- 1Michigan Tech Research Institute, Michigan Tech University, Ann Arbor, MI, United States
- 2NASA Glenn Research Center, Cleveland, OH, United States
The states of Michigan and Ohio issued shutdown orders in mid-March 2020 in an attempt to slow the spread of the coronavirus (COVID-19), resulting in widespread disruption to economic and human activity. This study, which was commissioned by NASA headquarters, utilized satellite remote sensing data from the Visible Infrared Imaging Radiometer Suite sensor onboard the Suomi National Polar-orbiting Partnership satellite to investigate whether these changes in activity led to any short-term changes in water quality in the Great Lakes region by comparing 2020 data to a historic baseline. The water quality parameters examined included chlorophyll-a (CHL) and total suspended solids (TSS) concentrations, water clarity, and harmful algal bloom (HAB) extent. These parameters were investigated in two Great Lakes basins which experience significant anthropogenic pressure: the western basin of Lake Erie (WBLE) and Saginaw Bay in Lake Huron (SBLH). TSS concentrations in April 2020 were below the historic baseline in both basins, and largely remained low until September. SBLH also experienced elevated CHL concentrations in April which persisted through the summer. Additionally, the WBLE HAB extent was down in 2020 after an early end to the growing season. However, this investigation found that the COVID-19 shutdowns were likely not a direct driver of these short-term anomalies. Instead, recent trends in the indicators and co-occurring anomalies in hydrological and meteorological conditions (e.g., lake temperature, river discharge, and wind speed) appeared to be more responsible for the detected water quality changes. Future work will investigate whether the shutdowns have a long-term or delayed impact on Great Lakes water quality.
Introduction
The coronavirus disease (COVID-19) was initially identified in January 2020 (Zhu et al., 2020). It spread slowly at first but the number of infections began to grow rapidly: from 44 confirmed cases on January 3, 2020 to 282 on January 20 (World Health Organization [WHO], 2020a) and over 9,826 confirmed cases in 20 countries by the end of January, prompting the World Health Organization (WHO) to issue a public health emergency of international concern (World Health Organization [WHO], 2020b).
The United States reported its first confirmed case of COVID-19 on January 20, 2020 (Holshue et al., 2020), and by February 7, 2021, had confirmed over 26 million infections and over 450,000 deaths due to the disease according to the WHO COVID-19 dashboard.1 The states of Michigan and Ohio reported their first positive cases in the initial weeks of March 2020 (Pelzer and Hancock, 2020; State of Michigan, 2020a). Despite efforts to slow its spread, both states experienced a rapid increase in infections and deaths in the ensuing weeks, leading the governors of both states to issue temporary shutdown orders beginning on March 23rd in Ohio (Ohio Department of Health, 2020) and 24th in Michigan (State of Michigan, 2020b). During these shutdowns, most economic activity was shut down and people were encouraged to stay at home. Surveys conducted in two subsequent weeks surrounding the start of the Michigan shutdown (March 21–22, 2020 and March 28–29, 2020) revealed the immediate impact of the shutdowns on resident’s activities. The percentage of respondents that reported staying at home all day for each of the prior 5 days increased from 19 to 47% and the percentage of respondents who went into work or a volunteer site at least once in the prior 5 days decreased from 46 to 30% (Cassidy-Bushrow et al., 2021). An analysis of mobile device data revealed that mobility decreased during the social distancing periods throughout both Michigan and Ohio (Garnier et al., 2021).
Initially intended to last just a few weeks, the shutdowns were extended several times as the disease continued to spread. Even as the shutdowns began to be lifted in the summer of 2020, many industries remained closed or opened at limited capacities (Bischoff, 2020; Hutchinson, 2020). According to US Bureau of Labor Statistics (BLS) data,2 the shutdowns resulted in massive employment losses in the Michigan and Ohio manufacturing industries, with 28 and 14% declines from March 2020 to April, respectively. These numbers had somewhat recovered by June, but were still below the pre-pandemic levels (11 and 6%, respectively) (Supplementary Figure 1). Daily testing data from the Centers for Disease Control and Prevention3 (CDC) reveal that the shutdowns helped to reduce the spread of COVID-19 until both states experienced resurgences in mid-summer (Supplementary Figure 2).
Shutdowns were a common approach used throughout the United States and in other nations. In addition to slowing the spread of COVID-19, there have been numerous reports of these shutdowns having a significant environmental impact. Several studies found that the declines in activity, including reduced vehicular traffic and industrial emissions, resulted in air quality improvements in parts of India, Italy, and Southeast Asia (Collivignarelli et al., 2020; Kanniah et al., 2020; Soni, 2021). Other studies identified improvements in marine and inland water quality coinciding with the periods of reduced activity. Many of these studies utilized remote sensing data in their analyses due to its ability to collect data at broad spatial and temporal scales and because it can be acquired without the potential health risks of in situ sampling. Multiple studies used Landsat 8 imagery to identify significant decreases in suspended particulate matter (SPM, used as an indicator of water pollution) in Indian inland waters (Aman et al., 2020; Yunus et al., 2020). These decreases were speculated to be due to reductions in industrial and tourism-related pollution as a result of the COVID-19 shutdowns. Another study utilized a selection of Sentinel-3 Ocean and Land Colour Imagery (OLCI) images and identified a reduction in chlorophyll-a (CHL) concentrations in the coastal waters off of India (extending over 100 km off shore in places) which was attributed to shutdown-related changes in urban and atmospheric nutrient deposition (Mishra et al., 2020). An analysis of Sentinel-2 imagery revealed improvements in water clarity in the Venice Lagoon due to the reduction in public transit and tourism-related boat traffic and a decline in wastewater discharge due to the lack of tourists (Braga et al., 2020). Finally, Cherif et al. (2020) utilized Sentinel-3-derived water temperature data to identify improved coastal water quality in Morocco as a result of COVID-19 related industrial discharge reductions. Each of these studies compared satellite-derived water quality metrics from imagery collected during the shutdown period to “normal” water quality as defined by a collection of historic images. However, these studies either used a single image from multiple years or multiple images from a single year (2019) to define the historic normal, potentially biasing their results if the images selected did not adequately represent the true normal state of the study areas.
As in the regions described above, human activity has been shown to have a large impact on water quality in the Great Lakes region, especially in regard to nutrient inputs. In response to widespread eutrophication in the lakes, the bi-national Great Lakes Water Quality Agreement (GLWQA) was signed in 1972 which resulted in significant reductions in point source nutrient inputs, largely due to improvements in wastewater treatment plants (Watson et al., 2016). These improvements in municipal wastewater treatment likely limit the impact of reductions in urban runoff which were hypothesized to cause some of the water quality improvements in India and Morocco. However, since the passage of the GLWQA, non-point source contributions of nutrients, including from agricultural runoff and residential septic systems, have become a major driver of water quality in several parts of the Great Lakes including the western basin of Lake Erie (WBLE) and Saginaw Bay in Lake Huron (SBLH) (He et al., 2014; Selzer et al., 2014; Stow et al., 2015). It has been estimated that 10–25% of Michigan’s million-plus on-site wastewater treatment (or septic) systems are in some level of failure (Michigan Office of the Great Lakes [OGL], 2016; Public Sector Consultants [PSC], 2018). In the five counties surrounding SBLH, this results in annual discharges of untreated or partially treated wastewater between 0.5 and 1.26 billion gallons (Public Sector Consultants [PSC], 2018). Rao and Schwab also note the occurrence of storm sewers draining directly into the Great Lakes which can impact the nearshore nutrient levels (2007). Additionally, changes in agricultural practices in response to the GLWQA have led to increased loadings of bioavailable phosphorus in WBLE (Scavia et al., 2014), which along with climate change and the introduction of zebra and quagga mussels, has led to the resurgence of the WBLE harmful algal bloom (HAB) in recent years (Watson et al., 2016).
Remote sensing has been a widely used tool for studying water quality in the Great Lakes due to their vast size and regional importance (the Laurentian Great Lakes make up over 20% of the freshwater on Earth and provide drinking water to over 35 million people; Herdendorf, 1982; Bootsma, 2018). The enhanced spatial and temporal sampling capabilities provided by remote sensing have helped further develop the link between anthropogenic forcing and water quality in the region (Michalak et al., 2013; Shuchman et al., 2017). Because of the greater optical complexity of these waters relative to the open ocean (Bukata, 2005; Sayers et al., 2019a), researchers have developed a range of empirical and semi-analytical water quality algorithms that are specifically tuned to the waters of the Great Lakes. Algorithms have been developed to study a range of parameters, including the concentrations of color producing agents (i.e., CHL, suspended sediments, and CDOM) (Binding et al., 2012; Shuchman et al., 2013), primary production (Warner and Lesht, 2015; Fahnenstiel et al., 2016), and the intensity and extent of HABs (Stumpf et al., 2012; Sayers et al., 2016).
The goal of this study is to use satellite imagery to understand whether the widespread disruptions caused by the COVID-19 pandemic had an observable impact on Great Lakes water quality. Due to the GLWQA-driven improvements to the municipal water treatment systems, it is unlikely that the shutdown-driven decrease in industrial activity would have a notable impact on the nutrient loads to the study areas. However, there are other ways in which nutrient loading could be impacted by the shutdowns. The transition to working at home and ceasing of most urban activity will shift some of the wastewater treatment loads from the highly effective municipal treatment plants to at-home septic systems which are more likely to release un-treated wastewater into the environment. Additionally, potential changes to the agricultural calendar, including the timing of plantings or fertilizer applications, could impact the nutrient loads. Because in situ sampling was heavily restricted due to the pandemic, this study used remote sensing to investigate the presence of any short-term water quality anomalies in the Great Lakes region that may be due to the COVID-19 shutdowns. For this analysis, short-term anomalies are defined as anomalies that become apparent in the weeks to months following the shutdowns. The analysis focused on water quality parameters which had been observed to be impacted elsewhere, including the concentrations of CHL and total suspended solids (TSS) and photic zone depth (PZD) was used as an indicator of water clarity. These parameters were investigated in WBLE and SBLH, two basins with an extensive history of anthropogenic impact. The HAB extent in WBLE was also investigated due to its regional importance and dependence on nutrient loading during the spring (Stumpf et al., 2012; Sayers et al., 2016, 2019b).
Materials and Methods
Study Areas
Two basins within the Laurentian Great Lakes region (WBLE and SBLH) were analyzed for impacts related to activity changes due to the COVID-19 pandemic (Figure 1).
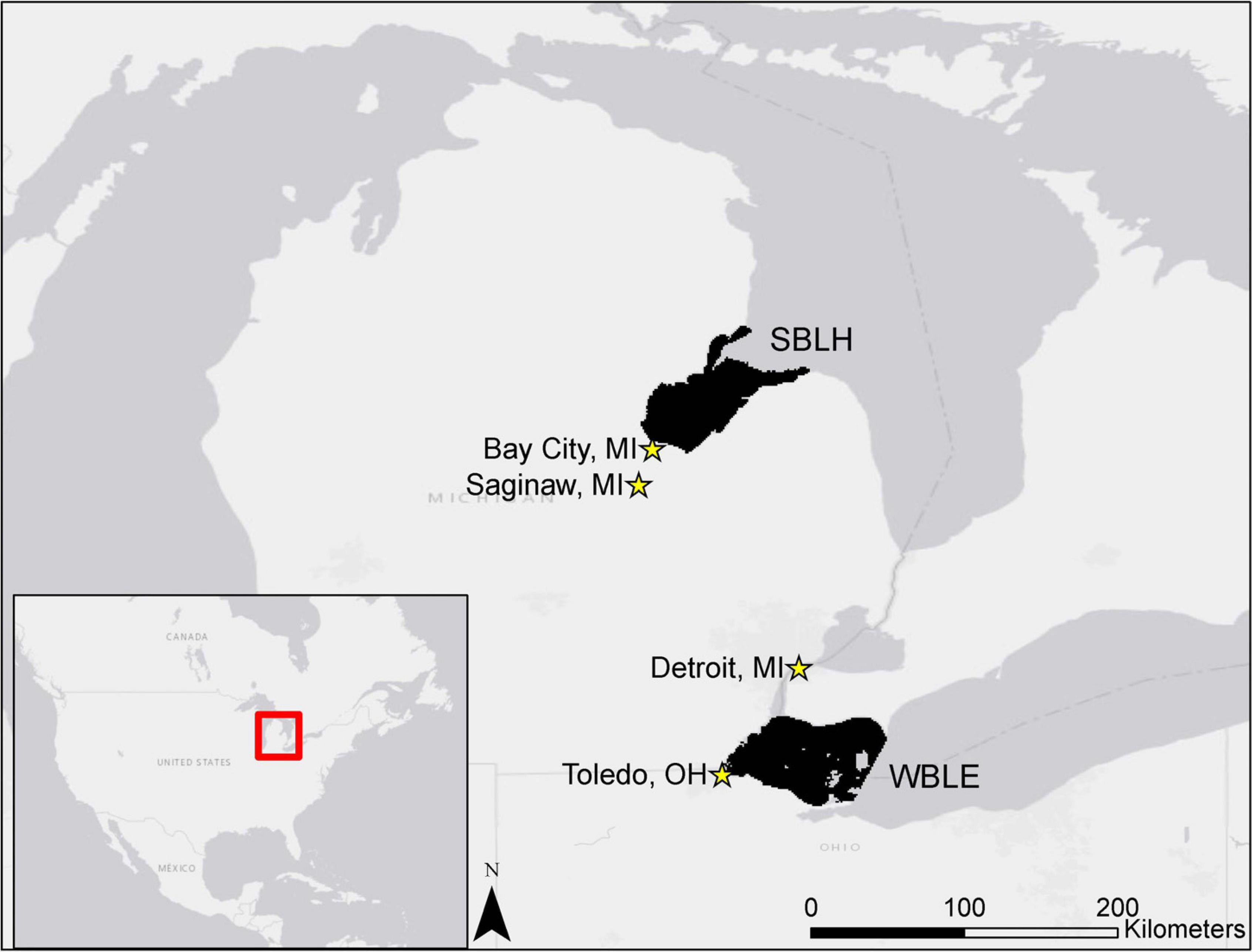
Figure 1. Regions studied for impacts related to the coronavirus (COVID-19) pandemic. The western basin of Lake Erie (WBLE) and Saginaw Bay in Lake Huron (SBLH) are heavily impacted by anthropogenic factors. The major population centers around each basin are also shown, including Toledo, Ohio and Detroit, Michigan (WBLE); and Bay City, Michigan and Saginaw, Michigan (SBLH).
The western basin of Lake Erie and SBLH were selected as they are two of the more eutrophic basins within the Great Lakes, Both have extended histories of anthropogenic impacts (Makarewicz and Bertram, 1991; Dolan, 1993; Stow et al., 2014) and HABs (Fahnenstiel et al., 2008; Bridgeman et al., 2013; Sayers et al., 2016, 2019b; Wynne et al., 2021). Much of the anthropogenic impact is driven by nutrient-rich runoff from heavily farmed watersheds and the nearby population centers (Michalak et al., 2013; Selzer et al., 2014). There are notable differences between the two regions as well, with the SBLH watershed having 60% lower population density than the WBLE watershed (Table 1). The land use within the watersheds differs as well, with SBLH having less agriculture and urban area (27 and 62% less than WBLE, respectively) and 3.6 times the forested area (Table 1). The SBLH coastline also consists of an extensive wetlands system which serves as a highly effective nutrient sink (Wynne et al., 2021). Due to the greater agricultural area and less forested area and wetlands within the watershed, WBLE experiences greater nutrient loads than SBLH (Wynne et al., 2021).
Satellite Data Acquisition and Pre-processing
The water quality metrics used for this study were derived from remote sensing imagery. The National Oceanic and Atmospheric Administration’s (NOAA) Visible Infrared Imaging Radiometer Suite (VIIRS) sensor onboard the Suomi National Polar-orbiting Partnership (SNPP) satellite was the source of imagery for this analysis, providing daily revisits at a 750-m resolution dating back to January 2012. Despite its coarse resolution, the VIIRS sensor has been used to study water quality in the Great Lakes due to its high temporal resolution (Binding et al., 2020; Son and Wang, 2020) which is necessary due to the frequent cloud cover in the region (Ackerman et al., 2013) and highly variable nature of the water conditions in the more eutrophic basins (Sayers et al., 2019a).
For each region, all intersecting imagery from March through December was acquired through the National Aeronautics and Space Administration (NASA) Ocean Biology Processing Group (OBPG) OceanColor Web data portal.4 In total, over 4,300 images were processed for each of the two study regions. Data was acquired at Level 1, with no atmospheric corrections having been applied, along with the corresponding geo-location files. Using the Level 1 and geo-location data, the VIIRS images were subset to the regions of interest and processed to Level 2 using OBPG’s L2gen module, applying a fixed model pair atmospheric correction. Atmospheric correction has been a key concern in freshwater remote sensing (Binding et al., 2020), particularly in the blue spectral bands (Budd and Warrington, 2004; Shuchman et al., 2013; Binding et al., 2019). Shuchman et al. evaluated eight different atmospheric correction techniques by comparing satellite-retrieved reflectance to in situ reflectance collected in a range of Great Lakes water types including sediment-dominant and chlorophyll-dominant waters (2013). The fixed model pair approach tested in their analysis performed well in each setting and produced the most accurate reflectance in intense HAB scenarios, where other correction techniques interpreted the elevated near infrared reflectance associated with near-surface HABs as atmospheric contamination (Shuchman et al., 2013; Sayers et al., 2016).
Derivation and Validation of Water Quality Metrics
The Color Producing Agents Algorithm (CPA-A; Shuchman et al., 2013) was used to estimate concentrations of CHL and TSS as well as CDOM absorption at 443 nm from the satellite-derived remote sensing reflectance at the following bands: 443, 486, 551, and 671 nm. This algorithm uses lake-specific parameterizations based on the range of observed inherent optical properties (IOPs) and has been shown to produce reliable results (Shuchman et al., 2013; Fahnenstiel et al., 2016), including root mean square error (RMSE) values ranging from 0.13 mg/m3 in Lake Huron to 2.18 mg/m3 in Lake Erie (Shuchman et al., 2013). PZD, defined as the depth where 1% of surface light remains, was used as an indicator of water clarity and was calculated from the CPA-A results as described in Fahnenstiel et al. (2016). Briefly, bulk absorption and backscatter are derived from the CPA-A retrievals and used to estimate the light attenuation coefficient at 490 nm (Kd490) using methods proposed by Lee et al. (2005). KdPAR was derived empirically from Kd490 following methods from Saulquin et al. (2013). The inverse of KdPAR is defined as one optical depth, and the PZD is calculated as 4.605 optical depths (Lee et al., 2007).
Harmful algal bloom extents in WBLE were derived from the CHL measurements. Using surface water measurements of CHL and phycocyanin pigment (PC, which can be used as an indicator of HAB presence), Sayers et al., found that minimal PC was observed at CHL concentrations below 18 mg/m3 (2016). Above that threshold, the PC increased linearly with the CHL concentration, indicating that increased CHL is associated with HAB presence (Sayers et al., 2016). This threshold has been used to map WBLE HAB extents in multiple studies (Sayers et al., 2016, 2019b; Manning et al., 2019) with results comparing well to in situ surveys (87% mapping accuracy, Sayers et al., 2016). During the HABs growing season, defined as lasting from July through October (Bridgeman et al., 2013; Wynne and Stumpf, 2015), HAB extents were calculated by first converting CHL concentration maps to binary maps using the 18 mg/m3 threshold, with each pixel indicating HAB presence or absence. The HAB extent was then calculated as the count of pixels classified as a HAB multiplied by the pixel size.
While the water quality algorithms used in this analysis were specifically calibrated for the Great Lakes, significant changes in water quality due to the COVID-19 shutdowns could render these prior calibrations obsolete and add uncertainty to the 2020 retrievals. Due to COVID-19 restrictions, there is limited in situ data available from early 2020 in order to validate the derived water quality metrics. However, near-weekly water quality sampling in WBLE and SBLH started in June and July 2020, respectively, and continued through the start of October. Sampling was conducted by scientists from the Cooperative Institute for Great Lakes Research (CIGLR) and NOAA’s Great Lakes Ecological Research Laboratory (GLERL). This data, which included extracted CHL concentrations and Secchi disk depths, were acquired from the NOAA-GLERL website5 and used to assess the performance of the remote sensing algorithms in light of the potential water quality changes. Summary statistics for these observations are reported in Table 2. These in situ metrics were compared to same-day derived products, averaged over a 3 × 3 grid surrounding the sampling location. Because both the in situ and remotely sensed CHL retrievals have associated uncertainty, the relationship between the two variables was assessed using type II linear regression with the lmodel2 package in R (Legendre, 2018). While Secchi disk depth and PZD are fundamentally different metrics, both are indicators of water clarity and have been shown to be related (Lee et al., 2018). For this analysis, the correlation between remote sensing-derived PZD and in situ Secchi disk depth was used to assess the general accuracy of the remote sensing product and its ability to track changes in water clarity.
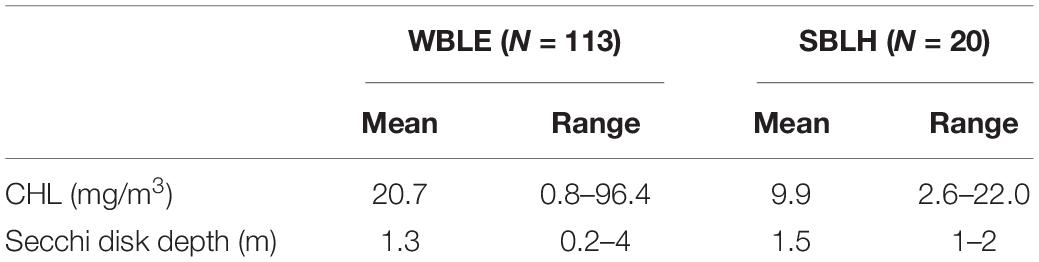
Table 2. Summary statistics for the in situ chlorophyll-a (CHL) and Secchi disk depth measurements collected in western basin of Lake Erie (WBLE) and Saginaw Bay in Lake Huron (SBLH) during the 2020 field season.
Assessment of Water Quality Change
For each of the remote sensing-derived metrics, data from 2020 was compared to a historical baseline in order to assess change. The immediate impacts of the shutdowns were evaluated by comparing data from April 2020 to April data from prior years. April was chosen because both the Michigan and Ohio shutdowns were in place for the duration of the month and this also coincided with when the social distancing mobility decreases were peaking (Garnier et al., 2021). The metrics were also evaluated in 10-day windows from March 1 through the end of the year to assess whether any water quality changes identified during April were present prior to the shutdowns or continued as the shutdowns began to be lifted.
Two filters were applied to the remote sensing-derived data before generating the 10-day and monthly composites. First, any pixels where the CPA-A optimization failed to retrieve a valid CHL or TSS value were eliminated. Second, any CHL value where the corresponding TSS concentration was greater than 5 mg/L was removed since the heavy sediment signature can overwhelm any impact of varying CHL concentrations on the spectral signature. The 5 mg/L threshold was determined through a validation using in situ CHL and TSS concentrations from the CIGLR routine water quality monitoring dataset (Cooperative Institute for Great Lakes Research et al., 2019). CPA-A derived CHL estimates were compared to in situ CHL measurements and grouped by the corresponding TSS concentrations in 1 mg/L bins. The RMSE was then calculated within each bin, revealing a distinct shift at the 5 mg/L TSS level. The mean RMSE for TSS bins below the threshold was 6.1 mg/L compared to an RMSE of 29.8 mg/L when TSS exceeded the threshold.
Composite maps were generated in the same way for both 10-day windows and monthly data. For each year in the study period (2012–2020), composite maps were generated by calculating the mean of all images from that year within the given date range. To assess the historical significance of the observed post-shutdown water quality, the basin-wide median values from 2012 to 2019 were compared to those from April 2020 using a Wilcoxon signed-rank test (Wilcoxon, 1945) with an alpha value of 0.05 used to determine significance. Historic baseline composite maps were generated by calculating the mean of all 2012–2019 images within the given date range. Anomaly maps were then calculated for each analysis period by comparing the 2020 composite to the historic baseline composite. For each pixel in the study region, the anomaly was derived as the percent change from the baseline to the 2020 metric value (calculated as the difference between the 2020 and baseline values divided by the baseline value, and multiplied by 100). A positive anomaly indicated that the 2020 metric value was elevated relative to the historic baseline. For CHL and TSS, this represented increased concentrations, and a positive PZD anomaly indicated clearer water. In order for a 10-day window to be included in the time series, the 2020 composite map needed to have valid data in at least 50% of the pixels for that region. This requirement was put in place in order to avoid seemingly anomalous data points caused by sparse data coverage.
Harmful algal bloom extents in WBLE were generated from each of the 10-day CHL composite maps during the HABs growing season. For each 10-day window, the mean and standard deviation of HAB extent from past years were calculated to determine the historic baseline. The HAB extent anomaly was then calculated as the percent difference between the 2020 HAB extent and the baseline HAB extent.
Ancillary Indicators
In addition to the primary water quality indicators (CHL, TSS, PZD, and WBLE HAB extent), other metrics were investigated as potential drivers of water quality change. These included lake surface temperature (LST), which is known to impact CHL production and HAB growth (Behrenfeld and Falkowski, 1997; Paerl and Huisman, 2008; Fahnenstiel et al., 2016). 2012–2020 LST data from the VIIRS sensor onboard the SNPP satellite was downloaded from the OBPG OceanColor Web at Level 2. The SST_triple product, using the default atmospheric correction (Minnett et al., 2014), was used to generate the composites and anomalies. Non-remote sensing-derived explanatory indicators included river discharge and wind speed which have been shown to impact sediment loading and HAB growth (Rao and Schwab, 2007; Stumpf et al., 2012; Sayers et al., 2016; Niu et al., 2018). Mean daily discharge data was acquired from the United States Geological Survey (USGS) National Water Information System (NWIS) using gages on the Maumee River (WBLE, gage 04193500), Detroit River (WBLE, gage 04165710), and Saginaw River (SBLH, gage 04157005). Hourly wind speeds were downloaded from buoys in NOAA’s National Data Buoy Center (NDBC) network (WBLE: THL01; SBLH: SBLM4). Baseline (2012–2019) and 2020 metrics, as well as anomalies (10-day and April) were calculated for each of the explanatory indicators using the same methods as for the water quality indicators. Finally, agricultural planting data were acquired from the United States Department of Agriculture (USDA) National Agricultural Statistics Service (NASS) 2015–2020 Historical Crop Progress reports.6 From this data, 2020 planting progress was evaluated against the prior 5-year average for three different crops (oats, corn, and soybeans) to determine if the shutdowns impacted agricultural activity.
Results
Of the 134 surface water measurements collected in WBLE and SBLH in 2020, there were only 20 with valid same-day remote sensing estimates (15 in WBLE, 5 in SBLH) (Figure 2). The in situ CHL concentrations within these 20 matchups ranged from 1.7 to 31.3 mg/m3. The Type II linear regression revealed strong agreement between the measured and estimated concentrations (R2 = 0.66, p < 0.01). Similarly, there was a strong positive correlation between the in situ Secchi disk depth and the remotely sensed PZD (Spearman’s rho = 0.69, p < 0.01) indicating that the PZD metric used in this analysis was adequately capturing changes in water clarity.
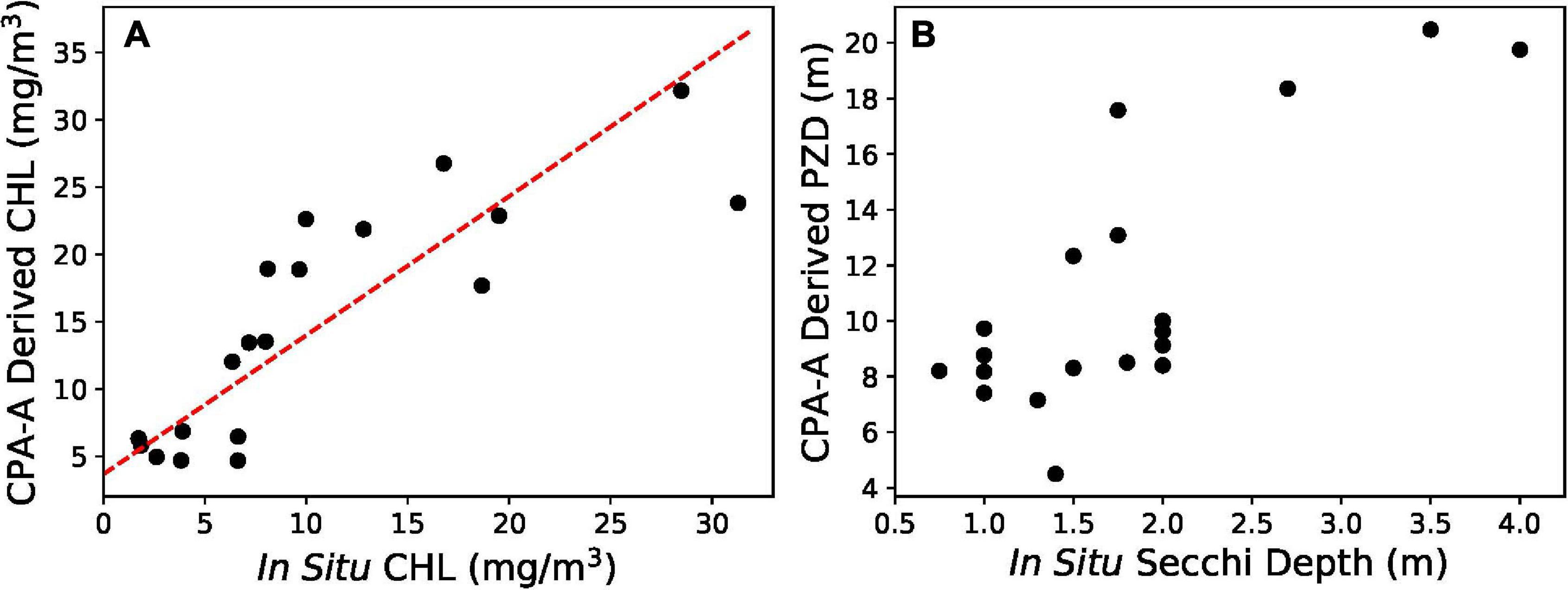
Figure 2. Comparisons between remote sensing estimates and in situ measurements in 2020. Panel (A) shows a comparison between remotely sensed and in situ chlorophyll-a (CHL), with the type II regression best fit line displayed in red (y = 3.67 + 1.03 × x; R2 = 0.66; p < 0.01). Panel (B) shows the comparison between remotely sensed photic zone depth (PZD) and in situ Secchi disk depth.
The annual time series of basin-wide median April indicator values (calculated from the annual April composite maps) were used to assess how water quality immediately after the shutdown began compared to prior years and whether the 2020 values were a continuation of a trend that preceded the COVID-19 pandemic (Figure 3). Using these plotted values, a statistical comparison was made between the 2020 observations and those from prior years in the study record. The basin-wide 2020 metric value was found to be significantly different from the historic record for all three metrics in SBLH and CHL in WBLE (Wilcoxon signed-rank test statistics and corresponding p-values reported in Table 3). Of all the metrics investigated, only SBLH CHL (Figure 3B) had a 2020 value falling more than two standard deviations away from the 2012–2019 mean, though this metric also appears to be in the midst of a multi-year increasing trend that pre-dates the pandemic. The 2020 TSS indicators for both basins fell within the two standard deviation window but were also at the low-end of the recent historic range after experiencing a steady decline in the past 3–4 years (Figures 3C,D), with the inverse being true for PZD (Figures 3E,F).
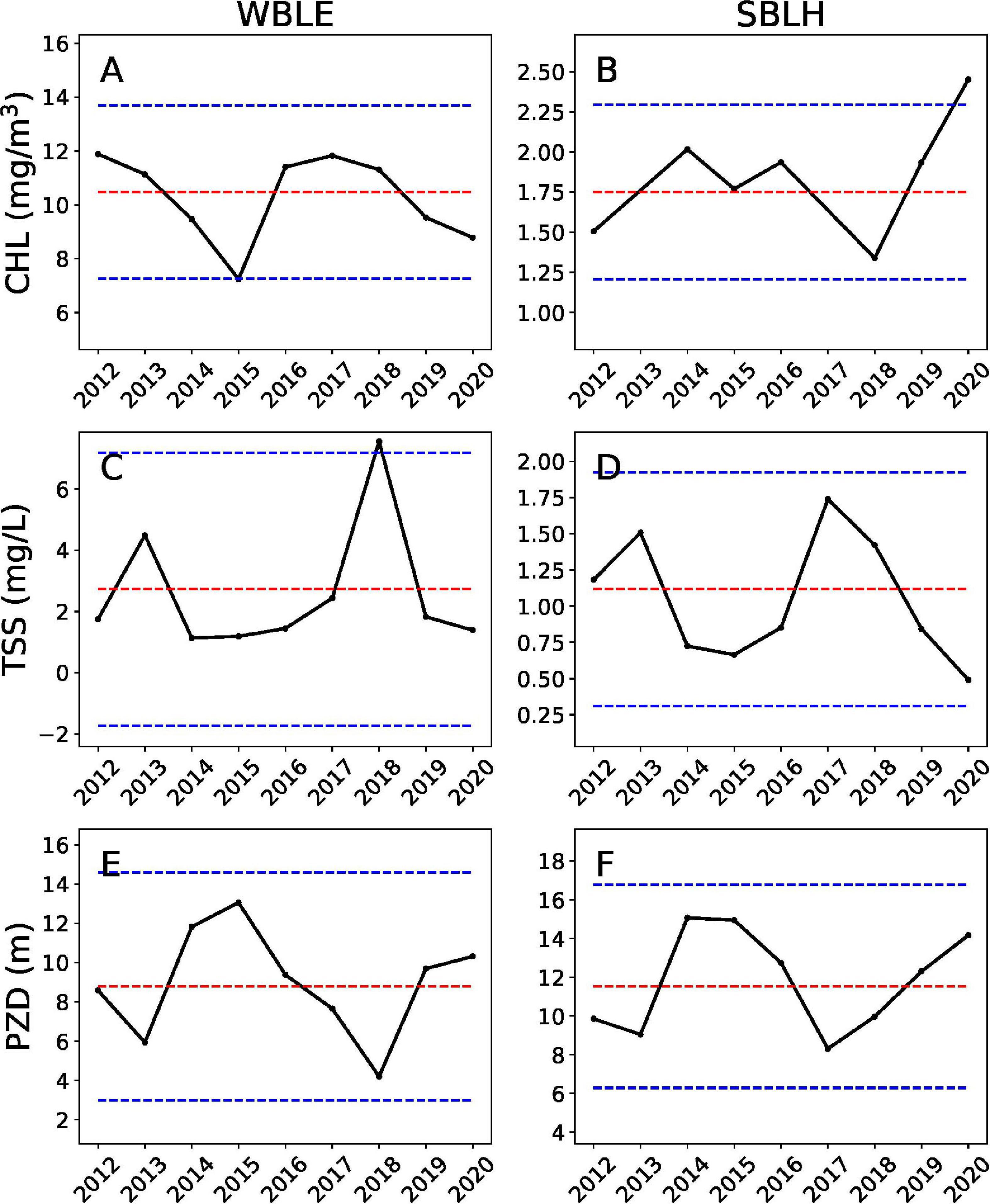
Figure 3. Median April indicator values plotted over the sensor data record (2012–2020). Years in which less than 50% of the regional data pixels have data are excluded. The 2012–2019 mean is shown as the dashed red line, with the dashed blue lines representing the 2012–2019 mean plus/minus two times the 2012–2019 standard deviation. (A,C,E) The CHL, TSS, and PZD indicator time series for WBLE. (B,D,F) The CHL, TSS, and PZD indicator time series for SBLH.
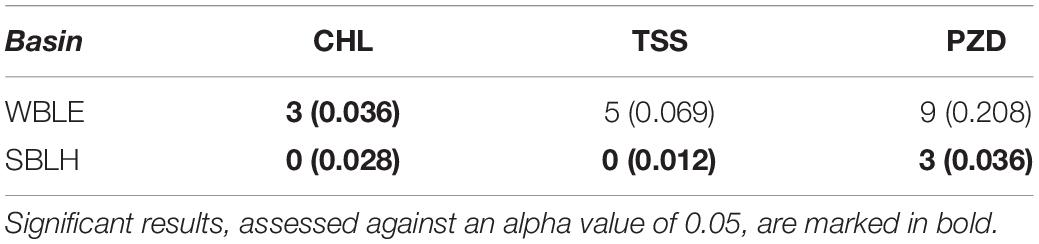
Table 3. Wilcoxon signed-rank test statistics (W) and p-values (in parentheses) for the analyses comparing basin-wide median metric values from April 2012–2019 to 2020 values.
Mapping the anomaly data revealed spatial variability across each study basin (Figure 4). The majority of WBLE CHL concentrations (Figure 4A) were near or below the historic baseline except near the mouth of the Maumee River where the anomalies were largely positive. The SBLH CHL anomaly map (Figure 4D) showed widespread positive anomalies, with no clear spatial trend relative to the Saginaw River. Rather, positive anomalies were observed in most pixels except for the center of the bay, which rarely experiences significant phytoplankton accumulation due to the bay’s circulation patterns (Wynne et al., 2021). TSS anomalies in both WBLE and SBLH were widely negative with a few exceptions, including near the mouths of the Maumee and Saginaw Rivers (Figures 4B,E). The PZD anomalies in both regions were mostly positive, indicating increased water clarity in April 2020 (Figures 4C,F). Median basin-wide April anomaly values for each primary indicator are displayed in Table 4.
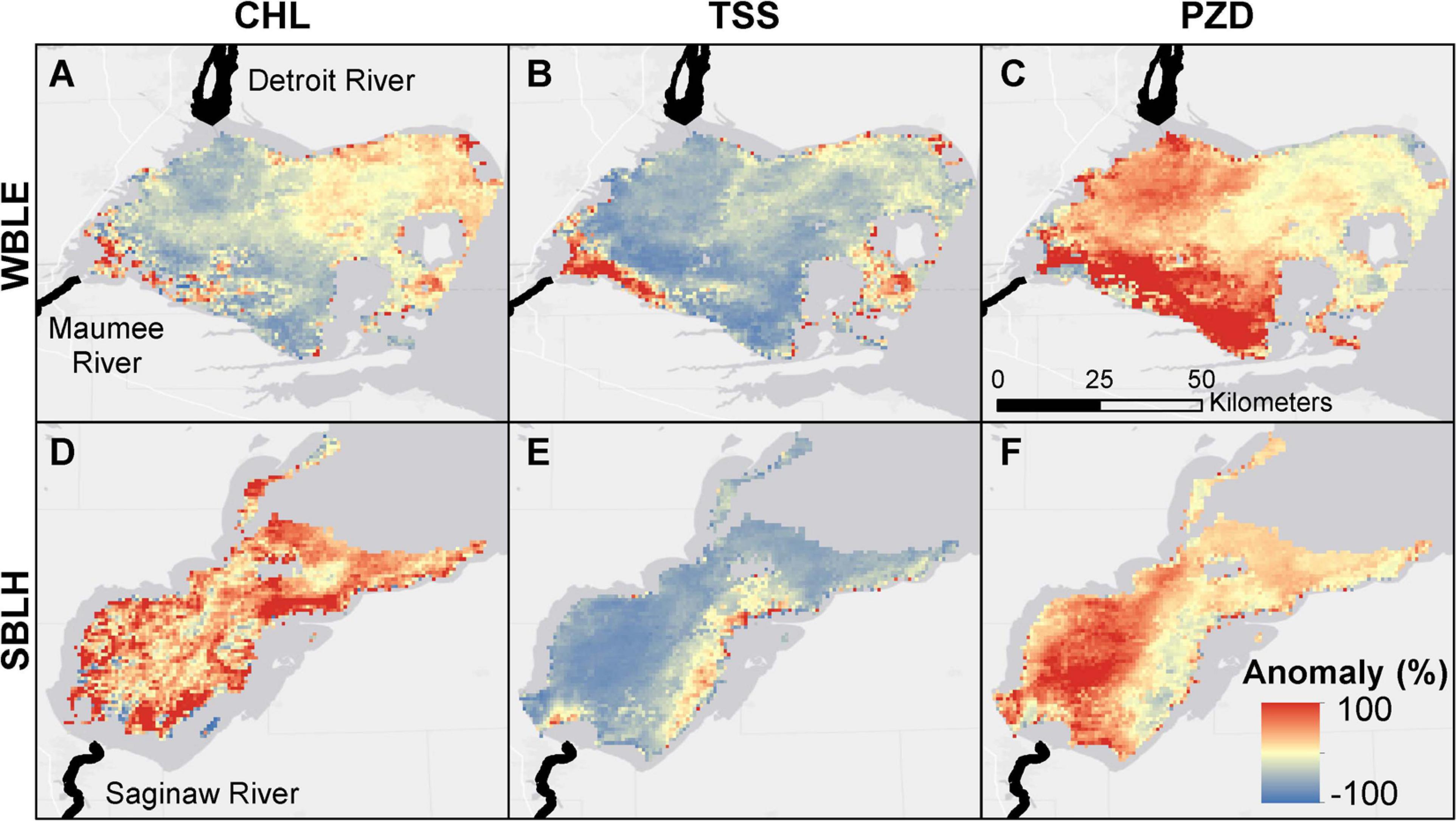
Figure 4. April anomaly maps for CHL, total suspended solids (TSS), and PZD in the two study basins. All maps are scaled from blue (large negative anomaly) to red (large positive anomaly). The locations of each basin’s major rivers (Maumee and Detroit Rivers in WBLE; Saginaw River in SBLH) and where they enter the basins are displayed in black. (A–C) The WBLE April anomaly maps for CHL, TSS, and PZD, respectively. (D–F) The SBLH April anomaly maps for CHL, TSS, and PZD, respectively.
Much of the spatial variability observed in Figure 4 appeared to be related to proximity to the major river mouths. Plotting the April 2020 water quality anomalies against this distance reveals several distinct relationships (Figure 5). As seen in Figure 4, the SBLH TSS anomaly is highest within 5 km of the Saginaw River mouth where it is approximately equal to the historic baseline, but is highly negative outside of this area (Figure 5F). This trend is reversed for the SBLH PZD anomalies (Figure 5I). The trends in WBLE are slightly more complex due to the presence of two major rivers flowing into the basin. The CHL and TSS anomalies are most positive within 5–10 km of the Maumee River mouth (Figures 5A,D). However, both indicators experience a strong negative anomaly just outside of that range, with concentrations moving toward the historic baseline as distance increases. The trends relative to the Detroit River are more clear, with the strongest negative anomalies occurring near the river mouth and gradually increasing with distance (Figures 5B,E).
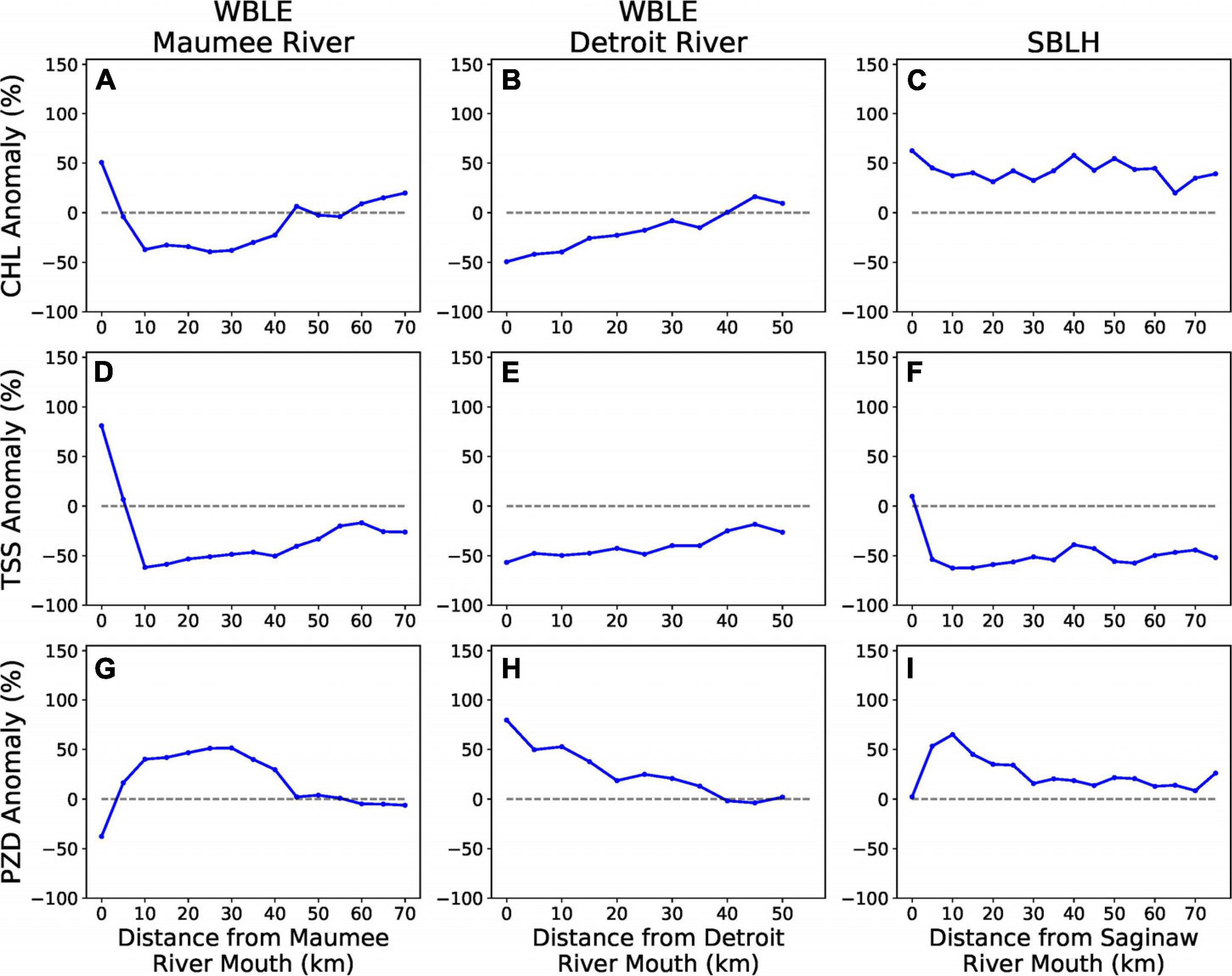
Figure 5. Median April anomaly value is plotted based on distance from the nearest major river mouth (Maumee and Detroit Rivers for WBLE; Saginaw River for SBLH). The distances are binned in 5-km increments. (A,D,G) The average CHL, TSS, and PZD anomalies in WBLE based on distance from the Maumee River. (B,E,H) The average CHL, TSS, and PZD anomalies in WBLE based on distance from the Detroit River. (C,F,I) The average CHL, TSS, and PZD anomalies in SBLH based on distance from the Saginaw River.
The water quality trends observed in April were generally also present throughout the year, as seen in the 10-day anomaly time series (Figure 6). These plots begin with the 10-day window starting on day 61 (March 1 in leap years; March 2 otherwise). The boxplots (Figures 6C,F,I) show the range of median anomaly values across the valid windows (a window was considered valid if the percent of pixels with data exceeded 50%). WBLE and SBLH had similar seasonal anomaly trends for both TSS and PZD. The TSS anomalies (Figures 6D,E) were consistently negative in both basins until early September aside from two outlier events in late-May and early-June. Conversely, the PZD time series (Figures 6G,H) revealed consistently positive anomalies aside from a few isolated events. Slight differences between the two regions were revealed in the CHL anomaly time series (Figures 6A,B). While the CHL anomaly in SBLH was almost uniformly positive from March through August, the CHL anomaly in WBLE fluctuated around zero until mid-August, before experiencing a brief extreme positive anomaly in late-August (+79%). Both basins experienced prolonged periods of lower anomalies in September and October, though WBLE anomalies were up to 62% below the historic baseline and near zero in SBLH. As seen in the CHL anomaly boxplots (Figure 6C), SBLH had higher anomalies on average than WBLE (14.7 and −6.3%, respectively), and also experienced a much tighter range of anomalies (from −6% in June to +56% in November) while WBLE experienced anomalies ranging from −62 to +86%.
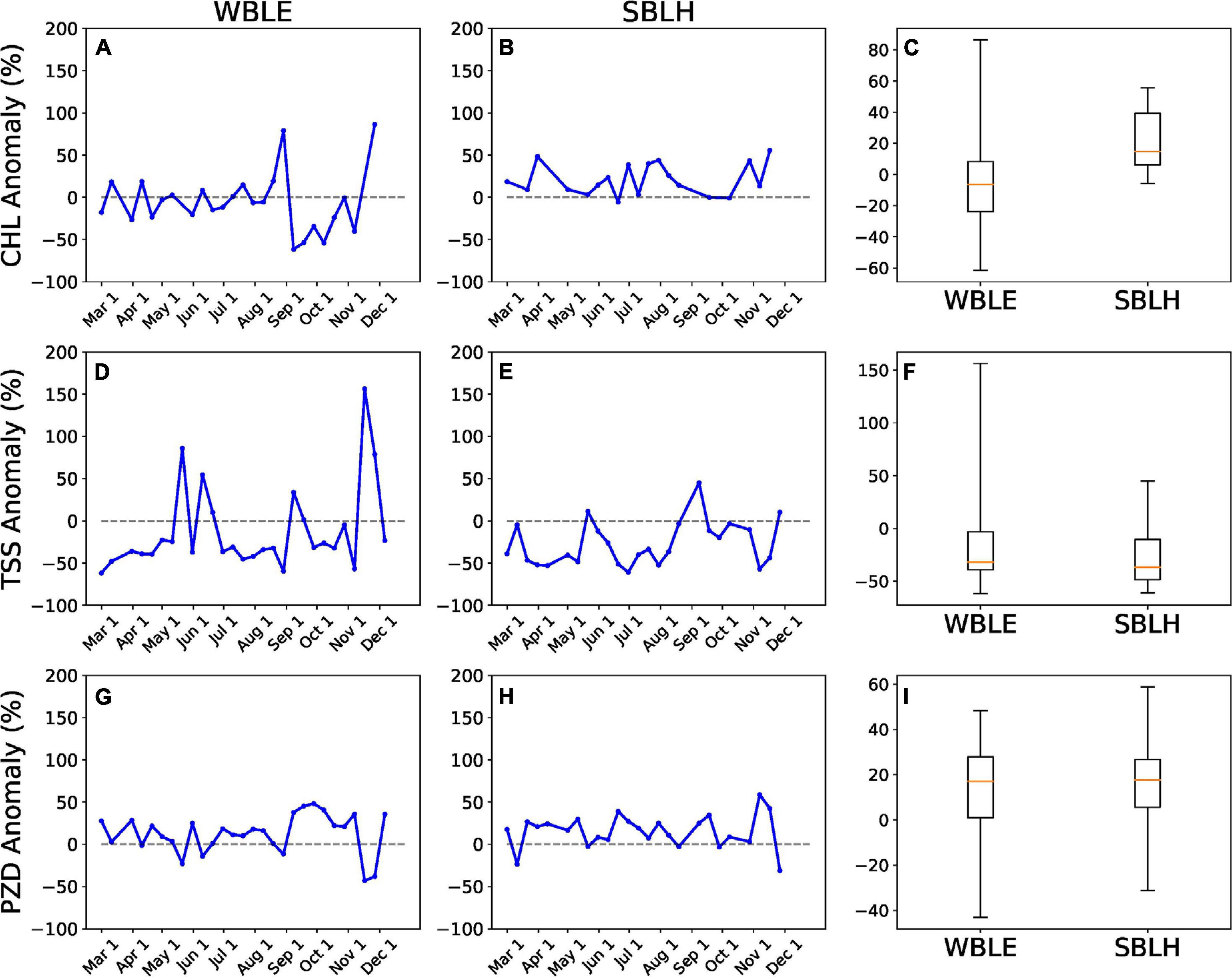
Figure 6. Median basin-wide anomalies for the 10-day windows starting from March 1 to December 26. Windows in which less than 50% of the regional data pixels have data are excluded. (A,D,G) The CHL, TSS, and PZD anomaly time series for WBLE. (B,E,H) The CHL, TSS, and PZD anomaly time series for SBLH. (C,F,I) The distribution of CHL, TSS, and PZD anomalies in both basins from March through December.
The seasonal progression of the WBLE HAB extent anomaly (Figure 7) was nearly identical to that of the WBLE CHL anomaly. Through July and the first few weeks of August, the 2020 HAB was slightly below the baseline extent, but within the normal range of variability. However, by mid-August, the extent began increasing rapidly, peaking in the first week of September 122% above the historic mean and several weeks earlier than the typical peak. The following week saw a rapid decline in extent, with a near total absence (mean anomaly of −86%) through the rest of the HAB season.
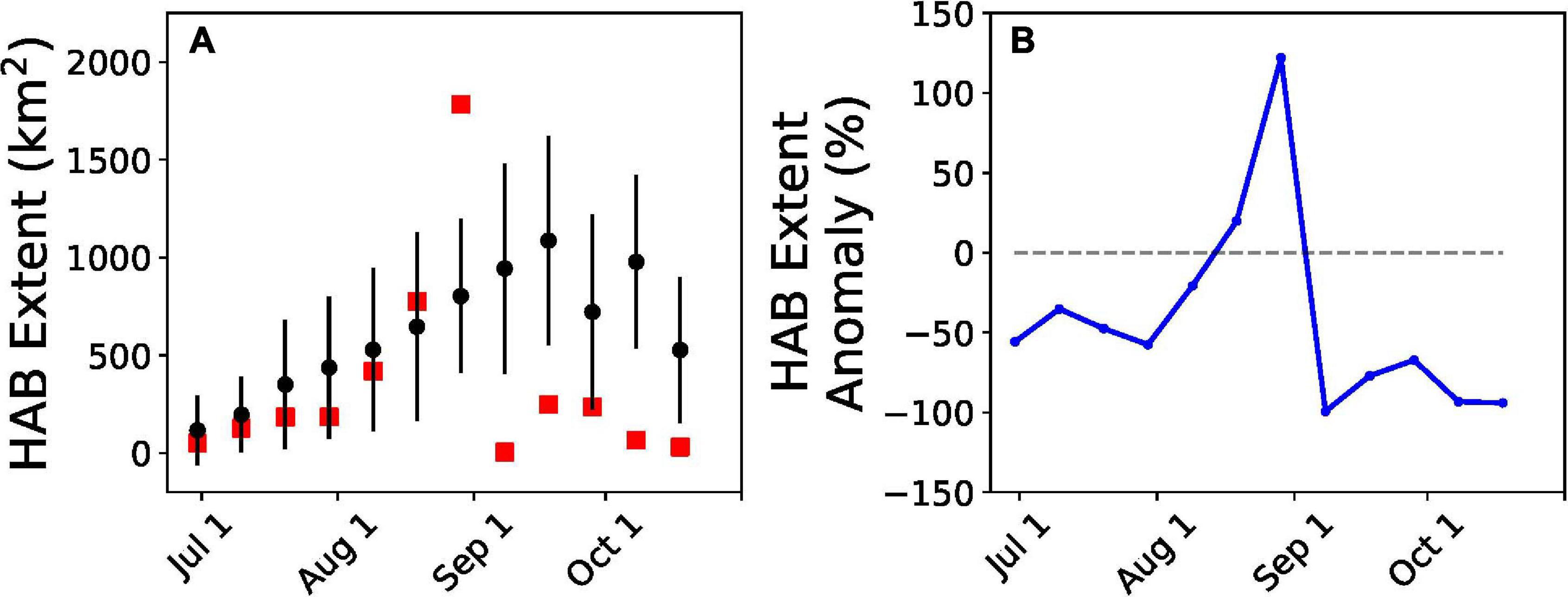
Figure 7. Panel (A) shows median WBLE harmful algal bloom (HAB) extent for the 10-day windows starting from June 30 to October 17. The red squares represent the 2020 extents and the black dots represent the mean of prior years’ (2012–2019) extents. The vertical black lines represent the standard deviations of the prior years’ extents. Panel (B) shows the anomaly time series for the HAB extent.
Seasonal and April anomalies were also assessed for three of the explanatory indicators. River discharge observed the most drastic anomalies, with the Maumee and Saginaw Rivers having April 2020 flow rates 46 and 52% below the 2012–2019 baseline, respectively, and the Detroit River with an above-average flow rate (+21%) (Table 5). Less extreme negative anomalies were also observed for the LST (−8 and −6% in WBLE and SBLH, respectively) and wind speed (−10 and −2%) metrics.
The seasonal anomaly trends for the explanatory indicators revealed similar results between WBLE and SBLH (Figure 8). LST in both basins (Figures 8A,B) was above average through most of March followed by negative anomalies from mid-April into June and again from September through mid-October. Each basin also experienced prolonged positive anomalies from mid-June through August. These anomalies were relatively small compared to those from the primary water quality indicators, with both regions showing a mean absolute anomaly across all valid windows of less than 10%.
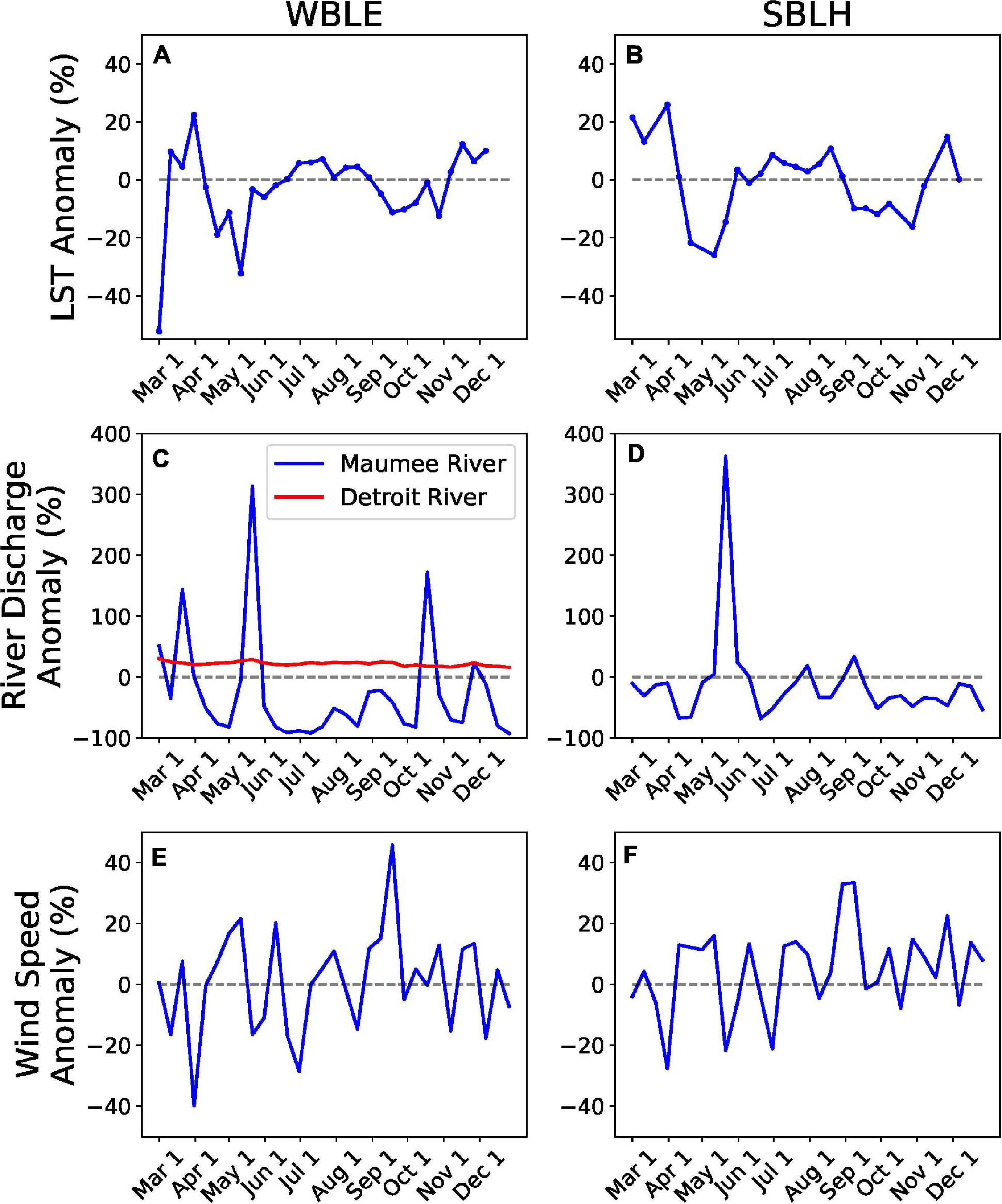
Figure 8. Median 10-day anomalies for the explanatory indicators, including lake surface temperature (A,B), river discharge (C,D), and wind speed (E,F).
The Detroit River (Figure 8C) had a consistently positive discharge anomaly (ranging from 15 to 30% above the historic baseline), but both the Maumee River (Figure 8C) and Saginaw River (Figure 8D) generally observed negative or near-zero discharge anomalies from April through September aside from a few extreme positive anomalies in mid-May. The wind speed time series (Figures 8E,F) showed no consistent anomalies throughout the season, fluctuating back and forth around the baseline. The largest observed anomaly was in WBLE at the start of September, when 2020 wind speeds were more than 40% higher than the historic baseline. This extreme wind event, along with the negative LST anomaly that started in September, likely contributed to the rapid decline in WBLE HAB extent.
The agricultural planting progress data was also analyzed to determine if the pandemic shutdowns had any impact on when oats, corn, or soybeans were planted. Some discrepancies were observed between the 2020 and 2015–2019 baseline, though these depended on the crop (Supplementary Figure 3). Throughout the data record, oats were the earliest crops to be planted. In 2020, despite the pandemic-driven shutdowns, planting progress for the oats was generally at least 25% ahead of the historic average through the first week of May. Corn and soybean plantings began at the end of April. The planting progress for corn was behind the baseline for several weeks but caught up by mid-May. Meanwhile 2020 soybean planting progress was ahead of the historic schedule throughout the season.
Discussion
This study highlights the importance of remote sensing for environmental monitoring. In a typical year in the Great Lakes, NOAA-GLERL and CIGLR conduct routine vessel and buoy-based water quality sampling in WBLE and SBLH (see text footnote 5). Due to the health and safety concerns surrounding the COVID-19 pandemic, both vessel-based sampling and buoy deployment were delayed and limited in capacity. Satellite-based remote sensing makes it possible to investigate water quality without the health concerns of in situ sampling, and it provides data at a broader spatial and temporal scale than is possible with boat- and buoy-based measurements. The extensive remote sensing historical data record also allows for the assessment of anomalies over broad spatial regions. Without this data, we would not have been able to assess how the COVID-19 shutdowns impacted Great Lakes water quality.
Extending beyond the Great Lakes region, polar-orbiting satellites like the one used in this analysis collect data on a global scale, allowing for coordinated analyses across the United States and internationally. NASA, in collaboration with the European Space Agency (ESA) and Japan Aerospace Exploration Agency (JAXA), assembled research teams from across the world to assess how the pandemic has impacted a range of environmental and societal indicators. Results of this collaboration, including the data presented in this article, are available on NASA’s COVID-19 dashboard.7
This investigation identified statistically significant short-term anomalies in several Great Lakes water quality metrics in the weeks and months following the start of the COVID-19 shutdowns, yet these anomalies cannot be directly attributed to the shutdowns as an examination of meteorological and hydrological variables has provided other plausible explanations for the observed changes Both WBLE and SBLH experienced large declines in TSS levels relative to the historic baseline in April 2020 as peak social distancing was occurring. However, co-occurring anomalies in the ancillary indicators could help explain this observation more than the changes in human activity. Both regions also experienced below average wind speed and river discharge rates in April 2020. The Detroit River was the exception, with above average discharge rates in April 2020. However, the Detroit River contributes very little sediment to WBLE, providing over 90% of the discharge but less than 6% of the sediment input, while the Maumee River provides 3% of the discharge and 45% of the sediment load (Niu et al., 2018). Because river discharge has a key role in nearshore sediment loading (Rao and Schwab, 2007) and high turbidity events in the offshore waters in WBLE are driven primarily by wind-driven resuspension (Niu et al., 2018) it is likely that natural factors (i.e., reduced wind speed and discharge) were driving the TSS reductions more than any COVID-19 impacts.
Investigating the TSS anomalies spatially revealed that despite the decreased discharge, increased TSS levels were observed in the 5–10 km nearest to the urban-adjacent Maumee and Saginaw River mouths and more negative anomalies were observed with increased distance. It is these nearshore areas that are most likely to be impacted by anthropogenic impacts such as urban or agricultural runoff (Rao and Schwab, 2007; Niu et al., 2018). However, there are plausible natural explanations for these trends as well. The reduced river discharge likely resulted in a greater concentration of the sediment nearer to the river mouths, unable to propel it further outward into the basins. This is particularly likely in WBLE, where the elevated sediment-poor Detroit River flow would further limit the extent of the diminished sediment-rich Maumee River plume (Jiang et al., 2015).
Saginaw Bay in Lake Huron also experienced a statistically significant CHL anomaly in April, with an increase of over 40% relative to the historic baseline. If this CHL increase were related to the pandemic, it would likely be due to an anomalously large influx of non-urban nutrients. It is unlikely that an increase in agricultural nutrient inputs would lead to positive anomalies basin-wide, particularly due to the greatly diminished Saginaw River discharge rates. And although the increased usage of residential septic systems during the shutdowns may have resulted in increased nutrient inputs along the SBLH coastline, this impact would most likely be observed in the very nearshore waters which are not visible with the coarse resolution satellite used in this analysis. As with TSS, there are several potential explanations for the CHL anomaly aside from the COVID-19 shutdowns. The time series of April CHL concentrations in SBLH (Figure 3B) indicated that the basin-wide median was stable from 2012 to 2018, but the last 2 years have seen a large increase, perhaps indicating an ongoing trend un-related to COVID-19. The decreased TSS concentrations may also be contributing to the increased CHL. Suspended sediment concentrations have been shown to be highly correlated with KdPAR (Millie et al., 2003), which is likely driving the significantly increased water clarity throughout the basin (Figure 4H). Other research in the Great Lakes has shown that increases in water clarity can cause increased phytoplankton production (Bierman and Dolan, 1981; Lohrenz et al., 2004; Jiang et al., 2015). WBLE also had a statistically significant CHL anomaly in April 2020. However, unlike SBLH, WBLE had a negative basin-wide CHL anomaly, but this varied considerably with distance from the Maumee River mouth. The 5 km nearest to the river mouth experienced a positive anomaly, while negative anomalies were observed from 5 to 45 km. Despite the increased water clarity similar to SBLH (Figure 4C), the decreased CHL is likely due to a nutrient limitation caused by the combination of increased nutrient-poor Detroit River discharge and decreased Maumee River discharge (Jiang et al., 2015). The stronger Detroit River plume would force the nutrient-rich Maumee River waters along the coastlines, consistent with the observed locations of increased CHL concentrations.
If the anomalies observed in April 2020 were caused by the pandemic-driven shift in activity, then it would be expected that they would dissipate in the weeks prior to and after the peak social distancing period. Instead, the trends that were observed in April tended to also be true in March and persisted further into the year. This was also generally true for the ancillary indicators which provided alternative explanations for the anomalies. WBLE and SBLH each observed negative TSS anomalies in over 80% of all qualifying 10-day windows from March 1 until the start of September. Deviations from this trend were also well explained by the ancillary indicators. Both basins observed an elevated TSS event in late May and early June, coinciding with large discharge anomalies due to an extreme storm event in late May which led to a 500-year flood event in mid-Michigan (French, 2020). Another positive TSS anomaly occurred in the first week of September, coinciding with the largest positive wind anomaly in each basin which we would expect to cause significant sediment resuspension (Niu et al., 2018).
2020 also resulted in an anomalously low HAB extent in WBLE, with a reduced severity relative to what was forecasted based on the observed levels of springtime discharge (National Oceanic and Atmospheric Administration [NOAA], 2020). While a negative anomaly was observed in a majority of windows, there was an extreme positive anomaly in late August before the bloom suddenly dissipated. Like the other observed anomalies, this trend seems to be well explained by variables not directly related to the COVID-19 shutdown. The upward trend in HAB extent coincided with a prolonged positive LST anomaly from June 29 through August 27, and the rapid bloom decline occurred simultaneously to the start of a negative LST anomaly which lasted from September 7 through the end of the typical HAB season. This decline also coincided with the aforementioned positive wind anomaly during the first weeks of September. Heavy winds force vertical mixing of the blooms which can reduce the amount of bloom visible to the satellite sensors and also limits the formation of highly concentrated surface scums until the winds calm (Wynne et al., 2010; Bosse et al., 2019; Sayers et al., 2019b). Elevated September winds also resulted in early ends to the HAB season in 2018 and 2019 (National Oceanic and Atmospheric Administration [NOAA], 2020). Prior years had seen HABs continue through October, often peaking in September (Bridgeman et al., 2013; Wynne and Stumpf, 2015; Sayers et al., 2019b).
Several other studies in Europe and the United States also found that observed environmental changes during the shutdown periods were just as well explained by long-term trends, meteorological factors, or other confounding factors (Ordóñez et al., 2020; Tobías et al., 2020; Zangari et al., 2020). In complex ecosystems like the Great Lakes, the water quality indicators that we examined are driven by a combination of natural factors (e.g., river discharge, LST, and wind speed, etc.) in addition to the anthropogenic drivers. This can result in significant spatial and temporal variability on yearly and even weekly time scales (Sayers et al., 2019a). Because of the observed variability in each of the ancillary factors studied, it is difficult to assign responsibility for any of the anomalies to changes in human behavior.
In addition to these ancillary variables, there are several other reasons why the shutdowns may not have resulted in observable water quality changes. For one, the regions being studied in this analysis are much larger than some of the other areas where changes were observed. Three studies looked at waters within approximately 3 km of shore (Aman et al., 2020; Braga et al., 2020; Yunus et al., 2020) and another went out to approximately 10 km (Cherif et al., 2020). These more targeted areas were also directly in the vicinity of key anthropogenic influences, including large cities and wastewater treatment plants. In contrast, the WBLE and SBLH study regions were an order of magnitude larger (approximately 3,200 and 2,700 km2, respectively). The waters studied in this analysis only extended to approximately 20 km offshore, but pixels on the eastern edges of SBLH and WBLE were up to 60–70 km away from what we would expect to be the primary drivers of change (major metropolitan areas and large river mouths). Additionally, the coarse resolution satellite used in this analysis limited the retrievals in nearshore waters where the anthropogenic influence would be magnified. VIIRS was used rather than higher resolution sensors such as the Landsat sensors or Sentinel-2 because the higher temporal resolution (1 day as compared to 5–16 days) is needed in the Great Lakes due to the frequent cloud cover, particularly in spring (Ackerman et al., 2013; Wynne et al., 2013). Sentinel-3 provides improved spatial resolution over VIIRS (300 vs 750 m) with a near-daily revisit, but this sensor has a limited historical record (launched in 2016) and the CPA-A has not yet been adequately calibrated or validated for this sensor.
Finally, the anthropogenic influence in WBLE and SBLH differs from that seen in some of the other regions where anomalies were identified. Several studies identified reduced industrial pollution and wastewater discharge as the drivers of water quality improvement (Aman et al., 2020; Cherif et al., 2020; Yunus et al., 2020) and boat traffic reductions in the normally busy Venetian Lagoon were cited as a primary cause of the observed water clarity increases (Braga et al., 2020). While motorized boat traffic was suspended in April as part of Michigan’s COVID-19 response (State of Michigan, 2020c), their impact on basin-wide water quality is likely negligible due to their reduced density relative to the Venice case. The changes in industrial pollution and wastewater discharge are also less relevant in the Great Lakes region due to the stringent controls placed on these systems as part of the GLWQA. This study hypothesized that the major drivers of change would be a transition from the highly effective municipal wastewater treatment systems to on-site septic systems and changes in the agricultural calendar. The heavier usage of septic systems may have resulted in increased nutrient loads to the basin, but this impact would likely be spread out across the basins (as opposed to the larger wastewater treatment plants which would have a single outflow point) and concentrated in the nearshore waters that were not resolvable in this analysis. And even though slight changes were observed in the agricultural planting calendar, the declaration of farmers as essential workers limited the impact of the shutdowns on this source of nutrient loading.
This analysis did not identify any water quality changes clearly attributable to the COVID-19 shutdowns, but it is possible that there were changes that were subtler than could be picked up by our algorithm. The CPA-A is parameterized on a per-lake basis using a generalized set of IOPs meant to capture the observed range of optical conditions in each lake. Sayers et al. (2019a) found issues with this approach in WBLE, as large shifts in the phytoplankton community can result in changes to the specific IOPs. The CPA-A should be able to effectively capture shutdown-driven changes in the magnitude of the color producing agents. However, if the changes in human activity resulted in significant shifts in the phytoplankton community, the optical properties could shift outside the range of our historical observations, introducing additional uncertainty into our results. While we do not have any in situ data from spring 2020 to validate our retrievals, comparisons against in situ data from mid-summer through early-fall 2020 generate confidence in the late-season CHL and PZD estimates.
Additionally, by focusing on short-term, immediate impacts of the shutdowns, this analysis ignores the contribution of legacy drivers of water quality. Prior research has shown that legacy nutrients in the soil within the watershed can contribute up to 49% of the annual total phosphorus load from the Maumee River (Kast et al., 2021) and phosphorus loadings from prior years also play a significant role in determining the size of phytoplankton blooms in WBLE (Ho and Michalak, 2017). This implies that the impacts of behavior changes due to the COVID-19 shutdowns may be muted by past activity and also that they could have impacts on water quality in the coming years. These water quality indicators, in particular the HAB extent in WBLE, should continue to be studied in subsequent years to assess whether there are any potential long-term or delayed impacts of the shutdowns.
Conclusion
The Visible Infrared Imaging Radiometer Suite satellite imagery from 2020 was compared to imagery from 2012 to 2019 to assess whether water quality in the Great Lakes’ eutrophic basins was impacted by the COVID-19 pandemic shutdowns. Indicator values in April 2020, when social distancing was at its peak, revealed significant changes in both SBLH (increased CHL, decreased TSS, increased PZD) and WBLE (decreased CHL). However, these shifts continued through the year even as shutdown restrictions eased. Comparisons to trends in other indicators (e.g., wind speed and river discharge) led to the conclusion that the observed water quality changes were more likely related to natural variability rather than being a result of the shutdowns. Future research will address some of the limitations of this analysis that may have masked some potential impacts related to the change in human activity. These include the addition of higher resolution sensors to assess nearshore water quality where significant impacts may be expected and the inclusion of data past 2020 to determine if the behavioral changes had any delayed or long-term impacts.
While this study found that there was likely no relationship between the COVID-19-induced shutdowns and short-term Great Lakes water quality, it has shown the utility of using the lengthy remote sensing data record to identify water quality anomalies. This approach has been used extensively in marine systems for identifying anomalies in sea surface temperature (Stock et al., 2015), phytoplankton blooms (Wang et al., 2021), sediment/turbidity (Dogliotti et al., 2016), and HAB presence (Stumpf et al., 2003; Tomlinson et al., 2009). However, there has been limited application of the method for water quality monitoring in the Great Lakes. Going forward, utilizing this approach as imagery becomes available will allow for the identification of water quality anomalies in near real time. This can provide a better understanding of the historical significance of any given sediment plume or phytoplankton bloom and guide in situ sampling efforts. It can also be expanded to the broader Great Lakes region, generating a more complete understanding of the current regional water quality conditions.
Data Availability Statement
The raw data supporting the conclusions of this article will be made available by the authors, without undue reservation.
Author Contributions
KB acquired, processed, and analyzed the data, and wrote the first draft of the manuscript. All authors contributed to the conception and design of the study, manuscript revision, read, and approved the submitted version.
Funding
This study was funded by NASA under contract # 80NSSC20P2097.
Conflict of Interest
The authors declare that the research was conducted in the absence of any commercial or financial relationships that could be construed as a potential conflict of interest.
Publisher’s Note
All claims expressed in this article are solely those of the authors and do not necessarily represent those of their affiliated organizations, or those of the publisher, the editors and the reviewers. Any product that may be evaluated in this article, or claim that may be made by its manufacturer, is not guaranteed or endorsed by the publisher.
Acknowledgments
We would like to thank Carol Tolbert and Leah Nakley for shepherding the project, Zane Almquist and Quelyn Bekkering for assistance with data acquisition and processing, and Laura Lorenzoni for inviting our team to be a part of the NASA COVID-19 dashboard.
Supplementary Material
The Supplementary Material for this article can be found online at: https://www.frontiersin.org/articles/10.3389/fmars.2021.673989/full#supplementary-material
Footnotes
- ^ https://covid19.who.int/region/amro/country/us
- ^ https://data.bls.gov/
- ^ https://data.cdc.gov/Case-Surveillance/United-States-COVID-19-Cases-and-Deaths-by-State-o/9mfq-cb36
- ^ https://oceancolor.gsfc.nasa.gov/
- ^ https://www.glerl.noaa.gov/res/HABs_and_Hypoxia/habsMon.html
- ^ https://www.nass.usda.gov/Statistics_by_State/Ohio/Publications/Crop_Progress_&_Condition/index.php
- ^ https://earthdata.nasa.gov/covid19/
References
Ackerman, S. A., Heidinger, A., Foster, M. J., and Maddux, B. (2013). Satellite regional cloud climatology over the Great Lakes. Remote Sens. 5, 6223–6240. doi: 10.3390/rs5126223
Aman, M. A., Salman, M. S., and Yunus, A. P. (2020). COVID-19 and its impact on environment: improved pollution levels during the lockdown period–A case from Ahmedabad, India. Remote Sens. Appl. 20:100382. doi: 10.1016/j.rsase.2020.100382
Behrenfeld, M. J., and Falkowski, P. G. (1997). Photosynthetic rates derived from satellite-based chlorophyll concentration. Limnol. Oceanogr. 42, 1–20. doi: 10.4319/lo.1997.42.1.0001
Bierman, V. J. Jr., and Dolan, D. M. (1981). Modeling of phytoplankton-nutrient dynamics in Saginaw Bay, Lake Huron. J. Great Lakes Res. 7, 409–439. doi: 10.1016/S0380-1330(81)72069-0
Binding, C. E., Greenberg, T. A., and Bukata, R. P. (2012). An analysis of MODIS-derived algal and mineral turbidity in Lake Erie. J. Great Lakes Res. 38, 107–116. doi: 10.1016/j.jglr.2011.12.003
Binding, C. E., Stumpf, R. P., Shuchman, R. A., and Sayers, M. J. (2020). “Advances in Remote Sensing of Great Lakes Algal Blooms,” in Contaminants of the Great Lakes, eds J. Crossman and C. Weisener (Cham: Springer), 217–232. doi: 10.1007/698_2020_589
Binding, C. E., Zastepa, A., and Zeng, C. (2019). The impact of phytoplankton community composition on optical properties and satellite observations of the 2017 western Lake Erie algal bloom. J. Great Lakes Res. 45, 573–586. doi: 10.1016/j.jglr.2018.11.015
Bischoff, L. (2020). Coronavirus timeline: a look at the orders changing life in Ohio. Dayton Daily News. Available online at: https://www.daytondailynews.com/news/local/timeline-coronavirus-prompts-orders-changing-everyday-life-ohio/gpnVSADPxZxMltlDVyqKEP/ (Accessed February 9, 2021)
Bootsma, H. A. (2018). Oceans, lakes, and inland seas: a virtual issue on the large lakes of the world. Limnol. Oceanogr. Bull. 27, 87–88. doi: 10.1002/lob.10230
Bosse, K. R., Sayers, M. J., Shuchman, R. A., Fahnenstiel, G. L., Ruberg, S. A., Fanslow, D. L., et al. (2019). Spatial-temporal variability of in situ cyanobacteria vertical structure in Western Lake Erie: implications for remote sensing observations. J. Great Lakes Res. 45, 480–489. doi: 10.1016/j.jglr.2019.02.003
Braga, F., Scarpa, G. M., Brando, V. E., Manfè, G., and Zaggia, L. (2020). COVID-19 lockdown measures reveal human impact on water transparency in the Venice Lagoon. Sci. Total Environ. 736:139612. doi: 10.1016/j.scitotenv.2020.139612
Bridgeman, T. B., Chaffin, J. D., and Filbrun, J. E. (2013). A novel method for tracking western Lake Erie Microcystis blooms, 2002–2011. J. Great Lakes Res. 39, 83–89. doi: 10.1016/j.jglr.2012.11.004
Budd, J. W., and Warrington, D. S. (2004). Satellite-based sediment and chlorophyll a estimates for Lake Superior. J. Great Lakes Res. 30, 459–466. doi: 10.1016/S0380-1330(04)70406-2
Bukata, R. P. (2005). Satellite monitoring of inland and coastal water quality: retrospection, introspection, future directions. Boca Raton: CRC Press.
Cassidy-Bushrow, A. E., Baseer, M., Kippen, K., Levin, A. M., Li, J., Loveless, I., et al. (2021). Social distancing during the COVID-19 pandemic: quantifying the practice in Michigan–a “hotspot state” early in the pandemic–using a volunteer-based online survey. BMC Public Health 21:245. doi: 10.1186/s12889-021-10287-w
U. S. Census Bureau, (2016). American Community Survey, 2016 ACS 5-Year Estimates, Table B01003. Available online at: https://data.census.gov/cedsci/ (Accessed December 10, 2020).
Cherif, E. K., Vodopivec, M., Mejjad, N., Esteves da Silva, J. C., Simonovič, S., and Boulaassal, H. (2020). COVID-19 pandemic consequences on coastal water quality using WST Sentinel-3 Data: case of Tangier, Morocco. Water 12:2638. doi: 10.3390/w12092638
Collivignarelli, M. C., Abbà, A., Bertanza, G., Pedrazzani, R., Ricciardi, P., and Miino, M. C. (2020). Lockdown for CoViD-2019 in Milan: what are the effects on air quality? Sci. Total Environ. 732:139280. doi: 10.1016/j.scitotenv.2020.139280
Cooperative Institute for Great Lakes Research [CIGLR], University of Michigan and NOAA Great Lakes Environmental Research Laboratory. (2019). Physical, chemical, and biological water quality monitoring data to support detection of Harmful Algal Blooms (HABs) in western Lake Erie, collected by the Great Lakes Environmental Research Laboratory and the Cooperative Institute for Great Lakes Research since 2012. Maryland: NOAA National Centers for Environmental Information. Dataset. doi: 10.25921/11da-3x54
Dogliotti, A. I., Ruddick, K., and Guerrero, R. (2016). Seasonal and inter-annual turbidity variability in the Río de la Plata from 15 years of MODIS: El Niño dilution effect. Estuar. Coast. Shelf Sci. 182, 27–39. doi: 10.1016/j.ecss.2016.09.013
Dolan, D. M. (1993). Point source loadings of phosphorus to Lake Erie: 1986–1990. J. Great Lakes Res. 19, 212–223. doi: 10.1016/S0380-1330(93)71212-5
European Space Agency [ESA]. (2018). Land Cover CCI Version 2.1.1. Available online at: https://cds.climate.copernicus.eu/cdsapp#!/dataset/satellite-land-cover (Accessed December 10, 2020).
European Space Agency [ESA]. (2017). Land Cover CCI Product User Guide Version 2. Tech. Rep. Available online at: maps.elie.ucl.ac.be/CCI/viewer/download/ESACCI-LC-Ph2-PUGv2_2.0.pdf (Accessed December 10, 2020).
Fahnenstiel, G. L., Millie, D. F., Dyble, J., Litaker, R. W., Tester, P. A., McCormick, M. J., et al. (2008). Microcystin concentrations and cell quotas in Saginaw Bay, Lake Huron. Aquat. Ecosyst. Health Manag. 11, 190–195. doi: 10.1080/14634980802092757
Fahnenstiel, G. L., Sayers, M. J., Shuchman, R. A., Yousef, F., and Pothoven, S. A. (2016). Lake-wide phytoplankton production and abundance in the Upper Great Lakes: 2010–2013. J. Great Lakes Res. 42, 619–629. doi: 10.1016/j.jglr.2016.02.004
French, C. (2020). How a spring rainstorm became a 500-year flood event in mid-Michigan. MLive. Available online at: https://www.mlive.com/news/saginaw-bay-city/2020/05/how-a-spring-rainstorm-became-a-500-year-flood-event-in-mid-michigan.html (Accessed January 15, 2021)
Garnier, R., Benetka, J. R., Kraemer, J., and Bansal, S. (2021). Socioeconomic Disparities in Social Distancing During the COVID-19 Pandemic in the United States: observational Study. J. Med. Int. Res. 23:e24591. doi: 10.2196/24591
He, C., Zhang, L., DeMarchi, C., and Croley, T. E. II (2014). Estimating point and non-point source nutrient loads in the Saginaw Bay watersheds. J. Great Lakes Res. 40, 11–17. doi: 10.1016/j.jglr.2014.01.013
Herdendorf, C. E. (1982). Large lakes of the world. J. Great Lakes Res. 8, 379–412. doi: 10.1016/S0380-1330(82)71982-3
Ho, J. C., and Michalak, A. M. (2017). Phytoplankton blooms in Lake Erie impacted by both long-term and springtime phosphorus loading. J. Great Lakes Res. 43, 221–228. doi: 10.1016/j.jglr.2017.04.001
Holshue, M. L., DeBolt, C., Lindquist, S., Lofy, K. H., Wiesman, J., Bruce, H., et al. (2020). First case of 2019 novel coronavirus in the United States. N. Engl. J. Med. 382, 929–936. doi: 10.1056/NEJMoa2001191
Hutchinson, D. (2020). Michigan stay-at-home order timeline: 70 days, 4 extensions, ever-changing restrictions. Click On Detroit. Available online at: https://www.clickondetroit.com/news/local/2020/06/02/michigan-stay-at-home-order-timeline-70-days-4-extensions-ever-changing-restrictions/ (Accessed February 9, 2021)
Jiang, L., Xia, M., Ludsin, S. A., Rutherford, E. S., Mason, D. M., Jarrin, J. M., et al. (2015). Biophysical modeling assessment of the drivers for plankton dynamics in dreissenid-colonized western Lake Erie. Ecol. Model. 308, 18–33. doi: 10.1016/j.ecolmodel.2015.04.004
Kanniah, K. D., Zaman, N. A. F. K., Kaskaoutis, D. G., and Latif, M. T. (2020). COVID-19’s impact on the atmospheric environment in the Southeast Asia region. Sci. Total Environ. 736:139658. doi: 10.1016/j.scitotenv.2020.139658
Kast, J. B., Apostel, A. M., Kalcic, M. M., Muenich, R. L., Dagnew, A., Long, C. M., et al. (2021). Source contribution to phosphorus loads from the Maumee River watershed to Lake Erie. J. Environ. Manag. 279:111803. doi: 10.1016/j.jenvman.2020.111803
Lee, Z., Du, K., Arnone, R., Liew, S., and Penta, B. (2005). Penetration of solar radiation in the upper ocean: a numerical model for oceanic and coastal waters. J. Geophys. Res. Oceans 110:13. doi: 10.1029/2004JC002780
Lee, Z., Shang, S., Du, K., and Wei, J. (2018). Resolving the long-standing puzzles about the observed Secchi depth relationships. Limnol. Oceanogr. 63, 2321–2336. doi: 10.1002/lno.10940
Lee, Z., Weidemann, A., Kindle, J., Arnone, R., Carder, K. L., and Davis, C. (2007). Euphotic zone depth: its derivation and implication to ocean-color remote sensing. J. Geophys. Res. Oceans 112:C03009. doi: 10.1029/2006JC003802
Lohrenz, S. E., Fahnenstiel, G. L., Millie, D. F., Schofield, O. M., Johengen, T., and Bergmann, T. (2004). Spring phytoplankton photosynthesis, growth, and primary production and relationships to a recurrent coastal sediment plume and river inputs in southeastern Lake Michigan. J. Geophys. Res. Oceans 109:C10S14. doi: 10.1029/2004JC002383
Makarewicz, J. C., and Bertram, P. (1991). Evidence for the restoration of the Lake Erie ecosystem. Bioscience 41, 216–223. doi: 10.2307/1311411
Manning, N. F., Wang, Y. C., Long, C. M., Bertani, I., Sayers, M. J., Bosse, K. R., et al. (2019). Extending the forecast model: predicting western Lake Erie harmful algal blooms at multiple spatial scales. J Great Lakes Res. 45, 587–595. doi: 10.1016/j.jglr.2019.03.004
Michalak, A. M., Anderson, E. J., Beletsky, D., Boland, S., Bosch, N. S., Bridgeman, T. B., et al. (2013). Record-setting algal bloom in Lake Erie caused by agricultural and meteorological trends consistent with expected future conditions. Proc. Natl. Acad. Sci. U. S. A. 110, 6448–6452. doi: 10.1073/pnas.1216006110
Michigan Office of the Great Lakes [OGL] (2016). Sustaining Michigan’s Water Heritage: A Strategy for the Next Generation. Available online at: https://www.michigan.gov/documents/deq/deq-ogl-waterstrategy_538161_7.pdf (Accessed March 14, 2021)
Millie, D. F., Fahnenstiel, G. L., Lohrenz, S. E., Carrick, H. J., Johengen, T. H., and Schofield, O. M. (2003). Physical-biological coupling in southern Lake Michigan: influence of episodic sediment resuspension on phytoplankton. Aquat. Ecol. 37, 393–408. doi: 10.1023/B:AECO.0000007046.48955.70
Minnett, P. J., Evans, R. H., Podestá, G. P., and Kilpatrick, K. A. (2014). “Sea-surface temperature from Suomi-NPP VIIRS: Algorithm development and uncertainty estimation,” in Proceedings of SPIE - - The International Society for Optical Engineering, Vol. 9111, (Bellingham, WA: SPIE), 91110C. doi: 10.1117/12.2053184
Mishra, D. R., Kumar, A., Muduli, P. R., Equeenuddin, S., Rastogi, G., Acharyya, T., et al. (2020). Decline in Phytoplankton Biomass along Indian Coastal Waters due to COVID-19 Lockdown. Remote Sens. 12:2584. doi: 10.3390/rs12162584
National Oceanic and Atmospheric Administration [NOAA]. (2020). NOAA Western Lake Erie Harmful Algal Bloom Seasonal Assessment. Available online at: https://cdn.coastalscience.noaa.gov/hab-data/bulletins/lake-erie/2020/finalAssessment_2020-09.pdf (Accessed January 30, 2021).
Niu, Q., Xia, M., Ludsin, S. A., Chu, P. Y., Mason, D. M., and Rutherford, E. S. (2018). High-turbidity events in Western Lake Erie during ice-free cycles: contributions of river-loaded vs. resuspended sediments. Limnol. Oceanogr. 63, 2545–2562. doi: 10.1002/lno.10959
Ohio Department of Health. (2020). Director’s Stay At Home Order [Press release]. Available online at: https://coronavirus.ohio.gov/static/publicorders/DirectorsOrderStayAtHome.pdf (Accessed February 9, 2021).
Ordóñez, C., Garrido-Perez, J. M., and García-Herrera, R. (2020). Early spring near-surface ozone in Europe during the COVID-19 shutdown: meteorological effects outweigh emission changes. Sci. Total Environ. 747:141322. doi: 10.1016/j.scitotenv.2020.141322
Paerl, H. W., and Huisman, J. (2008). Blooms like it hot. Science 320, 57–58. doi: 10.1126/science.1155398
Pelzer, J., and Hancock, L. (2020). Three Ohioans, All From Cuyahoga County, Have Coronavirus, Gov. Mike DeWine says. cleveland.com. Available online at: https://www.cleveland.com/healthfit/2020/03/three-ohioans-have-coronavirus-gov-mike-dewine-says.html (accessed August 12, 2021).
Public Sector Consultants [PSC]. (2018). An assessment of failing septic systems in the Saginaw Bay region. Lansing (MI): Public Sector Consultants. Available online at: https://publicsectorconsultants.com/an-assessment-of-failing-septic-systems-in-the-saginaw-bay-region/ (Accessed March 14, 2021).
Rao, Y. R., and Schwab, D. J. (2007). Transport and mixing between the coastal and offshore waters in the Great Lakes: a review. J. Great Lakes Res. 33, 202–218.
Saulquin, B., Hamdi, A., Gohin, F., Populus, J., Mangin, A., and d’Andon, O. F. (2013). Estimation of the diffuse attenuation coefficient KdPAR using MERIS and application to seabed habitat mapping. Remote Sens. Environ. 128, 224–233. doi: 10.1016/j.rse.2012.10.002
Sayers, M., Fahnenstiel, G. L., Shuchman, R. A., and Whitley, M. (2016). Cyanobacteria blooms in three eutrophic basins of the Great Lakes: a comparative analysis using satellite remote sensing. Int. J. Remote Sens. 37, 4148–4171. doi: 10.1080/01431161.2016.1207265
Sayers, M. J., Bosse, K. R., Shuchman, R. A., Ruberg, S. A., Fahnenstiel, G. L., Leshkevich, G. A., et al. (2019a). Spatial and temporal variability of inherent and apparent optical properties in western Lake Erie: implications for water quality remote sensing. J. Great Lakes Res. 45, 490–507. doi: 10.1016/j.jglr.2019.03.011
Sayers, M. J., Grimm, A. G., Shuchman, R. A., Bosse, K. R., Fahnenstiel, G. L., Ruberg, S. A., et al. (2019b). Satellite monitoring of harmful algal blooms in the Western Basin of Lake Erie: a 20-year time-series. J. Great Lakes Res. 45, 508–521. doi: 10.1016/j.jglr.2019.01.005
Scavia, D., Allan, J. D., Arend, K. K., Bartell, S., Beletsky, D., Bosch, N. S., et al. (2014). Assessing and addressing the re-eutrophication of Lake Erie: central basin hypoxia. J. Great Lakes Res. 40, 226–246. doi: 10.1016/j.jglr.2014.02.004
Selzer, M. D., Joldersma, B., and Beard, J. (2014). A reflection on restoration progress in the Saginaw Bay watershed. J. Great Lakes Res. 40, 192–200. doi: 10.1016/j.jglr.2013.11.008
Shuchman, R. A., Bosse, K. R., Sayers, M. J., Fahnenstiel, G. L., and Leshkevich, G. (2017). Satellite Observed Water Quality Changes In The Laurentian Great Lakes Due To Invasive Species, Anthropogenic Forcing, And Climate Change. Int. Arch. Photogramm. Remote Sens. Spatial Inf. Sci. XLII-3, 189–195. doi: 10.5194/isprs-archives-XLII-3-W2-189-2017
Shuchman, R. A., Leshkevich, G., Sayers, M. J., Johengen, T. H., Brooks, C. N., and Pozdnyakov, D. (2013). An algorithm to retrieve chlorophyll, dissolved organic carbon, and suspended minerals from Great Lakes satellite data. J. Great Lakes Res. 39, 14–33. doi: 10.1016/j.jglr.2013.06.017
Son, S., and Wang, M. (2020). Water quality properties derived from VIIRS measurements in the Great Lakes. Remote Sens. 12:1605. doi: 10.3390/rs12101605
Soni, P. (2021). Effects of COVID-19 lockdown phases in India: an atmospheric perspective. Environ. Dev. Sustain. 1–12. doi: 10.1007/s10668-020-01156-4 [Online ahead of print]
State of Michigan. (2020a). Michigan announces first presumptive positive cases of COVID-19 [Press release]. Available online at: https://www.michigan.gov/coronavirus/0,9753,7-406-98158-521365–,00.html (Accessed February 9, 2021)
State of Michigan. (2020b). Governor Whitmer Signs “Stay Home, Stay Safe” Executive Order [Press release]. Available online at: https://www.michigan.gov/whitmer/0,9309,7-387-90499_90640-522625–,00.html (Accessed February 9, 2021)
State of Michigan. (2020c). Governor Whitmer Extends, Expands “Stay Home, Stay Safe” Executive Order to Save Lives [Press release]. Available online at: https://www.michigan.gov/whitmer/0,9309,7-387-90499_90640-525173–,00.html (Accessed April 10, 2021)
Statistics Canada (2019). Census Profile for Census Subdivisions in Ontario, 2016 Census, Catalogue no. 98-401-X2016066. Available online at: https://www12.statcan.gc.ca/census-recensement/2016/dp-pd/index-eng.cfm (Accessed December 10, 2020).
Stock, C. A., Pegion, K., Vecchi, G. A., Alexander, M. A., Tommasi, D., Bond, N. A., et al. (2015). Seasonal sea surface temperature anomaly prediction for coastal ecosystems. Prog. Oceanogr. 137, 219–236. doi: 10.1016/j.pocean.2015.06.007
Stow, C. A., Cha, Y., Johnson, L. T., Confesor, R., and Richards, R. P. (2015). Long-term and seasonal trend decomposition of Maumee River nutrient inputs to western Lake Erie. Environ. Sci. Technol. 49, 3392–3400. doi: 10.1021/es5062648
Stow, C. A., Dyble, J., Kashian, D. R., Johengen, T. H., Winslow, K. P., Peacor, S. D., et al. (2014). Phosphorus targets and eutrophication objectives in Saginaw Bay: a 35 year assessment. J. Great Lakes Res. 40, 4–10. doi: 10.1016/j.jglr.2013.10.003
Stumpf, R. P., Culver, M. E., Tester, P. A., Tomlinson, M. C., Kirkpatrick, G. J., Pederson, B., et al. (2003). Monitoring Karenia brevis blooms in the Gulf of Mexico using satellite ocean color imagery and other data. Harmful Algae 2, 147–160. doi: 10.1016/S1568-9883(02)00083-5
Stumpf, R. P., Wynne, T. T., Baker, D. B., and Fahnenstiel, G. L. (2012). Interannual variability of cyanobacterial blooms in Lake Erie. PLoS One 7:e42444. doi: 10.1371/journal.pone.0042444
Tobías, A., Carnerero, C., Reche, C., Massagué, J., Via, M., Minguillón, M. C., et al. (2020). Changes in air quality during the lockdown in Barcelona (Spain) one month into the SARS-CoV-2 epidemic. Sci. Total Environ. 726:138540. doi: 10.1016/j.scitotenv.2020.138540
Tomlinson, M. C., Wynne, T. T., and Stumpf, R. P. (2009). An evaluation of remote sensing techniques for enhanced detection of the toxic dinoflagellate, Karenia brevis. Remote Sens. Environ. 113, 598–609. doi: 10.1016/j.rse.2008.11.003
Wang, M., Jiang, L., Mikelsons, K., and Liu, X. (2021). Satellite-derived global chlorophyll-a anomaly products. Int. J. Appl. Earth Obs. Geoinf. 97:102288. doi: 10.1016/j.jag.2020.102288
Warner, D. M., and Lesht, B. M. (2015). Relative importance of phosphorus, invasive mussels and climate for patterns in chlorophyll a and primary production in Lakes Michigan and Huron. Freshw. Biol. 60, 1029–1043. doi: 10.1111/fwb.12569
Watson, S. B., Miller, C., Arhonditsis, G., Boyer, G. L., Carmichael, W., Charlton, M. N., et al. (2016). The re-eutrophication of Lake Erie: harmful algal blooms and hypoxia. Harmful Algae 56, 44–66. doi: 10.1016/j.hal.2016.04.010
Wilcoxon, F. (1945). Individual Comparisons by Ranking Methods. Biometrics Bull. 1, 80–83. doi: 10.2307/3001968
World Health Organization [WHO]. (2020a). Coronavirus disease 2019 (COVID-19): Situation report, 1. Available online at: https://www.who.int/docs/default-source/coronaviruse/situation-reports/20200121-sitrep-1-2019-ncov.pdf?sfvrsn=20a99c10_4 (Accessed February 9, 2021)
World Health Organization [WHO]. (2020b). Coronavirus disease 2019 (COVID-19): Situation report, 11. Available online at: https://www.who.int/docs/default-source/coronaviruse/situation-reports/20200131-sitrep-11-ncov.pdf?sfvrsn=de7c0f7_4 (Accessed February 9, 2021)
Wynne, T. T., and Stumpf, R. P. (2015). Spatial and temporal patterns in the seasonal distribution of toxic cyanobacteria in western Lake Erie from 2002–2014. Toxins 7, 1649–1663. doi: 10.3390/toxins7051649
Wynne, T. T., Stumpf, R. P., and Briggs, T. O. (2013). Comparing MODIS and MERIS spectral shapes for cyanobacterial bloom detection. Int. J. Remote Sens. 34, 6668–6678. doi: 10.1080/01431161.2013.804228
Wynne, T. T., Stumpf, R. P., Litaker, R. W., and Hood, R. R. (2021). Cyanobacterial bloom phenology in Saginaw Bay from MODIS and a comparative look with western Lake Erie. Harmful Algae 103:101999. doi: 10.1016/j.hal.2021.101999
Wynne, T. T., Stumpf, R. P., Tomlinson, M. C., and Dyble, J. (2010). Characterizing a cyanobacterial bloom in western Lake Erie using satellite imagery and meteorological data. Limnol. Oceanogr. 55, 2025–2036. doi: 10.4319/lo.2010.55.5.2025
Yunus, A. P., Masago, Y., and Hijioka, Y. (2020). COVID-19 and surface water quality: improved lake water quality during the lockdown. Sci. Total Environ. 731:139012. doi: 10.1016/j.scitotenv.2020.139012
Zangari, S., Hill, D. T., Charette, A. T., and Mirowsky, J. E. (2020). Air quality changes in New York City during the COVID-19 pandemic. Sci. Total Environ. 742:140496. doi: 10.1016/j.scitotenv.2020.140496
Keywords: coronavirus – COVID-19, water quality, Great Lakes, anomaly, remote sensing
Citation: Bosse KR, Sayers MJ, Shuchman RA, Lekki J and Tokars R (2021) Measuring the Impact of the COVID-19 Shutdown on Great Lakes Water Quality Using Remote Sensing. Front. Mar. Sci. 8:673989. doi: 10.3389/fmars.2021.673989
Received: 28 February 2021; Accepted: 19 July 2021;
Published: 24 August 2021.
Edited by:
Deepak R. Mishra, University of Georgia, United StatesReviewed by:
Junyu He, Zhejiang University, ChinaD. Swain, Indian Institute of Technology Bhubaneswar, India
Copyright © 2021 Bosse, Sayers, Shuchman, Lekki and Tokars. This is an open-access article distributed under the terms of the Creative Commons Attribution License (CC BY). The use, distribution or reproduction in other forums is permitted, provided the original author(s) and the copyright owner(s) are credited and that the original publication in this journal is cited, in accordance with accepted academic practice. No use, distribution or reproduction is permitted which does not comply with these terms.
*Correspondence: Karl R. Bosse, a3Jib3NzZUBtdHUuZWR1