- 1Department of Clinical Tropical Medicine, Faculty of Tropical Medicine, Mahidol University, Bangkok, Thailand
- 2Tropical Immunology and Translational Research Unit (TITRU), Department of Clinical Tropical Medicine, Faculty of Tropical Medicine, Mahidol University, Bangkok, Thailand
- 3Thammasat Multi-Organ Transplant Center, Thammasat University Hospital, Faculty of Medicine, Thammasat University, Pathumthani, Thailand
- 4Division of Nephrology, Department of Medicine, Faculty of Medicine, Thammasat University, Pathumthani, Thailand
- 5Center of Excellence on Translational Research in Inflammation and Immunology (CETRII), Department of Microbiology, Chulalongkorn University, Bangkok, Thailand
- 6Department of Microbiology, Faculty of Medicine, Chulalongkorn University, Bangkok, Thailand
Although current regimens of immunosuppressive drugs are effective in renal transplant recipients, long-term renal allograft outcomes remain suboptimal. For many years, the diagnosis of renal allograft rejection and of several causes of renal allograft dysfunction, such as chronic subclinical inflammation and infection, was mostly based on renal allograft biopsy, which is not only invasive but also possibly performed too late for proper management. In addition, certain allograft dysfunctions are difficult to differentiate from renal histology due to their similar pathogenesis and immune responses. As such, non-invasive assays and biomarkers may be more beneficial than conventional renal biopsy for enhancing graft survival and optimizing immunosuppressive drug regimens during long-term care. This paper discusses recent biomarker candidates, including donor-derived cell-free DNA, transcriptomics, microRNAs, exosomes (or other extracellular vesicles), urine chemokines, and nucleosomes, that show high potential for clinical use in determining the prognosis of long-term outcomes of kidney transplantation, along with their limitations.
1 Introduction
A kidney transplant is typically the best option for patients with end-stage renal disease (ESRD). Kidney transplant (KT) recipients have a life expectancy that is more than double that of people on dialysis, and they also have a significant improvement in their quality of life (1). Furthermore, kidney transplantation is the most cost-effective long-term therapy for people with ESRD. Treatment developments have led to a steady decline in long-term allograft failure over the past 15 years: the kidney allograft failure rates five years post-transplantation in recipients receiving kidneys from deceased donors (DD) and live donors (LD) dropped to 14% and 9%, respectively in the periods from 1996 to 2012. The long-term survival of DD recipients has increased from 8.2 years (between 1995 and 1999) to 11.7 years (between 2014 and 2017) (2). Data from the National Kidney Transplantation Registry of Thailand in 2019 revealed the renal allograft survival rates at one, five, and ten years for DD recipients were 95.9%, 78.5%, and 58.5%, respectively. Meanwhile LD recipients showed a better renal allograft outcome than that of DD recipients (renal allograft survival rates were 98.2%, 92.6%, and 77.8%, respectively) (3). Of note, the leading causes of early graft failure within five years were rejection (56%) and interstitial fibrosis and tubular atrophy (IF/TA) (22%) followed by vascular or urologic complications (11%). IF/TA were the leading causes of late allograft failure (46.3%), followed by rejection (33%) and recurrent glomerular diseases (9%) (3). Thus, the major etiology of returning to dialysis in KT recipients is still dialysis reinstitution due to the failure of the renal allograft (3, 4). Despite advances in immunosuppressants and the management of acute kidney allograft rejection, a better understanding of several aspects of kidney transplantation is still needed, especially to improve long-term renal allograft survival. As such, donor characteristics and recipient variables (age, gender, dialysis vintage, and comorbidity), immunosuppressive drug monitoring, and immunological aspects such as human leucocyte antigen (HLA) mismatch, delayed graft function (DGF), cold ischemia period, and acute rejection during the first year of transplantation, have all been linked to long-term graft survival (5–8). Currently, several noninvasive biomarkers, including molecules, proteins, and immune responses, in combination or as single factors, have been developed to identify the risk of allograft rejection (9–12).
In response to the growing use of minimally invasive biomarkers in clinical transplantation, the Banff Minimally Invasive Biomarkers Working Group was established in early 2021 to examine the application of biomarkers in the diagnosis and categorization of renal allograft rejection. In the Banff 2005 and 2017 classification, donor-specific antibody (DSA) was introduced as a criterion for antibody-mediated rejection (AMR) (13, 14), and the classification of AMR and T cell-mediated rejection (TCMR) was greatly modified in the Banff 2019 classification (15). Currently, non-DSA biomarkers are mentioned in the Banff classification as screening tests to: i) rule out rejection, ii) expedite a confirmatory renal biopsy, or iii) directly diagnose rejection, either alone or in conjunction with histology (15, 16). Hence, the ideal biomarkers for diagnosis of allograft rejection should be able to distinguish rejection from non-rejection, be specific to rejection, replace biopsies or add information to the biopsy, and lastly, demonstrate prognostic value. The biomarker should also be able to discriminate between AMR and TCMR, which are induced through different immunopathogenic mechanisms. Several biomarkers include donor-derived cell-free deoxyribonucleic acid (dd-cfDNA), transcriptomic patterns, micro ribonucleic acids (microRNAs), exosomes, extracellular vesicles, chemokines, and nucleosomes are mentioned.
Our aim in writing this review was to summarize the most current research regarding novel biomarkers in the kidney transplantation field in terms of allograft rejection and their relevance to outcomes. Currently, novel biomarker use can be classified into two categories as immunological biomarkers and non-immunological biomarkers. The immunological biomarkers identify immune dysfunctions ranging from subclinical to overt rejection, whereas the non-immunological biomarkers indicate adverse transplant outcomes, such as delayed graft function, cardiovascular events, infection, and cancer, in which immune dysfunction is not the primary abnormality. Accordingly, although the non-immunological testing is necessary for long-term renal allograft outcomes, these biomarkers are outside the scope of this review.
2 Pathophysiology of renal allograft rejection
2.1 T cell-mediated rejection
Both innate and adaptive immune response components contribute to T cell-mediated graft injury. As such, the damage-associated molecular patterns (DAMPs) that are released in response to the ischemia during the graft preparation are recognized by pattern recognition receptors (PRRs) of phagocytic cells of the innate immunity leading to the upregulation of costimulatory molecules and secretion of pro-inflammatory cytokines (17). Mismatched HLA epitopes on the graft are recognized subsequently by host T cell receptors via direct, indirect, and semi-direct pathways (Figure 1) and act in concert with innate immunity-derived stimuli to activate and expand recipient T cell clones with inflammatory or regulatory functions (17). The production and release of soluble mediators, including interleukin (IL)-15, IL-17, granzyme B, perforin, Fas ligand which is also known as tumor necrosis factor (TNF) ligand superfamily member 6, interferon (IFN)-γ, TNF, CXC-chemokine ligand (CXCL) 10, CC-chemokine ligand (CCL) 2, CCL3, CCL4, CCL5, and CX3CL1, potentiates the inflammatory injury that is the characteristics of acute allograft rejection (17). Then, the activated mononuclear cells accumulate in the renal interstitium, tubules, and, rarely, in the arteries of the graft (leading to endarteritis), whereas glomerulitis may occur in more severe cases of rejection and is accompanied by apoptosis of vascular endothelial cells and mesangiolysis.
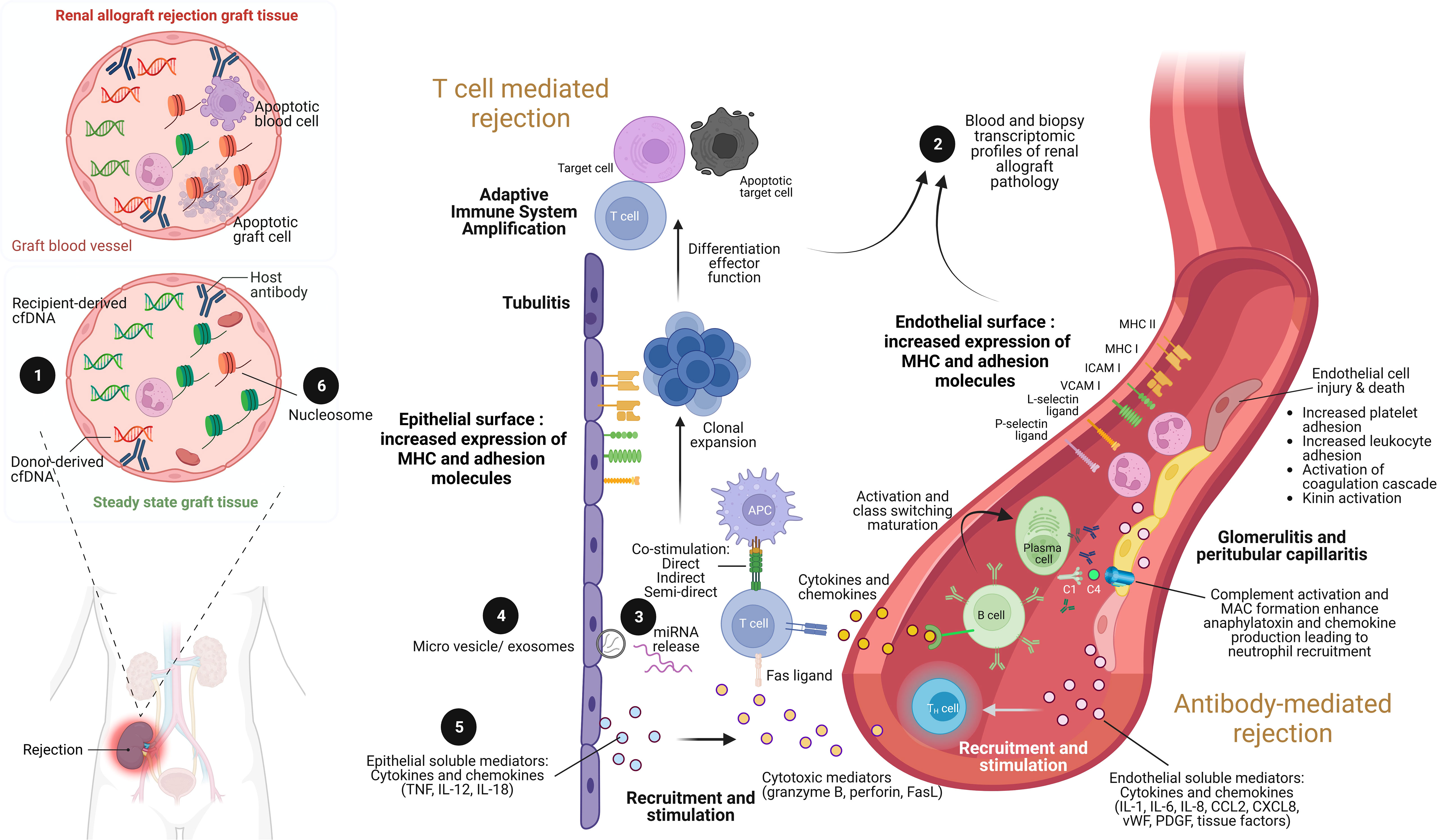
Figure 1 The illustration of renal allograft rejection and the application of biomolecular biomarkers from immunological pathogenesis. While the main pathogenesis of acute cellular mediated rejection (TCMR) is epithelial cell injury enhancement leading to adaptive immune system amplification, acute antibody-mediated rejection (AMR) is endothelial cell injury through antibody and complement enhancement. As a result, the culprit pathologic characteristic of TCMR is tubulitis compared to glomerulitis and peritubular capillaritis in AMR. Both patterns can be concurrently found in severe combined TCMR and AMR case. During renal allograft rejection process, both innate and adaptive immune system are activated from the imbalance differentiation between donor and recipient cell-free deoxyribonucleic acid (cfDNA) molecules ❶ (left panel). The final products of both T cell and B cell activation can be detected their signals and cellular origin of either peripheral blood or renal allograft tissue by transcriptomic profiles ❷. Indeed, plenty of mediators are produced during overwhelming inflammatory process from both TCMR and AMR, including the production of miRNAs ❸ within extracellular vesicles and exosomes ❹, and soluble mediators (cytokines and chemokines) ❺. Interestingly, the epigenetic control of gene expression by circulating cell-free nucleosome may play as a crucial step of renal allograft rejection activation ❻. Imbalance between donor- and recipient-derived nucleosomes with histone alteration is currently postulated as one of pathogenesis in renal allograft rejection. ICAM, intracellular adhesion molecule; IL, interleukin; MHC, major histocompatibility complex; VCAM, vascular cell adhesion molecule; TNF, tumor necrosis factor. Picture is created by BioRender.com.
Currently, the Banff classification (13) stratifies TCMR-induced graft injury into three classifications based on the presence of i) interstitial inflammation in the non-scarred area of the cortex, ii) tubulitis in cortical tubules within the non-scarred cortex, and iii) endarteritis (intimal and transmural arteritis with fibrinoid necrotic change) as well as medial smooth muscle necrosis with lymphocytic infiltration in the vessel (18). Despite the fact that TCMR normally responds rapidly to immunosuppressive drugs, persistent inflammation in the areas of IF/TA is frequently associated with sustained expression of gene transcripts characteristic of acute kidney injury and predicts progression to chronic-active TCMR (19, 20).
2.2 Antibody-mediated rejection
Antibody-mediated rejection (AMR) is the most severe and destructive form of immune-mediated graft injury which is observed in approximately 30% of all patients with rejection (21). As such, AMR may occur with or without TCMR and can be detected early or late in the transplantation process, ranging from acute AMR with rapid and severe graft injury to chronic AMR with progressive graft destruction (21). Recipient CD4+ T cells, which are activated by epitopes expressed on graft antigens, assist in the activation of graft-specific B cells, which is followed by class switching and affinity maturation; T cell assistance is mediated by costimulatory factors and receptors, including inducible T cell costimulator, CD40 ligand, CD80, and CD86 (22). These activated B cells generate plasmablasts and plasma cells that produce DSAs (Figure 1). It has been reported that 15% of KT recipients developed de novo DSAs over 4 years after transplantation, and graft survival at 10 years was diminished by 40% compared to patients without de novo DSAs due to chronically active AMR (23). Solid-phase assays can be used to detect DSAs and enable precise determination of alloreactivity, which is frequently directed against HLA class II epitopes but has also been observed against non-HLA targets such as type 1 angiotensin II receptor, perlecan, and collagen (24). The antibody against HLA is frequently initially circumscribed to mismatched epitopes expressed on the graft; however, repeated stimulation may enhance sensitization and broaden the epitope repertoire via intramolecular and intermolecular antigen spreading (epitope spreading) (25).
As highlighted by the Banff criteria, DSA binding to target epitopes expressed on the vascular endothelium led to acute microvascular injury that can be characterized by endothelial cell enlargement, vacuolization, loss of fenestrations, detachment from the basement membrane, and apoptosis (26). Mobilization of endothelial vesicles externalizes P-selectin, facilitates the binding of several cells at the site of injury, including platelets, neutrophils, macrophages, natural killer cells, and T cells, contributes to intimal arteritis which is a major characteristic of AMR injury (26). The formation of the membrane attack complex (C5b–C9), which exacerbates injury to the endothelium and other graft tissues (27), is triggered by the binding of the complement C1 complex to activate the classic complement pathway (Figure 1). Immunoglobulin subclasses 1 and 4 of the DSA are associated with enhanced C1 binding capacity and the degree of complement activation and may therefore determine the severity of the injury. Hence, C4d is frequently deposited at the site of complement activation, whereas C3a and C5a function as anaphylatoxins enhancing infiltration in the kidney with innate immune cells (neutrophils and mononuclear inflammatory cells) that exacerbate the injury. Additionally, the complement-independent pathways may also be involved in AMR (21). As such, AMR is currently classified as active, smoldering, or chronic mechanisms and either the smoldering or chronic AMR is frequently resistant to treatment. Although none of the current therapeutic interventions has shown promising results in AMR, removal of circulating antibodies by plasmapheresis with the concurrent intravenous immunoglobulin administration to downregulate B cell activity is currently a standard of care (21) with inadequately supported evidence. Moreover, proteasome inhibitors, C1q or C5 inhibitors, anti-CD20 biologics, and cleaving endopeptidases have proven to be ineffective (28).
3 Immunological biomarkers
3.1 Donor-derived cell-free deoxyribonucleic acid
Donor-derived cell-free deoxyribonucleic acid (dd-cfDNA) has been proposed as a noninvasive marker for the early detection of rejection before clinical allograft dysfunction (an increase in serum creatinine). Cell-free DNA (cfDNA) is a DNA fragment released from cells with a fast turnover, making it a useful tool for real-time monitoring of allograft damage. In KT recipients, the total cf-DNA in blood can be derived from the cells of the host and donor (allograft), and the differentiation between the cf-DNA from the allograft (donor cells) or recipient cells (host cells) is essential for determining allograft dysfunction. Large quantities of donor cells are found in recipients with graft injury and/or rejection caused by cell death. Similar to the clearance of serum creatinine, the clearance of dd-cfDNA from an individual’s body is comparable to that of cell-free DNA; however, additional study is required. In the circulation, cell-free DNA has a half-life of 16 minutes to 2.5 hours (29, 30). The DNase I enzyme present inside the liver and spleen can cause the entry of cell-free DNA and breakdown by the macrophages there (31). Cell-free DNA can also be excreted via the urine.
The blood level of dd-cfDNA is reported as the percentage of dd-cfDNA to the total cf-DNA, and its usefulness has been explored in several publications. In uncomplicated KT, high blood dd-cfDNA levels are encountered, with a median value of approximately 20% immediately (within hours) after renal engraftment and rapidly decreases on the first postoperative day to approximately 5% and then subsequently to below 1% (32). The level of dd-cfDNA depends on cell lysis (cell damage) (33) from any causes, including inflammation, infection, drug toxicity (calcineurin inhibitors), and disease recurrence. Due to its rapid change, dd-cfDNA can be used to obtain an immediate diagnosis of posttransplant rejection; however, the reported efficacy has varied among different studies (34–37). Sigdel et al. (35) demonstrate a new dd-cfDNA approach that employs a next generation sequencing (NGS) assay with single nucleotide polymorphisms (SNP)-based massively multiplex polymerase chain reaction (mmPCR) in a single-center retrospective analysis. The researchers examine 300 plasma samples acquired from 193 KT patients, including those with routine biopsies. The 217 biopsy-matched plasma samples from 193 KT patients, including 38 active rejection, 72 borderline TCMR rejection, 82 stable allografts, and 25 patients with other damages. Then, mmPCR is used to target 13,392 SNPs in dd-cfDNA. The test is able to distinguish acute allograft rejection (both AMR and TCMR) from non-rejection with an area under the curve (AUC) for the receiver-operator characteristic (ROC) curve (AUROC) curve of 0.87 with 88.7% sensitivity, 72.6% specificity, negative predictive value (NPV) 95.1%, and positive predictive value (PPV) of 51.9% and a stated cutoff of 1%. Unlike other dd-cfDNA technologies, the test is able to differentiate among TCMR, AMR and non-rejection causes (toxic damage or viral infection). Technical advancements enable a highly sophisticated mmPCR method allowing the use of over 13,000 SNP markers (35).
According to a meta-analysis, the sensitivity for AMR diagnosis is high at a fractional threshold of 1%, but less sensitive for TCMR, which generally needs a concentration higher than a 1% threshold, especially if the rejection is more severe than Banff 1B (38). With a cutoff of 0.69-1% for a positive test, most studies with commercially available dd-cfDNA assays demonstrate an AUC at 0.71-0.85, with a sensitivity and specificity of 45-89% and 69-85%, respectively, and a positive and negative predictive value of 52-77% and 66-95%, respectively, when compared with renal pathology, depending on the pretest probability of rejection (39).
Notably, most of the current studies on dd-cfDNA are ad hoc tests on patients who probably have a high pre-test risk of rejection. Categorization of blood dd-cfDNA into high (>1%) (35 cases), moderate (0.5-1%) (43 cases), and low (0.5%) (239 cases) among patients at 1-48 months post-transplantation revealed allograft rejection (biopsy within 2 months of dd-cfDNA measurement) in 24 of 62 cases (20%) among patients with moderate or high dd-cfDNA levels (40). The rejection was mostly demonstrated in patients with high (6 in 25 cases; 17%) and moderate dd-cfDNA (5 in 43 cases; 12%) when compared with the low level (13 in 239 cases; 5%) with no difference in the 1.6-year short-term graft outcomes using estimated glomerular filtration rate (eGFR) and de novo donor-specific antibodies (DSAs) (40). Most patients with high dd-cfDNA without allograft rejection remain stable without eGFR decline or graft loss (40). By contrast, a recent large multicenter study with approximately 1,100 kidney transplant patients indicated that patients with dd-cfDNA >0.5% had a greater risk of eGFR decline over 3 years and increased de novo DSA after follow-up (41).
A strong correlation is evident between high dd-cfDNA (>1%) and subclinical AMR using the Molecular Microscope Diagnostic System (MMDx; molecular tissue gene expression), but not by histopathology, among sensitized recipients (high risk of rejection), as indicated by DSAs, flow crossmatch at transplant, or documented non-adherence medication (42). These findings are also supported by the multicenter Trifecta trial (43). Likewise, Huang and colleagues (44) demonstrated that dd-cfDNA discriminated KT recipients with AMR (median 1.35%, interquartile range (IQR) 1.10% to 1.90%) from those without AMR (median 0.38%, IQR 0.26% to 1.10%), p <0.001. Interestingly, dd-cfDNA could not discriminate KT recipients with TCMR from those without rejection (44). A study by Whitlam et al. (45) provides further support, as 61 KT recipients with AMR showed receiver-operator characteristic AUC for graft-derived cfDNA concentration and graft fraction that were predictive of AMR (AUC = 0.91 (95% confidence interval (CI) 0.82 to 0.98) and 0.89 (95% CI 0.79 to 0.98). Again, both measures failed to diagnose borderline or type 1A TCMR (45).
High-normal dd-cfDNA (> 0.5%) can also identify individuals with borderline TCMR 1A histology who are likely to experience deteriorating kidney function (37). Indeed, the majority of patients with high dd-cfDNA and retained allograft function remained stable throughout the study without deterioration of function or graft loss (40). These publications support the preliminary use of dd-cfDNA as a screening test for renal biopsy and for categorizing rejection grading. Nevertheless, KT recipients with high DSA levels, BK poliomavirus (BKV) nephropathy, urinary traction infections, acute tubular necrosis, and post-renal allograft biopsy may also show increases in their dd-cfDNA levels (34, 46). Notably, absolute dd-cfDNA quantification in copies/mL might be more effective than the dd-cfDNA level as the percentage of total cf-DNA for discriminating allograft rejection (36). More studies would be interesting.
In summary, dd-cfDNA is a robust biomarker for the diagnosis of renal allograft rejection. Although dd-cfDNA alone cannot replace renal biopsy, it does provide a noninvasive way of identifying the potential causes of allograft failure in certain recipients, thereby enhancing the ability to predict long-term renal allograft outcomes. Increases in several regular biomarkers, including creatinine, proteinuria, and/or newly increased DSAs, are now indications for further dd-cfDNA tests (47). A routine cross-sectional dd-cfDNA testing of patients with a low pretest chance of rejection might be beneficial, and high dd-cfDNA levels are more common in DSA-positive recipients, highlighting the usefulness of dd-cfDNA in monitoring highly sensitized individuals (48). With the introduction of Allosure® and other comparable tests, dd-cfDNA is already being used as a supporting tool for diagnosis and therapy in clinical practice. The effects of repeated dd-cfDNA surveillance in kidney transplant recipients are currently being assessed in two prospective studies (The Ongoing Kidney Allograft Outcomes Registry (KOAR; NCT03984747), and The Prospera Kidney Transplant ACTIVE Rejection Assessment Registry (PROACTIVE; NCT03984747)
3.2 Transcriptomics
Several difficulties arise when attempting renal allograft rejection classification from kidney histology, including a lack of tissue, poor repeatability, and a dearth of well-trained pathologists. For this reason, transcriptome analysis has been the most highly feasible candidate technique for overcoming these limitations, as indicated by the use of C4d (49) and AMR-specific molecular panels (50, 51) for AMR diagnosis. Currently, the Molecular Microscope Diagnostic System (MMDx) is the gold standard for transcriptome analysis of kidney transplantation for AMR and TCMR (52, 53) with the identified key cellular pathways that contribute to rejection. However, many challenges remain in translating molecular diagnostics into clinical practice, including a large number of redundant gene sets that raise a need for standardization of various molecular diagnostic panels on gene analysis (e.g., microarrays and quantitative real-time polymerase chain reaction [qRT-PCR]), as well as an ongoing debate on rejection gene sets between AMR and TCMR (13).
Unlike the microarray gene-based MMDx platform, the NanoString nCounter platform needs only 100 ng of mRNA from formalin-fixed paraffin-embedded (FFPE) biopsies, without a requirement for a biopsy core, to detect mRNA target molecules within two days, allowing large-scale transcriptomic results from biopsy samples (54). The Banff Molecular Diagnostics Working Group developed molecular consensus gene sets for TCMR and AMR in 2015 (55) and proposed several molecular panels in 2017 (13). It subsequently launched the commercially available Banff-Human Organ Transplant (B-HOT) panel for transplantation in several organs (kidney, lung, heart, and liver) in 2019 without centralized molecular profiling (56). The incorporation of molecular pathology into clinical practice may use NanoString technology with the B-HOT panel for better diagnosis, categorization, and normalization, as demonstrated by the different gene expressions observed between no rejection versus AMR and TCMR (57).
Using the most predictive genes from the B-HOT and Element analysis, regression models based on the two least absolute shrinkage and selection operators are being developed to classify biopsies as AMR versus no AMR (57). These classifications include borderline rejection, TCMR, or no rejection, with a receiver-operating characteristic area under the curves (AUC) of 0.994 and 0.894, sensitivity of 0.821 and 0.480, and specificity of 1.00 and 0.979 during cross-validation compared with the gold standard renal biopsy (57). In addition, principal component analysis (PCA) of the microarray gene sets can identify the main categories of renal diagnosis and a comparable relationship between pathological diagnosis and molecular sets (58). As a result, non-chronic antibody-mediated rejection with high expression of endothelial genes can be detected by PC clustering with cell type analysis that is also able to reveal differences in genes from B-cells and plasma cells (58).
In addition, there are several tests that measure immunological activity by looking at the gene expression of circulating immune cells. A widely integrated gene expression profile (GEP) assay is AlloMap, which has been made available as a monitoring tool for heart transplant recipients since 2005 (59) with a high negative predictive value (NPV). However, immune system gene expression profiling in KT has been difficult to use as a consistently accurate and repeatable indicator of renal allograft rejection because the data remains controversial (9, 60–62). A most recent study from Akalin et al. (63) demonstrates the validation of a blood GEP developed to differentiate immune quiescence from both TCMR and AMR. On the basis of 56 peripheral blood samples, a five-gene classifier (DCAF12, MARCH8, FLT3, IL1R2, and PDCD1) is created and validated on two separate sample sets outside of the training cohort. The main validation set includes 18 rejection examples—7 TCMR, 10 AMR, and one mixed rejection—and 98 quiescence samples. The second validation set has 11 rejection samples—7 TCMR, 2 AMR, and 2 mixed rejection—and eight quiescence samples. Interestingly, quiescence and rejection are distinguished significantly by AlloMap Kidney classifier scores in the primary validation set (median, 9.49; IQR, 7.68-11.53 and 11.25-15.28, respectively). The medians in the second validation set are similar to those in the first validation set, although the cohorts are significantly different (p =0.03). The primary validation’s AUC for separating rejection from quiescence is 0.786, and the secondary validation’s AUC is 0.800 (63). Thus, blood GEP and dd-cfDNA contribute independent signals and inform on different aspects of allograft rejection.
On the other hand, the Kidney Solid Organ Response Test (kSORT) is a microarray-based assay designed to identify recipients at high risk for acute rejection (64) using quantitative polymerase chain reaction (PCR) to measure the relative mRNA expression levels of 17 genes that are associated with acute renal allograft rejection or leukocyte trafficking in peripheral blood. An algorithm based on correlation is then used to generate risk scores and classify patients as having a high, medium, or uncertain risk of acute rejection. The kSORT assay is initially evaluated in a large multicenter study of 436 adult and pediatric kidney transplant recipients (Assessment of Acute Rejection in Renal Transplantation [AART]) with paired peripheral blood samples and kidney allograft biopsies (performed for allograft dysfunction or as part of a clinical protocol) using a case-control study design of selected recipients (64). With a sensitivity and specificity of 92% and 93%, respectively, the kSORT assay is able to identify patients at high risk of either TCMR or AMR. In addition, kSORT is able to identify subclinical rejection in 75% of biopsies and clinical rejection in over 60% of samples collected within three months prior to the diagnosis of biopsy-confirmed acute renal allograft rejection. Nonetheless, the test fails to differentiate between acute TCMR and AMR.
Moreover, the TruGraf® v1 assay is a DNA microarray-based gene expression blood test that is developed as an alternative to surveillance biopsies to rule out subclinical rejection in recipients with sustained graft function (65). Blood samples coupled with protocol biopsies from prevalent cohorts are utilized for the entirety of the discovery and external validation of the TruGraf® test. However, the performance of the test in recipients with renal allograft dysfunction has not been evaluated and must be studied further. Interestingly, combining the TruGraf® assay with dd-cfDNA enhances the detection of subclinical renal allograft rejection (66). Of note, by using multivariable logistic regression, the AUC is 0.81, which is substantially greater than the gene expression profile (p <0.001) or dd-cfDNA alone (p =0.006). Notably, when cases are divided according to rejection type, the gene expression profile is significantly better at detecting TCMR (AUC 0.80 versus 0.62; p =0.001), whereas the dd-cfDNA is significantly better at detecting AMR (AUC 0.84 versus 0.71; p =0.003) (66).
To sum up, at present, transcriptomic analysis is revealing the possible molecular mechanisms that might improve outcomes and be useful as precision diagnostic indicators in renal transplantation.
3.3 MicroRNAs
MicroRNAs (miRs) are a class of short, noncoding RNAs that can regulate gene expression (57). They can be detected by several different methods, including qRT-PCR, microarray, and next-generation sequencing analysis (global miR profiling) (67) in the blood (cells), serum/plasma, and urine (68, 69). Ischemic reperfusion injury during KT increases urine miR-146a content to higher levels in renal transplant recipients implanted from deceased donors than from living donors (70). Acute TCMR increases miR-223 and miR-142–3p in allografts and in peripheral blood mononuclear cells (PBMCs) of recipients (71). Patients with TCMR demonstrate higher miR-223, miR-10a (72), miR-99a, and miR-100 levels in blood samples (73), but lower levels of miR-99a expression in kidney allografts (74, 75), implying a possible difference in miR levels between renal tissue and blood samples. Interestingly, multivariable logistic regression analysis of a panel of blood miRs (miR-15b, miR-16, miR103a, miR106a, and miR-107) was able to differentiate acute vascular rejection (Banff II–III) from stable graft function (76). In acute TCMR, urinary miR-10a is upregulated, while miR-10b and miR-210 are downregulated. The urinary level of miR-210 (a cellular aging biomarker) is correlated with the severity of biopsy-proven rejection, but with low specificity and sensitivity, unfortunately (69). Increased levels of miR-142–5p are reported in the PBMCs of recipients with chronic, but not acute, AMR (77) and with acute TCMR (71, 74). Interestingly, alteration of miR levels between pre- and post-renal allograft rejection has been reported by Millán and colleague study group (78). As such, urinary levels of miR-142-3p and miR-155-5p significantly increase, while miR-210-3p decrease in allograft rejection. The miR-155-5p at the threshold values of 0.51 demonstrates sensitivity and specificity at 85% and 86%, respectively, and the analyses of receiver operating characteristic (AUC) effectively differentiate the recipients with versus without allograft rejection (AUC = 0.875; p =0.046) (78). Also, there is a good correlation between miR-155-5p and glomerular filtration rate or renal allograft restoration (78).
Additionally, the content of miR-211, miR-204, and miR-142–3p in the urine exosomes of patients with biopsy-proven IF/TA show a correlation between miRs in urine and renal tissue (79). Downregulation of miR-200b, miR-375, and miR-193b and upregulation of miR-423–5p and miR-345 are also detected in the urine of patients with IF/TA (one-year follow-up) without the association between miR-200b expression and proteinuria (68). Downregulation of miR-200b (80) and downregulation of miR-21 are observed in plasma from patients with IF/TA (81).
In summary, many miRs have been proposed as biomarkers for renal allograft dysfunction due to miR stability; however, assessment using receiver-operator characteristic areas under the curves (sensitivity and specificity) is limited. Nevertheless, a five-miR panel is able to distinguish T cell–mediated vascular rejection from stable graft function following kidney transplantation (76), implying possible benefits of combined miR (panels). MiRs from allograft biopsy tissue provide greater accuracy for rejection diagnosis, suggesting that tissue-derived miRs may have the potential to substitute for histology. More studies are warranted.
3.4 Extracellular vesicles (EVs) and exosomes
Extracellular vesicles (EVs) are bilayer lipid membranes released by all cells in the body and can include exosomes, microvesicles (MVs), ectosomes, oncosomes, and apoptotic bodies. In general, the term “EV” seems to be a generic label for a “secreted vesicle” (82). The EVs in body fluids operate as carriers in signal transmission between cells for the regulation of immunological responses, inflammation, and other cell activities (83, 84). Because all cells can generate EVs, the EVs in urine should be correlated with the cells with direct urine contact (e.g., the urinary epithelium, endothelium, and immune cells). By contrast, the source of cells that produce EVs in blood could be more difficult to determine. The determination of EVs from urine requires strict normalization, and normalization by the duration of urine collection (time normalization), especially 24-hour urine, seems to be mostly appropriate; however, unfortunately, the correlation observed between EVs in urine and other normalization biomarkers (creatinine, total proteins, number of EVs) remains inconclusive (85). The duration of urine in the bladder before urine collection might also alter the EVs in the urine sample, because bladder cells can also produce EVs, and those EVs could be altered by urine characteristics (pH, concentration, and excreted substances) (85). Nevertheless, EVs from both blood and urine are being extensively studied for biomarkers.
Among all the EV types, exosomes were observed for the first time in a multivesicular endocytic compartment in 1983 by Harding et al. (86). Since then, these EVs have undergone the most extensive exploration. Exosomes are 40-100 nm in diameter (82) and are formed as lipid bilayers that can protect several molecules inside. For example, several RNA types, including miRs, long noncoding RNAs (lncRNAs), small nuclear RNAs (snRNAs), and circular RNAs (circRNAs), are found in EVs and can be used as biomarkers (87). Current omics technology, including transcriptomics, proteomics, and metabolomics, is now used for the genetic association analysis of expression quantitative trait loci (eQTL), protein quantitative trait loci (pQTL), and methylation quantitative trait loci (mQTL) (88). This has made possible the expanded use of exosomes and EVs for locating potential sites in allografts that produce EVs (89). Despite the large number of EVs in the plasma (roughly 102–1.013 vesicles per mL) (90), the tiny size, limited contents, and possible difference in contents inside each particle (referred to as “liquid biopsy”) are limitations for the use of EVs as biomarkers. However, next-generation sequencing (NGS) and mass spectrometry can now amplify and detect the molecules within the vesicles or the intra-vesicular contents of EVs and have revealed several interesting aspects of EVs.
One example is the profile of urinary EVs from living-donor renal transplantation, which demonstrates that the EVs are derived from the nephron (glomeruli and other parts; descending limb of Henle’s loop, the collecting tubules, etc.), epithelium, and endothelium (91). This categorization is established by the detection of several molecules, such as megalin, aquaporin (AQP), podocalyxin (PODXL), ion cotransporters, synaptotagmin 17 (SYT17), CD3, and CD133, which are expressed only at specific sites and might therefore be useful as biomarkers (92–94). Increases in these molecules in EVs from urine or blood mostly indicate that some damage has occurred to renal allografts.
Interestingly, the EV molecules related to epithelial cell differentiation seem to be upregulated in TCMR, while proteins of acute inflammation or antigen presentation are more related to AMR (95). Likewise, the levels of the sodium-chloride cotransporter (NCC) and Na-K-Cl cotransporter (NKCC2), the transporters commonly found in renal tubular cells, are higher in the EVs (exosomes) from patients treated with calcineurin inhibitors (CNIs; drugs with tubular toxicity) than with non-CNI regimens (96, 97). Similarly, miRNA-enriched EVs are reported in patients who experience long ischemic times during transplantation (98), implying that EVs might be directly related to ischemic mechanisms through the delivery of miRs and other molecules from one cell to others (99, 100).
In 2017, a landmark study by Park and colleagues reports the use of EVs in renal allograft rejection as T cell-derived EVs in urine might indicate renal tubular T cells infiltration during TCMR (101). Thus, an EVs-based diagnostic platform recognizing T cell-derived urinary EVs (uEVs), refer to as iKEA (integrated kidney exosome assay), is mentioned as TCMR biomarker. As such, CD3 is used to identify T cell-derived uEVs and the CD3-based iKEA demonstrates diagnostic accuracy of 91.1% in a discovery group of 30 recipients and 83.7% in a validation cohort of 14 recipients in subsequent clinical trials (101). Accordingly, iKEA might be a powerful noninvasive serial monitoring in kidney transplant recipients for better long-term renal allograft function. A subsequent well-design, large cohort study from El Fekih et al. established the rejection signatures using approximately 200 samples of the matched urinary exosomal mRNAs with the tissue of renal allograft biopsy for a powerful noninvasive liquid biopsy to identify renal allograft rejection (102). For the diagnosis of all-cause renal allograft rejection, the AUC of renal biopsy is 0.93 (95% CI, 0.87 to 0.98), while the AUCof eGFR is 0.57 (95% CI, 0.49 to 0.65). In parallel, the AUC of urinary exosome-based signature is 0.87 (95% CI 0.76 to 0.97) with positive and negative predictive values at 86.2% and 93.3%, respectively. Additionally, the exosome-based signature distinguishes recipients with TCMR from those with AMR with positive and negative predictive values at 77.8% and 90.6%, respectively (102). Despite a lower AUC than the gold standard renal allograft biopsy, the urine-based exosome measurement is noninvasive and can be frequently measured.
On the other hand, an elevation of EV numbers containing CD31 (glycosylated immunoglobulin-like membrane receptor of leucocytes, platelets, and endothelial cells) or CD81 (Tetraspanin; expressed in several cells except for erythrocytes, platelets, and neutrophils) is correlated with the length of cold ischemia, increased donor age, and reduced renal allograft blood flow (103). This suggests that the removal of EVs in KT recipients who experience long cold ischemic times before renal engraftment might be beneficial (104). The EVs may also transmit viruses through en bloc transmission of several viral genomes, which could modulate viral fitness and protect viruses within the lipid membrane (105). Viral particles in EVs might also dilute the physiologic contents and interfere with normal cell–cell communication (106). One virus, the BK polyomavirus (BKV), is an important cause of renal allograft failure (107). Its presence in exosomes could encode the host’s miRs and downregulate some host genes required for viral evasion processes (108), as elevated levels of miR-B1-5p and miR-B1-3p in urinary exosomes indicate possible BKV infection (109, 110).
Several challenges remain for the use of exosomes or EVs as biomarkers. These include methods for the purification and isolation of EVs (or exosomes) that preserve their integrity (111), the normalization, and the time-consuming procedure for 24-hour urine collection. Regarding the therapeutic aspects, EVs also represent possible vehicles for delivering therapeutic molecules to specific target cells (112), while the removal of EV-mediated ischemic responses might improve the long-term outcomes of KT (104). More clinical trials involving several candidates undergoing pre-clinical studies will be very interesting.
3.5 Urine and circulating chemokines
Inflammation is a response to cell damage, and detection of inflammation in renal allografts, especially with other biomarkers or clinical characteristics, possibly indicates allograft rejection. For example, urinary CXCL9 and CXCL10 are both increased in AMR and TCMR compared with patients with no rejection (113–115), elevated urinary CXCL10 predicts rejection (78), and treatment of allograft rejection reduces CXCL10 (78, 113, 116). However, combining CXCL9 with CXCL10 does not enhance the prediction ability compared with each molecule alone (114, 117). As an indicator of allograft rejection, urinary CXCL9 demonstrates sensitivity and specificity of 58-86% and 64-80%, respectively, while the values for CXCL10 are 59-84% and 76-90%, respectively (78, 113–115, 117). However, urinary CXCL10 seems to be associated with tubulointerstitial inflammation and peritubular capillaritis, rather than glomerulitis or isolated vascular inflammation (118) and urinary CXCL10, but not CXCL9, correlates with subclinical rejection (AUC 0.64; 95% CI, 0.55-0.73) (116). Both urinary CXCL9 and CXCL10 distinguish rejection from other non-rejection causes of graft dysfunction, with AUCs of 0.72 and 0.74, respectively (116). The urine CXCL10/creatinine ratio, together with the mean fluorescence intensity (MFI) of DSAs, predicts AMR and graft loss better than the DSA MFI alone, with a net reclassification increase of 73% (119). Nevertheless, urinary CXCL10 is not specific for rejection, although it is a good indicator of renal inflammation, as urinary CXCL10 is also elevated to similar levels in patients with BK viremia and in patients with tubulitis from rejection (113, 114). Interestingly, urinary CXCL10 is not increased in cytomegalovirus (CMV)-infected subjects (118), perhaps because of the greater genitourinary specificity of the BK virus compared with CMV. Urine CXCL9 and CXCL10 are also increased in patients with isolated leukocyturia and urinary tract infections (120) and leukocyturia with increased CXCL10 demonstrates more severe inflammation than leukocyturia alone (113). Notably, the levels of urinary CXCL9 and CXCL10 in both absolute terms and after adjustment to urine creatinine (urine creatinine normalization) are useful.
Urinary chemokines are enhanced before rejection becomes clinically apparent, implying that they are good candidates for screening tests (116, 121). Recipients with high urine CXCL10 levels have been divided into renal biopsy or regular surveillance in an ongoing multicenter trial (NCT 03206801). This trial could provide an opportunity to determine whether urinary chemokine levels, when considered alongside histologic variables, can improve the prediction of renal allograft outcomes. A test using urinary chemokines as KT biomarkers will be interesting. Recently, the Barcelona Consensus on Biomarker-Based Immunosuppressive Drugs Management in Solid Organ Transplantation has a preliminary proposal for using urinary chemokine CXCL9 and CXCL10 to guide and individualize immunosuppressive regimens, predict acute and chronic TCMR and AMR, and may be a useful tool for risk stratifying recipients. However, the standard immunoassay platforms are needed (122).
Circulating or plasmatic chemokines, CXCL10 is also a promising biomarker for renal allograft rejection determination. Due to the prevalence of clinical confounding factors, the utility of serum CXCL10 as a potential biomarker for assessing the risk of rejection remains controversial (123, 124). High serum CXCL10 during the pre-transplantation period is associated with long-term graft loss after kidney transplantation (123). As such, Xu et al. (125) demonstrate that serum CXCL10 measured on the fourth and seventh days after kidney transplantation are substantially higher in recipients with acute renal allograft rejection than those without rejection. The most recent study conducted in 28 recipients experienced rejection (14 TCMR cases and 14 recipients with AMR), 8 cases of subclinical rejection, 13 BKV infection, and 16 cases of CMV. Accordingly, in comparison with non-rejection, pre-transplantation circulating CXCL10 is significantly higher in TCMR and AMR. In post-transplantation, increased circulating CXCL10 is demonstrated in TCMR, AMR, and subclinical rejection. All CMV infected recipients show elevated circulating CXCL10 above the rejection threshold, whereas 80% of BKV infected recipients have CXCL10 concentration approximately at 100 pg/mL (126). Indeed, circulating CXCL10 can be used for pre-transplanted stratification and the selection of immunosuppressive regimens following the risk of rejection according to CXCL10 levels. However, BKV and CMV infection must be firstly excluded when using CXCL10 as a rejection biomarker (126).
On the other hand, urinary concentrations of neutrophil gelatinase-associated lipocalin (NGAL), during the early post-transplantation period, have been extensively examined as a predictor of delayed graft function in kidney transplantation (127, 128). Likewise, urine NGAL is demonstrated as a predictor of acute kidney injury in the later period after transplantation (129, 130) and an indicator of allograft loss after acute kidney injury (131). However, the diagnostic utility of NGAL in kidney transplant patients after the first year of transplantation with chronic processes of injury (a steadily deteriorated renal function) is demonstrated by only a few studies (132, 133). Additionally, the difference in urine NGAL assays in various studies makes it difficult for comparison and to propose the cut-off values using data from different studies. A recent study by Kielar et al. (134) demonstrates 2 folds higher urinary NGAL after 1-year post-transplantation in recipients with at least a 10% reduction in eGFR compared to those with stable or improved function of the transplanted kidney. Independent of baseline eGFR and albuminuria, baseline NGAL strongly predicts the relative and absolute changes in eGFR as well as the mean eGFR during the follow-up. Furthermore, high urine NGAL levels in clinically stable kidney transplant recipients after the first year may be interpreted as a warning sign, prompting a search for transitory or chronic causes of graft failure or urinary tract infection (134).
While urinary NGAL might be associated with delayed graft function (127), the relationship between urinary kidney injury molecular-1 (uKIM-1) and renal allograft is not clear (135). As such, recipients with lower KIM-1 in the first week post-transplantation take a longer time to stabilize their renal function compared to the cases with normal uKIM-1. In addition, a prospective cohort study by Zhu et al. (136), in 160 recipients scheduled for kidney transplantation, is conducted to evaluate the predictive power of uKIM-1 for renal allograft prognosis. They discover that recipients with higher uKIM-1 levels on the first day after transplantation had a 23.5% higher risk of developing functioning delayed graft function and a 27.3% higher chance of having a longer renal allograft survival. Hence, it is possible that KIM-1 has a potential role in post-transplant renoprotection (137, 138).
3.6 Nucleosomes
The smallest structural component of chromatin is called a nucleosome and usually consists of 8 histone proteins and 146 DNA base pairs (139). The histone-encased DNA plays a crucial role in the epigenetic control of gene expression by modifying the “tail” regions of histones by methylation, acetylation, ubiquitination, and phosphorylation (140). After cell death, nucleosomes are released into the blood, modified by some enzymes, and are then referred to as “circulating cell-free nucleosomes” (CCFN) (141). The epigenetic signature of histones (histone alterations) in CCFNs might be able to differentiate between regular versus pathological cell deaths, as mentioned in cancer studies (142). For example, the addition of DNA modification (5-methylcytosine) and histone modifications (H2AZ, H2A1.1, and H3K4Me2) increased the diagnostic values of carbohydrate antigen (CA) 19-9, a conventional cancer biomarker, in pancreatic malignancy (143). Likewise, increases in nucleosomes with histone alterations are observed in acute renal allograft rejection (144). Indeed, the levels of CCFNs containing citrullinated histone H3 (Cit-H3), a biomarker of neutrophil extracellular traps (NETs) (145–147) important in AMR (118), are increased within several hours after AMR and can be detected using a modest quantity of sample (10 μL) (143). However, serial readings of histone-modified CCFN might be necessary, as the levels may fluctuate in the setting of acute renal allograft rejection. Notably, total nucleosome concentrations (absolute total CCFNs, regardless of histone modification) are only an indicator of cell damage, while CCFNs with specific nucleosome modifications can determine the cause of cell damage and possibly serve as useful markers for renal allograft rejection. More studies on this topic will be interesting.
4 The utility of molecular immune monitoring for renal allograft rejection in clinical practice
4.1 PROS and CONS
The most advantage of molecular immune monitoring for renal allograft rejection is the superior sensitivity and specificity to the conventional markers (serum creatinine, eGFR, proteinuria, and DSAs) which can reduce unnecessary invasive renal allograft biopsy (148). With conventional markers, detection of subclinical changes is difficult due to the lower sensitivity. Although serum creatinine at one-year post-transplantation reflects long-term renal allograft outcome (149), an individual serum creatinine level is neither sensitive nor specific for early renal allograft injury, particularly compared to urine chemokines (114). Likewise, both albuminuria and proteinuria are nonspecific markers of renal allograft injury without a demonstrable association with renal allograft pathology (150, 151). Although current data support the use of de novo DSAs post-transplantation which is associated with decreased renal allograft survival (23, 152), the utilization of DSAs as a noninvasive diagnosis of AMR or a predictor for the long-term renal allograft outcomes has not been clearly elucidated (23). As such, innovative strategies (molecular immune monitoring methods) have been developed to overcome these limitations of the existing biomarkers. Most noninvasive molecular immune monitoring tools, including miR, gene expression, or protein level detection of molecular markers, have been proposed using the easily accessible biologic fluids (blood, serum, plasma, or urine) through a wide spectrum of platforms, mostly for frequent assessment of recipient’s immune status. However, the translation and validation of these discoveries and their implementation into standard transplantation clinical practice remain challenging. More large, prospective, interventional clinical trials are robustly needed to demonstrate the use of these molecular immune monitoring biomarkers for the improvement of renal allograft outcomes. In general, significant limitations of using these novel noninvasive molecular markers in clinical practice are regulatory issues, reimbursement from the Centers for Medicare and Medicaid Service, generalizability, cost, interpretation of the test, and, most importantly, the identification of beneficial populations compared with the conventional standard-of-care surveillance (153).
4.2 Combined molecular immune monitoring and the clinical parameters as a predictive score for renal allograft rejection
Due to the complexity and variability of immune responses, a panel of biomarkers (such as chemokines, DSAs, dd-cfDNA, and several miRs) might be more powerful than a single indicator for the prediction and diagnosis of renal allograft rejection and the differentiation between TCMR and AMR. For example, the Common Rejection Module that consists of 11 genes might be overexpressed in the biopsy samples from various solid organ transplants, including renal allograft rejection (154). Additionally, the urinary gene expression-based score (mRNA of these 11 genes) using urine from 150 renal transplant recipients with concurrent renal biopsies, including 43 stable biopsies, 45 acute rejections (TCMR or AMR or mixed), 19 ambiguous rejections, and 43 BKV, demonstrates 95% and 98% sensitivity and specificity, respectively, for the diagnosis of acute rejection (155). The sensitivity of the urinary gene expression-based score for diagnosis of acute renal rejection is reduced to 87.1% with an addition of the cases with ambiguous renal rejection into the stable biopsy and is decreased to 77% sensitivity with an addition of BKV nephropathy cases, with an unchanged specificity (155). Then, the urinary gene expression-based score may be useful for the non-invasive monitoring of acute renal allograft rejection.
Indeed, the addition of potential confounding cases (such as urinary tract infection and BK virus reactivation) in the stable biopsy as “a diagnostic multi-parametric model” improves the performance of the biomarkers (120). As such, a model with the combination of eight parameters (recipient age, gender, eGFR, DSA, signs of urinary tract infection, blood BKV viral load, urine CXCL9, and CXCL10) is able to diagnose acute renal allograft rejection with high accuracy (AUC: 0.85, 0.80–0.89). These results are paving the way for future studies using the combining urinary biomarkers with clinical characteristics to achieve the highest clinical relevance and provide targeted therapy for KT recipients (120). Recently, a research group from the University of California San Francisco demonstrates another comprehensive noninvasive tool for diagnosing and predicting renal allograft rejection (156). They explore the performance of target markers in a Kidney Injury Test assay for chronic kidney disease (CKD) staging in the native and non-transplanted kidney (157) and develop a Q-Score from these data for the detection of acute renal allograft rejection. Based on measurements of six urinary DNA, protein, and metabolic biomarkers, a noninvasive, spot urine-based diagnostic assay is proposed. On a cohort of 601 distinct urine samples with kidney injury (both native kidneys and renal allografts), the urinary composite score enables the diagnosis of acute renal allograft rejection, with an AUC of 0.99 for the receiver-operator characteristic (ROC) curve. Interestingly, the clinical utility of the assay can predict acute renal allograft rejection better than an increased serum creatinine resulting in an earlier rejection diagnosis than the current standard-of-care tests (156).
In summary, the use of a combination of multiple variables with mathematical approaches to calculating rejection probability, but not using only biomarkers of “graft functional impairment” alone might be very useful for an early diagnosis of rejection and might also be helpful for the selection of immunosuppressive protocols. Additionally, the rapid and routine monitoring of renal allografts is possibly enabled by the noninvasive assays, especially with sensitive and quantitative methods.
5 Future directions
While the establishment of a worldwide consensus framework (i.e., the Banff criteria) is still ongoing, a great deal of progress has been made in the field of the diagnostic evaluations of allograft pathology. In the foreseeable future, a molecular diagnostic model for renal allograft pathology should show significant steps toward the final development of a decentralized multi-platform compatible system. This could significantly impact clinical practice and outcomes by placing particular emphasis on the complex normalization pipelines required to compare gene expression data generated by different technologies. The creation of this system must integrate the efforts of the whole transplantation community for its validation to ensure that these molecular technologies provide optimal performance. In addition, the continuous updating of diagnostic criteria for renal allograft rejection and related lesions has improved diagnostic accuracy and clinicopathologic correlations, while also helping to clarify the limitations of histology and immunohistology in renal allograft biopsy interpretation. This has highlighted the need for the development of additional diagnostic modalities, including molecular diagnostics.
6 Conclusions
New-generation biomarkers in kidney transplantation are a collection of advanced indicators that provide a more comprehensive understanding of the status of a renal allograft. This has enabled the prognosis of the ultimate long-term renal allograft outcomes through the early detection of renal allograft rejection or dysfunction (Table 1). Although these biomarkers are now promising, further study is required to establish their therapeutic relevance and to find appropriate procedures for measuring and interpreting the data, especially in kidney transplant recipients. The choice of biomarkers may rely on the specific research topic, the type of accessible sample, and the isolation and analysis procedures employed. Interestingly, the integration of numerous indicators for a complete approach may improve accuracy and provide a bird’s-eye perspective of the condition of kidney allografts in individual recipients.

Table 1 Summary of the novel biomarker studies of immunologic monitoring in kidney transplant rejection.
Author contributions
The followings are the authors’ contribution: conceptualization, WC, OT, and AL. Writing—original draft preparation, WC. Writing—review and editing, WC, OT, AL AT. Funding acquisition, WC; and supervision, AL and AT. All authors contributed to the article and approved the submitted version.
Funding
This research project is supported by the Health System Research Institute (HSRI)-Flagship Project Fund: fiscal year 2020 (HSRI 63-081).
Conflict of interest
The authors declare that the research was conducted in the absence of any commercial or financial relationships that could be construed as a potential conflict of interest.
Publisher’s note
All claims expressed in this article are solely those of the authors and do not necessarily represent those of their affiliated organizations, or those of the publisher, the editors and the reviewers. Any product that may be evaluated in this article, or claim that may be made by its manufacturer, is not guaranteed or endorsed by the publisher.
References
1. Wolfe RA, Ashby VB, Milford EL, Ojo AO, Ettenger RE, Agodoa LY, et al. Comparison of mortality in all patients on dialysis, patients on dialysis awaiting transplantation, and recipients of a first cadaveric transplant. N Engl J Med (1999) 341(23):1725–30. doi: 10.1056/NEJM199912023412303
2. Poggio ED, Augustine JJ, Arrigain S, Brennan DC, Schold JD. Long-term kidney transplant graft survival-Making progress when most needed. Am J Transplant (2021) 21(8):2824–32. doi: 10.1111/ajt.16463
3. Larpparisuth N, Cheungpasitporn W, Lumpaopong A. Global perspective on kidney transplantation: Thailand. Kidney360 (2021) 2(7):1163–5. doi: 10.34067/KID.0002102021
4. Lamb K, Lodhi S, Meier-Kriesche HU. Long-term renal allograft survival in the United States: a critical reappraisal. Am J Transplant (2011) 11(3):450–62. doi: 10.1111/j.1600-6143.2010.03283.x
5. Loupy A, Aubert O, Orandi BJ, Naesens M, Bouatou Y, Raynaud M, et al. Prediction system for risk of allograft loss in patients receiving kidney transplants: international derivation and validation study. Br Med J (2019) 366:l4923. doi: 10.1136/bmj.l4923
6. Saengram W, Vadcharavivad S, Poolsup N, Chancharoenthana W. Extended release versus immediate release tacrolimus in kidney transplant recipients: a systematic review and meta-analysis. Eur J Clin Pharmacol (2018) 74:1249–60. doi: 10.1007/s00228-018-2512-7
7. Schnitzler MA, Johnston K, Axelrod D, Gheorghian A, Lentine KL. Associations of renal function at 1-year after kidney transplantation with subsequent return to dialysis, mortality, and healthcare costs. Transplantation (2011) 91(12):1347–56. doi: 10.1097/TP.0b013e31821ab993
8. Clayton PA, McDonald SP, Russ GR, Chadban SJ. Long-term outcomes after acute rejection in kidney transplant recipients: An ANZDATA analysis. J Am Soc Nephrol (2019) 30(9):1697–707. doi: 10.1681/ASN.2018111101
9. Li L, Khatri P, Sigdel TK, Tran T, Ying L, Vitalone MJ, et al. A peripheral blood diagnostic test for acute rejection in renal transplantation. Am J Transplant (2012) 12(10):2710–8. doi: 10.1111/j.1600-6143.2012.04253.x
10. Hirt-Minkowski P, Amico P, Ho J, Gao A, Bestland J, Hopfer H, et al. Detection of clinical and subclinical tubulo-interstitial inflammation by the urinary CXCL10 chemokine in a real-life setting. Am J Transplant (2012) 12(7):1811–23. doi: 10.1111/j.1600-6143.2012.03999.x
11. Bestard O, Nickel P, Cruzado JM, Schoenemann C, Boenisch O, Sefrin A, et al. Circulating alloreactive T cells correlate with graft function in longstanding renal transplant recipients. J Am Soc Nephrol (2008) 19(7):1419–29. doi: 10.1681/ASN.2007050539
12. Cherkassky L, Lanning M, Lalli PN, Czerr J, Siegel H, Danziger-Isakov L, et al. Evaluation of alloreactivity in kidney transplant recipients treated with antithymocyte globulin versus IL-2 receptor blocker. Am J Transplant (2011) 11(7):1388–96. doi: 10.1111/j.1600-6143.2011.03540.x
13. Haas M, Loupy A, Lefaucheur C, Roufosse C, Glotz D, Seron D, et al. The Banff 2017 Kidney Meeting Report: Revised diagnostic criteria for chronic active T cell-mediated rejection, antibody-mediated rejection, and prospects for integrative endpoints for next-generation clinical trials. Am J Transplant (2018) 18(2):293–307. doi: 10.1111/ajt.14625
14. Solez K, Colvin RB, Racusen LC, Sis B, Halloran PF, Birk PE, et al. Banff '05 Meeting Report: differential diagnosis of chronic allograft injury and elimination of chronic allograft nephropathy ('CAN'). Am J Transplant (2007) 7(3):518–26. doi: 10.1111/j.1600-6143.2006.01688.x
15. Loupy A, Haas M, Roufosse C, Naesens M, Adam B, Afrouzian M, et al. The Banff 2019 Kidney Meeting Report (I): Updates on and clarification of criteria for T cell– and antibody-mediated rejection. Am J Transplant (2020) 20(9):2318–31. doi: 10.1111/ajt.15898
16. Loupy A, Mengel M, Haas M. Thirty years of the International Banff Classification for Allograft Pathology: the past, present, and future of kidney transplant diagnostics. Kidney Int (2022) 101(4):678–91. doi: 10.1016/j.kint.2021.11.028
17. Callemeyn J, Lamarthée B, Koenig A, Koshy P, Thaunat O, Naesens M. Allorecognition and the spectrum of kidney transplant rejection. Kidney Int (2022) 101(4):692–710. doi: 10.1016/j.kint.2021.11.029
18. Roufosse C, Simmonds N, Clahsen-van Groningen M, Haas M, Henriksen KJ, Horsfield C, et al. A 2018 reference guide to the banff classification of renal allograft pathology. Transplantation (2018) 102(11):1795–814. doi: 10.1097/TP.0000000000002366
19. Nankivell BJ, Shingde M, Keung KL, Fung CL, Borrows RJ, O'Connell PJ, et al. The causes, significance and consequences of inflammatory fibrosis in kidney transplantation: The Banff i-IFTA lesion. Am J Transplant (2018) 18(2):364–76. doi: 10.1111/ajt.14609
20. Nankivell BJ, Agrawal N, Sharma A, Taverniti A, P'Ng CH, Shingde M, et al. The clinical and pathological significance of borderline T cell-mediated rejection. Am J Transplant (2019) 19(5):1452–63. doi: 10.1111/ajt.15197
21. Loupy A, Lefaucheur C. Antibody-mediated rejection of solid-organ allografts. N Engl J Med (2018) 379(12):1150–60. doi: 10.1056/NEJMra1802677
22. Cornell LD, Smith RN, Colvin RB. Kidney transplantation: mechanisms of rejection and acceptance. Annu Rev Pathol (2008) 3:189–220. doi: 10.1146/annurev.pathmechdis.3.121806.151508
23. Wiebe C, Gibson IW, Blydt-Hansen TD, Karpinski M, Ho J, Storsley LJ, et al. Evolution and clinical pathologic correlations of de novo donor-specific HLA antibody post kidney transplant. Am J Transplant (2012) 12(5):1157–67. doi: 10.1111/j.1600-6143.2012.04013.x
24. Dragun D, Catar R, Philippe A. Non-HLA antibodies against endothelial targets bridging allo- and autoimmunity. Kidney Int (2016) 90(2):280–8. doi: 10.1016/j.kint.2016.03.019
25. Cornaby C, Gibbons L, Mayhew V, Sloan CS, Welling A, Poole BD. B cell epitope spreading: mechanisms and contribution to autoimmune diseases. Immunol Lett (2015) 163(1):56–68. doi: 10.1016/j.imlet.2014.11.001
26. Valenzuela NM, Reed EF. Antibody-mediated rejection across solid organ transplants: manifestations, mechanisms, and therapies. J Clin Invest (2017) 127(7):2492–504. doi: 10.1172/JCI90597
27. Montgomery RA, Loupy A, Segev DL. Antibody-mediated rejection: New approaches in prevention and management. Am J Transplant (2018) 18 Suppl 3:3–17. doi: 10.1111/ajt.14584
28. Wan SS, Ying TD, Wyburn K, Roberts DM, Wyld M, Chadban SJ. The treatment of antibody-mediated rejection in kidney transplantation: An updated systematic review and meta-analysis. Transplantation (2018) 102(4):557–68. doi: 10.1097/TP.0000000000002049
29. Bronkhorst AJ, Ungerer V, Holdenrieder S. The emerging role of cell-free DNA as a molecular marker for cancer management. Biomol Detect Quantif (2019) 17:100087. doi: 10.1016/j.bdq.2019.100087
30. Diehl F, Schmidt K, Choti MA, Romans K, Goodman S, Li M, et al. Circulating mutant DNA to assess tumor dynamics. Nat Med (2008) 14(9):985–90. doi: 10.1038/nm.1789
31. Alekseeva LA, Mironova NL, Brenner EV, Kurilshikov AM, Patutina OA, Zenkova MA. Alteration of the exDNA profile in blood serum of LLC-bearing mice under the decrease of tumour invasion potential by bovine pancreatic DNase I treatment. PloS One (2017) 12(2):e0171988. doi: 10.1371/journal.pone.0171988
32. Shen J, Zhou Y, Chen Y, Li X, Lei W, Ge J, et al. Dynamics of early post-operative plasma ddcfDNA levels in kidney transplantation: a single-center pilot study. Transpl Int (2019) 32(2):184–92. doi: 10.1111/tri.13341
33. Xie WY, Kim K, Goussous N, Drachenberg CB, Scalea JR, Weir MR, et al. Causes of renal allograft injury in recipients with normal donor-derived cell-free DNA. Transplant Direct (2021) 7(4):e679. doi: 10.1097/TXD.0000000000001135
34. Bloom RD, Bromberg JS, Poggio ED, Bunnapradist S, Langone AJ, Sood P, et al. Cell-free DNA and active rejection in kidney allografts. J Am Soc Nephrol (2017) 28(7):2221–32. doi: 10.1681/ASN.2016091034
35. Sigdel TK, Archila FA, Constantin T, Prins SA, Liberto J, Damm I, et al. Optimizing detection of kidney transplant injury by assessment of donor-derived cell-free DNA via massively multiplex PCR. J Clin Med (2018) 8(1):19. doi: 10.3390/jcm8010019
36. Oellerich M, Shipkova M, Asendorf T, Walson PD, Schauerte V, Mettenmeyer N, et al. Absolute quantification of donor-derived cell-free DNA as a marker of rejection and graft injury in kidney transplantation: Results from a prospective observational study. Am J Transplant (2019) 19(11):3087–99. doi: 10.1111/ajt.15416
37. Stites E, Kumar D, Olaitan O, John Swanson S, Leca N, Weir M, et al. High levels of dd-cfDNA identify patients with TCMR 1A and borderline allograft rejection at elevated risk of graft injury. Am J Transplant (2020) 20(9):2491–8. doi: 10.1111/ajt.15822
38. Xiao H, Gao F, Pang Q, Xia Q, Zeng X, Peng J, et al. Diagnostic accuracy of donor-derived cell-free DNA in renal-allograft rejection: A meta-analysis. Transplantation (2021) 105(6):1303–10. doi: 10.1097/TP.0000000000003443
39. Garg N, Mandelbrot DA, Parajuli S, Aziz F, Astor BC, Chandraker A, et al. The clinical value of donor-derived cell-free DNA measurements in kidney transplantation. Transplant Rev (2021) 35(4):100649. doi: 10.1016/j.trre.2021.100649
40. Huang E, Haas M, Gillespie M, Sethi S, Peng A, Najjar R, et al. An assessment of the value of donor-derived cell-free DNA surveillance in patients with preserved kidney allograft function. Transplantation (2023) 107(1):274–82. doi: 10.1097/TP.0000000000004267
41. Bu L, Gupta G, Pai A, Anand S, Stites E, Moinuddin I, et al. Clinical outcomes from the Assessing Donor-derived cell-free DNA Monitoring Insights of kidney Allografts with Longitudinal surveillance (ADMIRAL) study. Kidney Int (2022) 101(4):793–803. doi: 10.1016/j.kint.2021.11.034
42. Gupta G, Moinuddin I, Kamal L, King AL, Winstead R, Demehin M, et al. Correlation of donor-derived cell-free DNA with histology and molecular diagnoses of kidney transplant biopsies. Transplantation (2022) 106(5):1061–70. doi: 10.1097/TP.0000000000003838
43. Halloran PF, Reeve J, Madill-Thomsen KS, Demko Z, Prewett A, Billings P. The trifecta study: Comparing plasma levels of donor-derived cell-free DNA with the molecular phenotype of kidney transplant biopsies. J Am Soc Nephrol (2022) 33(2):387–400. doi: 10.1681/ASN.2021091191
44. Huang E, Sethi S, Peng A, Najjar R, Mirocha J, Haas M, et al. Early clinical experience using donor-derived cell-free DNA to detect rejection in kidney transplant recipients. Am J Transplant (2019) 19(6):1663–70. doi: 10.1111/ajt.15289
45. Whitlam JB, Ling L, Skene A, Kanellis J, Ierino FL, Slater HR, et al. Diagnostic application of kidney allograft-derived absolute cell-free DNA levels during transplant dysfunction. Am J Transplant (2019) 19(4):1037–49. doi: 10.1111/ajt.15142
46. Kyeso Y, Bhalla A, Smith AP, Jia Y, Alakhdhair S, Ogir SC, et al. Donor-derived cell-free DNA kinetics post-kidney transplant biopsy. Transplant Direct (2021) 7(6):e703. doi: 10.1097/TXD.0000000000001149
47. Obrișcă B, Butiu M, Sibulesky L, Bakthavatsalam R, Smith KD, Gimferrer I, et al. Combining donor-derived cell-free DNA and donor specific antibody testing as non-invasive biomarkers for rejection in kidney transplantation. Sci Rep (2022) 12(1):15061. doi: 10.1038/s41598-022-19017-7
48. Jordan SC, Bunnapradist S, Bromberg JS, Langone AJ, Hiller D, Yee JP, et al. Donor-derived cell-free DNA identifies antibody-mediated rejection in donor specific antibody positive kidney transplant recipients. Transplant Direct (2018) 4(9):e379. doi: 10.1097/TXD.0000000000000821
49. Haas M, Sis B, Racusen LC, Solez K, Glotz D, Colvin RB, et al. Banff 2013 meeting report: inclusion of c4d-negative antibody-mediated rejection and antibody-associated arterial lesions. Am J Transplant (2014) 14(2):272–83. doi: 10.1111/ajt.12590
50. Venner JM, Hidalgo LG, Famulski KS, Chang J, Halloran PF. The molecular landscape of antibody-mediated kidney transplant rejection: evidence for NK involvement through CD16a Fc receptors. Am J Transplant (2015) 15(5):1336–48. doi: 10.1111/ajt.13115
51. Adam B, Afzali B, Dominy KM, Chapman E, Gill R, Hidalgo LG, et al. Multiplexed color-coded probe-based gene expression assessment for clinical molecular diagnostics in forMalin-fixed paraffin-embedded human renal allograft tissue. Clin Transplant (2016) 30(3):295–305. doi: 10.1111/ctr.12689
52. Halloran PF, Pereira AB, Chang J, Matas A, Picton M, De Freitas D, et al. Potential impact of microarray diagnosis of T cell-mediated rejection in kidney transplants: The INTERCOM study. Am J Transplant (2013) 13(9):2352–63. doi: 10.1111/ajt.12387
53. Halloran PF, Reeve J, Akalin E, Aubert O, Bohmig GA, Brennan D, et al. Real time central assessment of kidney transplant indication biopsies by microarrays: The INTERCOMEX study. Am J Transplant (2017) 17(11):2851–62. doi: 10.1111/ajt.14329
54. Geiss GK, Bumgarner RE, Birditt B, Dahl T, Dowidar N, Dunaway DL, et al. Direct multiplexed measurement of gene expression with color-coded probe pairs. Nat Biotechnol (2008) 26(3):317–25. doi: 10.1038/nbt1385
55. Loupy A, Haas M, Solez K, Racusen L, Glotz D, Seron D, et al. The banff 2015 kidney meeting report: Current challenges in rejection classification and prospects for adopting molecular pathology. Am J Transplant (2017) 17(1):28–41. doi: 10.1111/ajt.14107
56. Mengel M, Loupy A, Haas M, Roufosse C, Naesens M, Akalin E, et al. Banff 2019 Meeting Report: Molecular diagnostics in solid organ transplantation-Consensus for the Banff Human Organ Transplant (B-HOT) gene panel and open source multicenter validation. Am J Transplant (2020) 20(9):2305–17. doi: 10.1111/ajt.16059
57. Varol H, Ernst A, Cristoferi I, Arns W, Baan CC, van Baardwijk M, et al. Feasibility and potential of transcriptomic analysis using the nanoString nCounter technology to aid the classification of rejection in kidney transplant biopsies. Transplantation (2023) 107(5):903–12. doi: 10.1097/TP.0000000000004372
58. Smith RN, Rosales IA, Tomaszewski KT, Mahowald GT, Araujo-Medina M, Acheampong E, et al. Utility of banff human organ transplant gene panel in human kidney transplant biopsies. Transplantation (2023) 107(5):1188–99. doi: 10.1097/TP.0000000000004389
59. Deng MC, Eisen HJ, Mehra MR, Billingham M, Marboe CC, Berry G, et al. Noninvasive discrimination of rejection in cardiac allograft recipients using gene expression profiling. Am J Transplant (2006) 6(1):150–60. doi: 10.1111/j.1600-6143.2005.01175.x
60. Kurian SM, Williams AN, Gelbart T, Campbell D, Mondala TS, Head SR, et al. Molecular classifiers for acute kidney transplant rejection in peripheral blood by whole genome gene expression profiling. Am J Transplant (2014) 14(5):1164–72. doi: 10.1111/ajt.12671
61. Zhang W, Yi Z, Keung KL, Shang H, Wei C, Cravedi P, et al. A peripheral blood gene expression signature to diagnose subclinical acute rejection. J Am Soc Nephrol (2019) 30(8):1481–94. doi: 10.1681/ASN.2018111098
62. Van Loon E, Gazut S, Yazdani S, Lerut E, de Loor H, Coemans M, et al. Development and validation of a peripheral blood mRNA assay for the assessment of antibody-mediated kidney allograft rejection: A multicentre, prospective study. EBioMedicine (2019) 46:463–72. doi: 10.1016/j.ebiom.2019.07.028
63. Akalin E, Weir MR, Bunnapradist S, Brennan DC, Delos Santos R, Langone A, et al. Clinical validation of an immune quiescence gene expression signature in kidney transplantation. Kidney360 (2021) 2(12):1998–2009. doi: 10.34067/KID.0005062021
64. Roedder S, Sigdel T, Salomonis N, Hsieh S, Dai H, Bestard O, et al. The kSORT assay to detect renal transplant patients at high risk for acute rejection: results of the multicenter AART study. PloS Med (2014) 11(11):e1001759. doi: 10.1371/journal.pmed.1001759
65. Marsh CL, Kurian SM, Rice JC, Whisenant TC, David J, Rose S, et al. Application of truGraf v1: A novel molecular biomarker for managing kidney transplant recipients with stable renal function. Transplant Proc (2019) 51(3):722–8. doi: 10.1016/j.transproceed.2019.01.054
66. Park S, Guo K, Heilman RL, Poggio ED, Taber DJ, Marsh CL, et al. Combining blood gene expression and cellfree DNA to diagnose subclinical rejection in kidney transplant recipients. Clin J Am Soc Nephrol (2021) 16(10):1539–51. doi: 10.2215/CJN.05530421
67. Bartel DP. MicroRNAs: genomics, biogenesis, mechanism, and function. Cell (2004) 116(2):281–97. doi: 10.1016/S0092-8674(04)00045-5
68. Lorenzen JM, Volkmann I, Fiedler J, Schmidt M, Scheffner I, Haller H, et al. Urinary miR-210 as a mediator of acute T-cell mediated rejection in renal allograft recipients. Am J Transplant (2011) 11(10):2221–7. doi: 10.1111/j.1600-6143.2011.03679.x
69. Maluf DG, Dumur CI, Suh JL, Scian MJ, King AL, Cathro H, et al. The urine microRNA profile may help monitor post-transplant renal graft function. Kidney Int (2014) 85(2):439–49. doi: 10.1038/ki.2013.338
70. Amrouche L, Desbuissons G, Rabant M, Sauvaget V, Nguyen C, Benon A, et al. MicroRNA-146a in human and experimental ischemic AKI: CXCL8-dependent mechanism of action. J Am Soc Nephrol (2017) 28(2):479–93. doi: 10.1681/ASN.2016010045
71. Soltaninejad E, Nicknam MH, Nafar M, Ahmadpoor P, Pourrezagholi F, Sharbafi MH, et al. Differential expression of microRNAs in renal transplant patients with acute T-cell mediated rejection. Transpl Immunol (2015) 33(1):1–6. doi: 10.1016/j.trim.2015.05.002
72. Betts G, Shankar S, Sherston S, Friend P, Wood KJ. Examination of serum miRNA levels in kidney transplant recipients with acute rejection. Transplantation (2014) 97(4):e28–30. doi: 10.1097/01.TP.0000441098.68212.de
73. Tao J, Yang X, Han Z, Lu P, Wang J, Liu X, et al.Serum microRNA-99a helps detect acute rejection in renal transplantation. Transplant Proc. (2015) 47(6):1683–7. doi: 10.1016/j.transproceed.2015.04.094.
74. Anglicheau D, Sharma VK, Ding R, Hummel A, Snopkowski C, Dadhania D, et al. MicroRNA expression profiles predictive of human renal allograft status. Proc Natl Acad Sci U.S.A. (2009) 106(13):5330–5. doi: 10.1073/pnas.0813121106
75. Oghumu S, Bracewell A, Nori U, Maclean KH, Balada-Lasat J-M, Brodsky S, et al. Acute pyelonephritis in renal allografts–a new role for MicroRNAs? Transplantation (2014) 97(5):559. doi: 10.1097/01.TP.0000441322.95539.b3
76. Matz M, Fabritius K, Lorkowski C, Dürr M, Gaedeke J, Durek P, et al. Identification of T cell–mediated vascular rejection after kidney transplantation by the combined measurement of 5 specific microRNAs in blood. Transplantation (2016) 100(4):898–907. doi: 10.1097/TP.0000000000000873
77. Danger R, Paul C, Giral M, Lavault A, Foucher Y, Degauque N, et al. Expression of miR-142-5p in peripheral blood mononuclear cells from renal transplant patients with chronic antibody-mediated rejection. PloS One (2013) 8(4):e60702. doi: 10.1371/journal.pone.0060702
78. Millán O, Budde K, Sommerer C, Aliart I, Rissling O, Bardaji B, et al. Urinary miR-155-5p and CXCL10 as prognostic and predictive biomarkers of rejection, graft outcome and treatment response in kidney transplantation. Br J Clin Pharmacol (2017) 83(12):2636–50. doi: 10.1111/bcp.13399
79. Scian M, Maluf D, David K, Archer K, Suh J, Wolen A, et al. MicroRNA profiles in allograft tissues and paired urines associate with chronic allograft dysfunction with IF/TA. Am J Transplant (2011) 11(10):2110–22. doi: 10.1111/j.1600-6143.2011.03666.x
80. Vahed SZ, Zonouzi AP, Mahmoodpoor F, Samadi N, Ardalan M, Omidi Y. Circulating miR-150, miR-192, miR-200b, and miR-423-3p as non-invasive biomarkers of chronic allograft dysfunction. Arch Med Res (2017) 48(1):96–104. doi: 10.1016/j.arcmed.2017.03.004
81. Glowacki F, Savary G, Gnemmi V, Van der Hauwaert C, Lo-Guidice J-M, et al. Increased circulating miR-21 levels are associated with kidney fibrosis. PloS One (2013) 8(2):e58014. doi: 10.1371/journal.pone.0058014
82. Pisitkun T, Shen RF, Knepper MA. Identification and proteomic profiling of exosomes in human urine. Proc Natl Acad Sci U.S.A. (2004) 101(36):13368–73. doi: 10.1073/pnas.0403453101
83. Robbins PD, Morelli AE. Regulation of immune responses by extracellular vesicles. Nat Rev Immunol (2014) 14(3):195–208. doi: 10.1038/nri3622
84. Panich T, Chancharoenthana W, Somparn P, Issara-Amphorn J, HIrankarn N, Leelahavanichkul A. Urinary exosomal activating transcriptional factor 3 as the early diagnostic biomarker for sepsis-induced acute kidney injury. BMC Nephrol (2017) 18(1):10. doi: 10.1186/s12882-016-0415-3
85. Erdbrügger U, Blijdorp CJ, Bijnsdorp IV, Borràs FE, Burger D, Bussolati B, et al. Urinary extracellular vesicles: A position paper by the Urine Task Force of the International Society for Extracellular Vesicles. J Extracell Vesicles (2021) 10(7):e12093. doi: 10.1002/jev2.12093
86. Harding C, Heuser J, Stahl P. Receptor-mediated endocytosis of transferrin and recycling of the transferrin receptor in rat reticulocytes. J Cell Biol (1983) 97(2):329–39. doi: 10.1083/jcb.97.2.329
87. Veziroglu EM, Mias GI. Characterizing extracellular vesicles and their diverse RNA contents. Front Genet (2020) 11:700. doi: 10.3389/fgene.2020.00700
88. Quaglia M, Dellepiane S, Guglielmetti G, Merlotti G, Castellano G, Cantaluppi V. Extracellular vesicles as mediators of cellular crosstalk between immune system and kidney graft. Front Immunol (2020) 11:74. doi: 10.3389/fimmu.2020.00074
89. Soekmadji C, Li B, Huang Y, Wang H, An T, Liu C, et al. The future of Extracellular Vesicles as Theranostics - an ISEV meeting report. J Extracell Vesicles (2020) 9(1):1809766. doi: 10.1080/20013078.2020.1809766
90. Vykoukal J, Sun N, Aguilar-Bonavides C, Katayama H, Tanaka I, Fahrmann JF, et al. Plasma-derived extracellular vesicle proteins as a source of biomarkers for lung adenocarcinoma. Oncotarget (2017) 8(56):95466–80. doi: 10.18632/oncotarget.20748
91. Turco AE, Lam W, Rule AD, Denic A, Lieske JC, Miller VM, et al. Specific renal parenchymal-derived urinary extracellular vesicles identify age-associated structural changes in living donor kidneys. J Extracell Vesicles (2016) 5:29642. doi: 10.3402/jev.v5.29642
92. Dimuccio V, Ranghino A, Praticò Barbato L, Fop F, Biancone L, Camussi G, et al. Urinary CD133+ extracellular vesicles are decreased in kidney transplanted patients with slow graft function and vascular damage. PloS One (2014) 9(8):e104490. doi: 10.1371/journal.pone.0104490
93. Jung HY, Lee CH, Choi JY, Cho JH, Park SH, Kim YL, et al. Potential urinary extracellular vesicle protein biomarkers of chronic active antibody-mediated rejection in kidney transplant recipients. J Chromatogr B Analyt Technol BioMed Life Sci (2020) 1138:121958. doi: 10.1016/j.jchromb.2019.121958
94. Takada Y, Kamimura D, Jiang JJ, Higuchi H, Iwami D, Hotta K, et al. Increased urinary exosomal SYT17 levels in chronic active antibody-mediated rejection after kidney transplantation via the IL-6 amplifier. Int Immunol (2020) 32(10):653–62. doi: 10.1093/intimm/dxaa032
95. Pisitkun T, Gandolfo MT, Das S, Knepper MA, Bagnasco SM. Application of systems biology principles to protein biomarker discovery: urinary exosomal proteome in renal transplantation. Proteomics Clin Appl (2012) 6(5-6):268–78. doi: 10.1002/prca.201100108
96. Esteva-Font C, Guillén-Gómez E, Diaz JM, Guirado L, Facundo C, Ars E, et al. Renal sodium transporters are increased in urinary exosomes of cyclosporine-treated kidney transplant patients. Am J Nephrol (2014) 39(6):528–35. doi: 10.1159/000362905
97. Tutakhel OAZ, Moes AD, Valdez-Flores MA, Kortenoeven MLA, Vrie MVD, Jeleń S, et al. NaCl cotransporter abundance in urinary vesicles is increased by calcineurin inhibitors and predicts thiazide sensitivity. PloS One (2017) 12(4):e0176220. doi: 10.1371/journal.pone.0176220
98. Ponticelli C. Ischaemia-reperfusion injury: a major protagonist in kidney transplantation. Nephrol Dial Transplant (2014) 29(6):1134–40. doi: 10.1093/ndt/gft488
99. Gatti S, Bruno S, Deregibus MC, Sordi A, Cantaluppi V, Tetta C, et al. Microvesicles derived from human adult mesenchymal stem cells protect against ischaemia-reperfusion-induced acute and chronic kidney injury. Nephrol Dial Transplant (2011) 26(5):1474–83. doi: 10.1093/ndt/gfr015
100. Li ZL, Lv LL, Tang TT, Wang B, Feng Y, Zhou LT, et al. HIF-1α inducing exosomal microRNA-23a expression mediates the cross-talk between tubular epithelial cells and macrophages in tubulointerstitial inflammation. Kidney Int (2019) 95(2):388–404. doi: 10.1016/j.kint.2018.09.013
101. Park J, Lin HY, Assaker JP, Jeong S, Huang CH, Kurdi T, et al. Integrated kidney exosome analysis for the detection of kidney transplant rejection. ACS Nano (2017) 11(11):11041–6. doi: 10.1021/acsnano.7b05083
102. El Fekih R, Hurley J, Tadigotla V, Alghamdi A, Srivastava A, Coticchia C, et al. Discovery and validation of a urinary exosome mRNA signature for the diagnosis of human kidney transplant rejection. J Am Soc Nephrol (2021) 32(4):994–1004. doi: 10.1681/ASN.2020060850
103. Woud WW, Arykbaeva AS, Alwayn IPJ, Baan CC, Minnee RC, Hoogduijn MJ, et al. Extracellular vesicles released during normothermic machine perfusion are associated with human donor kidney characteristics. Transplantation (2022) 106(12):2360–9. doi: 10.1097/TP.0000000000004215
104. Gregorini M, Corradetti V, Pattonieri EF, Rocca C, Milanesi S, Peloso A, et al. Perfusion of isolated rat kidney with Mesenchymal Stromal Cells/Extracellular Vesicles prevents ischaemic injury. J Cell Mol Med (2017) 21(12):3381–93. doi: 10.1111/jcmm.13249
105. Altan-Bonnet N, Perales C, Domingo E. Extracellular vesicles: Vehicles of en bloc viral transmission. Virus Res (2019) 265:143–9. doi: 10.1016/j.virusres.2019.03.023
106. Altan-Bonnet N. Extracellular vesicles are the Trojan horses of viral infection. Curr Opin Microbiol (2016) 32:77–81. doi: 10.1016/j.mib.2016.05.004
107. Chancharoenthana W, Leelahavanichkul A. Innate immunity response to BK virus infection in polyomavirus-associated nephropathy in kidney transplant recipients. Transplantology (2022) 3(1):20–32. doi: 10.3390/transplantology3010003
108. Martelli F, Wu Z, Delbue S, Weissbach FH, Giulioli MC, Ferrante P, et al. BK polyomavirus microRNA levels in exosomes are modulated by non-coding control region activity and down-regulate viral replication when delivered to non-infected cells prior to infection. Viruses (2018) 10(9):466. doi: 10.3390/v10090466
109. Kim MH, Lee YH, Seo JW, Moon H, Kim JS, Kim YG, et al. Urinary exosomal viral microRNA as a marker of BK virus nephropathy in kidney transplant recipients. PloS One (2017) 12(12):e0190068. doi: 10.1371/journal.pone.0190068
110. Huang Y, Zeng G, Randhawa PS. Detection of BKV encoded mature MicroRNAs in kidney transplant patients: Clinical and biologic insights. J Clin Virol (2019) 119:6–10. doi: 10.1016/j.jcv.2019.07.006
111. Ashcroft J, Leighton P, Elliott TR, Hosgood SA, Nicholson ML, Kosmoliaptsis V. Extracellular vesicles in kidney transplantation: a state-of-the-art review. Kidney Int (2022) 101(3):485–97. doi: 10.1016/j.kint.2021.10.038
112. Manca S, Upadhyaya B, Mutai E, Desaulniers AT, Cederberg RA, White BR, et al. Milk exosomes are bioavailable and distinct microRNA cargos have unique tissue distribution patterns. Sci Rep (2018) 8(1):11321. doi: 10.1038/s41598-018-29780-1
113. Jackson JA, Kim EJ, Begley B, Cheeseman J, Harden T, Perez SD, et al. Urinary chemokines CXCL9 and CXCL10 are noninvasive markers of renal allograft rejection and BK viral infection. Am J Transplant (2011) 11(10):2228–34. doi: 10.1111/j.1600-6143.2011.03680.x
114. Rabant M, Amrouche L, Lebreton X, Aulagnon F, Benon A, Sauvaget V, et al. Urinary C-X-C motif chemokine 10 independently improves the noninvasive diagnosis of antibody-mediated kidney allograft rejection. J Am Soc Nephrol (2015) 26(11):2840–51. doi: 10.1681/ASN.2014080797
115. Blydt-Hansen TD, Sharma A, Gibson IW, Wiebe C, Sharma AP, Langlois V, et al. Validity and utility of urinary CXCL10/Cr immune monitoring in pediatric kidney transplant recipients. Am J Transplant (2021) 21(4):1545–55. doi: 10.1111/ajt.16336
116. Rabant M, Amrouche L, Morin L, Bonifay R, Lebreton X, Aouni L, et al. Early low urinary CXCL9 and CXCL10 might predict immunological quiescence in clinically and histologically stable kidney recipients. Am J Transplant (2016) 16(6):1868–81. doi: 10.1111/ajt.13677
117. Schaub S, Nickerson P, Rush D, Mayr M, Hess C, Golian M, et al. Urinary CXCL9 and CXCL10 levels correlate with the extent of subclinical tubulitis. Am J Transplant (2009) 9(6):1347–53. doi: 10.1111/j.1600-6143.2009.02645.x
118. Ho J, Schaub S, Wiebe C, Gao A, Wehmeier C, Koller MT, et al. Urinary CXCL10 chemokine is associated with alloimmune and virus compartment-specific renal allograft inflammation. Transplantation (2018) 102(3):521–9. doi: 10.1097/TP.0000000000001931
119. Mühlbacher J, Doberer K, Kozakowski N, Regele H, Camovic S, Haindl S, et al. Non-invasive chemokine detection: improved prediction of antibody-mediated rejection in donor-specific antibody-positive renal allograft recipients. Front Med (2020) 7:114. doi: 10.3389/fmed.2020.00114
120. Tinel C, Devresse A, Vermorel A, Sauvaget V, Marx D, Avettand-Fenoel V, et al. Development and validation of an optimized integrative model using urinary chemokines for noninvasive diagnosis of acute allograft rejection. Am J Transplant (2020) 20(12):3462–76. doi: 10.1111/ajt.15959
121. Hricik DE, Formica RN, Nickerson P, Rush D, Fairchild RL, Poggio E, et al. Adverse outcomes of tacrolimus withdrawal in immune-quiescent kidney transplant recipients. J Am Soc Nephrol (2015) 26(12):3114–22. doi: 10.1681/ASN.2014121234
122. Brunet M, Shipkova M, van Gelder T, Wieland E, Sommerer C, Budde K, et al. Barcelona consensus on biomarker-based immunosuppressive drugs management in solid organ transplantation. Ther Drug Monit (2016) 38 Suppl 1:S1–20. doi: 10.1097/FTD.0000000000000287
123. Lazzeri E, Rotondi M, Mazzinghi B, Lasagni L, Buonamano A, Rosati A, et al. High CXCL10 expression in rejected kidneys and predictive role of pretransplant serum CXCL10 for acute rejection and chronic allograft nephropathy. Transplantation (2005) 79(9):1215–20. doi: 10.1097/01.TP.0000160759.85080.2E
124. Heidt S, Shankar S, Muthusamy AS, San Segundo D, Wood KJ. Pretransplant serum CXCL9 and CXCL10 levels fail to predict acute rejection in kidney transplant recipients receiving induction therapy. Transplantation (2011) 91(8):e59–61. doi: 10.1097/TP.0b013e318210de6b
125. Xu C-X, Shi B-Y, Jin Z-K, Hao JJ, Duan WL, Han F, et al. Multiple-biomarkers provide powerful prediction of early acute renal allograft rejection by combination of serum fractalkine, IFN-γ and IP-10. Transpl Immunol (2018) 50:68–74. doi: 10.1016/j.trim.2018.08.003
126. Millán O, Rovira J, Guirado L, Espinosa C, Budde K, Sommerer C, et al. Advantages of plasmatic CXCL-10 as a prognostic and diagnostic biomarker for the risk of rejection and subclinical rejection in kidney transplantation. Clin Immunol (2021) 229:108792. doi: 10.1016/j.clim.2021.108792
127. Hollmen ME, Kyllönen LE, Inkinen KA, Lalla ML, Merenmies J, Salmela KT. Deceased donor neutrophil gelatinase-associated lipocalin and delayed graft function after kidney transplantation: a prospective study. Crit Care (2011) 15(3):R121. doi: 10.1186/cc10220
128. Li YM, Li Y, Yan L, Wang H, Wu XJ, Tang JT, et al. Comparison of urine and blood NGAL for early prediction of delayed graft function in adult kidney transplant recipients: a meta-analysis of observational studies. BMC Nephrol (2019) 20(1):291. doi: 10.1186/s12882-019-1491-y
129. Kaufeld JK, Gwinner W, Scheffner I, Haller HG, Schiffer M. Urinary NGAL ratio is not a sensitive biomarker for monitoring acute tubular injury in kidney transplant patients: NGAL and ATI in renal transplant patients. J Transplant (2012) 2012:563404. doi: 10.1155/2012/563404
130. Ramirez-Sandoval JC, Barrera-Chimal J, Simancas PE, Correa-Rotter R, Bobadilla NA, Morales-Buenrostro LE. Tubular urinary biomarkers do not identify aetiology of acute kidney injury in kidney transplant recipients. Nephrol (Carlton) (2014) 19(6):352–8. doi: 10.1111/nep.12240
131. Ramírez-Sandoval JC, Barrera-Chimal J, Simancas PE, Rojas-Montaño A, Correa-Rotter R, Bobadilla NA, et al. Urinary neutrophil gelatinase-associated lipocalin predicts graft loss after acute kidney injury in kidney transplant. Biomarkers (2014) 19(1):63–9. doi: 10.3109/1354750X.2013.867536
132. Cassidy H, Slyne J, O'Kelly P, Traynor C, Conlon PJ, Johnston O, et al. Urinary biomarkers of chronic allograft nephropathy. Proteomics Clin Appl (2015) 9(5-6):574–85. doi: 10.1002/prca.201400200
133. Lacquaniti A, Caccamo C, Salis P, Chirico V, Buemi A, Cernaro V, et al. Delayed graft function and chronic allograft nephropathy: diagnostic and prognostic role of neutrophil gelatinase-associated lipocalin. Biomarkers (2016) 21(4):371–8. doi: 10.3109/1354750X.2016.1141991
134. Kielar M, Dumnicka P, Gala-Błądzińska A, Będkowska-Prokop A, Ignacak E, Maziarz B, et al. Urinary ngal measured after the first year post kidney transplantation predicts changes in glomerular filtration over one-year follow-up. J Clin Med (2020) 10(1):43. doi: 10.3390/jcm10010043
135. Tabernero G, Pescador M, Ruiz Ferreras E, Morales AI, Prieto M. Evaluation of NAG, NGAL, and KIM-1 as prognostic markers of the initial evolution of kidney transplantation. Diagnostics (Basel) (2023) 13(11):1843. doi: 10.3390/diagnostics13111843
136. Zhu M, Chen Z, Wei Y, Yuan Y, Ying L, Zhou H, et al. The predictive value of urinary kidney injury molecular-1 for long-term graft function in kidney transplant patients: a prospective study. Ann Transl Med (2021) 9(3):244. doi: 10.21037/atm-20-2215a
137. van Timmeren MM, Vaidya VS, van Ree RM, Oterdoom LH, de Vries AP, Gans RO, et al. High urinary excretion of kidney injury molecule-1 is an independent predictor of graft loss in renal transplant recipients. Transplantation (2007) 84(12):1625–30. doi: 10.1097/01.tp.0000295982.78039.ef
138. Malyszko J, Koc-Zorawska E, Malyszko JS, Mysliwiec M. Kidney injury molecule-1 correlates with kidney function in renal allograft recipients. Transplant Proc (2010) 42(10):3957–9. doi: 10.1016/j.transproceed.2010.10.005
139. Verhoeven JG, Boer K, Van Schaik RH, Manintveld OC, Huibers MMH, Baan CC, et al. Liquid biopsies to monitor solid organ transplant function: a review of new biomarkers. Ther Drug Monit (2018) 40(5):515–25. doi: 10.1097/FTD.0000000000000549
140. Strahl BD, Allis CD. The language of covalent histone modifications. Nature (2000) 403(6765):41–5. doi: 10.1038/47412
141. Chen R, Kang R, Fan X, Tang D. Release and activity of histone in diseases. Cell Death Dis (2014) 5(8):e1370–e. doi: 10.1038/cddis.2014.337
142. McAnena P, Brown JA, Kerin MJ. Circulating nucleosomes and nucleosome modifications as biomarkers in cancer. Cancers (2017) 9(1):5. doi: 10.3390/cancers9010005
143. Bauden M, Pamart D, Ansari D, Herzog M, Eccleston M, Micallef J, et al. Circulating nucleosomes as epigenetic biomarkers in pancreatic cancer. Clin Epigenet (2015) 7(1):1–7. doi: 10.1186/s13148-015-0139-4
144. Verhoeven JG, Baan CC, Peeters AM, Clahsen-van Groningen MC, Nieboer D, Herzog M, et al. Circulating cell-free nucleosomes as biomarker for kidney transplant rejection: a pilot study. Clin Epigenet (2021) 13:1–8. doi: 10.1186/s13148-020-00969-4
145. Wang Y, Li M, Stadler S, Correll S, Li P, Wang D, et al. Histone hypercitrullination mediates chromatin decondensation and neutrophil extracellular trap formation. J Cell Biol (2009) 184(2):205–13. doi: 10.1083/jcb.200806072
146. Scozzi D, Ibrahim M, Menna C, Krupnick AS, Kreisel D, Gelman AE. The role of neutrophils in transplanted organs. Am J Tranplant (2017) 17(2):328–35. doi: 10.1111/ajt.13940
147. Saithong S, Worasilchai N, Saisorn W, Udompornpitak K, Bhunyakarnjanarat T, Chindamporn A, et al. Neutrophil extracellular traps in severe SARS-coV-2 infection: A possible impact of LPS and (1→3)-β-D-glucan in blood from gut translocation. Cells (2022) 11(7):1103. doi: 10.3390/cells11071103
148. Henderson LK, Nankivell BJ, Chapman JR. Surveillance protocol kidney transplant biopsies: their evolving role in clinical practice. Am J Transplant (2011) 11(8):1570–5. doi: 10.1111/j.1600-6143.2011.03677.x
149. Hariharan S, McBride MA, Cherikh WS, Tolleris CB, Bresnahan BA, Johnson CP. Post-transplant renal function in the first year predicts long-term kidney transplant survival. Kidney Int (2002) 62(1):311–8. doi: 10.1046/j.1523-1755.2002.00424.x
150. Amer H, Lieske JC, Rule AD, Kremers WK, Larson TS, Franco Palacios CR, et al. Urine high and low molecular weight proteins one-year post-kidney transplant: relationship to histology and graft survival. Am J Transplant (2013) 13(3):676–84. doi: 10.1111/ajt.12044
151. Tsampalieros A, Knoll GA. Evaluation and management of proteinuria after kidney transplantation. Transplantation (2015) 99(10):2049–60. doi: 10.1097/TP.0000000000000894
152. Wiebe C, Gibson IW, Blydt-Hansen TD, Pochinco D, Birk PE, Ho J, et al. Rates and determinants of progression to graft failure in kidney allograft recipients with de novo donor-specific antibody. Am J Transplant (2015) 15(11):2921–30. doi: 10.1111/ajt.13347
153. Cravedi P, Mannon RB. Noninvasive methods to assess the risk of kidney transplant rejection. Expert Rev Clin Immunol (2009) 5(5):535–46. doi: 10.1586/eci.09.36
154. Khatri P, Roedder S, Kimura N, De Vusser K, Morgan AA, Gong Y, et al. A common rejection module (CRM) for acute rejection across multiple organs identifies novel therapeutics for organ transplantation. J Exp Med (2013) 210(11):2205–21. doi: 10.1084/jem.20122709
155. Sigdel TK, Yang JYC, Bestard O, Schroeder A, Hsieh SC, Liberto JM, et al. A urinary Common Rejection Module (uCRM) score for non-invasive kidney transplant monitoring. PloS One (2019) 14(7):e0220052. doi: 10.1371/journal.pone.0220052
156. Yang JYC, Sarwal RD, Sigdel TK, Damm I, Rosenbaum B, Liberto JM, et al. A urine score for noninvasive accurate diagnosis and prediction of kidney transplant rejection. Sci Transl Med (2020) 12(535):eaba2501. doi: 10.1126/scitranslmed.aba2501
157. Watson D, Yang JY, Sarwal RD, Sigdel TK, Liberto JM, Damm I, et al. A novel multi-biomarker assay for non-invasive quantitative monitoring of kidney injury. J Clin Med (2019) 8(4):499. doi: 10.3390/jcm8040499
158. Mayer KA, Omic H, Weseslindtner L, Doberer K, Reindl-Schwaighofer R, Viard T, et al. Levels of donor-derived cell-free DNA and chemokines in BK polyomavirus-associated nephropathy. Clin Transplant (2022) 36(11):e14785. doi: 10.1111/ctr.14785
159. Dauber EM, Kollmann D, Kozakowski N, Rasoul-Rockenschaub S, Soliman T, Berlakovich GA, et al. Quantitative PCR of INDELs to measure donor-derived cell-free DNA-a potential method to detect acute rejection in kidney transplantation: a pilot study. Transpl Int (2020) 33(3):298–309. doi: 10.1111/tri.13554
160. Friedewald JJ, Kurian SM, Heilman RL, Whisenant TC, Poggio ED, Marsh C, et al. Development and clinical validity of a novel blood-based molecular biomarker for subclinical acute rejection following kidney transplant. Am J Transplant (2019) 19(1):98–109. doi: 10.1111/ajt.15011
161. Crespo E, Roedder S, Sigdel T, Hsieh SC, Luque S, Cruzado JM, et al. Molecular and functional noninvasive immune monitoring in the ESCAPE study for prediction of subclinical renal allograft rejection. Transplantation (2017) 101(6):1400–9. doi: 10.1097/TP.0000000000001287
162. First MR, Peddi VR, Mannon R, Knight R, Marsh CL, Kurian SM, et al. Investigator assessment of the utility of the truGraf molecular diagnostic test in clinical practice. Transplant Proc (2019) 51(3):729–33. doi: 10.1016/j.transproceed.2018.10.024
163. Matz M, Lorkowski C, Fabritius K, Durek P, Wu K, Rudolph B, et al. Free microRNA levels in plasma distinguish T-cell mediated rejection from stable graft function after kidney transplantation. Transpl Immunol (2016) 39:52–9. doi: 10.1016/j.trim.2016.09.001
164. Matz M, Heinrich F, Lorkowski C, Wu K, Klotsche J, Zhang Q, et al. MicroRNA regulation in blood cells of renal transplanted patients with interstitial fibrosis/tubular atrophy and antibody-mediated rejection. PloS One (2018) 13(8):e0201925. doi: 10.1371/journal.pone.0201925
165. Ledeganck KJ, Gielis EM, Abramowicz D, Stenvinkel P, Shiels PG, Van Craenenbroeck AH. MicroRNAs in AKI and kidney transplantation. Clin J Am Soc Nephrol (2019) 14(3):454–68. doi: 10.2215/CJN.08020718
166. Qamri Z, Pelletier R, Foster J, Kumar S, Momani H, Ware K, et al. Early posttransplant changes in circulating endothelial microparticles in patients with kidney transplantation. Transpl Immunol (2014) 31(2):60–4. doi: 10.1016/j.trim.2014.06.006
167. Sigdel TK, Bestard O, Tran TQ, Hsieh SC, Roedder S, Damm I, et al. A computational gene expression score for predicting immune injury in renal allografts. PloS One (2015) 10(9):e0138133. doi: 10.1371/journal.pone.0138133
168. Zhang H, Huang E, Kahwaji J, Nast CC, Li P, Mirocha J, et al. Plasma exosomes from HLA-sensitized kidney transplant recipients contain mRNA transcripts which predict development of antibody-mediated rejection. Transplantation (2017) 101(10):2419–28. doi: 10.1097/TP.0000000000001834
169. Lim JH, Lee CH, Kim KY, Jung HY, Choi JY, Cho JH, et al. Novel urinary exosomal biomarkers of acute T cell-mediated rejection in kidney transplant recipients: A cross-sectional study. PloS One (2018) 13(9):e0204204. doi: 10.1371/journal.pone.0204204
170. Freitas RCC, Bortolin RH, Genvigir FDV, Bonezi V, Hirata TDC, Felipe CR, et al. Differentially expressed urinary exo-miRs and clinical outcomes in kidney recipients on short-term tacrolimus therapy: a pilot study. Epigenomics (2020) 12(22):2019–34. doi: 10.2217/epi-2020-0160
Keywords: chemokine, donor-derived cell-free DNA, exosomes, extracellular vesicles, MicroRNAs, molecular immune monitoring, nucleosome, transcriptomics
Citation: Chancharoenthana W, Traitanon O, Leelahavanichkul A and Tasanarong A (2023) Molecular immune monitoring in kidney transplant rejection: a state-of-the-art review. Front. Immunol. 14:1206929. doi: 10.3389/fimmu.2023.1206929
Received: 16 April 2023; Accepted: 31 July 2023;
Published: 22 August 2023.
Edited by:
Long Zheng, The Second Affiliated Hospital of Zhejiang University School of Medicine, ChinaReviewed by:
Olga Millán, Carlos III Health Institute (ISCIII), SpainEnver Akalin, Montefiore Medical Center, United States
Copyright © 2023 Chancharoenthana, Traitanon, Leelahavanichkul and Tasanarong. This is an open-access article distributed under the terms of the Creative Commons Attribution License (CC BY). The use, distribution or reproduction in other forums is permitted, provided the original author(s) and the copyright owner(s) are credited and that the original publication in this journal is cited, in accordance with accepted academic practice. No use, distribution or reproduction is permitted which does not comply with these terms.
*Correspondence: Wiwat Chancharoenthana, d2l3YXQuY2hhQG1haGlkb2wuYWMudGg=; Asada Leelahavanichkul, YWxlZWxhaGF2YW5pdEBnbWFpbC5jb20=