- 1Department of Environmental Biology, Sapienza University of Rome, Rome, Italy
- 2Institute for the Study of Anthropogenic Impacts and Sustainability in the Marine Environment, National Research Council, Trapani, Italy
- 3Department of Statistical Sciences, Sapienza University of Rome, Rome, Italy
- 4Istituto Superiore per la Protezione e la Ricerca Ambientale (ISPRA), Italian Institute for Environmental Protection and Research, Rome, Italy
- 5Department of Integrated Marine Ecology, Stazione Zoologica Anton Dohrn, Naples, Italy
- 6Department of Mathematical Sciences, Norwegian University of Science and Technology, Trondheim, Norway
Assessing the distribution of marine apex–predators is pivotal to understanding community interactions and defining management goals. However, several challenges arise in both estimates and predictions considering the distinctive and mutable biological/ecological requirements of these species and the influence of human activities. Thus, efforts to study apex–predators’ spatial distribution patterns must deal with inherent uncertainty. Relying on different data sources (research programs and social media reports), physiographic and environmental covariates (depth, slope, surface temperature and chlorophyll–a), and specific source–related detection functions, this study selected a Spatial Log–Gaussian Cox Process to model the distribution patterns of an opportunistic apex–predator, the common bottlenose dolphin (Tursiops truncatus), over 14 years (2008−2021) in the Mediterranean Sea (Italy) using a total of 955 encounters. Both depth and slope showed a significant (95% significance) reduction effect in the encounters when deeper and steeper, respectively. Temperature (parabolic) shows a positive effect (90% significance), while chlorophyll–a values did not seem to have a significant effect on encounter intensities within each season. The estimated posterior mean and the coefficient of variation surfaces for the intensity by season showed higher intensity in summer near the Tiber River estuary than other regions. Almost homogeneous predictions were observed in winter, with marginal greater intensities where lower temperatures and higher chlorophyll–a concentration were observed. The relatively low variance was predicted in the more coastal parts of the study area within each season, while higher uncertainty was instead revealed in the southernmost offshore area. This study highlighted the persistent presence of the common bottlenose dolphin in the investigated area both winter and summer, with a coherent distribution within each season, and rare transient occurrences in deeper waters (where uncertainty increases). Thanks to its versatile characteristics, the species seems to well adapt to different seasonal conditions and maintain its distributional range.
Introduction
Multiple roles are recognized for apex predators in the marine environment, fulfilling key ecological, economic, and cultural functions (Hammerschlag et al., 2019). Apex predators are indeed affected by bottom−up processes and can influence food webs via consumptive effects on prey (top−down effects) (Heithaus et al., 2008; Steneck, 2012; Kiszka et al., 2022). Hence, assessing their distribution is pivotal to understanding community interactions and defining management goals to be implemented (Hammerschlag et al., 2019). However, several challenges arise in both distribution estimates and predictions (Pace et al., 2019; Martino et al., 2021), generated by high movement ability and large home–ranges covered by these species, spatio-temporal knowledge gaps and the increasing use of different data sources to model their distribution (Watson et al., 2019). Considering also the distinctive and changing biological and ecological requirements of these species (Forcada, 2018; Pace et al., 2018), and the influence of threats related to human activities (Nelms et al., 2021), efforts to study spatial distribution patterns must deal with inherent uncertainty (Ansong et al., 2017; Stephenson et al., 2021).
The common bottlenose dolphin (Tursiops truncatus) is a cosmopolitan marine apex predator inhabiting a variety of habitats, including nearshore waters, harbors, estuaries, and deeper waters over the continental shelf worldwide (Wells and Scott, 2018; Wells et al., 2019). The species range poleward of 45° in northern Europe and southern New Zealand, but it has been reported as far south as 53-55°S in South America and as far north as British Columbia (50°N) (Wells et al., 2019). Different elements seem to drive its distribution in space and time and habitat use, being under the influence of environmental [e.g., sea surface temperature (SST) and bathymetry], ecological [e.g., prey distribution], social [e.g., inter- and intraspecific interactions/relationships] and anthropogenic variables [e.g., fishing activities and boat traffic] (e.g., see Bennington et al., 2020; Diaz Lopez, 2019; Greller et al., 2021; Haughey et al., 2021; Zanardo et al., 2017 and references herein). Considering their widespread distribution and prominent presence in the coastal marine ecosystems, common bottlenose dolphins could have a significant role in the structure and function of these ecosystems (Diaz Lopez, 2019). However, significant gaps impede our capability to fully determine several critical attributes of the function of these predators, particularly their fine–scale distribution and movements, feeding rates, and prey selection (Kiszka et al., 2022).
In the Mediterranean Sea (30-41°N), the common bottlenose dolphin is widespread across the entire basin, occurring primarily in coastal habitats with depths<100 m (Natoli et al., 2021), often in correspondence with highly productive systems with significant ecological importance in the marine life environment. In the basin, the species shows a remarkable level of ecological and behavioral plasticity, an erratic distribution, and variable habitat use and residency degrees depending on local conditions, resources availability and social factors (Blasi and Boitani, 2012; Giannoulaki et al., 2017; Vassallo et al., 2020; Pace et al., 2021). Several studies reported different distribution patterns, where individuals may present high site fidelity or, conversely, show movements on a scale of hundreds of kilometers (e.g., Gnone et al., 2011; Pulcini et al., 2014; Pleslić et al., 2019; Pace et al., 2021; Labach et al., 2022). Many environmental factors, physiographic characteristics, and seasonal patterns appear to drive the observed fine–scale coastal distribution and seem to best predict suitable habitats for the species (Blasi and Boitani, 2012; Marini et al., 2015; La Manna et al., 2016; Laran et al., 2017; Giannoulaki et al., 2017; Vassallo et al., 2020; Gnone et al., 2022). In addition, the highly opportunistic, versatile, and resilient nature of the common bottlenose dolphin diet and foraging strategies (Giménez et al., 2017; Borrell et al., 2021; Carmen et al., 2021; Natoli et al., 2021) enable the species to also adjust its behavior as a consequence of resources’ availability. This opportunistic behavior can be also applied to resources related to human activities (e.g., fisheries and aquaculture) (Bonizzoni et al., 2021; Pace et al., 2022a; Pace et al., 2019; Triossi et al., 2013). All these characteristics may facilitate the development of discrete geographical units in the Mediterranean population (Carnabuci et al., 2016; Vassallo et al., 2020), making distribution assessments more difficult and increasing the uncertainty when predicting scenarios.
The proximity to human activities in the Mediterranean coastal areas makes the common bottlenose dolphin susceptible to various anthropogenic threats (e.g., bycatch, vessel traffic, overfishing, contaminants, and noise pollution) (Natoli et al., 2021). Consequently, the species was included in Annex II of the EU Habitats Directive (92/43/CEE) as priority species and was listed as Least Concern in the last IUCN Red List of Threatened species regional assessment (Natoli et al., 2021). This new assessment imperatively recommended continuously monitoring the effects of human–related stressors on the common bottlenose dolphin to ensure the maintenance of intra–species diversity and the survival across its range (Natoli et al., 2021).
Considering the species characteristics and the variations of the natural and anthropogenic conditions, a large number of occurrence data is required to support studies investigating common bottlenose dolphin distribution, as well as a robust analytical approach capable of coping with heterogeneous data and the variability of predictors (Martino et al., 2021). Relying on different data sources (research programs and social media reports), physiographic and environmental covariates (depth, slope, surface temperature and chlorophyll–a), and specific source–related detection functions, this study select a Spatial Log–Gaussian Cox Process to model common bottlenose dolphin distribution patterns using 14-years data (2008−2021) collected in the Mediterranean Sea. Here, to effectively manage and solve the complex issue of presence-only data, the suggestions offered by Warton and Shepherd (2010) and, in the ecological framework, by Renner et al. (2015) were used, adopting a point processes approach where pseudo-absences generation is not required. Since anthropogenic pressures (i.e., coastal population, fishing and tourism; see Figures S5 and S6 in the Supplementary Materials; http://dati.istat.it/, https://www.politicheagricole.it) on the study area were constant within each season in the considered time window of 14 years, a spatial model with a seasonal effect was adopted. Applying the approach and the methodology developed and tested in Martino et al. (2021), this study investigates the common bottlenose dolphin seasonal distribution in a broader area than previously reported and discusses the flexibility of the species to ecological drivers and anthropogenic forces.
Materials and methods
Study site
The study area is located in the western Mediterranean Sea, within the Ligurian and central Tyrrhenian Sea (Figure 1), off the Tuscany and Lazio coasts (Italy). The area covers almost 60,000 km2 and is a complex marine region including various environmental features (e.g., continental shelf, slope, canyons, seamounts) and various habitats (seagrass meadows, hard‐bottom communities with coastal banks, cliffs, sand, and mud). The northern section spans mainly over the continental shelf. It is characterized by shallow waters and coastal shoals surrounding several islands, which form the Tuscan Archipelago (i.e., Capraia, Elba, Giannutri, Giglio, Gorgona, Montecristo, and Pianosa Islands). The Elba, Capraia, and Pianosa Islands area presents mainly shallow waters within the 100 m bathymetry. In contrast, the west–southern area of the Archipelago is characterized by steeper slopes and deep canyons, reaching more than 500 m depths (Ricevuto et al., 2011). Between Capraia and Gorgona Islands, the continental shelf is crossed by the Elba canyon, which descends deeply toward the North–West. The seabed between Elba and Argentario consists of a single basin, bordered to the west by the Elba ridge, to the east by the Tuscany coast, and to the north by the Piombino canal and Elba. The central–southern section of the study area includes the continental shelf areas off the Lazio coast and, toward the south, the islands of Ponza, Palmarola, and Ventotene (Pontine Archipelago).
Many seamounts are included or border the entire study area, such as the Santa Lucia and Occhiali seamounts in the north, the Cialdi, Etruschi, and Tiberino in the middle, and the Albano seamount in the south (Würtz and Rovere, 2015). Seamounts attract a rich associated fauna and strongly influence the distribution of pelagic top predators, which can find optimal foraging areas around them (Würtz and Rovere, 2015; Bo et al., 2020). The several river mouths present in the area affect the coastal marine ecosystems as they are major sources of organic matter for the adjacent marine waters. They include the Arno river (in the northern part of the study area), the Tiber river (in the middle of the study area), and the Garigliano and Volturno rivers (in the southern part of the study area).
Part of the study area (Figure 1) is included in the international Pelagos Sanctuary for the protection of Marine Mammals and is classified as a Specially Protected Area of Mediterranean Importance (SPAMI) under the Barcelona Convention Protocol for Specially Protected Areas and Biological Diversity in the Mediterranean (SPA/BD Protocol). Additionally, many Marine Protected Areas, including several Sites of Community Importance and Special Protection Areas under the Natura 2000 European network of the Habitat Directive (92/43/EEC), are comprised in the study area. Twelve Natura 2000 sites are found in the northern portion (10 SCZ and 2 SCI), including the new designated Natura 2000 SCI site ‘Tutela del Tursiops truncatus’ − IT5160021, located just off the Tuscany coast, for the protection of the common bottlenose dolphin. Two marine protected areas (MPAs) can be found in the southern portion of the study area: the MPA Islands of Ventotene and S. Stefano in the Pontine Archipelago [recently acknowledged by the International Union for Conservation of Nature (IUCN) as Important Marine Mammal Area (IMMA, IUCN-MMPATF, 2017)] and the MPA Tor Paterno bank near Rome. The entire study area is under constant anthropogenic pressures only partially mitigated by the above-mentioned protective measures (see section S5 in the Supplementary Materials for further information on the demographic, fishing and touristic variations in the study area).
Data sources
Following the approach used by Martino et al. (2021), three types of sighting data over 14 years (2008–2021) were used considering two seasons (summer: April−September; winter: October−March). Data included: 1) information derived from conventional visual/acoustic research protocols (adaptive sampling) using motor/sailing boats (Pace et al., 2021; Pace et al., 2021; Papale et al., 2021; Pace et al., 2022a) the resulting dataset was labeled UNIRM; 2) information originated from standardized monitoring protocols (distance sampling) using platforms of opportunity within the project “FLT Mediterranean Monitoring Network” (ISPRA, 2016; Arcangeli et al., 2019; Pace et al., 2019); the resulting dataset was labeled FERRY-FLT; 3) information extracted from social media (Facebook and YouTube) using reports by sea–users (Pace et al., 2019); the resulting dataset was labeled SM. Detailed elements on SM data collection procedures and selection are provided in Pace et al. (2019) and Martino et al. (2021). As the SM dataset also included details on other cetacean species than the common bottlenose dolphin (see Table S1 in the Supplementary Materials), this information was used as a proxy to infer boat densities potentially able to record the animals’ presence (see the following ”Modeling approach” paragraph). The issue of estimating boats’ (especially smaller ones) density was here further developed to explore additional potential sources of information (see section S3 in the Supplementary Materials) to extend and enhance what already reported in Martino et al. (2021).
Physiographic and environmental covariates
The following covariates were initially selected as reasonable proxies for the species’ ecological needs (La Manna et al., 2016): salinity, depth, slope, sea surface temperature (SST), and chlorophyll–a. Salinity was not significant for modeling, thus only the last four were used. Depth data were downloaded from GEBCO (General bathymetric Chart of the Ocean – https://www.gebco.net); the slope was computed from depth data through the terrain() function of the R package “terra” (https://www.r–project.org/; Hijmans, 2022); SST and chlorophyll–a were retrieved from COPERNICUS platform (https://marine.copernicus.eu/) as monthly average. The retrieved datasets and data handling procedures are reported in Martino et al. (2021) and the Supplementary Materials (see section S1).
Modeling approach
Dolphin sightings were aggregated over time into two seasons (summer and winter) and viewed as two-point patterns over space. Therefore, a point process model was used to describe how those points are generated. To integrate data from all available sources and manage possible detection bias in each dataset (see section S2 in the Supplementary Materials), a Spatial Log–Gaussian Cox Process (LGCP) (Renner et al., 2015) incorporating different detection functions for each data source was built (see Martino et al., 2021 for details). It was assumed that sighting patterns, i.e., locations of dolphin groups in space ( s∈S⊂R2 ) and season t=1, 2 (t is the season), were properly described by a point process whose intensity function (s,t) is additive on the log–scale:
where X(s,t) is a set of covariates detected at location s and time t with linear effects β to be estimated, and ω(s) is a zero–mean Gaussian process with Matérn covariance function of order 1, describing the residual spatial variation. Notice that both the effect of covariates β and the spatial process ω(s) are assumed to be constant and are estimated jointly using data from both seasons.
The vector X included both time constant (depth and slope) and time dependent (SST and chlorophyll–a) covariates. Temperature has been included both as a linear and quadratic term. To distinguish between a possible seasonal effect and the spatial effect of the covariate within one season, we created a standardized version of both temperature and chlorophyll–a as:
where sst(t) and chl(t) are the seasonal means of surface temperature and chlorophyll–a over the whole domain of interest, defined as:
The model for the log intensity was then formalized as:
Where β0 is a global mean, β1 a seasonal effect and I(summer) is an indicator variable for the summer season and the rest of symbols are explained above.
It was assumed that the above process was observed in three different ways, conditionally independent given λ(s,t). Thus, three observed intensities were defined as:
where gj(s,t) is the detection function (with values between 0 and 1) which determines the thinning of the original process (Martino et al., 2021). The detection functions were defined as follows:
For the adaptive sampling (UNIRM) data:
where d1(s) is the distance (Km) between point s and the position of the boat when the groups were sighted. K = 4 Km was defined as the maximum distance measured between the location of the first visual sight of a dolphin group by researchers.
For the distance sampling (FERRY-FLT) data, the half normal detection function was used, defined as:
where, d2(s) is the perpendicular distance (Km) to the ferry track and ξ2 is a scale parameter.
Finally, for the SM data, the sighting probability was assumed to be larger with higher number of citizens’ small boats, so that the detection function was defined as:
Where Ф is the cumulative distribution function of a standard normal distribution, d3(s,t) is the log–intensity of small boats at point s and time t, and µ3 and ξ3 are location and scale parameters.
The intensity of the small boats d3(s,t) is unknown. Following Martino et al. (2021), this function was estimated using sightings of all cetacean species included in the SM dataset (see Table S1 and Figure S2 in the Supplementary Materials), and accounting for seasonality (more small boats in summer than in winter are expected). The parameters of the detection function ξ3 and µ3 were kept constant between seasons (see Supplementary S2 section for details).
An alternative estimation of the density of small boats d3(s,t) was attempted using images of Copernicus Sentinel–1 satellite radar. Such an approach was proposed in Martino et al. (2021) as a future development but has not been successful in the present study, as the intensity surfaces derived from satellite data reported several artifacts (see section S3 in the Supplementary Materials for details).
The model was fitted in a Bayesian setting using the inlabru R package (Yuan et al., 2017; Bachl et al., 2019). The approach allows for the estimation of all model components, jointly including the parameters in the detection functions, and therefore accounting for all uncertainties in a coherent way (see section S4 in the Supplementary Materials for prior specifications and details about the implementation).
Once the model was fitted, the estimated mean number of sightings was predicted over the whole area of interest as:
Such an integral can be estimated using Monte Carlo sampling from the fitted model.
Results
A total of 955 common bottlenose dolphin encounters was collected by research projects and social media reports (Table 1) over a period of 14 years, with a higher number documented in summer (N = 759) than winter (N = 196).
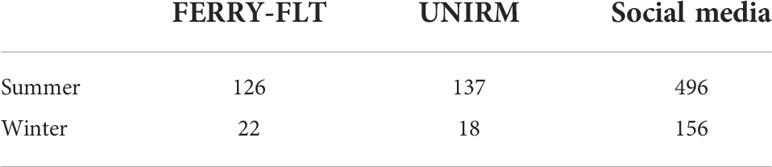
Table 1 Total number of common bottlenose dolphins recorded by the two research programs [onboard ferries FLT Net (FERRY-FLT) and a dedicated survey platform UNIRM)] and by social media.
The total research effort in the two research programs (UNIRM and FERRY-FLT datasets) was 23,920 Km during summer (21,479 Km by FERRY-FLT; 2,441 by UNIRM) and 3,477 Km during winter (3,010 Km by FERRY-FLT; 467 by UNIRM). Total effort by season is shown in Figure 2.
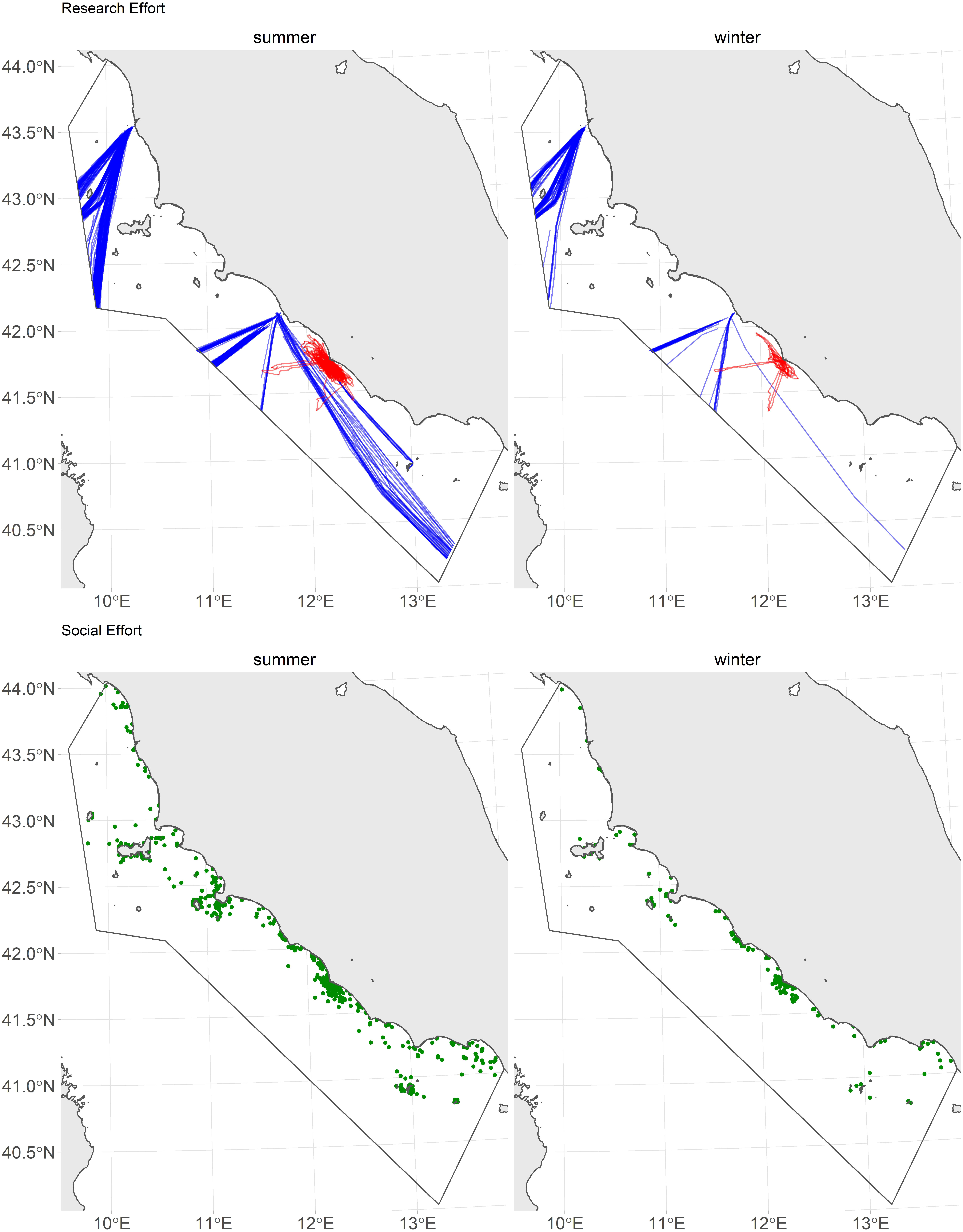
Figure 2 Total research effort by UNIRM (red) and FLT Net (blue) research programs and point location of social media sightings (green dots).
Maps showing covariates used within the model are shown in Figure 3 (depth and slope) and Figure 4 (seasonal sea surface temperature and chlorophyll–a). Estimated maps of observation process intensity used in the detection functions are presented in Figure 5. The estimated value for the posterior means together with 90 and 95% credible interval (CI) of the model’s fixed effects are shown in Table 2. Importance or significance of variables can be deducted by examining the overlap of their 90 or 95% CI with zero. Both depth and slope showed a significant reduction effect in the encounters when deeper and steeper, respectively (90 and 95% CI do not contain zero). The seasonal effect (Seasonal(β1) has fully positive 90 and 95% CI) indicated an increase in the encounter intensity during the summer season. The two space–time varying covariates, SST and chlorophyll–a values, were not significant within each season at the 95% significance level (CI contain zero). However, the parabolic effect of SST was significant at 90% level, suggesting a highly variable, but positive effect of the surface temperature. Spatial field’s parameters are reported in the Supplementary Material (see S4 section).
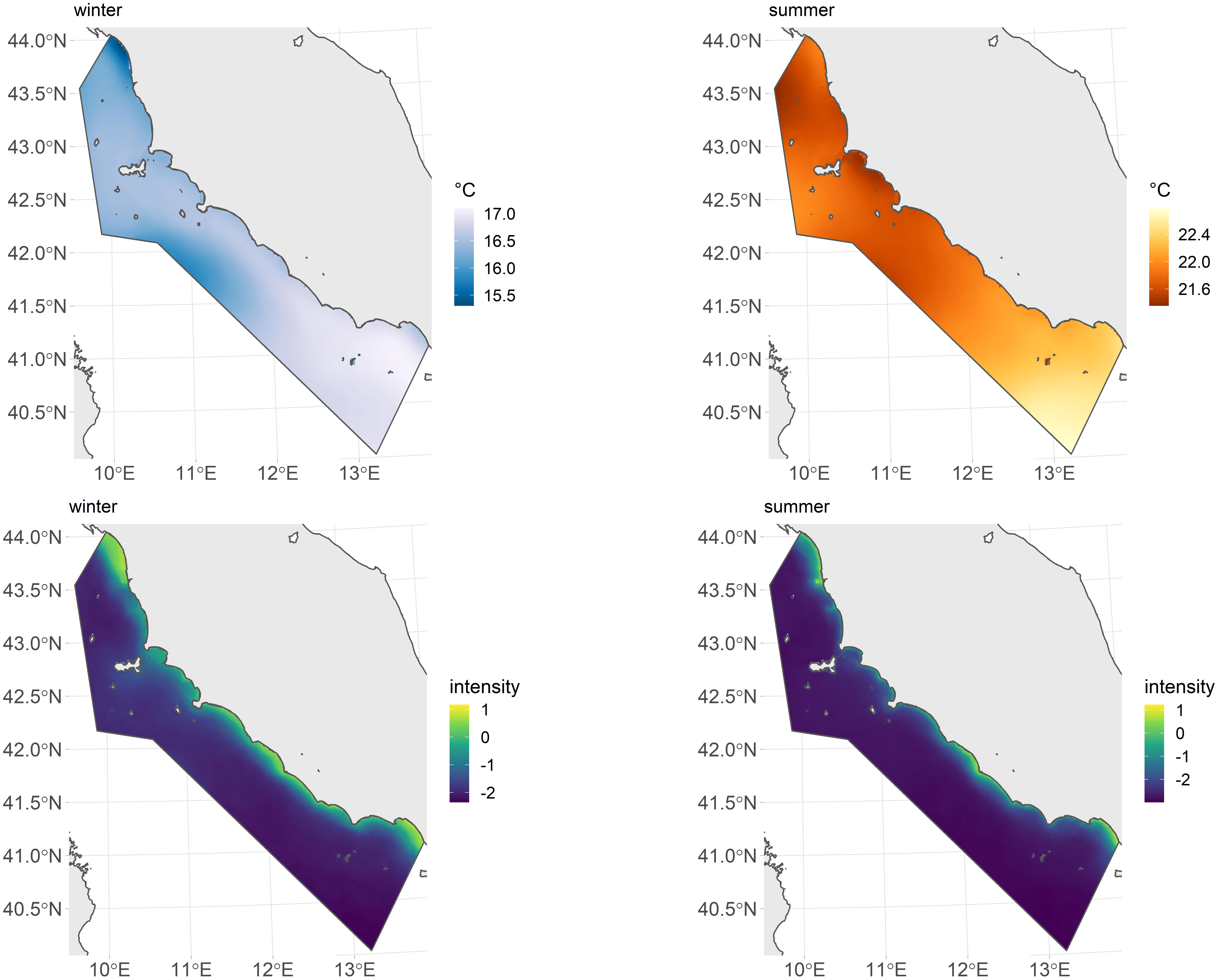
Figure 4 Sea surface temperature (SST) distribution in winter (upper left panel) and summer (upper right panel), and chlorophyll–a log–scale values in winter (lower left panel) and summer (lower right panel) in the study area.
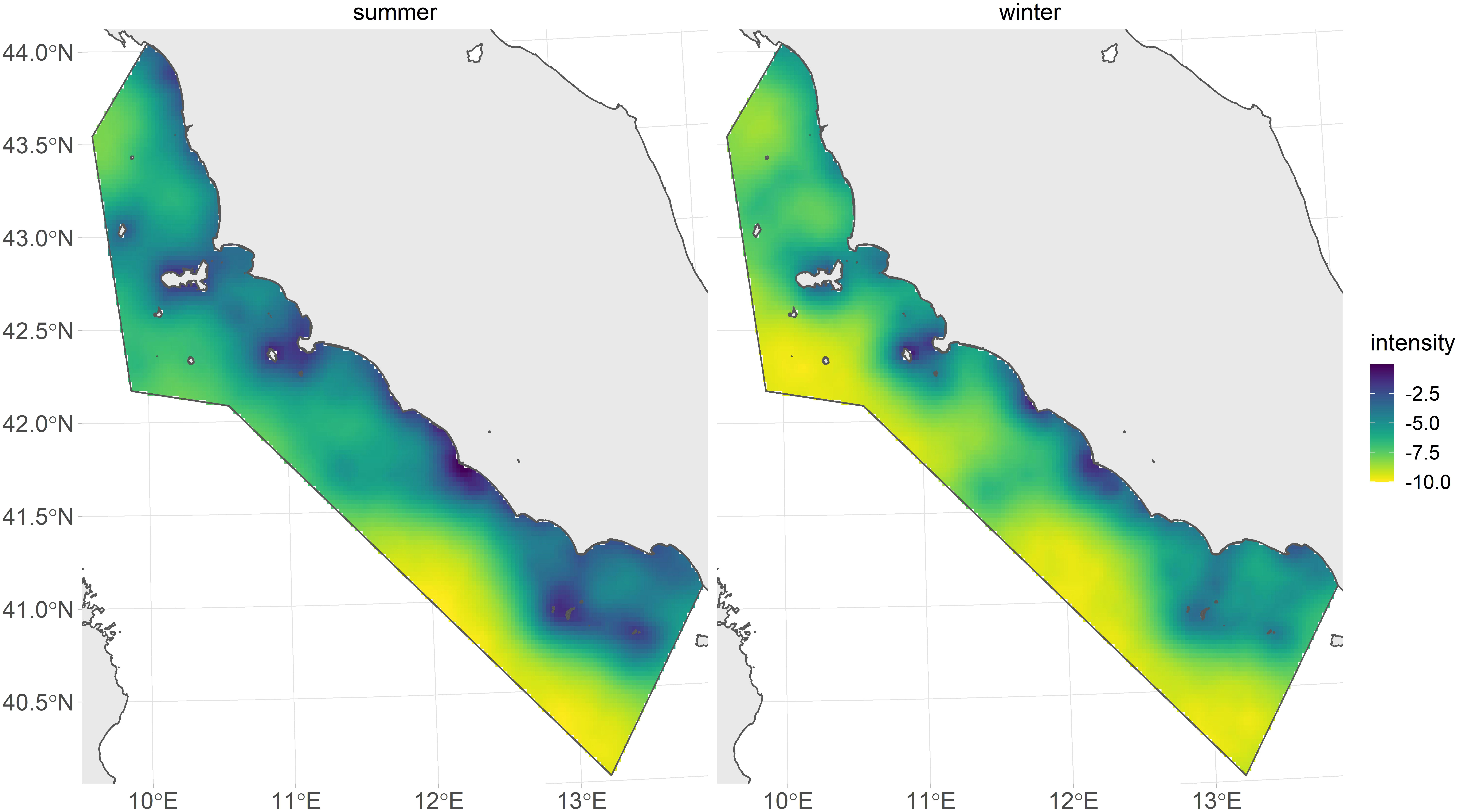
Figure 5 Estimated maps of observation process intensity used in the detection functions (summer: left panel; winter: right panel).
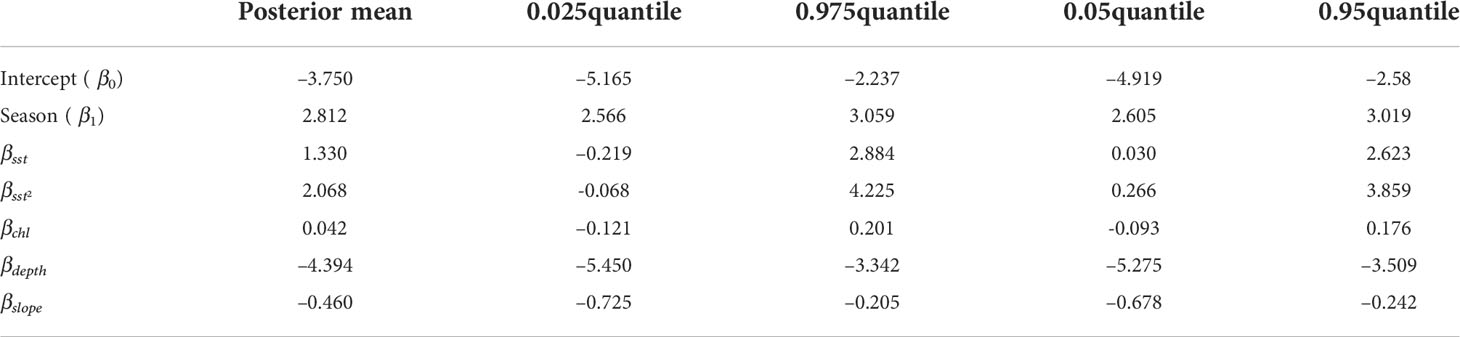
Table 2 Estimated posterior means together with 90 and 95% credible interval (CI) for the fixed effects parameters in Equation (1).
The estimated posterior mean and coefficient of variation (CV) surfaces for the intensity of the common bottlenose dolphin distribution by season are reported in Figure 6. In summer, the Tiber River estuary (nearly in the middle of the study area) showed higher intensity than other coastal regions. In winter, almost homogeneous predictions were observed, with marginal greater intensities at the two extremes of the study area (the Arno estuary in the northern part and Gaeta Gulf in the south), where lower temperatures and slightly higher chlorophyll–a concentration were observed. The relatively low variance was predicted in the more coastal parts of the study area within each season, while higher uncertainty was instead revealed in the southernmost offshore area.
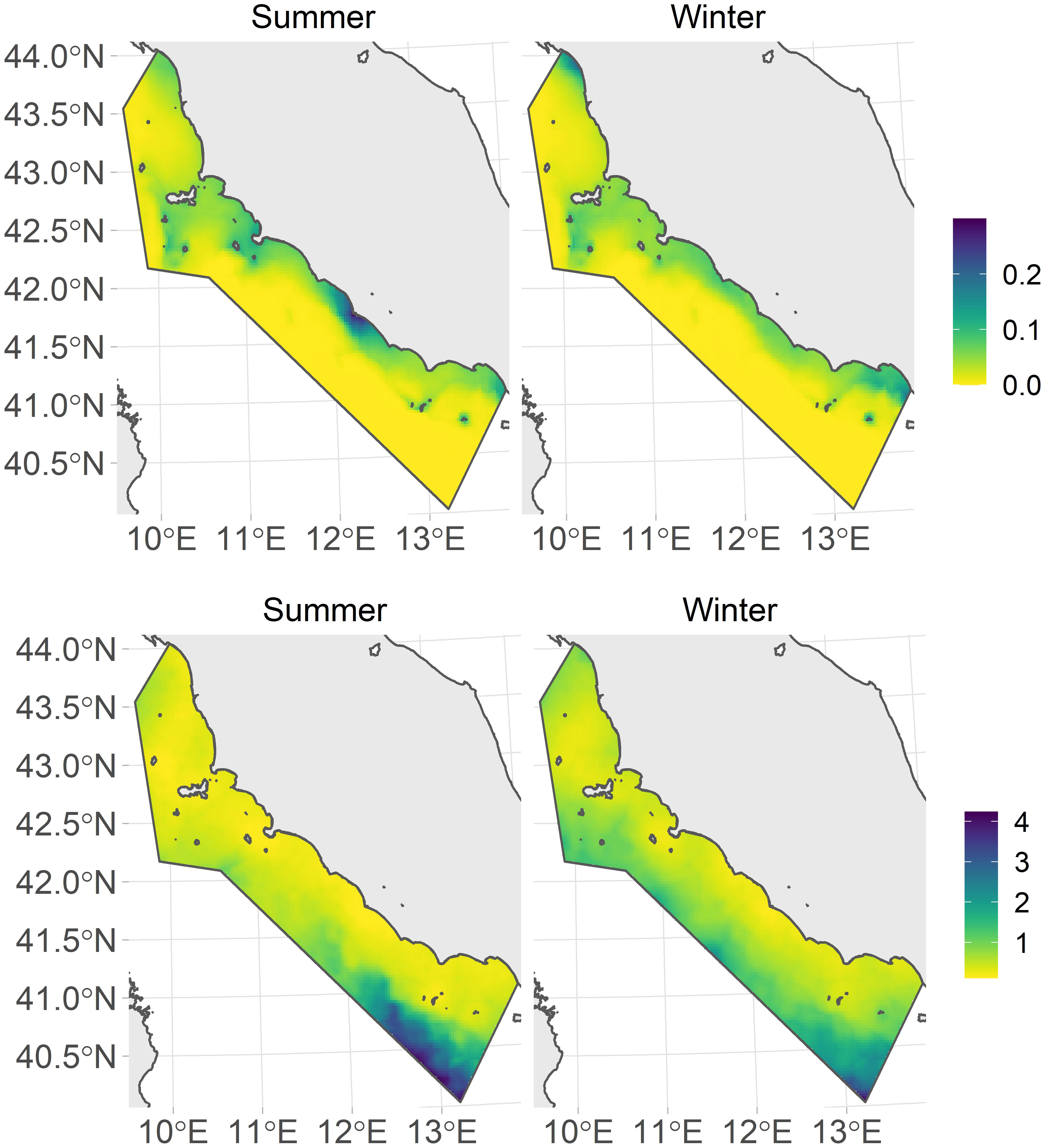
Figure 6 Posterior means of the predicted intensity surface for bottlenose dolphin distribution during the summer (upper left panel) and winter (upper right panel) seasons and the coefficient of variation (CV) for each season (summer: lower left panel; winter: lower right panel).
Finally, the distribution of the expected number of sightings by each season over the whole area, computed using Equation 3, is reported in Figure 7. While the expected number of sightings is fairly similar during summer and winter, a much larger variance is observed for the winter estimate.
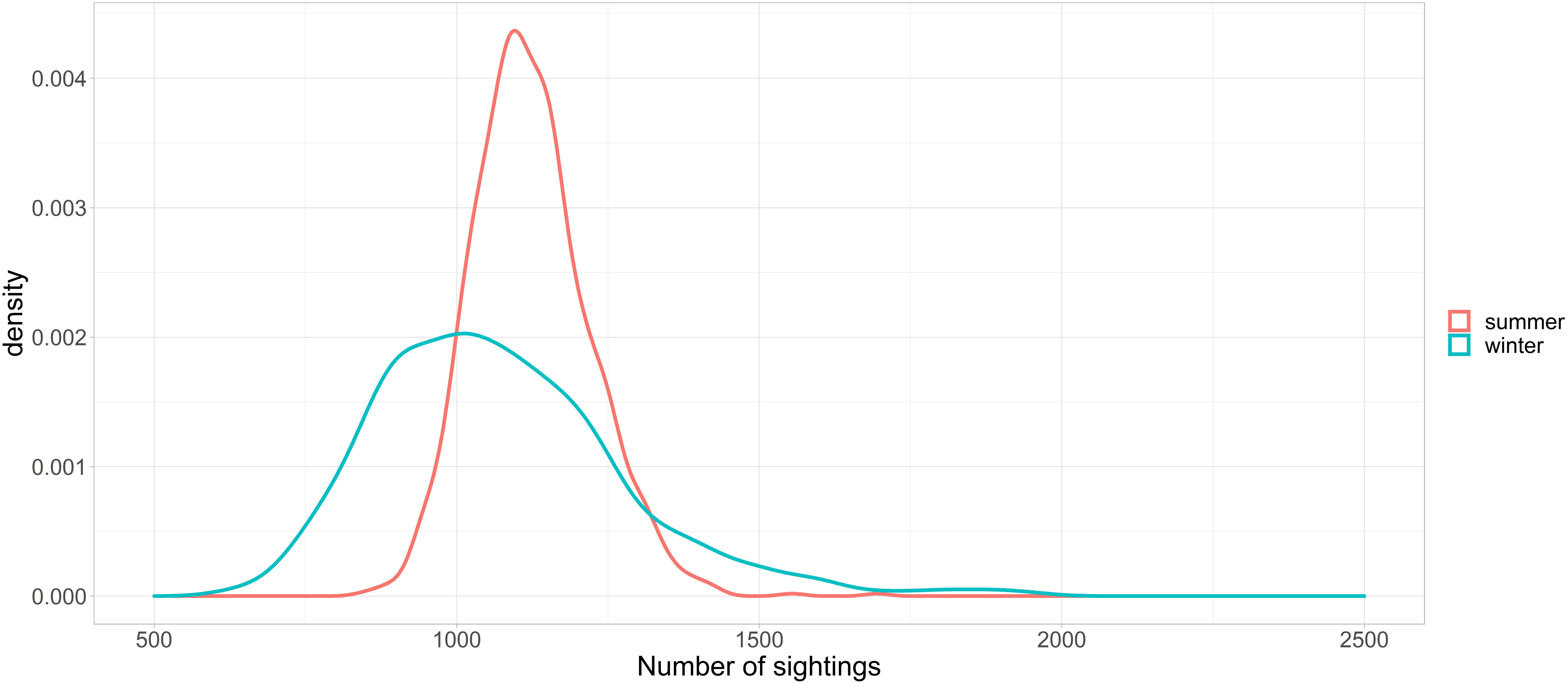
Figure 7 Distribution of the expected number of sightings over the whole area during summer (red) and winter (blue).
Discussion
The modeling approach of spatial data integration, able to carefully consider and minimize datasets biases, has been used in this study, offering a more precise picture of the seasonal common bottlenose dolphin distribution in the western Mediterranean Sea. As Dorazio (2014) pointed out, several statistical models have been proposed to integrate presence-only data from different research protocols to obtain reliable predictions of species distribution. However, these models have overlooked the effects of imperfect detectability and survey bias. Recently, Martino et al. (2021) showed that bias in these estimates, induced by multiple detection mechanisms related to data collection, could be reduced by correcting for detectability issues, thus allowing multiple sources of information to be integrated. In this study, new advancements with respect to Martino et al. (2021) were presented, testing a different approach to better define the presence–only (social media data source) detection function (see section S3 in the Supplementary Materials) and describing a possible seasonal effect thanks to a larger amount of data and a more extended study area. However, the relatively low number of sightings currently available, highly scattered in time, makes it difficult to connect to the detailed temporal pattern in the environmental covariates. The number of sightings per year seems insufficient to allow a full space-time modeling to capture possible temporal dynamics in the area.
The model here estimated includes all sources of uncertainty in one framework, allowing for a rigorous evaluation of the overall prediction uncertainty. It is known that when predicting animal distribution, uncertainty – both epistemic (i.e., the recognized ignorance due to imperfect knowledge; Brown, 2004) and stochastic (i.e., always present when dealing with nature; Walker et al., 2003) – is an unavoidable factor to deal with. This is obviously a critical point in the development of conservation and management measures, as without a proper uncertainty evaluation, weak and inefficient choices could be made (Stephenson et al., 2021). For the distribution of marine apex predators like the common bottlenose dolphin this is a crucial aspect, as these species can travel for great distances or reside in specific coastal locations. These versatile habits entail significant variations in the environmental characteristics and expose the species to a large variety of human pressures overlapping their ecologically important areas.
This study highlighted that the common bottlenose dolphin seems to well adapt to different seasonal conditions and maintain its distributional range, being constantly present along the coasts of the study area over the investigated 14-years period and showing differences in the intensities of its distribution between the two seasons (winter and summer), while the spatial pattern remain constant. A much larger variance for the winter vs summer estimates emerged, likely related to the reduced observation effort in the winter season due to bad weather conditions. Even not statistically significant, the lowering temperature and the slight rise of chlorophyll–a concentration detected in winter seem to increase the probability of dolphin presence. More specifically, the chlorophyll–a concentration was higher in two locations, near the Arno estuary in the north and the Garigliano/Volturno estuaries in the south of the study area, respectively. Being responsible for primary production, chlorophyll–a could be used as an indicator for other biotic features, such as the zooplankton distribution or plantophagous fish presence (e.g., La Manna et al., 2016). In the southern Mediterranean Sea, chlorophyll–a was found to be the second strongest predictor for bottlenose dolphin spatial distribution patterns, representing a good proxy for prey availability and thus a highly useful parameter in identifying relevant aggregation hotspots for dolphins (La Manna et al., 2016). Most likely, it is not by chance that the main estuaries in the study area were sites with the greatest predicted bottlenose dolphin densities, as estuaries have been shown to act as significant habitats for the genus Tursiops worldwide (e.g., Sprogis et al., 2016; Hartel et al., 2020). Estuaries are key aspects of the coastal ecosystems because of their unique characteristics and the variability induced by mixing and stratifying fresh and saltwater (McLusky and Elliott, 2004; Lin et al., 2013). Such processes are known to trigger fish aggregations and movements (Krumme, 2004) as they adapt to these changes, and dolphin paths as well, as they follow their prey (i.e., prey availability and distribution in turn influence common bottlenose dolphin distribution; Karczmarski et al., 2000; Soldevilla et al., 2011; Lin et al., 2013). In addition, other peculiar, fixed features of the study area such as islands, headlands and seamounts may have played a role in the constant distributional pattern over seasons here observed, as they are known to permanently generate eddies, fronts and water masses circulation, enhancing the amount of available nutrients and aggregating different species able to attract apex predators (Johnston and Read, 2007; Bailey and Thompson, 2010; Dinis et al., 2016).
Bathymetry is considered a proxy for prey availability indirectly linked to the common bottlenose dolphin distribution and habitat selection as well (Marini et al., 2015; Gnone et al., 2022). Here, bathymetry appeared to be the best predictor of the species distribution in the investigated area: the probability of sighting a group of common bottlenose dolphin increased in shallow (coastal) waters and decreased with increasing depth. This result is in accordance with the relatively consistent preferences in terms of bottom topography and water depth observed for the common bottlenose dolphin in the Mediterranean Sea (Natoli et al., 2021), although some transient occurrences in deeper waters, where uncertainty in predictions increases, were detected. Coastal waters may be more suitable habitats for common bottlenose dolphin mother–calf pairs than deeper ones, where females with newborns could form stable resident groups as observed in the study area near the Tiber River estuary by both researchers and sea users (Pace et al., 2019; Pace et al., 2021; Pace et al., 2022a; Pedrazzi et al., 2022).
Marine apex predators are, in general, highly mobile species, which raises issues in identifying their habitat boundaries for conservation actions (Cribb et al., 2015; Pace et al., 2018). The heterogeneous distribution over a wide range of habitats characterizes these species as indicators to estimate the effects of human activities on ecosystem functions (Arcangeli et al., 2015; Carlucci et al., 2016). Coastal dolphins are known to be affected by different anthropogenic threats such as bycatch, entanglement in fishing gears or marine litter, physical disturbance by shipping, unregulated dolphin watching or coastal development, anthropogenic noise, chemical contaminants, or overfishing (Natoli et al., 2021). These multiple pressures are acting in the highly anthropized study area (Gnone et al., 2022), although it encompasses several levels of spatial protection measures such as the SPAMI Pelagos Sanctuary for the protection of Marine Mammals, Marine Protected Areas, and Natura 2000 sites including the ‘Tutela del Tursiops truncatus’ − IT5160021 specifically established for protecting a recognized important site for bottlenose dolphin just off the Tuscany coast. To date, a complete assessment of species conservation status and intervention to assure the favorable status is reached or maintained is mandatory within the recently established Habitat Directive N2000 IT5160021 site (Arcangeli et al., 2021). Our findings underline the importance of the study area for the bottlenose dolphin also well outside the limit of the N2000 site and call for a reinforcement of effective mitigation measures to preserve the species especially along the whole coastal area. The results shown by our integrative modeling effort highlight the importance of using all available data to better understand the distribution of the species (Pace et al., 2014; Pace et al., 2019; Gnone et al., 2022) and, in turn, the characteristics of the marine ecosystem they are part of. Additional efforts to enlarge and reinforce the existing protective regulations in the study area are urgent priorities (to encompass at least the whole coastal area), as well as further investigations and continuous monitoring activities to identify effective mitigation actions for the local common bottlenose dolphin population.
Data availability statement
The raw data supporting the conclusions of this article will be made available by the authors, without undue reservation.
Ethics statement
Ethical review and approval was not required for the animal study because we used observational data only.
Author contributions
DSP, AA, GJ, and SMa designed the study and managed the funding acquisition. DSP and AA performed the field work. GP, SMo, GJ, and SMa analyzed data. All authors wrote the first version of the manuscript, discussed the results and implications, commented on the manuscript at all stages and contributed extensively to the work here presented.
Funding
In 2017–2018, UNIRM work was supported by OceanCare (Switzerland) and in 2019–2020 by Cooperativa Pelagos (Italy). In 2020, part of the data was collected within the framework of the “DelPHEos” project funded by the Lazio Region (Italy) within PO FEAMP 2014/2020–Misura 1.40 framework and the “JCDM” project funded by Sapienza University of Rome (Grant no. RM1201729F23D51B).
Acknowledgments
UNIRM team would like to thank the Secche di Tor Paterno (MPA) and Roma Natura for the logistic support; “I Barcaroli del Dollaro” and the local professional/recreational fishing community for their help; all students for their assistance in 2017–2021 field seasons; Giancarlo Giacomini, Giulia Pedrazzi and Margherita Silvestri for their support in data collection; Maria Cristina Gambi, Raffaella Tizzi, Caterina Lanfredi, Junio Fabrizio Borsani, Sabina Airoldi and Francesca Triossi for the constant encouragements. The dataset from ferry were collected within the FLT Med Network (ISPRA) programme with the collaboration of University of Pisa, Accademia del Leviatano, and Ketos. The authors would like to thank all the prefessional observers running the monitoring and the Corsica Sardinia and Grimaldi ferry companies hosting the researchers onboard. A special thank to Eleonora Pignata who extracted and first managed the SM data in Tuscany.
Conflict of interest
The authors declare that the research was conducted in the absence of any commercial or financial relationships that could be construed as a potential conflict of interest.
Publisher’s note
All claims expressed in this article are solely those of the authors and do not necessarily represent those of their affiliated organizations, or those of the publisher, the editors and the reviewers. Any product that may be evaluated in this article, or claim that may be made by its manufacturer, is not guaranteed or endorsed by the publisher.
Supplementary material
The Supplementary Material for this article can be found online at: https://www.frontiersin.org/articles/10.3389/fmars.2022.939692/full#supplementary-material
References
Ansong J., Gissi E., Calado H. (2017). An approach to ecosystem–based management in maritime spatial planning process. Ocean Coast. Manage. 141, 65–81. doi: 10.1016/j.ocecoaman.2017.03.005
Arcangeli A., Aissi M., Atzori F., Azzolin M., Campana I., Carosso L., et al. (2019). Fixed line transect mediterranean monitoring network (flt med net), an international collaboration for long term monitoring of macro–mega fauna and main threats fixed line transect mediterranean monitoring network. Biol. Mar. Mediterr. 26 (1), 400–401.
Arcangeli A., Crosti R., Campana I., Carosso L., Gregorietti M., Mainardi G., et al. (2021). Long–term monitoring for the surveillance of the conservation status of Tursiops truncatus in an EU Natura2000 site in the Mediterranean sea. a pilot study in the Tuscan archipelago. Mediterr. Mar. Sci. 22 (2), 340–346. doi: 10.12681/mms.24562
Arcangeli A., Orasi A., Carcassi S., Crosti C. (2015). Exploring thermal and trophic preference of balaenoptera physalus in the central tyrrhenian Sea: a new summer feeding ground? Mar. Biol. 161, 427–436. doi: 10.1007/s00227-013-2348-8
Bachl F. E., Lindgren F., Borchers D. L., Illian J. B. (2019). Inlabru: an r package for bayesian spatial modelling from ecological survey data. Methods Ecol. Evol. 10, 760–766. doi: 10.1111/2041-210X.13168
Bailey H., Thompson P. (2010). Effect of oceanographic features on fine–scale foraging movements of bottlenose dolphins. Mar. Ecol. Prog. Ser. 418, 223–233. doi: 10.3354/meps08789
Bennington S., Rayment W., Dawson S. (2020). Putting prey into the picture: improvements to species distribution models for bottlenose dolphins in doubtful sound, new Zealand. Mar. Ecol. Prog. Ser. 653, 191–204. doi: 10.3354/meps13492
Blasi M., Boitani L. (2012). Modelling fine–scale distribution of the bottlenose dolphin tursiops truncatus using physiographic features on filicudi (southern thyrrenian Sea, Italy). Endanger. Species Res. 17, 269–288. doi: 10.3354/esr00422
Bo M., Coppari M., Betti F., Massa F., Gay G., Cattaneo–Vietti R., et al. (2020). Unveiling the deep biodiversity of the janua seamount (Ligurian sea): first Mediterranean sighting of the rare Atlantic bamboo coral Chelidonisis aurantiaca stude. Deep Sea Res. Part I Oceanogr. Res. Pap. 156, 103186. doi: 10.1016/j.dsr.2019.103186
Bonizzoni S., Furey N. B., Bearzi G. (2021). Bottlenose dolphins (Tursiops truncatus) in the north-western Adriatic Sea: Spatial distribution and effects of trawling. Aquat. Conserv.: Mar. Freshw. Ecosyst. 31 (3), 635–650. doi: 10.1002/aqc.3433
Bonizzoni S., Hamilton S., Reeves R. R., Genov T., Bearzi G. (2022). Odontocete cetaceans foraging behind trawlers, worldwide. Rev. Fish Biol. Fish. 32, 827–877. doi: 10.1007/s11160-022-09712-z
Borrell A., Vighi M., Genov T., Giovos I., Gonzalvo J. (2021). Feeding ecology of the highly threatened common bottlenose dolphin of the gulf of ambracia, Greece, through stable isotope analysis. Mar. Mamm. Sci. 37 (1), 98–110. doi: 10.1111/mms.12725
Brown J. D. (2004). Knowledge, uncertainty and physical geography: towards the development of methodologies for questioning belief. Trans. Inst. Br. Geogr. 29 (3), 367–381. doi: 10.1111/j.0020-2754.2004.00342.x
Carlucci R., Fanizza C., Cipriano G., Paoli C., Russo T., Vassallo P. (2016). Modeling the spatial distribution of the striped dolphin (Stenella coeruleoalba) and common bottlenose dolphin (Tursiops truncatus) in the gulf of taranto (Northern Ionian Sea, central–eastern Mediterranean Sea). Ecol. Indic. 69, 707–721. doi: 10.1016/j.ecolind.2016.05.035
Carmen M., Berrow S. D., O’Brien J. M. (2021). Foraging behavior of bottlenose dolphins in the Shannon estuary, Ireland as determined through static acoustic monitoring. J. Mar. Sci. Eng. 9, 275. doi: 10.3390/jmse9030275
Carnabuci M., Schiavon G., Bellingeri M., Fossa F., Paoli C., Vassallo P., et al. (2016). Connectivity in the network macrostructure of Tursiops truncatus in the pelagos sanctuary (NW Mediterranean sea): does landscape matter? Popul. Ecol. 58 (2), 249–264. doi: 10.1007/s10144-016-0540-7
Cribb N., Miller C. E., Seuront L. (2015). Towards a standardized approach of cetacean habitat: past achievements and future directions. Open J. Mar. Sci. 5, 335–357. doi: 10.4236/ojms.2015.53028
Diaz Lopez B. (2019). “Hot deals at sea”: responses of a top predator (Bottlenose dolphin, Tursiops truncatus) to human-induced changes in the coastal ecosystem. Behav. Ecol. 30 (2), 291–300. doi: 10.1093/beheco/ary162
Dinis A., Alves F., Nicolau C., Ribeiro C., Kaufmann M., Cañadas A., et al. (2016). Bottlenose dolphin Tursiops truncatus group dynamics, site fidelity, residency and movement patterns in the Madeira archipelago (North–East Atlantic). Afr. J. Mar. Sci. 38 (2), 151–160. doi: 10.2989/1814232X.2016.1167780
Dorazio R. M. (2014). Accounting for imperfect detection and survey bias in statistical analysis of presence-only data. Glob. Ecol. Biogeogr. 23 (12), 1472–1484. doi: 10.1111/geb.12216
Forcada J. (2018). “Distribution,” in Encyclopedia of marine mammals. Eds. Wursig B., Thewissen J. G. M., Kovacs K. (London: Elsevier), 259–262.
Giannoulaki M., Markoglou E., Valavanis V. D., Alexiadou P., Cucknell A., Frantzis A. (2017). Linking small pelagic fish and cetacean distribution to model suitable habitat for coastal dolphin species, Delphinus delphis and Tursiops truncatus, in the Greek seas (Eastern Mediterranean). Aquat. Conserv.: Mar. Freshw. Ecosyst. 27 (2), 436–451. doi: 10.1002/aqc.2669
Giménez J., Marçalo A., Ramírez F., Verborgh P., Gauffier P., Esteban R., et al. (2017). Diet of bottlenose dolphins (Tursiops truncatus) from the gulf of cadiz: insights from stomach content and stable isotope analyses. PloS One 12 (9), e0184673. doi: 10.1371/journal.pone.0184673
Gnone G., Bellingeri M., Dhermain F., Dupraz F., Nuti S., Bedocchi D., et al. (2011). Distribution, abundance, and movements of the bottlenose dolphin (Tursiops truncatus) in the pelagos sanctuary MPA (north-west Mediterranean Sea). Aquat. Conserv.: Mar. Freshw. Ecosyst. 21 (4), 372–388. doi: 10.1002/aqc.1191
Gnone G., Bellingeri M., Molinari Y., Dhermain F., Labach H., Díaz López B., et al. (2022). The seabed makes the dolphins. physiographic features shape the size and structure of the bottlenose dolphin geographical units. J. Mar. Sci. Eng. 10, 1036. doi: 10.3390/jmse10081036
Greller R., Mazzoli M., Titcomb E., Nelson B., Paperno R., Markwith S. H. (2021). Environmental drivers of habitat use by common bottlenose dolphins (Tursiops truncatus) in the Indian river lagoon, Florida, USA. Mar. Mamm. Sci. 37 (2), 512–532. doi: 10.1111/mms.12767
Hammerschlag N., Schmitz O. J., Flecker A. S., Lafferty K. D., Sih A., Atwood T. B., et al. (2019). Ecosystem function and services of aquatic predators in the anthropocene. Trends Ecol. Evol. 34 (4), 369–383. doi: 10.1016/j.tree.2019.01.005
Hartel E. F., Durden W. N., O’Corry–Crowe G. (2020). Testing satellite telemetry within narrow ecosystems: nocturnal movements and habitat use of bottlenose dolphins within a convoluted estuarine system. Anim. Biotelemetry 8 (1), 1–16. doi: 10.1186/s40317-020-00200-4
Haughey R., Hunt T. N., Hanf D., Passadore C., Baring R., Parra G. J. (2021). Distribution and habitat preferences of indo-pacific bottlenose dolphins (Tursiops aduncus) inhabiting coastal waters with mixed levels of protection. Front. Mar. Sci. 8. doi: 10.3389/fmars.2021.617518
Heithaus M. R., Frid A., Wirsing A. J., Worm B. (2008). Predicting ecological consequences of marine top predator declines. TREE 23 (4), 202–210. doi: 10.1016/j.tree.2008.01.003
Hijmans R. J. (2022) Terra: Spatial data analysis. Available at: https://CRAN.R-project.org/package=terra.
ISPRA (2016). Fixed line transect monitoring using ferries as platform of observation for marine mega and macro fauna and main threats. monitoring protocol for cetaceans and sea turtles. – ISPRA agreement – technical annex 1 (ISPRA), 19. Available at: https://www.isprambiente.gov.it/files2021/progetti/technical-annex-i_monitoring-protocol_2015.pdf
IUCN-MMPATF (2017) Campanian and pontino archipelagos IMMA. Available at: https://drive.google.com/file/d/0BxEdFMHwpjLLa1ZKMkNrYnhldlk/view.
Johnston D. W., Read A. J. (2007). Flow-field observations of a tidally driven island wake used by marine mammals in the bay of fundy, Canada. Fish. Oceanogr. 16 (5), 422–435. doi: 10.1111/j.1365-2419.2007.00444.x
Karczmarski L., Cockcroft V. G., Mclachlan A. (2000). Habitat use and preferences of indo-pacific humpback dolphins Sousa chinensis in algoa bay, south Africa. Mar. Mammal Sci. 16 (1), 65–79. doi: 10.1111/j.1748-7692.2000.tb00904.x
Kiszka J. J., Woodstock M. S., Heithaus M. R. (2022). Functional roles and ecological importance of small cetaceans in aquatic ecosystems. Front. Mar. Sci. 9, 163. doi: 10.3389/fmars.2022.803173
Krumme U. (2004). Patterns in tidal migration of fish in a Brazilian mangrove channel as revealed by a split–beam echosounder. Fish. Res. 70 (1), 1–15. doi: 10.1016/j.fishres.2004.07.004
Labach H., Azzinari C., Barbier M., Cesarini C., Daniel B., David L., et al. (2022). Distribution and abundance of common bottlenose dolphin (Tursiops truncatus) over the French Mediterranean continental shelf. Mar. Mamm. Sci. 38 (1), 212–222. doi: 10.1111/mms.12874
La Manna G., Ronchetti F., Sarà G. (2016). Predicting common bottlenose dolphin habitat preference to dynamically adapt management measures from a marine spatial planning perspective. Ocean Coast. Manage. 130, 317–327. doi: 10.1016/j.ocecoaman.2016.07.004
Laran S., Pettex E., Authier M., Blanck A., David L., Dorémus G., et al. (2017). Seasonal distribution and abundance of cetaceans within French waters – part I: The north–Western Mediterranean, including the pelagos sanctuary. Deep–Sea Res. II: Top. Stud. Oceanogr. 141, 20–30. doi: 10.1016/j.dsr2.2016.12.011
Lin T. H., Akamatsu T., Chou L. S. (2013). Tidal influences on the habitat use of indo–pacific humpback dolphins in an estuary. Mar. Biol. 160 (6), 1353–1363. doi: 10.1007/s00227-013-2187-7
Marini C., Fossa F., Paoli C., Bellingeri M., Gnone G., Vassallo P. (2015). Predicting bottlenose dolphin distribution along liguria coast (northwestern Mediterranean Sea) through different modeling techniques and indirect predictors. J. Environ. Manage. 150, 9–20. doi: 10.1016/j.jenvman.2014.11.008
Martino S., Pace D. S., Moro S., Casoli E., Ventura D., Frachea A., et al. (2021). Integration of presence-only data from several sources: a case study on dolphins’ spatial distribution. Ecography 44 (10), 1533–1543. doi: 10.1111/ecog.05843
McLusky D. S., Elliott M. (2004). The estuarine ecosystem: ecology, threats and management (Oxford: OUP).
Natoli A., Genov T., Kerem D., Gonzalvo J., Holcer D., Labach H., et al. (2021) Tursiops truncatus (Mediterranean subpopulation) (The IUCN Red List of Threatened Species 2021) (Accessed 22 July 2022).
Nelms S. E., Alfaro-Shigueto J., Arnould J. P., Avila I. C., Nash S. B., Campbell E., et al. (2021). Marine mammal conservation: over the horizon. Endang. Species Res. 44, 291–325. doi: 10.3354/esr01115
Pace D. S., Arcangeli A., Mussi B., Vivaldi C., Ledon C., Lagorio S., et al. (2018). Habitat suitability modeling in different sperm whale social groups. J. Wildl. Manage. 82 (5), 1062–1073. doi: 10.1002/jwmg.21453
Pace D. S., Di Marco C., Giacomini G., Ferri S., Silvestri M., Papale E., et al. (2021). Capitoline dolphins: Residency patterns and abundance estimate of Tursiops truncatus at the Tiber river estuary (Mediterranean Sea). Biology 10 (4), 275. doi: 10.3390/biology10040275
Pace D. S., Ferri S., Giacomini G., Di Marco C., Papale E., Silvestri M., et al. (2022a). Resources and population traits modulate the association patterns in the common bottlenose dolphin living nearby the Tiber river estuary (Mediterranean Sea). Front. Mar. Sci 9, 935235. doi: 10.3389/fmars.2022.935235
Pace D. S., Giacomini G., Campana I., Paraboschi M., Pellegrino G., Silvestri M., et al. (2019). An integrated approach for cetacean knowledge and conservation in the central Mediterranean Sea using research and social media data sources. Aquat. Conserv.: Mar. Freshw. Ecosyst. 29 (8), 1302–1323. doi: 10.1002/aqc.3117
Pace D. S., Mussi B., Wurtz M., Gordon J. D. C. (2014). Foreword. Aquat. Conserv.: Mar. Freshw. Ecosyst. 24, 1–3. doi: 10.1002/aqc.2457
Pace D. S., Pulcini M., Triossi F. (2012). Anthropogenic food patches and association patterns of Tursiops truncatus at lampedusa island, Italy. Behav. Ecol. 23 (2), 254–264. doi: 10.1093/beheco/arr180
Pace D. S., Tumino C., Silvestri M., Giacomini G., Pedrazzi G., Pavan G., et al (2022b). Bray-Call Sequences in the Mediterranean Common Bottlenose Dolphin (Tursiops truncatus) Acoustic Repertoire. Biology 11 (3), 367. doi: 10.3390/biology11030367
Papale E., Alonge G., Caruso F., Grammauta R., Mazzola S., Mussi B., et al(2021). The higher, the closer, the better? influence of sampling frequency and distance on the acoustic properties of short-beaked common dolphins burst pulses in the Mediterranean Sea. Aquat. Conserv. Mar. Freshw. Ecosyst. 31, 51–60. doi: 10.1002/aqc.3158
Pedrazzi G., Giacomini G., Pace D. S. (2022). First report of epimeletic and acoustic behaviour in Mediterranean common bottlenose dolphins (Tursiops truncatus) carrying dead calves. Biology 11 (2), 337. doi: 10.3390/biology11020337
Pleslić G., Rako-Gospić N., Miočić-Stošić J., Blazinić Vučur T., Radulović M., Mackelworth P., et al. (2019). Social structure and spatial distribution of bottlenose dolphins (Tursiops truncatus) along the Croatian Adriatic coast. Aquat. Conserv.: Mar. Freshw. Ecosyst. 29 (12), 2116–2132. doi: 10.1002/aqc.3213
Pulcini M., Pace D. S., La Manna G., Triossi F., Fortuna C. M. (2014). Distribution and abundance estimates of bottlenose dolphins (Tursiops truncatus) around lampedusa island (Sicily channel, italy): implications for their management. J. Mar. Biolog. Assoc. UK 94 (6), 1175–1184. doi: 10.1017/S0025315413000842
Renner I. W., Elith J., Baddeley A., Fithian W., Hastie T., Phillips S. J., et al. (2015). Point process models for presence-only analysis. Methods Ecol. Evol. 6, 366–379. doi: 10.1111/2041-210X.12352
Ricevuto E., Tognotti M., Trombetti C. (2011). Scientific activities’ programme: monitoring species and surveys on anthropogenic impacts on marine–coastal habitats. Biol. Mar. Medit. 18, 159–160.
Soldevilla M. S., Wiggins S. M., Hildebrand J. A., Oleson E. M., Ferguson M. C. (2011). Risso’s and pacific white–sided dolphin habitat modeling from passive acoustic monitoring. Mar. Ecol. Prog. Ser. 423, 247–260. doi: 10.3354/meps08927
Sprogis K. R., Raudino H. C., Rankin R., MacLeod C. D., Bejder L. (2016). Home range size of adult indo-pacific bottlenose dolphins (Tursiops aduncus) in a coastal and estuarine system is habitat and sex-specific. Mar. Mamm. Sci. 32 (1), 287–308. doi: 10.1111/mms.12260
Steneck R. S. (2012). Apex predators and trophic cascades in large marine ecosystems: learning from serendipity. PNAS 109 (21), 7953–7954. doi: 10.1073/pnas.1205591109
Stephenson F., Hewitt J. E., Torres L. G., Mouton T. L., Brough T., Goetz K. T., et al. (2021). Cetacean conservation planning in a global diversity hotspot: dealing with uncertainty and data deficiencies. Ecosphere 12 (7), e03633. doi: 10.1002/ecs2.3633
Triossi F., Willis T. J., Pace D. S. (2013). Occurrence of bottlenose dolphins Tursiops truncatus in natural gas fields of the northwestern Adriatic Sea. Mar. Ecol. 34 (3), 373–379. doi: 10.1111/maec.12020
Vassallo P., Marini C., Paoli C., Bellingeri M., Dhermain F., Nuti S., et al. (2020). Species-specific distribution model may be not enough: The case study of bottlenose dolphin (Tursiops truncatus) habitat distribution in pelagos sanctuary. Aquat. Conserv.: Mar. Freshw. Ecosyst. 30 (8), 1689–1701. doi: 10.1002/aqc.3366
Walker W. E., Harremoës P., Rotmans J., van der Sluijs J. P., Van Asselt M. B., Janssen P., et al. (2003). Defining uncertainty: a conceptual basis for uncertainty management in model–based decision support. Integr. Assess. 4 (1), 5–17. doi: 10.1076/iaij.4.1.5.16466
Warton D. I., Shepherd L. C. (2010). Poisson point process models solve the “pseudo-absence problem” for presence-only data in ecology. Ann. Appl. Stat. 4, 1383–1402. doi: 10.1214/10-AOAS331
Watson J., Joy R., Tollit D., Thornton S. J., Auger–Méthé M. (2019). Estimating animal utilization distributions from multiple data types: a joint spatio–temporal point process framework. arXiv preprint arXiv 1911, 00151. doi: 10.48550/arXiv.1911.00151
Wells R. S., Natoli A., Braulik G. (2019) Tursiops truncatus (errata version published in 2019) (The IUCN Red List of Threatened Species 2019) (Accessed 27 July 2022).
Wells R. S., Scott M. D. (2018). “Bottlenose dolphin, tursiops truncatus, common bottlenose dolphin,” in Encyclopedia of marine mammals. Eds. Wursig B., Thewissen J. G. M., Kovacs K. (London: Elsevier), 118–125.
Würtz M., Rovere M. (2015). Atlas of the Mediterranean seamounts and seamount–like structures (Gland, Switzerland: IUCN).
Yuan Y., Bachl F. E., Lindgren F., Borchers D. L., Illian J. B., Buckland S. T., et al. (2017). Point process models for spatio–temporal distance sampling data from a large–scale survey of blue whales. Ann. Appl. Stat. 11, 2270–2297. doi: 10.1214/17-AOAS1078
Keywords: distribution modeling, Spatial Log–Gaussian Cox Process, uncertainty, common bottlenose dolphin, Mediterranean Sea, conservation, ecology
Citation: Pace DS, Panunzi G, Arcangeli A, Moro S, Jona-Lasinio G and Martino S (2022) Seasonal distribution of an opportunistic apex predator (Tursiops truncatus) in marine coastal habitats of the Western Mediterranean Sea. Front. Mar. Sci. 9:939692. doi: 10.3389/fmars.2022.939692
Received: 09 May 2022; Accepted: 25 August 2022;
Published: 15 September 2022.
Edited by:
Maritza Sepulveda, Universidad de Valparaiso, ChileReviewed by:
Bruno Díaz López, Bottlenose Dolphin Research Institute (BDRI), Spain;Ding Zhang, University of Michigan, United StatesCopyright © 2022 Pace, Panunzi, Arcangeli, Moro, Jona-Lasinio and Martino. This is an open-access article distributed under the terms of the Creative Commons Attribution License (CC BY). The use, distribution or reproduction in other forums is permitted, provided the original author(s) and the copyright owner(s) are credited and that the original publication in this journal is cited, in accordance with accepted academic practice. No use, distribution or reproduction is permitted which does not comply with these terms.
*Correspondence: Sara Martino, c2FyYS5tYXJ0aW5vQG50bnUubm8=
†These authors share first authorship
‡These authors share senior authorship