- 1School of Ocean Science and Engineering, The University of Southern Mississippi, Ocean Springs, MS, United States
- 2Environmental Science and Policy Department, George Mason University, Fairfax, VA, United States
- 3Bureau of Ocean Energy Management, New Orleans, LA, United States
- 4Oceaneering International, Inc., Lafayette, LA, United States
- 5Naval Research Laboratory, Stennis Space Cente, Hancock County, MS, United States
Exposure to oil from the Deepwater Horizon spill may have lasting impacts on preservation of historic shipwrecks in the Gulf of Mexico. Submerged steel structures, including shipwrecks, serve as artificial reefs and become hotspots of biodiversity in the deep sea. Marine biofilms on submerged structures support settlement of micro- and macro-biota and may enhance and protect against corrosion. Disruptions in the local environment, including oil spills, may impact the role that biofilms play in reef preservation. To determine how the Deepwater Horizon spill potentially impacted shipwreck biofilms and the functional roles of the biofilm microbiome, experiments containing carbon steels disks (CSDs) were placed at five historic shipwreck sites located within, and external to the benthic footprint of the Deepwater Horizon spill. The CSDs were incubated for 16 weeks to enable colonization by biofilm-forming microorganisms and to provide time for in situ corrosion to occur. Biofilms from the CSDs, as well as sediment and water microbiomes, were collected and analyzed by 16S rRNA amplicon gene sequencing to describe community composition and determine the source of taxa colonizing biofilms. Biofilm metagenomes were sequenced to compare differential gene abundances at spill-impacted and reference sites. Biofilms were dominated by Zeta-, Alpha-, Epsilon-, and Gamma-proteobacteria. Sequences affiliated with the Mariprofundus and Sulfurimonas genera were prolific, and Roseobacter, and Colwellia genera were also abundant. Analysis of 16S rRNA sequences from sediment, water, and biofilms revealed sediment to be the main known source of taxa to biofilms at impacted sites. Differential gene abundance analysis revealed the two-component response regulator CreC, a gene involved in environmental stress response, to be elevated at reference sites compared to impacted sites within the spill plume fallout area on the seafloor. Genes for chemotaxis, motility, and alcohol dehydrogenases were differentially abundant at reference vs. impacted sites. Metal loss on CSDs was elevated at sites within the spill fallout plume. Time series images reveal that metal loss at a heavily impacted site, the German Submarine U-166, has accelerated since the spill in 2010. This study provides evidence that spill residues on the seafloor may impact biofilm communities and the preservation of historic steel shipwrecks.
Introduction
Biofilms form on surfaces in the marine environment when microorganisms adhere to solid substrates and develop a thin layer of cells and extracellular polymeric substances (EPS) (Qian et al., 2007). EPS provide stability, protection, nutrition, and recruitment cues to the microbiome, and promote the recruitment of macro-organisms on artificial reefs (Svane and Petersen, 2001). Biofilm resiliency, spatial organization, and interspecies interaction are determined by physical, biological, and chemical factors in the local environment (Garrett et al., 2008; Brauer et al., 2015). Accordingly, the dominant phylotypes in a biofilm are dictated by environmental conditions and the substrate to which they attach.
Historic shipwrecks provide substrate for attachment of marine biofilms. As the biofilm matures, fauna recruit to the shipwreck’s surface, creating an artificial reef that becomes a diverse community of organisms. Marine biofilm maturation in shallow water (less than 200 m) may occur rapidly (4–30 days) (Teitzel and Parsek, 2003; Acuña et al., 2006; Barraud et al., 2006; Bermont-Bouis et al., 2007; Nithya et al., 2010), although little is known about the formation of marine biofilms in the deep sea (McBeth and Emerson, 2016).
Carbon steel was used to construct World War II-era ships, and is still used in ship hull construction and other marine infrastructure (McBeth et al., 2011). Carbon steel is inexpensive, easy to cast, and has high tensile strength. However, due to its high iron content (99%), it is susceptible to corrosion. The corrosive effects of microbial biofilms on steel surfaces in shallow environments have been extensively studied (Little et al., 2008, and references therein). Microbial biofilms have been linked to ennoblement of corrosion potential and sulfide derivitization of metal resulting from the metabolism of sulfate-reducing bacteria (SRB) that leads to surface corrosion. Microbially-induced corrosion (MIC) is a biologically-mediated process by which conditions for corrosion at a metal surface are enhanced by bacteria in the environment (Cullimore and Johnston, 2008; McBeth et al., 2011). MIC couples corrosion and microbiological processes, yet the underlying mechanisms are poorly known (Little and Lee, 2014).
Metal surfaces including carbon steel are a source of Fe(II) ions, and serve as a substrate for iron-oxidizing bacteria (FeOB) (McBeth et al., 2011). FeOB form a symbiotic relationship with iron-reducing bacteria (FeRB) to use the metal surface as a source of energy. As a result, iron cycling occurs at the metal surface, which eventually leads to pitting (Lee et al., 2013). As oxygen is utilized in a biofilm and it shifts from an aerobic to anaerobic niche, SRB become the dominant phylotype in the MIC process. As SRB reduce sulfate to hydrogen sulfide, iron sulfides are formed which are highly corrosive to carbon steel (AlAbbas et al., 2013). Previous studies have observed higher corrosion rates associated with SRB biofilms in field and laboratory experiments (Beech and Campbell, 2008; Landoulsi et al., 2008; Little et al., 2008; Enning et al., 2012; Enning and Garrelfs, 2014; Vigneron et al., 2016). In addition, SRB and iron-related bacteria (i.e., FeOB, FeRB) have also been shown to be active in MIC of steel shipwrecks in the Gulf of Mexico (Cullimore and Johnston, 2008).
While biofilms on metal accelerate corrosion above that expected from abiotic processes, some biofilms form protective surfaces through deposition of biomass and EPS which may decrease access for abiotic corrosion. Accordingly, biofilms may assist with historic preservation of metal shipwrecks (Beech and Cheung, 1995). However, the local environment dictates the characteristics of marine biofilms (Garrett et al., 2008; Brauer et al., 2015), and thus, perturbations may lead to changes in biofilm composition and function (Salerno et al., 2018).
The in situ effects of oil exposure to marine biofilms following a major spill has not been studied. Crude oil may provide metabolites to biofilm-forming anaerobic bacteria that utilize carbon and sulfur from oil (Aktas et al., 2010). As anaerobic niches in biofilms emerge, oil-degrading bacteria may consume oil and generate metabolites which could potentially accelerate MIC (Little et al., 2008; Pi et al., 2017). Recently, Salerno et al. (2018) assessed the potential impacts of oil on biofilm composition and MIC in laboratory experiments. The study revealed that prolonged oil exposure resulted in a shift in microbial community composition toward oil-degrading taxa. This was accompanied by an increase in metal loss on exposed surfaces, as compared to unexposed surfaces. This suggests that exposure of steel surfaces, including shipwrecks, to oil could negatively impact preservation through enhancement of MIC.
During the Deepwater Horizon spill, the deep-water plume of oil that originated from the Macondo wellhead persisted for nearly 16 weeks as it traveled southwest. The spill interacted with the seafloor through deposition of oil snow from the plume and oil-contaminated surface water, affecting numerous deep-sea communities (Girard et al., 2018). An acute footprint of oil spill-derived contamination was in an area ∼16 km southwest of the Macondo wellhead (Stout et al., 2017). This area contains two steel-hulled historic shipwrecks, the German submarine U-166, and the passenger and freight steamer Robert E. Lee (Damour et al., 2016; Hamdan et al., 2018). Hamdan et al. (2018) provides evidence that U-166 was exposed to the fallout of oil from the spill, and that the microbiomes of sediment surrounding U-166 still presented evidence of contamination 4 years after the spill. The proximity of these shipwrecks to the Macondo wellhead, the observed shift in sediment microbiomes toward oil-degrading bacteria, and location within the spill footprint raises questions about the potential for oil-enhanced MIC and impacts to the preservation of historic shipwrecks.
To date, the Bureau of Ocean Energy Management’s (BOEM) non-public historic shipwreck database lists more than 2,000 vessel losses in the northern Gulf of Mexico between the 16th and 20th centuries. The database compiles information from archival repositories, newspaper accounts, oil and gas-related geophysical surveys since the 1970s, and other sources. Potentially thousands more shipwrecks remain undiscovered, thus, the preservation of other sites may be at risk. Accordingly, the goal of this study was to understand the impacts to deep-sea biofilms caused by in situ exposure to residual spill contaminants through a comparative study involving historic shipwrecks within and external to the acute spill footprint.
Materials and Methods
Field Work and Site Selection
Work was conducted during two expeditions on board R/V Pelican: PE14-15 and PE15-02 in March and July 2014, respectively, as part of the Gulf of Mexico Shipwreck Corrosion, Hydrocarbon Exposure, Microbiology, and Archaeology (GOM-SCHEMA) project. Five historic shipwrecks were selected (Figure 1) (Damour et al., 2016; Hamdan et al., 2018). A sixth site, the Viosca Knoll Wreck (Hamdan et al., 2018) was planned, but weather delays during PE14-15 did not allow for placement of biofilm experiments. Shipwrecks were selected based on location relative to the Macondo wellhead, hull type, age, and if they had a pre-spill archaeological investigation (Hamdan et al., 2018). Consideration was also given to the presence of natural seeps in the study areas. At one site, U-166, the closest known natural seep is 0.55 nm away. At all other sites, the nearest natural seep is at least 1 nm away (information provided by BOEM). Three steel-hulled World War II-era (1942–1944) shipwrecks and two 19th century wooden-hulled and copper-sheathed shipwrecks were investigated. Shipwrecks were in three areas described by proximity to the Macondo wellhead: heavily impacted, defined as within 20 km from the wellhead (U-166 and Mica wreck), moderately impacted, defined as within 75 km (Anona), and reference sites, at 100–150 km (Halo and Ewing Bank wreck) (Figure 1). Stout et al. (2017) described the footprint of spill-derived hydrocarbons on the seafloor across the study area, and an acute fallout plume in the Mississippi Canyon lease area, where U-166 and the Mica wreck are located, shortly after the spill, and in 2014, when the experiments in this study were initiated. The residual impacts to the seabed at sites in this study are described in detail in Hamdan et al. (2018). There is geological and biogeochemical evidence of spill residues at the U-166 site, as revealed by a ∼5 cm layer of oil flocculant on the seafloor that has a radiocarbon signature inconsistent with riverine sediment. Sedimentation rates at that location were also ∼7x greater than expected for the area, after the spill. While the Mica wreck is ∼12 km from the wellhead, our prior work did not reveal biogeochemical or geological evidence of residual impacts at the time of this study. However, the microbiome in surface sediments at Mica and U-166 are highly similar (Hamdan et al., 2018). Hopane and PAH data from Stout et al. (2017) and sedimentation rate data (Hamdan et al., 2018) indicate spill impacts were also evident at Anona at the time of this study.
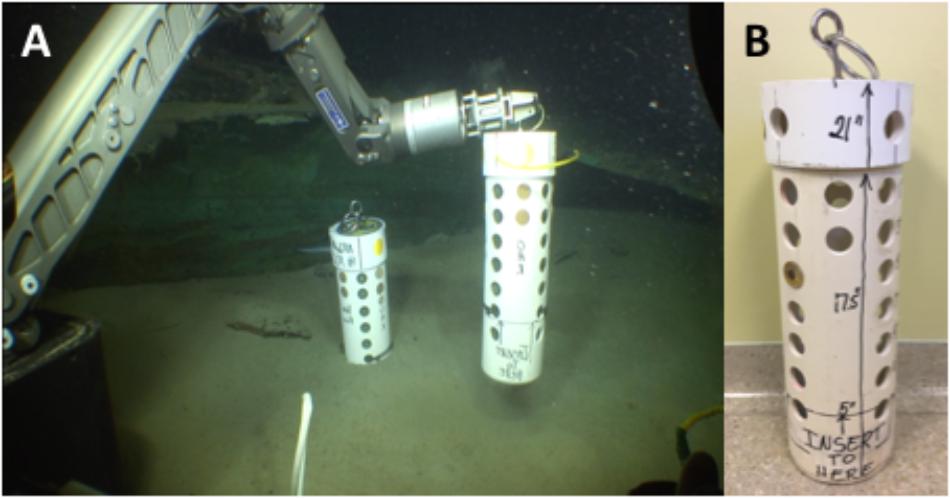
Figure 2. (A) Deployment of BMPs at the bow of Anona using the Orion 7-function manipulator arm of the ROV Global Explorer. (B) Biofilm monitoring platform (BMP) used to hold carbon steel disks (CSDs) deployed at each shipwreck site.
This study focused on built features that arrive at the seafloor by accident; because of this and the constraint of pre-spill investigations, the number of sites that could be used was limited, which created variability in depth across sites. The impacts of variable depth on the interpretation of results is mitigated to some extent by pair-matching wooden-hulled (Ewing Bank and Mica) and steel-hulled (Anona and U-166) shipwrecks that vary in depth by less than 250 m. The exception is Halo, which unlike all other sites is located on the continental shelf.
Sediment sampling and biofilm experiments were facilitated with the remotely operated vehicle (ROV) Global Explorer (Deep Sea Systems International, an Oceaneering Company). Visual surveys of shipwrecks and the seafloor were performed to identify areas for sampling and biofilm monitoring platform (BMP) placement devoid of archaeological materials. To evaluate hull degradation, high resolution still images of specific areas of the shipwrecks were obtained by autonomous underwater vehicle (AUV) surveys. Images were aligned and compared to images from prior AUV surveys in 2003 and 2009 using AutoCAD to measure metal loss.
Sediment was collected with 6.35 cm. inner diameter (ID) push corers and the ROV’s Orion 7-function manipulator arm (Figure 2A). Cores were collected within 2 m of shipwrecks, in the same area BMPs were placed. Water was collected with a CTD-rosette ∼10 m above each shipwreck.
Biofilm monitoring platforms were 53 cm tall and constructed of 15-cm ID PVC pipe with 3-cm. holes on four sides (Figure 2B). The holes were used to hold Unified Numbering System (UNS) C1020 mild carbon steel disks (CSDs) in EpoThinTM 2 epoxy (Buehler) with a 2 cm2 area of exposed steel. Immediately before deployment by ROV, the exposed steel surface and surrounding epoxy of the CSDs were cleaned with molecular grade 100% ethanol to remove grease used to prevent corrosion prior to deployment, and any biofilm that may have developed during storage. The CSDs entered the water column devoid of any biofilm, and thus the source of biofilms was the surrounding marine environment (water or sediment). Two BMPs were then deployed at each site, each containing six replicate CSDs. Disks provided settlement surfaces for biofilms and permitted measurement of metal loss. BMPs were placed vertically on the seafloor by ROV and inserted 5 inches into the sediment. BMPs were deployed in March 2014 and recovered in July 2014, permitting 118 to 131 days for colonization and exposure.
Sample Storage and Handling
Sediment cores were extruded into clean polycarbonate tubes and the upper 4 cm was sampled at 2 cm resolution (Hamdan et al., 2018). New, sterile tongue depressors were used to place ∼10 ml of sediment into sterile, PCR clean conical tubes, which were immediately frozen at -20°C, and stored at -80°C.
Water was transferred from CTD Niskin bottles into clean polycarbonate bottles, and cells in 2.5 L of water were concentrated on Sterivex filters (Millipore) using a peristaltic pump (Hamdan et al., 2013). Filters were stored as described above for sediment. Filters were cracked, the filter aseptically removed, and extracted as described below for genetic analyses.
Carbon steels disks were immediately removed from BMPs upon recovery of the ROV. The CSDs were placed upright in sterile plastic containers. Samples for genetic analysis were stored as described for sediment. Samples for metal loss were air dried for 2 days under a sterile glass beaker at room temperature.
DNA Extraction and Quantitation
DNA was extracted from all samples using the FastDNATM protocol, modified as described elsewhere for sediment (Hamdan et al., 2018), water (Hamdan et al., 2013), and biofilms (Salerno et al., 2018). Purified template was quantitated on a Qubit 2.0 Fluorometric Quantitation system following the manufacturer’s protocol (Invitrogen). A NanoDrop spectrophotometer was used to assess the quantity and purity of extracted DNA (ThermoFisher).
16S rRNA Gene Amplification and Sequencing
Extracted genomic DNA was submitted to the Integrated Microbiome Resource (IMR) facility at Dalhousie University for 16S rRNA gene amplicon sequencing on an Illumina MiSeq according to Comeau et al. (2011) and Salerno et al. (2018). The V6-V8 variable regions of the 16S rRNA gene were selectively targeted for amplification using primer sets B969F/BA1406 and A956F/A1401R for bacteria and archaea, respectively.
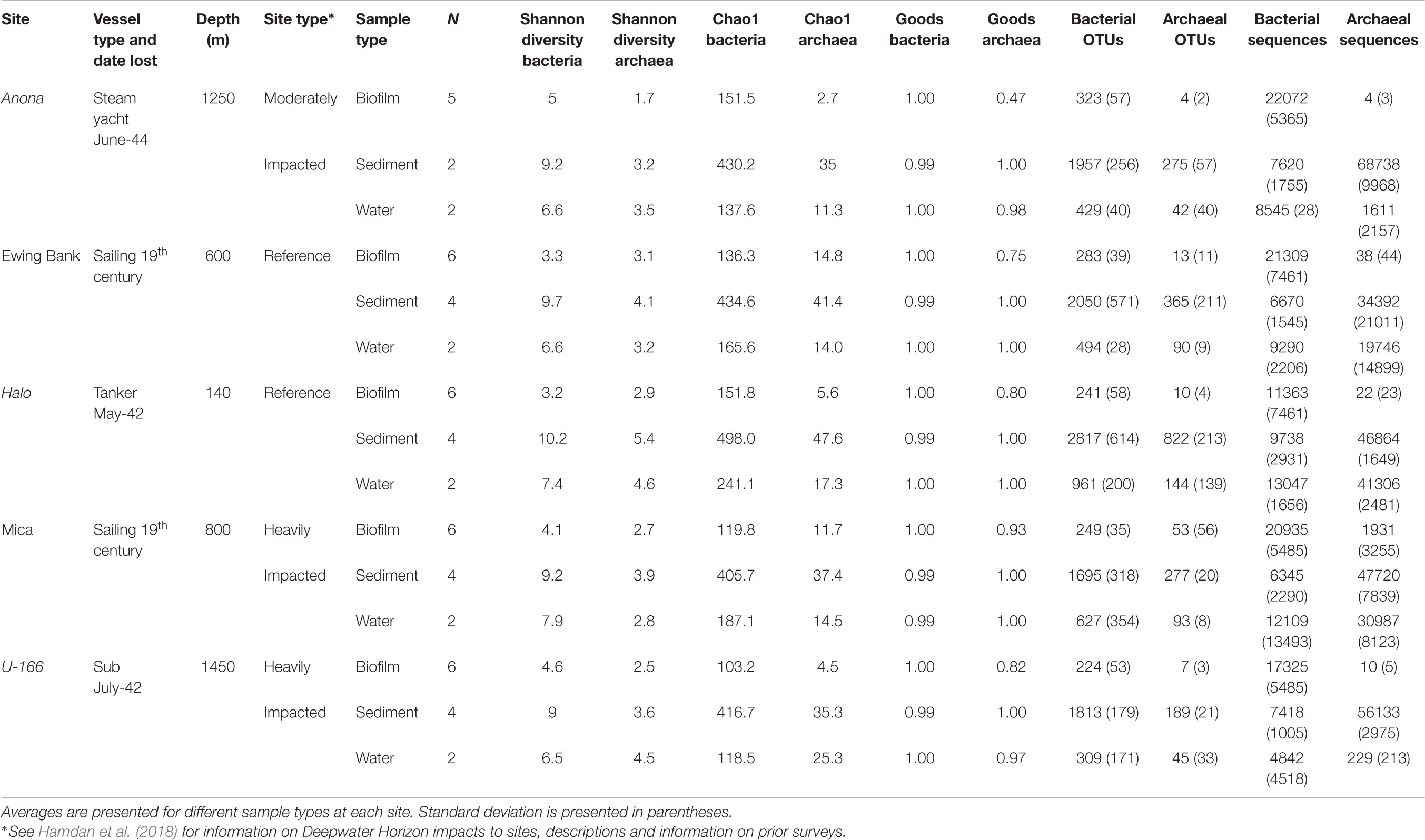
Table 1. Descriptive statistics for sediment, water, and biofilms from BMPs collected proximate to shipwrecks.
The pipeline generated with UPARSE (Edgar, 2013) and Quantitative Insights into Microbial Ecology (QIIME) (Caporaso et al., 2010) described in Salerno et al. (2018) was used to analyze bacterial and archaeal amplicon sequences. Paired-end sequences were merged, quality filtered, dereplicated and unique sequences served as operational taxonomic units (OTUs). Sequences were assigned to OTUs at ≥97% similarity, and chimeras were removed. An OTU abundance table was constructed, and UCLUST and the GreenGenes reference database (v 13.8) (DeSantis et al., 2006) were used to assign taxonomy. The Chao1 and Good’s coverage indices were calculated at the species level in QIIME (Caporaso et al., 2010). A core microbiome in ≥95% of biofilm samples was identified with the compute core microbiome script in QIIME. Archaeal sequence count in biofilm samples from 4 of 5 sites were very low (<22 total; Table 1) and thus, excluded from further analysis. The same was observed for archaea in biofilms on CSDs in Salerno et al. (2018).
SourceTracker (v 0.9.5) (Knights et al., 2011) was used to model the source (surface sediments at 0–4 cm vs. overlying water) of OTUs to biofilms on CSDs using a Bayesian inference. The microbiome on CSDs was treated as a ‘sink’ and modeled as a mixture of OTUs originating from water, sediment and unknown sources. SourceTracker estimated the proportion of the CSD microbiome originating from those sources. Because the sediment at impacted sites, specifically Anona and U-166 was physically modified by spill contaminants, showing anomalously high surface porosities for sites exceeding 1000 m deep (Hamdan et al., 2018), we hypothesized that this could result in greater physical interaction between CSDs and sediment, and thus greater import of OTUs to CSDs from a sediment source. This hypothesis was informed by prior studies which demonstrate that physical constraints, i.e., porosity and density, dictate microbiome dispersal and composition (Hamdan et al., 2013).
PRIMER (v.6.13) was used to calculate Bray–Curtis dissimilarities from the OTU table after fourth root transformation. Non-metric multidimensional scaling (NMDS) plots were constructed to visualize community structure. Hierarchical clustering (CLUSTER) based on the group average linkage was used to illustrate similarity. A permutational analysis of variance (PERMANOVA) identified differences in sample groups (e.g., impact and hull type), using depth as a covariate. PERMANOVA was run using Type I (sequential) sum of squares, fixed effects sum to zero, permutation of residuals under a reduced model, and 9999 permutations.
Metagenome Amplification Sequencing and Bioinformatics
Prior to metagenome analysis, biofilm samples from each site were pooled if replicates fell within 2 standard deviations for diversity statistics (Shannon, Chao1, total sequences). Pooling protocols are described in Comeau et al. (2011). At IMR, DNA libraries were prepared with the Nextera XT Library Preparation Kit (Illumina) modified to use the Just-a-PlateTM 96 PCR Purification and Normalization Kit (Charm Biotech). Sequencing was performed on an Illumina NextSeq 550, generating 150 bp, paired-end sequences.
Analysis of metagenomes followed the Microbiome Helper (Comeau et al., 2017) procedure. Raw sequences were quality filtered (Andrews, 2010), assembled into paired-end reads (Zhang et al., 2013), trimmed (Bolger et al., 2014), and screened for contaminants (Langmead and Salzberg, 2012). MetaPhlAn2 was used to assign taxonomy (Truong et al., 2015). Phylogenetic trees were created to the genus level in GraPhlAn to visualize and compare communities (Asnicar et al., 2015). HUMAnN2 was used to profile genes and gene families using the UniRef90 database, and associated pathways and abundance using the MetaCyc Metabolic Pathway database (Abubucker et al., 2012). Prior to analysis in MEGAN, reads were aligned to a DIAMOND database created from the UniRef90 protein blast database (Buchfink et al., 2014). Metagenome aligned reads were imported to MEGAN v 6.11.1 as normalized counts and interactively compared using the NCBI database for taxonomy and the SEED database for gene counts, pathways, and abundances of gene families in samples (Huson et al., 2016).
The normalization approach used the relative log expression (RLE) and the trimmed mean of M-values (TMMs), implemented in DESeq2 and EdgeR, respectively (Table 2). This approach was selected because of its high performance in a review of methods for gene abundance (Pereira et al., 2018). Both methods calculate a dataset-specific scaling factor versus randomly removing gene fragments. DESeq2 and EdgeR assume that no genes will be differentially abundant. Prior to statistical analysis, the biological coefficient of variation (BCV) was estimated in EdgeR to accurately fit a negative binomial model to the dataset (BCV = 0.6). Differentially abundant genes (DAGs) were screened using the TMMs method in the EdgeR package in R (Robinson and Oshlack, 2010) and the RLE method in the DESeq2 package in R (Love et al., 2014). Both methods used absolute gene counts imported from MEGAN to normalize data. The DESeq2 workflow was executed twice and yielded the same results. To test for DAGs in DESeq2 and EdgeR, the analysis must be set by group, treatment, or condition, and include replicates (Chen et al., 2014). As replicates were pooled for metagenome sequencing, the differential abundance test in DESeq2 was performed by impact (reference, moderately impacted, heavily impacted), and by reference against impacted sites in EdgeR. DESeq2 calculated DAGs based on log fold change values and statistical significance (P < 0.05) between reference sites and the moderately impacted site, and between reference sites and heavily impacted sites. EdgeR served as a check on DESeq2. A table of the top 20 DAGs across all sites was generated based on conversion of copies per million (CPM) to log counts to correct for library size differences. EdgeR returned a table of top 10 DAGs between reference and impacted sites using a negative binomial model based on common and tagwise dispersion estimates. All sequences (16S rRNA and metagenomes) were submitted to the NCBI SRA under BioProject PRJNA481022.
Metal Loss
Prior to mounting in epoxy, CSDs were weighed to calculate weight loss. Following field experiments and drying, disks were removed from epoxy mounts, and acid-cleaned to remove corrosion products according to ASTM Standard G1-03 (2003). Four acid-cleaned disks from each site were weighed and compared to pre-exposure weights to estimate weight loss.
Results
Microbiome Composition in Sediment and Water
A total of 875,835 sequences (average length 437 bp) in sediment and water were retained after quality filtering. Archaeal sequences in sediment were more abundant than bacteria; however, bacterial diversity and OTU richness exceeded archaeal metrics at all sites. In general, sediment diversity was higher at reference sites relative to impacted sites, and Shannon diversity in sediment samples exceeded that in biofilm and water samples (Table 1). The Good’s coverage index averaged 0.99 for bacteria and archaea in sediment and water samples indicating excellent coverage of the community, including singletons.
The Proteobacteria were highly abundant in surface sediments at all sites, and Delta-, Gamma-, and Alpha-proteobacteria accounted for over 50% of sequences (Supplementary Figure S1). The class Deltaproteobacteria was most abundant at reference sites (18–22%), and the Alphaproteobacteria averaged 11% (7–14%) of sequences at moderately and heavily impacted sites. Gammaproteobacteria dominated moderately and heavily impacted sites (23–29%) and were most abundant in surface sediments from U-166.
One Gammaproteobacteria OTU, related to the Piscirickettsiaceae, accounted for an average 11% of sediment sequences. The second and third most abundant OTUs were Flammeovirgaceae-related sequences, and a Deltaproteobacteria affiliated with the NB1-I family.
Water samples at Halo had the highest average bacterial diversity, with an average of 961 bacterial OTUs, possibly attributed to the shallow depth at this site (Table 1). Archaeal sequence abundance in water samples generally exceeded bacterial sequence abundance with the exception of Anona and U-166.
The Proteobacteria accounted for the majority of bacteria in water samples at all sites (Supplementary Figure S2). Alpha- and Gamma-proteobacteria were most abundant, followed by Deltaproteobacteria. The SAR 406 cluster accounted for over 7% of sequences at all sites. At all locations, the most abundant OTU was affiliated with the Pelagibacteraceae family (21–41%). Gammaproteobacterial OTUs related to the Alteromonas genus and the Oceanospirillales order accounted for an average of 6% of sequences at all sites.
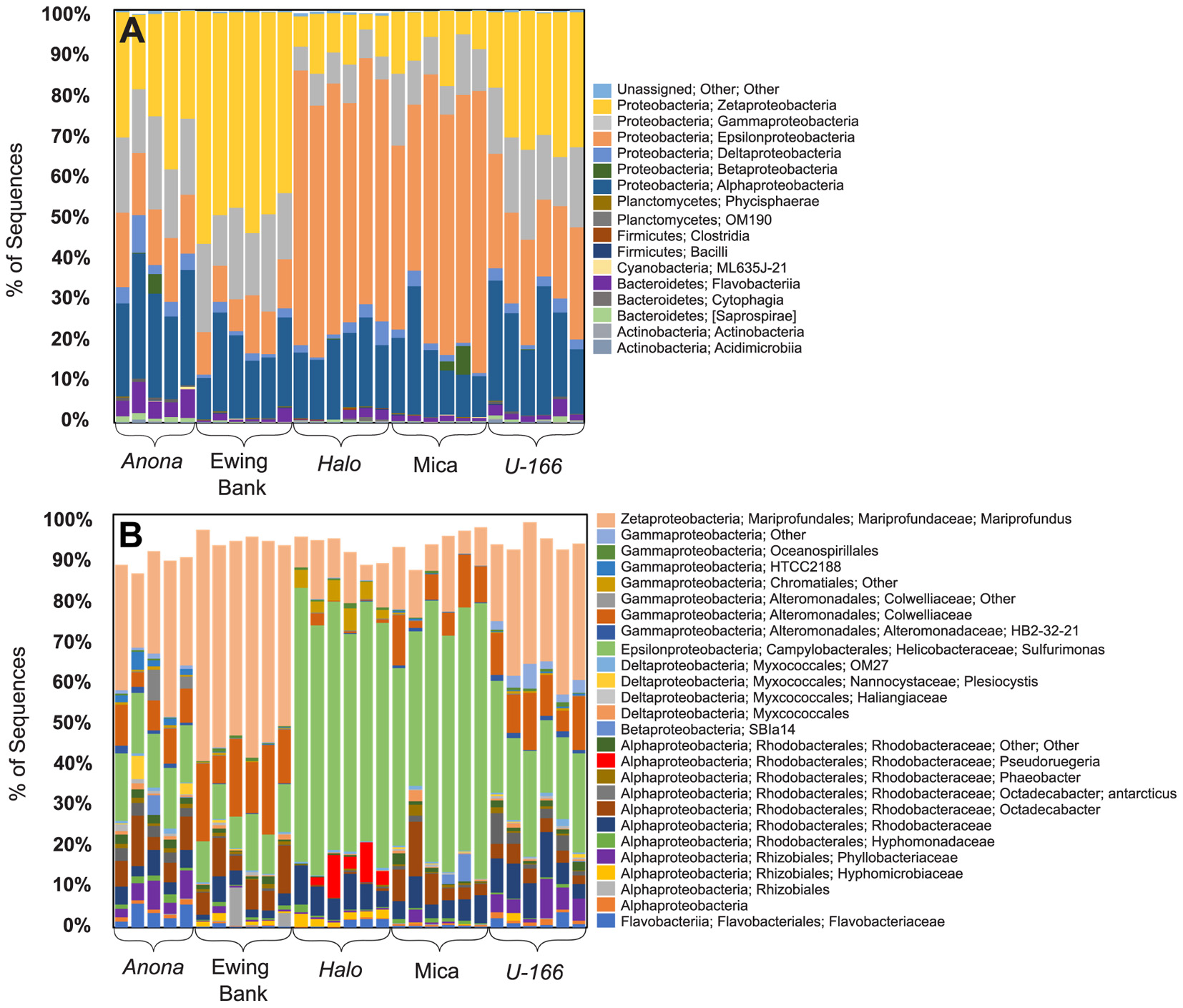
Figure 3. Class level (A) and OTU level (B) bacterial community composition (16S rRNA) in replicate biofilm samples.
Microbiome Composition in Biofilms
Bacterial species richness and Shannon diversity in biofilms were highest at Anona and lowest at Halo (Tables 1, 2). In contrast to results for sediment and water samples, diversity in biofilms on CSDs was generally higher at impacted sites compared to reference sites. All biofilm communities were dominated by Proteobacteria (85–97%) (Figure 3A). The Epsilon- and Zeta-proteobacteria were most abundant among the Proteobacteria, although Gamma- and Alpha-proteobacteria were also abundant. Generally, two bacterial OTUs from the Sulfurimonas genus (Gammaproteobacteria) and Mariprofundus genus (Zetaproteobacteria) accounted for the majority of sequences in the total biofilm dataset (Figure 2B). The Sulfurimonas OTU was highly abundant at Halo and the Mica wreck. Cultured members of this group grow chemolithoautotrophically using zero valent sulfur, molecular hydrogen, or reduced sulfur compounds as electron donors and nitrate, nitrite, and oxygen as electron acceptors (Inagaki et al., 2004). The Mariprofundus OTU was highly abundant at Anona, Ewing Bank, and U-166. Mariprofundus is the only genus in the class Zetaproteobacteria, and cultured isolates can grow by oxidizing ferrous to ferric iron.
Similar to the 16S rRNA-based sequence data, metagenome analysis revealed that biofilms were dominated by Proteobacteria. Gamma-, Epsilon-, and Alpha-proteobacteria were present at all sites. As described for the 16S rRNA results, Zetaproteobacteria were highly abundant at Anona, Ewing Bank, and U-166 (Figure 4). Taxonomic diversity trees corroborate Anona as being the most diverse site and Halo as the least diverse. Unlike other locations, Betaproteobacteria were present in Mica samples. Sulfurimonas dominated metagenomes at all sites (Figure 3). The order Rhodobacterales, which includes the Roseobacter, a microorganism known for biofilm formation and potential oil degradation, was abundant at Anona, Ewing Bank, Halo, and U-166. The Alteromonadales, including the family Pseudoalteromonadaceae, which is associated with oil degradation, accounted for 3–10% of metagenomes at all sites except Halo. The 16S rRNA results (Figure 3) also indicated an elevated abundance of Altermonadales at all sites except Halo; however, the two most abundant OTUs were affiliated with the Colwelliaceae.
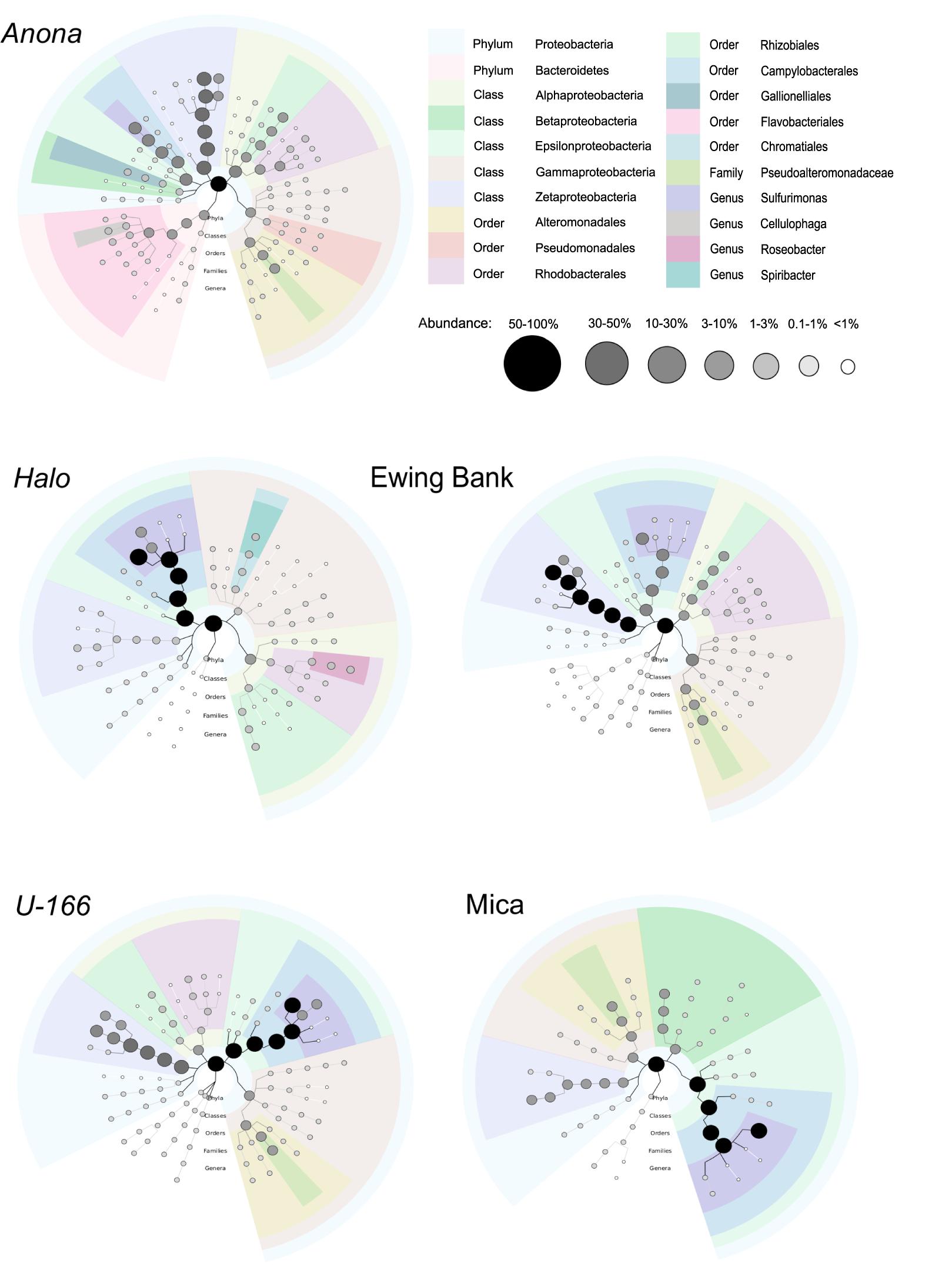
Figure 4. Biofilm taxonomy from metagenome sequences of pooled replicate samples. Shaded bubbles represent percentage of abundance and colors represent taxonomic groups. Plots generated in GraPhlAn.
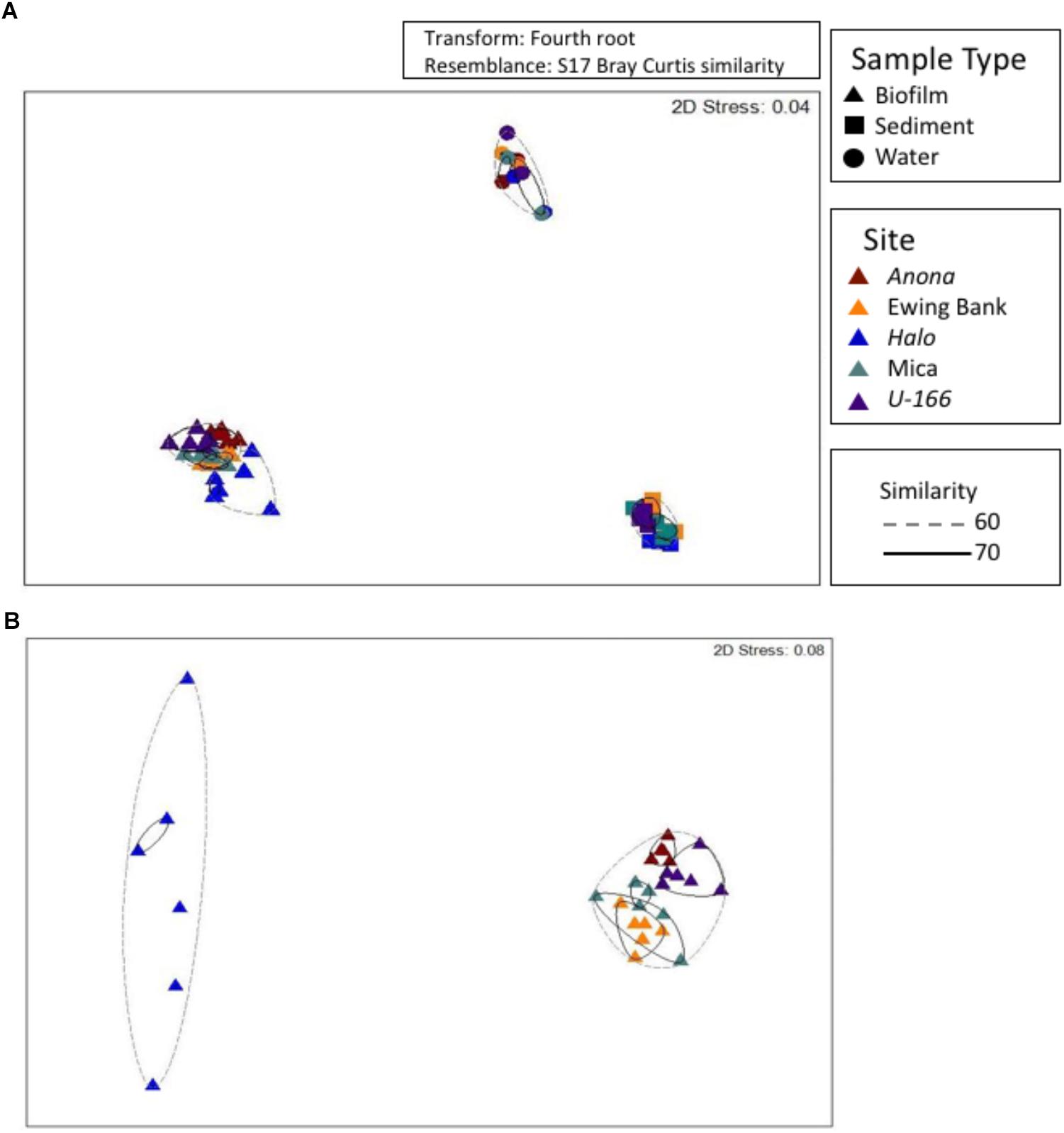
Figure 5. Non-metric multidimensional scaling analysis of bacterial communities at all sites and in all sample types (A). A subset of biofilm samples from BMPs (B). Hierarchical clustering used to obtain similarity dendrograms, based on group average linkage are projected on the plot as contours.
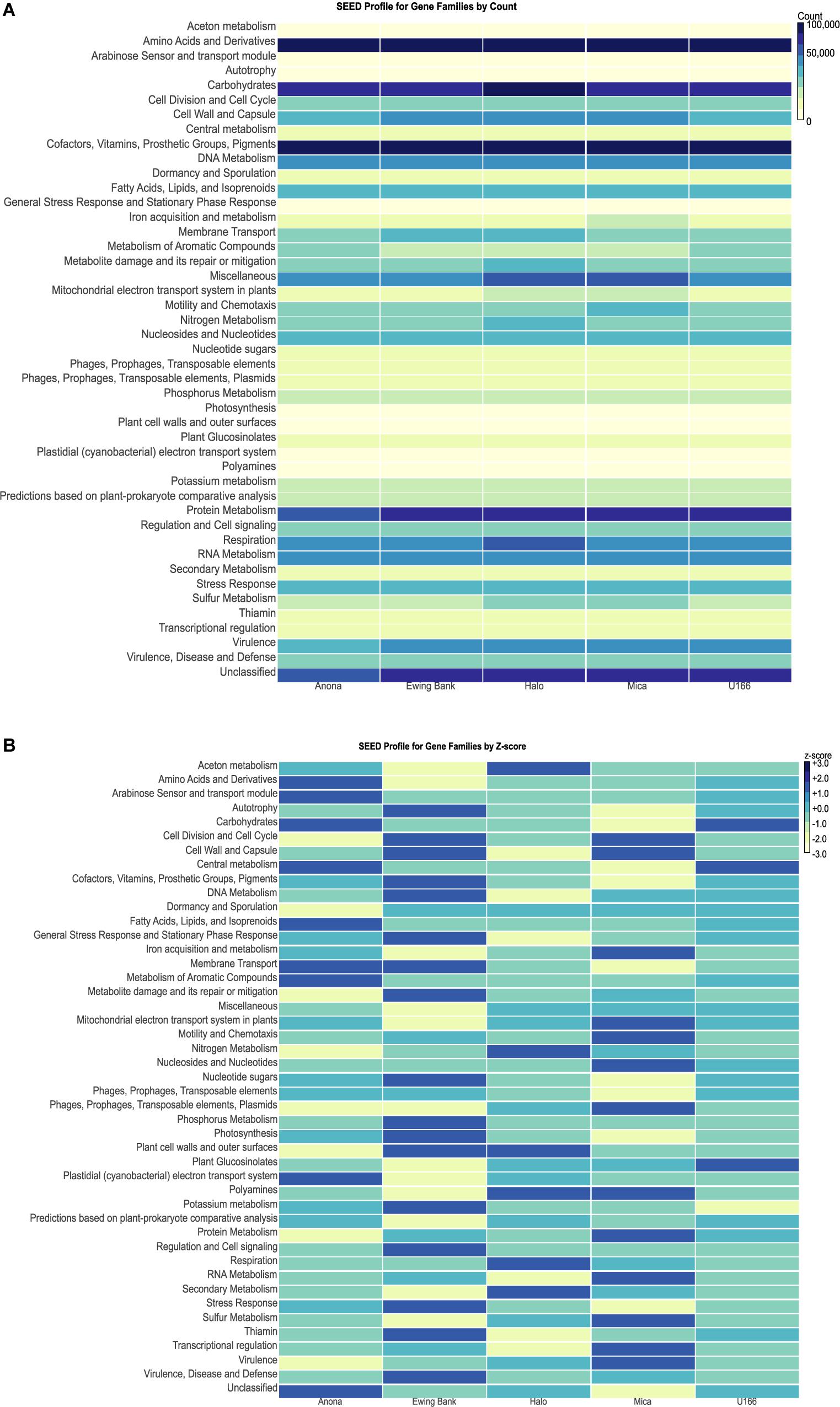
Figure 6. MEGAN heatmaps of functional gene families classified using the SEED database. (A) Heatmap by count of gene reads in each family. Light colors represent smaller counts while dark colors represent larger counts. (B) SEED profile of gene families normalized by Z-score.
Features Driving Biofilm Composition
Bacterial communities in biofilm, sediment, and water formed distinct clusters that were significantly different based on sample type (Figure 5A and Supplementary Table S1). At 60% similarity, biofilm samples formed two clusters (Figure 5B) with Halo distinct from other sites. PERMANOVA revealed that sites contain distinct communities based on depth as a covariate, and impact and hull type as factors (Supplementary Table S1). Communities at heavily impacted sites differed significantly from reference sites (P= 0.0001, Supplementary Table S1). The shipwreck’s hull material (wood or steel) proximate to BMPs also drove dissimilarity among sites (P= 0.0001, Supplementary Table S1).
SourceTracker revealed that surface sediment was the primary known source of OTUs to biofilms (Supplementary Figure S3). The highest predicted contribution of sediment OTUs to biofilms was at Anona (average 22.3%) and U-166 (average 18.2%), while the lowest predicted contributions were at Halo (average 1.6%). The majority of biofilm OTUs sourced from sediment were affiliated with the oil-degrading Colwelliaceae family, followed by the Rhodobacteraceae family, which includes biofilm formers, and the iron-oxidizing genus Mariprofundus (Supplementary Table S2).
The biofilm core microbiome contained 11 OTUs (Supplementary Table S3), seven of which were likely sourced from sediment. Most core microbiome OTUs were related to the Rhodobacteraceae and Mariprofundaceae families. The functional roles of cultured representatives of core microbiome OTUs include biofilm formation, iron oxidation, and sulfate reduction.
Gene Families in Biofilm Samples
The highest gene counts, as revealed by MEGAN, were classified to housekeeping families including amino acids and derivatives, carbohydrates, cofactors, vitamins, prosthetic groups, pigments, and protein metabolism (Supplementary Figure S4). Heatmaps were used to compare gene family abundance between sites. Mica had higher counts of genes clustering to iron acquisition and metabolism and motility and chemotaxis relative to other sites (Figure 6A). Sulfur metabolism counts were elevated at Halo and Mica, and nitrogen metabolism and respiration were highest at Halo. Ewing Bank and Halo showed the highest count of membrane transport genes. Anona and U-166 had the highest count of genes involved in metabolism of aromatic compounds. The heatmap, when scaled by Z-score (number of standard deviations from mean) for each gene family revealed U-166 to have the most Z-scores closest to zero (Figure 6B).
Differentially Abundant Genes Across Sites
The differential abundance analysis of reference sites vs. a moderately impacted site revealed significant differences in the abundance of protein hydE, quinone-reactive Ni/Fe-hydrogenase small chain precursor, periplasmic nitrate reductase component NapL, two-component response regulator CreC, and quinone-reactive Ni/Fe-hydrogenase large chain genes (Table 3). Only one gene, the two-component response regulator CreC, was significantly different in the comparison of reference sites to heavily impacted sites.
Metagenome absolute sequence counts were analyzed in EdgeR to test the robustness of differential abundance results from DESeq2 in site to site comparisons. Prior to normalizing for total library size, absolute counts were converted to counts per million (CPM) to normalize for sequencing depth across sites. A table of the top 20 most variable genes across samples was generated by calculating the variance for each gene in the dataset, and then creating a new matrix to display the 20 most variable genes in all samples (Table 4). Variable genes included metal-dependent hydrolase involved in phosphonate metabolism, motility accessory factor, and flagellar transcriptional activator FlhC. Following normalization by the TMMs method, differential abundance analysis was performed in EdgeR by groups of sites (reference versus impacted) to discover DAGs, reported in terms of log fold change (log FC), CPM, P-value, and false discovery rate (FDR) (Supplementary Table S4). A negative log fold change value indicates that the gene had higher counts at the reference sites, while a positive value reflects a higher gene count at impacted sites. The only statistically significant (P< 0.05) DAG with a low FDR value (FDR < 0.05) was the two-component response regulator CreC.
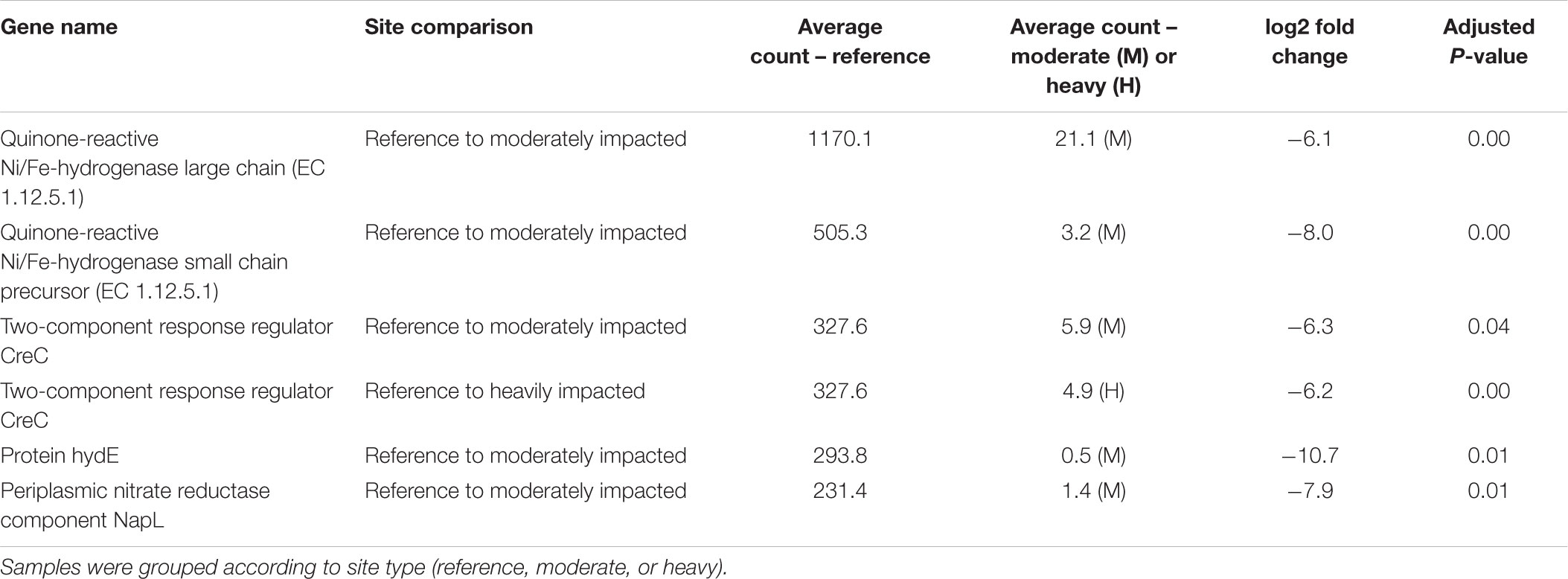
Table 3. Statistically significant (P< 0.05) differentially abundant genes (DAGs) analyzed in DESeq2.
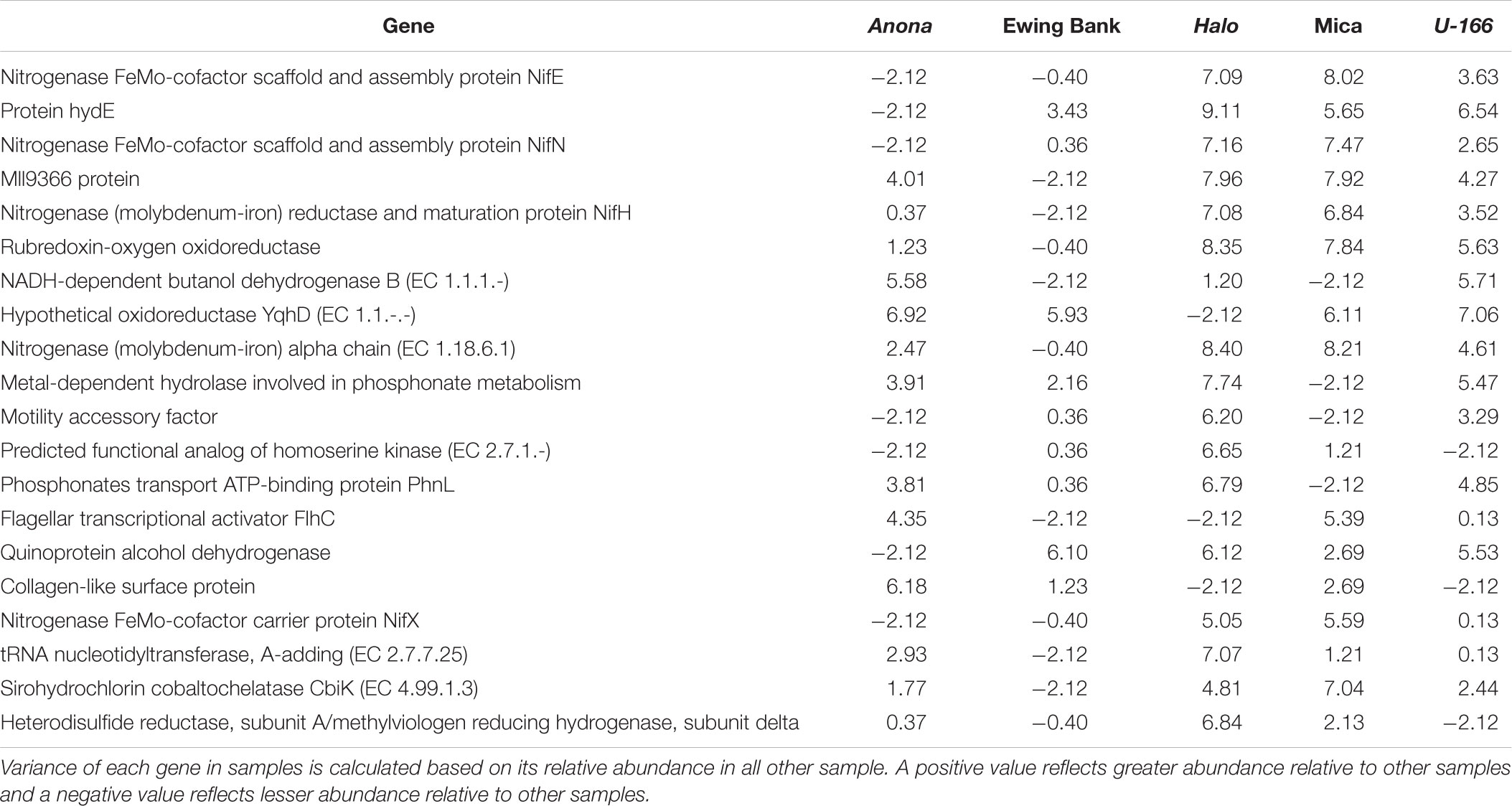
Table 4. Top 20 variable genes between metagenome samples after differential abundance analysis in EdgeR.
Metal Loss
Metal loss was estimated by weight difference at all sites (Supplementary Figure S5). Average metal loss was lowest at Halo (0.04 ± 0.01 g), and highest at U-166 (0.12 ± 0.01 g), and Mica (0.10 ± 0.01 g).
During a study initiated prior to the Deepwater Horizon spill, images of the deck of U-166 were collected for archaeological studies. During the SCHEMA study, additional images were obtained to document impacts from the spill. These data were used to calculate metal loss. Data are not available for other sites in this study because this analysis required images from at least two observation events prior to the spill, thus these results focus only on U-166. Areas of metal loss on the deck of the U-166 were quantified by comparing images from 2003, 2009, and 2013. An area of metal loss that created a breach in the port side hull of the U-166 stern section (Figure 7A, area 1) measured 57.9 cm × 25.7 cm in 2003. By 2009 the area had widened along the port side gap by 4.5 cm. Between 2009 and 2013 the gap widened an additional 9.8 cm, twice the amount previously observed, in only 4 years. Another breach in the deck (Figure 7A, area 2) on the starboard side measured 47.3 cm at its widest in 2003. In 2009, in the same breach, a 5.2 cm wide portion of the starboard edge had deteriorated away; in 2013 a 24.5 cm × 5.5 cm section along the edge completely disappeared. The metal loss in the four-year span encompassing the post spill period is five times that observed in the six years prior to the spill. The full extent of deterioration is unknown, as the section was covered in a layer of sediment not observed prior to 2013. Aft of area 2, an additional previously undetected area of deterioration (area 4) was found in 2013. Areas of additional deck deterioration were also imaged aft of the 37-mm gun (Figure 7B) that was first discovered and documented in 2003. The deterioration had expanded by 2009 and new holes were observed that were not present in 2003. In 2013, these areas had continued to widen, and additional holes not present in 2009 were found. A 5 cm hole first observed in 2003 had no discernible change in size in 2009. By 2013, metal deterioration had expanded the hole to more than twice its size as documented in 2003 and 2009.
Discussion
Spill Impacts on Biofilm Taxonomy
Formation of biofilms in the marine environment assist in the survival, adaptation, and propagation of bacteria (Awan et al., 2018). In some cases, formation is enhanced after introduction of sub-lethal stress or nutritional-stress factors in the environment. Oil spills alter microbial communities in the marine environment (Joye et al., 2016; Hamdan et al., 2018). Hamdan et al. (2018) demonstrated that residual spill contaminants remained at U-166 at the time BMPs were deployed and recovered, and that impacts to sediment microbiomes were evident at moderately and heavily impacted sites. The presence of residual oil may promote bacterial growth and enhance biofilm formation, indicating a community response under adverse environmental conditions (Dombrowski et al., 2016; Awan et al., 2018).
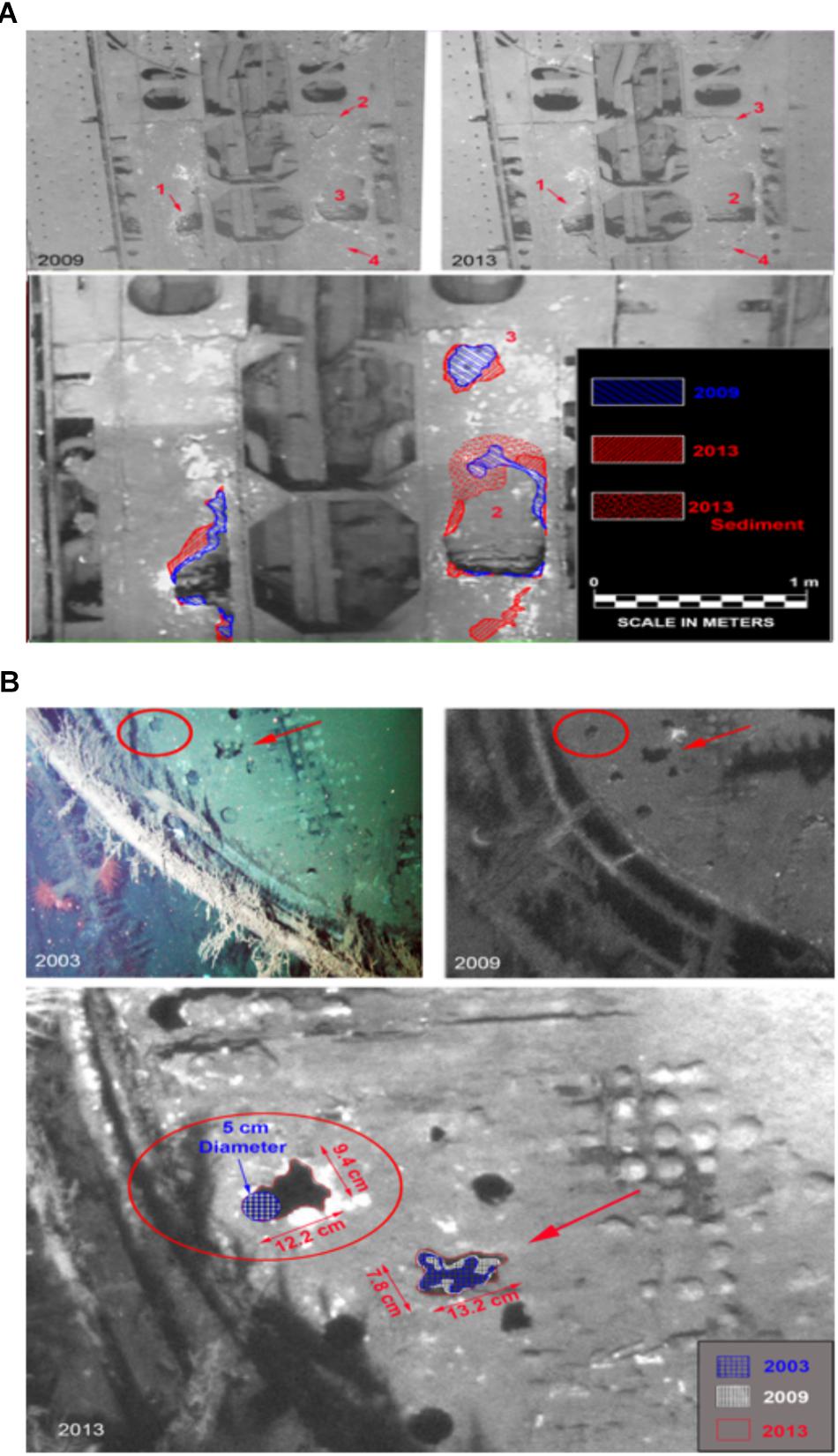
Figure 7. Metal deterioration on the deck of the U-166. (A) Deterioration in U-166’s aft deck from 2003, 2009, and 2013. (Upper) 2009 and 2013 image showing section of the deck, and (Lower) 2003 photo with 2009 (Blue) and 2013 (Red) shown as hatched overlay. (B) Deterioration on the deck of U-166’s conning tower. (Upper) 2003 and 2009 images, and (Lower) prior images shown as hatched overlay of 2013 image.
Previous studies have shown Alteromonadales to be early and dominant colonizers of marine biofilms (Arora and Boon, 2012; Dombrowski et al., 2016; Rampadarath et al., 2017), with some Alteromonas spp. protecting biofilms against contaminants. Metagenome taxonomy data showed that Alteromonadales accounted for 3–10% of the bacterial community at all sites except Halo. While the relative abundance of Alteromonadales OTUs was elevated at the Ewing Bank wreck, a reference site, the diversity of this order was reduced at both reference sites relative to impacted sites, suggesting that spill residues may have impacted the diversity of this group. The 16S rRNA data identified similar trends for Alteromonadales with minimal sequence abundance at Halo. The most abundant Alteromonadales OTU in this study was affiliated with the Colwelliaceae family, which dominated the water column plume during the Deepwater Horizon spill (Valentine et al., 2014). Colwellia has been shown to respond to the presence of oil in field and lab experiments, and may metabolize a variety of hydrocarbon compounds (Mason et al., 2014; Brakstad et al., 2015a; Hu et al., 2017). Unlike other locations, two Colwelliaceae OTUs were abundant at Anona and U-166 (Figure 2). The presence of Colwellia in biofilms may imply that biodegradation of recalcitrant oil compounds was occurring at these impacted sites. PERMANOVA analysis revealed that 16S rRNA-based bacterial community composition in reference vs. heavily impacted sites differed significantly (P= 0.0001). The Phyllobacteriaceae family, which were enriched at Anona and U-166 (Figure 3), two of the deepest sites, contributed to the observed differences. An OTU affiliated with the Phyllobacteriaceae accounted for 1–10% of 16S rRNA sequences in biofilms at these locations. The novel Phyllobacteriaceae family, of the Rhizobiales, has been associated with hydrocarbon degradation in the deep sea and in oil-contaminated backwaters (Vila et al., 2010; Lai et al., 2011; Rahul et al., 2015). Its elevated presence at locations with confirmed spill impacts (Hamdan et al., 2018) supports a hypothesis of oil metabolism in biofilms at U-166 and Anona. We note that natural seepage of oil is common in the Gulf of Mexico, and that there are numerous natural oil and gas seeps in the Mississippi Canyon lease area (Orcutt et al., 2010). The presence of natural oil is likely to increase the abundance of oil-degrading bacteria in environmental samples. However, there are no natural seeps in direct contact with sites in this study.
In studies of microbial community succession during oil biodegradation, Gamma- and Alpha-proteobacteria are often dominant (Jiménez et al., 2011; Brakstad et al., 2015b; Rampadarath et al., 2017). Gammaproteobacteria dominate during initial exposure to hydrocarbons, while Alphaproteobacteria become more prevalent as hydrocarbons biodegrade (Marietou et al., 2018). Co-dominance of both Gamma- and Alphaproteobacteria at Anona and U-166 suggests that the communities at these sites may have colonized biofilms to utilize residual oil from the spill or are readily sourced from the local environment.
The Zetaproteobacteria class of iron-oxidizing marine bacteria are widely distributed in the deep ocean (McBeth et al., 2011; Singer et al., 2011; McBeth and Emerson, 2016). The first genome of a Zetaproteobacterium, Mariprofundus ferrooxydans PV-1, sequenced in 2011, revealed this microorganism’s ability to rapidly detect and respond to environmental stimuli (Singer et al., 2011). Comparative metagenomics with other marine iron-oxidizing bacteria indicate that Zetaproteobacteria harbor genes involved in signal transduction, response regulation, and heavy metal resistance. In the current study, Zetaproteobacteria were abundant at all sites, including U-166, suggesting their tolerance to oil residues.
SourceTracker analysis has been used in environmental studies to evaluate possible sources of dispersal for local communities (Hamdan et al., 2013; Storesund et al., 2017; Comte et al., 2018). This tool was applied to evaluate if physical modification of sediment at spill impacted sites resulted in greater dispersal of sediment OTUs to CSDs, impacting colonization of CSDs and microbiome composition of biofilms. At all locations, the predominant known source of OTUs was sediment, indicating that ordinarily, sediment biodiversity will dictate the microbiome forming on structures resting on the seafloor. This study revealed that the two sites with the greatest source contribution from sediment were Anona and U-166. In a previous study (Hamdan et al., 2018), Pb-210 analysis was used to predict the rate of sedimentation, and to inform if sites in this study experienced fallout from the Deepwater Horizon spill. The rate of sedimentation at Anona and U-166 was 3 to 7 times higher than expected for the northern Gulf of Mexico (Yeager et al., 2004) and deviated from other locations. The sedimentation rate observations for Anona and U-166 were consistent with the findings of Brooks et al. (2015) concerning a sedimentation pulse following the Deepwater Horizon spill. Surface porosity at Anona and U-166 was higher than other sites and attributed to fallout of highly porous oil snow, likely from the spill. The presence of fresh, non-compacted material likely increased the potential of sediment-associated OTUs to encounter CSDs and may explain the greater source contribution of OTUs from sediment at impacted sites. These results indicate that the effects of an oil spill on metal structures resting on the seafloor may persist even after labile hydrocarbons are below detection limits. Greater dispersal of sediment OTUs to metal surfaces, including CSDs, shipwrecks, and other marine infrastructure may change the structure of biofilm communities, their potential function, and the physical interaction between community and surface, all of which could impact preservation.
Spill Impacts on Functional Potential
Many bacteria use the widespread two-component system (TCS) to detect and respond to changes in the environment (Arora and Boon, 2012; Awan et al., 2018). The TCS is deployed as a defense and stress response mechanism, where the cell detects stimuli and responds through selected processes (e.g., quorum sensing, light detection, and chemotaxis). Some environmental changes, including oil spills, may result in a temporary or permanent change in local conditions, triggering bacterial stress responses (Awan et al., 2018). The two-component response regulator CreC is part of the TCS. Differential abundance analysis of gene counts in both DESeq2 and EdgeR returned CreC as significantly elevated at reference sites as compared to the moderately impacted site (P= 0.043) and heavily impacted sites (P= 0.003), possibly due to the absence of oil (stressor) at the reference sites.
Bacterial chemotaxis and motility genes play significant roles in biofilm formation, maturation, and dispersal (Awan et al., 2018). Flagellar formation and regulation genes help bacteria swim toward nutrient sources and detect and adhere to surfaces (Li and Wang, 2011). They may also assist in avoiding stressful conditions, through detachment and relocation to a more favorable environment (Singer et al., 2011). The table of the top 20 variable genes (Table 4) between all sites identified the flagellar transcriptional activator FlhC gene as more variable and generally more prevalent at impacted sites compared to reference sites. These differences in variation and prevalence of the FlhC gene may be indicative of a response by biofilm community members to escape unfavorable environmental conditions.
Since the 2010 Deepwater Horizon oil spill, several studies have documented the ability of specific groups of bacteria to thrive in oil-contaminated environments by using oil compounds as a carbon and energy source (Bælum et al., 2012; Gao et al., 2015; Dombrowski et al., 2016). In this study, MEGAN analysis of functional gene families revealed higher counts of aromatic degradation genes at Anona and U-166 compared to the reference sites (Supplementary Figure S4). The differential abundance analysis also revealed that NADH-dependent butanol dehydrogenase, an alcohol dehydrogenase, had greater abundance at Anona and U-166 than other sites. Mason et al. (2014) demonstrated that alcohol dehydrogenase, including butanol dehydrogenase genes, were frequently observed in a Colwellia phylotype enriched during the spill. Alcohol dehydrogenases are enzymes that are crucial for the degradation of hydrocarbon compounds, and their elevation at Anona and U-166 may imply that biodegradation of residual oil compounds was still occurring at these contaminated sites (Sierra-García et al., 2014).
Spill Impacts on Shipwreck Preservation
One goal of this study was to understand how exposure to oil on the seafloor impacts the preservation of historic shipwrecks, and potentially enhances MIC. While Zetaproteobacteria abundance was elevated in biofilms relative to sediment and water, no functional iron-bacteria interactions were observed. In a previous work using CSDs in a microcosm study, Salerno et al. (2018) observed greater metal loss after exposure to oil, likely attributed to MIC resulting from sulfur metabolism and the production of corrosive metabolites. While this study does not provide evidence of enhanced sulfur metabolism at impacted sites, greater metal loss on CSDs at U-166 was observed relative to other locations.
Hamdan et al. (2018) provides definitive evidence of oil residues from the spill in sediment collected around U-166. Even though other environmental factors, such as depth, temperature, and nutrient availability may be involved in determining total metal loss, the presence of oil flocculant on the seafloor, and the increased physical interaction between seafloor and surface as revealed by SourceTracker, should be considered. At the time of BMP collection at U-166, high-resolution images of the shipwreck were obtained to compare with images collected in 2003 and 2009, prior to the spill. These images are relevant to the current study which documents impacts to biofilm composition, colonization and metal loss at U-166. Over a decade of progressive deterioration has been observed on the aft deck and conning tower of U-166 (Figure 7). Ordinarily, the corrosion rate of carbon steels and other low-alloy steels in seawater follow non-linear dependence upon exposure time with the highest corrosion rates at the earliest exposure times (Heiser and Soo, 1995; Melchers, 2003). This is contrary to observations at U-166 in 2013. On the aft deck, areas of corrosion on the steel hull first observed in 2003 expanded to a greater degree during a shorter interval (2009–2013) after the spill than compared to before (2003–2009) (Figure 6A). The deck of the conning tower shows continuous deterioration over 10 years, with new holes in 2013 (Figure 6B) and a doubling in size of existing holes between 2009 and 2013. In both areas, sediment not observed prior to 2013 covered sections where new holes were emerging, providing another line of evidence of a sedimentation pulse at this location after the spill. Although these data are only available for U-166, a wreck that has been on the seafloor for 76 years, the corrosion rate at this site, revealed through still images, appears orders of magnitude higher than rates reported in the literature, and has accelerated across the time series presented here (Heiser and Soo, 1995; Melchers, 2003). This is unexpected and suggests a connection between spill fallout and metal loss of historic shipwrecks on the seafloor.
Conclusion
This study reveals increased connectivity between sediment and carbon steel biofilm microbiomes in spill-impacted areas. This connectivity may lead to changing functional interactions between biofilms and steel surfaces in the marine environment, possibly leading to oil-induced MIC. Future studies are needed to continue to investigate the interaction between oil spills and the functional capability of bacteria in sediment, water, and biofilms, in general. Exposure of shipwreck surfaces to residual spill contaminants has the potential to alter biofilm taxonomy and functional potential, which may place the biodiversity and the preservation of historic structures in the deep sea at risk.
Author Contributions
LH designed the study. LH and MD carried out field experiments. JS, RM, and LH conducted laboratory analyses. RM, MB, JS, and LH conducted microbiome data analyses. JL participated in metal loss analysis. RC conducted imagery analysis for metal loss estimates. LH and RM wrote the manuscript. All authors contributed to the manuscript.
Funding
Funding was provided by the U.S. Department of the Interior, Bureau of Ocean Energy Management, Environmental Studies Program under Cooperative agreement number M13AC00015 and Interagency agreement number M13PG00020. Ship time was provided through the Naval Research Laboratory Platform Support Program.
Conflict of Interest Statement
RC was employed by C & C Technologies, Inc., now owned by Oceaneering International, Inc.
The remaining authors declare that the research was conducted in the absence of any commercial or financial relationships that could be construed as a potential conflict of interest.
Acknowledgments
The authors thank the Captain and Crew of the R/V Pelican, and the crew of the Global Explorer ROV for support during field work. They are grateful to Ricky Ray for designing and building BMPs, and Christine Figan, Zeima Kassahun, Matthew Johnson and Christine McGown for laboratory assistance. Daniel Warren provided site plans instrumental to the sampling effort. They also thank Yoko Furukawa, Lisa Fitzgerald and Chris Horrell for input on the study.
Supplementary Material
The Supplementary Material for this article can be found online at: https://www.frontiersin.org/articles/10.3389/fmars.2019.00048/full#supplementary-material
References
Abubucker, S., Segata, N., Goll, J., Schubert, A. M., Izard, J., Cantarel, B. L., et al. (2012). Metabolic reconstruction for metagenomic data and its application to the human microbiome. PLoS Comput. Biol. 8:e1002358. doi: 10.1371/journal.pcbi.1002358
Acuña, N., Ortega-Morales, B. O., and Valadez-González, A. (2006). Biofilm colonization dynamics and its influence on the corrosion resistance of austenitic UNS S31603 stainless steel exposed to gulf of Mexico seawater. Mar. Biotechnol. 8, 62–70. doi: 10.1007/s10126-005-5145-7
Aktas, D. F., Lee, J. S., Little, B. J., Ray, R. I., Davidova, I. A., Lyles, C. N., et al. (2010). Anaerobic metabolism of biodiesel and its impact on metal corrosion. Energy Fuels 24, 2924–2928. doi: 10.1021/ef100084j
AlAbbas, F. M., Williamson, C., Bhola, S. M., Spear, J. R., Olson, D. L., Mishra, B., et al. (2013). Influence of sulfate reducing bacterial biofilm on corrosion behavior of low-alloy, high-strength steel (API-5L X80). Int. Biodeterior. Biodegradation 78, 34–42. doi: 10.1016/j.ibiod.2012.10.014
Andrews, S. (2010). FASTQC: A Quality Control Tool For High Throughput Sequence Data. Available at: http://www.bioinformatics.babraham.ac.uk/
Arora, D. P., and Boon, E. M. (2012). Nitric oxide regulated two-component signaling in Pseudoalteromonas atlantica. Biochem. Biophys. Res. Commun. 421, 521–526. doi: 10.1016/j.bbrc.2012.04.037
Asnicar, F., Weingart, G., Tickle, T. L., Huttenhower, C., and Segata, N. (2015). Compact graphical representation of phylogenetic data and metadata with GraPhlAn. PeerJ 3:e1029. doi: 10.7717/peerj.1029
Awan, F., Dong, Y., Wang, N., Liu, J., Ma, K., and Liu, Y. (2018). The fight for invincibility: environmental stress response mechanisms and Aeromonas hydrophila. Microb. Pathog. 116, 135–145. doi: 10.1016/j.micpath.2018.01.023
Barraud, N., Hassett, D. J., Hwang, S.-H., Rice, S. A., Kjelleberg, S., and Webb, J. S. (2006). Involvement of nitric oxide in biofilm dispersal of Pseudomonas aeruginosa. J. Bacteriol. 188, 7344–7353. doi: 10.1128/JB.00779-06
Beech, I. B., and Campbell, S. A. (2008). Accelerated low water corrosion of carbon steel in the presence of a biofilm harbouring sulphate-reducing and sulphur-oxidising bacteria recovered from a marine sediment. Electrochim. Acta 54, 14–21. doi: 10.1016/j.electacta.2008.05.084
Beech, I. B., and Cheung, C. W. S. (1995). Interactions of exopolymers produced by sulfate-reducing bacteria with metal-ions. Int. Biodeterior. Biodegradation 35, 59–72. doi: 10.1016/0964-8305(95)00082-G
Bermont-Bouis, D., Janvier, M., Grimont, P., Dupont, I., and Vallaeys, T. (2007). Both sulfate-reducing bacteria and Enterobacteriaceae take part in marine biocorrosion of carbon steel. J. Appl. Microbiol. 102, 161–168. doi: 10.1111/j.1365-2672.2006.03053.x
Bolger, A. M., Lohse, M., and Usadel, B. (2014). Trimmomatic: a flexible trimmer for Illumina sequence data. Bioinformatics 30, 2114–2120. doi: 10.1093/bioinformatics/btu170
Brakstad, O. G., Nordtug, T., and Throne-Holst, M. (2015a). Biodegradation of dispersed macondo oil in seawater at low temperature and different oil droplet sizes. Mar. Pollut. Bull. 93, 144–152. doi: 10.1016/j.marpolbul.2015.02.006
Brakstad, O. G., Throne-Holst, M., Netzer, R., Stoeckel, D. M., and Atlas, R. M. (2015b). Microbial communities related to biodegradation of dispersed M acondo oil at low seawater temperature with norwegian coastal seawater. Microb. Biotechnol. 8, 989–998. doi: 10.1111/1751-7915.12303
Brauer, J. I., Makama, Z., Bonifay, V., Aydin, E., Kaufman, E. D., Beech, I. B., et al. (2015). Mass spectrometric metabolomic imaging of biofilms on corroding steel surfaces using laser ablation and solvent capture by aspiration. Biointerphases 10, 019003. doi: 10.1116/1.4906744
Brooks, G. R., Larson, R. A., Schwing, P. T., Romero, I., Moore, C., Reichart, G.-J., et al. (2015). Sedimentation pulse in the NE Gulf of Mexico following the 2010 DWH blowout. PLoS One 10:e0132341. doi: 10.1371/journal.pone.0132341
Buchfink, B., Xie, C., and Huson, D. H. (2014). Fast and sensitive protein alignment using DIAMOND. Nat. Methods 12, 59–60. doi: 10.1038/nmeth.3176
Bælum, J., Borglin, S., Chakraborty, R., Fortney, J. L., Lamendella, R., Mason, O. U., et al. (2012). Deep-sea bacteria enriched by oil and dispersant from the deepwater horizon spill. Environ. Microbiol. 14, 2405–2416. doi: 10.1111/j.1462-2920.2012.02780.x
Caporaso, J. G., Kuczynski, J., Stombaugh, J., Bittinger, K., Bushman, F. D., Costello, E. K., et al. (2010). QIIME allows analysis of high-throughput community sequencing data. Nat. Methods 7, 335–336. doi: 10.1038/nmeth.f.303
Chen, Y., Mccarthy, D., Robinson, M., and Smyth, G. K. (2014). edgeR: Differential Expression Analysis of Digital Gene Expression Data User’s Guide. Available at: https://www.bioconductor.org/packages/devel/bioc/vignettes/edgeR/inst/doc/edgeRUsersGuide.pdf
Comeau, A. M., Douglas, G. M., and Langille, M. G. (2017). Microbiome helper: a custom and streamlined workflow for microbiome research. mSystems 2, e127–16. doi: 10.1128/mSystems.00127-16
Comeau, A. M., Li, W. K. W., Tremblay, J. E., Carmack, E. C., and Lovejoy, C. (2011). Arctic ocean microbial community structure before and after the 2007 record sea ice minimum. PLoS One 6:e27492. doi: 10.1371/journal.pone.0027492
Comte, J., Culley, A. I., Lovejoy, C., and Vincent, W. F. (2018). Microbial connectivity and sorting in a high arctic watershed. ISME J. 12, 2988–3000. doi: 10.1038/s41396-018-0236-4
Cullimore, D. R., and Johnston, L. A. (2008). Microbiology of concretions, sediments and mechanisms influencing the preservation of submerged archaeological artifacts. Int. J. Hist. Archaeol. 12, 120–132. doi: 10.1007/s10761-008-0045-y
Damour, M., Church, R., Warren, D., Horrell, C., and Hamdan, L. (2016). “Gulf of mexico shipwreck corrosion, hydrocarbon exposure, microbiology, and archaeology (GOM-SCHEMA) project: studying the effects of a major oil spill on submerged cultural resource,” in Proceedings for the 2015 Society for Historical Archaeology Annual Conference, (Germantown, MD: Society for Historical Archaeology), 51–61.
DeSantis, T. Z., Hugenholtz, P., Larsen, N., Rojas, M., Brodie, E. L., Keller, K., et al. (2006). Greengenes, a chimera-checked 16S rRNA gene database and workbench compatible with ARB. Appl. Environ. Microbiol. 72, 5069–5072. doi: 10.1128/AEM.03006-05
Dombrowski, N., Donaho, J. A., Gutierrez, T., Seitz, K. W., Teske, A. P., and Baker, B. J. (2016). Reconstructing metabolic pathways of hydrocarbon-degrading bacteria from the deepwater horizon oil spill. Nat. Microbiol. 1:16057. doi: 10.1038/nmicrobiol.2016.57
Edgar, R. C. (2013). UPARSE: highly accurate OTU sequences from microbial amplicon reads. Nat. Methods 10, 996–998. doi: 10.1038/nmeth.2604
Enning, D., and Garrelfs, J. (2014). Corrosion of iron by sulfate-reducing bacteria: new views of an old problem. Appl. Environ. Microbiol. 80, 1226–1236. doi: 10.1128/AEM.02848-13
Enning, D., Venzlaff, H., Garrelfs, J., Dinh, H. T., Meyer, V., Mayrhofer, K., et al. (2012). Marine sulfate-reducing bacteria cause serious corrosion of iron under electroconductive biogenic mineral crust. Environ. Microbiol. 14, 1772–1787. doi: 10.1111/j.1462-2920.2012.02778.x
Gao, X., Gao, W., Cui, Z., Han, B., Yang, P., Sun, C., et al. (2015). Biodiversity and degradation potential of oil-degrading bacteria isolated from deep-sea sediments of south mid-atlantic ridge. Mar. Pollut. Bull. 97, 373–380. doi: 10.1016/j.marpolbul.2015.05.065
Garrett, T. R., Bhakoo, M., and Zhang, Z. (2008). Bacterial adhesion and biofilms on surfaces. Prog. Nat. Sci. 18, 1049–1056. doi: 10.1016/j.pnsc.2008.04.001
Girard, F., Shea, K., and Fisher, C. R. (2018). Projecting the recovery of a long-lived deep-sea coral species after the deepwater horizon oil spill using state-structured models. J. Appl. Ecol. 55, 1812–1822. doi: 10.1111/1365-2664.13141
Hamdan, L. J., Coffin, R. B., Sikaroodi, M., Greinert, J., Treude, T., and Gillevet, P. M. (2013). Ocean currents shape the microbiome of arctic marine sediments. ISME J. 7, 685–696. doi: 10.1038/ismej.2012.143
Hamdan, L. J., Salerno, J. L., Reed, A., Joye, S. B., and Damour, M. (2018). The impact of the deepwater horizon blowout on historic shipwreck-associated sediment microbiomes in the northern gulf of Mexico. Sci. Rep. 8:9057. doi: 10.1038/s41598-018-27350-z
Heiser, J. H., and Soo, P. (1995). Corrosion of Barrier Materials in Seawater Environments. New York: Brookhaven National Lab.
Hu, P., Dubinsky, E. A., Probst, A. J., Wang, J., Sieber, C. M., Tom, L. M., et al. (2017). Simulation of deepwater horizon oil plume reveals substrate specialization within a complex community of hydrocarbon degraders. Proc. Natl. Acad. Sci. U.S.A. 114, 7432–7437. doi: 10.1073/pnas.1703424114
Huson, D. H., Beier, S., Flade, I., Górska, A., El-Hadidi, M., Mitra, S., et al. (2016). MEGAN community edition-interactive exploration and analysis of large-scale microbiome sequencing data. PLoS Comput. Biol. 12:e1004957. doi: 10.1371/journal.pcbi.1004957
Inagaki, F., Takai, K., Nealson, K. H., and Horikoshi, K. (2004). Sulfurovum lithotrophicum gen. nov., sp. nov., a novel sulfur-oxidizing chemolithoautotroph within the 𝜀-Proteobacteria isolated from okinawa trough hydrothermal sediments. Int. J. Syst. Evol. Microbiol. 54, 1477–1482. doi: 10.1099/ijs.0.03042-0
Jiménez, N., Viñas, M., Guiu-Aragonés, C., Bayona, J. M., Albaigés, J., and Solanas, A. M. (2011). Polyphasic approach for assessing changes in an autochthonous marine bacterial community in the presence of prestige fuel oil and its biodegradation potential. Appl. Microbiol. Biotechnol. 91, 823–834. doi: 10.1007/s00253-011-3321-4
Joye, S. B., Kleindienst, S., Gilbert, J. A., Handley, K. M., Weisenhorn, P., Overholt, W. A., et al. (2016). Responses of microbial communities to hydrocarbon exposures. Oceanography 29, 136–149. doi: 10.5670/oceanog.2016.78
Knights, D., Kuczynski, J., Charlson, E. S., Zaneveld, J., Mozer, M. C., Collman, R. G., et al. (2011). Bayesian community-wide culture-independent microbial source tracking. Nat. Methods 8, 761–763. doi: 10.1038/nmeth.1650
Lai, Q., Wang, L., Liu, Y., Yuan, J., Sun, F., and Shao, Z. (2011). Parvibaculum indicum sp. nov., isolated from deep-sea water. Int. J. Syst. Evol. Microbiol. 61, 271–274. doi: 10.1099/ijs.0.021899-0
Landoulsi, J., Kirat, K. E., Richard, C., Feron, D., and Pulvin, S. (2008). Enzymatic approach in microbial-influenced corrosion: a review based on stainless steels in natural waters. Environ. Sci. Technol. 42, 2233–2242. doi: 10.1021/es071830g
Langmead, B., and Salzberg, S. L. (2012). Fast gapped-read alignment with Bowtie 2. Nat. Methods 9, 357–359. doi: 10.1038/nmeth.1923
Lee, J. S., Mcbeth, J. M., Ray, R. I., Little, B. J., and Emerson, D. (2013). Iron cycling at corroding carbon steel surfaces. Biofouling 29, 1243–1252. doi: 10.1080/08927014.2013.836184
Li, J., and Wang, N. (2011). Genome-wide mutagenesis of Xanthomonas axonopodis pv. citri reveals novel genetic determinants and regulation mechanisms of biofilm formation. PLoS One 6:e21804. doi: 10.1371/journal.pone.0021804
Little, B. J., and Lee, J. S. (2014). Microbiologically influenced corrosion: an update. Int. Mater. Rev. 59, 384–393. doi: 10.1179/1743280414Y.0000000035
Little, B. J., Lee, J. S., and Ray, R. I. (2008). The influence of marine biofilms on corrosion: a concise review. Electrochim. Acta 54, 2–7. doi: 10.1016/j.electacta.2008.02.071
Love, M. I., Huber, W., and Anders, S. (2014). Moderated estimation of fold change and dispersion for RNA-seq data with DESeq2. Genome Biol. 15:550. doi: 10.1186/s13059-014-0550-8
Marietou, A., Chastain, R., Scoma, A., Hazen, T. C., and Bartlett, D. H. (2018). The effect of hydrostatic pressure on enrichments of hydrocarbon degrading microbes from the gulf of mexico following the deepwater horizon oil spill. Front. Microbiol. 9:808. doi: 10.3389/fmicb.2018.00808
Mason, O. U., Han, J., Woyke, T., and Jansson, J. K. (2014). Single-cell genomics reveals features of a Colwellia species that was dominant during the deepwater horizon oil spill. Front. Microbiol. 5:332. doi: 10.3389/fmicb.2014.00332
McBeth, J. M., and Emerson, D. (2016). In situ microbial community succession on mild steel in estuarine and marine environments: exploring the role of iron-oxidizing bacteria. Front. Microbiol. 7:767. doi: 10.3389/fmicb.2016.00767
McBeth, J. M., Little, B. J., Ray, R. I., Farrar, K. M., and Emerson, D. (2011). Neutrophilic iron-oxidizing “Zetaproteobacteria” and mild steel corrosion in nearshore marine environments. Appl. Environ. Microbiol. 77, 1405–1412. doi: 10.1128/AEM.02095-10
Melchers, R. E. (2003). Modeling of marine immersion corrosion for mild and low-alloy steels—Part 1: Phenomenological model. Corrosion 59, 319–334. doi: 10.5006/1.3277564
Nithya, C., Begum, M. F., and Pandian, S. K. (2010). Marine bacterial isolates inhibit biofilm formation and disrupt mature biofilms of Pseudomonas aeruginosa PAO1. Appl. Microbiol. Biotechnol. 88, 341–358. doi: 10.1007/s00253-010-2777-y
Orcutt, B. N., Joye, S. B., Kleindienst, S., Knittel, K., Ramette, A., Reitz, A., et al. (2010). Impact of natural oil and higher hydrocarbons on microbial diversity, distribution, and activity in gulf of mexico cold-seep sediments. Deep Sea Res. Part 2 Top. Stud. Oceanogr. 57, 2008–2021. doi: 10.1016/j.dsr2.2010.05.014
Pereira, M. B., Wallroth, M., Jonsson, V., and Kristiansson, E. (2018). Comparison of normalization methods for the analysis of metagenomic gene abundance data. BMC Genomics 19:274. doi: 10.1186/s12864-018-4637-6
Pi, Y., Chen, B., Bao, M., Fan, F., Cai, Q., Ze, L., et al. (2017). Microbial degradation of four crude oil by biosurfactant producing strain rhodococcus sp. Bioresour. Technol. 232, 263–269. doi: 10.1016/j.biortech.2017.02.007
Qian, P.-Y., Lau, S. C., Dahms, H.-U., Dobretsov, S., and Harder, T. (2007). Marine biofilms as mediators of colonization by marine macroorganisms: implications for antifouling and aquaculture. Mar. Biotechnol. 9, 399–410. doi: 10.1007/s10126-007-9001-9
Rahul, K., Azmatunnisa, M., Sasikala, C., and Ramana, C. V. (2015). Hoeflea olei sp. nov., a diesel-oil-degrading, anoxygenic, phototrophic bacterium isolated from backwaters and emended description of the genus Hoeflea. Int. J. Syst. Evol. Microbiol. 65, 2403–2409. doi: 10.1099/ijs.0.000277
Rampadarath, S., Bandhoa, K., Puchooa, D., Jeewon, R., and Bal, S. (2017). Early bacterial biofilm colonizers in the coastal waters of Mauritius. Electron. J. Biotechnol. 29, 13–21. doi: 10.1016/j.ejbt.2017.06.006
Robinson, M. D., and Oshlack, A. (2010). A scaling normalization method for differential expression analysis of RNA-seq data. Genome Biol. 11:R25. doi: 10.1186/gb-2010-11-3-r25
Salerno, J. L., Little, B., Lee, J., and Hamdan, L. J. (2018). Exposure to crude oil and chemical dispersant may impact marine microbial biofilm composition and steel corrosion. Front. Mar. Sci. 5:196. doi: 10.3389/fmars.2018.00196
Sierra-García, I. N., Alvarez, J. C., De Vasconcellos, S. P., De Souza, A. P., Dos Santos Neto, E. V., and De Oliveira, V. M. (2014). New hydrocarbon degradation pathways in the microbial metagenome from Brazilian petroleum reservoirs. PLoS One 9:e90087. doi: 10.1371/journal.pone.0090087
Singer, E., Emerson, D., Webb, E. A., Barco, R. A., Kuenen, J. G., Nelson, W. C., et al. (2011). Mariprofundus ferrooxydans PV-1 the first genome of a marine Fe (II) oxidizing Zetaproteobacterium. PloS one 6:e25386. doi: 10.1371/journal.pone.0025386
Storesund, J. E., Sandaa, R.-A., Thingstad, T. F., Asplin, L., Albretsen, J., and Erga, S. R. (2017). Linking bacterial community structure to advection and environmental impact along a coast-fjord gradient of the Sognefjord, western Norway. Progr. Oceanogr. 159, 13–30. doi: 10.1016/j.pocean.2017.09.002
Stout, S. A., Rouhani, S., Liu, B., Oehrig, J., Ricker, R. W., Baker, G., et al. (2017). Assessing the footprint and volume of oil deposited in deep-sea sediments following the Deepwater Horizon oil spill. Mar. Pollut. Bull. 114, 327–342. doi: 10.1016/j.marpolbul.2016.09.046
Svane, I., and Petersen, J. K. (2001). On the problems of epibioses, fouling and artificial reefs, a review. Mar. Ecol. 22, 169–188. doi: 10.1046/j.1439-0485.2001.01729.x
Teitzel, G. M., and Parsek, M. R. (2003). Heavy metal resistance of biofilm and planktonic Pseudomonas aeruginosa. Appl. Environ. Microbiol. 69, 2313–2320. doi: 10.1128/AEM.69.4.2313-2320.2003
Truong, D. T., Franzosa, E. A., Tickle, T. L., Scholz, M., Weingart, G., Pasolli, E., et al. (2015). MetaPhlAn2 for enhanced metagenomic taxonomic profiling. Nat. Methods 12, 902–903. doi: 10.1038/nmeth.3589
Valentine, D. L., Fisher, G. B., Bagby, S. C., Nelson, R. K., Reddy, C. M., Sylva, S. P., et al. (2014). Fallout plume of submerged oil from deepwater horizon. Proc. Natl. Acad. Sci. U.S.A. 111, 15906–15911. doi: 10.1073/pnas.1414873111
Vigneron, A., Alsop, E. B., Chambers, B., Lomans, B. P., Head, I. M., and Tsesmetzis, N. (2016). Complementary microorganisms in highly corrosive biofilms from an offshore oil production facility. Appl. Environ. Microbiol. 82, 2545–2554. doi: 10.1128/AEM.03842-15
Vila, J., Nieto, J. M., Mertens, J., Springael, D., and Grifoll, M. (2010). Microbial community structure of a heavy fuel oil-degrading marine consortium: linking microbial dynamics with polycyclic aromatic hydrocarbon utilization. FEMS Microbiol. Ecol. 73, 349–362. doi: 10.1111/j.1574-6941.2010.00902.x
Yeager, K. M., Santschi, P. H., and Rowe, G. T. (2004). Sediment accumulation and radionuclide inventories (239,240 Pu, 210Pb and 234Th) in the northern gulf of mexico, as influenced by organic matter and macrofaunal density. Mar. Chem. 91, 1–14. doi: 10.1016/j.marchem.2004.03.016
Keywords: biofilm, shipwreck, microbiome, metagenome, corrosion, Gulf of Mexico, Deepwater Horizon oil spill, deep sea
Citation: Mugge RL, Brock ML, Salerno JL, Damour M, Church RA, Lee JS and Hamdan LJ (2019) Deep-Sea Biofilms, Historic Shipwreck Preservation and the Deepwater Horizon Spill. Front. Mar. Sci. 6:48. doi: 10.3389/fmars.2019.00048
Received: 06 October 2018; Accepted: 29 January 2019;
Published: 14 February 2019.
Edited by:
Dennis A. Bazylinski, University of Nevada, Las Vegas, United StatesReviewed by:
Zhanfei Liu, The University of Texas at Austin, United StatesAndrew R. Thurber, Oregon State University, United States
Copyright © 2019 Mugge, Brock, Salerno, Damour, Church, Lee and Hamdan. This is an open-access article distributed under the terms of the Creative Commons Attribution License (CC BY). The use, distribution or reproduction in other forums is permitted, provided the original author(s) and the copyright owner(s) are credited and that the original publication in this journal is cited, in accordance with accepted academic practice. No use, distribution or reproduction is permitted which does not comply with these terms.
*Correspondence: Leila J. Hamdan, leila.hamdan@usm.edu