- 1Divisions of Epidemiology and Biostatistics, School of Public Health, University of California, Berkeley, CA, United States
- 2Energy and Resources Group, University of California, Berkeley, CA, United States
- 3Berkeley Institute for Data Science, University of California, Berkeley, CA, United States
- 4Innovative Genomics Institute, University of California, Berkeley, CA, United States
Mosquito-borne diseases such as malaria continue to pose a major global health burden, and the impact of currently-available interventions is stagnating. Consequently, there is interest in novel tools to control these diseases, including gene drive-modified mosquitoes. As these tools continue to be refined, decisions on whether to implement them in the field depend on their alignment with target product profiles (TPPs) that define product characteristics required to achieve desired entomological and epidemiological outcomes. TPPs are increasingly being used for malaria and vector control interventions, such as attractive targeted sugar baits and long-acting injectable drugs, as they progress through the development pipeline. For mosquito gene drive products, reliable predictions from mathematical models are an essential part of these analyses, as field releases could potentially be irreversible. Here, we review the prior use of mathematical models in developing TPPs for malaria and vector control tools and discuss lessons from these analyses that may apply to mosquito gene drives. We recommend that, as gene drive technology gets closer to field release, discussions regarding target outcomes engage a wide range of stakeholders and account for settings of interest and vector species present. Given the relatively large number of parameters that describe gene drive products, machine learning approaches may be useful to explore parameter space, and an emphasis on conservative fitness estimates is advisable, given the difficulty of accurately measuring these parameters prior to field studies. Modeling may also help to inform the risk, remediation and cost dimensions of mosquito gene drive TPPs.
Introduction
Mosquito-borne diseases such as malaria, dengue and yellow fever continue to pose a major public health burden throughout much of the world. These diseases primarily impact low and middle-income countries, with over 90% of malaria cases and deaths occurring in sub-Saharan Africa (1). While malaria incidence in Africa has significantly declined since the wide-scale distribution of interventions beginning in 2000 (2), disease transmission is now stagnating at an unacceptably high level (1). In addition to increasing access to long-lasting insecticide-treated nets (LLINs) and other currently-available tools such as artemisinin combination therapy drugs (ACTs), it is clear that new tools will be needed to meet Global Technical Strategy milestones for reductions in malaria incidence and mortality, with two of the most promising novel tools currently being malaria vaccines and gene drive-modified mosquitoes. Gene drive approaches bias inheritance in favor of an introduced allele intended to spread through the mosquito population, and fall into two main categories: i) “population suppression,” whereby the introduced allele induces a fitness load or sex bias, reducing mosquito numbers, and ii) “population modification,” whereby the introduced allele disrupts pathogen transmission, reducing mosquito vector competence (3).
In order for gene drive mosquito products to advance from laboratory to field studies, their characteristics will be assessed against target product profiles (TPPs) - planning tools that provide a list of preferred characteristics and minimum criteria products must satisfy as they progress through the development pipeline. TPPs for gene drive mosquitoes are becoming increasingly relevant as the technology matures and moves closer to release. A draft TPP for a population modification gene drive product has been proposed (4), and a workshop hosted by the Foundation for the National Institutes of Health (FNIH) discussed TPPs for gene drive products at length (5). A common theme from these publications is that, while TPPs should be based as much as possible on empirical studies, rigorous modeling will be needed where empirical data is not available. Key outcomes for vector control tools are entomological (i.e., effects on mosquito populations) and epidemiological (i.e., effects to human health outcomes) and can only be observed following a release, meaning that the initial decision to release will be based on model predictions.
Fortunately, there has been a growth in malaria modeling over the last 10-15 years, with several detailed models being published that concisely describe malaria transmission dynamics in the mosquito vector and human host. These include OpenMalaria (6, 7) and the Imperial College London malaria model (8, 9). Over this same period, other novel malaria control tools have also been developed and advanced through stages of laboratory and field testing - most recently and visibly, attractive targeted sugar baits (ATSBs) (10) and malaria vaccines (11). These provide case studies for the application of mathematical models to TPPs, and we draw from these previous analyses to explore the role that mathematical models should play in informing TPPs for mosquito gene drive products.
Model-Informed Target Product Profiles for Other Malaria Control Tools
As the goals of TPPs are product-focused, much of the modeling work in this area does not feature in academic journals. We therefore focus on a handful of published modeling analyses, each conducted by a different research group, that have supported TPP specification for ATSBs (12), odor-baited traps (13), and long-acting injectable drugs (LAIs) for seasonal malaria prevention (14). We summarize these analyses, and additional TPP modeling analyses for malaria vaccines (15) and vector control pesticides (16, 17), in Table 1.
Lessons From Attractive Targeted Sugar Baits
ATSBs were proposed as an outdoor vector control strategy to complement existing indoor tools such as LLINs and indoor residual spraying with insecticides (IRS), and work by attracting mosquitoes to the fruity or flowery scent of a bait laced with a combination of sugar and an oral toxin (18). In 2008, a field study demonstrated that ATSBs were capable of reducing Anopheles gambiae mosquito populations by 90% in Bandiagara, a semi-arid area of Mali (19). Then in 2017, a randomized controlled trial (RCT) involving 14 villages in Mali demonstrated that ATSBs were capable of significantly reducing An. gambiae populations, including sporozoite-infected females, when LLINs were already present (10). Both studies had focused on entomological outcomes (i.e., mosquito density and sporozoite infection), and a mathematical model was used to estimate the expected epidemiological impact based on the RCT results (12) prior to planning epidemiological RCTs.
Predicting outcomes, especially epidemiological ones, based on product parameters embodies the primary role that mathematical models play in informing TPPs. For ATSBs, the key product parameter is the excess daily mosquito mortality rate caused by the intervention, which was estimated to be 0.09 per mosquito per day from the Mali RCT (12). In this study, the Imperial College London malaria model (8, 9) was used to predict all-ages malaria prevalence and clinical incidence following intervention with ATSBs in a range of transmission settings (baseline malaria prevalence ranging from 10-50% with varying degrees of seasonal transmission). This malaria model includes acquired and maternal human immunity, symptomatic and asymptomatic infection, human age structure, mosquito biting heterogeneity, and antimalarial drug therapy and prophylaxis (8). A detailed mosquito life history model is also included, incorporating vector control tools such as LLINs and IRS (20, 21). Predictions from this model suggest that the RCT-inferred excess mosquito mortality rate due to ATSBs should result in reductions in malaria prevalence exceeding 30% and reductions in clinical incidence exceeding 50% for the range of transmission settings considered (12).
This analysis addressed questions directly informative of a TPP for ATSBs. An excess mortality rate due to ATSBs of ~0.05 per mosquito per day or higher was predicted to result in a >30% reduction in clinical incidence in a range of settings, and an excess mortality rate of ~0.1 per mosquito per day or higher was found to result in a >30% reduction in malaria prevalence (12). Depending on the target outcome, either of these excess mortality rates could be considered a minimum criterion that would enable approval of an epidemiological field trial. An important caveat to note is that the excess mortality rate is expected to vary by environmental setting. For instance, in lush settings with an abundance of alternative sugar sources, the excess mortality rate due to ATSBs is expected to be lower. The key product parameter is therefore location-specific, and an environmental assay, such as the feeding rate on attractive sugar baits marked with dyes, would be needed to assess TPP alignment in a new location. That said; the analysis clearly demonstrates the strength of mathematical models in predicting target epidemiological outcomes for a given product parameter in a range of transmission settings.
Lessons From Odor-Baited Traps
Odor-baited traps have long been discussed as a form of vector control (22), although their use to date has been limited to mosquito monitoring (23). In 2010, a series of papers were published detailing odor-baited traps that are more attractive to mosquitoes than humans (24, 25), and a deterministic model describing mosquito host-seeking and ovipositing behavior (26) was adapted to model vector control with odor-baited traps in addition to LLINs (13). One of the motivations for this mathematical analysis was to inform a TPP for odor-baited traps, as a prototype version (25), while potentially effective, was considered too expensive for a community-scale trial. The modeling analysis considered the entomological inoculation rate (EIR) - the rate at which people are exposed to infectious mosquito bites - as the outcome of interest, and calibrated the model to a baseline EIR greater than 200 infective bites per person per year, representing locations in Africa where transmission is consistently high.
To produce a TPP for odor-baited traps, key intervention parameters were explored - the attractiveness of traps to mosquitoes, and the number of traps per 1000 people that would produce a reduction in EIR equivalent to that achieved by 50% coverage with LLINs (a level of coverage considered generally attainable throughout Africa). Given the number of traps required, a corresponding maximum cost per trap was calculated in order for the traps to be at least as cost-effective as LLINs. The analysis concluded that the traps should be more attractive than humans, and that 20-130 traps per 1000 people would be needed to produce the target EIR reduction. This equated to a maximum cost per trap of $4 to $27, which includes costs of production, transport, installation, operation and maintenance (13). An interesting point raised by this and many TPP analyses is that target product characteristics can be traded off against each other - i.e., a more attractive trap can afford to be more expensive as less traps are required. The analysis also emphasized the importance of a comparative cost analysis, and this was influential in preventing these odor-baited traps from being adopted at scale.
Lessons From Long-Acting Injectable Drugs for Seasonal Malaria Prevention
LAIs have been proposed as an alternative to monthly seasonal malaria chemoprevention (SMC) with oral anti-malarials in the hope that longer-lasting injectable drugs can remedy the spread of drug-resistance and the low adherence rates and high deployment costs of SMC (27). In order for LAIs to be implemented, they must be shown to be non-inferior to existing interventions, and in lieu of clinical studies, a preliminary modeling analysis was conducted (14). In this study, the OpenMalaria model (6, 7) was used to predict clinical malaria cases averted by LAIs compared to SMC in the intervention age group (children 0-5 years of age). Simulations were conducted for settings that resemble Mali and Senegal in terms of seasonality, mosquito species and interventions, with these two countries being chosen because they are locations where SMC is implemented and clinical trials are frequently conducted (14). The OpenMalaria model is an individual-based simulation of malaria in humans, including heterogeneity in mosquito biting, human immunity and disease susceptibility, and is linked to a deterministic model of mosquito life history (6, 7).
Key product parameters that were varied in this analysis describe initial LAI protective efficacy (i.e., the chance that a malaria case is prevented upon treatment with a LAI) and the shape and half-life of decay in protective efficacy. LAI coverage (i.e., the proportion of the intervention age group receiving the LAI at the beginning of a transmission season) was also varied. Results suggest that the shape of decay in protective efficacy is key, with protective efficacy profiles that remain high for an extended period (e.g. sigmoidal efficacy profiles) being essential to establish non-inferiority of LAIs. The required half-life of protective efficacy mirrored the duration of seasonal transmission in each setting, and a trade-off was observed between protective efficacy and coverage. Given the importance of decay in LAI protective efficacy, it was recommended that studying this phenomenon be prioritized in potential clinical studies (14). Another interesting aspect of this analysis was the use of machine learning to infer non-inferior tool profiles and parameter sensitivities based on a database of TPP malaria model simulations (28).
Special Considerations for Mosquito Gene Drive Products
For mosquito gene drive products, reliable predictions from mathematical models will be required to inform TPPs prior to the first field release, as any release could potentially be irreversible. This elevates the need for rigorous modeling analyses, as accurate predictions of safety and efficacy will be required in the absence of field testing. Here, we describe some of the special considerations that apply to mosquito gene drive products when developing model-informed TPPs, in particular concerning product parameters, target outcomes, biosafety and cost (Figure 1).
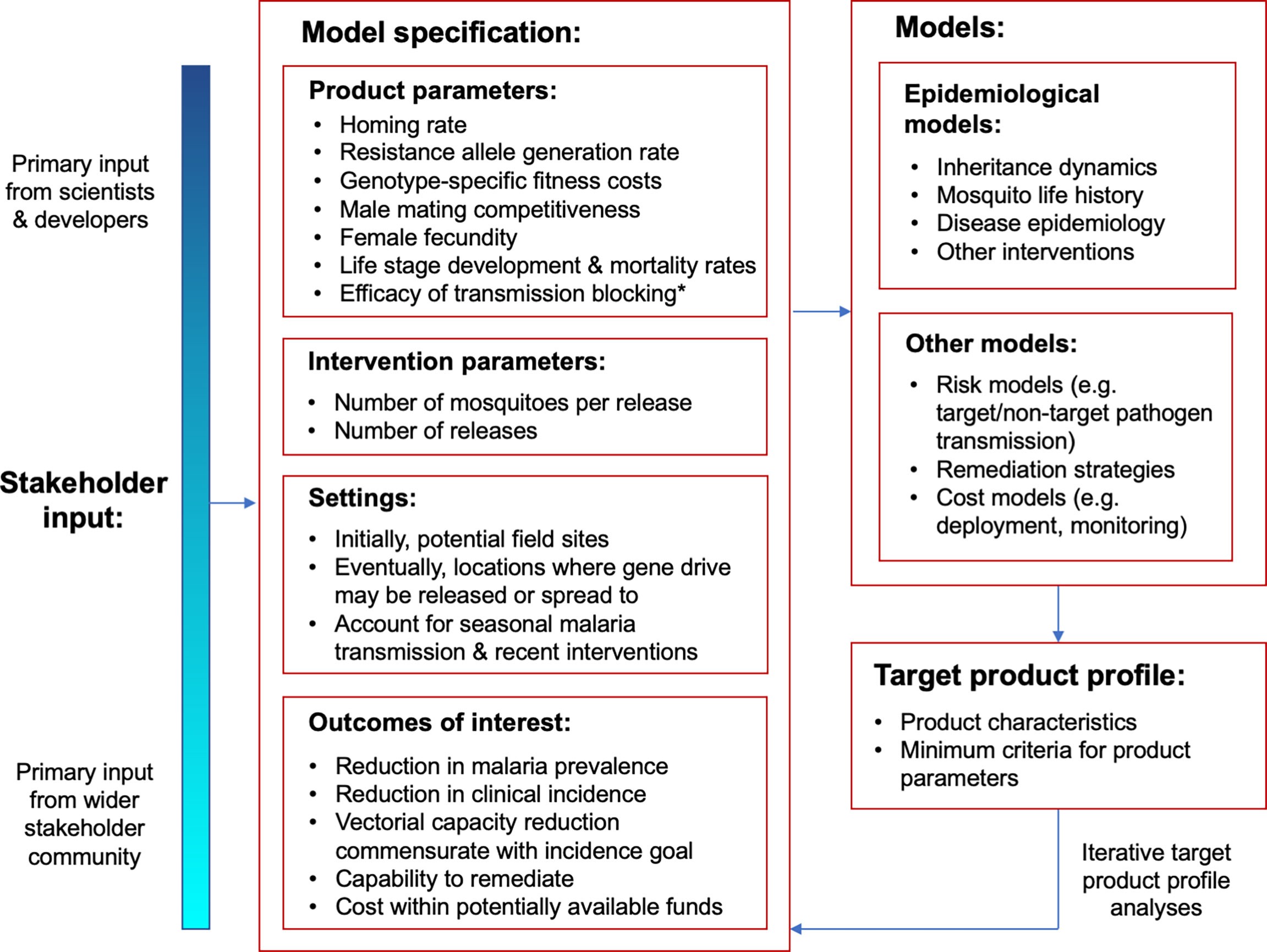
Figure 1 Schematic representation of the role of mathematical models in informing target product profiles (TPPs) for gene drive mosquitoes. Stakeholder input follows a gradient with scientists and developers providing primary input on product parameters and the wider stakeholder community providing primary input on outcomes of interest. The flowchart applies to both population modification and population suppression gene drive systems, although the product parameter for efficacy of transmission blocking* only applies to population modification systems.
Product Parameters
Gene drive mosquito products can be described by a large number of parameters. The FNIH workshop on TPPs, for instance, listed homing rate, rate of functional resistance allele generation, male mating competitiveness, female fecundity, and the development and mortality rates of each life stage all as important product parameters (5). Additionally, intervention parameters such as the number and size of releases require specification. In order to provide meaningful criteria for each parameter, consultation with molecular biologists and field ecologists will be essential to narrow the space of parameter exploration, and a machine learning approach will likely assist in exploring the refined parameter space, as it did for LAIs (28). Prior modeling studies can also help to refine parameter space (29). For example, the EMOD individual-based model of mosquito population dynamics (30) was used to determine the homing rate, resistance allele generation rate and female fecundity parameters required for persistent reduction of disease-competent mosquitoes in sub-Saharan African settings (31). Fitness parameters consistently emerge as highly influential on gene drive model outcomes, and given the inaccuracy of estimating these prior to a field release, a conservative approach would be to focus on their lower bounds when generating criteria for other parameters.
Target Outcomes
Ongoing discussions will likely be needed to define target outcomes for gene drive mosquito products. Epidemiological outcomes, such as reductions in clinical incidence and prevalence, are likely to be required by stakeholders such as the World Health Organization, with questions remaining over exactly what the target reduction should be. TPPs are blunt instruments, and any target reduction decided upon will likely represent a compromise between demonstrating significant public health benefit, and having an achievable goal that will enable the technology to progress along the product development pipeline. The precedent from the ATSB analysis is a 30% reduction in clinical malaria incidence or prevalence (12), while at the FNIH workshop on TPPs, a 20-50% reduction in clinical malaria incidence was discussed (5). Such target decisions should involve a wider range of stakeholders as the technology gets closer to field release, and should include consideration of national malaria control targets, and recent and current interventions used.
Key decisions also need to be made regarding the modeled transmission settings, as these will have a significant influence on TPP specification. The LAI analysis presents an interesting case study by selecting two locations where comparable interventions and field studies have been conducted (14). For gene drive mosquito projects, similar reasoning would support modeling population suppression products in Mali, Burkina Faso, Kenya or Uganda (field sites of the Target Malaria project), and population modification products in São Tomé and Príncipe or the Union of the Comoros (field sites of the UC Irvine Malaria Initiative). Models in these settings should take into account seasonal malaria transmission profiles alongside current interventions being implemented. If the scale of gene drive interventions grows, then TPPs should consider a representative range of settings, spanning a diversity of local vector ecologies, and malaria transmission and intervention profiles.
For settings with more than one malaria vector present, entomological outcomes may be more suitable than epidemiological ones. To illustrate this, an alternative malaria vector could hypothetically prevent elimination of a target vector species from resulting in a 20-50% reduction in clinical malaria incidence due to the nonlinear relationship between the EIR and malaria incidence (32). To remedy this, the target outcome could be specified as an inferred 20-50% reduction in malaria incidence due to the target species, calculated in terms of a reduction in species-specific vectorial capacity commensurate with the epidemiological goal. Other target outcomes are also important, and may include a rate of spread expected to produce the desired epidemiological impact within the time frame of a field trial (perhaps two years), and a minimum duration of effect of perhaps three years (5).
Biosafety and Cost Considerations
Finally, modeling may play a role in assessing some of the biosafety and cost dimensions of gene drive mosquito TPPs. One aspect of biosafety is the availability of products and strategies to remediate gene drive-modified organisms from the environment in the event of unwanted consequences or a shift in public opinion. The need and capability to remediate gene drive organisms is still being discussed (33, 34); however, in the absence of extensive field data, modeling can provide insights to determine minimum criteria and capabilities for insecticide-based campaigns or genetic systems such as ERACR (element reversing the autocatalytic chain reaction) (35, 36) in order to achieve a defined level of transgene confinement or removal.
Some aspects of a model-informed TPP may overlap with risk assessment. For instance, whether there is a tolerable mosquito biting rate that would not be expected to enhance transmission of target and non-target pathogens (37). Lastly, as demonstrated by case studies of odor-baited traps and LAIs, costing is another important dimension of TPPs that modeling may help to inform. Analyses focusing on deployment costs suggest that, due to their self-propagating nature, highly effective gene drives are expected to be more cost-effective than currently-available tools (38); however, similarly detailed analyses have yet to be conducted for monitoring requirements, which are expected to be a cost driver for the technology (39). That said; gene drives occupy a distinct niche in the malaria control toolkit due to their ability to spread and be effective despite compliance rates; therefore, costs may be best assessed against potentially available funds rather than the costs of other interventions.
Discussion
As gene drive mosquito products mature, TPPs will guide their advancement from lab to field, and mathematical models will necessarily inform TPP criteria. The potential irreversibility of gene drive releases heightens the need for rigorous modeling to assess their safety and efficacy. Recent advances in malaria modeling will facilitate prediction of epidemiological outcomes, data from ecological studies will facilitate predictions of safety, and input from a wide range of stakeholders will facilitate refinement of the target outcomes that modeling seeks to predict.
Data Availability Statement
The original contributions presented in the study are included in the article. Further inquiries can be directed to the corresponding author.
Author Contributions
All authors listed have made a substantial, direct, and intellectual contribution to the work, and approved it for publication.
Funding
This work was supported by funds from the Bill & Melinda Gates Foundation (INV-017683), the UC Irvine Malaria Initiative, and a DARPA Safe Genes Program Grant (HR0011-17-2-0047) awarded to JM. The funders had no role in the study design, decision to publish, or preparation of the manuscript.
Conflict of Interest
The authors declare that the research was conducted in the absence of any commercial or financial relationships that could be construed as a potential conflict of interest.
Publisher’s Note
All claims expressed in this article are solely those of the authors and do not necessarily represent those of their affiliated organizations, or those of the publisher, the editors and the reviewers. Any product that may be evaluated in this article, or claim that may be made by its manufacturer, is not guaranteed or endorsed by the publisher.
Acknowledgments
The authors would like to thank Drs. Fredros Okumu, Melissa Penny, Keith Fraser, Julian Entwistle, Derric Nimmo and Jason Richardson for helpful discussions and dialogue that informed the manuscript.
References
1. World Health Organization. “World Malaria Report 2020: 20 Years of Global Progress and Challenges”. Geneva, Switzerland: WHO Press (2020).
2. Bhatt S, Weiss DJ, Cameron E, Bisanzio D, Mappin B, Dalrymple U, et al. The Effect of Malaria Control on Plasmodium Falciparum in Africa Between 2000 and 2015. Nature (2015) 526:207–11. doi: 10.1038/nature15535
3. Wang G-H, Gamez S, Raban RR, Marshall JM, Alphey L, Li M, et al. Combating Mosquito-Borne Diseases Using Genetic Control Technologies. Nat Commun (2021) 12:4388. doi: 10.1038/s41467-021-24654-z
4. Carballar-Lejarazú R, James AA. Population Modification of Anopheline Species to Control Malaria Transmission. Pathog Glob Health (2017) 111:424–35. doi: 10.1080/20477724.2018.1427192
5. James SL, Marshall JM, Christophides GK, Okumu FO, Nolan T. Toward the Definition of Efficacy and Safety Criteria for Advancing Gene Drive-Modified Mosquitoes to Field Testing. Vector Borne Zoonotic Dis (2020) 20:237–51. doi: 10.1089/vbz.2019.2606
6. Chitnis N, Smith T, Steketee R. A Mathematical Model for the Dynamics of Malaria in Mosquitoes Feeding on a Heterogeneous Host Population. J Biol Dynamics (2008) 2:259–85. doi: 10.1080/17513750701769857
7. Chitnis N, Hardy D, Smith T. A Periodically-Forced Mathematical Model for the Seasonal Dynamics of Malaria in Mosquitoes. Bull Math Biol (2012) 74:1098–124. doi: 10.1007/s11538-011-9710-0
8. Griffin JT, Deirdre Hollingsworth T, Okell LC, Churcher TS, White M, Hinsley W, et al. Reducing Plasmodium Falciparum Malaria Transmission in Africa: A Model-Based Evaluation of Intervention Strategies. PloS Med (2010) 7:e1000324. doi: 10.1371/journal.pmed.1000324
9. Walker PGT, Griffin JT, Ferguson NM, Ghani AC. Estimating the Most Efficient Allocation of Interventions to Achieve Reductions in Plasmodium Falciparum Malaria Burden and Transmission in Africa: A Modelling Study. Lancet Glob Health (2016) 4:e474–84. doi: 10.1016/S2214-109X(16)30073-0
10. Traore MM, Junnila A, Traore SF, Doumbia S, Revay EE, Kravchenko VD, et al. Large-Scale Field Trial of Attractive Toxic Sugar Baits (ATSB) for the Control of Malaria Vector Mosquitoes in Mali, West Africa. Malar J (2020) 19:72. doi: 10.1186/s12936-020-3132-0
11. Hogan AB, Winskill P, Ghani AC. Estimated Impact of RTS,S/AS01 Malaria Vaccine Allocation Strategies in Sub-Saharan Africa: A Modelling Study. PloS Med (2020) 17:e1003377. doi: 10.1371/journal.pmed.1003377
12. Fraser KJ, Mwandigha L, Traore SF, Traore MM, Doumbia S, Junnila A, et al. Estimating the Potential Impact of Attractive Targeted Sugar Baits (ATSBs) as a New Vector Control Tool for Plasmodium Falciparum Malaria. Malar J (2021) 20:151. doi: 10.1186/s12936-021-03684-4
13. Okumu FO, Govella NJ, Moore SJ, Chitnis N, Killeen GF. Potential Benefits, Limitations and Target Product-Profiles of Odor-Baited Mosquito Traps for Malaria Control in Africa. PloS One (2010) 5:e11573. doi: 10.1371/journal.pone.0011573
14. Burgert L, Reiker T, Golumbeanu M, Möhrle JJ, Penny MA. Model-Informed Target Product Profiles of Long Acting-Injectables for Use as Seasonal Malaria Prevention. medRxiv (2021). doi: 10.1101/2021.07.05.21250483
15. Hogan AB, Winskill P, Verity R, Griffin JT, Ghani AC. Modelling Population-Level Impact to Inform Target Product Profiles for Childhood Malaria Vaccines. BMC Med (2018) 16:109. doi: 10.1186/s12916-018-1095-6
16. Killeen GF, Chitnis N, Moore SJ, Okumu FO. Target Product Profile Choices for Intra-Domiciliary Malaria Vector Control Pesticide Products: Repel or Kill? Malar J (2011) 10:207. doi: 10.1186/1475-2875-10-207
17. Killeen GF, Moore SJ. Target Product Profiles for Protecting Against Outdoor Malaria Transmission. Malar J (2012) 11:17. doi: 10.1186/1475-2875-11-17
18. Müller G, Schlein Y. Sugar Questing Mosquitoes in Arid Areas Gather on Scarce Blossoms That Can be Used for Control. Int J Parasitol (2006) 36:1077–80. doi: 10.1016/j.ijpara.2006.06.008
19. Müller GC, Beier JC, Traore SF, Toure MB, Traore MM, Bah S, et al. Successful Field Trial of Attractive Toxic Sugar Bait (ATSB) Plant-Spraying Methods Against Malaria Vectors in the Anopheles Gambiae Complex in Mali, West Africa. Malar J (2010) 9:210. doi: 10.1186/1475-2875-9-210
20. Le Menach A, Takala S, McKenzie FE, Perisse A, Harris A, Flahault A, et al. An Elaborated Feeding Cycle Model for Reductions in Vectorial Capacity of Night-Biting Mosquitoes by Insecticide-Treated Nets. Malar J (2007) 6:10. doi: 10.1186/1475-2875-6-10
21. White MT, Griffin JT, Churcher TS, Ferguson NM, Basáñez M-G, Ghani AC. Modelling the Impact of Vector Control Interventions on Anopheles Gambiae Population Dynamics. Parasitol Vectors (2011) 4:153. doi: 10.1186/1756-3305-4-153
22. Day JF, Sjogren RD. Vector Control by Removal Trapping. Am J Trop Med Hyg (1994) 50:126–33. doi: 10.4269/ajtmh.1994.50.126
23. Constantini C, Gibson G, Brady J, Merzagora L, Coluzzi M. A New Odor Baited Trap to Collect Host Seeking Mosquitoes. Parassitologia (1993) 35:5–9.
24. Okumu FO, Killeen GF, Ogoma S, Biswaro L, Smallegange RC, Mbeyela E, et al. Development and Field Evaluation of a Synthetic Mosquito Lure That Is More Attractive Than Humans. PloS One (2010) 5:e8951. doi: 10.1371/journal.pone.0008951
25. Okumu FO, Madumla EP, John AN, Lwetoijera DW, Sumaye RD. Attracting, Trapping and Killing Disease-Transmitting Mosquitoes Using Odor-Baited Stations - The Ifakara Odor-Baited Stations. Parasitol Vectors (2010) 3:12. doi: 10.1186/1756-3305-3-12
26. Killeen GF, Smith TA, Ferguson HM, Mshinda H, Abdulla S, Lengeler C, et al. Preventing Childhood Malaria in Africa by Protecting Adults From Mosquitoes With Insecticide-Treated Nets. PloS Med (2007) 4:e229. doi: 10.1371/journal.pmed.0040229
27. Macintyre F, Ramachandruni H, Burrows JN, Holm R, Thomas A, Möhrle JJ, et al. Injectable Anti-Malarials Revisited: Discovery and Development of New Agents to Protect Against Malaria. Malar J (2018) 17:402. doi: 10.1186/s12936-018-2549-1
28. Golumbeanu M, Yang G, Camponovo F, Stuckey EM, Hamon N, Mondy M, et al. Combining Machine Learning and Mathematical Models of Disease Dynamics to Guide Development of Novel Disease Interventions. medRxiv (2021). doi: 10.1101/2021.01.05.21249283v1.abstract
29. North AR, Burt A, Godfray HCJ. Modelling the Potential of Genetic Control of Malaria Mosquitoes at National Scale. BMC Biol (2019) 17:26. doi: 10.1186/s12915-019-0645-5
30. Eckhoff PA. A Malaria Transmission-Directed Model of Mosquito Life Cycle and Ecology. Malar J (2011) 10:303. doi: 10.1186/1475-2875-10-303
31. Eckhoff PA, Wenger EA, Godfray HCJ, Burt A. Impact of Mosquito Gene Drive on Malaria Elimination in a Computational Model With Explicit Spatial and Temporal Dynamics. Proc Natl Acad Sci USA (2017) 114:E255–64. doi: 10.1073/pnas.1611064114
32. Smith DL, McKenzie FE, Snow RW, Hay SI. Revisiting the Basic Reproductive Number for Malaria and Its Implications for Malaria Control. PloS Biol (2007) 5:e42. doi: 10.1371/journal.pbio.0050042
33. National Academies of Sciences, Engineering, and Medicine, Division on Earth and Life Studies, Board on Life Sciences, and Committee on Gene Drive Research in Non-Human Organisms: Recommendations for Responsible Conduct. Gene Drives on the Horizon: Advancing Science, Navigating Uncertainty, and Aligning Research With Public Values. National Academies Press (2016).
34. Marshall JM, Akbari OS. Can CRISPR-Based Gene Drive Be Confined in the Wild? A Question for Molecular and Population Biology. ACS Chem Biol (2018) 13:424–30. doi: 10.1021/acschembio.7b00923
35. Vella MR, Gunning CE, Lloyd AL, Gould F. Evaluating Strategies for Reversing CRISPR-Cas9 Gene Drives. Sci Rep (2017) 7:11038. doi: 10.1038/s41598-017-10633-2
36. Xu X-RS, Bulger EA, Gantz VM, Klanseck C, Heimler SR, Auradkar A, et al. Active Genetic Neutralizing Elements for Halting or Deleting Gene Drives. Mol Cell (2020) 80:246–62.e4. doi: 10.1016/j.molcel.2020.09.003
37. Connolly JB, Mumford JD, Fuchs S, Turner G, Beech C, North AR, et al. Systematic Identification of Plausible Pathways to Potential Harm via Problem Formulation for Investigational Releases of a Population Suppression Gene Drive to Control the Human Malaria Vector Anopheles Gambiae in West Africa. Malar J (2021) 20:170. doi: 10.1186/s12936-021-03674-6
38. Metchanun N, Borgemeister C, Amzati G, von Braun J, Nikolov M, Selvaraj P, et al. Modeling Impact and Cost-Effectiveness of Gene Drives for Malaria Elimination in the Democratic Republic of the Congo. medRxiv (2021). doi: 10.1101/2020.06.29.20142760
Keywords: attractive targeted sugar baits, gene drive mosquitoes, long-acting injectable drugs, malaria, odor-baited traps, population modification, population suppression
Citation: Mondal A, Vásquez VN and Marshall JM (2022) Target Product Profiles for Mosquito Gene Drives: Incorporating Insights From Mathematical Models. Front. Trop. Dis 3:828876. doi: 10.3389/fitd.2022.828876
Received: 04 December 2021; Accepted: 14 January 2022;
Published: 01 March 2022.
Edited by:
Adam E. Vorsino, United States Fish and Wildlife Service, United StatesReviewed by:
Cynthia Kon, Swinburne University of Technology Sarawak Campus, MalaysiaCopyright © 2022 Mondal, Vásquez and Marshall. This is an open-access article distributed under the terms of the Creative Commons Attribution License (CC BY). The use, distribution or reproduction in other forums is permitted, provided the original author(s) and the copyright owner(s) are credited and that the original publication in this journal is cited, in accordance with accepted academic practice. No use, distribution or reproduction is permitted which does not comply with these terms.
*Correspondence: John M. Marshall, am9obi5tYXJzaGFsbEBiZXJrZWxleS5lZHU=