al. Predictive value of monocyte histocompatibility leukocyte antigen-DR expression and plasma interleukin-4 and -10 levels in critically ill patients with sepsis. Shock (Augusta Ga) (2003) 20(1):1–4. doi: 10.1097/01.shk.0000068322.08268.b4
72. Darkwah S, Nago N, Appiah MG, Myint PK, Kawamoto E, Shimaoka M, et al. Differential Roles of Dendritic Cells in Expanding CD4 T Cells in Sepsis. Biomedicines (2019) 7(3):52. doi: 10.3390/biomedicines7030052
73. Scumpia PO, McAuliffe PF, O’Malley KA, Ungaro R, Uchida T, Matsumoto T, et al. CD11c+ dendritic cells are required for survival in murine polymicrobial sepsis. J Immunol (Baltimore Md 1950) (2005) 175(5):3282–6. doi: 10.4049/jimmunol.175.5.3282
74. Venet F, Pachot A, Debard AL, Bohe J, Bienvenu J, Lepape A, et al. Human CD4+CD25+ regulatory T lymphocytes inhibit lipopolysaccharide-induced monocyte survival through a Fas/Fas ligand-dependent mechanism. J Immunol (Baltimore Md 1950) (2006) 177(9):6540–7. doi: 10.4049/jimmunol.177.9.6540
75. Scumpia PO, Delano MJ, Kelly-Scumpia KM, Weinstein JS, Wynn JL, Winfield RD, et al. Treatment with GITR agonistic antibody corrects adaptive immune dysfunction in sepsis. Blood (2007) 110(10):3673–81. doi: 10.1182/blood-2007-04-087171
76. Gabrilovich DI. Myeloid-Derived Suppressor Cells. Cancer Immunol Res (2017) 5(1):3–8. doi: 10.1158/2326-6066.cir-16-0297
77. Cuenca AG, Delano MJ, Kelly-Scumpia KM, Moreno C, Scumpia PO, Laface DM, et al. A paradoxical role for myeloid-derived suppressor cells in sepsis and trauma. Mol Med (Cambridge Mass) (2011) 17(3-4):281–92. doi: 10.2119/molmed.2010.00178
78. Albertsmeier M, Prix NJ, Winter H, Bazhin A, Werner J, Angele MK. Monocyte-Dependent Suppression of T-Cell Function in Postoperative Patients and Abdominal Sepsis. Shock (Augusta Ga) (2017) 48(6):651–6. doi: 10.1097/shk.0000000000000924
79. Uhel F, Azzaoui I, Grégoire M, Pangault C, Dulong J, Tadié JM, et al. Early Expansion of Circulating Granulocytic Myeloid-derived Suppressor Cells Predicts Development of Nosocomial Infections in Patients with Sepsis. Am J Respir Crit Care Med (2017) 196(3):315–27. doi: 10.1164/rccm.201606-1143OC
80. Leach DR, Krummel MF, Allison JP. Enhancement of antitumor immunity by CTLA-4 blockade. Sci (N Y NY) (1996) 271(5256):1734–6. doi: 10.1126/science.271.5256.1734
81. Hoos A, Ibrahim R, Korman A, Abdallah K, Berman D, Shahabi V, et al. Development of ipilimumab: contribution to a new paradigm for cancer immunotherapy. Semin Oncol (2010) 37(5):533–46. doi: 10.1053/j.seminoncol.2010.09.015
82. Arce Vargas F, Furness AJS, Litchfield K, Joshi K, Rosenthal R, Ghorani E, et al. Fc Effector Function Contributes to the Activity of Human Anti-CTLA-4 Antibodies. Cancer Cell (2018) 33(4):649–63.e4. doi: 10.1016/j.ccell.2018.02.010
83. Hotchkiss RS, Moldawer LL. Parallels between cancer and infectious disease. New Engl J Med (2014) 371(4):380–3. doi: 10.1056/NEJMcibr1404664
84. Voerman HJ, van Schijndel RJ, Groeneveld AB, de Boer H, Nauta JP, van der Veen EA, et al. Effects of recombinant human growth hormone in patients with severe sepsis. Ann Surg (1992) 216(6):648–55. doi: 10.1097/00000658-199212000-00006
85. Vara-Thorbeck R, Guerrero JA, Rosell J, Ruiz-Requena E, Capitán JM. Exogenous growth hormone: effects on the catabolic response to surgically produced acute stress and on postoperative immune function. World J Surg (1993) 17(4):530–7; discussion 7-8. doi: 10.1007/bf01655116
86. Takala J, Ruokonen E, Webster NR, Nielsen MS, Zandstra DF, Vundelinckx G, et al. Increased mortality associated with growth hormone treatment in critically ill adults. New Engl J Med (1999) 341(11):785–92. doi: 10.1056/nejm199909093411102
87. McAuley DF, Cross LM, Hamid U, Gardner E, Elborn JS, Cullen KM, et al. Keratinocyte growth factor for the treatment of the acute respiratory distress syndrome (KARE): a randomised, double-blind, placebo-controlled phase 2 trial. Lancet Respir Med (2017) 5(6):484–91. doi: 10.1016/s2213-2600(17)30171-6
88. Döcke WD, Randow F, Syrbe U, Krausch D, Asadullah K, Reinke P, et al. Monocyte deactivation in septic patients: restoration by IFN-gamma treatment. Nat Med (1997) 3(6):678–81. doi: 10.1038/nm0697-678
89. Bo L, Wang F, Zhu J, Li J, Deng X. Granulocyte-colony stimulating factor (G-CSF) and granulocyte-macrophage colony stimulating factor (GM-CSF) for sepsis: a meta-analysis. Crit Care (2011) 15(1):R58–R. doi: 10.1186/cc10031
90. Mackall CL, Fry TJ, Gress RE. Harnessing the biology of IL-7 for therapeutic application. Nat Rev Immunol (2011) 11(5):330–42. doi: 10.1038/nri2970
91. Venet F, Demaret J, Blaise BJ, Rouget C, Girardot T, Idealisoa E, et al. IL-7 Restores T Lymphocyte Immunometabolic Failure in Septic Shock Patients through mTOR Activation. J Immunol (Baltimore Md 1950) (2017) 199(5):1606–15. doi: 10.4049/jimmunol.1700127
92. Francois B, Jeannet R, Daix T, Walton AH, Shotwell MS, Unsinger J, et al. Interleukin-7 restores lymphocytes in septic shock: the IRIS-7 randomized clinical trial. JCI Insight (2018) 3(5):e98960. doi: 10.1172/jci.insight.98960
93. Bretscher P, Cohn M. A theory of self-nonself discrimination. Sci (N Y NY) (1970) 169(3950):1042–9. doi: 10.1126/science.169.3950.1042
94. Francisco LM, Sage PT, Sharpe AH. The PD-1 pathway in tolerance and autoimmunity. Immunol Rev (2010) 236:219–42. doi: 10.1111/j.1600-065X.2010.00923.x
95. Dai S, Jia R, Zhang X, Fang Q, Huang L. The PD-1/PD-Ls pathway and autoimmune diseases. Cell Immunol (2014) 290(1):72–9. doi: 10.1016/j.cellimm.2014.05.006
96. Francisco LM, Salinas VH, Brown KE, Vanguri VK, Freeman GJ, Kuchroo VK, et al. PD-L1 regulates the development, maintenance, and function of induced regulatory T cells. J Exp Med (2009) 206(13):3015–29. doi: 10.1084/jem.20090847
97. Topalian SL, Hodi FS, Brahmer JR, Gettinger SN, Smith DC, McDermott DF, et al. Safety, activity, and immune correlates of anti-PD-1 antibody in cancer. New Engl J Med (2012) 366(26):2443–54. doi: 10.1056/NEJMoa1200690
98. Wolchok JD, Kluger H, Callahan MK, Postow MA, Rizvi NA, Lesokhin AM, et al. Nivolumab plus ipilimumab in advanced melanoma. New Engl J Med (2013) 369(2):122–33. doi: 10.1056/NEJMoa1302369
99. Vincent JL. Emerging therapies for the treatment of sepsis. Curr Opin Anaesthesiol (2015) 28(4):411–6. doi: 10.1097/aco.0000000000000210
100. Zhang Y, Li J, Lou J, Zhou Y, Bo L, Zhu J, et al. Upregulation of programmed death-1 on T cells and programmed death ligand-1 on monocytes in septic shock patients. Crit Care (2011) 15(1):R70–R. doi: 10.1186/cc10059
101. Shao R, Fang Y, Yu H, Zhao L, Jiang Z, Li CS. Monocyte programmed death ligand-1 expression after 3-4 days of sepsis is associated with risk stratification and mortality in septic patients: a prospective cohort study. Crit Care (2016) 20(1):124. doi: 10.1186/s13054-016-1301-x
102. Huang X, Venet F, Wang YL, Lepape A, Yuan Z, Chen Y, et al. PD-1 expression by macrophages plays a pathologic role in altering microbial clearance and the innate inflammatory response to sepsis. Proc Natl Acad Sci United States America (2009) 106(15):6303–8. doi: 10.1073/pnas.0809422106
103. Zhang Y, Zhou Y, Lou J, Li J, Bo L, Zhu K, et al. PD-L1 blockade improves survival in experimental sepsis by inhibiting lymphocyte apoptosis and reversing monocyte dysfunction. Crit Care (2010) 14(6):R220. doi: 10.1186/cc9354
104. Chang KC, Burnham CA, Compton SM, Rasche DP, Mazuski RJ, McDonough JS, et al. Blockade of the negative co-stimulatory molecules PD-1 and CTLA-4 improves survival in primary and secondary fungal sepsis. Crit Care (2013) 17(3):R85. doi: 10.1186/cc12711
105. Brahmamdam P, Inoue S, Unsinger J, Chang KC, McDunn JE, Hotchkiss RS. Delayed administration of anti-PD-1 antibody reverses immune dysfunction and improves survival during sepsis. J Leukocyte Biol (2010) 88(2):233–40. doi: 10.1189/jlb.0110037
106. Hotchkiss RS, Colston E, Yende S, Crouser ED, Martin GS, Albertson T, et al. Immune checkpoint inhibition in sepsis: a Phase 1b randomized study to evaluate the safety, tolerability, pharmacokinetics, and pharmacodynamics of nivolumab. Intensive Care Med (2019) 45(10):1360–71. doi: 10.1007/s00134-019-05704-z
107. Hotchkiss RS, Colston E, Yende S, Angus DC, Moldawer LL, Crouser ED, et al. Immune Checkpoint Inhibition in Sepsis: A Phase 1b Randomized, Placebo-Controlled, Single Ascending Dose Study of Antiprogrammed Cell Death-Ligand 1 Antibody (BMS-936559). Crit Care Med (2019) 47(5):632–42. doi: 10.1097/ccm.0000000000003685
108. Vivier E, Artis D, Colonna M, Diefenbach A, Di Santo JP, Eberl G, et al. Innate Lymphoid Cells: 10 Years On. Cell (2018) 174(5):1054–66. doi: 10.1016/j.cell.2018.07.017
109. Sonnenberg GF, Hepworth MR. Functional interactions between innate lymphoid cells and adaptive immunity. Nat Rev Immunol (2019) 19(10):599–613. doi: 10.1038/s41577-019-0194-8
110. Akama Y, Satoh-Takayama N, Kawamoto E, Ito A, Gaowa A, Park EJ, et al. The Role of Innate Lymphoid Cells in the Regulation of Immune Homeostasis in Sepsis-Mediated Lung Inflammation. Diagnostics (Basel Switzerland) (2020) 10(10):808. doi: 10.3390/diagnostics10100808
111. Akama Y, Park EJ, Satoh-Takayama N, Gaowa A, Ito A, Kawamoto E, et al. Sepsis Induces Deregulation of IL-13 Production and PD-1 Expression in Lung Group 2 Innate Lymphoid Cells. Shock (Augusta Ga) (2020). doi: 10.1097/shk.0000000000001647
112. Matsukawa A, Hogaboam CM, Lukacs NW, Lincoln PM, Evanoff HL, Strieter RM, et al. Expression and contribution of endogenous IL-13 in an experimental model of sepsis. J Immunol (Baltimore Md 1950) (2000) 164(5):2738–44. doi: 10.4049/jimmunol.164.5.2738
113. Nascimento DC, Melo PH, Piñeros AR, Ferreira RG, Colón DF, Donate PB, et al. IL-33 contributes to sepsis-induced long-term immunosuppression by expanding the regulatory T cell population. Nat Commun (2017) 8:14919. doi: 10.1038/ncomms14919
114. Poggio M, Hu T, Pai CC, Chu B, Belair CD, Chang A, et al. Suppression of Exosomal PD-L1 Induces Systemic Anti-tumor Immunity and Memory. Cell (2019) 177(2):414–27.e13. doi: 10.1016/j.cell.2019.02.016
115. Park EJ, Appiah MG, Myint PK, Gaowa A, Kawamoto E, Shimaoka M. Exosomes in Sepsis and Inflammatory Tissue Injury. Curr Pharm Design (2019) 25(42):4486–95. doi: 10.2174/1381612825666191116125525
116. Kawamoto E, Masui-Ito A, Eguchi A, Soe ZY, Prajuabjinda O, Darkwah S, et al. Integrin and PD-1 Ligand Expression on Circulating Extracellular Vesicles in Systemic Inflammatory Response Syndrome and Sepsis. Shock (Augusta Ga) (2019) 52(1):13–22. doi: 10.1097/shk.0000000000001228
117. Levy MM, Evans LE, Rhodes A. The Surviving Sepsis Campaign Bundle: 2018 Update. Crit Care Med (2018) 46(6):997–1000. doi: 10.1097/ccm.0000000000003119
118. Beam AL, Kohane IS. Big Data and Machine Learning in Health Care. Jama (2018) 319(13):1317–8. doi: 10.1001/jama.2017.18391
119. Sidey-Gibbons JAM, Sidey-Gibbons CJ. Machine learning in medicine: a practical introduction. BMC Med Res Method (2019) 19(1):64. doi: 10.1186/s12874-019-0681-4
120. Avendaño-Ortiz J, Maroun-Eid C, Martín-Quirós A, Lozano-Rodríguez R, Llanos-González E, Toledano V, et al. Oxygen Saturation on Admission Is a Predictive Biomarker for PD-L1 Expression on Circulating Monocytes and Impaired Immune Response in Patients With Sepsis. Front Immunol (2018) 9:2008. doi: 10.3389/fimmu.2018.02008
121. Reyna MA, Josef CS, Jeter R, Shashikumar SP, Westover MB, Nemati S, et al. Early Prediction of Sepsis From Clinical Data: The PhysioNet/Computing in Cardiology Challenge 2019. Crit Care Med (2020) 48(2):210–7. doi: 10.1097/ccm.0000000000004145
122. Burdick H, Pino E, Gabel-Comeau D, Gu C, Roberts J, Le S, et al. Validation of a machine learning algorithm for early severe sepsis prediction: a retrospective study predicting severe sepsis up to 48 h in advance using a diverse dataset from 461 US hospitals. BMC Med Inf Decision Making (2020) 20(1):276. doi: 10.1186/s12911-020-01284-x
123. Malhotra R, Siew ED. Biomarkers for the Early Detection and Prognosis of Acute Kidney Injury. Clin J Am Soc Nephrol (2017) 12(1):149–73. doi: 10.2215/CJN.01300216
124. Tomašev N, Glorot X, Rae JW, Zielinski M, Askham H, Saraiva A, et al. A clinically applicable approach to continuous prediction of future acute kidney injury. Nature (2019) 572(7767):116–9. doi: 10.1038/s41586-019-1390-1
125. Zeiberg D, Prahlad T, Nallamothu BK, Iwashyna TJ, Wiens J, Sjoding MW. Machine learning for patient risk stratification for acute respiratory distress syndrome. PloS One (2019) 14(3):e0214465. doi: 10.1371/journal.pone.0214465
126. Le S, Pellegrini E, Green-Saxena A, Summers C, Hoffman J, Calvert J, et al. Supervised machine learning for the early prediction of acute respiratory distress syndrome (ARDS). J Crit Care (2020) 60:96–102. doi: 10.1016/j.jcrc.2020.07.019
127. Komorowski M, Celi LA, Badawi O, Gordon AC, Faisal AA. The Artificial Intelligence Clinician learns optimal treatment strategies for sepsis in intensive care. Nat Med (2018) 24(11):1716–20. doi: 10.1038/s41591-018-0213-5
128. Seymour CW, Kennedy JN, Wang S, Chang CH, Elliott CF, Xu Z, et al. Derivation, Validation, and Potential Treatment Implications of Novel Clinical Phenotypes for Sepsis. Jama (2019) 321(20):2003–17. doi: 10.1001/jama.2019.5791
129. Yipp BG, Kubes P. NETosis: how vital is it? Blood (2013) 122(16):2784–94. doi: 10.1182/blood-2013-04-457671
130. Shimomura Y, Suga M, Kuriyama N, Nakamura T, Sakai T, Kato Y, et al. Recombinant human thrombomodulin inhibits neutrophil extracellular trap formation in vitro. J Intensive Care (2016) 4:48. doi: 10.1186/s40560-016-0177-9
131. Hasegawa D, Nishida O. Individualized recombinant human thrombomodulin (ART-123) administration in sepsis patients based on predicted phenotypes. Crit Care (2019) 23(1):231. doi: 10.1186/s13054-019-2521-7
Keywords: sepsis - diagnostics, immunomodulation, immunoparalysis, PD-1, PD-L, immune checkpoints inhibitors, artificial intelligence, machine learning
Citation: Nakamori Y, Park EJ and Shimaoka M (2021) Immune Deregulation in Sepsis and Septic Shock: Reversing Immune Paralysis by Targeting PD-1/PD-L1 Pathway. Front. Immunol. 11:624279. doi: 10.3389/fimmu.2020.624279
Received: 31 October 2020; Accepted: 29 December 2020;
Published: 17 February 2021.
Edited by:
Andreas Von Knethen, Goethe University Frankfurt, GermanyReviewed by:
Domenico V. Delfino, University of Perugia, ItalyRoslyn Kemp, University of Otago, New Zealand
Copyright © 2021 Nakamori, Park and Shimaoka. This is an open-access article distributed under the terms of the Creative Commons Attribution License (CC BY). The use, distribution or reproduction in other forums is permitted, provided the original author(s) and the copyright owner(s) are credited and that the original publication in this journal is cited, in accordance with accepted academic practice. No use, distribution or reproduction is permitted which does not comply with these terms.
*Correspondence: Yuki Nakamori, eW5ha2Ftb3JpQGNsaW4ubWVkaWMubWllLXUuYWMuanA=; Motomu Shimaoka, c2hpbWFva2FAZG9jLm1lZGljLm1pZS11LmFjLmpw