- 1Etteplan Sweden Akitebolag (AB), Uppsala, Sweden
- 2IDLab, Department of Electronics and Information Systems, Ghent University - Imec, Ghent, Belgium
- 3Akkodis, Temse, Belgium
- 4Ethiopian Public Health Institute, Addis Ababa, Ethiopia
- 5Department of Medical Laboratory Sciences, Institute of Health, Jimma University, Jimma, Ethiopia
- 6Vector Control Division, Kampala, Uganda
- 7Bridges to Development, Geneva, Switzerland
- 8Lygature, Utrecht, Netherlands
- 9Merck Global Health Institute, Geneva, Switzerland
- 10Department of Translational Physiology, Infectiology and Public Health, Ghent University, Merelbeke, Belgium
- 11Janssen Global Public Health, Janssen Research and Development (R&D), Beerse, Belgium
The World Health Organization (WHO) recently published target product profiles (TPPs) for neglected tropical diseases (NTDs) to inform and accelerate the development of diagnostics tools necessary to achieve targets in the decade ahead. These TPPs describe the minimal and ideal requirements for various diagnostic needs related to NTD specific use-cases. An early step towards the manufacture and implementation of new diagnostics is to critically review the TPPs and translate these into an initial design and ultimately into user requirement specifications (URS). Artificial intelligence-based digital pathology (AI-DP) may overcome critical shortcomings of current standards for most NTDs reliant on microscopy, such as poor reproducibility and error-prone manual read-out. Furthermore, a digitalised workflow can create opportunities to reduce operational costs via increased throughput and automated data capture, analysis, and reporting. Despite these promising benefits, a critical review of the NTD TPPs with consideration to an AI-DP diagnostic solution is lacking. We present a systematic analysis of one of the WHO TPPs with the aim to inform the development of a URS for an AI-DP solution for NTDs. As a case study we focused on monitoring and evaluation (M&E) of programs designed to control soil-transmitted helminths (STHs). To this end, we start by outlining a brief overview of diagnostic needs for STHs, after which we systematically analyse the recently published WHO TPPs, highlighting the technical considerations for an AI-DP diagnostic solution to meet the minimal requirements for this TPP. Finally, we further reflect on the feasibility of an AI-DP informing STH programs towards the WHO 2030 targets in due time.
1 Introduction
The recent publication of the World Health Organization (WHO) 2021 - 2030 road map for neglected tropical diseases (NTDs) (1), has put the diagnostic technologies high on the priority list of the NTD-community. Indeed, to reach the four 2030 targets (Target #1: a 90% reduction in people requiring interventions against NTDs, Target #2: a 75% reduction in disability-adjusted life years attributable to NTDs, Target #3 elimination of at least one NTD in 100 countries, Target #4 eradication of two NTDs), a more performant diagnostic armamentarium will be of utmost importance.
To guide the development of new diagnostics, WHO has published target product profiles (TPPs) for 10 NTDs (Buruli ulcer (2), dermal leishmaniasis (3), human African trypanosomiasis (4, 5), lymphatic filariasis (6, 7), mycetoma (8), onchocerciasis (9), scabies (10), schistosomiasis (SCH) (11), soil-transmitted helminthiasis (STH) (12) and yaws (13)). These TPPs describe the minimal and ideal requirements for various diagnostic needs (e.g., simplicity, performance, price of the test) related to NTD specific use-cases, including starting, monitoring and evaluation (M&E) control program performance (STH, onchocerciasis, scabies and SCH), stopping of program (lymphatic filariasis, onchocerciasis and scabies), surveillance (lymphatic filariasis and SCH), confirmation of cases (Buruli ulcer, dermal leishmaniasis, human African trypanosomiasis and mycetoma) and detection of drug resistance (yaws).
Although the TPPs are an important first step, there are a number of critical development steps that one must go through before a new diagnostic (or any other medical device) can be manufactured/repurposed and implemented in NTD control programs. Since the United States Food and Drug Administration (FDA) issued its Design Control Guidance For Medical Device Manufacturers (14) in 1997, various manufacturers have been applying the so-called waterfall design process as a basis for their medical device development process. While there are many variations of this waterfall design process, a typical example for such development processes is depicted in Figure 1 (the names for process, documents, stages and acronyms may differ depending on the manufacturer, region and regulatory bodies). Generally, the process defines a sequence of steps where outputs of one step are reviewed before becoming the input for the next step, resembling a waterfall effect. The process supports iterative improvements in and quality assurance of the device.
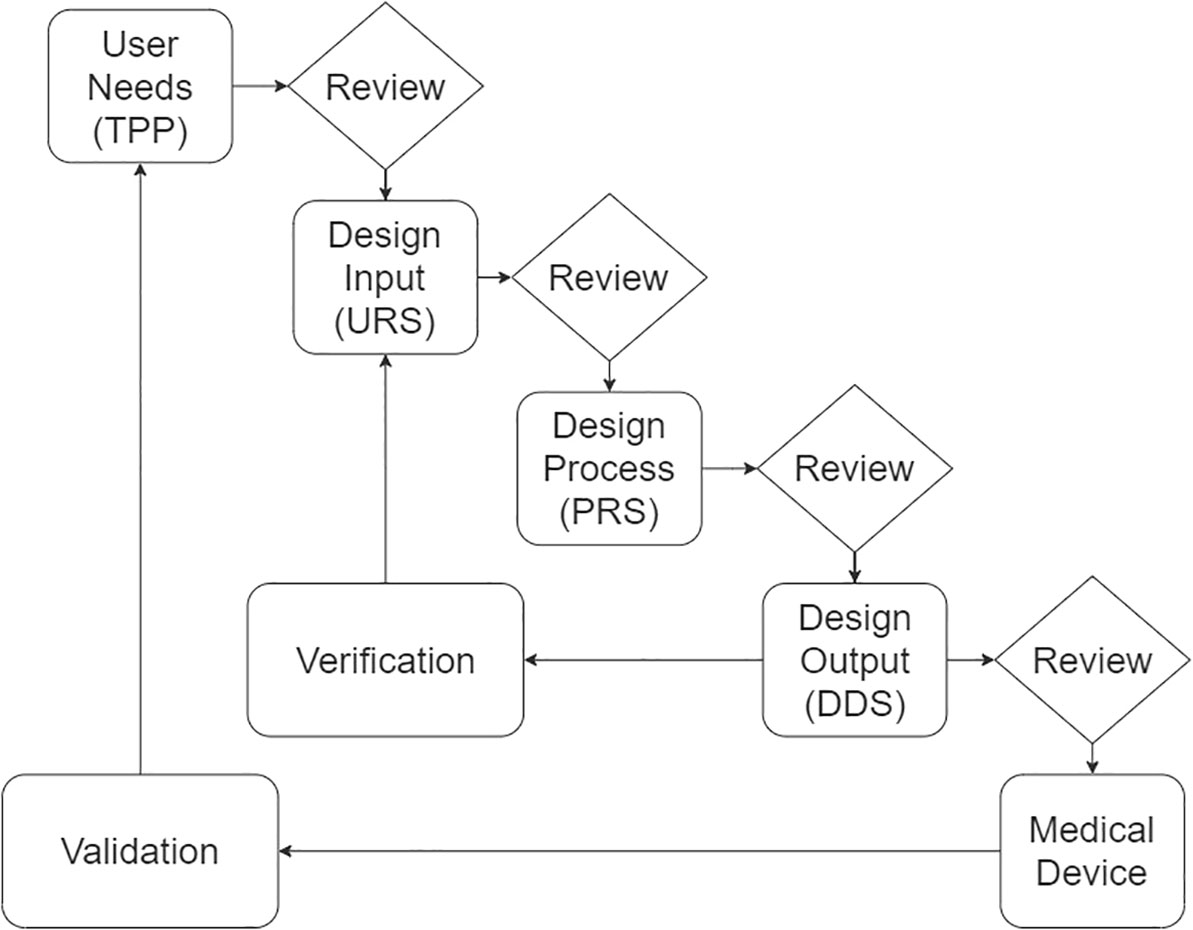
Figure 1 A typical waterfall design process for the development of medical devices, as presented in (14), with key design documents noted in brackets. TPP, target product profile; URS, user requirement specifications; PRS, product requirement specifications; DDS, detailed design specifications. The documents are maintained as a design history file.
As can be seen, the process starts with a TPP, which provides a thorough assessment of the user needs agnostic to a solution or technology. Following the review, design input can be used to generate a user requirement specification (URS). The URS defines the functionality the users will get from the medical device and is used as input to the design process. This results in the generation of a product requirement specification (PRS). The design output results in the generation of a detailed design specification (DDS), which includes the full design details required to manufacture the product. While the product requirement specifications defines what the device will or will not do, the detailed design specification defines what the device is, and provides a reference for the manufacturer to verify whether the design output meets the design input. Following verification of the design output, the medical device can be manufactured and validated against the user needs, for example through clinical trials. All design and review documentation produced during the device lifecycle are maintained and controlled as a design history file. From this figure it is clear that the next critical step towards new diagnostics for NTDs involves the review of the TPPs and the generation of a URS.
A diagnostic platform that is receiving increasing interest is artificial intelligence-based digital pathology (AI-DP) (15). Indeed, AI-DP is a revolutionising concept in general clinical pathology and a few regulated introductions were recently introduced (16, 17). AI-DP may also significantly contribute to NTD-programs, particularly as most programs today still rely heavily on visual and microscopic examination of specimens for most NTDs (including those listed above). Generally, a field application of AI-DP may consist of (EDC) electronic data capture tools, a whole slide imaging (WSI) scanner, an AI model, and a data reporting system. As illustrated in Figure 2, this field application of AI-DP may not only overcome the critical shortcomings of current microscopic diagnostic standards [e.g., poor reproducibility and error-prone manual read-out (18)], but may also reduce operational costs by increasing throughput and reducing labour demands, and may automate data capture, analysis and reporting (19). Despite these promising features, a critical review of the NTD TPPs towards URSs specific to AI-DP is lacking.
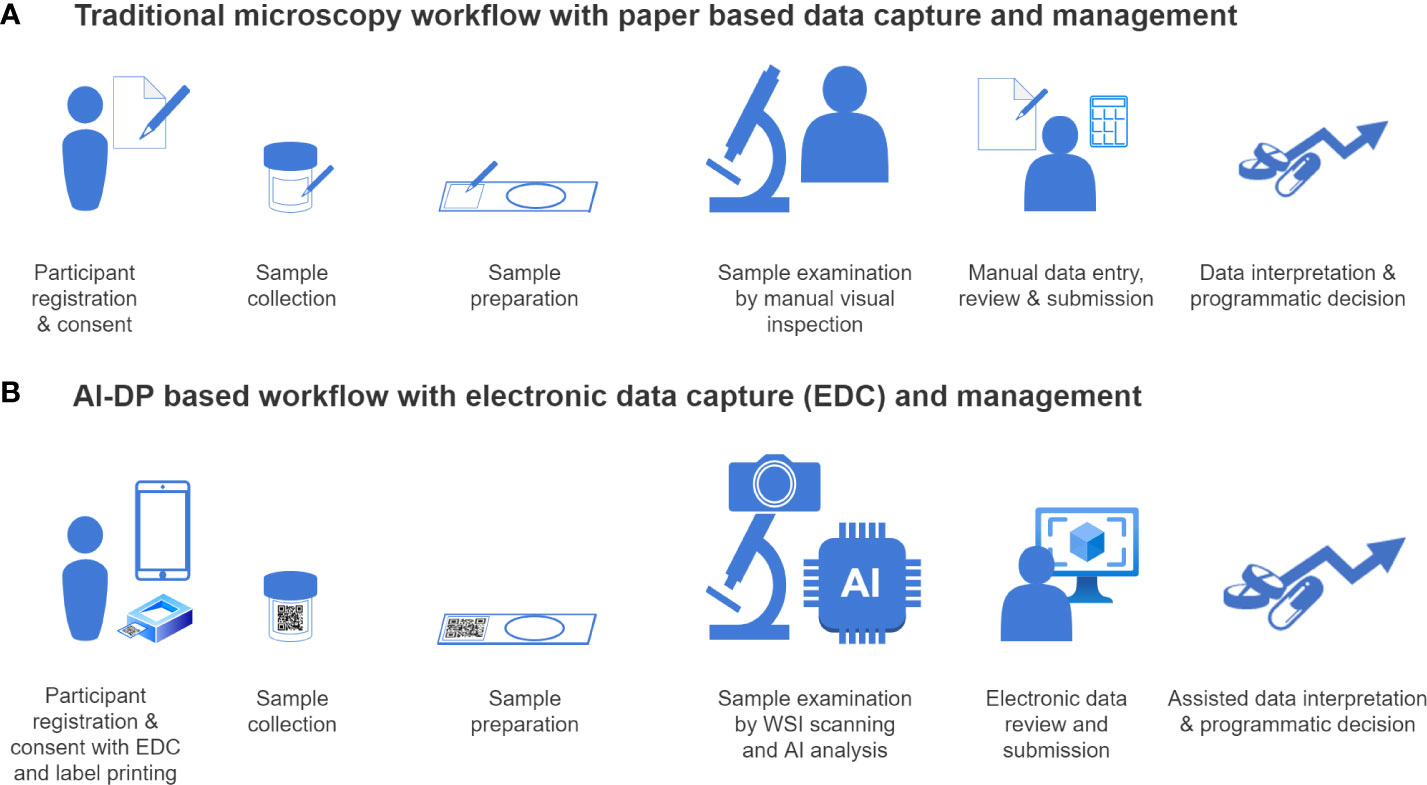
Figure 2 Workflow comparison between traditional microscopy and AI-DP. This figure schematically demonstrates the differences between the traditional microscopy-based test (A) and artificial-based digital pathology (AI-DP) (B) workflows. Electronic data capture (EDC) tools can be used to collect and track data (data management) throughout the process (for example by using labels containing QR codes). While use of electronic data capture in the traditional workflow is generally limited to participant registration and consent, sample collection and sample preparation, the AI-DP workflow can take advantage of electronic data management from start to finish with a higher degree of quality, less manual interactions and faster time to result. Target objects in the prepared slides are digitalized with whole slide image (WSI) scanning, and the images are processed by AI to identify the features of interest (AI-analysis). These features may be visually confirmed or rejected by a trained laboratory technician (electronic data review), and the final results can be made directly available in an online healthcare system database, after which a treatment decision can be taken (assisted data interpretation).
We present here an analysis of one of the WHO TPPs with the aim to inform the development of a URS for an AI-DP specific solution for NTDs. As a case study we focused on M&E of STH control programs. To this end, we start with a brief overview of diagnostic needs for STH, after which we systematically analysed the recently published WHO TPP, highlighting the technical considerations for an AI-DP diagnostic solution to meet the minimal requirements for this TPP. In a final section, we further reflect on the feasibility of an AI-DP informing STH programs towards the WHO 2030 targets in due time.
2 Diagnostic needs for monitoring and evaluation of soil-transmitted helminthiasis control program
STH is caused by a group of parasitic worms or helminths [Ascaris lumbricoides, Trichuris trichiura, Necator americanus and Ancylostoma duodenale (hookworms)] that are transmitted through infectious stages residing in soil (referring to the common name of the disease). It is estimated that more than 1.5 billion people, or 24% of the world’s population, are affected by STH (20). The main strategy to control the STH-morbidity are large scale deworming programs (preventive chemotherapy (PC) (12, 21, 22)), during which safe and broad-spectrum drugs are periodically administered as a single tablet to school-age-children. The frequency and nature of drug administration are determined by the prevalence of any helminth species, and a detailed decision tree to initiate, scale down frequency, and ultimately stop drug distribution is provided by WHO (12, 22). The previous WHO STH-specific target was to ensure a drug-administration program coverage of at least 75% in all endemic countries by 2020. In contrast, the main target for the next decade is to reduce the prevalence of moderate-to-heavy intensity infection to below 2% (12, 22). This shift from merely program-coverage target towards a disease-intensity target implies that diagnostic tools should not only be able to accurately quantify infections (traditionally done by estimating the number of helminth eggs in one gram of stool (EPG)) but should also be able to differentiate worm species. This is because the thresholds defining the intensity of infections are worm specific due to differences in both fecundity and morbidity (i.e., for the same number of worms, the impact depends on the species) (23). Currently, microscopically detecting and quantifying worm eggs in a stool smear applying the Kato-Katz (KK) technique is the WHO recommended diagnostic mean for STH control programs. In this microscopy-based test, a known amount of stool is used to generate a stool thick-smear on a standard glass slide, which trained microscopists use to manually visualize and count worm eggs in the stool sample using a standard microscope. The resulting statistic from this test is EPG, which is then used to refer to known WHO ranges of egg counts to determine the level of infection intensity.
While Strongyloides stercoralis is a species in the STH group, the WHO TPP for STH explicitly did not include Strongyloides. It has been proven that larvae are not detected in the KK stool thick smear method (24–27), and therefore not considered for the AI-DP approach. A separate TPP might be required for Strongyloides.
WHO summarises its diagnostic TPP for M&E of STH control programmes as follows: “The target product is an in-vitro/ex-vivo laboratory-based (minimum) or point-of-sampling (ideal) test that allows for quantitative detection of analytes specific to soil-transmitted helminths in all age groups. For laboratory-based tests, tests can be performed in regional or national diagnostic testing laboratories by trained laboratory technicians (< 1-week training); specific requirements for portability and transport should not exceed those of standard laboratory equipment. For point-of-sampling tests, health personnel and community health workers should be able to perform and interpret the test with only a single day of training; any equipment used for reading the test should be highly portable and battery powered if it needs electricity at all. The test should be specific (≥94%) to each Ascaris, Trichuris and hookworm and have a sensitivity of at least 60% for each of the three helminth species, although different sensitivity/specificity combinations are possible. The test should allow for a throughput of at least seven samples per hour and its cost should not exceed US$ 3.”
Below we assess these requirements step-by-step as they are presented in the WHO STH TPP document.
3 Systematic review of the WHO TPP for M&E of STH control programs with consideration of an AI-DP diagnostic solution
Given the availability of a WHO TPP for M&E of STH control programmes, we analysed and reviewed the implications of each requirement with respect to an AI-DP workflow involving the KK stool thick smear. For each TPP item, as presented below, we do not repeat the listed requirements, instead we provide additional considerations and constraints imposed with the application of an AI-DP specific solution. Therefore, we recommend the reader to read each of the minimal TPP items in parallel with our commentary.
3.1 Product use summary
3.1.1 Intended use
At least two use case scenarios are possible: (i) a laboratory-based testing configuration; and (ii) an outside of standard laboratory environment, for example during surveys in remote areas. KK stool thick smears are already routine practice for both scenarios. Application of AI-DP to these scenarios should be at least as informative for M&E purposes as the currently used standard procedure.
3.1.2 Target population
The KK stool thick smear procedure is dependent on the availability of a stool sample from the individuals residing in the surveyed area. Obtaining a stool sample for the KK stool thick smear from adults and non-enrolled school-age children is more difficult than from school-age children, however there are no implications for an AI-DP system other than ensuring that demographic data can include all ages.
3.1.3 Lowest infrastructure level
An AI-DP solution should come as a ready-to-use system. This means that in principle all components to make the system operational should be made available when the system is installed. The lowest infrastructure level for an ideal AI-DP solution is therefore seen as a remote area without laboratory infrastructure. The dependency on stable electricity and internet connectivity might push the technology into next level of infrastructure. Given the limited access to internet services in remote settings, the lowest infrastructure levels will require the AI-DP solution to generate all results on site without reliance on cloud computing.
3.1.4 Lowest level of user
An AI-DP solution will require properly trained users for preparation of KK stool thick smears and operation of the AI-DP system.
3.1.5 Training requirements
For proper use of the AI-DP solution, at least the following training modules will be needed and required: system setup-up and pack-down, system operation, system troubleshooting, system maintenance, result analysis and interpretation (including verification of the AI-selected features and training on recognising features from digitalized images), and data management best practices.
3.2 Design
3.2.1 Portability
For point-of-sampling tests in remote locations (ideal TPP), an AI-DP solution will need to be transported in a suitable transport case that protects the equipment from shock and vibration, water, humidity, temperature and dust ingress. The level of protection for an AI-DP solution may require a higher standard compared to traditional light microscopes. The size of the transport case should take into consideration travel ease internationally and domestically. Typically, in aviation, a maximum weight permissible for a single piece of baggage is 32 kg, and the sum of the 3 dimensions of the box must not exceed 158 cm (28). This will also permit equipment to be transported in a vehicle. Envisioning the use-case scenario at the lowest infrastructure level, the components inside the transport case could include the following: WSI scanner(s), AI processing unit (could be built into scanners, standalone, or computer based), a handheld device for verification of AI-detected helminth eggs (e.g., laptop, table or mobile phone), a battery and/or power management system to support 8 hrs operation (however, power generation equipment such as diesel, solar or wind generators are not expected to be transported within the transport case), a portable label maker for data management, an internet connected device for final result upload (e.g., a 4G modem or mobile phone), and cables and chargers for each component where required.
3.2.2 Instrument/power requirements
Independent of the use case scenario, AI-DP solutions will require power for the laptops and/or tablets, tablets supporting internet connectivity, label printers, WSI scanners and AI processing units. For laboratory use case scenario, the mains electricity can be assumed. However, to protect scanning activities in case of power outages, an uninterruptable power supply is needed (can either be internal to or external to the product). For field use case scenarios without connection to a power grid, alternative energy sources like solar power, or diesel/petrol generator could be considered. A battery and uninterruptable power supply should also be used to stabilize intermittent loads during operation in laboratory and field conditions. In the lowest infrastructure settings, the ideal requirement for the system is at least 8 hrs of operation on battery, while as a minimum requirement the system must support operation from portable power sources (e.g., portable diesel generator, portable solar setup, portable wind turbine or car charger).
AI determinations are provided by processing thousands of microscopic field-of-views (FOVs) per KK stool thick smear, the total compressed image data typically exceeding 1 GB in size (29–32). In laboratory settings, the transfer of images for AI processing with cloud services is considered time and cost prohibitive due to the quantity and size of the image data generated, while in the lowest infrastructure areas, internet connection is not guaranteed. Therefore, the AI-DP system cannot depend on the availability of the internet connection for result generation. The AI-DP system will need to store the image data locally, process the images locally with an AI model, and finally transmit the verified result data (e.g., quantification of eggs per slides or EPG) to the health care systems when an internet connection is established.
3.2.3 Water requirement
The KK stool thick smear and the desk cleaning might require water depending on the existing standard operating procedures (SOPs), but the AI-DP procedure by itself should not require any water. Occasionally, when the system gets contaminated by stool or reagents used in the KK thick smear preparation, cleaning water might be required.
3.2.4 Maintenance and calibration
As AI-DP solutions involve electrical equipment in mobile settings, the system will require return shipment to the manufacturer or locally certified repair centres in cases of failure or systematic errors. Equipment beyond a life expectancy of standard electrical equipment should not be serviced for repair but replaced. Calibration will be necessary to have a correct positioning of the KK stool thick smear in the scanning equipment. An on-board calibration procedure should be installed to monitor the correct surface scanning of the stool smear. Calibration on the focus depth should be included. Calibration procedures for AI models and quantification algorithms should be included (e.g., control and negative samples with a known number of eggs).
3.2.5 Sample type/collection
Tests are performed on KK stool thick smears. The SOPs to prepare the stool thick smears (33–35) should be strictly followed because the AI-DP process of scanning and AI-interpretation is fully dependent on the preparation of the KK stool thick smear. The stool thick smear preparation is outside of the AI-DP system.
3.2.6 Sample preparation/transfer device
Materials and reagents for KK stool thick smear preparations are not included in the AI-DP system. Sample collection is done by the study coordinator using the SOPs. For the data entry of the study design and participant information, the following should be considered: a tablet/mobile app to collect study information, including but not limited to a unique identifier (ID) for the study, a participant ID (not name) and participant demographic data (e.g., school/community, age and sex); a QR code containing the slide ID is then generated. The QR codes are printed and attached to the stool sample collection/transfer container and to the microscope glass slides which are prepared with the stool samples having the corresponding QR code. All QR codes also require human readable labels, typically containing the study ID, participant ID and an optional stool thick smear reference (e.g., A or B in case of duplicate KK stool thick smears). Additional QR labels can optionally be used for traceability on paper forms.
3.2.7 Sample quantity
The KK thick stool smear preparation is not part of the AI-DP system, but the WHO SOP should be followed. The preparation of the KK stool thick smear is done on filtered stool (mesh size of 200 μm). Only a very small amount of stool (41.7 mg) is used for each KK stool thick smear.
3.2.8 Target analyte
The biomarker used in KK stool thick smear-based AI-DP is the helminth egg. There is no direct parameter to measure STH morbidity (36). Since it is impossible to measure the exact number of worms in a host, the relationship between the number of worms and morbidity remains elusive. However, there is a relationship between the number of worms and the number of eggs in stool (37), although this relationship is not always strong, and has many weaknesses (egg production is not constant, the presence of eggs in stool is not homogeneous (38)). In absence of any better morbidity measurement, expressing fecal egg counts in EPG, remains the best proxy for morbidity.
3.2.9 Type of analysis
The AI-DP principles assume that AI is capable of detecting, classifying and quantifying egg-like features. Quantifying egg-like features by AI allows to classify the infection intensity over the complete range, from no infection, to low, moderate and heavy intensity infection. This can be done for A. lumbricoides, T. trichiura and hookworm (differentiation between two hookworm species based on the morphology on eggs is impossible), and any other helminth species that can be found in stool. This capability of the AI component introduces the multiplexing capacity within the same sample for each worm egg that has been trained with. Automated analysis of the AI detection results generates a quantitative output (expressed as EPG) for all targeted helminth species, but at the same time generates a semi-quantitative output of the individual infection as either one of the following of the three levels of intensity no, low, OR moderate-to-heavy intensity infection.
3.2.10 Detection
Digitalizing a KK stool thick smear may result in >1,000 FOVs. Due to the thickness of KK smears, every FOV may require the collection of multiple focal planes stored as a focus stack allowing users to observe features in different focal planes during review of the identified helminth eggs. Image contrasts for each FOV image in the focus stack should be sufficiently clear to allow visual inspection of the egg-like features independent of the stool consistency (Bristol stool chart) and clearing time (i.e., between 30 to 60 min for hookworm, and 24 hr for all other helminth species). A digital zoom function should be available to inspect features at a higher magnitude without significant loss in granularity (image pixelation). Every FOV should be presented with a micrometre scale to allow deduction of the size range of the selected egg-like feature. The scale is adapted to the digital zoom function.
3.2.11 Quality control
Quality control for the AI-DP solution should include the following: (i) storage of each FOV until they are removed by the study coordinator; (ii) intrinsic quality control of the AI-detected egg-like features by means of visual verification (e.g., a tablet based application to visually observe the size, shape and appearance of the feature); (iii) for every egg-like feature presented to the operator for verification, the operator has ability to overrule the AI-based detection and classification; (iv) a tool to label helminth eggs that were missed by the AI model; (iv) in case of visual inspection of eggs by multiple operators, the software should allow for conflict resolution when discrepancies occur.
3.2.12 Supplies needed
The reagents to prepare a KK stool thick smear are not included. All technology components for the AI-DP solution should be included in the equipment travel box (see section 3.2.1. Portability).
3.2.13 Safety
KK stool thick smears preparations are prepared according to WHO SOPs, including all safety precautions. The normal use of the AI-DP system should not create any personal, environmental or biosafety hazards. Devices making connection to a governmental network should use encryption.
3.3 Performance
3.3.1 Species differentiation/detection
The detection of the target species (A. lumbricoides, T. trichiura, and hookworm (A. duodenale and N. americanus) is dependent on the availability of samples, as the performance of the AI model depends on the availability of representative and appropriate data and variables being included in the AI training. The variables include: stool composition (colour, density, liquidity, food components and environmental (e.g., microparticles (silica) and pollen (39)), variability in egg morphology (e.g., fertile vs. infertile eggs, corticated vs. decorticated eggs, embryonated vs. non-embryonated eggs), geographical location (e.g., Trichuris vs. Capillaria spp (40).
The AI module used for egg-like feature detection should be validated on a reference set, being a large collection of images that contain ground truth labels. The following parameters are important to consider: (i) a large collection (e.g., >10,000 images) collected from a large participant sample size (e.g., >500 participants with different scores on the Bristol stool scale) from several regions (e.g., >2 different countries where diets may differ) for each target species; (ii) an analytical analysis of the AI model on the ground truth should result in a precision (true positive (TP)/(TP + false positive (FP))) and a recall (TP/(TP + false negative (FN))) of > 95% as minimum desired performance and >98% for ideal performance). This should be demonstrated on an unseen image set from the ground truth database of at least 10% (e.g., at least 1,000 eggs) for each species.
Beside STH, the multiplexing capacity of KK technique should allow to detect, classify and enumerate other parasite features in stool, including but not limited to Schistosoma mansoni, S. japonicum, S. mekongi, and S. intercalatum, Fasciola hepatica, Taenia spp, and Hymenolepis spp. The same validation processes for these helminth species apply as for the STH species.
3.3.2 Diagnostic/clinical sensitivity
As a benchmark, manual reading of single KK stool thick smear results in a clinical sensitivity for Ascaris of 71.9%, for Trichuris 88.1%, and for hookworms 72.6% at any intensity level, but this is markedly less for the low infection intensity levels (respectively 55.6%, 79.6%, and 69.4%) (41, 42). Since examination of a single KK stool thick smear has a limit of detection (LOD) of 24 EPG (= 1/0.0417 gram), it is anticipated that screening a duplicate KK stool thick smear will result in a LOD of 12 EPG (= 1/(2 x 0.0417 gram)), and therefore it is expected that clinical sensitivity of at least >60% should be reached for all helminth species.
The AI to be evaluated for clinical sensitivity should have a minimum recall of >95% (see above in 3.3.1) to ensure almost all eggs are detected. Sensitivity testing should be completed by scanning KK stool thick smears in field setting. The smears should be freshly prepared from a stool sample that was not used for the training of the AI model. Using the manual procedure (reference method), the total amount of egg-like features should be determined and compared to the AI- predicted true positive features. This should be completed in a head-to-head comparison for all helminth species and over the no, low and moderate-to-heavy infection intensity levels. Sensitivity results should be equal or better than the reference method procedure. In order to demonstrate the clinical sensitivity of >60%, sufficient samples should be tested to reach statistical significance for the sensitivity claim.
3.3.3 Diagnostic/clinical specificity
As a benchmark, the manual examination of a single KK stool thick smear results in a clinical specificity of >95% for all STHs and levels of intensity, irrespective of the intensity level (43). AI models evaluated for clinical specificity should have a precision of >95% on the ground truth, and preferably >98% (see above in 3.3.1). Specificity testing should be completed by scanning KK stool thick smears in field setting. The smears should be freshly prepared from a stool sample that was not used for the training of the AI model.
Using the manual procedure (reference method), the total amount of egg-like features should be determined and compared to the AI-predicted TP and FP features. This should be completed in a head-to-head comparison for all parasites and over the no, low and moderate-to-heavy intensity infection levels. Specificity is determined by the total number of FP in the prediction model. FP may appear as a consequence of several independent factors (e.g., egg-like artefacts such as pollen, stool particles, or out of focus artefacts). The FPs number is determined by the learning process. In order to reduce the number of FP to below an acceptable range (e.g., less than 5% of all predictions = 95% specificity), a large effort in evaluating all different stool samples from different geographical areas should be included. The WSI optical settings (e.g., magnification, depth of focus, light brightness and iris diaphragm aperture) and control algorithms (e.g., focus range and FOV overlap) should be optimised to reduce the number of FPs.
With specificity being considered the most important parameter (44, 45), there may be a need to further validate the AI-generated detections to bring the specificity above 95%. In order to do so, a hybrid AI/human review software tool should be made available to remove FP from the final result. The final call will be made by human operators, who are trained laboratory technicians/technologists. As an example, for heavy intensity infections of:
A. lumbricoides: Heavy intensity infection: >50,000 EPG or > 2085 eggs per slide (41.7 mg KK stool thick smear); a 5% FP rate would require the operator to decide on a total of 2,189 eggs (of which 104 are negative (non-egg) features);
T. trichiura: Heavy intensity infection: >10,000 EPG or >417 eggs per KK stool thick smear; a 5% FP rate would require the operator to decide on a total of 438 eggs (of which 21 ARE negative features);
Hookworm: Heavy intensity infection: >4,000 EPG or > 167 eggs per KK stool thick smear; a 5% FP rate would require the operator to decide on a total of 175 eggs (with 8 negative features).
Under the assumption that any slide can contain heavy intensity infections of all parasites at the same time, the total sum of eggs to be reviewed with a 5% FP rate would be 2,802 eggs (with 133 negative features). This software tool should be designed such that decision making on presented features is intuitive, simple and fast. The curation of the list of predictions should not significantly increase the time of making the final determination. As a final test, egg-negative stool samples from healthy individuals analysed using this hybrid AI/human software program should not result in verified egg features. This hybrid AI/human decision software should bring the specificity to >99%.
3.3.4 Time to results
As a benchmark, a highly accurate microscopist can read an average of 80 slides in an 8-hour working day (with the expected benchmark sensitivity and specificity), and excluding data entry.
Setup of the system at the lowest infrastructure level should be less than 20 min by trained personnel. Both the data entry and initiation of the KK stool thick smear should be completed in less than 10 min/smear. Scanning of a single smear should not take longer than 20 min. The interpretation of the AI predictions could be done locally in real-time, or at the end of the day (a batch analysis), or at the end of the study. This should not take more than 5 min/smear. Trained personnel should be able to set-up and pack-down within less than 20 min. The total turn-around-time (from setting-up the system to data-report available) for one single KK stool thick smear at the lowest infrastructure level should be less 90 min. Turn-around times should become gradually less when more slides need to be scanned within one experiment. The AI-DP system time-to-result should be equal or better as compared to the benchmark.
3.3.5 Result stability
Scanned KK thick smears are stored locally on a hard drive. Images should be transferrable to an external storage medium (e.g., portable hard drive or cloud server). Images should be stored until the study manager decides that the interpretation is completed, and the images have no further value to the study.
3.3.6 Throughput
A typical survey might include up to 100 smears/day/geographical area. The AI-DP system should allow to complete the scanning procedure in a time frame that is comparable or better than the standard manual procedure. Knowing that the scanning procedure is the limiting step, and to support this turn-around-time, there should be a possibility to connect multiple scanners in parallel to achieve the desired throughput. Storing and AI processing should not interrupt or delay the scanning procedures when multiple scanners are used. The following scenario needs to be considered: one scanner is capable of scanning 4 smears/hr (15 min/scan). An 8-hour scanning operation day would allow to digitalize 32 smears. A survey having 3 scanners would provide a throughput capacity of 96 slides including result verification. The flexibility of the AI-DP configuration allows for cost-effective scalability of throughput.
3.3.7 Target shelf-life/stability
The AI-DP system should have no difference in shelf-life or stability from that of standard computers, modems, and power supplies.
3.3.8 Ease of use
The AI-DP system should be seen as a sample-in and result-out tool, with a minimal number of steps to load and unload the scanner. The egg verification tool should be a graphical user interface software visualizing the scanned image, the AI interpretation, the support tools, and the decision-making option. An optimal user experience with minimal steps for egg verification is needed. Data transfer from the equipment to the health care system data warehouse should be simple, intuitive, and not more than ten or fewer steps.
3.3.9 Ease of results interpretation
The interpretation should be aided by the AI tool. The AI tool presents features to the end user, preferably a trained laboratory technician/technologist, that can make an interpretation of each of the presented features. Interpretation should be facilitated by the availability of the reference card, a micrometre scale, a digital zoom, and a focus adjustment. The AI tool should indicate a confidence level of the prediction. Presentation of features for end-user decision should be configurable by decreasing or increasing confidence values. The AI tool and supporting software should indicate when a threshold between low and moderate infection intensity is crossed. The operator should have the decision power to stop the validation once a threshold is crossed. A summary report should then be prepared by the AI-DP system, so that the report can be reviewed on the system, and finally transmitted to the health care system data warehouse.
3.3.10 Operating temperature
The AI-DP system should be operational at ambient temperature and relative humidity.
3.4 Product configuration
3.4.1 Shipping conditions
The AI-DP system might be seen as a medical device, and shipping conditions should comply with international and national regulations for shipment of medical devices. Several components of the slide scanner are mobile parts and should be protected from damages introduced by overland transportation. For AI-DP there is no requirement for cold-chain. Considerations for dimensions of a storage and shipping container are discussed in 3.2.1.
3.4.2 Storage conditions
The AI-DP system should be stored at ambient temperature and relative humidity.
3.4.3 Service and support
The AI-DP system should log diagnostic data to support service and maintenance of the devices. Remote support should be available.
3.4.4 Waste disposal
AI-DP is seen as a hardware device. Non-functional devices (broken/damaged) should be either sent back to the manufacturer or locally destroyed according to country-specific government regulations for electronic equipment. Waste disposal of the KK stool thick smear falls outside the AI-DP system and should be disposed following institutional biosafety regulations.
3.4.5 Labelling and instructions for use
AI-DP should meet compliance per CE/In Vitro Diagnostic Regulation and WHO prequalification guidance if required. AI-DP should include product insert in relevant local language(s) and shall include instructions for use for the test.
3.5 Product cost and channels
3.5.1 Target pricing per test
The cost to prepare a KK stool thick smear should be similar to that of the reference method, which is at max around US$0.5, with the reported cost of performing a duplicate KK test varying between 2.67 and 12.48 US$ (46, 47). In AI-DP, cost/test is comprised on KK standard test materials, personnel costs, depreciation of WSI, and AI accessory materials:
Cost per test on the WSI equipment: As an example: 4 scans/hr x 8 hr/day and 150 operational days per year, and a total operational time of 2 years will result in 9,600 scans during the minimum expected lifetime of a WSI scanner. With a capital cost of max $5,000 per WSI scanner, this would result in US$0.52 per test performed to cover the capital costs of the equipment. This will bring the target price to the ideal <US$1 target, not including the strongly reduced time of the field operator, and hence the costs for salary.
3.5.2 Capital cost
AI-DP devices for STH needs proper design choices to make the technology solution affordable and reliable in use case environments. A market price of US$5,000 for a complete system (containing 3 WSI scanners to meet desired throughput) in a transportation box is challenging. Therefore, the final configuration for the AI-DP solution might be very different in design than currently available commercial WSI equipment and cloud-based AI platforms.
3.5.3 Product lead times
Lead times for consumables are limited to those necessary to perform the KK technique, which is considered outside the scope of an AI-DP system.
3.5.4 Target launch countries
Countries prioritized in consultation with WHO.
3.5.5 Product registration
The system must be of assured quality, but the level of regulatory and WHO pre-qualification oversight remains unclear for an AI-DP system. Epidemiological research is not dependent on the new regulation In Vitro Diagnostic Medical Devices Regulation (IVDR) EU 2017/746 (48). Epidemiological research has no medical application as intended under the regulation. The regulation introduces the classification into risk classes A, B, C, and D. An AI-DP tool for STH epidemiology might be seen as risk class B. Another approach can be taken via the research use only (RUO) label. The consequences of using an RUO label is that there is no need to follow the regulatory controls (49), but other directives might be in place for example electro-magnetic exposure, hazardous substances and waste. Other regulations to consider for an AI-DP solution include the General Data Protection Regulation (GDPR) and the proposed Artificial Intelligence Act by the European Parliament and of the Council of the EU.
Real world data may potentially be used as some - or all - of the evidence necessary for understanding the medical device performance at different points in the product life cycle. In many cases, an approved investigational device exemption (IDE; investigational use only, exempt from IVDR requirements) is required before initiating a clinical investigation. An investigation is defined as a clinical investigation or research involving one or more subjects to determine the safety or effectiveness of a device.
However, for treatment decisions the technology should be developed according to the IVDR, and the pre-qualification process of WHO if applicable, as a low-risk device (class B). Manufacturing following ISO 13485 is necessary for medical devices having an IVD label. Depending on the interpretation of the technology, the software and AI model might be seen as a software as a medical device. Under the EU regulatory framework it would not be covered by the Medical Devices Regulation so long as it is not a standalone software and it is not used for treatment of individual patients (50, 51).
4 Discussion
To complement the launch of the WHO 2030 roadmap, explorations are ongoing by our group and many others to present new diagnostic tools to confirm the reduction or elimination of STH and SCH in both control and elimination programs. Currently, the reference method remains the manual examination of KK stool thick smears, although several research activities are ongoing to identify new biomarkers for infection and/or morbidity, and conversion of these biomarkers into diagnostic technologies.
The monitoring of the impact of the drug donation programs is another key aspect requiring a more focused exploration for new diagnostic tools. Drug donations may vary between countries and from situation to situation. Therefore, drug donations must be based on a sound analysis of the needs, and their selection and distribution must fit with existing drug policies and administrative purposes (52). The recipient of the donated drugs (endemic countries) needs to specify the needs for donated drugs as much as possible, including the required quantities and prioritizations. As is visualized in the online maps, the impact of the interventions on the STH prevalence is limited, for the majority part because evaluations are either not done, results of evaluations are not available, or the data are completely absent (53).
For all these reasons, the importance for increasing the diagnostic test armamentarium is imperative, and the guidance in the WHO TPPs are crucial to guide test development. Previously, we have argued that the new test development in support of the 2030 roadmap is largely hampered by either lack of readily available biomarkers, or lack of funding to initiate the discovery and/or development process (19). In our assessment, the KK technique is currently fit for purpose, but the method should be improved with digitalised and automated data capture, analysis and reporting.
Based on our observations with the previously published AI-DP prototype (29), we have provided design considerations for an AI-DP workflow that builds on the existing KK stool thick smear procedure. The STH TPP was used as a starting document as we complete the URS for each TPP item. Following this description, we will try to implement the requirements into our ongoing research and development of an AI-DP system.
An additional comment could be introduced related to the mansoni detection in KK stool thick smears. The WHO TPP for SCH mentions that the desire is to avoid testing reliant on stool or venous blood. The most obvious candidates are urine or finger-stick blood; while other non-stool, non-venous blood samples (e.g., saliva, breath) would also be acceptable if they meet test performance criteria. However, for the purposes of AI-DP on KK stool thick smears, the URS are equally relevant for stool-based egg detection of S.mansoni. S. haematobium filters made with filtration technique can also be read. The slide is quickly made from a total volume of 10 ml of urine. Glycerine or mounting medium may be added to the egg containing filter membranes on a glass slide, allowing later examination. Hence this equipment should not be limited to KK for STH and S. mansoni but should include filtration technique for S. haematobium too (54, 55). The capability of the AI component introduces the multiplexing capacity within the same sample for each determinant that it has been trained with. Automated analysis of the AI detection results generates a quantitative output (EPG or eggs per 10 mL of urine) for all targeted species, but at the same time generates a semi-quantitative output of the individual infection as either one of the following “no/low/moderate/high” intensity of infection.
In conclusion, this document proposes a translation from the WHO TPP towards concrete guidelines for AI-DP design and development when shifting from a manual examination of the KK stool thick smear towards application of AI-DP. We systematically show how such solution fits within the envelope of the WHOs diagnostic TPP for M&E of STH control programmes.
Furthermore, our considerations for AI-DP may be applicable to many other NTDs where microscopic visual examination is already routine practice.
Data availability statement
The original contributions presented in the study are included in the article/supplementary material. Further inquiries can be directed to the corresponding author.
Author contributions
PW and PD: contributed the design requirements from a hardware configuration approach. LB and KO: evaluated the design requirements from a programmatic approach. PW, PD, and SH: evaluated the software and AI requirements. PW, GL, ZM, BN, NK, BL: evaluated the requirements from a country perspective, in Uganda and Ethiopia, from a centralized lab perspective, and as a field-based test. AB and MG: evaluated the requirements from a technology, access approach, and stakeholder interactions. MG and LS: evaluation of the complete program design, and program supervision. PW, BL, and LS: concept, original design, writing, editing and finalizing the manuscript. All authors contributed to the article and approved the submitted version.
Funding
The authors declare that this study received funding from Johnson & Johnson Foundation (grant agreement No 66552075) and Merck as a consortium activity (see AI4NTD.org). The funder was not involved in the study design, collection, analysis, interpretation of data, the writing of this article, or the decision to submit it for publication.
Acknowledgments
This study is part of the R&D activities of the AI4NTD Consortium, https://www.AI4NTD.org.
Conflict of interest
Authors PW, PD were employed by Etteplan Sweden Akitebolag (AB). Author LAB was employed by Akkodis. Author MG was employed by company Merck Global Health Institute. Author LJS was employed by Janssen Global Public Health.
The remaining authors declare that the research was conducted in the absence of any commercial or financial relationships that could be construed as a potential conflict of interest.
Publisher’s note
All claims expressed in this article are solely those of the authors and do not necessarily represent those of their affiliated organizations, or those of the publisher, the editors and the reviewers. Any product that may be evaluated in this article, or claim that may be made by its manufacturer, is not guaranteed or endorsed by the publisher.
Abbreviations
AI-DP, artificial intelligence-digital pathology; DDS, detailed design specification; EPG, eggs per gram stool; FDA, food and drug administration; GDPR, General Data Protection Regulation; IVDR, In Vitro Diagnostic Medical Devices Regulation; KK: Kato-Katz; FOV, field of view; M&E, monitoring and evaluation; NTDs, neglected tropical diseases; PC, preventive chemotherapy; RUO, research use only; SOP, standard operating procedure; STH, soil-transmitted helminths; TPP, target product profile; URS, user requirement specification; WHO, World Health Organization; WSI, whole slide imaging. IDE, investigational device exemption; SCH, Schistosoma; TP, true positive; FP, false positive; FN, fasle negative; PRS, product requirement specification; EDC, electronic data capturing; ID, identifier; LOD, limit of detection.
References
1. World Health Organization. Ending the neglect to attain the sustainable development goals – a road map for neglected tropical diseases 2021–2030. (Geneva: World Health Organization) (2021). Available at: https://www.who.int/publications/i/item/9789240010352.
2. World Health Organization. Target product profile for a rapid test for diagnosis of buruli ulcer at the primary health-care level. (Geneva: World Health Organization). (2022). Available at: https://www.who.int/publications/i/item/9789240043251.
3. World Health Organization. Target product profile for a point-of-care diagnostic test for dermal leishmaniases (2022). (Geneva: World Health Organization). Available at: https://www.who.int/publications/i/item/9789240045224.
4. World Health Organization. Target product profile for a test for rhodesiense human African trypanosomiasis diagnosis usable in peripheral health facilities. (Geneva: World Health Organization). (2021). Available at: https://www.who.int/publications/i/item/9789240029378.
5. World Health Organization. Target product profile for a gambiense human African trypanosomiasis test to identify individuals to receive widened treatment. (Geneva: World Health Organization). (2022). Available at: https://www.who.int/publications/i/item/9789240043299.
6. World Health Organization. Diagnostic test for surveillance of lymphatic filariasis target product p rofile (2021). (Geneva: World Health Organization). Available at: https://www.who.int/publications/i/item/9789240018648.
7. World Health Organization. Diagnostic test for lymphatic filariasis to support decisions for stopping triple-therapy mass drug administration: target product profile (2021). Available at: https://www.who.int/publications/i/item/9789240018624.
8. World Health Organization. Target product profile for a rapid test for diagnosis of mycetoma at the primary health-care level. (Geneva: World Health Organization). (2022). Available at: https://www.who.int/publications/i/item/9789240047075.
9. World Health Organization. Onchocerciasis: diagnostic target product profile to support preventive chemotherapy. (Geneva: World Health Organization). (2021). Available at: https://www.who.int/publications/i/item/9789240024496.
10. World Health Organization. Target product profile for scabies to start and stop mass drug administration. (Geneva: World Health Organization). (2022). Available at: https://www.who.int/publications/i/item/9789240045026.
11. World Health Organization. Diagnostic target product profiles for monitoring, evaluation and surveillance of schistosomiasis control programmes. (Geneva: World Health Organization). (2021). Available at: https://www.who.int/publications/i/item/9789240031104.
12. World Health Organization. Diagnostic target product profile for monitoring and evaluation of soil-transmitted helminth control programmes. (Geneva: World Health Organization). (2021). Available at: https://www.who.int/publications/i/item/9789240031227.
13. World Health Organization. Target product profile for the detection of a case of yaws and the detection of azithromycin resistance. (Geneva: World Health Organization). (2022). Available at: https://www.who.int/publications/i/item/9789240045743.
14. Food and Drug Administration Center for Devices and Radiological Health (1997). Design control guidance for medical device manufacturers. In: Design history file (1997) Rockville): Food and Drug Administration). Available at: https://www.fda.gov/media/116573/download.
15. Niazi MKK, Parwani AV, Gurcan MN. Digital pathology and artificial intelligence. Lancet Oncol (2019) 20(5):e253–61. doi: 10.1016/S1470-2045(19)30154-8
16. FDA Allows marketing of first whole slide imaging system for digital pathology | FDA (2017). Available at: https://www.fda.gov/news-events/press-announcements/fda-allows-marketing-first-whole-slide-imaging-system-digital-pathology.
17. FDA Clears leica biosystems’ digital pathology system | 2019-06-04 | FDANews . Available at: https://www.fdanews.com/articles/191523-fda-clears-leica-biosystems-digital-pathology-system.
18. O’Meara WP, Barcus M, Wongsrichanalai C, Muth S, Maguire JD, Jordan RG, et al. Reader technique as a source of variability in determining malaria parasite density by microscopy. Malar J (2006) 5:1–7. doi: 10.1186/1475-2875-5-118
19. Stuyver LJ, Levecke B. The role of diagnostic technologies to measure progress toward WHO 2030 targets for soil-transmitted helminth control programs. PloS Negl Trop Dis (2021) 15(6):e0009422. doi: 10.1371/journal.pntd.0009422
20. World Health Organisation. Soil-transmitted helminth infections (2020). Available at: https://www.who.int/news-room/fact-sheets/detail/soil-transmitted-helminth-infections.
21. Montresor A, Mupfasoni D, Mikhailov A, Mwinzi P, Lucianez A, Jamsheed M, et al. The global progress of soil-transmitted helminthiases control in 2020 and world health organization targets for 2030. PloS Negl Trop Diseases. (2020) 14:1–17. doi: 10.1371/journal.pntd.0008505
22. World Health Organization. 2030 targets for soil-transmitted helminthiases control programmes (2020). Available at: https://www.who.int/publications/i/item/9789240000315.
23. World Health Organization. Guidelines for the evaluation of soil-transmitted helminthiasis and schistosomiasis at community level: A guide for managers of control programmes. (Geneva: World Health Organization). (1998). Available at: https://apps.who.int/iris/handle/10665/63821.
24. World Health Organization. Diagnostic methods for the control of strongyloidiasis virtual meeting. (Geneva: World Health Organization). (2020). Available at: https://apps.who.int/iris/rest/bitstreams/1336813/retrieve.
25. Steinmann P, Zhou XN, Du ZW, Jiang JY, Wang LB, Wang XZ, et al. Occurrence of strongyloides stercoralis in yunnan province, China, and comparison of diagnostic methods. PloS Negl Trop Dis (2007) 1(1):e75. doi: 10.1371/journal.pntd.0000075
26. Utzinger J, Becker SL, Knopp S, Blum J, Neumayr AL, Keiser J, et al. Neglected tropical diseases: Diagnosis, clinical management, treatment and control. Swiss Med Wkly (2012) 142:19–22. doi: 10.4414/smw.2012.13727
27. Knopp S, Mgeni AF, Khamis IS, Steinmann P, Stothard JR, Rollinson D, et al. Diagnosis of soil-transmitted helminths in the era of preventive chemotherapy: Effect of multiple stool sampling and use of different diagnostic techniques. PloS Negl Trop Dis (2008) 2(11):e33. doi: 10.1371/journal.pntd.0000331
28. International Air Transport Association. IATA - passenger baggage rules (2022). Available at: https://www.iata.org/en/programs/ops-infra/baggage/check-bag/.
29. Ward P, Dahlberg P, Lagatie O, Larsson J, Tynong A, Vlaminck J, et al. PLOS neglected tropical diseases affordable artificial intelligence-based digital pathology for neglected tropical Diseases : a proof-of-Concept for the detection of soil-transmitted helminths and schistosoma mansoni eggs in kato-Katz stool thick smears. PloS Negl Trop Dis (2022) 16(6):e00. doi: 10.1371/journal.pntd.0010500
30. Yagi Y, Yoshioka S, Kyusojin H, Onozato M, Mizutani Y, Osato K, et al. An ultra-high speed whole slide image viewing system. Anal Cell Pathol (2012) 35:65–73. doi: 10.1155/2012/626025
31. Hanna MG, Reuter VE, Hameed MR, Tan LK, Chiang S, Sigel C, et al. Whole slide imaging equivalency and efficiency study: experience at a large academic center. Mod Pathol (2019) 32:916–28. doi: 10.1038/s41379-019-0205-0
32. Zarella MD, Bowman D, Aeffner F, Farahani N, Xthona A, Absar SF, et al. A practical guide to whole slide imaging: A white paper from the digital pathology association. Arch Pathol Lab Med (2019) 143(2):222–34. doi: 10.5858/arpa.2018-0343-RA
33. World Health Organization. Bench aids for the diagnosis of intestinal parasites, second edition. (Geneva: World Health Organization). (2019). p.32. Available at: https://www.who.int/publications/i/item/9789241515344.
34. Demeulenaere D. Tutorial kato-Katz thick smear (2013). Available at: https://www.youtube.com/watch?v=WpcZejHa_jM.
35. DeWorm3. Kato-Katz preparation and analysis . Available at: https://depts.washington.edu/deworm3/wordpress/wp-content/uploads/2020/09/DeWorm3_SOP_707.-Kato-Katz-preparation-and-analysis-V1.pdf.
36. Campbell SJ, Nery SV, McCarthy JS, Gray DJ, Soares Magalhães RJ, Clements ACA. A critical appraisal of control strategies for soil-transmitted helminths. Trends Parasitol (2016) 32(2):97–107. doi: 10.1016/j.pt.2015.10.006
37. Malizia V, Giardina F, de Vlas SJ, Coffeng LE. Appropriateness of the current parasitological control target for hookworm morbidity: a statistical analysis of individual-level data. PloS Negl Trop Dis (2022) 16(6):e00. doi: 10.1371/journal.pntd.0010279
38. Krauth SJ, Coulibaly JT, Knopp S, Traoré M, N’Goran EK, Utzinger J. An in-depth analysis of a piece of shit: Distribution of schistosoma mansoni and hookworm eggs in human stool. PloS Negl Trop Dis (2012) 6(12):e1969. doi: 10.1371/journal.pntd.0001969
39. Camacho M, Reinhard KJ. Confusing a pollen grain with a parasite egg: An appraisal of “paleoparasitological evidence of pinworm (enterobius vermicularis) infection in a female adolescent residing in ancient tehran. ” Korean J Parasitol (2019) 57(6):621–5. doi: 10.3347/kjp.2019.57.6.621
40. Suwandittakul N, Mungthin M, Kuntawong K, Laojun S, Pimsuka S, Chaiphongpachara T. A novel use of a geometric morphometric technique to distinguish human parasite eggs of twelve different species. Exp Parasitol (2022) 238:108281. doi: 10.1016/j.exppara.2022.108281
41. Speich B, Ali SM, Ame SM, Albonico M, Utzinger J, Keiser J. Quality control in the diagnosis of trichuris trichiura and ascaris lumbricoides using the kato-Katz technique: Experience from three randomised controlled trials. Parasites Vectors. (2015) 8(1):1–8. doi: 10.1186/s13071-015-0702-z
42. Cools P, Vlaminck J, Albonico M, Ame S, Ayana M, Antonio BPJ, et al. Diagnostic performance of a single and duplicate kato-Katz, mini-FLOTAC, FECPAKG2 and qPCR for the detection and quantification of soil-transmitted helminths in three endemic countries. PloS Negl Trop Dis (2019) 13(8):1–22. doi: 10.1371/journal.pntd.0007446
43. Fenta A, Hailu T, Alemu M, Nibret E, Amor A, Munshea A. Evaluating the performance of diagnostic methods for soil transmitted helminths in the amhara national regional state, Northwest Ethiopia. BMC Infect Dis (2020) 20(1):1–8. doi: 10.1186/s12879-020-05533-2
44. Gass K. Time for a diagnostic sea-change: Rethinking neglected tropical disease diagnostics to achieve elimination. PloS Negl Trop Dis (2020) 14(12):1–6. doi: 10.1371/journal.pntd.0008933
45. Levecke B, Coffeng LE, Hanna C, Pullan RL, Gass KM. Assessment of the required performance and the development of corresponding program decision rules for neglected tropical diseases diagnostic tests: Monitoring and evaluation of soil-transmitted helminthiasis control programs as a case study. PloS Negl Trop Dis (2021) 15(9):e0009740. doi: 10.1371/journal.pntd.0009740
46. Worrell CM, Bartoces M, Karanja DMS, Ochola EA, Matete DO, Mwinzi PNM, et al. Cost analysis of tests for the detection of schistosoma mansoni infection in children in western Kenya. Am J Trop Med Hyg (2015) 92(6):1233–9. doi: 10.4269/ajtmh.14-0644
47. Turner HC, Bettis AA, Dunn JC, Whitton JM, Hollingsworth TD, Fleming FM, et al. Economic considerations for moving beyond the kato-Katz technique for diagnosing intestinal parasites as we move towards elimination. Trends Parasitol (2017) 33(6):435–43. doi: 10.1016/j.pt.2017.01.007
48. European Comission. In vitro diagnostic medical devices regulation (EU) 2017/746 (2017). Available at: https://ec.europa.eu/growth/single-market/european-standards/harmonised-standards/iv-diagnostic-medical-devices_en.
49. Johner Institute. Laboratory products for “Research use only” (RUO) – often a dangerous claim (2021). Available at: https://www.johner-institute.com/articles/regulatory-affairs/and-more/laboratory-products-for-research-use-only-ruo-often-a-dangerous-claim/.
50. Medical Device Coordination Group. MDCG 2019-11 guidance on qualification and classification of software. (Brussels: European Commission) (2019) p. 1–28. Available at: https://ec.europa.eu/docsroom/documents/37581/attachments/1/translations/en/renditions/native.
51. Directorate-General for Health and Food Safety. Is your software a Medical Device? (Brussels: European Commission). (2021). Available at: https://health.ec.europa.eu/latest-updates/infographic-your-software-medical-device-2021-03-23_en.
52. Health Product Policy and Standards, Medicines Selection, IP and Affordability. Guidelines for Medicine Donations Revised 2010 [Internet]. (Geneva: World Health Organization). (2011). Available at: https://www.who.int/publications/i/item/9789241501989.
53. World Health Organization Collaborating Centre for Diagnosis of Intestianal Helminths and Protozoa (2022) CREMOPAR l of p and PD. In: WHO collaborating centre for diagnosis of intestinal helminths and Protozoa. Available at: https://www.whocc.ita116.unina.it/.
54. Oyibo P, Jujjavarapu S, Meulah B, Agbana T, Braakman I, van Diepen A, et al. Schistoscope: An automated microscope with artificial intelligence for detection of schistosoma haematobium eggs in resource-limited settings. Micromachines. (2022) 13(5):643. doi: 10.3390/mi13050643
Keywords: soil-transmitted helminthiasis, neglected tropical diseases, artificial intelligence-based pathology, target product profiles, monitoring and evaluation
Citation: Ward P, Broadfield LA, Dahlberg P, Leta G, Mekonnen Z, Nabatte B, Kabatereine N, Brooks A, Orrling KM, Gomes M, Van Hoecke S, Levecke B and Stuyver LJ (2022) The development of an artificial intelligence-based digital pathology for neglected tropical diseases: A platform specific analysis of the World Health Organization diagnostic target product profile for soil-transmitted helminthiasis. Front. Trop. Dis 3:990304. doi: 10.3389/fitd.2022.990304
Received: 09 July 2022; Accepted: 07 November 2022;
Published: 20 December 2022.
Edited by:
Russ Russell Stothard, Liverpool School of Tropical Medicine, United KingdomReviewed by:
Chong Chin Heo, Universiti Teknologi MARA, MalaysiaPaul Erasto Kazyoba, National Institute for Medical Research (NIMR), Tanzania
Soeren Becker, Saarland University Hospital, Germany
Copyright © 2022 Ward, Broadfield, Dahlberg, Leta, Mekonnen, Nabatte, Kabatereine, Brooks, Orrling, Gomes, Van Hoecke, Levecke and Stuyver. This is an open-access article distributed under the terms of the Creative Commons Attribution License (CC BY). The use, distribution or reproduction in other forums is permitted, provided the original author(s) and the copyright owner(s) are credited and that the original publication in this journal is cited, in accordance with accepted academic practice. No use, distribution or reproduction is permitted which does not comply with these terms.
*Correspondence: Lieven J. Stuyver, bHN0dXl2ZXJAaXRzLmpuai5jb20=