- 1Department of Civil and Environmental Engineering, Stanford University, Stanford, CA, United States
- 2School of Biological Sciences, University of Hong Kong, Pok Fu Lam, Hong Kong
Coastal marine ecosystems provide numerous invaluable services and benefits to humankind. However, urbanization of coastal areas has homogenized and reduced the biodiversity of the surrounding marine environment and the sustainability of the multiple ecosystem services it provides. Studies have focused on single ecosystem functions despite human populations relying on several functions being delivered at once (known as multifunctionality). This study investigates five ecosystem functions (primary productivity, herbivory, predation, organic matter decomposition and carbon sequestration) and overall multifunctionality in four sites along a gradient of 16 environmental parameters. Ecosystem function varied significantly between sites that were farthest apart. In determining factors that drove ecosystem functioning, we found a positive relationship between coral cover and primary productivity but negative relationships between coral cover and levels of herbivory and predation intensity. Higher temperatures and greater concentrations of chlorophyll-a had a positive impact on predation and herbivory, respectively. Notably, we found a significant negative impact of total inorganic nitrogen and significant positive impact of total Kjeldahl nitrogen on carbon sequestration. Further, individual functions were compared with fish abundance (obtained from seawater eDNA), and benthic community composition (obtained from plate % coverage of autonomous reef monitoring structures). Increasing herbivorous fish abundance had a positive impact on Ulva mass loss. Overall, relative abundance of predatory, omnivorous and planktivorous fish exerted overriding influences on primary productivity and predation intensity, implying that fishing pressure and marine protected area status are important factors. Importantly, we found significant effects from environmental parameters indicating that reliably predicting the effects of future anthropogenic impacts will not be straightforward as multiple drivers are likely to have complex effects. Taken together, urbanized coastal ecosystems exhibit varying levels of multifunctionality depending on the extent of human impact, and the functional diversity of the benthic community present.
Introduction
Marine environments are significant contributors to both local and global economies, providing ecosystem services and jobs to local people on the one hand, and representing a great bank of undiscovered species, including many of potential importance for human health, on the other. However, of the many changes underway in the world today, one of the most striking is the decline and homogenization of marine biodiversity through overexploitation, climate change, habitat loss, nutrient pollution and species invasions (Meyer et al., 2016). This raises considerable concern, since human society depends on the ocean for its services and the loss of marine biodiversity has detrimental impacts on the environment. For example, Villnas et al. (2013) demonstrated that repetitive anthropogenic stressors result in the gradual degradation of individual functions in sedimentary ecosystems. Additionally, based on (a) experimental systems and (b) individual functions, Gamfeldt et al. (2015) concluded that loss of marine biodiversity likely decreases ecosystem function and impacts habitat resilience. Specific to coral reefs, Lefcheck et al. (2019) demonstrated that tropical fish diversity significantly impacts functioning and Brandl et al. (2019) observed the same relationship between biodiversity and ecosystem functioning. In the last decade, an increasing number of studies have arrived at similar conclusions about the biodiversity-ecosystem function relationship in natural systems (non-experimental, ‘real-world’ ecosystems; drylands – Maestre et al., 2012; Berdugo et al., 2017, grasslands – Soliveres et al., 2016, forests – Zhang and Wang, 2012).
Our understanding of ecosystem function, however, is not only far from complete but is also biased toward experiments that have been conducted (1) over a small-scale, (2) with a few species (3) across a few lower trophic levels (Duffy et al., 2007; Nelson et al., 2009; Berdugo et al., 2017) and (4) are biased toward single functions (Stachowicz et al., 2007; Cardinale et al., 2011; Byrnes et al., 2014). In the last decade, efforts have been made to change this trend, by expanding ecosystem function studies across spatial scales (Thompson et al., 2018; Gonzalez et al., 2020). A recent meta-analysis by Duffy et al. (2017) synthesizes these biodiversity effects from multiple ecosystem types. Specifically, Ptacnik et al. (2008); Mora et al. (2011), Zimmerman and Cardinale (2013); Duffy et al. (2015)Duffy et al. (2016), and García-Comas et al. (2016) present measured effects of eelgrass, fish species and plankton diversity on reef ecosystem functioning after statistically controlling for environmental covariables.
Ecosystem function components, when considered synergistically, or additively can have different and likely stronger effects on biodiversity (Hector and Bagchi, 2007; Hautier et al., 2017; Meyer et al., 2018). For instance, Maestre et al. (2012) used a natural experiment to test the biodiversity-ecosystem function relationship (BEF; Duffy, 2009; Gamfeldt and Roger, 2017) between plant species richness and multiple functions in semi-arid ecosystems. This proved to be timely because previous work on dryland systems had only assessed ecosystem functions individually. This led to the concept of a habitat’s “multifunctionality” which was defined as the “simultaneous provision of multiple functions” (Hector and Bagchi, 2007; Gamfeldt et al., 2008). Recently, in a study by Byrnes et al. (2014), the authors suggested that aspects of the BEF relationship, which continue to remain unresolved in several marine ecosystems, could be tested stringently when multifunctionality is taken into account and quantified. Taking this forward, Lefcheck et al. (2015) highlighted the importance of taking multiple ecosystem functions into consideration to understand the effect of biodiversity on integrated functioning, an aspect that is unclear when only individual functions are analyzed. By presenting evidence of biodiversity’s effect on multifunctionality, the authors demonstrated that communities with higher species richness maintain a higher number of ecosystem functions than those with lower species richness. In this manner, the concept of multifunctionality became useful to understand ecosystem functioning in fundamental and applied ecology.
The measurement of multifunctionality, however, has proven extremely challenging as it takes into consideration only a small subset of all possible individual ecosystem functions. To our knowledge, there is no standardized way of defining or carefully accounting for specific functions that will capture an ecosystem’s “true” multifunctionality (Manning et al., 2018). For instance, Alsterberg et al. (2014) illustrated that there were no effects of nutrient enrichment, toxicants, sedimentation and warming on marine ecosystem multifunctionality. However, a few years later, Alsterberg et al. (2017) demonstrated that habitat diversity has a direct effect on marine ecosystem functionality. As a result, data on ecosystem functioning of marine ecosystems are especially limited and fragmented, despite the fact that such information could help inform effective management of crucial environments (Manning et al., 2018).
The last decade has seen a surge in the development of methods to quantify ecosystem multifunctionality (Maestre et al., 2012 – the averaging approach; Gamfeldt et al., 2008; Byrnes et al., 2014 – single and multiple threshold approaches). Manning et al. (2018) introduced two additional components - ecosystem function (EF) multifunctionality and ecosystem services (ES) multifunctionality to help environmental managers and economists quantify the value of ecosystems using monetary units and life satisfaction. Recently, Hoölting et al. (2019) proposed a new way of measuring multifunctionality across spatial scales. Although these methods have been reasonably successful in assessing an ecosystem’s performance (Hensel and Silliman, 2013 reviews a few of the methods listed), the literature cited have not yet completely addressed how biodiversity when coupled with environmental factors influences ecosystem function, especially for urbanized coastal marine ecosystems. Therefore, it is crucial to understand the resilience of coastal marine ecosystems in response to global change and environmental stressors.
This is especially relevant to a coastal megacity such as Hong Kong, where eutrophication (nutrient-driven marine pollution) has contributed to a marked reduction in water quality (Duprey et al., 2017) and loss of critical habitats such as hard corals, thus reducing the complexity, diversity and function of benthic ecosystems (Scott, 1990; Fabricius and McCorry, 2006). For example, Tolo Harbour, in northeast Hong Kong was once a pristine, coral-fringed bay home to various coral communities and vibrant fisheries (Morton, 1989). However, several sites in the harbor have experienced a dramatic loss of coral cover from >60% to <10%. In 2015, we utilized stable isotope analysis to understand nitrogen source dynamics in both wastewater effluents and receiving seawaters in the Tolo Channel and found sewage effluent to be the dominant source of nitrogen pollution (Archana et al., 2016, 2018). Consistently, Wong et al. (2017) measured δ15N of hard corals from several sites in this region, which also revealed strong human-derived nutrient signals. Today, we see a punctuated gradient in water quality with nutrient concentrations decreasing with distance from coastal populations. Yet we have little understanding of how these eutrophic conditions affect the ecosystem function of such benthic marine communities and their multifunctionality.
Here, we analyzed ecosystem processes – primary productivity, herbivory, predation, organic matter decomposition, carbon sequestration and overall ecosystem multifunctionality along a gradient of environmental parameters in an urbanized coastal marine environment. We used environmental data (nutrient concentrations, dissolved oxygen, chlorophyll A, pH, salinity, turbidity, secchi depth, temperature, and suspended solids) to test the hypothesis that, ecosystem multifunctionality is significantly correlated with geochemical parameters. Next, we used abundance data of functional groups from annotated settlement plate photos of autonomous reef monitoring structures (ARMS) deployed in the same sites to test the hypothesis that increased abundance of benthic taxa is significantly correlated with ecosystem functioning. We used fish abundance data from eDNA of water samples collected from the same sites to test the hypothesis that increased fish abundance significantly alters ecosystem functioning.
Materials and Methods
Study Area
The study was undertaken over a period of 1 year across four field sites within Tolo Harbour, Hong Kong: Center Island – CI, Che Lei Pai – CLP, Port Island – PI, and Tung Ping Chau – TPC (Figure 1). These sites represent a gradient in coral cover, CI < CLP < PI < TPC (Duprey et al., 2016; Wong et al., 2017) and several environmental parameters. Center Island is the site closest to the harbor, with almost no hard corals, and is also the most polluted compared to the other sites. Tung Ping Chau, on the other hand is a marine reserve with over 60 species of hard corals. The study used a simple toolkit to allow the quantification of key ecosystem functions and overall multifunctionality.
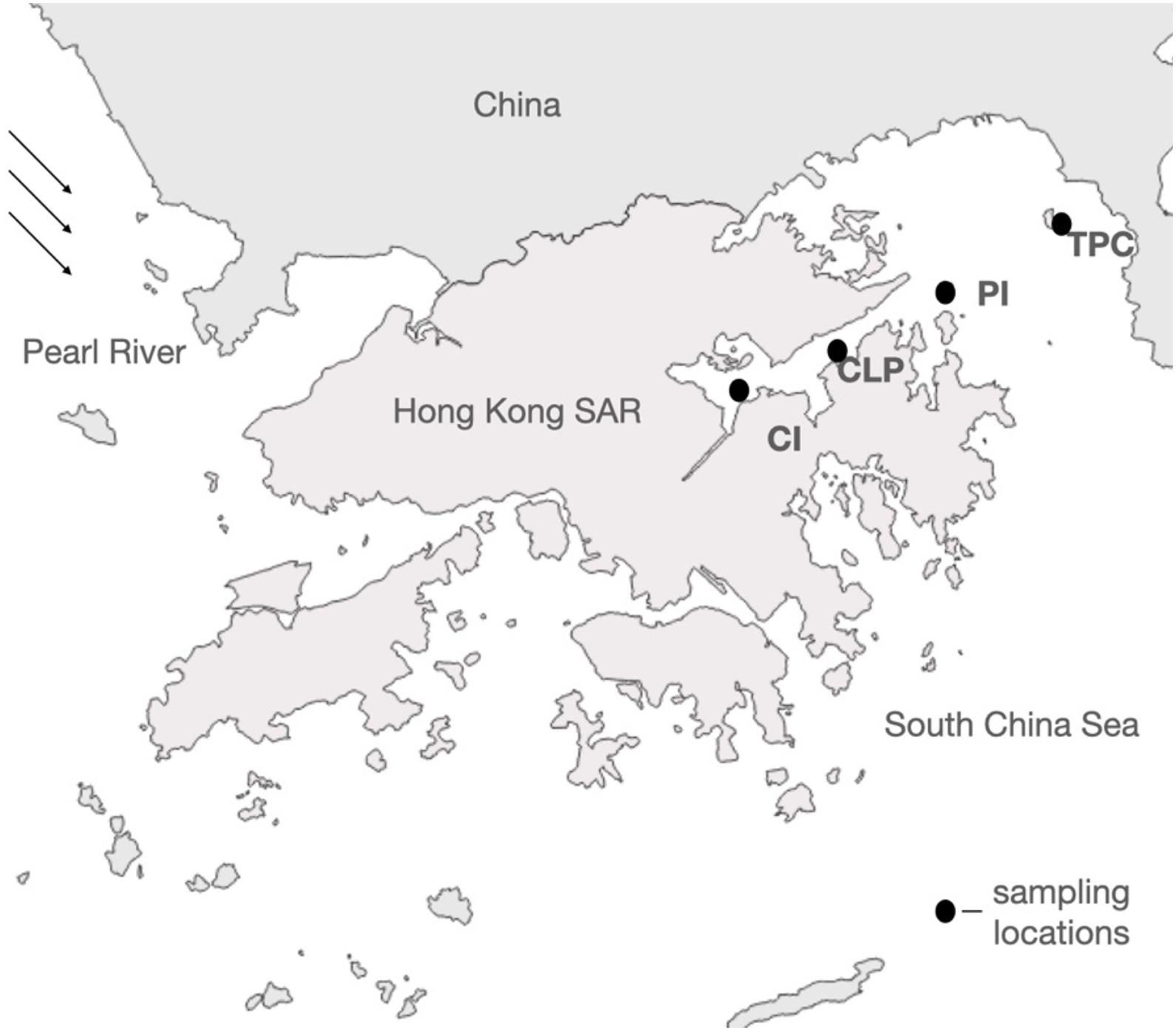
Figure 1. Map showing four study sites in Tolo Harbour (Center Island: CI, Che Lei Pai: CLP, Port Island: PI and Tung Ping Chau: TPC) in Hong Kong’s marine environment.
Primary Productivity
Primary productivity is the rate at which energy is stored as organic matter (Fahey and Knapp, 2007). We measured primary productivity as net mass gain (%) of macroalgae (Ulva) on substrata protected from grazers. We deployed four replicates at each site in plastic bottles with holes, 4 m from the ocean floor (to standardize light availability). Ropes were attached to bricks and deployed on the substratum at 5–10 m intervals (Figure 2). After 48 h, we collected the macroalgae, returned them to the laboratory and assessed their mass loss/gain (ΔM) as follows:
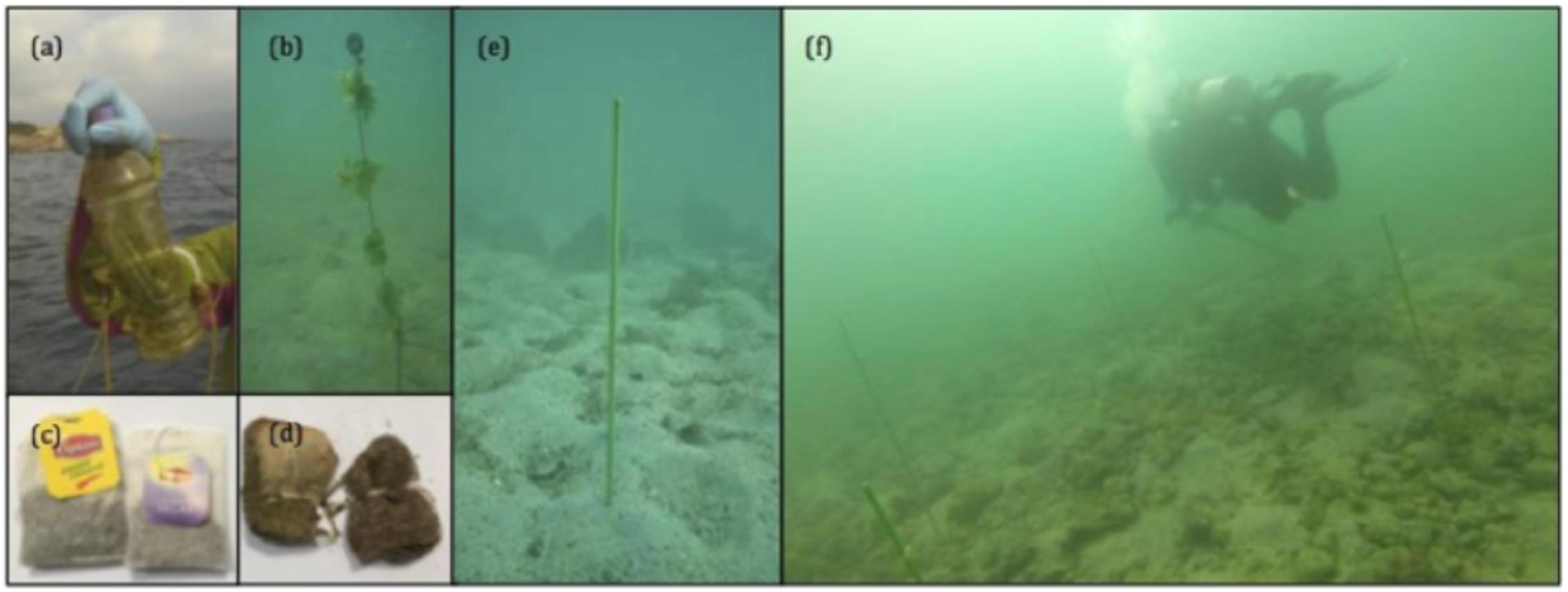
Figure 2. Ecosystem function assays for (a) primary productivity using Ulva in closed plastic bottles (b) herbivory using Ulva tied to ropes (c) green and rooibos tea bags shown before decomposition and upon retrieval (d) after 6 months of deployment (e) and (f) predation using squid pops.
ΔM = (Mf − Mi)/Mi
Where i and f refer to initial and final wet weights as per Rasher et al. (2013).
Herbivory/Grazing Intensity
Herbivores play a vital role in maintaining a healthy coral-dominated community through intense feeding and grazing of unwanted macroalgae that indirectly interfere with the growth, reproduction and survivorship of corals (Burkepile and Hay, 2008). To estimate herbivory, we exposed samples of macroalgae (referred to as “algae pops”) to determine their susceptibility to grazing. The macroalgae were deployed on 90 cm lengths of three-stranded nylon rope, upon which the algae had been grown for 2 weeks prior to use (Figure 2). At each site four replicates were deployed and spaced 5–10 m apart on the sea floor. Following exposure for 48 h, loss of biomass (ΔC) was calculated as follows:
ΔC = {[(1 + ΔM)∗Mi] − Mf}/Mi
Where i and f refer to initial and final wet weights as per Rasher et al. (2013) and ΔM is the % mass gain calculated from the primary productivity assay.
Predation
Fish feeding intensity is often used as a measure of predation in a shallow water ecosystem. A simple assay was used to assess predation – “the squid pop” (Duffy et al., 2015). This refers to a piece of dried squid deployed at the end of a tether tied to a stake as bait for fish. In order to standardize the squid pop assay, we cut the dried squid into disks that all had the same size. Following this, the disks were deployed from the seafloor by tethering them to individual metal stakes using fishing line. Squid pops were deployed at three sites (CI, CLP, PI; 25 replicates per site). Squid pops were not deployed in the fourth site – TPC due to inclement weather forecast and budget restrictions. One hour after deployment, we returned to observe whether or not fish consumed the bait. The degree to which the squid pops were consumed was used as an indicator of the level of predation (or the activity of fish feeding) in the marine ecosystem (Duffy et al., 2015; Figure 2).
Bait loss = 1 − [(Si − Sf)/Si]
Where i and f refer to the initial and final number of squid pops, respectively.
Organic Matter Decomposition and Carbon Sequestration
To measure organic matter decomposition and carbon sequestration potential, we used the tea bag assay as per Keuskamp et al. (2013). The tea bag assay was developed to measure organic matter degradability in a standardized, cost-effective manner across diverse ecosystems globally. Recent studies have demonstrated its usefulness and its applicability across aquatic and terrestrial ecosystems (Al-Maliki and Al-Masoudi, 2018; Mueller et al., 2018; Duddigan et al., 2020). Further, Seelen et al. (2019) demonstrated the applicability of the tea bag method to marine environments by incorporating a leaching factor (40% for green tea and 20% for rooibos tea).
We deployed 10 pairs of commercial rooibos tea bags and green tea bags (rooibos tea – ASIN: B00BAUF9KA, Lipton, Unilever, United Kingdom and green tea – B0173LLHNM, Lipton, Unilever, United Kingdom) in nylon mesh (200 μm) that were held within a porous plastic bottle and buried ∼10 cm beneath the sea floor for a period of 6 months in the four study sites (Figure 2). Upon retrieval, the tea bags were oven-dried at 60°C for at least 72 h and weighed after removing the mesh bags. Decomposition rate (k, d–1) of rooibos tea and organic matter stabilization (S, %) of green tea were calculated using the following equations:
k = −Ln [(final mass − initial mass)/duration of deployment]
s = 1 − [(final mass − initial mass)/initial mass of tea] × 100.
Multifunctionality
In order to assess the ability of a given site to perform multiple ecosystem functions, we calculated a multifunctionality index following a geometric mean approach and a multiple threshold based approach (Byrnes et al., 2014). For each of the five functions (primary productivity, herbivory, predation, organic matter decomposition and carbon sequestration), we defined “maximum functioning” as the highest value among the recorded observations. Following this we calculated the standardized percent functioning for each function and took the geometric mean of these values to get the multifunctionality index for every site. Next, we selected a multifunctionality threshold (50% of the calculated maximum functioning values) to evaluate the effect of coral species richness on ecosystem function. The rationale for this is that Gamfeldt et al. (2008) made reasonable observations assuming a 50% functioning threshold to study the effects of species loss on multifunctionality. We recorded 4 values between 0 and 1 for each site, where 1 represented a function was maintained over the selected threshold and 0 represented a function that was maintained below this threshold. We took the sum of these values for each site, which resulted in numbers between 0 and 4, with 4 indicating that the site was able to maintain all ecosystem functions above the specified threshold.
ARMS Plate Photo Annotations
Plate photos from 12 autonomous reef monitoring structures (ARMS), that were deployed in the same four study sites were analyzed and annotated by functional group. The ARMS are similar to mini apartment blocks that are made of 9 stacked PVC plates and are deployed on the sea floor. The structures have cave-like spaces for marine fauna to crawl in, hide and settle. Following deployment for 2 years, the ARMS were retrieved, and everything on the plates was collected and identified (Leray and Knowlton, 2014). During this step, high resolution photographs of individual plates were taken (Figure 3). Similar to settlement plates, ARMS plate photos can be used to assess the diversity, coverage and size of sessile taxa. Recently, David et al. (2019) validated ARMS as a promising monitoring tool for hard bottomed communities and to investigate environmental effects. An online software CoralNet, a repository and resource for benthic image analysis was used that helped generate 50 random points on each plate photo (Beijbom et al., 2015). The software uses computer algorithms that allows fully and semi automated annotation. Based on already identified taxa, the organisms on the plates were assigned to various taxonomic groups such as sponges, bryozoans, bivalves, etc. The assignments were made based on annotation categories used by the NOAA CRED program that is already available on CoralNet. In total, 204 plate photos were annotated at the functional level. Relationships between the abundance of various taxonomic groups on ARMS plates and individual ecosystem functions were assessed to understand the mechanisms that drive variability in functionality.
eDNA Data on Fish Abundance
Seawater Sampling
Seawater samples were collected in August 2016 from the surface in 1-L Nalgene bottles (3 replicates per site from the four study sites) and filtered through a 47 mm diameter PES filter (nominal pore size, 0.22 μm; Millipore Express). Filters were wrapped in aluminum foil and stored at −20°C for DNA extraction.
DNA Extraction
DNA was extracted in triplicate from every sampling site, using the DNeasy Blood and Tissue Kit (Qiagen) according to the manufacturer protocol and supplemented with a few steps: first, the filter was cut into 4 pieces and put into a UV-sterilized 1.5 mL tube. Next, 180 μL ATL was added to each tube. Following this, 20 μL Proteinase K was added to each tube individually and vortexed. The tubes were vortexed a few more times during the day and incubated at 55°C overnight. To increase the yield, the DNA was eluted with 100 μL Buffer AE twice. Next, following incubation at room temperature for 5 min, the spin column was spun at 8000 RPM for 1 min. For the remaining steps, we followed the manufacturer’s protocol. The extracted DNA was stored at −20°C. To monitor contamination, a blank filter was used as negative control. DNA from four fish species (Nemipterus bathybius, Gambusia affinis, Xiphophorus helleri, and Macropodus opercularis) was extracted and used as the positive control.
Library Preparation
We chose a set of universal PCR primers for metabarcoding eDNA from fishes in subtropical habitats (MiFish-U). The primers amplify an approximately 163–185 bp region of the 12S rRNA gene, which is known to resolve taxonomy of most fishes to the family level (Miya et al., 2015). We used a two-step PCR amplification protocol. In the first round of PCR amplification, 12 samples with the universal fish primer pairs (MiFish-U) were used. The PCR amplification was done in 30 cycles and the total reaction volume was 12 μL, containing 6 μL 2 × KAPA HiFi HotStart ReadyMix (KAPA Biosystems, Wilmington, MA, United States), 0.36 μL of each primer (10 μM), 2.0 μL of template and 3.28 μL of sterile distilled H2O. The thermal cycle profile was as follows: initial 3 min denaturation step at 95°C followed by denaturation at 98°C for 30 s; annealing at 63°C for 30 s; extension at 72°C for 20 s; and a final extension at 72°C for 5 min. Collected PCR products were purified using the MinElute Kit (Qiagen) with an elution volume of 10 μL. DNA fragments were recovered and ready to use in downstream analysis. The concentration of DNA was measured using a Thermo ScientificTMμDropTM Plate. The second round of PCR followed by library preparation and sequencing were performed at Genewiz (Suzhou, China) following protocols described in Miya et al. (2015). Indexed amplicon libraries were constructed, pooled and multiplexed sequenced using the Illumina MiSeq platform.
Sequencing Data Optimization
For base calling and preliminary analysis of the raw data, we used Bcl2fastq (v2.17.1.14). To optimize the raw sequencing data – (a) two sequences of each read pair were merged according to overlapping sequences, after which undetermined bases were removed (b) Primers and adapter sequences were removed (c) the 5′ and 3′ bases with Qphred score < 20 were trimmed (d) resulting sequences >200 bp in length passed this stage of optimization. Cutadapt (v1.9.6), Qiime (1.9.1), and Vsearch (1.9.6) were used (see Supplementary Information for raw data statistics).
Taxonomic Assignment
OTU refers to an operational definition of a classification unit (genus, species, grouping, etc.) used commonly for data analysis. All the sequences in a sample were classified to obtain information on species and genus. By classification, the sequences were grouped according to their similarity, and one group is an OTU. First, unique sequences were extracted from optimized sequences with read count information. Next, OTU clustering of unique sequences (read count > 1) was performed with similarity of 97%, and chimeric sequences were further removed to obtain representative OTU sequences. The RDP classifier was used to select and annotate the representative sequence for each OTU to obtain the community composition of each sample. Community composition was subsequently analyzed and summarized using Silva_128 12S rRNA database for species annotation. The relationship between fish community composition (Supplementary Appendix Tables A.4, A.5) and ecosystem function was characterized to understand the factors driving its variability.
Environmental Data
Environmental data was obtained from surface seawater samples collected from the four study sites by the Hong Kong Environmental Protection Department in 2016 (10 replicates per site, every month). The parameters used for the analysis were – turbidity, total phosphorous, total nitrogen, total Kjeldahl nitrogen (TKN), total inorganic nitrogen (TIN), temperature, suspended solids, secchi depth, salinity, pH, orthophosphate, nitrate-nitrogen, ammonia-nitrogen, dissolved oxygen, chlorophyll-a, and coral cover (Supplementary Appendix Table A.1).
Data Analysis
Data were screened for normality using a Shapiro–Wilk W test and for homoscedasticity using Levene’s test. One-way ANOVA was used to evaluate the variability of primary productivity (% mass gain), herbivory (% mass consumed), predation (% bait loss), decomposition rate and carbon sequestration by habitat. Post hoc comparisons to test for significant differences between sites were conducted using Student’s t-test and Tukey’s test. Generalized linear regression was performed with second-order AICc (Hurvich and Tsai, 1989) model selection to obtain the model that could best explain the relationship between the parameters. All statistical analyses were performed in JMP 15.0. Visualizations were carried out using Datagraph.
Results
We present our analyses on the level of individual functions and joint ecosystem functioning (or multifunctionality) in four habitats that have varying environmental parameters, fish abundance and benthic community composition. We analyzed the effect of urbanization, as reflected in the environmental variables on multiple ecosystem functions – primary productivity, predation, herbivory, decomposition and carbon storage. Next, we use two methods to assess multifunctionality – the average approach and the 0.5 threshold approach. Finally, we evaluate the effects of fish abundance and benthic community composition on individual ecosystem functions and overall multifunctionality.
Individual Ecosystem Functions
Net mass gained by protected Ulva (primary productivity), net mass loss by exposed Ulva (herbivory), loss of squid pop (bait) to predators (predation intensity), and carbon sequestration varied significantly among the four habitats (see Supplementary Materials 1–5 for one-way ANOVA results); One-way ANOVA; n = 47; F(3,44) = 8.9 p = 0.0001; One-way ANOVA; n = 47; F(3,44) = 301.2 p < 0.001; One-way ANOVA; n = 47; F(3,44) = 21.1 p < 0.001; n = 47; F(3,44) = 3.4 p = 0.03, respectively; Figure 4. Post hoc Tukey HSD tests revealed significant differences between the farthest sites (Tung Ping Chau and Center Island) for primary productivity (p = 0.0001), herbivory (p = 0.0001), and predation (p = 0.0001); and adjacent sites for carbon sequestration (Che Lei Pai and Port Island p = 0.04), primary productivity (Tung Ping Chau and Che Lei Pai p = 0.0007; Tung Ping Chau and Port Island p = 0.02), herbivory (all site pair comparisons p < 0.001) and predation (Tung Ping Chau and Che Lei Pai p < 0.0001; Center Island and Port Island p = 0.0001; Tung Ping Chau and Port Island p = 0.04).
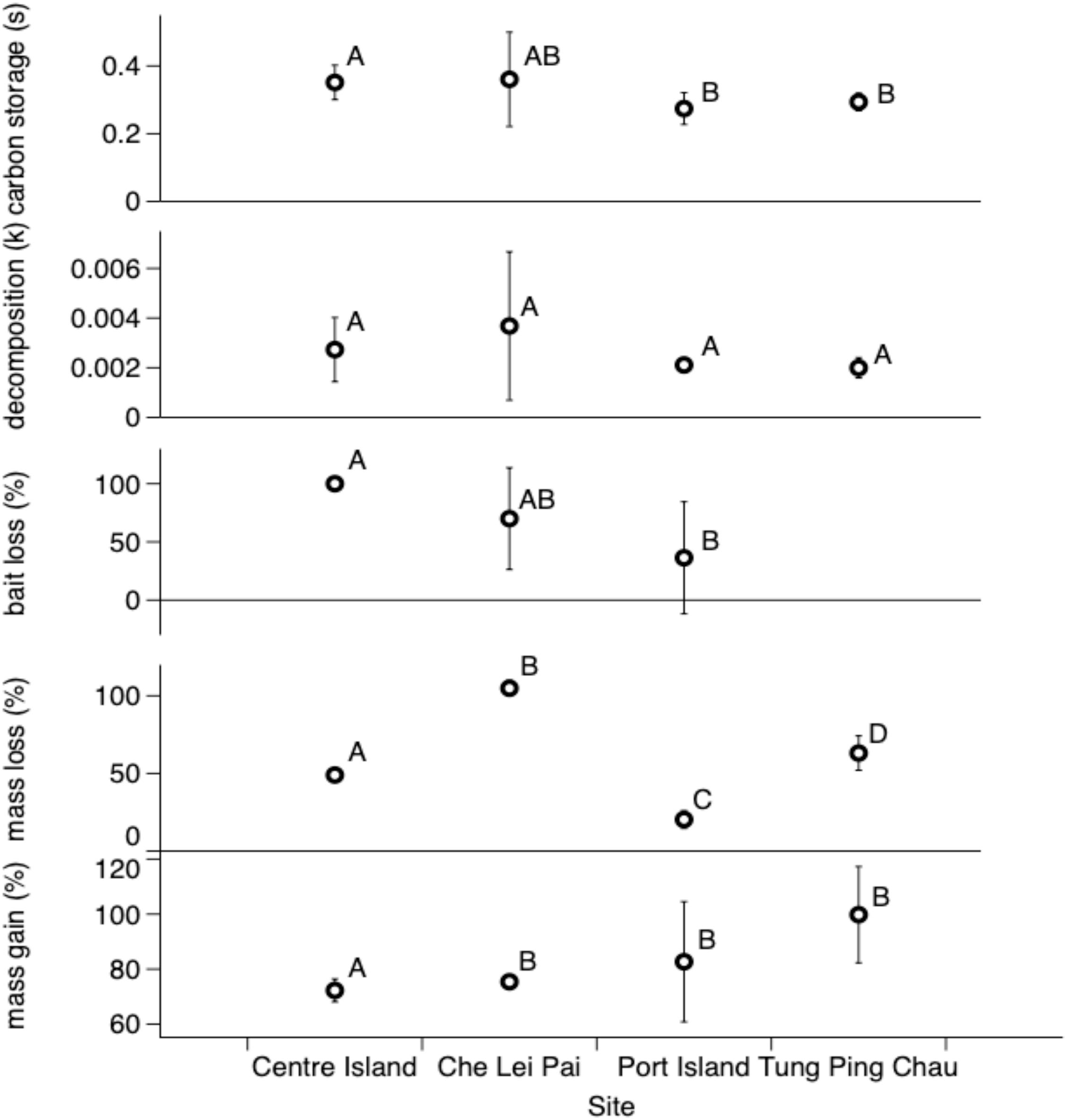
Figure 4. Ecosystem function data for primary productivity, herbivory, predation, organic matter decomposition and carbon sequestration across the four study sites (Center Island, Che Lei Pai, Port Island, Tung Ping Chau). Primary productivity was measured as the % gain in mass of macroalgae over a deployment period of 48 h; herbivory was measured as the % loss in mass of macroalgae over a deployment period of 48 h; predation was measured as the % loss in bait (dried squid) deployed over a period of 1 h – note: data missing from TPC; organic matter decomposition and carbon sequestration were calculated using the tea bag assay (refer to section “Materials and Methods”). Data is represented as the average value ± standard deviation. Connecting letters indicate significant differences between sites revealed by post hoc Tukey HSD tests (α = 0.05).
To identify the key environmental factors driving the variability, step-wise linear regression with model selection using the second-order Akaike Information Criterion (AICc) was performed (see Supplementary Material for detailed step-wise linear regression results with AICc scores: Supplementary Tables 6A–E). Chlorophyll-a and coral cover were significant drivers (p < 0.05) of primary productivity, on the other hand inorganic nitrogen and dissolved oxygen were closely significant (p = 0.05; best-fit model r2 = 0.44). Coral cover was also critical and the most significant (p < 0.05) for determining herbivory, however, secchi depth, an indicator of light (p = 0.05) could not be eliminated (best-fit model r2 = 0.96). Temperature and coral cover (p < 0.05) were important in predicting predation intensity (best-fit model r2 = 0.66). In determining factors that drove the variability of organic matter decomposition, no significant environmental parameters were observed. However, inorganic nitrogen significantly impacted the carbon sequestration potential in the sites (p < 0.05; best-fit model r2 = 0.44).
Analysis of Plate Imagery: Abundance of Sessile Fauna on ARMS Plates
ARMS plate photo analysis has been proven to be a powerful way to compare marine benthic communities (David et al., 2019). We observed 11 broad groups of organisms present on all ARMS deployed. This included several types of algae – chlorophytes, rhodophytes, and phaeophytes, tunicates, bivalves, and tube worms. To identify the key groups of benthic organisms driving the observed ecosystem function variability, step-wise linear regression with model selection using the second-order Akaike Information Criterion (AICc) was performed (see Supplementary Table 7). We observed no significant effect of the ARMS plates community composition on herbivory, primary productivity, carbon sequestration and organic matter decomposition. On the other hand, the relative abundance of green macroalgae (p = 0.00001), red upright macroalgae (p = 0.00251), soft tube worms (p = 1.46e–5) and tunicates (p = 0.00044) had a significant effect on the intensity of predation (best-fit model r2 = 0.95).
eDNA Data on Fish Abundance
Illumina MiSeq analysis yielded an average of 196540 million reads. High quality reads were obtained upon using a Phred score cutoff of 90% on the paired end pre-processed reads (Supplementary Appendix Tables A.4, A.5). Overall, ∼75% of all reads were annotated to local fish species. Samples from Center Island (the innermost site) did not amplify during the second PCR reaction. Therefore, these samples were excluded from library preparation.
eDNA sequences of a total of 91 taxa were obtained, in 44 families and 14 orders. Over 98% were registered under the Hong Kong Register of Marine Species, a comprehensive database for marine species in Hong Kong waters (Astudillo et al., 2018). Commercially valuable fish species were abundant, such as golden threadfin bream Nemipterus virgatus, flathead gray mullet Mugil cephalus, and yellow croaker Larimichthys crocea. Pollution-tolerant species including Mugil cephalus, and Siganus canaliculatus were also obtained from the samples. When compared with other conventional fish surveillance methods such as trawling (Leung, 1997), line fishing and netting (Hksar Agricultural, Fisheries and Conservation Department, 2016), as well as underwater visual census surveys (Sadovy and Cornish, 2000), eDNA captured ∼75% of taxa previously recorded in the same study sites. Moreover, there were 11 species that were only recorded using the eDNA method, but not observed in the conventional methods (Tsang, 2018).
Relative abundance of all fish varied significantly between sites (One-way ANOVA n = 11; F(3,8) = 103.5 p < 0.0001). Post hoc Tukey HSD revealed significant differences between the farthest sites Che Lei Pai and Tung Ping Chau and also all adjacent site pairs except Port Island and Tung Ping Chau (Figure 5). It is worth noting that OTU relative abundance is only correlated but does not reflect the relative abundance of the fish species under discussion. This is due to limitations with sequencing methods that yields platform specific data (reads), therefore making relative abundance analysis challenging (Harrison et al., 2020). Nevertheless, acknowledging these constraints imposed by compositional data is vital when analyzing sequencing data.
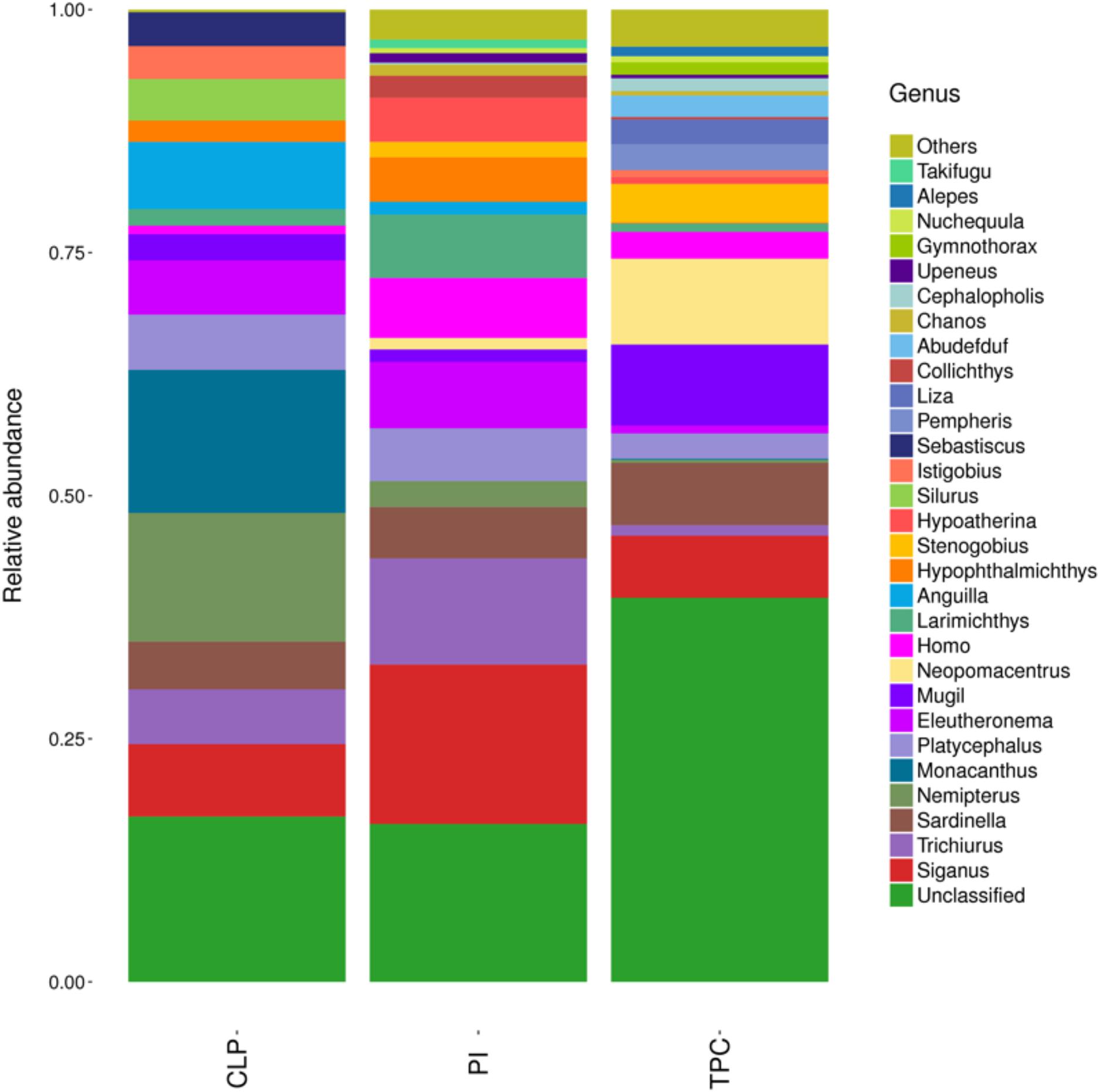
Figure 5. Relative abundance of top 30 genera of fish obtained from eDNA analysis of seawater samples collected from the same study sites. We recorded no fish in Center Island (CLP - Che Lei Pai; PI – Port Island; TPC – Tung Ping Chau).
We classified the observed fish species according to their dietary habits to herbivorous, omnivorous, planktivorous and predatory fish using FishBase (Figure 6). Specifically, relative abundance of herbivorous, omnivorous and planktivorous fish varied significantly between sites, however, the relative abundance of predatory fish showed no significant variability.
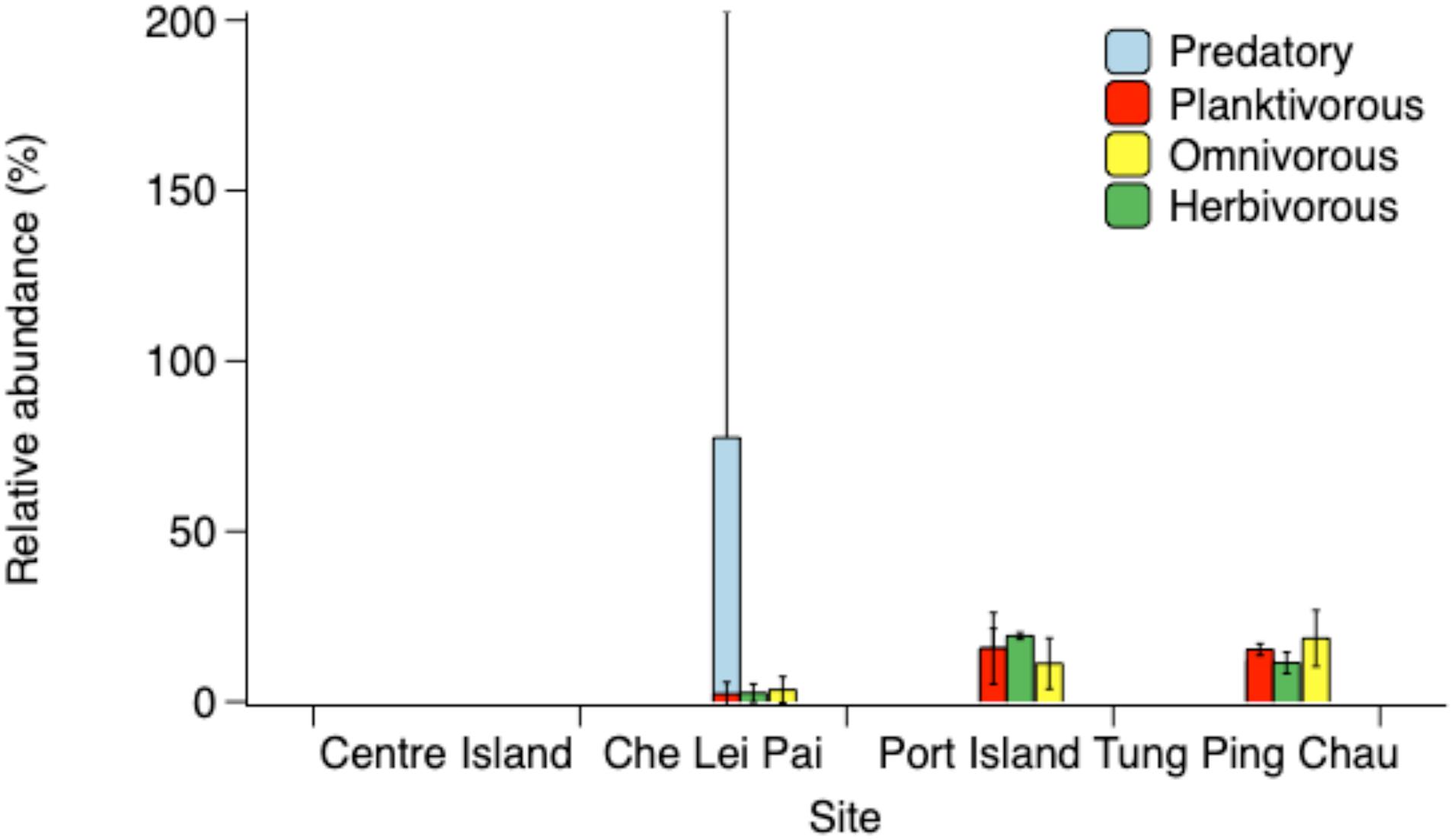
Figure 6. Results of relative abundance of predatory, herbivorous, planktivorous and omnivorous fish obtained from eDNA analysis of seawater samples collected from the same study sites. We recorded no fish in Center Island. Data is represented as the average value ± standard deviation.
We performed step-wise linear regression with model selection using the second-order Akaike Information Criterion (AICc), to characterize the relationship between fish abundance, fish diversity, dietary preference and ecosystem function (see Supplementary Tables 8A–E). Mass loss significantly depended on the relative abundance of herbivorous fish (p = 0.00061; best fit model r2 = 0.22). Mass gain significantly depended on the relative abundance of the following 12 genera of predatory fish (p = 0.00369; best fit model r2 = 0.46): Platycephalus indicus, Protonibea diacanthus, Trichiurus japonicus, Larimichthys polyactis, Polynemus dubius, Anguilla japonica, Nemipterus virgatus, Collichthys lucidus, Silurus meridionalis, Istigobius campbelli, Cephalopholis boenak, and Larimichthys crocea. The relative abundance of all planktivorous fish (p = 0.01198), omnivorous fish (p = 0.00179), and certain genera of predatory fish (A. japonica, I. campbelli, N. virgatus, C. lucidus, L. crocea, P. indicus, P. dubius, T. japonicus, S. meridionalis, C. boenak, L. polyactis, P. diacanthus; p = 0.00431) were significant drivers of bait loss variability (best fit model r2 = 0.52). The relative abundance of 12 genera of predatory fish – C. boenak, C. lucidus, I. campbelli, L. polyactis, N. virgatus, P. indicus, P. dubius, S. meridionalis, T. japonicus, A. japonica, L. crocea, and P. diacanthus significantly drove the variability of organic matter decomposition (p = 0.00126; best fit model r2 = 0.24). Finally, N. virgatus, P. indicus, A. japonica, L. polyactis, P. diacanthus, C. boenak, L. crocea, T. japonicus, P. dubius, S. meridionalis, C. lucidus, and I. campbelli significantly determined carbon sequestration variability (p = 0.00224; best fit model r2 = 0.18).
Overall Ecosystem Multifunctionality
In this study, overall multifunctionality was calculated using two approaches – average approach and threshold approach. Multifunctionality using the average approach was calculated without bait loss, as there was no data for Tung Ping Chau. Overall multifunctionality index was 0.70 for Center Island, 0.72 for Tung Ping Chau, 0.92 for Che Lei Pai and 0.51 for Port Island. The number of functions that could be maintained above a 50% threshold was 4, 5, 3, 4, respectively for Center Island, Che Lei Pai, Port Island and Tung Ping Chau (Table 1).
Discussion
In the last decade, research into the concept of multifunctionality (ability of ecosystems to provide multiple ecosystem functions) has increased owing to tremendous anthropogenic pressure on natural resources. This has resulted in the need to design and manage ecosystems so that they can efficiently provide multiple ecosystem functions simultaneously (Manning et al., 2018). However, not all ecosystem functions exhibit the same response to environmental drivers (Bradford et al., 2014). Given the complexity and diversity of ecosystem functions, the present study discusses five vital functions (primary productivity, herbivory, predation, organic matter decomposition and carbon sequestration) in an urbanized coastal marine environment. Data from each individual ecosystem function has been used to derive the overall multifunctionality for the human-impacted habitat. Linkages between multifunctionality, individual functions, environmental factors, fish abundance and benthic community composition datasets have been presented here.
Habitat as a Driver of Ecosystem Function
Coral reefs and coral communities are vital components of the Earth’s biosphere dominating several coastal habitats (Knowlton et al., 2010). In recent years, however, these habitats have been destroyed and homogenized owing to unsustainable coastal development, increased levels of pollution, overfishing and disease. This decline in coral cover is projected to continue even if the goals of the December 2015 Paris Agreement are implemented (Hoegh-Guldberg et al., 2019). Changes in habitat (coral reef complexity, coral cover, coral species richness, etc.) can have important implications for the functions that coral reefs provide such as productivity, sediment generation and so on (Perry et al., 2018). Our study sites in Hong Kong are along a gradient of coral cover (Center Island has almost no hard coral species to Tung Ping Chau which has ∼65 hard coral species) and serve as natural laboratories to discuss how changes to coral cover can affect multifunctionality in an ecosystem. Specifically, in testing for the effects of habitat on ecosystem function, we observed a positive relationship between coral cover and primary productivity. This is consistent with several literature showing that homogenized reef communities can jeopardize ecosystem functioning and productivity (Alvarez-Filip et al., 2015; Hughes et al., 2018; Richardson et al., 2018).
Functional Diversity as a Driver of Ecosystem Function
Functional diversity has been proven to be an indicator of ecosystem function (Petchey et al., 2004) and has been correlated to productivity (Cadotte et al., 2009). When coral communities shift, due to stressors, some visible changes often occur. One such example is the shift from a coral-dominated community to an algae-dominated one (Brooker et al., 2016). We observed this visually during scuba dives in Center Island and validated it through our analysis on the relative abundance of food sources as captured by the ARMS plates. Center Island recorded high relative abundance of algae, but no hard-coral species. On the other hand, Tung Ping Chau recorded relatively high abundance of both corals and algae (Figure 7).
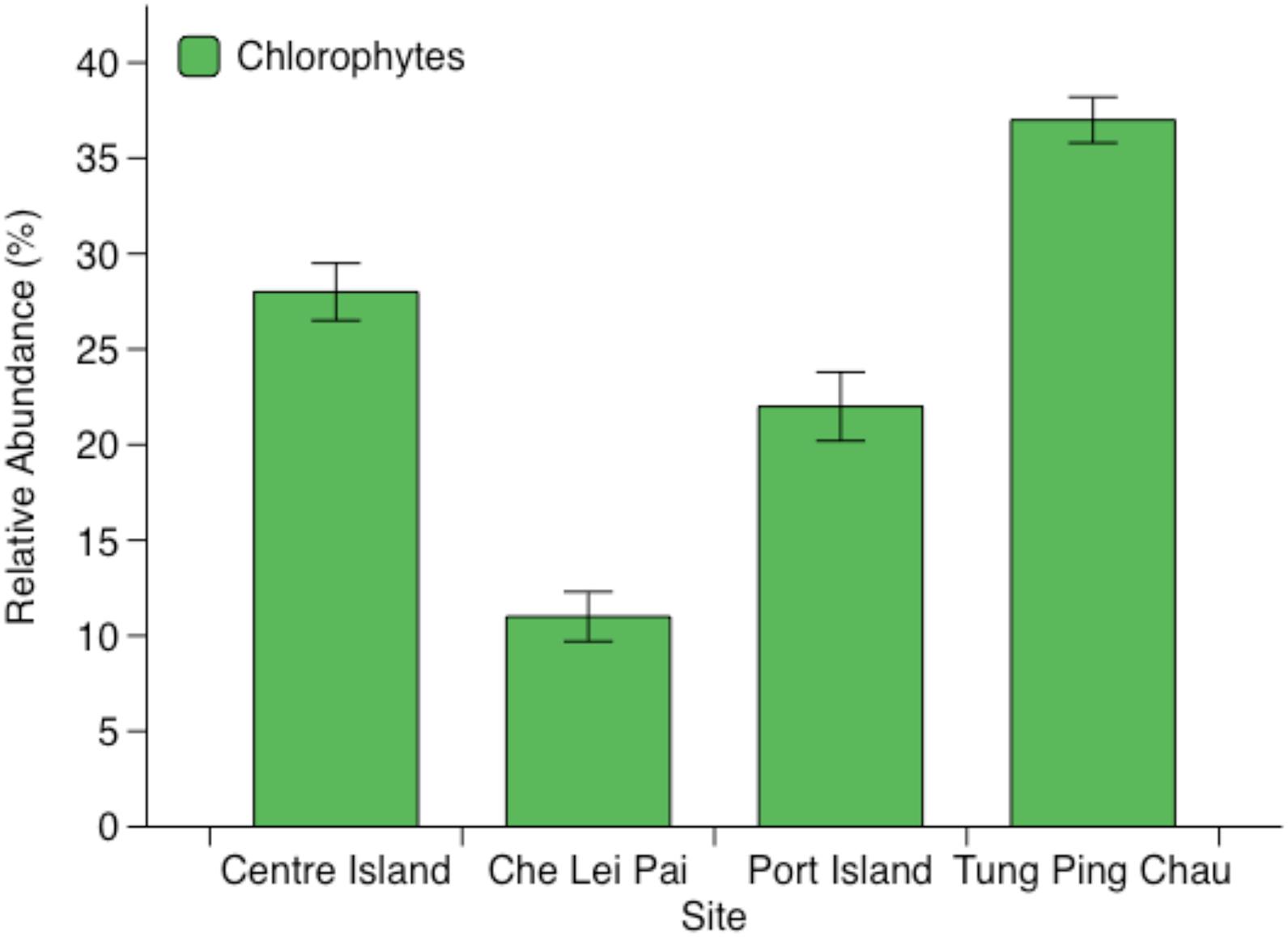
Figure 7. Results of annotations of plate photos revealing average relative abundance of chlorophytes from Autonomous Reef Monitoring Structures (ARMS) deployed in the same study sites.
When looking for functional diversity drivers of overall multifunctionality, the relative abundance of benthic filter-feeders emerged significant, outweighing effects from abiotic factors. This is consistent with literature citing bivalves as pivotal players in ecosystem functioning owing to their contribution to nutrient regeneration and productivity (Norkko et al., 2013).
Abiotic Factors as Drivers of Ecosystem Function
Nitrogen is the biological nutrient limiting factor in marine ecosystems, as it is generally less available for ocean plants and animals. In this study, there were significant inorganic nitrogen mediated effects on primary productivity and carbon sequestration in the study sites. Consistent with Zhang et al. (2015) and Mueller et al. (2018) increased inorganic nitrogen significantly reduced organic carbon preservation in coastal marine sediments. The results were also was consistent with other studies such as Bristow et al. (2017) which observed that nitrogen exerts a critical control on primary productivity. On the other hand, we observed the opposite relationship between total Kjeldahl nitrogen (TKN: a measure of organic nitrogen) and carbon sequestration, where carbon storage decreased with decreasing TKN concentration. TKN is directly related to the source of bottom deposition. Wastewater discharges often contain relatively high concentrations of organic nitrogen and Hong Kong discharges over 3 million cubic meters of treated wastewater into its surrounding marine environment every day. Archana et al. (2016) recorded that wastewater was indeed the dominant source of the nitrogen pool using stable isotopes. Therefore, characterizing the effect of bottom sediment TKN is valuable in this highly urbanized coastal system.
We observed no nutrient-driven effects on primary productivity, herbivory, predation and organic matter decomposition. This is consistent with Alsterberg et al. (2014), which summarized findings from some studies that demonstrated no effects of nutrients on ecosystem multifunctionality in a marine habitat. We hypothesize that nutrient-driven effects were masked by effects from other significant abiotic factors such as secchi depth (an indicator of light), chlorophyll-a, dissolved oxygen and temperature.
The Coral-Algae-Herbivore Triangle
The complexity of the coral-algae-herbivore relationship is well known (Holbrook et al., 2016). A popular hypothesis (that is also somewhat controversial) is that herbivore fishes help corals thrive in a reef benthos by managing the distribution and abundance of algae (Hixon, 2015). However, overfishing of herbivorous fishes can degrade this association (Heenan et al., 2016). The data recorded from the herbivory assay showed significant variability between the four sites. We observed relatively more algal consumption in Tung Ping Chau and Che Lei Pai when compared to what was observed in Center Island and Port Island. We hypothesize that this could be due to several reasons – firstly, we propose that the lack of consumption in Center Island and Port Island could be due to the availability and preference for other food sources, such as Sargassum, which was also present at some sites. This was confirmed by the ARMS plate photo analysis, which revealed several types of algae (Figure 7) in varying abundances at all the four study sites. Secondly, we observed herbivorous rabbitfish and pufferfish at the sites that recorded more herbivory. This was corroborated by fish abundance from eDNA analysis (Figure 6), which suggested the presence of herbivorous and omnivorous fish in increasing abundances from Che Lei Pai to Tung Ping Chau. However, it was unusual that we observed the presence of herbivorous and omnivorous fish in the eDNA analysis at Port Island, yet did not observe any algal mass loss in the herbivory assay. As the coral-algal-herbivore interaction does not occur in isolation of the rest of the ecosystem, we suspect the variability in our dataset to be due to a combination of abiotic factors, other organisms and excessive fishing.
Relative Feeding Intensity of Generalist Predators
There continues to be some controversy on whether algal distribution and growth in the marine benthos is controlled by top-down herbivory/predation or bottom-up nutrient cycling (Lapointe et al., 2004; Poore et al., 2012). While bottom-up control by resources is relatively well understood, it is necessary to characterize the geography of top-down control by predators and herbivores through space and time (Meyer et al., 2016). One way to do this is to measure prey vulnerability – however, across geographies and larger scales, it is desirable to have a standardized food type and a simpler tool to measure the relative feeding intensity of generalist predators. In line with this, we utilized the squid pop technique as per Duffy et al. (2015). Feeding intensity (bait loss from squid pops) was higher in the sites with higher hard coral species richness. A recent study that employed the squid pop technique found increased feeding intensity to be directly correlated with fish abundance. This pattern could therefore reflect a higher level of predation in Port Island, a site where visual observations have also recorded more fish compared to inshore sites. Consistently, fish abundance from the same sites revealed a similar pattern, thereby corroborating our findings. However, we did not obtain predation intensity for Tung Ping Chau. Therefore, we did not obtain a holistic picture for the difference across habitats in relative predation intensity. We believe these limitations are compensated by the assay’s ease of applicability and standardization, making it possible to replicate the experiment and obtain data fairly quickly.
Intermediate Disturbance Affects Overall Ecosystem Multifunctionality
To determine the overall ecosystem multifunctionality, we followed two standard approaches – the average approach and the threshold approach. Both these approaches include all available measures of ecosystem functions in a given habitat. Consistently across both approaches, Che Lei Pai recorded a relatively higher multifunctionality index and multifunctionality threshold while Port Island recorded a relatively lower index and threshold compared to the other sites. The site with the least disturbance (Tung Ping Chau) did not record the highest multifunctionality index or threshold, neither did the site with the most disturbance (Center Island) record the lowest multifunctionality index or threshold. The site with intermediate disturbance (Che Lei Pai) recorded the highest multifunctionality index and threshold. This was contrary to our hypothesis (multifunctionality increases from inshore – more human impacts to open ocean - less human impacts). The intermediate disturbance hypothesis was presented originally to describe species-mediated effects on corals and trees (Connell, 1978). It predicts that sites with maximum disturbance and least disturbance will have low diversity and that intermediate disturbance will maximize diversity. In line with this, we propose that intermediate disturbance maximizes diversity and correspondingly multifunctionality in Che Lei Pai when compared to the other sites. However, it must be noted that both approaches to characterize ecosystem multifunctionality are not without limitations and multivariate methods for quantification is recommended.
In testing for drivers of multifunctionality, we observed abiotic-mediated (turbidity) and functional diversity-mediated (benthic filter feeder abundance) effects. However, maintaining high levels of multifunctionality in an ecosystem is not likely to be driven solely by these two factors. The maximization of functions favored by the habitat is likely to be largely responsible as per Hensel and Silliman (2013). The findings from our study revealed that when human impact decreases, the consequences to overall ecosystem functioning depend not only on which single functions are taken into consideration. This implies that ecosystem multifunctionality is also driven by the presence or absence of other metazoans and microbial organisms present that drive trophic level interactions and functions such as herbivory and predation. This is consistent with Gamfeldt et al. (2008) who proposed that even in eutrophication impacted coastal marine environments, overall multifunctionality is more susceptible to species loss than are individual ecosystem functions. So, taking these factors into consideration and shifting focus to a variety of functions provided by a diversity of species is likely to provide a holistic picture of ecosystem multifunctionality and will subsequently help in conservation management.
Conclusion and Implications for Conservation Management
The results from this study have important implications for understanding processes regulating the structure of human-impacted coastal marine habitats. By characterizing important ecosystem functions (primary productivity, herbivory, predation, decomposition and carbon sequestration) and overall multifunctionality, the findings significantly expand on what we already know about urbanization impacts on key ecosystem processes. The highlight of this study is the finding that urbanization impacts multifunctionality in coastal marine systems. However, these results warrant a more detailed investigation on the links between multifunctionality and community composition (microbial and metazoan). Further research into the role of decomposition in overall ecosystem multifunctionality and associated carbon storage mechanisms can be used to help in global carbon inventory management. Moreover, research into functional level responses, microbial interactions and additional ecosystem services (Manning et al., 2018) such as shoreline stabilization, biogeochemical cycling and carbon sequestration will help shed light on the holistic properties of the ecosystem.
Data Availability Statement
All data generated for this study has been made publicly available in the Appendix and Supplementary Material of this article.
Author Contributions
AA and DB conceptualized the research. AA conducted the fieldwork and did the analysis. DB reviewed the manuscript and analysis. Both authors contributed to the article and approved the submitted version.
Funding
We are grateful to the International Coral Reef Society for the graduate student fellowship awarded to AA that helped fund this work. We are grateful to the Environment Conservation Fund (ECF, Grant 67-2016) and Collaborative Research Fund (CRF, C7013-19GF) that helped fund the work on the autonomous reef monitoring structures (ARMS) and eDNA. This paper is contribution 67 to the Smithsonian’s MarineGEO-TMON program.
Conflict of Interest
The authors declare that the research was conducted in the absence of any commercial or financial relationships that could be construed as a potential conflict of interest.
Acknowledgments
We would like to express our sincere gratitude to Tsang, Hin Hung Rainbow for sharing her eDNA data on fish diversity in the Tolo channel from her Final Year Project report. We would like to express our heartfelt thanks to Rinaldi Gotama for sharing the data on taxa annotations from the ARMS plate photos. This data has been used to analyze and interpret the data on ecosystem multifunctionality. We are especially grateful to Taihun Kim for assisting with the tea bag deployments. We want to thank Jane, Inga, Phil, Taihun, Chloe, and department colleagues Laura, Briony, and Rhyn for participating and graciously offering their help during the lab dive-cruise where we collected all the data on ecosystem functioning. Lastly, we are very grateful to the International Coral Reef Society’s graduate fellowship awarded to AA, that helped fund the fieldwork.
Supplementary Material
The Supplementary Material for this article can be found online at: https://www.frontiersin.org/articles/10.3389/fmars.2020.557145/full#supplementary-material
References
Al-Maliki, S., and Al-Masoudi, M. (2018). Interactions between Mycorrhizal Fungi, tea wastes, and algal biomass affecting the microbial community, soil structure, and alleviating of salinity stress in corn yield (Zea mays L.). Plants 6:63. doi: 10.3390/plants7030063
Alsterberg, C., Roger, F., Sundbäck, K., Juhanson, J., Hulth, S., Hallin, S., et al. (2017). Habitat diversity and ecosystem multifunctionality—the importance of direct and indirect effects. Sci. Adv. 3:e1601475. doi: 10.1126/sciadv.1601475
Alsterberg, C., Sundback, K., and Gamfeldt, L. (2014). Multiple stressors and multifunctionality: limited effects on an illuminated benthic system. Biol. Lett. 10:20140640. doi: 10.1098/rsbl.2014.0640
Alvarez-Filip, L., Paddack, M. J., Collen, B., Robertson, D. R., and Côté, I. M. (2015). Simplification of caribbean reef-fish assemblages over decades of coral reef degradation. PLoS One 10:e0126004. doi: 10.1371/journal.pone.0126004
Archana, A., Luo, L., Kao, S. J., Thibodeau, B., and Baker, D. M. (2016). Variation in nitrate isotopic composition of wastewater effluents by treatment type in Hong Kong. Mar. Pollut. Bull. 111, 143–152. doi: 10.1016/j.marpolbul.2016.07.019
Archana, A., Thibodeau, B., Geeraert, N., Xu, M. N., Kao, S. J., and Baker, D. M. (2018). Nitrogen sources and cycling revealed by dual isotopes of nitrate in a complex urbanized environment. Wat. Res. 142, 459–470. doi: 10.1016/j.watres.2018.06.004
Astudillo, J. C., Williams, G. A., Leung, K. M. Y., Cannicci, S., Yasuhara, M., Yau, C., et al. (2018). Hong Kong Register of Marine Species. Available online at: http://www.marinespecies.org/hkrms/ (accessed November 23, 2020).
Beijbom, O., Edmunds, P. J., Roelfsema, C., Smith, J., Kline, D. I., Neal, B. P., et al. (2015). Towards automated annotation of benthic survey images: variability of human experts and operational modes of automation. PLoS One 10:e0130312. doi: 10.1371/journal.pone.0130312
Berdugo, M., Keìfi, S., Soliveres, S., and Maestre, F. T. (2017). Plant spatial patterns identify alternative ecosystem multifunctionality states in global drylands. Nat. Ecol. Evolut. 1:3.
Bradford, M. A., Wood, S. A., Bardgett, R. D., Black, H. I. J., Bonkowski, M., Eggers, T., et al. (2014). Discontinuity in the response of ecosystem processes and multifunctionality to altered soil community composition. Proc. Natl. Acad. Sci. U.S.A. 111, 14478–14483. doi: 10.1073/pnas.1413707111
Brandl, S. J., Rasher, D. B., Côté, I. M., Casey, J. M., Darling, E. S., Lefcheck, J. S., et al. (2019). Coral reef ecosystem functioning: eight core processes and the role of biodiversity. Front. Ecol. Environ. 17, 445–454. doi: 10.1002/fee.2088
Bristow, L. A., Mohr, W., Ahmerkamp, S., and Kuypers, M. M. M. (2017). Nutrients that limit growth in the ocean. Curr. Biol. 27, R431–R510.
Brooker, R. M., Hay, M. E., and Dixson, D. L. (2016). Chemically cued suppresion of coral reef resilience: where is the tipping point? Coral Reefs 35, 1263–1270. doi: 10.1007/s00338-016-1474-4
Burkepile, D. E., and Hay, M. E. (2008). Herbivore vs. nutrient control of marine primary producers: context-dependent effects. Ecology 87, 3128–3139. doi: 10.1890/0012-9658(2006)87[3128:hvncom]2.0.co;2
Byrnes, J. E. K., Gamfeldt, L., Isbell, F., Lefcheck, J. S., Griffin, J. N., Hector, A., et al. (2014). Investigating the relationship between biodiversity and ecosystem multifunctionality: challenges and solutions. Methods Ecol. Evolut. 5, 111–124. doi: 10.1111/2041-210x.12143
Cadotte, M. W., Cavender-Bares, J., Tilman, D., and Oakley, T. H. (2009). Using phylogenetic, functional and trait diversity to understand patterns of plant community productivity. PLoS One 4:e5695. doi: 10.1371/journal.pone.005695
Cardinale, B. J., Matulich, K. L., Hooper, D. U., Byrnes, J. E., Duffy, E., Gamfeldt, L., et al. (2011). The functional role of producer diversity in ecosystems. Am. J. Bot. 98, 572–592. doi: 10.3732/ajb.1000364
Connell, J. H. (1978). Diversity in tropical rain forests and coral reefs. Science 199, 1302–1310. doi: 10.1126/science.199.4335.1302
David, R., Uyarra, M. C., Carvalho, S., Anlauf, H., Borja, A., Cahill, A. E., et al. (2019). Lessons from photo analyses of autonomous reef monitoring structures as tools to detect (bio-)geographical, spatial and environmental effects. Mar. Pollut. Bull. 141, 420–429. doi: 10.1016/j.marpolbul.2019.02.066
Duddigan, S., Shaw, L. J., Alexander, P. D., and Collins, C. D. (2020). Chemical underpinning of the Tea Bag Index: an examination of the decomposition of tea leaves. Appl. Environ. Soil Sci. 20:6085180.
Duffy, E. J., Godwin, C. M., and Cardinale, B. J. (2017). Biodiversity effects in the wild are common and as strong as key drivers of productivity. Nature 549, 261–264. doi: 10.1038/nature23886
Duffy, E. J., Ziegler, S. L., Campbell, J. E., Bippus, P. M., and Lefcheck, J. S. (2015). Squidpops: a simple tool to crowdsource a global map of marine predation intensity. PLoS One 10:e0142994. doi: 10.1371/journal.pone.0142994
Duffy, J. E. (2009). Why biodiversity is important to the functioning of real-world ecosystems. Front. Ecol. Environ. 7, 437–444. doi: 10.1890/070195
Duffy, J. E., Cardinale, B. J., France, K. E., Mclntyre, P. B., Thebault, E., and Loreau, M. (2007). The functional role of biodiversity in ecosystems: incorporating trophic complexity. Ecol. Lett. 10, 522–538. doi: 10.1111/j.1461-0248.2007.01037.x
Duffy, J. E., Lefcheck, J. S., Stuart-Smith, R. D., Navarrete, S. A., and Edgar, G. J. (2016). Biodiversity enhances reef fish biomass and resistance to climate change. Proc. Natl. Acad. Sci. U.S.A. 113, 6230–6235. doi: 10.1073/pnas.1524465113
Duprey, N. N., McIlroy, S. E., Ng, T. P. T., Thompson, P. D., Kim, T., Wong, J. C. Y., et al. (2017). Facing a wicked problem with optimism: issues and priorities for coral conservation in Hong Kong. Biodivers. Conserv. 26, 2521–2545. doi: 10.1007/s10531-017-1383-z
Duprey, N. N., Yasuhara, M., and Baker, D. M. (2016). Reefs of tomorrow: eutrophication reduces coral biodiversity in an urbanized seascape. Glob. Chang. Biol. 22, 3550–3565. doi: 10.1111/gcb.13432
Fabricius, K. E., and McCorry, D. (2006). Changes in octocoral communities and benthic cover along a water quality gradient in the reefs of Hong Kong. Mar. Pollut. Bull. 52, 22–33. doi: 10.1016/j.marpolbul.2005.08.004
Fahey, T., and Knapp, A. (2007). Principles and Standards for Measuring Primary Production. New York, NY: Oxford University Press.
Gamfeldt, L., Hillebrand, H., and Jonsson, P. R. (2008). Multiple functions increase the importance of biodiversity for overall ecosystem functioning. Ecol. Soc. Am. 89, 1223–1231. doi: 10.1890/06-2091.1
Gamfeldt, L., Lefcheck, J. S., Byrnes, J. E. K., Cardinale, B. J., Duffy, J. E., and Griffin, J. N. (2015). Marine biodiversity and ecosystem functioning: what’s known and what’s next? Oikos 124, 252–265. doi: 10.1111/oik.01549
Gamfeldt, L., and Roger, F. (2017). Revisiting the biodiversity-ecosystem multifunctionality relationship. Nat. Ecol. Evol. 1:0168. doi: 10.1038/s41559-017-0168
García-Comas, C., Sastri, A., Ye, L., Channg, C. Y., Lin, F. S., Su, M. S., et al. (2016). Prey size diversity hinders biomass trophic transfer and predator size diversity promotes it in Planktonic communities. Proc. R. Soc. B Biol. Sci. 283:20152129. doi: 10.1098/rspb.2015.2129
Gonzalez, A., Germain, R. M., Srivastava, D. S., Filotas, E., Dee, L. E., Gravel, D., et al. (2020). Scaling-up biodiversity-ecosytem functioning research. Ecol. Lett. 23, 757–776. doi: 10.1111/ele.13456
Harrison, J. G., Calder, W. J., Shuman, B., and Buerkle, C. A. (2020). The quest for absolute abundance: the use of internal standards for DNA-based community ecology. Mol. Ecol. Resourc. 1–14. doi: 10.1111/1755-0998.13247
Hautier, Y., Isbell, F., Borer, E. T., Seablom, E. W., Harpole, W. S., Lind, E. M., et al. (2017). Local loss and spatial homogenization of plant diversity reduce ecosystem multifunctionality. Nat. Ecol. Evol. 2, 50–56.
Hector, A., and Bagchi, R. (2007). Biodiversity and ecosystem multifunctionality. Nature 448, 188–190. doi: 10.1038/nature05947
Heenan, A., Hoey, A. S., Williams, G. J., and Williams, I. D. (2016). Natural bounds on herbivorous coral reef fishes. Proc. R. Soc. B Biol. Sci. 283:20161716. doi: 10.1098/rspb.2016.1716
Hensel, M. J. S., and Silliman, B. R. (2013). Consumer diversity across kingdoms supports multiple functions in a coastal ecosystem. Proc. Natl. Acad. Sci. U.S.A. 110, 20621–20626. doi: 10.1073/pnas.1312317110
Hixon, M. A. (2015). “Reef fishes, seaweeds, and corals: a complex triange,” in Coral Reefs in the Anthropocene, ed. C. Birkeland (Dordrecht: Springer), 195–215. doi: 10.1007/978-94-017-7249-5_10
Hksar Agricultural, Fisheries and Conservation Department (2016). Major Species of Fish Catch (Adult Fish) Port Survey. Available online at: https://www.afcd.gov.hk/english/fisheries/fish_cap/fish_cap_latest/files/common/PS201617_ENG.pdf (accessed October 03, 2020).
Hoegh-Guldberg, O., Jacob, D., Taylor, M., Bolanos, T. G., Bindi, M., Brown, S., et al. (2019). The human imperative of stabilizing global climate change at 1.5°C. Science 365:eaaw6974.
Holbrook, S. J., Schmitt, R. J., Adam, T. C., and Brooks, A. J. (2016). Coral reef resilience, tipping points and the strength of herbivory. Sci. Rep. 6:35817.
Hoölting, L., Jacobs, S., Felipe-Lucia, M., Maes, J., Norstroöm, A., Plieninger, T., et al. (2019). Measuring ecosystem multifunctionality across scales. Environ. Res. Lett. 14:123083.
Hughes, T. P., Kerry, J. T., Baird, A. H., Connolly, S. R., Dietzel, A., Eakin, C. M., et al. (2018). Global warming transforms coral reef assemblages. Nature 556, 492–496. doi: 10.1038/s41586-018-0041-2
Hurvich, C. M., and Tsai, C.-L. (1989). Regression and time series model selection in small samples. Biometrika 76, 297–307. doi: 10.1093/biomet/76.2.297
Keuskamp, J. A., Dingemans, B. J. J., Lehtinen, T., Sarneel, J. M., and Hefting, M. M. (2013). Tea bag index: a novel approach to collect uniform decomposition data across ecosystems. Methods Ecol. Evol. 4, 1070–1075. doi: 10.1111/2041-210x.12097
Knowlton, N., Brainard, R. E., Fisher, R., Moews, M., Plaisance, L., and Caley, M. J. (2010). “Coral reef biodiversity,” in Life in the World’s Oceans: Diversity, Distribution, and Abundance, ed. A. D. Macintyre (Oxford: Wiley-Blackwell), 65–77.
Lapointe, B. E., Barile, P. J., Yentsch, C. S., Littler, M. M., Littler, D. S., and Kakuk, B. (2004). The relative importance of nutrient enrichment and herbivory on macroalgal communities near Norman’s Pond Cay, Exumas Cays, Bahamas: a “natural” enrichment experiment. J. Exper. Mar. Biol. Ecol. 298, 275–301. doi: 10.1016/s0022-0981(03)00363-0
Lefcheck, J. S., Byrnes, J. E. K., Isbell, F., Gamfeldt, L., Griffin, J. N., Eisenhauer, N., et al. (2015). Biodiversity enhances ecosystem multifunctionality across trophic levels and habitats. Nat. Commun. 6:6936.
Lefcheck, J. S., Innes-Gold, A. A., Brandl, S. J., Steneck, R. S., Torres, R. E., and Rasher, D. B. (2019). Tropical fish diversity enhances coral reef functioning across multiple scales. Sci. Adv. 5:eaav6420. doi: 10.1126/sciadv.aav6420
Leray, M., and Knowlton, N. (2014). DNA barcoding and metabarcoding of standardized samples reveal patterns of marine benthic diversity. Proc. Natl. Acad. Sci. U.S.A. 112, 2076–2081. doi: 10.1073/pnas.1424997112
Leung, A. W. Y. (1997). “The epibenthic ichthyofauna of Tolo Harbour and Hong Kong s northeastern waters a long term record of change,” in Proceedings of the Eighth International Marine Biological Workshop: The Marine Flora and Fauna of Hong Kong and Southern China, ed. B. Morton (Hong Kong: Hong Kong University Press), 463–497.
Maestre, F. T., Quero, J. L., Gotelli, N. J., Escudero, A., Ochoa, V., and Delgado-Baquerizo, M. (2012). Plant species richness and ecosystem multifunctionality in global drylands. Science 335, 214–218.
Manning, P., van der Plas, F., Soliveres, S., Allan, E., Maestre, F. T., Mace, G., et al. (2018). Redefining ecosystem multifunctionality. Nat. Ecol. Evol. 2, 427–436. doi: 10.1038/s41559-017-0461-7
Meyer, S. T., Ebeling, A., Eisenhauer, N., Hertzog, L., Hillebrand, H., Milcu, A., et al. (2016). Effects of biodiversity strengthen over time as ecosystem functioning decines at low and increases at high biodiversity. Ecosphere 7:e01619.
Meyer, S. T., Ptacnik, R., Hillebrand, H., Bessler, H., Buchmann, N., Ebeling, A., et al. (2018). Biodiversity-multifunctionality relationships depend on identity and number of measured functions. Nat. Ecol. Evol. 2, 44–49. doi: 10.1038/s41559-017-0391-4
Miya, M., Sato, Y., Fukunaga, T., Sado, T., Poulsen, J. Y., Sato, K., et al. (2015). MiFish, a set of universal PCR primers for metabarcoding environmental DNA from fishes: detection of more than 230 subtropical marine species. R. Soc. Open Sci. 2:150088. doi: 10.1098/rsos.150088
Mora, C., Tittensor, D. P., Adl, S., Simpson, A. G. B., and Worm, B. (2011). How many species are there on Earth and in the ocean? PLoS Biol. 9:e1001127. doi: 10.1371/journal.pone.1001127
Morton, B. (1989). Editorial: Hong Kong’s first marine disaster. Mar. Pollut. Bull. 19, 299–300. doi: 10.1016/0025-326x(88)90406-7
Mueller, P., Schile-Beers, L. M., Mozder, T. J., Chmura, G. L., Dinter, T., Kuzyakov, Y., et al. (2018). Global-change effects on early-stage decomposition processes in tidal wetlands: implications from a global survey using standardized litter. Biogeosciences 15, 3189–3202. doi: 10.5194/bg-15-3189-2018
Nelson, E., Mendoza, G., Regetz, J., Polasky, S., Tallis, H., Cameron, D. R., et al. (2009). Modeling multiple ecosystem services, biodiversity conservation, commodity production, and tradeoffs at landscape scales. Front. Ecol. Environ. 7, 4–11. doi: 10.1890/080023
Norkko, A., Billnas, A., Norkko, J., Valanko, S., and Pilditch, C. (2013). Size matters: implications of the loss of large individuals for ecosystem function. Sci. Rep. 3:2646.
Perry, C. T., Alvarez-Filip, L., Graham, N. A. J., Mumby, P. J., Wilson, S. K., Kench, P. S., et al. (2018). Loss of coral reef growth capacity to track future increases in sea level. Nature 558, 396–400. doi: 10.1038/s41586-018-0194-z
Petchey, O. L., Hector, A., and Gaston, K. J. (2004). How do different measures of functional diversity perform? Ecology 85, 847–857. doi: 10.1890/03-0226
Poore, A. G. B., Campbell, A. H., Coleman, R. A., Edgar, G. J., Jormalainen, V., Reynolds, P. L., et al. (2012). Global patterns in the impacts of marine herbivores on benthic primary producers. Ecol. Lett. 15, 912–922. doi: 10.1111/j.1461-0248.2012.01804.x
Ptacnik, R., Solimini, A. G., Andersen, T., Tamminen, T., Brettum, P., Lepisto, L., et al. (2008). Diversity predicts stability and resource use efficiency in natural phytoplankton communities. Proc. Natl. Acad. Sci. U.S.A. 105, 5134–5138. doi: 10.1073/pnas.0708328105
Rasher, D. B., Hoey, A. S., and Hay, M. E. (2013). Consumer diversity interacts with prey defences to drive ecosystem function. Ecology 94, 1347–1358. doi: 10.1890/12-0389.1
Richardson, L. E., Graham, N. A. J., Pratchett, M. S., Eurich, J. C., and Hoey, A. S. (2018). Mass coral bleaching causes biotic homogenization of reef fish assemblages. Glob. Chang. Biol. 24, 3117–3129. doi: 10.1111/gcb.14119
Sadovy, Y., and Cornish, A. (2000). Reef Fishes of Hong Kong. Hong Kong: Hong Kong University Press.
Scott, P. J. B. (1990). Chronic pollution recorded in coral skeletons in Hong Kong. J. Exper. Mar. Biol. Ecol. 139, 51–64. doi: 10.1016/0022-0981(90)90038-e
Seelen, L. M. S., Flaim, G., Keuskamp, J., Teurlincx, S., Font, R. A., Tolunay, D., et al. (2019). An affordable and reliable assessment of decomposition: tailoring the tea bag index to surface waters. Wat. Res. 151, 31–43. doi: 10.1016/j.watres.2018.11.081
Soliveres, S., Manning, P., Prati, D., Gossner, M. M., Alt, F., Arndt, H., et al. (2016). Locally rare species influence grassland ecosystem multifunctionality. Philos. Trans. R. Soc. B Biol. Sci. 371:20150269.
Stachowicz, J. J., Bruno, J. F., and Duffy, J. E. (2007). Understanding the effects of marine biodiversity on communities and ecosystems. Ann. Rev. Ecol. Evol. Syst. 38, 739–766. doi: 10.1146/annurev.ecolsys.38.091206.095659
Thompson, P. L., Isbell, F., Loreau, M., O’Connor, M. I., and Gonzalez, A. (2018). The strength of the biodiversity-ecosystem function relationship depends on spatial scale. Proc. R. Soc. B 285:20180038. doi: 10.1098/rspb.2018.0038
Tsang, H. H. (2018). Using Environmental DNA to Reveal the Local Fish Communities at Tolo Harbor and Channel, Hong Kong. Final Year Project Thesis, University of Hong Kong, Pok Fu Lam.
Villnas, A., Norkko, J., Hietanen, S., Josefson, A. B., Lukkari, K., and Norkko, A. (2013). The role of recurrent disturbances for ecosystem multifunctionality. Ecology 94, 2275–2287. doi: 10.1890/12-1716.1
Wong, C. W. M., Duprey, N. N., and Baker, D. M. (2017). New insights on the nitrogen footprint of a coastal megalopolis from coral-hosted Symbiodinium δ15N. Environ. Sci. Technol. 51, 1981–1987. doi: 10.1021/acs.est.6b03407
Zhang, J. T., and Wang, C. (2012). Biodiversity and ecosystem functioning: exploring large-scale patterns in mainland China. iForest Biogeosci. For. 5, 230–234. doi: 10.3832/ifor0627-005
Zhang, Y., Wang, L., Hu, Y., Xi, X., Tang, Y., Chen, J., et al. (2015). Water organic pollution and eutrophication influence soil microbial processes, increasing soil respiration of estuarine wetlands: site study in Jiuduansha wetland. PLoS One 10:e0126951. doi: 10.1371/journal.pone.0126951
Keywords: urban, marine, ecosystem, multifunctionality, coral, eutrophication, coastal
Citation: Archana A and Baker DM (2020) Multifunctionality of an Urbanized Coastal Marine Ecosystem. Front. Mar. Sci. 7:557145. doi: 10.3389/fmars.2020.557145
Received: 29 April 2020; Accepted: 11 November 2020;
Published: 03 December 2020.
Edited by:
Marius Nils Müller, Federal University of Pernambuco, BrazilReviewed by:
Rodney Forster, University of Hull, United KingdomAndrew Martin Lohrer, National Institute of Water and Atmospheric Research (NIWA), New Zealand
Copyright © 2020 Archana and Baker. This is an open-access article distributed under the terms of the Creative Commons Attribution License (CC BY). The use, distribution or reproduction in other forums is permitted, provided the original author(s) and the copyright owner(s) are credited and that the original publication in this journal is cited, in accordance with accepted academic practice. No use, distribution or reproduction is permitted which does not comply with these terms.
*Correspondence: Archana Anand, YXJjaGFuYWFAc3RhbmZvcmQuZWR1