- 1German Aerospace Center (DLR), Remote Sensing Technology Institute, Berlin, Germany
- 2Foundation for Research and Technology - Hellas (FORTH), Institute of Applied and Computational Mathematics, Heraklion, Greece
- 3Marine Explorers Society - 20000 Leagues, Zadar, Croatia
- 4Andromède Océanologie, Mauguio, France
- 5MARBEC, Univ Montpellier, CNRS, IFREMER, IRD, labcom InToSea, Montpellier, France
- 6Institute of Marine Biology, University of Montenegro, Kotor, Montenegro
- 7Department of Earth, Environment and Life Sciences (DISTAV), University of Genoa, Genoa, Italy
- 8EqEL – UMR CNRS 6134 SPE, University of Corsica Pascal Paoli, Corte, France
- 9Bodega Marine Laboratory-University of California, Davis, Bodega Bay, CA, United States
- 10Bigelow Laboratory for Ocean Sciences, East Boothbay, ME, United States
- 11German Aerospace Center (DLR), Earth Observation Center (EOC), Weßling, Germany
The seagrass Posidonia oceanica is the main habitat-forming species of the coastal Mediterranean, providing millennial-scale ecosystem services including habitat provisioning, biodiversity maintenance, food security, coastal protection, and carbon sequestration. Meadows of this endemic seagrass species represent the largest carbon storage among seagrasses around the world, largely contributing to global blue carbon stocks. Yet, the slow growth of this temperate species and the extreme projected temperature and sea-level rise due to climate change increase the risk of reduction and loss of these services. Currently, there are knowledge gaps in its basin-wide spatially explicit extent and relevant accounting, therefore accurate and efficient mapping of its distribution and trajectories of change is needed. Here, we leveraged contemporary advances in Earth Observation—cloud computing, open satellite data, and machine learning—with field observations through a cloud-native geoprocessing framework to account the spatially explicit ecosystem extent of P. oceanica seagrass across its full bioregional scale. Employing 279,186 Sentinel-2 satellite images between 2015 and 2019, and a human-labeled training dataset of 62,928 pixels, we mapped 19,020 km2 of P. oceanica meadows up to 25 m of depth in 22 Mediterranean countries, across a total seabed area of 56,783 km2. Using 2,480 independent, field-based points, we observe an overall accuracy of 72%. We include and discuss global and region-specific seagrass blue carbon stocks using our bioregional seagrass extent estimate. As reference data collections, remote sensing technology and biophysical modelling improve and coalesce, such spatial ecosystem extent accounts could further support physical and monetary accounting of seagrass condition and ecosystem services, like blue carbon and coastal biodiversity. We envisage that effective policy uptake of these holistic seagrass accounts in national climate strategies and financing could accelerate transparent natural climate solutions and coastal resilience, far beyond the physical location of seagrass beds.
Introduction
The Mediterranean coastal seascape hosts 18% of all known marine species, 31% of the global tourism, and 2% of the global population across 48,300 km of coastline (Coll et al., 2010; UNEP/MAP, 2012). Seagrasses—marine flowering plants forming submerged meadows up to 40 m deep—and especially the endemic and most common species in the basin Posidonia oceanica—have been the bioengineers of this structurally complex seascape framework for millennia (Arnaud-Haond et al., 2012). Covering less than 2% of the total Mediterranean seabed, the P. oceanica seagrass meadows provide a plethora of ecosystem services valued between 57,000 to 184,000 €/ha/year (Paoli et al., 2018; Rigo et al., 2021) and are globally significant carbon sinks, with greater organic carbon density than the observed one in estuarine mangroves, peatlands or tropical forests (IUCN, 2021). The large efficiency of such vegetated coastal ecosystems in absorbing and storing carbon, and thus reducing atmospheric carbon dioxide concentration, has led to initiatives to include them in climate change mitigation strategies like REDD+ (Reducing Emissions from Deforestation and Forest Degradation) (Duarte et al., 2013). Such initiatives are further supported by the observation that P. oceanica seagrass meadows could have sequestered up to 42% of the carbon emitted by all Mediterranean countries since the onset of the Industrial Revolution (Pergent et al., 2014).
Due to numerous anthropogenic impacts, including coastal development, eutrophication, anchoring, and illegal fishing, the Mediterranean seascape has experienced a net loss of 6,990 ha in the coverage of P. oceanica meadows between 1869 and 2016, with, however, a reversed decline trend since the 1990s (de los Santos et al., 2019). A regional climate warming of 1.5°C above pre-industrial levels coupled with P. oceanica’s slow growth (1 cm yr -1) (Marbà and Duarte, 1998) would accelerate its loss (Jordà et al., 2012) with associated risks to biodiversity, food security, livelihoods, tourism, and, ultimately, coastal protection. The observed decline of the P. oceanica meadows and the lack of suitable spatially explicit monitoring necessitate accurate and continuous mapping and monitoring of their extent, trajectory of change, and condition. Such monitoring efforts can enable a better understanding and detection of hotspots of sensitivity and resilience not only for effective management and protection but also for climate change mitigation and adaptation strategies across the basin.
In the past five years, advances in Earth Observation technology—high spatial and temporal satellite data archives—as well as cloud computing power and artificial intelligence (AI) have enabled data-driven measurements, monitoring, and change detection in the distribution, trends, and health of the coastal environment, from a regional to global scale (Bunting et al., 2018; Murray et al., 2019; Purkis et al., 2019; Lyons et al., 2020). Such large-scale Earth Observation efforts require consistent, accurate, and well-distributed reference data of extensive magnitude in space and time to calibrate and validate their mapping products. These mapping products could enable seascape management and conservation at national and global scales, and climate change mitigation schemes like the Nationally Determined Contributions (United Nations Framework Convention on Climate Change, 2021); and could support the efficacy of the Sustainable Development Goals relevant to the coastal marine environment—namely Goal 6, 13 and 14—by 2030 (United Nations, 2015).
In this study, we have expanded an end-to-end cloud-native—built and run entirely within a cloud computing environment—Earth Observation algorithmic framework (Traganos et al., 2018) using the cloud geospatial platform of the Google Earth Engine (GEE) (Gorelick et al., 2017). We utilized this algorithmic framework to map, for the first time, the P. oceanica seagrass ecosystem extent at its whole bioregion scale of 56,783 km2 that includes 22 countries. The GEE cloud geospatial platform enabled the storage, processing, and analysis of a mosaic of 279,186 open satellite Sentinel-2 images (Gascon et al., 2017) between 2015 and 2019, the mosaic classification via the cloud-based machine learning (ML) algorithm of the Random Forests (RF) (Gislason et al., 2006), and the development of an inventory of human-labeled training data to guide the ML-based classification. For the latter, we annotated 1,748 training blocks consisting of 62,928 100-m2 pixels indicative of the different Mediterranean benthic classes i.e., seagrass meadows, rocky reefs and sandy bottom, and optically deep water. Optically deep waters are regions where the remote sensing signal does not provide any bottom information due to the light attenuation from the water column. To validate the mapping results, we collected and utilized an independent (both spatially and source-wise) inventory of existing field-based data (2,480 points).
Methods
The Cloud-Native Earth Observation Framework
As part of this study, we improved a recent cloud-native algorithmic framework (Traganos et al., 2018). This framework was built upon the advances in Earth Observation technology in terms of open petabyte-scale satellite datasets, high-performance cloud computational power, supervised machine learning, web-based visualization capabilities, and human-guided design of large-scale training data suitable for the supervision of the ML component—all of which are powered by the cloud infrastructure of the GEE.
The cloud-native existence of the evolved Earth Observation framework (Figure 1) allowed a time- and cost-efficient scalability in three dimensions: space (e.g., region, country, basin), time (e.g., monthly, seasonal, annual, multiannual), and satellite data input. The time dimension here encompassed multi-temporal analytics based on the combination of metadata selection and statistical analysis of all available satellite images within a given period. The result of multi-temporal analytics is a pseudo-image whose pixels contain the least amount of clouds, aerosols, waves, and reflection from the sea surface—all of the above being common natural obstacles within satellite scenes over coastal waters.
The Multi-Temporal Sentinel-2 Satellite Image Mosaic
The aforementioned three components were adjusted according to our mapping task. To map the basin-scale seagrass distribution of P. oceanica, we scaled up the framework (Traganos et al., 2018) throughout the first 25 m of the depth of the Mediterranean, a total of 56,783 km2. We identified this depth range utilizing the EMODnet Bathymetry Digital Terrain Model (DTM) for the European Seas of 2018 (https://www.emodnet.eu/new-high-resolution-digital-terrain-model), which is a composite of bathymetric datasets from different sources at ~115m x 115 m resolution. We empirically selected the 25 m cut off depth as the most representative deep limit of seabed detection of the Sentinel-2 satellite—guided by water quality and human expert knowledge—in both the western and eastern basin part of the Mediterranean (Poursanidis et al., 2019) (Supplementary Table S2; Figures S3−S8). This depth limit is a balance between removing as many optically deep water pixels as possible without accidentally misidentifying and removing seagrass pixels, since both classes have similar spectral values. A deeper limit would include many more optically deep pixels, which could lead to potential false positives of seagrass presence; while a shallower limit would conversely mask many potential true positives of P. oceanica seagrass regions, especially within the optically shallower eastern basin.
Additionally, we manually masked extensive regions of turbid waters (mainly in north Italy, Egypt, Syria, and southeast Turkey), which would obscure the seabed detection. Finally, pixels from 279,186 satellite image tiles of Sentinel-2—100x100 km2 images—acquired between 23 June 2015 and 31 December 2019 synthesized the multi-annual mosaic at 10 m resolution—a full-archive synthesis of Sentinel-2 images over the Mediterranean at the time of its creation. The multi-temporal synthesis was essentially a statistical reduction of all land, cloud and deep-water-filtered images to the 25th percentile per pixel, a reduction deemed necessary to automatically filter out natural interferences like remaining clouds, haze, waves, sunglint and similar. As these Sentinel-2 images are top-of-the-atmosphere satellite data, we also employed the modified dark pixel subtraction method (Traganos et al., 2018) to account for the atmospheric effect in these data.
Training Data
Before describing the artificial intelligence component in the heart of our cloud-native framework, it is important to describe our training data design—essentially, the type of data that guides the AI. The training data were labeled following human-guided photointerpretation of the Sentinel-2 image mosaic by an expert on both Earth Observation image analysis and the nature of the Mediterranean coastal seascape. The expert spent 100 working hours within the cloud geospatial environment to annotate polygons indicative of the presence of three habitat classes: a) seagrasses (P. oceanica), b) optically deep water, and c) rocky and sandy seabed (Table 1). We decided to design polygons on P. oceanica seagrass meadows and not on other Mediterranean seagrass species (e.g., C. nodosa, Z. marina) as the sparse natural distribution of the latter species would have caused confusion on the 10 m spatial resolution of Sentinel-2. Therefore, it is expected that the spectral similarities between P. oceanica and other seagrass beds may have caused over-estimations and misclassification of the former class in our mapping effort. The human photointerpreter designed the training data by choosing polygons from all depths (shallow to deep), distribution (eastern and western basin), and density gradient (sparse to dense) of the studied habitats.
Sequentially, the final training dataset consisted of 1,748 polygons which were first reduced to centroids (Figure 2) and then were grown to blocks of a 30-m circular radius buffer around the centroids i.e., ~36 Sentinel-2-pixel blocks of 3,600 m2 each (Table 1). This means that we allocated 1.1 training point for each square kilometer of the mapped seabed, on average (62,928 training pixels for all 56,783 km2 of seabed area). This ensured a robust plethora of training blocks of pure pixels for each class.
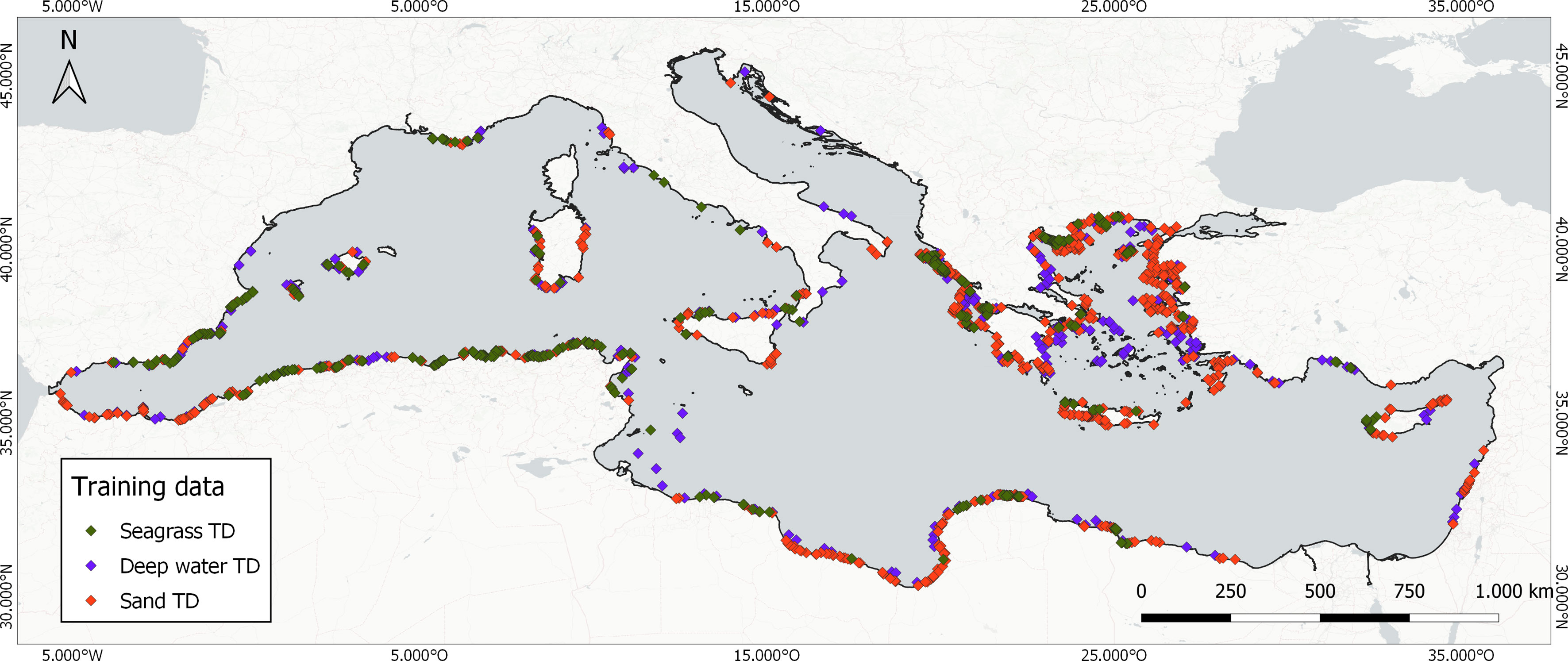
Figure 2 Distribution of the centroids of the 1,748 image-annotated training data blocks indicative of the presence of the three mapped classes.

Table 1 Name and definition of the human-labeled classes along with the number of designed training pixel blocks and points.
Artificial Intelligence
We employed the training data to guide the Random Forests artificial intelligence framework (Breiman, 2001) and classify the multi-annual Sentinel-2 mosaic at 10 m resolution. RF is an ensemble supervised machine learning classification algorithm that incorporates numerous self-learning decision trees that can handle both collinearity and non-linearity between predictor variables (e.g., the often non-linear border of P.oceanica seagrass meadows with the unconsolidated fine sediments). The rationale behind choosing RF was two-fold: a) their robustness against overtraining and noisy data (Gislason et al., 2006) that could still arise from our training data design; and b) their high accuracies in multi-scale coastal habitat mapping in local and serverless environments (Traganos and Reinartz, 2018a; Traganos and Reinartz, 2018b; Poursanidis et al., 2019; Lyons et al., 2020).
We parameterized and ran the RF within the GEE platform using the probability mode. This essentially creates an intermediate soft probability of presence or soft classification of each class varying between 0 and 100. This means that each pixel in this intermediate continuous layer represent probability of presence between 0 and 100%. We ran a series of quantitative and qualitative analyses to determine the best threshold value for the presence of seagrasses in both the western and eastern part of the basin. Then, we merged all classes into one hard probability habitat map containing all pixels—the hard classification. In contrast to the continuous soft probability layer, the hard probability/classification layer is a thematic product with each pixel representing a different class. This allowed us to delve into our classification experiments, further decreasing a possible over-estimation resulting from potential noise in the training data. Based on our analyses, the ideal threshold values for the soft-to-hard transformation were 45 in the West Mediterranean and 77 in the East. Figure 3 displays the spectral ranges of the training and validation data in both Western and Eastern Mediterranean across our utilized Sentinel-2 bands.
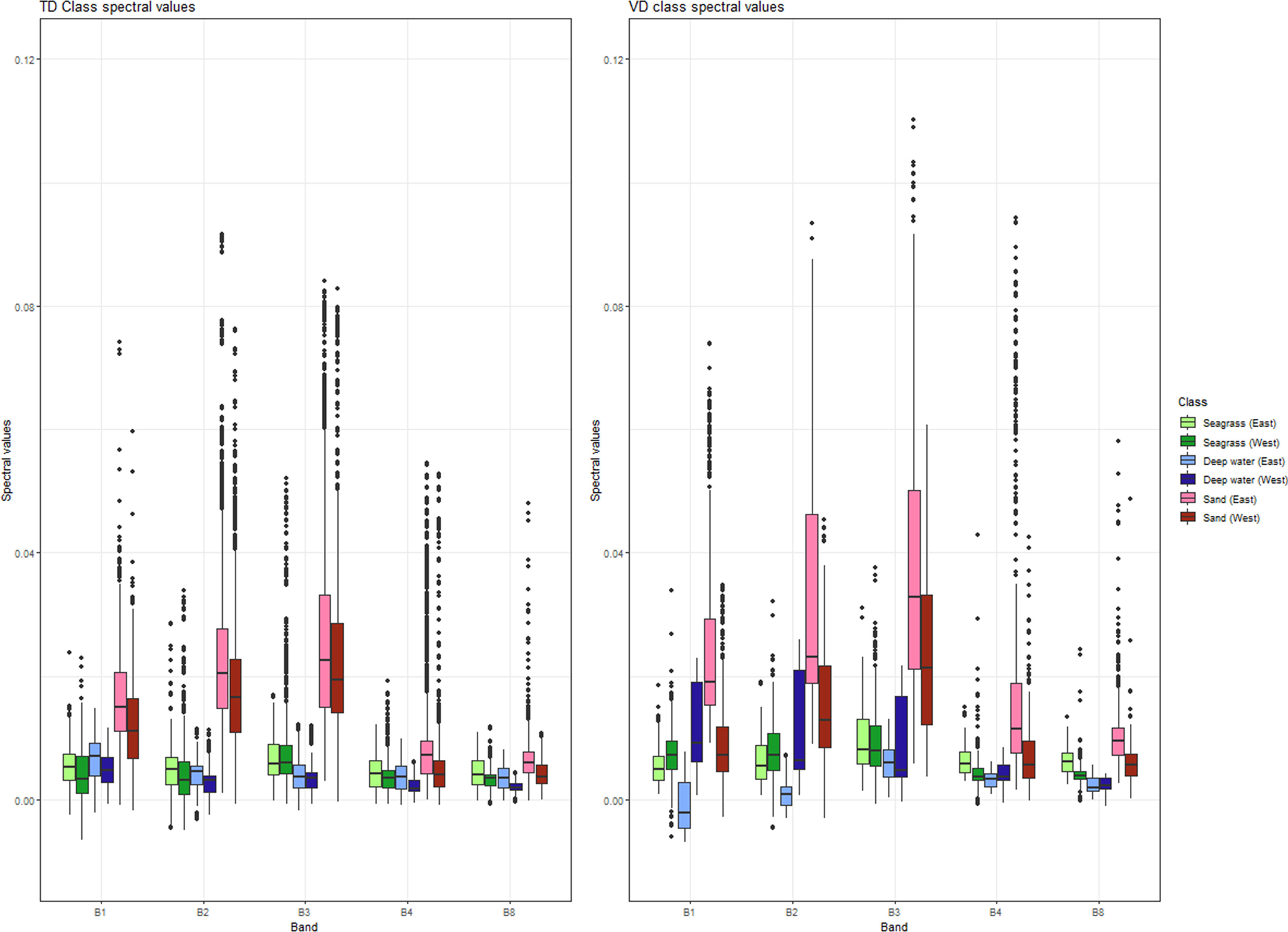
Figure 3 Spectral ranges of the training and validation data in both eastern and western Mediterranean across the B1-B4, and B8 bands of our multi-temporal Sentinel-2 data mosaic. Bright and dark hues of each color code reflect eastern and western reference data and green, blue, and red hues reflect the seagrass, deep water, and sand classes respectively. The split into eastern and western regions was in consideration of their dissimilar spectral reflectances (Supplementary Figure S8). This is more pronounced in the validation data where many of the interquartile ranges (the boxes of the boxplots) within each class in all the bands have either a small or no overlap. The lines within the boxes indicate the median.
Validation Data and Accuracy Assessment
To assess the accuracy of our bioregional map, we synthesized existing independent field-based validation data of P. oceanica seagrass meadows and neighboring habitats. During this synthesis, we collected high-resolution seagrass habitat maps developed previously in the Mediterranean by trained experts and scientists in the seagrass domain resulting in in situ data of total coverage of 3,274 km2. Table 2 indicates information on the coverage, temporal range, and source of the reference data. In addition to approaching our annotated data with as much independence as possible to reduce possible bias, the reference data featured higher quality and finer spatial resolution (Finegold et al., 2016) following field collection by trained experts via snorkeling, diving, and/or with the use of other technical equipment.
An independent analyst (not being the same person who annotated the training data) randomly selected data for the three habitat classes from the total amount of training data (3.8% on average), which resulted in 680 points for the seagrass class, 350 points for the optically deep water class, and 1,450 points for the sandy and rocky class (Figure 4). We decided to have a merged sandy and rocky class as the latter class was poorly represented across the entire basin based on both its natural distribution and the validation data availability and thus would have caused a rather biased representation in the confusion matrix and classification. The design was performed on a local GIS environment using three sources of data for guidance: a) the outline of the EMODnet DTM 0-25 m extent to ensure that all validation points fall within these limits; b) the higher spatial resolution satellite base maps of Google Earth Pro and the BING imagery platform independently from the Sentinel-2 data; and c) a hot-spot map based on the training data to ensure spatial independency between the latter and the validation data to further reduce potential spatial bias.
To assess the accuracy of our habitat mapping approach, we employed standard quantitative metrics in Earth Observation analysis: the Overall Accuracy—the proportion of area that is classified correctly; the Producer’s Accuracy—informing whether map classes were under-estimations; and the User’s Accuracy—reflecting whether the classified map is overestimated. We reported these metrics through the cross-tabulation of the labeled classes populated by the classification results and the reference data; the so-called “error matrix” (Supplementary Table S1).
Results
The Pan-Mediterranean Extent of P. oceanica Seagrass Meadows
We estimated a basin-wide seagrass area of 19,020 km2 between 0 and 25 m of depth in 22 countries with 72% overall accuracy—4,325 km2 in the Eastern basin (overall accuracy of 79%) and 14,694 km2 in the Western basin (overall accuracy of 64%). The producer’s and user’s accuracies of our seagrass product are 55% and 62% respectively (Supplementary Table S1). Our coverage estimate at the basin scale is 55.3% larger than the seagrass area synthesis in Telesca et al. (2015) (12,247 km2), 34.2% larger than the higher-confidence area synthesis of McKenzie et al. (2020) (14,167 km2), and around 1/6 of the MaxEnt-based modeled estimate of Jayathilake and Costello (2018) (118,913 km2). Figure 5 shows the bioregional extent of P. oceanica seagrass meadows across the entire Mediterranean.
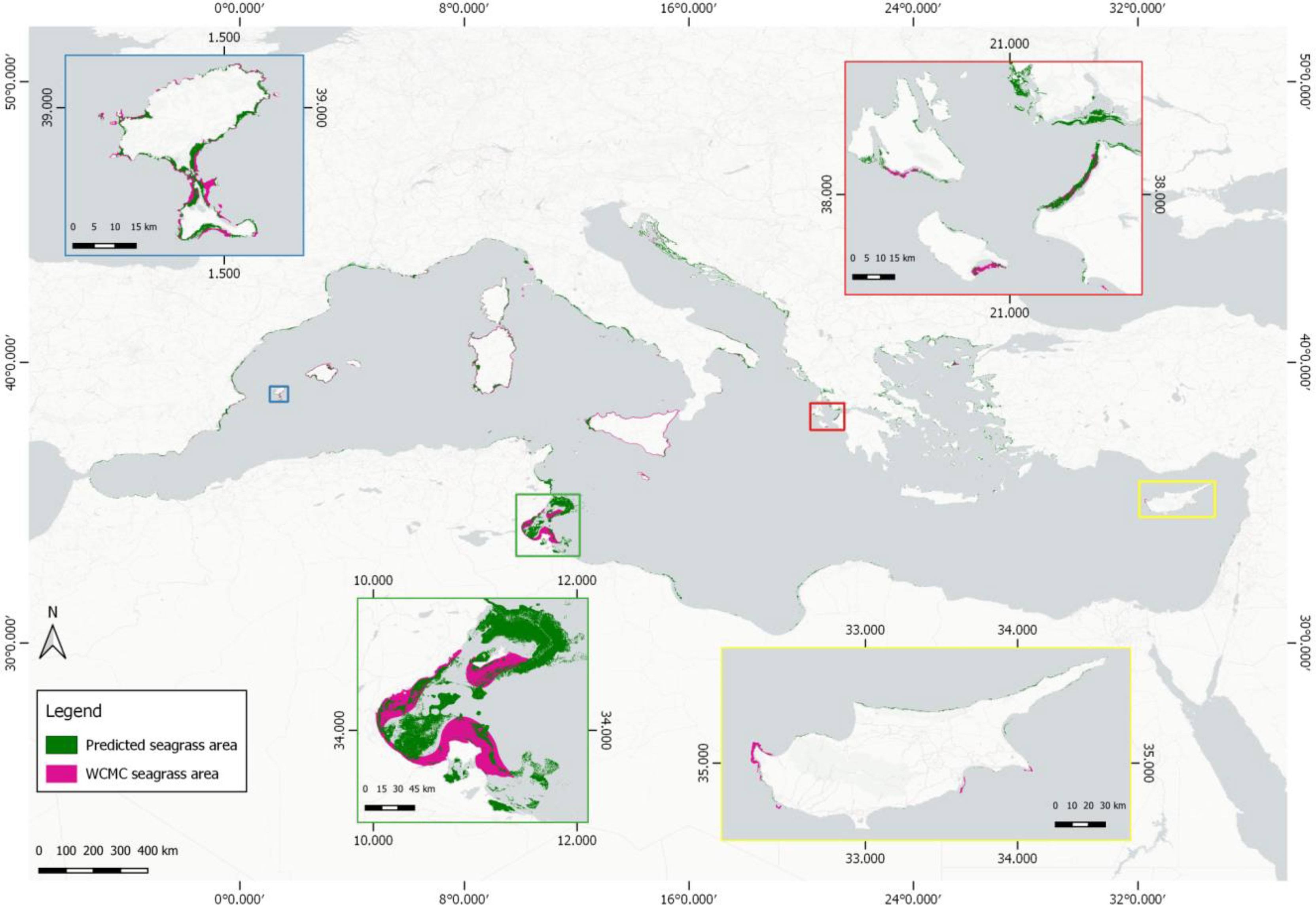
Figure 5 Pan-Mediterranean P. oceanica seagrass extent (this paper: green, pink (Telesca et al., 2015)). The four inset panels depict the two estimates of mapped seagrass extent in Ibiza and Formentera, Spain (blue inset), Gulf of Gabes, Tunisia (green inset), NW Peloponnese and South Ionian Sea, Greece (red inset), and the country scale of Cyprus (yellow inset).
Country-Scale P. oceanica Seagrass Area Inventories
Table 3 depicts the country-scale P. oceanica seagrass extent estimates across 22 Mediterranean countries between 0 and 25 m of depth, based on the spatial resolution of 10 m of Sentinel-2 data. The three countries with the largest P. oceanica seagrass meadows were Tunisia (6,369 km2), Italy (3,261 km2), and Greece (2,878 km2) (Table 3). The three countries with the largest seagrasses by km of Mediterranean coastline were Tunisia (3.4 km2/km), Montenegro (0.8 km2/km), and Croatia (0.5 km2/km). The 13 European countries with a Mediterranean coastline counted a total P. oceanica seagrass area of 10,932 km2 (57.5% of the total seagrass bioregional area). The five African countries have an estimated total seagrass area of 7,310 km2 (38.4% of the total bioregional seagrass area) and the five Asian countries a total seagrass extent of 778 km2 (4.1% of the pan-Mediterranean seagrass extent).
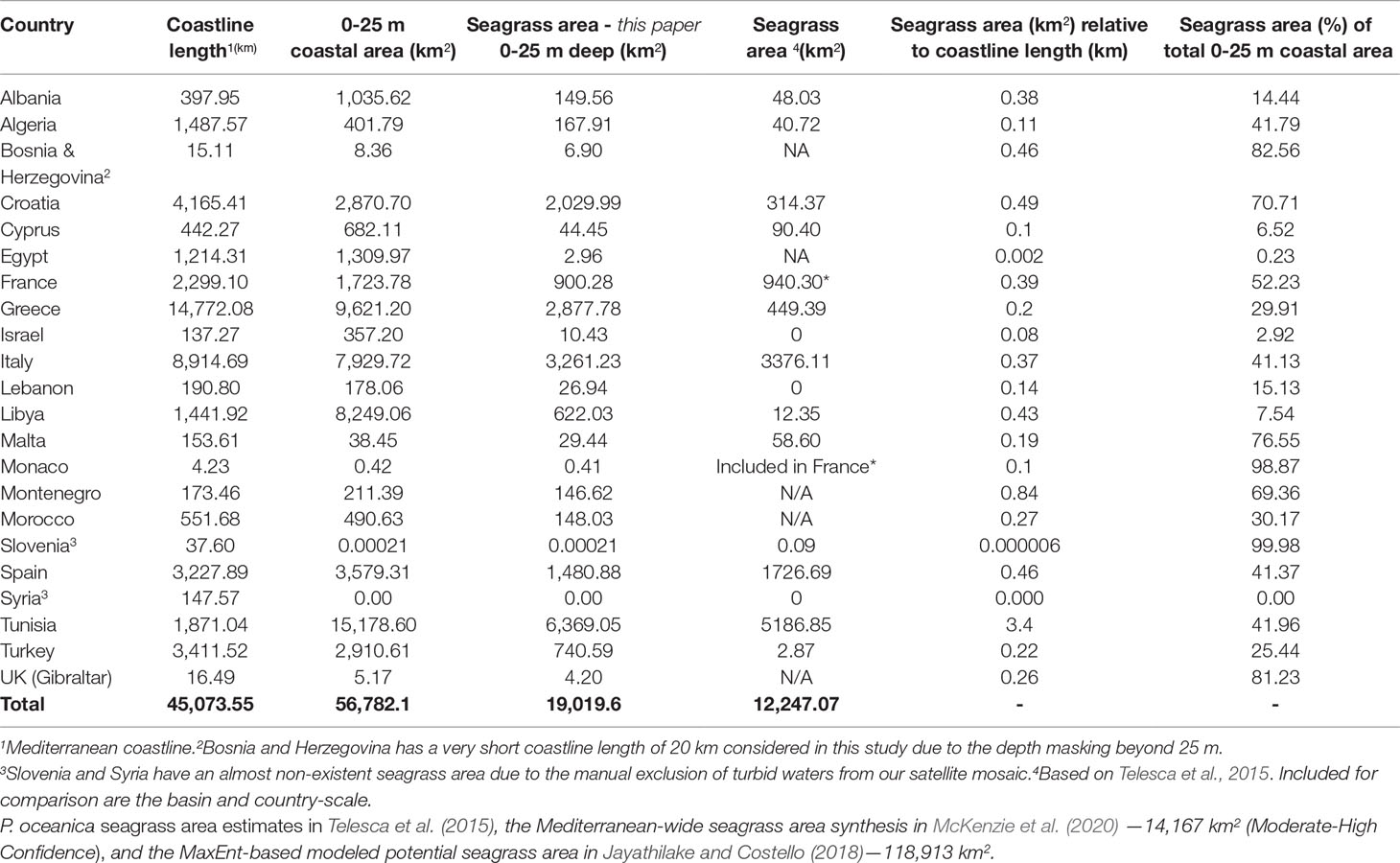
Table 3 Country-scale P. oceanica seagrass mapping estimates (km2) in the entire Mediterranean basin.
In comparison to the data gaps in P. oceanica seagrass meadows in Telesca et al. (2015) (46.7% featured missing data on seagrasses), our baseline spatially explicit estimate features: a greater coverage of the coastline of several countries (e.g., +92% in Greece, +89% in Libya, +86% in Croatia, and +84% in Algeria), one additional country-scale estimate (Bosnia and Herzegovina), and one additional overseas territory (UK - Gibraltar). Finally, in relation to the country-scale seagrass area of Greece, we estimated a 13.7% larger area than the estimation of 2,510 km2 (Traganos et al., 2018) that also used Sentinel-2 multi-temporal data within GEE.
Discussion
Using a cloud-native Earth Observation framework for big-satellite-data processing and analysis, we showcase here the first data-driven, spatially explicit seagrass extent accounting across an entire bioregional scale. Featuring a plethora of cutting-edge frameworks and algorithms in Earth Observation such as multi-temporal data analytics, machine learning, cloud computing, and big-data processing, we map 19,020 km2 of area of the seagrass P.oceanica across the entire basin using 10 m 279,186 open Sentinel-2 satellite image tiles (2015-2019) and 65,408 reference data points. Undoubtedly, the most important novelty of our mapping effort is the fact that we designed, synthesized, and employed a single and consistent data source—the multi-temporal Sentinel-2 data mosaic—within the moderately small temporal window of 4 years to account the bioregion-wide seagrass ecosystem extent.
The present mapping effort covers important gaps in the previous charting of the Mediterranean seagrasses. The previous Mediterranean-wide effort (Telesca et al., 2015) mapped 55.3% fewer seagrasses than our effort, lacking data on their distribution in 46.7% of the entire Mediterranean and 93% of the entire Eastern basin. Additionally, this effort featured multiple data sources based primarily on experts’ knowledge spanning 39 years (1972-2011) in contrast to the 4 years in this present study. An additional synthesis (McKenzie et al., 2020) used an existing inventory (UNEP-WCMC and Short, 2018) and only quantitative polygons with accompanied accuracy to calculate 14,167 km2 of Mediterranean seagrasses—that is 34.2% less than our calculation. The reliance of the two latter studies on multiple data sources and interpolated expert knowledge may have led to a possible under-estimation of the seagrass extent throughout the Mediterranean basin (United Nations Environment Programme, 2020). This was also highlighted in a recent national-scale seagrass mapping venture in the Greek territorial waters (Traganos et al., 2018) which yielded four times more seagrass habitats than the inventory of UNEP-WCMC and Short (2018). Last but not least, we calculated only one-sixth of the modeled-based area of Jayathilake and Costello (2018) (118,913 km2), which nonetheless was not an actual seagrass area estimate, but rather the potential regional occurrence of seagrasses in the basin according to their habitat suitability. Such model-guided output could complement the ability of the two former data syntheses to guide the scalability of data-driven mapping approaches like the one presented here. It is also worth noting that our basin-wide mapping estimate features a producer’s accuracy of 55% which increases the uncertainty in the aforementioned comparisons.
Our cloud-native framework was built on the latest technological advances in Earth Observation: cloud computing power, AI, and open and free satellite data. More specifically, we describe below four important technological innovations alongside their associated benefits and space for improvement, if applicable:
a) Scalability: We can scale up the cloud-native framework in terms of space (region, country, basin), time (monthly, seasonally, annually, multi-annually), as well as satellite data input depending on the final goal. The framework could be tuned to also ingest the multi-decadal satellite data archive of NASA/USGS Landsat series within GEE (Dwyer et al., 2018). The spatial scalability is demonstrated by the adaptation of the present Earth Observation system to ingest two orders of magnitude of Sentinel-2 tiles (1,000 to 100,000s) across tens of thousands of km² to map both the Greece-wide (Traganos et al., 2018) and the pan-Mediterranean seascape here.
b) Time efficiency: Through the high-performance computation within the Google Earth Engine, our framework requires less than 5 minutes (the maximum allocated time for on-demand interactive computations in the platform) to run its end-to-end big data processing to classify seagrasses; we estimate that just downloading 279,186 Sentinel-2 tiles, with an approximate size of 600MB/tile, would have taken somewhat less than 6 months on a 100 Mbps Fast Ethernet connection and 167.5TB of disk space, rendering the present venture unfathomable within a local server.
c) Multi-temporal Analytics: The cloud-native availability of the public satellite data archive of Sentinel-2 allowed the implementation of all available images within a selected period (> 4 years in this study) in a multi-temporal fashion. Following a statistical and metadata-based approach, this enabled the automated reduction of natural optical interferences (e.g., clouds, sunglint, waves, etc.) that impede the traditional off-the-shelf single-image approaches. In the future, the availability of larger temporal stacks within the cloud will enable more accurate multi-annual mapping analytics through the improved filtering of the aforementioned interferences.
d) Artificial intelligence: The Google Earth Engine platform contains a large set of available, ready-to-use, and easily-tuned ML algorithms, which ease the implementation and tuning of the AI component in the heart of our cloud-native algorithmic pipeline. Paired with hundreds of thousands of human-labeled training data, our selected algorithm of the Random Forests enabled a better classification result in comparison to the majority of applications in our targeted domain, most of which employ local-scale unsupervised and/or supervised classifiers in local servers. Looking into near-future potential AI developments, we expect that ensemble—voting systems consisting of several different ML classifiers which output the most voted class per pixel—and deep learning architectures could bring further breakthroughs in data-driven approaches in seagrass mapping. All of the above will be achieved by increasing automation and accuracy in the image annotation and streamlining of seagrass data and related reference data (Zhang, 2015; Islam et al., 2020). Nevertheless, deep learning techniques in Earth Observation have not yet reached the necessary maturity to provide a clear understanding of whether the extra scientific effort for a theoretically greater accuracy justifies the needed extra vast manual annotation and processing power compared to ML classifiers. Nor, in any way, have cloud-native earth observation frameworks within a coastal aquatic context matured.
We identify here four main challenges and associated uncertainties of the present framework along with potential solutions:
a) Over- and under-estimation of seagrass class: We carried out an intensive training data design to differentiate P. oceanica from other seagrass species present in the basin (e.g., Cymodocea nodosa and Zostera marina) and optically deep water. Nonetheless, based on a qualitative and quantitative assessment, clusters of over and under-estimation of the seagrass extent were inevitably identified. This could arise from several reasons. On one hand, over-estimation could have been produced from the pre-processing of our satellite mosaic to the level of the surface reflectance, accounting for the effect of the atmosphere and water surface media, but not of the water column medium. This causes spectral similarities across habitats with increasing depths (e.g., P. oceanica seagrass features a similar color to other seagrass habitats and also optically deep water on the satellite mosaic) leading to misclassifications of the P. oceanica class. We quantitatively confirmed the similar spectral ranges of the classes of P. oceanica meadows and deep water in Figure 4. The differences in spectral reflectances between the training and validation data for these two classes would further accentuate such misclassifications, namely the over-estimation of seagrass areas in our study. On the other hand, the possible under-estimation of our seagrass product is highlighted by two facts. First, its producer’s accuracy is 7% less than its user’s accuracy. This indicates that most of the P. oceanica in the map was also P. oceanica in the validation data, but that the map failed to capture a fair amount of this class. Second, masking out all depths deeper than 25 m (deemed necessary to reduce over-estimations due to the spectral confusion between seagrass and optically deep water) may have produced under-estimations. The mean lower depth limit of P. oceanica in the majority of the basin is around 35 m which means that our mapped area omitted 10 m of potential P. oceanica vertical habitat suitability. We evaluated the magnitude of this possible under-estimation, estimating 12,267 km² of coastal area between 25 and 35 m of depth. Using the seagrass coverage percentage per country of the total coastal area in the first 25 of depth, we estimated 3,964 km² of potential additional P. oceanica meadows across 22,984 km² at basin scale. This corresponds to 20.8% of possible under-estimation at the basin scale (Supplementary Table S2). The latter estimate is only 3.6% larger than the recent Mediterranean-wide estimate of Pergent-Martini et al. (2021) (22,161 km²) which was based on national seagrass areas. This increases the confidence in our cloud-based method and estimate as well as the calculation of the potential under-estimation here. Using the potential under-estimation of 3,964 km², the true bioregional blue carbon storage of P. oceanica beds increases to 872.7 million MgC, considering a Tier 2 assessment (Supplementary Table S4). Last but not least, other sources of over- and under-estimation of seagrass meadows and resulting uncertainties in our analysis could be potential confusion with macroalgae, existent seagrasses in waters of low to medium turbidity, varying seagrass density at the sub-pixel level, and, finally, relatively larger temporal differences between our mapping and implemented validation data (Table 2).
One way to alleviate these over and under-estimation trends could be to map bathymetry along with the habitat mapping and use cut-off depths for the optical presence of a certain class; for such a vast and diverse optical environment as the Mediterranean, this could be problematic due to the differences in optical properties between the western and eastern part. A second approach to solve this problem could be through forward modeling within the cloud to estimate the “true” seabed reflectance of seagrasses combined with the use of specific spectral indices that could allow differentiation between the seagrass and neighboring classes. A third way to resolve this could be the development of statistical thresholding on the attenuation coefficient layer which in turn will output only the optically shallow regions, offering the two-fold benefit of reduced computational power and improved detection accuracy.
b) Multi-temporal Analytics: Modern solutions are sometimes the forebears of equally modern problems. Our multi-temporal analytics employs a deep satellite image stack spanning more than 4 years. This allows us to address and correct natural optical interferences more efficiently. Yet, in baseline mapping estimations, this also means that such an approach would not detect any potential change within and across seagrasses or neighboring habitats over the studied period. Assuming no habitat loss, the slow growth of P. oceanica seagrass species is an advantage here. However, short-lived seagrasses in tropical regions undergo changes in much smaller time scales which would render obligatory the reduction of the temporal selection to monthly or seasonal composites to reflect their natural cycles. Nonetheless, tropical regions experience substantially more cloudy days than the Mediterranean, and thus the reduction of the time dimension would allow more clouds and cloud shadows to negatively impact our detection capabilities. Currently, this trade-off between change detection through multi-temporal analytics and the correction of environmental noise poses a very interesting scientific question in multi-temporal coastal aquatic mapping efforts within primarily tropical systems.
c) Training data: Large-scale mapping requires large-scale training data. The case of sufficient training data is one of the emerging issues due to the transition of seagrass mapping from the local to the cloud environment, and from the local to the bioregional mapping scale. Due to the absence of suitable and standardized training data to guide our ML component, we spent a labor-intensive effort to annotate an inventory that would match the multi-temporal Sentinel-2 mosaic. One possible approach to increase the time efficiency could be to design a machine or deep learning framework that, using the same satellite input with the mapping, could generate training data in a more automated way. Yet, to some extent, humans would still have to be involved in assessing the produced training data before these could be fed into the AI-based mapping. Anterior designed and implemented systems for semi-automated and automated interpretation of coastal and marine habitats do exist (Beijbom et al., 2015; González-Rivero et al., 2016; Griffin et al., 2017; Evans et al., 2018; Williams et al., 2019) and could be paired with our cloud-native framework in future endeavors.
d) Validation data: Large-scale mapping data also requires equally large-scale validation data. The four most important characteristics of suitable reference data for the validation of the Earth Observation mapping approaches include independence to the data source and location, higher quality and higher resolution than the training data in use, and temporal consistency of the source information with the mapping products. It was also very time-consuming to collect such reference data and transform them into a suitable format for the validation process (e.g., designing points within existing polygons in areas with sparse or no training data). Arguably, we could render the validation data design more time-efficient by developing a central user-friendly cloud-native tool (e.g., within Google Earth Engine), which could be used by experienced seagrass and seascape scientists for the design of large-scale, high-quality validation data on high-resolution satellite base maps such as the ones within Google Earth and/or Google Maps.
At present, we infer that based on the observed producer’s and user’s accuracy of our produced seagrass mapping data, which fluctuates between 40.2 and 69.1%, considerable efforts must be placed to improve our multi-temporal analytics and reference data design. Such efforts will enable the quantification of additional seagrass biophysical parameters with at least moderate confidence (e.g., leaf area index, above-ground biomass, cover, density, fragmentation, carbon stocks). These efforts would have to rely on not only a greater wealth of available and/or new field data collections to train AI frameworks, but also optical data of higher spatial resolution to detect and map sparser features than the 100 m2 pixel of Sentinel-2. It is without doubt that near-future collaborations with seagrass and coastal habitat scientists in and beyond the Mediterranean would expand our mapping capacity beyond just seagrass extent.
Our cloud-native framework can be adapted to account seagrasses and other coastal aquatic ecosystems beyond the geographic limits of the Mediterranean in both temperate and tropical waters. New mapping efforts using our framework can enrich the sparsity of existing large-scale coastal habitat mapping results. Notable examples focused only on tropical marine systems of >10,000 km2 include the pan-Caribbean seagrass mapping of Purkis et al. (2019), the merged geomorphic-benthic habitat product of Wabnitz et al. (2008) across 65,000 km2, and the coincident geomorphic and benthic habitat mapping of Lyons et al. (2020) across four orders of spatial magnitude. The latter multiscale Earth Observation framework is the only one, to the best of our knowledge, that can be compared to the herein framework as both use the same technological pillars (cloud computing, artificial intelligence, and satellite data) to produce scalable benthic habitat mapping products. Nevertheless, a qualitative and quantitative comparison of all the aforementioned mapping results would enable a better technical understanding of the challenges around these endeavors. Additionally, this would allow us to overcome the arising challenges towards continental and global-scale seagrass mapping following the examples of existing related global-scale EO frameworks and products: the Global Forest Watch (Hansen et al., 2013), the Global Mangrove Watch (Bunting et al., 2018), and the planetary-scale mapping of surface water (Pekel et al., 2016), and tidal flats (Murray et al., 2019).
Applying our pan-Mediterranean seagrass extent estimate of 19,020 km2 between 0 and 25 m and the region-specific seagrass carbon storage of Fourqurean et al. (2012), we estimate a 722.2 million MgC of blue carbon storage for all P. oceanica seagrass meadows in all 22 countries (Supplementary Table S3). Furthermore, applying our extrapolated bioregional extent estimate of 22,984 km2 between 0 and 35 m, we estimate 872.7 million MgC of P. oceanica seagrass blue carbon storage (Supplementary Table S4). While these bioregional and their underlying national blue carbon accounts are based on a single region-specific seagrass carbon estimate—and not on more accurate and dense country-specific data—we envisage that a broader availability of the latter data at the nationwide scale will unlock standardized, spatially explicit monitoring programs of seagrass blue carbon in and beyond the basin. These monitoring programs and their provided spatial accounts are expected to aid effective blue carbon policy actions and much-needed investments, especially in countries with large yet unaccounted seagrass carbon sinks (Macreadie et al., 2019; Macreadie et al., 2021).
Within the era of Space Renaissance that we are currently traversing, the stability and frequency of the cultural heritages that constitute the Copernicus Sentinel and Landsat satellite data collections could facilitate the transformation of comparable cloud-native frameworks into powerful, global decision support and knowledge systems that will:
a) Enhance the effective protection and management of seagrasses and their vital ecosystem services and functions.
b) Strengthen climate change resilience through the promotion and assessment of the role of seagrasses as a nature-based solution to climate change.
c) Assist the accounting of the seagrass-related Sustainable Development Goals and enforce their alignment with Nationally Determined Contributions and Ecosystem-based Adaptation approaches.
Namely, a new project entitled “Global Seagrass Watch” is established to impel this Earth Observation framework into a long-term standardized ecosystem accounting system. Its near-future implementation will empower scientists, governments, and policymakers to develop tangible solutions beyond their standard operating procedures in terms of communication, partnerships, and actions for the entirety of the coastal seascape environment.The latest advances in Earth Observation, namely the democratization and widespread availability of satellite data, AI, and cloud-based, large-scale satellite data processing paired with human-labeled reference data, allowed the adaptation of the cloud-native framework of this project in an innovative and scalable way. This scalability yielded in the present study bioregional seagrass ecosystem accounts with increased accuracy, cost and time-efficiency, as well as automation. Following the availability of suitable reference data and big satellite data analytics, we envisage that the present spatially-explicit seagrass ecosystem accounting effort will assist in paving the way towards future national to bioregional-scale accurate accounting of seagrass extent and related blue carbon stocks following recent mapping efforts (Murray et al., 2019; Serrano et al., 2019; Lyons et al., 2020) and needs (Macreadie et al., 2019).
Data Availability Statement
The original contributions presented in the study are included in the article/Supplementary Material. Further inquiries can be directed to the corresponding author.
Author Contributions
DT conceived the idea, designed the work, annotated the training data, and wrote the manuscript with the input of all co-authors. CL and DT designed, developed, and executed the cloud-based Earth Observation framework. DP coordinated the collection and design of the in situ validation data. HC, JD, VM, MM, GP, CP-M, and AR provided their validation data. AB ran the Tier 1 and Tier 2 blue carbon accounting. CL, AB, and DT designed all figures and tables. All authors contributed to the article and approved the submitted version.
Funding
DT acknowledges support from both a DLR-DAAD Research Fellowship (No. 57186656) and the DLR-funded Global Seagrass Watch Project. CL acknowledges support from a DLR-DAAD Research Fellowship (No. 57478193).
Conflict of Interest
The authors declare that the research was conducted in the absence of any commercial or financial relationships that could be construed as a potential conflict of interest.
Publisher’s Note
All claims expressed in this article are solely those of the authors and do not necessarily represent those of their affiliated organizations, or those of the publisher, the editors and the reviewers. Any product that may be evaluated in this article, or claim that may be made by its manufacturer, is not guaranteed or endorsed by the publisher.
Acknowledgments
In France, data used to build the 1:10000 marine habitat map were collected by Andromède Océanologie, Agence de l’Eau RMC, Conservatoire du Littoral, DREAL PACA; Egis Eau, ERAMM, GIS Posidonie, IFREMER, Institut océanographique Paul Ricard, Nice Côte d’Azur, TPM, Programme CARTHAM—Agence des Aires Marines Protégées, ASCONIT Consultants, COMEX-SA, EVEMAR, IN VIVO, Sentinelle, Stareso, Programme MEDBENTH, Université de Corse (EQEL), Ville de St Cyr-sur-mer, Ville de Cannes, Ville de Marseille, Ville de St Raphaël and Ville de St Tropez. We acknowledge and appreciate the assistance of an anonymous proof-reader towards improving the quality of this manuscript.
Supplementary Material
The Supplementary Material for this article can be found online at: https://www.frontiersin.org/articles/10.3389/fmars.2022.871799/full#supplementary-material
References
Andromède Océanologie (2019) Seabed Map, Donia Project. Available at: www.medtrix.fr.
Arnaud-Haond S., Duarte C. M., Diaz-Almela E., Marbà N., Sintes T., Serrão E. A. (2012). Implications of Extreme Life Span in Clonal Organisms: Millenary Clones in Meadows of the Threatened Seagrass Posidonia Oceanica. PLos One 7 (2), e30454. doi: 10.1371/Journal.pone.0030454
Atlas of Posidonia (2019). Available at: https://ideib.caib.es/posidonia/ [Accessed July 5, 2022].
Beijbom O., Edmunds P. J., Roelfsema C., Smith J., Kline D. I., Neal B. P., et al. (2015). Towards Automated Annotation of Benthic Survey Images: Variability of Human Experts and Operational Modes of Automation. PLos One 10 (7), e0130312. doi: 10.1371/journal.pone.0130312
Bunting P., Rosenqvist A., Lucas R. M., Rebelo L.-M., Hilarides L., Thomas N., et al. (2018). The Global Mangrove Watch — A New 2010 Global Baseline of Mangrove Extent. Remote Sens. Ecol. Conserv. 10 (10), 1669. doi: 10.3390/rs10101669
Coll M., Piroddi C., Steenbeek J., Kaschner K., Ben Rais Lasram F., Aguzzi J., et al. (2010). The Biodiversity of the Mediterranean Sea: Estimates, Patterns, and Threats. PLos One 5 (8), e11842. doi: 10.1371/journal.pone.0011842
de los Santos C. B., Krause-Jensen D., Alcoverro T., Marbà N., Duarte C. M., van Katwijk M. M., et al. (2019). Recent Trend Reversal for Declining European Seagrass Meadows. Nat. Commun. 10 (1), 3356. doi: 10.1038/s41467-019-11340-4
DFS Montenegro Engineering (2012). Start Up of “Katič” MPA in Montenegro and Assessment of Marine and Coastal Ecosystems Along the Coast.
Duarte C. M., Losada I. J., Hendriks I. E., Mazarrasa I., Marbà N. (2013). The Role of Coastal Plant Communities for Climate Change Mitigation and Adaptation. Nat. Climate Change 3 (11), 961–968. doi: 10.1038/nclimate1970
Duman M., Eronat A. H., İlhan T., Talas E., Küçüksezgin F. (2019). Mapping Posidonia Oceanica (Linnaeus) Meadows in the Eastern Aegean Sea Coastal Areas of Turkey: Evaluation of Habitat Maps Produced Using the Acoustic Ground Discrimination Systems. Int. J. Environ. Geoinformatics 6 (1), 67–75. doi: 10.30897/ijegeo.544695
Dwyer J. L., Roy D. P., Sauer B., Jenkerson C. B., Zhang H. K., Lymburner L. (2018). Analysis Ready Data: Enabling Analysis of the Landsat Archive. Remote Sens. 10 (9), 1363. doi: 10.3390/rs10091363
Evans S. M., Griffin K. J., Blick R. A., Poore A. G., Vergés A. (2018). Seagrass on the Brink: Decline of Threatened Seagrass Posidonia Australis Continues Following Protection. PLos One 13 (4), e0190370. doi: 10.1371/journal.pone.0190370
Finegold Y., Ortmann A., Lindquist E., d’Annunzio R., Sandker M. (2016). Map Accuracy Assessment and Area Estimation: A Practical Guide (Rome: Food Agriculture Organization of the United Nations).
Fourqurean J. W., Duarte C. M., Kennedy H., Marbà N., Holmer M., Mateo M. A., et al. (2012). Seagrass Ecosystems as a Globally Significant Carbon Stock. Nat. Geosci. 5 (7), 505–509. doi: 10.1038/ngeo1477
Gascon F., Bouzinac C., Thépaut O., Jung M., Francesconi B., Louis J., et al. (2017). Copernicus Sentinel-2A Calibration and Products Validation Status. Remote Sens. 9 (6), 584. doi: 10.3390/rs9060584
GENCAT (2021) Herbassars. Zones D’envolvents. Available at: http://agricultura.gencat.cat/ca/detalls/Article/Herbassars.-Zones-denvolvents.
Gislason P. O., Benediktsson J. A., Sveinsson J. R. (2006). Random Forests for Land Cover Classification. Pattern Recognition Lett. 27 (4), 294–300. doi: 10.1016/j.patrec.2005.08.011
González-Rivero M., Beijbom O., Rodriguez-Ramirez A., Holtrop T., González-Marrero Y., Ganase A., et al. (2016). Scaling Up Ecological Measurements of Coral Reefs Using Semi-Automated Field Image Collection and Analysis. Remote Sens. 8 (1), 30. doi: 10.3390/rs8010030
Gorelick N., Hancher M., Dixon M., Ilyushchenko S., Thau D., Moore R. (2017). Google Earth Engine: Planetary-Scale Geospatial Analysis for Everyone. Remote Sens. Environ. 202, 18–27. doi: 10.1016/j.rse.2017.06.031
Griffin K. J., Hedge L. H., González-Rivero M., Hoegh-Guldberg O. I., Johnston E. L. (2017). An Evaluation of Semi-Automated Methods for Collecting Ecosystem-Level Data in Temperate Marine Systems. Ecol. Evol. 7 (13), 4640–4650. doi: 10.1002/ece3.3041
Hansen M. C., Potapov P. V., Moore R., Hancher M., Turubanova S. A., Tyukavina A., et al. (2013). High-Resolution Global Maps of 21st-Century Forest Cover Change. Science 342 (6160), 850–853. doi: 10.1126/science.1244693
Islam K. A., Hill V., Schaeffer B., Zimmerman R., Li J. (2020). Semi-Supervised Adversarial Domain Adaptation for Seagrass Detection Using Multispectral Images in Coastal Areas. Data Sci. Eng. 5, 111–125. doi: 10.1007/s41019-020-00126-0
IUCN (2021). Manual for the Creation of Blue Carbon Projects in Europe and the Mediterranean. Ed. Otero M., 144 pages. Center for Mediterranean Cooperation, Malaga, Spain
Jayathilake D. R., Costello M. J. (2018). A Modelled Global Distribution of the Seagrass Biome. Biol. Conserv. 226, 120–126. doi: 10.1016/j.biocon.2018.07.009
Jordà G., Marbà N., Duarte C. M. (2012). Mediterranean Seagrass Vulnerable to Regional Climate Warming. Nat. Climate Change 2 (11), 821–824. doi: 10.1038/nclimate1533
Lyons M. B., Roelfsema C. M., Kennedy E. V., Kovacs E. M., Borrego-Acevedo R., Markey K., et al. (2020). Mapping the World’s Coral Reefs Using a Global Multiscale Earth Observation Framework. Remote Sens. Ecol. Conserv. 6 (4), 557–568. doi: 10.1002/rse2.157
Macreadie P. I., Anton A., Raven J. A., Beaumont N., Connolly R. M., Friess D. A., et al. (2019). The Future of Blue Carbon Science. Nat. Commun. 10 (1), 1–13. doi: 10.1038/s41467-019-11693-w
Macreadie P. I., Costa M. D. P., Atwood T. B., Friess D. A., Kelleway J. J., Kennedy H., et al. (2021). Blue Carbon as a Natural Climate Solution. Nat. Rev. Earth Environ. 2 (12), 826–839. doi: 10.1038/s43017-021-00224-1
Marbà N., Duarte C. M. (1998). Rhizome Elongation and Seagrass Clonal Growth. Mar. Ecol. Prog. Ser. 174, 269–280. doi: 10.3354/meps174269
McKenzie L. J., Nordlund L. M., Jones B. L., Cullen-Unsworth L. C., Roelfsema C., Unsworth R. K. (2020). The Global Distribution of Seagrass Meadows. Environ. Res. Lett. 15 (7), 074041. doi: 10.1088/1748-9326/ab7d06
Murray N. J., Phinn S. R., DeWitt M., Ferrari R., Johnston R., Lyons M. B., et al. (2019). The Global Distribution and Trajectory of Tidal Flats. Nature 565 (7738), 222–225. doi: 10.1038/s41586-018-0805-8
Paoli C., Povero P., Burgos E., Dapueto G., Fanciulli G., Massa F., et al. (2018). Natural Capital and Environmental Flows Assessment in Marine Protected Areas: The Case Study of Liguria Region (NW Mediterranean Sea). Ecol. Model. 368, 121–135. doi: 10.1016/j.ecolmodel.2017.10.014
Pekel J.-F., Cottam A., Gorelick N., Belward A. S. (2016). High-Resolution Mapping of Global Surface Water and its Long-Term Changes. Nature 540 (7633), 418–422. doi: 10.1038/nature20584
Pergent G., Bazairi H., Bianchi C. N., Boudouresque C. F., Buia M., Calvo S., et al. (2014). Climate Change and Mediterranean Seagrass Meadows: A Synopsis for Environmental Managers. Mediterr. Mar. Sci. 15 (2), 462–473. doi: 10.12681/mms.621
Pergent-Martini C., Pergent G., Monnier B., Boudouresque C.-F., Mori C., Valette-Sansevin A. (2021). Contribution of Posidonia Oceanica Meadows in the Context of Climate Change Mitigation in the Mediterranean Sea. Mar. Environ. Res. 165, 105236. doi: 10.1016/j.marenvres.2020.105236
Pergent-Martini C., Valette-Sansevin A., Pergent G. (2015). Cartographie Continue Des Habitats Marins En Corse / Résultats Cartographiques.
Poursanidis D., Traganos D., Reinartz P., Chrysoulakis N. (2019). On the Use of Sentinel-2 for Coastal Habitat Mapping and Satellite-Derived Bathymetry Estimation Using Downscaled Coastal Aerosol Band. Int. J. Appl. Earth Observation Geoinformation 80, 58–70. doi: 10.1016/j.jag.2019.03.012
Purkis S. J., Gleason A. C., Purkis C. R., Dempsey A. C., Renaud P. G., Faisal M., et al. (2019). High-Resolution Habitat and Bathymetry Maps for 65,000 Sq. Km of Earth’s Remotest Coral Reefs. Coral Reefs 38 (3), 467–488. doi: 10.1007/s00338-019-01802-y
Ricart A. M. (2016). Insights Into Seascape Ecology: Landscape Patterns as Drivers in Coastal Marine Ecosystems (Barcelona, Spain: Universitat de Barcelona).
Rigo I., Paoli C., Dapueto G., Pergent-Martini C., Pergent G., Oprandi A., et al. (2021). The Natural Capital Value of the Seagrass Posidonia Oceanica in the North-Western Mediterranean. Diversity 13 (10), 499. doi: 10.3390/d13100499
Serrano O., Lovelock C. E., Atwood T. B., Macreadie P. I., Canto R., Phinn S., et al. (2019). Australian Vegetated Coastal Ecosystems as Global Hotspots for Climate Change Mitigation. Nat. Commun. 10 (1), 1–10. doi: 10.1038/s41467-019-12176-8
Telesca L., Belluscio A., Criscoli A., Ardizzone G., Apostolaki E. T., Fraschetti S., et al. (2015). Seagrass Meadows (Posidonia Oceanica) Distribution and Trajectories of Change. Sci. Rep. 5 (1), 1–14. doi: 10.1038/srep12505
Traganos D., Aggarwal B., Poursanidis D., Topouzelis K., Chrysoulakis N., Reinartz P. (2018). Towards Global-Scale Seagrass Mapping and Monitoring Using Sentinel-2 on Google Earth Engine: The Case Study of the Aegean and Ionian Seas. Remote Sens. 10 (8), 1227. doi: 10.3390/rs10081227
Traganos D., Reinartz P. (2018a). Interannual Change Detection of Mediterranean Seagrasses Using RapidEye Image Time Series. Front. Plant Sci. 9. doi: 10.3389/fpls.2018.00096
Traganos D., Reinartz P. (2018b). Mapping Mediterranean Seagrasses With Sentinel-2 Imagery. Mar. pollut. Bull. 134, 197–209. doi: 10.1016/j.marpolbul.2017.06.075
UNEP/MAP (2012). State of the Mediterranean Marine and Coastal Environment (Athens: UNEP/MAP – Barcelona Convention).
UNEP-WCMC, Short F. T. (2018) Global Distribution of Seagrasses (Version 6.0). Sixth Update to the Data Layer Used in Green and Short, (2003). Available at: http://data.unep-wcmc.org/datasets/7.
United Nations (2015). Transforming Our World: The 2030 Agenda for Sustainable Development (New York, USA: United Nations).
United Nations Environment Programme (2020). Out of the Blue: The Value of Seagrasses to the Environment and to People (Nairobi, Kenya: UNEP).
United Nations Framework Convention on Climate Change (2021) Nationally Determined Contributions (NDCs). Available at: https://unfccc.int/process-and-meetings/the-paris-agreement/nationally-determined-contributions-ndcs/nationally-determined-contributions-ndcs (Accessed 11 January 2022).
Wabnitz C. C., Andréfouët S., Torres-Pulliza D., Müller-Karger F. E., Kramer P. A. (2008). Regional-Scale Seagrass Habitat Mapping in the Wider Caribbean Region Using Landsat Sensors: Applications to Conservation and Ecology. Remote Sens. Environ. 112 (8), 3455–3467. doi: 10.1016/j.rse.2008.01.020
Williams I. D., Couch C. S., Beijbom O., Oliver T. A., Vargas-Angel B., Schumacher B. D., et al. (2019). Leveraging Automated Image Analysis Tools to Transform Our Capacity to Assess Status and Trends of Coral Reefs. Front. Mar. Sci. 6. doi: 10.3389/fmars.2019.00222
Keywords: Mediterranean, Sentinel-2, Posidonia oceanica, Coastal ecosystem accounting, Google Earth Engine Seagrass, Earth Observation, Blue Carbon
Citation: Traganos D, Lee CB, Blume A, Poursanidis D, Čižmek H, Deter J, Mačić V, Montefalcone M, Pergent G, Pergent-Martini C, Ricart AM and Reinartz P (2022) Spatially Explicit Seagrass Extent Mapping Across the Entire Mediterranean. Front. Mar. Sci. 9:871799. doi: 10.3389/fmars.2022.871799
Received: 08 February 2022; Accepted: 22 June 2022;
Published: 22 July 2022.
Edited by:
Catherine Lovelock, The University of Queensland, AustraliaReviewed by:
Maria Laura Zoffoli, National Research Council (CNR), ItalyKonstantinos Topouzelis, University of the Aegean, Greece
Copyright © 2022 Traganos, Lee, Blume, Poursanidis, Čižmek, Deter, Mačić, Montefalcone, Pergent, Pergent-Martini, Ricart and Reinartz. This is an open-access article distributed under the terms of the Creative Commons Attribution License (CC BY). The use, distribution or reproduction in other forums is permitted, provided the original author(s) and the copyright owner(s) are credited and that the original publication in this journal is cited, in accordance with accepted academic practice. No use, distribution or reproduction is permitted which does not comply with these terms.
*Correspondence: Dimosthenis Traganos, ZGltb3N0aGVuaXMudHJhZ2Fub3NAZGxyLmRl