- Epidemiology, Public Health and Impact Unit, International Vaccine Institute, Seoul, South Korea
The importance of vaccines in combatting antimicrobial resistance (AMR) is commonly accepted. Although scientific reasoning supports the putative connection between vaccines and reduction of AMR, reliably measuring the magnitude and effect of vaccines on antimicrobial resistance is inherently challenging, especially in low resource settings. We review the intrinsic challenges in estimating the effect of vaccines on AMR and discuss the limitations and opportunities in current methods from the host, pathogen, and environment perspectives. We highlight advantages and pitfalls in different epidemiological study designs with a specific focus on impact studies in low resource settings and suggest how these perspectives could be considered in future research.
Introduction
Antimicrobial resistance (AMR) is a serious public health threat with over 700,000 people worldwide succumbing to resistant infections per year (1). Low- and middle- income countries (LMICs) are facing an unequal impact due to higher infectious disease burden of resistance prone pathogens (2), lower vaccine coverage (3), and higher rates of inappropriate use of antibiotics (4, 5).
The severity of the global threat of AMR has resulted in high level concerted efforts including the creation of a Global Action Plan in 2015 (6) with strategic directions for how to combat AMR, and an action framework envisioned in 2020 for leveraging vaccines to reduce antimicrobial use (AMU) and prevent AMR (7), which complements the global immunization strategy – Immunization Agenda 2030 (8). Given the important role of vaccines in infection prevention it has repeatedly been highlighted as one of the key tools to combatting AMR (7, 9, 10). However, lack in funding for development of new vaccines and barriers to access for existing vaccines have recently been highlighted as critical gaps in the continued AMR response over the next 5 to 10 years (1).
Despite the widely publicized importance of vaccines in fighting AMR, as illustrated by the multitude of scientific perspectives and narrative review pieces published in recent years (1, 7, 9–20), the actual research evidence is sparse, and not yet well established in LMICs. This is in large part because measuring the magnitude and direct effect of vaccines on AMR is inherently challenging and a number of these challenges even more difficult in low resource settings.
In this paper, we review the intrinsic challenges in estimating the effect of vaccines on AMR and discuss the limitations and opportunities in currently applied methods from the host, pathogen, and environment perspectives (Figure 1). We briefly describe which vaccines can contribute to combat AMR and how AMR and/or relevant proxies can be measured. We highlight advantages and pitfalls in different epidemiological study designs with a specific focus on impact studies in low resource settings and suggest how these perspectives could be considered in future research.
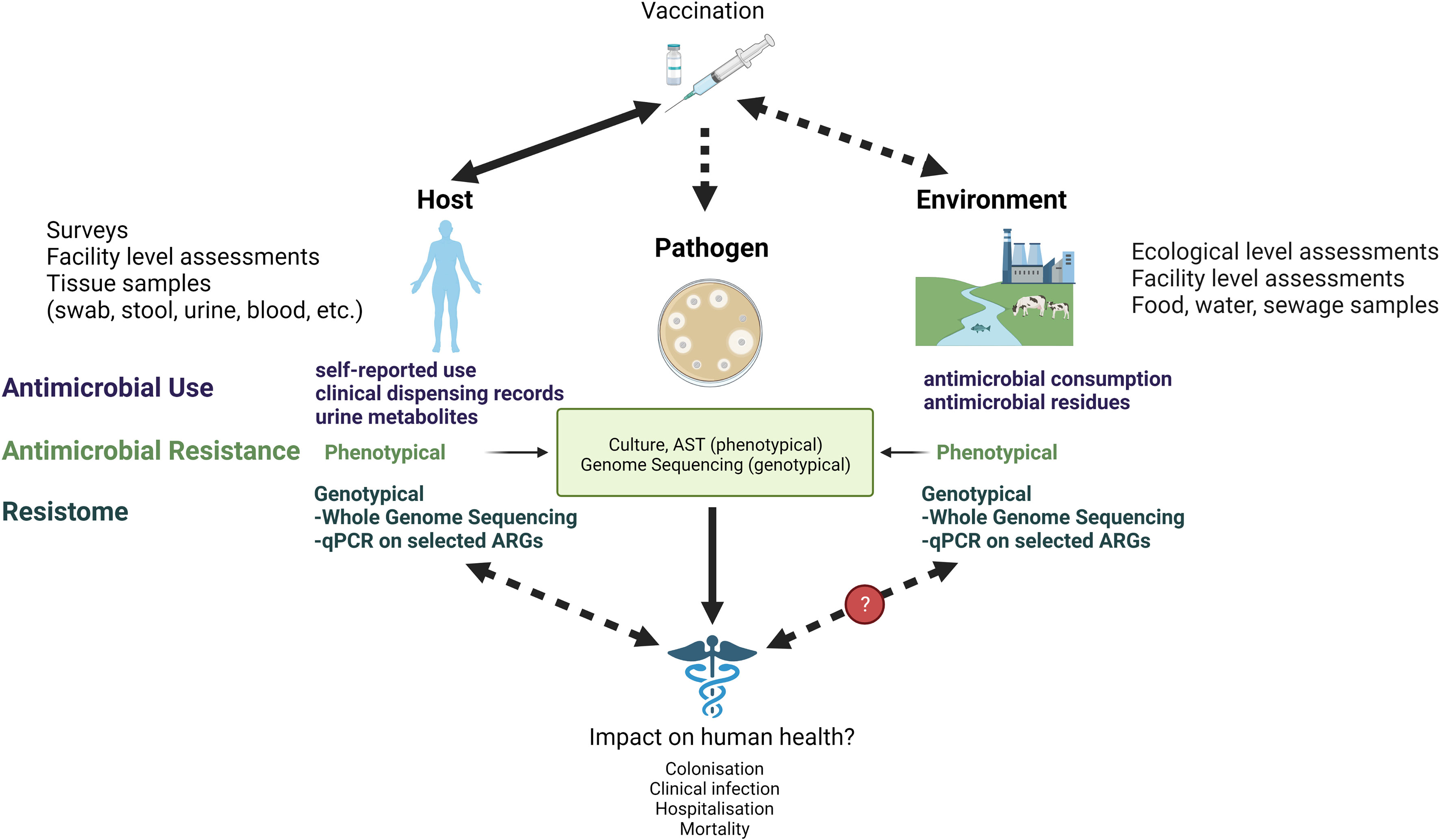
Figure 1 Measuring the link between vaccines and AMR at the host, pathogen, and environmental level. AST, Antimicrobial Susceptibility Testing; qPCR, quantitative polymerase chain reaction; ARG, Antimicrobial Resistance Genes.
Vaccines Against AMR
Vaccines are commonly considered to impact AMR either directly via preventing infection thereby reducing the risk of drug resistant infection and/or pathogen carriage, or through an indirect effect by preventing non-bacterial primary infections (e.g., viral), which are often wrongly treated with antibiotics. This indirect effect, in turn, reduces antibiotic misuse (21), which is considered an important driver of AMR (22) and/or prevents secondary (potentially drug resistant) bacterial co-infections.
There are four main categories of vaccines that may each prevent AMR in different ways:
Firstly, vaccines against bacterial pathogens causing (previously common) community acquired infections including diphtheria, tetanus, pertussis, Haemophilus influenzae type B (Hib) and Streptococcus pneumoniae and for which vaccines are currently administered as part of existing immunization programs have markedly reduced the incidence of infectious disease as well as the need for antimicrobial treatment (11). Within this category of vaccines, the direct association between vaccine and AMR reduction and associated medical complications has been best illustrated for Pneumococcal Conjugate Vaccine (PCV) and Hib vaccines (23–27).
Currently available licensed vaccines targeting the World Health Organization published list of “AMR priority bacterial pathogens” (2) are the pneumococcal conjugate vaccine (PCV), the Haemophilus influenzae type b (Hib) vaccine and the typhoid conjugate vaccine (TCV) (13).
Secondly, there are vaccines that prevent viral infections, which are often mistakenly treated with antibiotics. These vaccines therefore reduce misuse of antimicrobials as well as potential secondary bacterial co-infections that can arise after some viral infections (e.g., influenza, SARS-CoV-2). Examples of existing vaccines with illustrated effect on antimicrobial use are those against rotavirus (28) and influenza (29–31). Early evidence also suggests a substantial overprescribing of antibiotics in association with hospitalized cases of COVID-19 not justified by the risk of bacterial co-infection (32).
Thirdly, vaccines to prevent hospital-acquired infections with (often already resistant) pathogens such as Acinetobacter spp, Staphylococcus aureus, and Pseudomonas aeruginosa. Such infections are associated with considerable treatment challenges, hospitalization cost and high fatality (33) and are listed as either high (vancomycin resistant) or even critical (carbapenem resistant) on the WHO AMR priority pathogen list (34). Unfortunately, development of vaccines for these have so far been limited or unsuccessful and there are currently no licensed vaccines (12, 13). Considering how vaccines are perhaps the most crucial and final option against pathogens that have already acquired complete resistance (14) this is truly an urgent research and development need (10).
Finally, vaccines to prevent infections in animals may reduce the risk of emergence of resistance and reduce the need for use of antibiotics in agriculture including the incentive for using antimicrobials as growth promotors (35), which accounts for the largest proportion of global consumption (36) and considered a major driver of AMR (22). A successful, early example has been the introduction of furunculosis vaccines in salmon aquaculture in Norway (37).
Measuring the Link Between Vaccination and AMR Reduction
The prerequisite to demonstrate a link between vaccination and AMR is a solid way to measure and/or characterize “antimicrobial resistance”. This can be approached from the pathogen angle by measuring genotypic or phenotypic resistance in microorganisms, at the population or individual level by quantifying antimicrobial use, or by measuring the antimicrobial resistome in individuals or in the environment. These three complementary approaches are outlined below.
Measuring Antimicrobial Resistance
The traditional way to measure AMR is via classic bacteriology with isolation, pathogen identification and antimicrobial susceptibility testing (AST), and although methods have been standardized, it involves complex steps, is labor intensive, time consuming, and requires technical expertise to interpret results consistently. Such phenotypic testing has been the mainstay in clinical bacteriology for almost a century but is gradually being replaced by simplified and/or automated systems (38).
New and more advanced methods include molecular methods such as PCR, DNA microarray, whole genome sequencing (WGS), metagenomics and genotypical testing for resistance genes. These are often faster and more sensitive and can also be used for surveillance of AMR (39) but the limitation is that the presence of resistance genes is not necessarily correlated with resistant phenotype and these methods can only test for already known resistance genes. Also, the cost and expertise for application of these methods make them less accessible in low resource settings. Therefore, phenotypic methods of AST often remain the detection method of choice. However, it is a slow diagnostic and because sampling is often not possible/done in clinical settings, empirical antibiotic treatment based on symptoms is the most common practice leading to potential antimicrobial misuse and overuse (40).
In recent years there has also been a focus on developing more rapid and/or point-of care diagnostics for AMR, however, despite a busy pipeline very few tools have made it to clinical testing and use (41).
Obtaining reliable measures of AMR is also substantially challenged by inconsistent or incomplete sampling practices (42) as well as inadequate quality bacteriology diagnostics that do not meet accepted quality standards, a particular issue in low resource settings (43). Collection of an appropriate clinical sample for microbiological culture depends upon infection site and can be difficult to correctly obtain and process without access to highly skilled health professionals (e.g., cerebrospinal fluid, deep seated abscesses). Additionally, samples like sputum and stool pose extra challenges in identifying and differentiating pathogens from commensal flora, thereby demanding advanced technical expertise for interpretation of culture results. Blood cultures are indicated in suspected bloodstream infection and in most cases only one organism is isolated. This makes the laboratory process involved in blood culture straightforward to differentiate between pathogen and contaminant and therefore WHO has also recognized it as the priority specimen for surveillance of AMR (44). Although blood culture is considered the gold standard to measure blood borne infections it is not always reliable or feasible in limited resource settings where quality and operational challenges such as contamination, limited availability of culture bottles, electricity and hence temperature control, and human capacity constraints are common (45). Thereby, manual blood culture systems are most frequently used in LMICs while automated systems are currently standard in high-income countries (44).
Hence sufficient resources, standardized methods and quality assured clinical sampling and laboratory practices are important elements to ensure validity of bacteriology data when assessing the impact of vaccines on AMR.
In terms of quality assurance, although ISO (International Organization for Standardization) accreditation may not be feasible, and may not be required for research study laboratories, demonstration of technical and operational competence compatible with applicable local regulations can help improve the reliability of bacteriology data to assess changes in AMR patterns during vaccination campaigns and trials. Adherence to certain universally adapted quality guidelines and standards for antimicrobial susceptibility testing (AST) such as Clinical Laboratory Standard Institute (CLSI) and European Committee on Antimicrobial Susceptibility Testing (EUCAST) can help ensure this. The MICRO (Microbiology Investigation Criteria for Reporting Objectively) checklist is another welcome tool that can be used to ensure data quality when planning new studies (46).
Ultimately, assessing the presence and clinical significance of AMR relies on obtaining reliable information on vaccination status, clinical outcomes, and quality assured genotypic and phenotypic bacteriology data.
Measuring Antimicrobial Use
Antimicrobial use (AMU) is seen as one of the main drivers of AMR (22, 36) and certainly one of the most common proxy outcome measures used to study the potential impact of vaccines in reducing AMR (30, 31).
Measuring antimicrobial treatment can be done at the population level, which is often referred to as antimicrobial consumption (AMC) and can be assessed either at the most macro level using data sources such as import, production and export data or even national distribution records from regulatory authorities (47). Consumption can be assessed with more granularity when it captures information on procurement or supply at district or even health facility level. As such, consumption data is not able to distinguish treatment prescribed to individuals and hence these measurements in the association between vaccines and AMU are mostly relevant to ecological studies that consider correlations between trends in consumption in populations before and after introduction of vaccines.
An important caveat to keep in mind when considering population level assessments in LMICs is that national aggregate numbers may mask important local disparities in access, overuse and/or misuse (48, 49).
Nevertheless, population level measures of consumption can be useful in measuring the impact of newly introduced national vaccination campaigns on antimicrobial prescriptions among the target groups for vaccination.
Another way to use national data to obtain more granularity in the assessment is using standard national demographic and health surveys. A recent study used data from national household surveys across 77 countries (covering 944,173 children) between 2006 and 2018 to estimate the protection against antibiotic-treated episodes of acute respiratory infection conferred by selected vaccines (28). The study estimated that under the current global coverage levels rotavirus vaccines prevent 13.6 million episodes of antibiotic-treated diarrhea illness in children under 2 years of age, and pneumococcal vaccines prevent 23.8 million episodes of antibiotic-treated respiratory illness among children under five years of age in LMICs each year. Keeping in mind that the study relied on survey data on mother-reported disease outcomes and antibiotic use that has been criticized for yielding incomplete information of antibiotic treatment rates, especially in children with pneumonia (50), it still represents a significant step forward towards individual level assessments of the association between vaccines and AMU in low resource settings.
The findings from this study are also supported by similar observed trends illustrated at the individual level in high income settings where introduction of PCV correlated with reversing an otherwise steady increase in antibiotic prescriptions among children (25). However, this type of linkage and assessments of both vaccination status, illness episodes and antibiotic prescriptions at the individual level are currently only possible in very few high income countries with highly developed universal health care systems with long-standing and complete routine registration of health events (51). Therefore, using national standardized health surveys remains the most feasible choice elsewhere. Several publications provide guidance on how to ensure proper validation of assessments of respiratory infections (52) and diarrheal infections and treatment coverage among children in LMICs (53), which can be applied when designing individual level studies of vaccination and AMU in these settings.
Finally, the potential impact of antimicrobial use on the risk of environmental spread of AMR can be assessed by measuring antimicrobial residues in the environment and food samples. Although pollution with antibiotic residues is considered a growing area of concern across countries undergoing economic development where both production and use of antibiotics is substantial (54), data to determine the risk of residues in the environment are very limited and the link to development and transmission of antimicrobial resistance genes (ARG) only theoretical (55). Similarly, the implication of presence of ARGs in the environment on human health outcomes is also still largely unknown (56) and hence the measurement of this parameter not immediately applicable in vaccine studies.
Measuring antimicrobial use at the individual level can be done using data sources such as prescription data and/or records of dispensed antibiotics to individual patients, which ideally includes both patient- and clinical information on diagnoses and health outcomes (57). Such granular assessments of AMU are difficult due to complexities in considering multiple system levels and data sources. Therefore, gathering this very detailed level of information is challenging in most healthcare settings and often not obtained in standard surveys/surveillance.
Currently, the most feasible methods in use to asses antimicrobial treatment at the individual level in LMICs include hospital-based assessments such as point prevalence surveys (58), (hospital) pharmacy sales data (59, 60) and cross sectional survey data often available through standard national health surveys (such as Multiple Indicator Cluster Surveys and Demographic and Health Surveys) (48), however as mentioned above the latter ones may not catch the sufficient level of information and may also be associated with substantial recall bias.
Even if designing and conducting separate surveys to capture the relevant information directly as part of a vaccine introduction study, the limitation of self-reporting in community-based assessments in antimicrobial use remains and should therefore include validation measurements in urine (61).
The best way to assess AMU also requires careful consideration of setting and (vaccinated) population. There is large heterogeneity in antibiotic consumption across and within countries (5). Choosing settings where there is already a high level of misuse, and preferably also a high variation in use, would yield the highest statistical power to conduct studies on the effect of vaccine introduction on AMU. Triangulation of different data sources may also present an opportunity to ensure covering information on use including self-medication and medicine procured from unregulated outlets (62).
Finally, the methodological challenges of assessing AMU among children adds further uncertainty to the measurements between the currently available vaccines against priority pathogens and AMU as these are all administered primarily in very young children. Applying the Access, Watch, Reserve (AwaRe) categorization (63) rather than standard Anatomical Therapeutic Chemical (ATC) and Defined Daily Doses (DDD) methodology (64), which does not capture use in children well, represents a relevant alternative (5, 65).
Although AMU remains one of the most feasible ways to measure the potential (in-direct) impact of vaccines on AMR, the association can be difficult to reliably assess and is also subject to influences from various other parameters that need to be considered in parallel.
Measuring Antimicrobial Resistome
Measuring AMR has traditionally relied on assessing phenotypic resistance of clinically relevant bacteria in symptomatic patients visiting a health-care facility (66). Recently, measuring the resistome in humans, animals and/or the environment as a proxy for AMR has emerged as an option for population-based studies due to its putative link to resistance spread.
The resistome represents the collection of all antimicrobial resistance genes (ARGs) present in a certain ecological niche, in particular where microbial communities exist. Example of such niches are soil (often seen as the source of many ARGs), rivers (important for dissemination), wastewater (hotspot for proliferation of bacteria and ARGs, interface between humans and the environment), sites of agriculture/aquaculture (important exchange route for ARGs from human and animals to the environment, and back to human) or the gut microbiota of animals and humans (67). The human and animal gut resistomes are interim reservoirs, which contribute to ARGs dissemination and spread to the environment (68, 69) between individuals, and between bacteria within a single individual.
At the individual level, unequivocally linking the presence of a particular ARG in the human gut resistome to a bacteria species, a resistant phenotype or a clinical presentation is not straightforward. However, the possibility of horizontal ARGs transfer between bacteria, and in particular between commensal and pathogenic bacteria (70, 71), suggests that the resistome is associated with past and/or future spread of resistance (72). Therefore, defining the abundance and diversity of the resistome is often seen as a proxy for AMR in populations of interest, and could be an attractive readout for AMR in epidemiological and vaccine-studies.
Recent advances in culture-independent sequencing technologies have vastly improved the ability to detect ARGs and characterize the resistome of the gut microbiota using DNA from fecal samples (69, 72). Quantitative PCR and microarray hybridization have been widely used to screen for genes of interest and measure the presence, abundance and diversity of ARGs in the microbiota. However, these techniques give little clue about the genetic context, functionality, or host of the detected ARGs, and these methods can only detect known ARGs.
More recently, next generation sequencing methods have been applied to fully characterize the composition of the microbiota, and at the same time detect and quantify ARGs (70). However, ARG detection needs to be supported by downstream functional validation techniques such as functional metagenomics to better define the link between genotype (the presence of a gene) and phenotype (the realization of the resistance in the host bacteria). In addition, a deeper understanding of intra-population gene transfer is warranted, as the most worrisome event is the transfer of an ARG to a pathogenic bacterium.
The resistome can also be characterized in the environment, using molecular techniques similar to the ones applied to humans and animals by sampling sewage/wastewater including from well-defined outlets from particular buildings (73, 74). When an effect on AMR is expected in a defined population attached to a particular geographical area, measuring the wastewater resistome in a catchment area of interest (e.g., countries, states, neighborhoods, buildings, etc.) could provide valuable information in addition to other measures of AMR. Examples include ecological studies, impact assessment of interventions targeting defined geographical regions, cluster-randomized trials, or regional vaccine introductions. Longitudinal sampling could clarify the effect on AMR over time. In turn, understanding the link between the local environmental resistome and clinical presentation of bacterial infections in that same region should be an important research priority.
Due to both inter- and intra-individual variations, and difficulties in sample handling in general, which can challenge the accuracy, reliability and relevance of resistome ascertainment (75), individual samples may not be the ideal for resistome measurements. Therefore, pooling samples or sampling reservoirs representative of large populations and assessing differences in resistomes between populations (e.g., vaccinated and non-vaccinated) may be a more robust approach. Combining several sampling sources and modalities could be the answer.
While working with resistome data, it is important to keep in mind that not only pathogenic, but also commensal bacteria, harbor significant levels of ARGs. Delineating the clinically relevant ones and assessing their impact on health of individuals is difficult. However, it is safe to assume that higher prevalence of resistance plasmids in commensal bacteria increases the risk of horizontal transmission of resistance to pathogenic bacteria upon infection, and ultimately promotes the spread of AMR in pathogenic bacteria as well. Also, it is important to keep in mind that any effect on AMR might take time to be reflected in the resistome of a population/region, suggesting that realistic timelines must be defined to maximize the chance of capturing an effect on AMR by measuring the resistome of populations. Finally, as mentioned, the direct link between detection of ARGs in populations or the environment and impact of human health is uncertain (56). But if transmission is expected to ultimately impact health, it is likely to be more pronounced in places with poor sanitation and hygiene infrastructure and consequently more opportunities for contamination.
Despite the limitations outlined above, measuring the resistome is emerging as an attractive proxy measurement to complement other AMR or AMU measurement methods. This can be done in groups of individuals (such as intervention study arms) or catchment areas of interest during epidemiological studies (including pilot vaccine introduction or other vaccine effectiveness studies). The benefits of a non-invasive method (sample is stool), a simpler ethical framework (at least for sewage sampling), and the possibility of frequent measurements need to be balanced with the limitations such as data aggregation and the difficulty to link the presence of a gene to a pathogen or clinical syndrome.
Context and Study Design Considerations
In addition to the important methodological considerations for outcome measurements discussed above, contextual factors including setting, population, vaccine uptake and study design are also important to consider (76).
With regards to setting, it is important to consider the existing burden of infectious disease and the current prevalence of drug resistance. As such the priority settings to perform studies should be areas of relatively high prevalence of drug resistant infections where good epidemiological data are available. Further, not only detection of single pathogens but also clinical endpoints should be considered. Common clinical syndromes such as fever, gastroenteric-, urinary- and respiratory symptoms are non-specific and often of viral (or parasitological) etiology and hence most often associated with inappropriate antibiotic prescriptions (77) and/or self-medication, especially in LMICs (78).
Additional issues to pay special attention to when assessing health outcomes such as clinical syndrome and infection rates in LMICs is to ensure representativeness when healthcare coverage and utilization may challenge complete capture of cases. Appropriate prior census taking and ensuring sufficient follow up of the populations under study through comprehensive disease surveillance coverage is vital.
For all assessments, and for population level assessments in particular, it is also crucial to consider current vaccine deployment and coverage (3).
Several different epidemiological designs can be applied to assess the efficacy and effectiveness of vaccination on important outcome measures. Rarely will a vaccine study focus on AMR alone but rather this is often one among many parameters assessed as part of the overall vaccine evaluation. Different types of study designs hold different opportunities and limitations for the assessment of direct and indirect effects on AMR.
As an initial step, ecological studies can be valuable to generate hypotheses and compare rates of AMR prevalence and AMU between different regions with different vaccine coverage. However, such comparisons require robust and reliable AMR surveillance systems, which is currently lacking in most low resource settings (79).
The highest level of evidence from intervention studies are traditionally gained form randomized controlled trials. Randomization allows us to assume that all potential confounders such as AMU, non-targeted bacterial and viral infections, and other individual level risk factors, are randomly distributed and hence do not influence estimates. When new vaccines are introduced, this is often done through smaller individual efficacy trials and sometimes through larger cluster effectiveness trials. Although logistical and financial challenges mean we are only able to study limited number of participants, these randomized vaccine trials generate strong evidence since we can obtain more detailed and controlled measurements from individual participants and/or clusters. Including an antimicrobial readout (AMR, AMU and/or resistome) in vaccine trials of interest could generate additional evidence on the effect of vaccines on AMR. Which readout to include would depend on the trial design and setting. One caveat to be aware of will be the use of comparator vaccines, which might attenuate the association seen for non-pathogen specific AMR effects of the vaccine.
Assessing the impact of vaccines in non-randomized longitudinal intervention designs present several other advantages including ability to study larger populations, and in elimination campaigns also more complete immunization coverage, while allowing repeated host and environmental assessments both before, during and after vaccination. This design also has the added benefit of allowing longer follow up time, which is needed when assessing differences in AMR prevalence, which may take some time to appear and be measurable. The caveat here is that the longitudinal comparison assumes there are no other environmental, societal or disease burden changes that significantly affect the rates of resistant infections and presence of resistance markers in the environment or population of interest during the observation time. This might often be very difficult to ascertain in developing regions where other interventions and/or general improvements in livings standards normally would be associated with improved sanitation and hygiene, advances in health care quality and infection control. Measurements of such potential confounding parameters at both the individual and contextual levels would therefore be important to include so they can subsequently be considered in multilevel adjustment models estimating effect measures.
Cluster-randomized trials represent a particularly interesting opportunity, because most parameters, secular trends and living conditions can be assumed to be constant across the whole study region. In theory, only vaccination status would vary between clusters, limiting the risk of confounding. In addition, despite some unavoidable inter-cluster mobility, one can assume that the population of each cluster spends most of the time in their respective cluster, offering the possibility to triangulate multiple readouts such as AMR in pathogens, AMU for population and resistome for population and/or environment in each cluster. This multi-pronged approach (pathogen, population, geographic area) may generate more credible data on AMR and increase the likelihood to detect vaccine-associated changes in resistance patterns.
Choosing the appropriate population and vaccine within settings with well-defined resistance and disease burden and vaccine coverage patterns, while ensuring multiple different types and levels of outcome measurements that allows triangulation and cross validation of the different assessments, will allow for more scientifically rigorous assessments while improving the reliability of individual methods. Coupled with applying different design modalities across different settings would further minimize the influences of the biases inherent in individual ascertainment methods and study designs.
Finally, after quantifying the impact of vaccine introductions on both short and long-term resistance-related health outcomes, measuring the associated health sector as well as broader socioeconomic costs of these and comparing this to other non-vaccine interventions will be a crucial element to ascertaining the cost effectiveness and societal benefits of vaccines in prevention of AMR (80).
Conclusion
As highlighted in this paper, there are multiple reasons why the evidence base for the association between vaccines and AMR is still very limited including limited availability of vaccine candidates that target priority pathogens, and technical challenges in proper assessment and measurements of both direct effects on reduction of drug resistant infection rates as well as indirect effects on drug use and presence of resistance genes in both host and environment. The described systemic obstacles in low resource settings add further complexities.
However, we argue that carefully considering these challenges and taking a holistic approach in study design by including multiple assessments of several of these parameters simultaneously, while also carefully considering the relevant setting and design, will allow studies to further build the evidence base for the employment of vaccines as a crucial tool in combatting AMR.
Author Contributions
MH and RZ conceived the original concept of the article. MH, RZ, NP, KS, and HJ contributed to writing and generating graphical content to the manuscript. All authors provided critical revisions of the manuscript for important intellectual content. All authors read and approved the final version of this manuscript.
Funding
The Fleming Fund is a £265 million UK aid investment to tackle antimicrobial resistance in low- and middle-income countries around the world. The program is managed by the UK Department of Health and Social Care, in partnership with Mott MacDonald, the Fleming Fund Grants Management Agent. This work was supported by the Fleming Fund Regional Grants (grant numbers FF10-135, FF11-139, and FF-64). RZ is partly supported by a grant from the Bill & Melinda Gates Foundation (INV-029287).
Conflict of Interest
The authors declare that the research was conducted in the absence of any commercial or financial relationships that could be construed as a potential conflict of interest.
Publisher’s Note
All claims expressed in this article are solely those of the authors and do not necessarily represent those of their affiliated organizations, or those of the publisher, the editors and the reviewers. Any product that may be evaluated in this article, or claim that may be made by its manufacturer, is not guaranteed or endorsed by the publisher.
References
1. Wellcome Trust. The Global Response to AMR: Momentum, Success, and Critical Gaps. 2020 November. (2020), Report No.
2. (WHO) World Health Organisation. Prioritization of Pathogens to Guide Discovery, Research and Development of New Antibiotics for Drug-Resistant Bacterial Infections, Including Tuberculosis. Geneva: World Health Organization; 2017(WHO/EMP/IAU/2017.12). (Licence: CC BY-NC-SA 3.0 IGO. Geneva: World Health Organization (2017).
3. International Vaccine Access Center (IVAC). VIEW-Hub Report: Global Vaccine Introduction and Implementation. Baltimore: Johns Hopkins Bloomberg School of Public Health (2019). Available at: https://www.jhsph.edu/ivac/wp-content/uploads/2019/05/VIEW-hub_Report_Mar2019.pdf.
4. Klein EY, Milkowska-Shibata M, Tseng KK, Sharland M, Gandra S, Pulcini C, et al. Assessment of WHO Antibiotic Consumption and Access Targets in 76 Countries, 2000-15: An Analysis of Pharmaceutical Sales Data. Lancet Infect Dis (2021) 21(1):107–15. doi: 10.1016/S1473-3099(20)30332-7
5. Knowles R, Sharland M, Hsia Y, Magrini N, Moja L, Siyam A, et al. Measuring Antibiotic Availability and Use in 20 Low- and Middle-Income Countries. Bull World Health Organ (2020) 98(3):177–87C. doi: 10.2471/BLT.19.241349
6. (WHO) World Health Organisation. Global Action Plan on Antimicrobial Resistance. Geneva: WHO (2015).
7. World Health Organisation (WHO). Leveraging Vaccines to Reduce Antibiotic Use and Prevent Antimicrobial Resistance: An Action Framework: World Health; 2020. Licence: CC BY-NC-SA 3.0 IGO. Geneva: WHO (2020).
8. World Health Organisation. Immunization Agenda 2030: A Global Strategy To Leave No One Behind. Geneva: WHO (2020).
9. O’Neill J. The Review on Antimicrobial Resistance. Tackling Drug-Resistant Infections Globally: Final Report and Recommendations. (2016).
10. Wellcome Trust & BCG. Vaccines to Tackle Drug Resistant Infections. An Evaluation of R&D Opportunities. (2018).
11. Rahman-Shepherd A, Shaikh N, Dar O, Heyman DL. Establishing the Importance of Human and Animal Vaccines in Preventing Antimicrobial Resistance (2017). Available at: http://resistancecontrol.info/2018-frontpage/2018-3/establishing-the-importance-of-human-and-animal-vaccines-in-preventing-antimicrobial-resistance/.
12. Micoli F, Bagnoli F, Rappuoli R, Serruto D. The Role of Vaccines in Combatting Antimicrobial Resistance. Nat Rev Microbiol (2021) 19(5):287–302. doi: 10.1038/s41579-020-00506-3
13. Vekemans J, Hasso-Agopsowicz M, Kang G, Hausdorff WP, Fiore A, Tayler E, et al. Leveraging Vaccines to Reduce Antibiotic Use and Prevent Antimicrobial Resistance: A WHO Action Framework. Clin Infect Dis (2021) 73(4):e1011–7. doi: 10.1093/cid/ciab062
14. Clift C, Salisbury DM. Enhancing the Role of Vaccines in Combatting Antimicrobial Resistance. Vaccine. (2017) 35(48 Pt B):6591–3. doi: 10.1016/j.vaccine.2017.09.053
15. Buchy P, Ascioglu S, Buisson Y, Datta S, Nissen M, Tambyah PA, et al. Impact of Vaccines on Antimicrobial Resistance. Int J Infect Dis (2020) 90:188–96. doi: 10.1016/j.ijid.2019.10.005
16. Lipsitch M, Siber GR. How Can Vaccines Contribute to Solving the Antimicrobial Resistance Problem? mBio. (2016) 7(3):1–8. doi: 10.1128/mBio.00428-16
17. Bloom DE, Black S, Salisbury D, Rappuoli R. Antimicrobial Resistance and the Role of Vaccines. Proc Natl Acad Sci USA (2018) 115(51):12868–71. doi: 10.1073/pnas.1717157115
18. Jansen KU, Anderson AS. The Role of Vaccines in Fighting Antimicrobial Resistance (AMR). Hum Vaccin Immunother (2018) 14(9):2142–9. doi: 10.1080/21645515.2018.1476814
19. Jit M, Cooper B. The Role of Vaccines in Combating Antimicrobial Resistance. Challenges to Tackling Antimicrobial Resistance. Proc Natl Acad Sci USA (2020) 115:181–206. doi: 10.1017/9781108864121.009
20. Rosini R, Nicchi S, Pizza M, Rappuoli R. Vaccines Against Antimicrobial Resistance. Front Immunol (2020) 11:1048. doi: 10.3389/fimmu.2020.01048
21. Klugman KP, Black S. Impact of Existing Vaccines in Reducing Antibiotic Resistance: Primary and Secondary Effects. Proc Natl Acad Sci USA (2018) 115(51):12896–901. doi: 10.1073/pnas.1721095115
22. Holmes AH, Moore LSP, Sundsfjord A, Steinbakk M, Regmi S, Karkey A, et al. Understanding the Mechanisms and Drivers of Antimicrobial Resistance. Lancet (2016) 387(10014):176–87. doi: 10.1016/S0140-6736(15)00473-0
23. Klugman KP, Madhi SA, Huebner RE, Kohberger R, Mbelle N, Pierce N. A Trial of a 9-Valent Pneumococcal Conjugate Vaccine in Children With and Those Without HIV Infection. N Engl J Med (2003) 349(14):1341–8. doi: 10.1056/NEJMoa035060
24. Adam HJ, Richardson SE, Jamieson FB, Rawte P, Low DE, Fisman DN. Changing Epidemiology of Invasive Haemophilus Influenzae in Ontario, Canada: Evidence for Herd Effects and Strain Replacement Due to Hib Vaccination. Vaccine (2010) 28(24):4073–8. doi: 10.1016/j.vaccine.2010.03.075
25. Howitz MF, Harboe ZB, Ingels H, Valentiner-Branth P, Molbak K, Djurhuus BD. A Nationwide Study on the Impact of Pneumococcal Conjugate Vaccination on Antibiotic Use and Ventilation Tube Insertion in Denmark 2000-2014. Vaccine (2017) 35(43):5858–63. doi: 10.1016/j.vaccine.2017.09.006
26. Tomczyk S, Lynfield R, Schaffner W, Reingold A, Miller L, Petit S, et al. Prevention of Antibiotic-Nonsusceptible Invasive Pneumococcal Disease With the 13-Valent Pneumococcal Conjugate Vaccine. Clin Infect Dis (2016) 62(9):1119–25. doi: 10.1093/cid/ciw067
27. von Gottberg A, de Gouveia L, Tempia S, Quan V, Meiring S, von Mollendorf C, et al. Effects of Vaccination on Invasive Pneumococcal Disease in South Africa. N Engl J Med (2014) 371(20):1889–99. doi: 10.1056/NEJMoa1401914
28. Lewnard JA, Lo NC, Arinaminpathy N, Frost I, Laxminarayan R. Childhood Vaccines and Antibiotic Use in Low- and Middle-Income Countries. Nature (2020) 581(7806):94–9. doi: 10.1038/s41586-020-2238-4
29. van Heuvel L, Caini S, Dückers M, Paget J. Influenza Vaccination and Antimicrobial Resistance: Strategic Recommendations. Nivel: Netherlands Institute for Health Services Research, Utrecht, The Netherlands (2021).
30. Buckley BS, Henschke N, Bergman H, Skidmore B, Klemm EJ, Villanueva G, et al. Impact of Vaccination on Antibiotic Usage: A Systematic Review and Meta-Analysis. Clin Microbiol Infect (2019) 25(10):1213–25. doi: 10.1016/j.cmi.2019.06.030
31. Doherty TM, Hausdorff WP, Kristinsson KG. Effect of Vaccination on the Use of Antimicrobial Agents: A Systematic Literature Review. Ann Med (2020) 52(6):283–99. doi: 10.1080/07853890.2020.1782460
32. Langford BJ, So M, Raybardhan S, Leung V, Soucy JR, Westwood D, et al. Antibiotic Prescribing in Patients With COVID-19: Rapid Review and Meta-Analysis. Clin Microbiol Infect (2021) 27(4):520–31. doi: 10.1016/j.cmi.2020.12.018
33. Cassini A, Högberg LD, Plachouras D, Quattrocchi A, Hoxha A, Simonsen GS, et al. Attributable Deaths and Disability-Adjusted Life-Years Caused by Infections With Antibiotic-Resistant Bacteria in the EU and the European Economic Area in 2015: A Population-Level Modelling Analysis. Lancet Infect Dis (2019) 19(1):56–66. doi: 10.1016/s1473-3099(18)30605-4
34. Tacconelli E, Carrara E, Savoldi A, Harbarth S, Mendelson M, Monnet DL, et al. Discovery, Research, and Development of New Antibiotics: The WHO Priority List of Antibiotic-Resistant Bacteria and Tuberculosis. Lancet Infect Dis (2018) 18(3):318–27. doi: 10.1016/s1473-3099(17)30753-3
35. Hoelzer K, Bielke L, Blake DP, Cox E, Cutting SM, Devriendt B, et al. Vaccines as Alternatives to Antibiotics for Food Producing Animals. Part 1: Challenges and Needs. Vet Res (2018) 49(1):64. doi: 10.1186/s13567-018-0560-8
36. Laxminarayan R, Duse A, Wattal C, Zaidi AKM, Wertheim HFL, Sumpradit N, et al. Antibiotic Resistance the Need for Global Solutions. Lancet Infect Dis (2013) 13(12):1057–98. doi: 10.1016/S1473-3099(13)70318-9
37. World Health Organisation Regional Office for Europe. Vaccinating Salmon: How Norway Avoids Antibiotics in Fish Farming (2015). Available at: https://www.euro.who.int/en/countries/norway/news2/news/2015/10/vaccinating-salmon-how-norway-avoids-antibiotics-in-fish-farming.
38. Tang Y, Stratton C. Advanced Techniques in Diagnostic Microbiology. United States: Springer Science (2006).
39. Anjum MF, Zankari E, Hasman H. Molecular Methods for Detection of Antimicrobial Resistance. Microbiol Spectr (2017) 5(6):1–17. doi: 10.1128/microbiolspec.ARBA-0011-2017
40. (WHO) World Health Organisation. Global Antimicrobial Resistance Surveillance System: Manual for Early Implementation. Geneva: WHO (2015).
41. Shanmugakani RK, Srinivasan B, Glesby MJ, Westblade LF, Cardenas WB, Raj T, et al. Current State of the Art in Rapid Diagnostics for Antimicrobial Resistance. Lab Chip (2020) 20(15):2607–25. doi: 10.1039/D0LC00034E
42. Skodvin B, Wathne JS, Lindemann PC, Harthug S, Nilsen RM, Charani E, et al. Use of Microbiology Tests in the Era of Increasing AMR Rates- a Multicentre Hospital Cohort Study. Antimicrob Resist Infect Control (2019) 8:28. doi: 10.1186/s13756-019-0480-z
43. Seale AC, Gordon NC, Islam J, Peacock SJ, Scott JAG. AMR Surveillance in Low and Middle-Income Settings - A Roadmap for Participation in the Global Antimicrobial Surveillance System (GLASS). Wellcome Open Res (2017) 2:92. doi: 10.12688/wellcomeopenres.12527.1
44. Ombelet S, Barbe B, Affolabi D, Ronat JB, Lompo P, Lunguya O, et al. Best Practices of Blood Cultures in Low- and Middle-Income Countries. Front Med (Lausanne) (2019) 6:131. doi: 10.3389/fmed.2019.00131
45. Dailey PJ, Osborn J, Ashley EA, Baron EJ, Dance DAB, Fusco D, et al. Defining System Requirements for Simplified Blood Culture to Enable Widespread Use in Resource-Limited Settings. Diagnostics (Basel) (2019) 9(1):1–12. doi: 10.3390/diagnostics9010010
46. Turner P, Fox-Lewis A, Shrestha P, Dance DAB, Wangrangsimakul T, Cusack TP, et al. Microbiology Investigation Criteria for Reporting Objectively (MICRO): A Framework for the Reporting and Interpretation of Clinical Microbiology Data. BMC Med (2019) 17(1):70. doi: 10.1186/s12916-019-1301-1
47. (WHO) World Health Organisation. WHO Methodology for a Global Programme on Surveillance of Antimicrobial Consumption V1.0. Available at: https://www.who.int/medicines/areas/rational_use/WHO_AMCsurveillance_1.0.pdf.
48. Sulis G, Gandra S. Access to Antibiotics: Not a Problem in Some LMICs. Lancet Global Health (2021) 9(5):e561–2. doi: 10.1016/S2214-109X(21)00085-1
49. Queenan K, Chandler CIR, Goodman C. Meeting Report: Metrics and Methods for Assessing Antibiotic Use at the Granular Level in Humans and Livestock in LMICs. London: London School of Hygiene & Tropical Medicine. (2017).
50. Campbell H, El Arifeen S, Hazir T, O’Kelly J, Bryce J, Rudan I, et al. Measuring Coverage in MNCH: Challenges in Monitoring the Proportion of Young Children With Pneumonia Who Receive Antibiotic Treatment. PloS Med (2013) 10(5):e1001421. doi: 10.1371/journal.pmed.1001421
51. Schmidt M, Schmidt SAJ, Adelborg K, Sundboll J, Laugesen K, Ehrenstein V, et al. The Danish Health Care System and Epidemiological Research: From Health Care Contacts to Database Records. Clin Epidemiol (2019) 11:563–91. doi: 10.2147/CLEP.S179083
52. Lanata CF, Rudan I, Boschi-Pinto C, Tomaskovic L, Cherian T, Weber M, et al. Methodological and Quality Issues in Epidemiological Studies of Acute Lower Respiratory Infections in Children in Developing Countries. Int J Epidemiol (2004) 33(6):1362–72. doi: 10.1093/ije/dyh229
53. Levine GA, Walson JL, Atlas HE, Lamberti LM, Pavlinac PB. Defining Pediatric Diarrhea in Low-Resource Settings. J Pediatr Infect Dis Soc (2017) 6(3):289–93. doi: 10.1093/jpids/pix024
54. Lundborg CS, Tamhankar AJ. Antibiotic Residues in the Environment of South East Asia. BMJ (2017) 358:j2440. doi: 10.1136/bmj.j2440
55. Ben Y, Fu C, Hu M, Liu L, Wong MH, Zheng C. Human Health Risk Assessment of Antibiotic Resistance Associated With Antibiotic Residues in the Environment: A Review. Environ Res (2019) 169:483–93. doi: 10.1016/j.envres.2018.11.040
56. Stanton IC, Bethel A, Leonard AFC, Gaze WH, Garside R. What is the Research Evidence for Antibiotic Resistance Exposure and Transmission to Humans From the Environment? A Systematic Map Protocol. Environ Evid (2020) 9(1):12. doi: 10.1186/s13750-020-00197-6
57. Leung V, Langford BJ, Ha R, Schwartz KL. Metrics for Evaluating Antibiotic Use and Prescribing in Outpatient Settings. JAC Antimicrob Resist (2021) 3(3):dlab098. doi: 10.1093/jacamr/dlab098
58. (WHO) World Health Organisation. WHO Methodology for Point Prevalence Survey on Antibiotic Use in Hospitals V1.1. Geneva: WHO (2018).
59. Klein EY, Van Boeckel TP, Martinez EM, Pant S, Gandra S, Levin SA, et al. Global Increase and Geographic Convergence in Antibiotic Consumption Between 2000 and 2015. Proc Natl Acad Sci USA (2018) 115(15):E3463–70. doi: 10.1073/pnas.1717295115
60. Li G, Jackson C, Bielicki J, Ellis S, Hsia Y, Sharland M. Global Sales of Oral Antibiotics Formulated for Children. Bull World Health Organ (2020) 98(7):458–66. doi: 10.2471/BLT.19.235309
61. Alexandrova L, Haque F, Rodriguez P, Marrazzo AC, Grembi JA, Ramachandran V, et al. Identification of Widespread Antibiotic Exposure in Patients With Cholera Correlates With Clinically Relevant Microbiota Changes. J Infect Dis (2019) 220(10):1655–66. doi: 10.1093/infdis/jiz299
62. Do NTT, Vu HTL, Nguyen CTK, Punpuing S, Khan WA, Gyapong M, et al. Community-Based Antibiotic Access and Use in Six Low-Income and Middle-Income Countries: A Mixed-Method Approach. Lancet Global Health (2021) 9(5):e610–9. doi: 10.1016/S2214-109X(21)00024-3
63. (WHO) World Health Organisation. WHO 2019 AWaRe Classification Antibiotics World Health Organisation (2019). Available at: https://www.who.int/medicines/news/2019/WHO_releases2019AWaRe_classification_antibiotics/en/.
64. WHO Collaborating Centre for Drug Statistics Methodology. Guidelines for ATC Classification and DDD Assignment 2020. Oslo: WHO (2019).
65. Hsia Y, Lee BR, Versporten A, Yang Y, Bielicki J, Jackson C, et al. Use of the WHO Access, Watch, and Reserve Classification to Define Patterns of Hospital Antibiotic Use (AWaRe): An Analysis of Paediatric Survey Data From 56 Countries. Lancet Global Health (2019) 7(7):e861–71. doi: 10.1016/s2214-109x(19)30071-3
66. (WHO) World Health Organisation. Antimicrobial Resistance: Global Report on Surveillance. Geneva: WHO. (2014).
67. Lim MY, Cho Y, Rho M. Diverse Distribution of Resistomes in the Human and Environmental Microbiomes. Curr Genomics (2018) 19(8):701–11. doi: 10.2174/1389202919666180911130845
68. Hu Y, Gao GF, Zhu B. The Antibiotic Resistome: Gene Flow in Environments, Animals and Human Beings. Front Med (2017) 11(2):161–8. doi: 10.1007/s11684-017-0531-x
69. Kim DW, Cha CJ. Antibiotic Resistome From the One-Health Perspective: Understanding and Controlling Antimicrobial Resistance Transmission. Exp Mol Med (2021) 53(3):301–9. doi: 10.1038/s12276-021-00569-z
70. van Schaik W. The Human Gut Resistome. Philos Trans R Soc Lond B Biol Sci (2015) 370(1670):20140087. doi: 10.1098/rstb.2014.0087
71. Penders J, Stobberingh EE, Savelkoul PH, Wolffs PF. The Human Microbiome as a Reservoir of Antimicrobial Resistance. Front Microbiol (2013) 4:87. doi: 10.3389/fmicb.2013.00087
72. Crofts TS, Gasparrini AJ, Dantas G. Next-Generation Approaches to Understand and Combat the Antibiotic Resistome. Nat Rev Microbiol (2017) 15(7):422–34. doi: 10.1038/nrmicro.2017.28
73. Brinch C, Leekitcharoenphon P, Duarte ASR, Svendsen CA, Jensen JD, Aarestrup FM, et al. Long-Term Temporal Stability of the Resistome in Sewage From Copenhagen. mSystems (2020) 5(5):e00841–20. doi: 10.1128/mSystems.00841-20
74. Nieuwenhuijse DF, Oude Munnink BB, Phan MVT, Global Sewage Surveillance project c, Munk P, Venkatakrishnan S, et al. Setting a Baseline for Global Urban Virome Surveillance in Sewage. Sci Rep (2020) 10(1):13748. doi: 10.1038/s41598-020-69869-0
75. Gorzelak MA, Gill SK, Tasnim N, Ahmadi-Vand Z, Jay M, Gibson DL. Methods for Improving Human Gut Microbiome Data by Reducing Variability Through Sample Processing and Storage of Stool. PloS One (2015) 10(8):e0134802. doi: 10.1371/journal.pone.0134802
76. Pollard AJ, Bijker EM. A Guide to Vaccinology: From Basic Principles to New Developments. Nat Rev Immunol (2021) 21(2):83–100. doi: 10.1038/s41577-020-00479-7
77. Fleming-Dutra KE, Hersh AL, Shapiro DJ, Bartoces M, Enns EA, File TM Jr, et al. Prevalence of Inappropriate Antibiotic Prescriptions Among US Ambulatory Care Visits, 2010-2011. JAMA (2016) 315(17):1864–73. doi: 10.1001/jama.2016.4151
78. Nepal G, Bhatta S. Self-Medication With Antibiotics in WHO Southeast Asian Region: A Systematic Review. Cureus (2018) 10(4):e2428. doi: 10.7759/cureus.2428
79. Ashley EA, Shetty N, Patel J, van Doorn R, Limmathurotsakul D, Feasey NA, et al. Harnessing Alternative Sources of Antimicrobial Resistance Data to Support Surveillance in Low-Resource Settings. J Antimicrob Chemother (2019) 74(3):541–6. doi: 10.1093/jac/dky487
Keywords: antimicrobial resistance (AMR), vaccines, low-resource settings, antimicrobial use (AMU), antimicrobial resistome
Citation: Holm M, Zellweger RM, Poudyal N, Smith KHT, Joh HS and Marks F (2022) Measuring the Link Between Vaccines and Antimicrobial Resistance in Low Resource Settings – Limitations and Opportunities in Direct and Indirect Assessments and Implications for Impact Studies. Front. Trop. Dis 3:805833. doi: 10.3389/fitd.2022.805833
Received: 31 October 2021; Accepted: 06 January 2022;
Published: 15 February 2022.
Edited by:
Alicia Ponte-Sucre, Central University of Venezuela, VenezuelaReviewed by:
Jyoti Joshi, Center for Disease Dynamics, Economics & Policy (CDDEP), IndiaCopyright © 2022 Holm, Zellweger, Poudyal, Smith, Joh and Marks. This is an open-access article distributed under the terms of the Creative Commons Attribution License (CC BY). The use, distribution or reproduction in other forums is permitted, provided the original author(s) and the copyright owner(s) are credited and that the original publication in this journal is cited, in accordance with accepted academic practice. No use, distribution or reproduction is permitted which does not comply with these terms.
*Correspondence: Marianne Holm, bWFyaWFubmUuaG9sbUBpdmkuaW50