- Thomas H. Gosnell School of Life Sciences, Rochester Institute of Technology, Rochester, NY, United States
Approximately 2.8 million people worldwide are infected with bacteria that are deemed resistant to clinically relevant antibiotics. This accounts for 700,000 deaths every year and represents a major public health threat that has been on the rise for the past two decades. In contrast, the pace of antibiotic discovery to treat these resistant pathogens has significantly decreased. Most antibiotics are complex natural products that were isolated from soil microorganisms during the golden era of antibiotic discovery (1940s to 1960s) employing the “Waksman platform”. After the collapse of this discovery platform, other strategies and approaches emerged, including phenotype- or target-based screenings of large synthetic compound libraries. However, these methods have not resulted in the discovery and/or development of new drugs for clinical use in over 30 years. A better understanding of the structure and function of the molecular components that constitute the bacterial system is of paramount importance to design new strategies to tackle drug-resistant pathogens. Herein, we review the traditional approaches as well as novel strategies to facilitate antibiotic discovery that are chemical biology-focused. These include the design and application of chemical probes that can undergo bioorthogonal reactions, such as copper (I)-catalyzed azide-alkyne cycloadditions (CuAAC). By specifically interacting with bacterial proteins or being incorporated in the microorganism’s metabolism, chemical probes are powerful tools in drug discovery that can help uncover new drug targets and investigate the mechanisms of action and resistance of new antibacterial leads.
Introduction
Antibiotic resistance is rapidly increasing on a global scale. A recent study predicts that, by 2050, antibiotic-resistant infections will cause more deaths yearly when compared to the deaths caused by cancer (1). At the same time, the discovery rate of antibiotics belonging to novel chemical classes and with new mechanisms of action (MoAs) is declining. This is largely due to a combination of scientific, economic and policies challenges that include a lack of innovation in antibacterial drug discovery methods and low economic incentives from both the public and private sectors (2). Another reason for the lack of success in the discovery of new antibiotics is the poor understanding of the bacterial cell system as a whole, despite advances in bacterial genomics and drug discovery platforms (3).
Traditional Approaches Used in Antimicrobial Drug Discovery
The vast majority of antibiotics currently used in the clinics were discovered during the few decades after World War II, a period often referred to as the golden age of antibiotic discovery. At that time, the traditional approach for antibiotic discovery consisted of screening for compounds produced by soil-derived microbes that inhibited the growth of pathogenic bacteria in vitro. Through this method, also known as the “Waksman platform”, scientists discovered several natural product-derived antibiotics that are still in use today, including: streptomycin, vancomycin, tetracycline, and rifampicin (4) (Table 1). After about two decades using this platform, scientists began to encounter difficulties, with known compounds being repeatedly discovered and their efforts consistently yielding diminishing returns (3, 5). Following the collapse of the Waksman platform, the efforts to find new antibiotics shifted from isolating natural products to synthesizing new compounds and creating slightly modified analogs of existing drugs. These approaches resulted both in new medicines of the quinolone, oxazolidinone, and sulfa classes, for example, and in optimized drugs of preceding antibiotic chemical classes, which were very useful in the clinics but still susceptible to resistance (6, 7).
Rapid advances in genomics, bioinformatics, and medicinal chemistry in the 1990s gave rise to high-throughput screening (HTS) and, with it, target-based screening was introduced into antibiotic discovery programs in addition to the traditional phenotype-based screens (4, 8) (Table 1). While the latter involves selecting antibacterial compounds according to a specific phenotype they exhibit (Figure 1A), such as inhibition of bacterial growth, in the former method, compound libraries are screened against a pre-determined protein target for inhibition of its biological function (Figure 1B). Target identification and validation are crucial in both approaches to elucidate and better understand the inhibitor’s MoA. In phenotypic assays, however, this is usually determined much later in the discovery process and, in many cases, even after approval for clinical use. Conversely, despite knowing the drug target from the start in target-based approaches, these in vitro screens often identify lead inhibitors that fail to penetrate the bacterial cell wall and wind up being biologically inactive (4, 5).
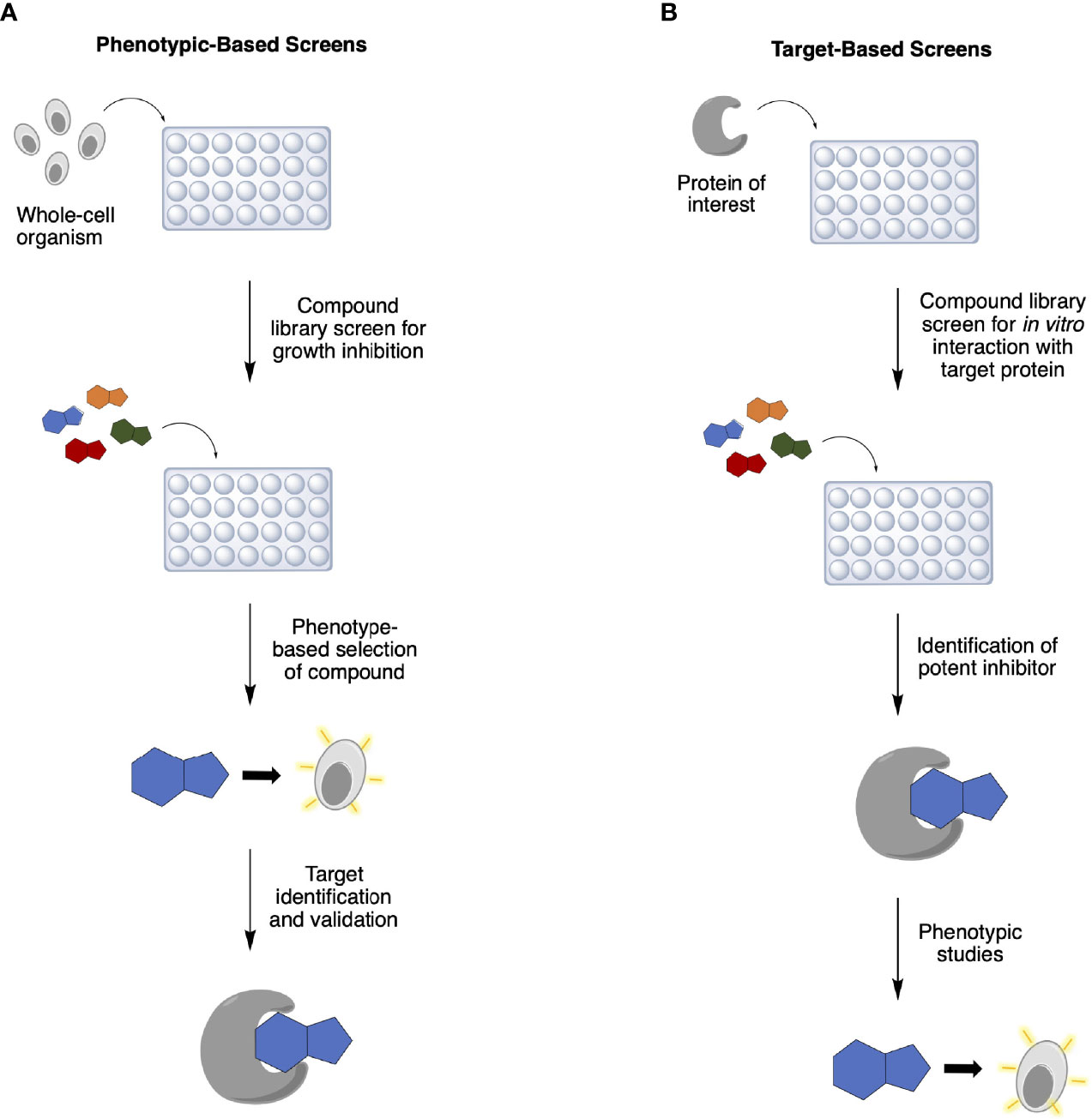
Figure 1 Traditional approaches to antibiotic discovery: (A) phenotypic-based and (B) target-based screens. Both methods follow similar steps later in the cascade: hit compounds evaluation, lead compound validation and optimization, and preclinical and clinical development.
The evolution of multi-drug resistant bacteria has been surpassing the rate of antibiotic discovery for many decades. Although the number of antibiotics to reach the clinics has increased in the past few years, it still represents a minor percentage (< 10%) of the total drugs approved by the Food and Drug Administration (FDA). For instance, in the period of 2018 and 2019, only 9 antibacterial agents were approved by the FDA, including: plazomicin; eravacycline; sarecycline; omadacycline; rifamycin; a combination therapy comprising of imipenem, cilastatin, and relebactam; pretomanid; lefamulin; and cefiderocol (9, 10). From this list, only pretomanid represents a first-in-class drug, while the others are either derivatives of existing compounds, such as tetracycline, or new combinations of existing drugs (10). The absence of new antibiotic classes with efficacy against Gram-negative pathogens is of particular concern. Consequently, the development of new strategies for antibiotic discovery with lower costs and increased success rates is fundamental to invert this dire scenario. Given the urgent need, recent research efforts have been devoted into antibiotic discovery and resulted in novel promising strategies and therapeutic leads.
Despite being underexplored for a period of time, natural product discovery has undergone a revival in the past few years. Scientists are now looking into unexploited and more diverse sources of antimicrobials, such as uncultured bacteria, invertebrates, and plants (11). For instance, the natural product teixobactin has been isolated and identified from the extracts of uncultured soil microbes (12). This compound was shown to inhibit bacterial cell wall synthesis in Gram-positive bacteria, such as Staphylococcus aureus and Mycobacterium tuberculosis, without detectable resistance. Another recently reported natural product, darobactin, was discovered from extracts of the gut microbiome of nematodes (13). Darobactin showed activity, both in vitro and in vivo, against Gram-negative pathogens; however, resistance was detected via mutations on its target protein, the β-barrel, outer membrane protein periplasmic chaperone (BamA) (13). Marine invertebrates have also been explored for antimicrobial natural products, and a variety of compounds from different chemical classes such as oxazolidinones and guanidinium alkaloids have been reported in recent literature (14).
Although no antibiotics discovered through HTS methods have made it to the clinics so far, recent improvements in the antibiotic discovery pipeline have resulted in promising new therapeutic leads (4). A combination of HTS with rational design holds great promise in producing new scaffolds to overcome resistance and expand pathogen scope given the advances in synthetic chemistry and an increased knowledge on the desired pharmacokinetic properties of antibiotics (3, 14, 15). Moreover, a switch from reductionist genes-to-drugs studies to more comprehensive systems-level approaches in antibacterial drug discovery has proven fundamental to identify antibiotics with novel MoAs and less prone to resistance (3). For example, the compound N3-Cyclopropyl-7-[[4-(1-methylethyl) phenyl] methyl]-7H-pyrrolo[3,2-f]quinazoline-1,3-diamine dihydrochloride, referred to as SCH-79797, was recently identified in a library screen of approximately 33,000 unique small molecules (16). It demonstrated broad-spectrum bactericidal activity, both in vitro and in vivo, with undetectably low resistance frequencies. By using a combination of quantitative imaging, proteomics, genetics, metabolomics, and whole-cell assays, the authors identified a dual-targeting MoA for SCH-79797. Finally, medicinal chemistry efforts resulted in a derivative of SCH-79797, Irresistin-16, with higher potency and reduced mammalian toxicity that demonstrated efficacy in treating a Neisseria gonorrhoeae animal infection model. Overall, this study showed that combining multiple targeting moieties and MoAs onto the same small molecule is a novel powerful method to tackle drug-resistant pathogens.
Another recent rational design study reported the discovery of antibiotics from the diazabicyclooctane (DBO) chemical class that showed activity against Gram-negative bacteria without detectable resistance (17). Initial modifications to the structure of avibactam, a known DBO β-lactamase inhibitor, resulted in a first lead compound that showed bactericidal activity against antibiotic-resistant pathogens in vitro, as well as potent in vivo efficacy against a Pseudomonas aeruginosa infection model. Additional structure optimization was necessary, however, to improve the compound’s permeation across bacterial outer membranes. After noticing that chemical features that enhanced target potency were also responsible for preventing compound uptake, the authors established an optimization strategy involving structure-porin permeation relationships (SPPR) that led to the design of the compound (4R,7S)-7-(N-hydroxy-N’-methylcarbamimidoyl)-1-methyl-4-(sulfooxyamino)-5,7-dihydro-4H-pyrazolo[3,4-c]pyridine-6-carboxylic acid, referred to as ETX0462. This compound demonstrated enhanced bacterial uptake with potent target inhibition in vitro, as well as in vivo activity against difficult-to-treat bacteria, such as the ESKAPE pathogens (Enterococcus faecium, S. aureus, Klebsiella pneumoniae, Acinetobacter baumannii, P. aeruginosa, and Enterobacter species), in addition to Stenotrophomonas maltophilia, and other pathogens.
Emerging Approaches and Tools Used in Antimicrobial Drug Discovery
Machine Learning-Based Approaches: Using Deep Neural Networks to Predict Antibiotic Activity
There has been a rising interest in developing and applying computational prediction algorithms in drug discovery over the past few years, and these approaches can be particularly advantageous for antibacterial drug discovery. They have the potential to decrease the costs of lead compound identification while simultaneously decreasing the time and labor needed to find ideal molecules and increasing the rate to identify structurally novel antibiotics (Table 2). In a recent study, a machine/deep learning approach was used to predict antibacterial molecules in silico from multiple structurally diverse chemical libraries (7). First, a small library composed of known antibiotic drugs served as a training set to teach a deep neural network to predict growth inhibition of E. coli (Figure 2, 1). After optimization, their model was applied to several chemical libraries to identify potential lead compounds with the desired phenotype (Figure 2, 2-4). Finally, the most promising candidates were chosen for empirical tests based on high prediction scores, chemical structure (distant from known antibiotics), availability, and low predicted toxicity.
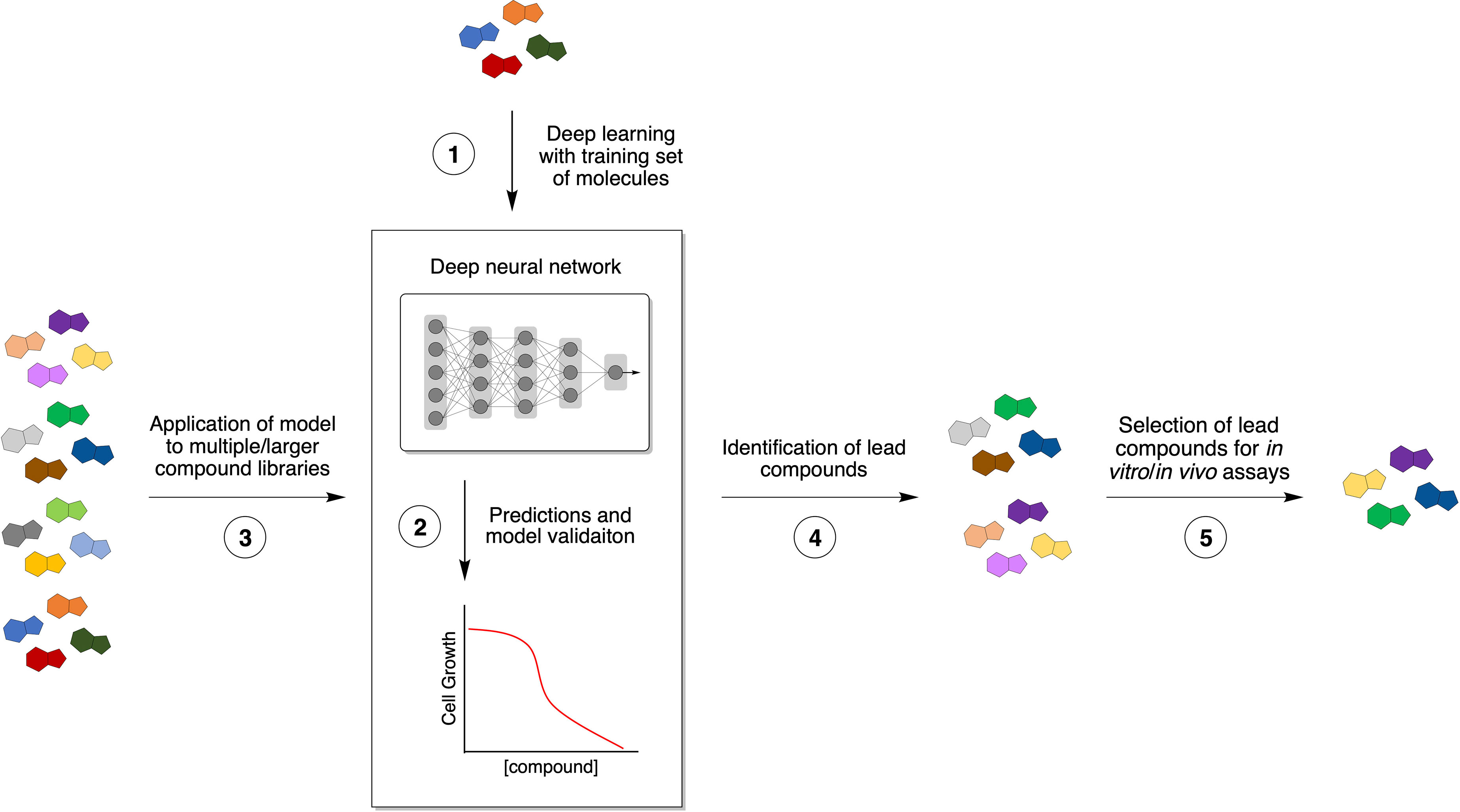
Figure 2 Novel approaches to antibiotic discovery. Machine/deep learning applied to antibiotic discovery affords rapid and inexpensive screening of compounds with potential antimicrobial activity. A small set of known antibiotics is used to train a deep neural network (1) to predict compounds that produce a specific phenotype, such bacterial growth inhibition (2). After optimization, the resulting model is applied to multiple chemical libraries (3) to identify potential lead compounds with antibiotic activity (4). Finally, promising candidates are chosen based on desired properties, such as chemical structure diversity, synthetic availability, and low predicted toxicity (5).
A small-molecule inhibitor of the c-Jun N-terminal kinase (JNK), named halicin, was discovered from the Drug Repurposing Hub as a compound with broad-spectrum in vitro and in vivo bactericidal activity using a deep learning approach (7). While halicin was identified from an initial test involving 2,300 candidates, a much larger library comprising more than 107 million molecules was screened and resulted in 23 hits that were further tested to confirm their activities. From this, eight antibacterial compounds with diverse chemical structures were identified, and two, in particular, displayed broad-spectrum activity even against resistant E. coli strains. Overall, the deep learning approach was to show the feasibility and plausibility of this approach, hence proving a model to facilitate antibiotic discovery.
For specific structural classes of well-known antibiotic compounds such as non-ribosomal peptides (NRPs), automated discovery from natural sources has been enabled by computational methods (18). The authors of this study created the NRPminer, an algorithm that uses the antibiotics and secondary metabolite analysis shell (antiSMASH) genome mining tool (19) to identify biosynthetic gene clusters (BGCs) in the genomes of organisms that encode for NRP synthetases (NRPS). They utilized this algorithm to search for post-assembly modified NRPs from large (meta)genomic and mass spectrometry databases. NRPminer discovered NRPs from different bacterial samples, including soil-associated microbes and the human microbiota. Furthermore, the authors validated two of the previously unreported NRP families predicted by the algorithm using nuclear magnetic resonance (NMR) and demonstrated their anti-parasite activities, although modest (IC50 value in the low millimolar range), against Trypanosoma brucei, the unicellular flagellated parasite that is the causative agent of the fatal tropical disease named sleeping sickness.
The success of machine/deep learning and other methods described here for antibiotic discovery is deeply connected to the number of tools and data available, as well as appropriate experimental designs. For this, understanding bacterial cell systems and the related chemistry is of paramount importance. Advances in bacterial genome sequencing and proteomic technologies have significantly enhanced our knowledge of microbial biochemistry by providing valuable insight on biochemical functions and mechanisms, as well as novel enzymes and metabolites (20). This information alone, however, is not sufficient to produce a comprehensive picture of how drug candidates interact with bacterial systems at the molecular level to uncover novel antibacterial leads and their mechanisms of action. In this context, the use of chemical tools to probe biology is a powerful strategy to investigate the targets and functions of antimicrobial drug candidates (3).
Chemical Biology-Based Approaches: Using Chemical Probes as Tools in Antibiotic Discovery
Chemical probes usually consist of a drug or metabolite functionalized with a reporter tag, such as a fluorescent dye or affinity tag, for imaging and/or proteomic applications (Figure 3A and Table 2). Similar to chemical probes, activity-based probes (ABPs) consist of three elements: (i) a recognition element, which promotes selectivity toward the target of interest; (ii) a reactive group (electrophilic or photoreactive) that covalently binds to the interacting protein(s); and (iii) a reporter tag that enables detection, enrichment, identification and/or visualization of the probe-labeled protein(s) (Figure 3A and Table 2) (21). Most bioorthogonal probes contain alkyne and azide groups for copper(I)-catalyzed azide-alkyne cycloadditions (CuAAC), but click reactions are versatile and can be performed between various pairs of compatible bioorthogonal reactive groups, such as triarylphosphines and azides (Staudinger ligation), amines and aldehydes or ketones (carbonyl condensation), among others (Figures 3A, B) (21). All of these elements should be carefully studied and considered when designing chemical reporters and ABPs so that they do not interfere with the probes’ ability to bind to potential targets (Table 2).
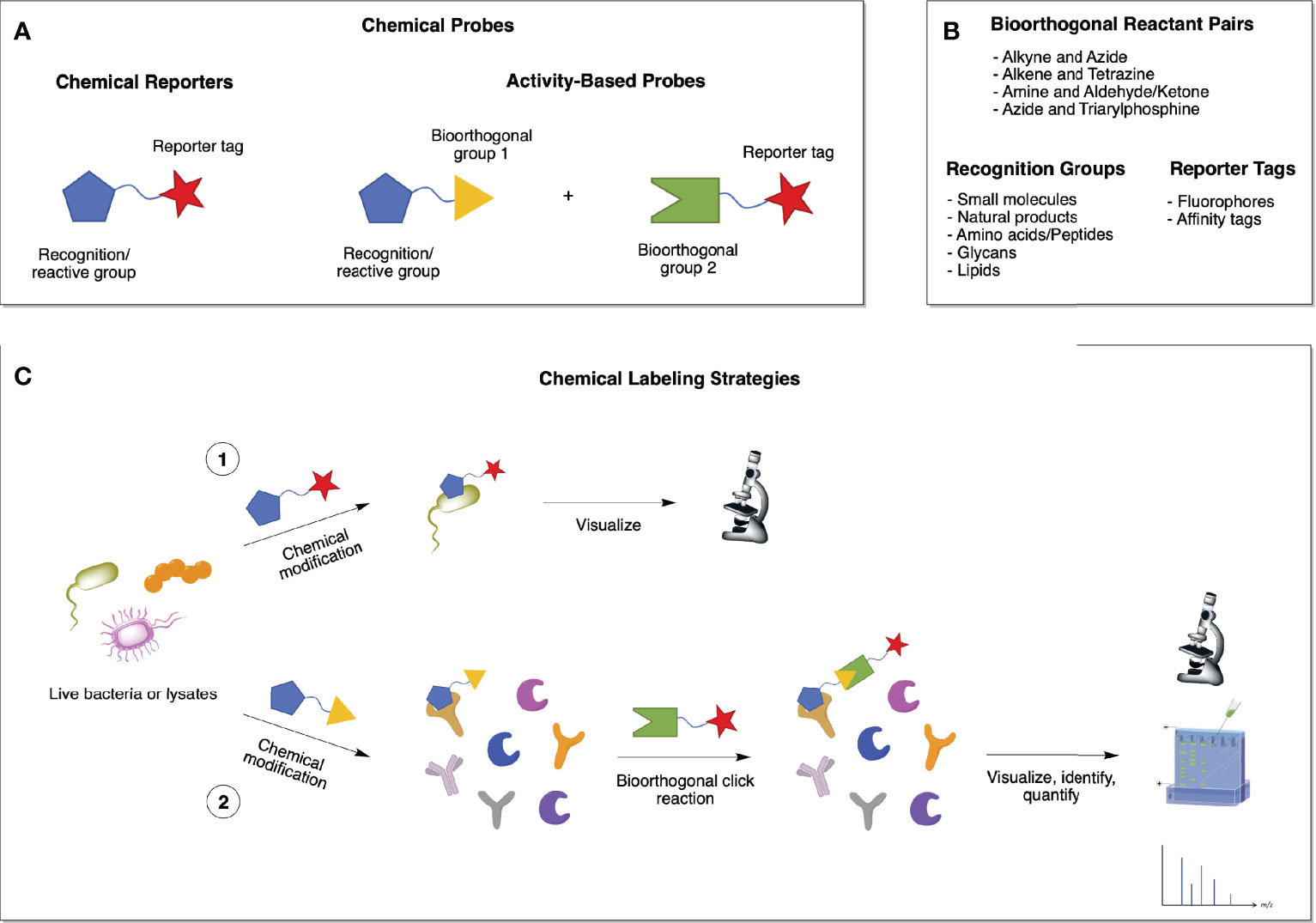
Figure 3 Chemical biology strategies for investigating biological processes in bacteria. (A) Chemical probes can be of two types: chemical reporters or activity-based probes (ABPs). The first ones consist of a drug or metabolite functionalized with a reporter tag, while the latter comprise a bioorthogonal group (1) that is complementary to a second bioorthogonal group (2) bearing a reporter tag. (B) Examples of the different elements that compose a chemical probe. (C) Chemical labeling of proteins in live bacterial cells or lysates can occur via 1) a one-step chemical modification with a functionalized probe that allows for the visualization of the reporter-labeled targets; or 2) a two-step bioorthogonal chemical ligation, by which the resulting covalent complex formed between the probe and its target proteins can be visualized, identified, and quantified using various methods.
Chemical probes can be introduced to whole cells or lysates to specifically label the biomolecules they interact with via either affinity binding alone (chemical reporters; one-step labeling strategy; Figure 3C, 1) or affinity binding combined with bioorthogonal (or “click”) reactions (activity-based probes; two-step labeling strategy; Figure 3C, 2). ABP profiling (ABPP) facilitates the investigation of microbiota-metabolite interactions, microbiome composition, and microbiome dynamics (22). Advances in chemoproteomic techniques have greatly facilitated the detection of covalent probe-tagged proteins following ABPP routine in the last few years (23). A number of chemical reporters and ABPs have been developed and applied to study various classes of microbes, including Gram-negative, Gram-positive, mycobacteria, and even more complex microbiota communities (Figure 4). Among bacterial molecules tagged by chemical reporters are surface glycans, peptidoglycans (PG), lipopolysaccharides (LPS), capsular polysaccharides (CPS), glycoproteins, amino acids, lipids, and secondary bile acids (produced by the microbial transformation of host bile acids) (22).
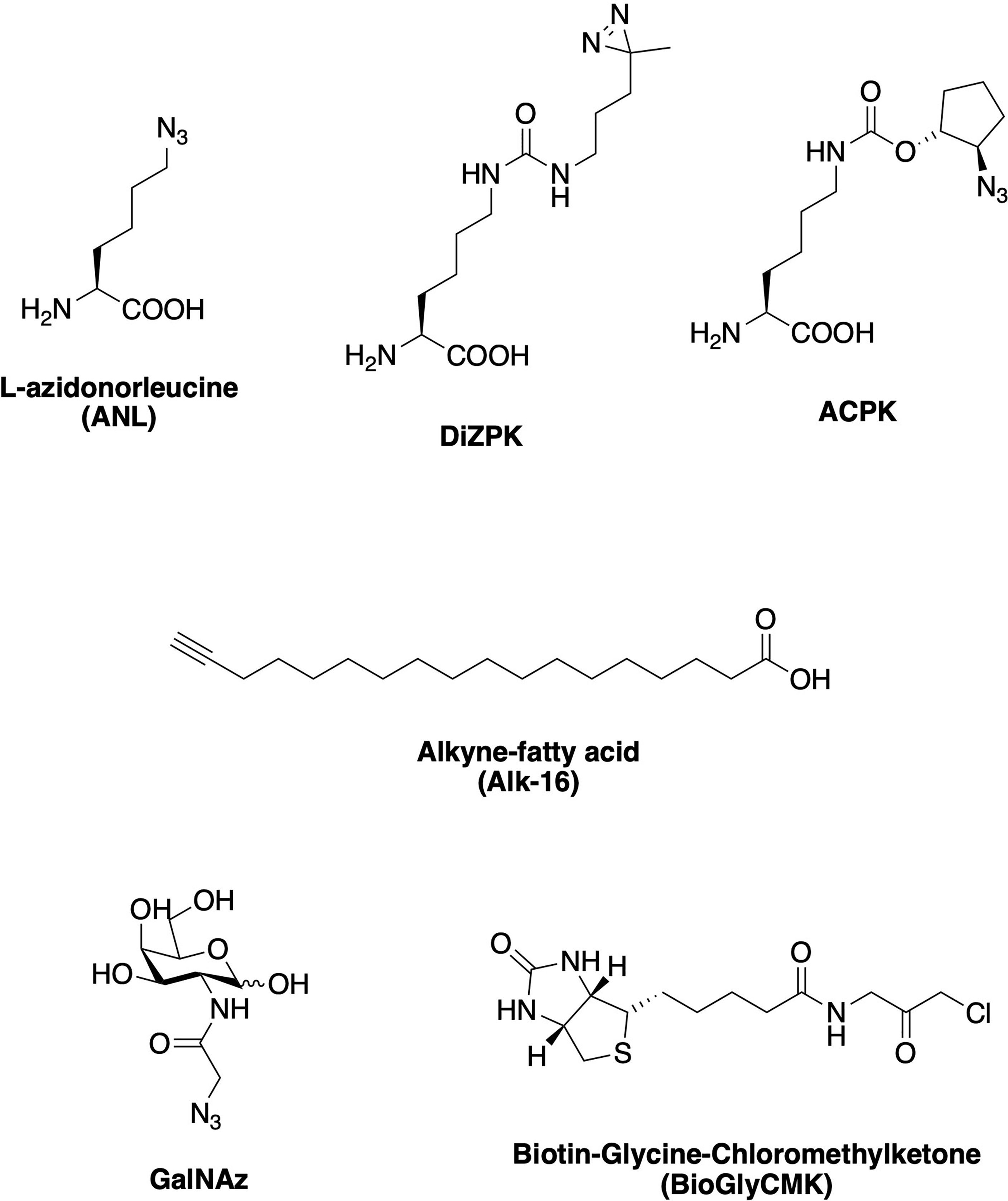
Figure 4 Examples of chemical reporters and probes that have been used to study various classes of microbes, including amino acid-, lipid, and sugar-based reporters, as well as activity-based probes (ABPs).
Chemical reporters are useful tools to visualize global events happening in a bacterial cell. For example, fluorescent probes have been employed to study PG biosynthesis, an important crosslinked glycopeptide polymer involved in maintaining cell shape and integrity in bacteria (24). Vancomycin and ramoplanin, two antibiotics known to bind PG precursors, were attached to fluorescent dyes to help determine the genes that regulate PG biosynthesis. By comparing the staining patterns of these probes in B. subtilis, the authors observed a concentration-dependent activity, suggesting that high concentrations of the antibiotics perturbed PG synthesis. Moreover, their data indicated that the cytoskeletal protein Mbl did not play an essential role in directing the localization of the PG biosynthetic machinery, contradicting their initial hypothesis. A recent study employed fluorescent probes to selectively target and differentiate vancomycin-resistant S. aureus (VRSA) from vancomycin-sensitive S. aureus (VSSA) strains (25). A dual-probe labeling protocol was described in which the siderophore staphyloferrin A (SA) was chosen as a specific ligand to target S. aureus, and vancomycin (Van) was used as the ligand to detect resistance. As a result, the SA-based probes labeled both VRSA and VSSA S. aureus strains while sparing bacteria from different species, such as B. subtilis, E. faecalis, and E. coli, and the Van-based probes showed selective labeling of VSSA over VRSA. An optimized dual-probe protocol was then utilized in diagnostic experiments in complex bacterial mixtures and proved successful in detecting VRSA in the presence of both VSSA and other bacterial species.
Amino acid chemical reporters have shown useful to monitor bacterial protein translation. Bioorthogonal noncanonical amino acid tagging (BONCAT) uses alkyne- and azide-modified amino acids and their respective mutant tRNA synthetases for selective proteomic analysis of bacterial translation. Such noncanonical amino acids, for example, have been used to study protein expression and antibiotic resistance profiles in biofilms of P. aeruginosa subpopulations (26). Unnatural amino acids bearing a photoactive diazirin group and an azide handle, DiZPK and ACPK respectively (Figure 4), were successfully incorporated into proteins in enteric bacteria from a mouse model of Inflammatory Bowel Disease (IBD) (27). Additionally, tracking newly synthesized proteins in situ inside complex gut microbial communities has been achieved by feeding the community with azide-bearing amino acids, which can then be converted into fluorescent probes in vivo via bioorthogonal chemistry (28). Another study reported the use of two fluorescent D-amino acid (FDAA) probes, TAMRA-amino-D-alanine (TADA) and Cy5-amino-D-alanine (Cy5ADA), for the live monitoring of bacterial growth and division patterns in the mouse gut (29). The same FDAA probes were employed to assess the viability of bacteria in fecal transplants (30). The use of bioorthogonal probes has greatly facilitated the analysis of fecal samples, which are notoriously difficult to study via conventional methods without prior sample treatment. In this case, bacteria were treated with one probe before the transplant and the recipient mice was fed a different probe subsequently. If the bacteria survived the transplantation process, they showed emission for both probes and could thereby be confirmed as viable. Other alanine-derived probes, modified with either alkyne or cyclooctyne reporters, have also been applied to investigate PG turnover in bacteria (31, 32).
Glycan chemical reporters have been employed to monitor sugar-containing macromolecules that decorate the bacterial cell surface, such as LPS and CPS, as well as post-translationally modified proteins. Azide-modified sugar units that are LPS building blocks have been developed to label its inner core structure via click reaction with an alkyne-bearing fluorophore (33). CPS are also present in the cell surface of many bacteria. Repeating units of monosaccharides such as N-acetylglucosamine (GlcNAc) and N-acetylgalactosamine (GalNAc) compose its structure, which vary greatly among different bacteria. In this context, azide-labeled GalNAc (named GalNAz, Figure 4) was employed to image anaerobic commensal CPS present in gut microbes in live mice (34). Using alkyne-containing fluorophores with distinct colors, the authors achieved differential labeling and tracking of three Bacteroides species, enabling the visualization of real-time interactions between the gut microbes and the host immune system.
A recent work described a multifunctional and structurally unique chemoselective probe that was developed to analyze femtomolar levels of metabolic amines in fecal samples (35). The probe contains both a previously unreported bioorthogonal cleavage site (p-nitrocinnamyloxycarbonyl) and a selective, amine-reactive “warhead” made up of a sulfo-N-hydroxysuccinimide (sulfo-NHS) group. The probe was also functionalized with magnetic beads in one end to allow for the direct extraction of metabolites from human fecal samples that could be detected via mass spectrometry analysis. Similarly, chemoselective probes have also made possible to determine the sources of certain metabolites in the gut microbiome. For example, sphinganines are bioactive components found in foods, but they are also produced by the resident gut microbiota. Thereby, creating alkyne-tagged sphinganines enabled the identification of the bacterial genus Bacteroides as the nearly exclusive source of the microbial sphinganines based on labeling followed by cell-sorting (36). Other lipid-based chemical reporters, such as fatty acid derivatives (Figure 4), have also been used to image membranes in bacteria (37), identify lipid-modified proteins (38), or even study bacterial-secreted virulence factors (39–42).
ABPs have been able to reveal specific interactions between probe-modified proteins and their client proteins via bioorthogonal reactions. For instance, these molecules can help to identify the protein targets involved in bacterial pathogenesis or acid-stress defense mechanisms in situ, which otherwise has been challenging to achieve using conventional methods. An ABP composed of a chloromethylketone linked to glycine and a biotin tag (BioGlyCMK, Figure 4) is a cysteine-reactive probe that showed labeling of several proteases and hydrolases in mice. This approach permitted the differentiation between humanized mice models harboring either a “healthy” or an IBD-affected human microbiome, since the proteins were tagged only in the IBD model and not in the “healthy” one (43). A versatile set of chemical probes for detecting bile salt hydrolase (BSH) activity was based on the bioluminescence of luciferin and has been employed to detect BSH activity in purified enzymes, bacterial cells and fecal slurries (44). They were also used to non-invasively detect BSH activity in mice and humans in the same study. When BSH activity was present, luciferin was released from the conjugated bile acid and assayed using luciferase. These bile acid-luciferin probes were used to both demonstrate that prebiotics stimulate BSH activity and facilitate the non-invasive detection of clinical IBD status in patients.
Conclusions and Perspectives
The rise in antibiotic resistant bacteria coupled with a decrease in the discovery and development of novel antibiotics is a global threat. Innovation in drug discovery methods and platforms are necessary to uncover novel chemical classes of antibiotics with novel MoAs that are able to effectively treat diseases caused by resistant pathogens and/or emerging pathogens. Additionally, new chemical tools and approaches are in constant need for development to examine the functions and/or roles of understudied microbial enzymes and biochemical pathways that are involved in anabolizing compounds that have bactericidal/bacteriostatic activities, and those that are attractive targets for inhibition from individual bacterial species and complex microbial communities. Advances in chemical and computational biology have enhanced current tools and our understanding to probe microorganisms and study biochemical pathways and enzymatic mechanisms via bioorthogonal small-molecule-protein interactions. The variety of chemical reactions these probes can undergo, combined with the remarkable diversity in disease-causing microbes (unique enzymes, pathways, metabolites), results in innumerous possibilities to create new tools to investigate understudied metabolic pathways and proteins in bacteria, therefore, revealing new targets for antibiotic discovery and development. Importantly, these tools allow for the generation of unique small molecules to specifically interact with protein targets in bacteria. This could help to better understand how bacterial cells react to or resist chemical perturbation, providing invaluable information on antimicrobial resistance mechanisms.
Some of the challenges involving the use of chemical probes, however, are worth mentioning. First, the chemical synthesis of probes can be difficult, time-consuming, and expensive due to the complexity and low stability of metabolites and natural-product derived antibiotics. Second, the addition of functional groups to drug/metabolite scaffolds can not only make the synthesis even more challenging but also jeopardize their biological activities by disrupting interactions with the target protein. Third, although chemical probes undergo selective bioorthogonal reactions in biological settings, off-target probe labeling that results in background noise is a possibility that should be considered. Nevertheless, chemical probes are versatile and tunable tools that could still be instrumental additions to drug discovery platforms already in use by researchers in both academia and industry. One potential application would be their incorporation into phenotypic screens (Figure 1A) to enrich for and identify the specific biological targets that drug candidates are interacting with to cause a pre-determined phenotype. Other applications would be to help elucidate and validate the protein target of interest, as well as evaluate target engagement, occupancy, and mechanisms of action and resistance, which are crucial steps in the preclinical stage of the drug discovery process.
Author Contributions
RM: conceptualization, writing, editing, revising. AP: conceptualization, writing. AH: conceptualization, editing, revising. All authors have given approval to the final version of the manuscript.
Funding
This work was supported by a National Institutes of Health (NIH) Grant (R15GM144862) to AH.
Conflict of Interest
The authors declare that the research was conducted in the absence of any commercial or financial relationships that could be construed as a potential conflict of interest.
Publisher’s Note
All claims expressed in this article are solely those of the authors and do not necessarily represent those of their affiliated organizations, or those of the publisher, the editors and the reviewers. Any product that may be evaluated in this article, or claim that may be made by its manufacturer, is not guaranteed or endorsed by the publisher.
Acknowledgments
The authors would like to thank the College of Science, The Thomas H. Gosnell School of Life Sciences for ongoing support of research in the Hudson laboratory.
Abbreviations
CuAAC, copper (I)-catalyzed azide-alkyne cycloaddition; MoA(s), mechanism(s) of action; HTS, high-throughput screening; FDA, Food and Drug Administration; BamA, component A of the β-barrel assembly machinery complex; DBO, diazabicyclooctane; SPRR, structure-porin permeation relationships; ESKAPE, Enterococcus faecium, Staphylococcus aureus, Klebsiella pneumoniae, Acinetobacter baumannii, Pseudomonas aeruginosa, and Enterobacter species; JNK, c-Jun N-terminal kinase; NRP(s), non-ribosomal peptide(s); antiSMASH, antibiotics and secondary metabolite analysis shell; BGC(s), biosynthetic gene cluster(s) (BGC); NRPS(s), non-ribosomal peptide synthetase(s); NMR, nuclear magnetic resonance; IC50, half maximal inhibitory concentration; ABP(s), activity-based probe(s); ABPP, activity-based probe profiling; PG, peptidoglycans; LPS, lipopolysaccharides; CPS, capsular polysaccharides; VRSA, vancomycin-resistant S. aureus; VSSA, vancomycin-sensitive S. aureus; SA, staphyloferrin A; BONCAT, bioorthogonal noncanonical amino acid tagging; DiZPK, ((3-(3-methyl-3H-diazirin-3-yl)propamino)carbonyl)-Nϵ-L-lysine; ACPK, Nϵ-((((1R,2R)-2-azidocyclopentyl)oxy)carbonyl)-L-lysine; IBD, inflammatory bowel disease; FDAA, fluorescent D-amino acid; TAMRA, tetramethylrhodamine azide; TADA, TAMRA-amino-D-alanine; Cy5ADA, Cyanine 5-amino-D-alanine; GlcNAc, N-acetylglucosamine; GalNAz, azido-labeled N-acetylglucosamine; sulfo-NHS, sulfo-N-hydroxysuccinimide; BioGlyCMK, biotin-glycine-chloromethylketone; BSH, bile salt hydrolase.
References
1. O’Neill J. Antimicrobial Resistance: Tackling a Crisis for the Health and Wealth of Nations. Rev Antimicrob Resist (2014) 1–20.
2. Lewis K. The Science of Antibiotic Discovery. Cell (2020) 181(1):29–45. doi: 10.1016/j.cell.2020.02.056
3. Farha MA, French S, Brown ED. Systems-Level Chemical Biology to Accelerate Antibiotic Drug Discovery. Acc Chem Res (2021) 54(8):1909–20. doi: 10.1021/acs.accounts.1c00011
4. Boyd NK, Teng C, Frei CR. Brief Overview of Approaches and Challenges in New Antibiotic Development: A Focus on Drug Repurposing. Front Cell Infect Microbiol (2021) 11:684515. doi: 10.3389/fcimb.2021.684515
5. Brotz-Oesterhelt H, Bandow JE, Labischinski H. Bacterial Proteomics and its Role in Antibacterial Drug Discovery. Mass Spectrom Rev (2005) 24(4):549–65. doi: 10.1002/mas.20030
6. Martin JK. Drug Discovery Needs to Change. Nature (2020) 586:S57. doi: 10.1038/d41586-020-02888-z
7. Stokes JM, Yang K, Swanson K, Jin W, Cubillos-Ruiz A, Donghia NM, et al. A Deep Learning Approach to Antibiotic Discovery. Cell (2020) 180(4):688–702 e13. doi: 10.1016/j.cell.2020.01.021
8. Lewis K. Platforms for Antibiotic Discovery. Nat Rev Drug Discov (2013) 12(5):371–87. doi: 10.1038/nrd3975
9. Butler MS, Blaskovich MA, Cooper MA. Antibiotics in the Clinical Pipeline at the End of 2015. J Antibiot (Tokyo) (2017) 70(1):3–24. doi: 10.1038/ja.2016.72
10. Andrei S, Droc G, Stefan G. FDA Approved Antibacterial Drugs: 2018-2019. Discover (Craiova) (2019) 7(4):e102. doi: 10.15190/d.2019.15
11. Mantravadi PK, Kalesh KA, Dobson RCJ, Hudson AO, Parthasarathy A. The Quest for Novel Antimicrobial Compounds: Emerging Trends in Research, Development, and Technologies. Antibiotics (Basel) (2019) 8(1):1–34. doi: 10.3390/antibiotics8010008
12. Ling LL, Schneider T, Peoples AJ, Spoering AL, Engels I, Conlon BP, et al. A New Antibiotic Kills Pathogens Without Detectable Resistance. Nature (2015) 517(7535):455–9. doi: 10.1038/nature14098
13. Imai Y, Meyer KJ, Iinishi A, Favre-Godal Q, Green R, Manuse S, et al. A New Antibiotic Selectively Kills Gram-Negative Pathogens. Nature (2019) 576(7787):459–64. doi: 10.1038/s41586-019-1791-1
14. Valdes-Pena MA, Massaro NP, Lin YC, Pierce JG. Leveraging Marine Natural Products as a Platform to Tackle Bacterial Resistance and Persistence. Acc Chem Res (2021) 54(8):1866–77. doi: 10.1021/acs.accounts.1c00007
15. Looper RE, Boger DL, Silver LL. Small Molecular Weapons Against Multi-Drug Resistance. Acc Chem Res (2021) 54(13):2785–7. doi: 10.1021/acs.accounts.1c00246
16. Martin JK 2nd, Sheehan JP, Bratton BP, Moore GM, Mateus A, Li SH, et al. A Dual-Mechanism Antibiotic Kills Gram-Negative Bacteria and Avoids Drug Resistance. Cell (2020) 181(7):1518–32.e14. doi: 10.1016/j.cell.2020.05.005
17. Durand-Reville TF, Miller AA, O’Donnell JP, Wu X, Sylvester MA, Guler S, et al. Rational Design of a New Antibiotic Class for Drug-Resistant Infections. Nature (2021) 597:1–5. doi: 10.1038/s41586-021-03899-0
18. Behsaz B, Bode E, Gurevich A, Shi YN, Grundmann F, Acharya D, et al. Integrating Genomics and Metabolomics for Scalable Non-Ribosomal Peptide Discovery. Nat Commun (2021) 12(1):3225. doi: 10.1038/s41467-021-23502-4
19. Blin K, Shaw S, Steinke K, Villebro R, Ziemert N, Lee SY, et al. antiSMASH 5.0: Updates to the Secondary Metabolite Genome Mining Pipeline. Nucleic Acids Res (2019) 47(W1):W81–7. doi: 10.1093/nar/gkz310
20. Kountz DJ, Balskus EP. Leveraging Microbial Genomes and Genomic Context for Chemical Discovery. Acc Chem Res (2021) 54(13):2788–97. doi: 10.1021/acs.accounts.1c00100
21. Parker CG, Pratt MR. Click Chemistry in Proteomic Investigations. Cell (2020) 180(4):605–32. doi: 10.1016/j.cell.2020.01.025
22. Zhang ZJ, Wang YC, Yang X, Hang HC. Chemical Reporters for Exploring Microbiology and Microbiota Mechanisms. Chembiochem (2020) 21(1-2):19–32. doi: 10.1002/cbic.201900535
23. Berger AB, Vitorino PM, Bogyo M. Activity-Based Protein Profiling: Applications to Biomarker Discovery, In Vivo Imaging and Drug Discovery. Am J Pharmacogenomics (2004) 4(6):371–81. doi: 10.2165/00129785-200404060-00004
24. Tiyanont K, Doan T, Lazarus MB, Fang X, Rudner DZ, Walker S. Imaging Peptidoglycan Biosynthesis in Bacillus Subtilis With Fluorescent Antibiotics. Proc Natl Acad Sci USA (2006) 103(29):11033–8. doi: 10.1073/pnas.0600829103
25. Wang TA, Chen PL, Chen YS, Hung HM, Huang JY. Selectively Targeting and Differentiating Vancomycin-Resistant Staphylococcus Aureus via Dual Synthetic Fluorescent Probes. ACS Infect Dis (2021) 7(9):2584–90. doi: 10.1021/acsinfecdis.1c00235
26. Babin BM, Atangcho L, van Eldijk MB, Sweredoski MJ, Moradian A, Hess S, et al. Selective Proteomic Analysis of Antibiotic-Tolerant Cellular Subpopulations in Pseudomonas Aeruginosa Biofilms. mBio (2017) 8(5):1–16. doi: 10.1128/mBio.01593-17
27. Lin S, Zhang Z, Xu H, Li L, Chen S, Li J, et al. Site-Specific Incorporation of Photo-Cross-Linker and Bioorthogonal Amino Acids Into Enteric Bacterial Pathogens. J Am Chem Soc (2011) 133(50):20581–7. doi: 10.1021/ja209008w
28. Hatzenpichler R, Scheller S, Tavormina PL, Babin BM, Tirrell DA, Orphan VJ. In Situ Visualization of Newly Synthesized Proteins in Environmental Microbes Using Amino Acid Tagging and Click Chemistry. Environ Microbiol (2014) 16(8):2568–90. doi: 10.1111/1462-2920.12436
29. Lin L, Wu Q, Song J, Du Y, Gao J, Song Y, et al. Revealing the In Vivo Growth and Division Patterns of Mouse Gut Bacteria. Sci Adv (2020) 6(36):1–9. doi: 10.1126/sciadv.abb2531
30. Wang W, Lin L, Du Y, Song Y, Peng X, Chen X, et al. Assessing the Viability of Transplanted Gut Microbiota by Sequential Tagging With D-Amino Acid-Based Metabolic Probes. Nat Commun (2019) 10(1):1317. doi: 10.1038/s41467-019-09267-x
31. Siegrist MS, Whiteside S, Jewett JC, Aditham A, Cava F, Bertozzi CR. (D)-Amino Acid Chemical Reporters Reveal Peptidoglycan Dynamics of an Intracellular Pathogen. ACS Chem Biol (2013) 8(3):500–5. doi: 10.1021/cb3004995
32. Shieh P, Siegrist MS, Cullen AJ, Bertozzi CR. Imaging Bacterial Peptidoglycan With Near-Infrared Fluorogenic Azide Probes. Proc Natl Acad Sci USA (2014) 111(15):5456–61. doi: 10.1073/pnas.1322727111
33. Dumont A, Malleron A, Awwad M, Dukan S, Vauzeilles B. Click-Mediated Labeling of Bacterial Membranes Through Metabolic Modification of the Lipopolysaccharide Inner Core. Angew Chem Int Ed Engl (2012) 51(13):3143–6. doi: 10.1002/anie.201108127
34. Geva-Zatorsky N, Alvarez D, Hudak JE, Reading NC, Erturk-Hasdemir D, Dasgupta S, et al. In Vivo Imaging and Tracking of Host-Microbiota Interactions via Metabolic Labeling of Gut Anaerobic Bacteria. Nat Med (2015) 21(9):1091–100. doi: 10.1038/nm.3929
35. Garg N, Conway LP, Ballet C, Correia MSP, Olsson FKS, Vujasinovic M, et al. Chemoselective Probe Containing a Unique Bioorthogonal Cleavage Site for Investigation of Gut Microbiota Metabolism. Angewandte Chemie Int Ed (2018) 57(42):13805–9. doi: 10.1002/anie.201804828
36. Lee MT, Le HH, Johnson EL. Dietary Sphinganine is Selectively Assimilated by Members of the Mammalian Gut Microbiome. J Lipid Res (2021) 62:100034. doi: 10.1194/jlr.RA120000950
37. Fishov I, Woldringh CL. Visualization of Membrane Domains in Escherichia Coli. Mol Microbiol (1999) 32(6):1166–72. doi: 10.1046/j.1365-2958.1999.01425.x
38. Rangan KJ, Yang YY, Charron G, Hang HC. Rapid Visualization and Large-Scale Profiling of Bacterial Lipoproteins With Chemical Reporters. J Am Chem Soc (2010) 132(31):10628–9. doi: 10.1021/ja101387b
39. Burnaevskiy N, Fox TG, Plymire DA, Ertelt JM, Weigele BA, Selyunin AS, et al. Proteolytic Elimination of N-Myristoyl Modifications by the Shigella Virulence Factor IpaJ. Nature (2013) 496(7443):106–9. doi: 10.1038/nature12004
40. Liu W, Zhou Y, Peng T, Zhou P, Ding X, Li Z, et al. N(epsilon)-Fatty Acylation of Multiple Membrane-Associated Proteins by Shigella IcsB Effector to Modulate Host Function. Nat Microbiol (2018) 3(9):996–1009. doi: 10.1038/s41564-018-0215-6
41. Zhou Y, Huang C, Yin L, Wan M, Wang X, Li L, et al. N(epsilon)-Fatty Acylation of Rho GTPases by a MARTX Toxin Effector. Science (2017) 358(6362):528–31. doi: 10.1126/science.aam8659
42. Burnaevskiy N, Peng T, Reddick LE, Hang HC, Alto NM. Myristoylome Profiling Reveals a Concerted Mechanism of ARF GTPase Deacylation by the Bacterial Protease IpaJ. Mol Cell (2015) 58(1):110–22. doi: 10.1016/j.molcel.2015.01.040
43. Mayers MD, Moon C, Stupp GS, Su AI, Wolan DW. Quantitative Metaproteomics and Activity-Based Probe Enrichment Reveals Significant Alterations in Protein Expression From a Mouse Model of Inflammatory Bowel Disease. J Proteome Res (2017) 16(2):1014–26. doi: 10.1021/acs.jproteome.6b00938
Keywords: antibiotic discovery, antimicrobial resistance, resistant pathogens, chemical biology, click chemistry, bioorthogonal probes
Citation: Miranda RR, Parthasarathy A and Hudson AO (2022) Exploration of Chemical Biology Approaches to Facilitate the Discovery and Development of Novel Antibiotics. Front. Trop. Dis 3:845469. doi: 10.3389/fitd.2022.845469
Received: 29 December 2021; Accepted: 31 January 2022;
Published: 03 March 2022.
Edited by:
Faith Mabiki, Sokoine University of Agriculture, TanzaniaReviewed by:
William Brittain, Durham University, United KingdomCynthia A. Danquah, Kwame Nkrumah University of Science and Technology, Ghana
Copyright © 2022 Miranda, Parthasarathy and Hudson. This is an open-access article distributed under the terms of the Creative Commons Attribution License (CC BY). The use, distribution or reproduction in other forums is permitted, provided the original author(s) and the copyright owner(s) are credited and that the original publication in this journal is cited, in accordance with accepted academic practice. No use, distribution or reproduction is permitted which does not comply with these terms.
*Correspondence: Renata Rezende Miranda, cnJtc2JpQHJpdC5lZHU=; André O. Hudson, YW9oc2JpQHJpdC5lZHU=