- 1School of Architecture and the Built Environment, KTH Royal Institute of Technology, Stockholm, Sweden
- 2Department of Geosciences, Virginia Tech, Blacksburg, VA, United States
- 3Deutsches Geodätisches Forschungsinstitut der Technischen Universität München, Munich, Germany
Satellite altimetry, satellite gravimetry, and in-situ subsurface salinity and temperature profiles are used to investigate the total, barystatic, and steric sea level variations in the Baltic Sea, respectively. To estimate the steric sea level, the density variations are weighted in deeper layers to prevent overestimation of their contribution. We show that the sum of barystatic and steric components exhibits excellent cross correlation (0.9) with satellite altimetry sea level variations and also explains up to 84% of total signal variability from 2002 to 2019. Considering the dominance of barystatic sea level variations in the basin and the limitation of satellite gravimetry in resolving the mass change in water-land transition zones (known as the leakage problem), the mismatch is likely attributed to the inadequate accuracy of the barystatic datasets. The total sea level and its contributors are further decomposed into seasonal, interannual, and decadal temporal components. It is shown that despite its insignificant contributions to seasonal and interannual changes, the steric sea level plays an important role in decadal variations. Additionally, we show that the interannual variations of the barystatic sea level are governed by the North Atlantic Oscillation in the basin. The sea level variation in the North Sea is also examined to deduce the water exchange patterns on different time scales. A drop in the North Sea level can be seen from 2005 to 2011 which is followed by the Baltic Sea level with a ~3-year lag, implying the outflow from the Baltic Sea to the North Sea.
Introduction
The need for a better understanding of regional drivers and patterns of sea level signifies as climate change shows severer impacts. To this extent, space-borne observations are now providing us with reliable data sets for the last three decades. Satellite altimetry and satellite gravimetry missions are two of these sources that measure the total and barystatic sea level changes, respectively. The barystatic sea level is the mass component of the sea level, representing the change in the hydrostatic pressure above the ocean bottom (Gregory et al., 2019; Dobslaw et al., 2020). The difference in observation systems of these two space-borne techniques makes them complementary tools to conduct analyses, draw conclusions, and validate their results (Barnoud et al., 2021).
The Baltic Sea is a semi-enclosed shallow basin in northern Europe and is connected to the North Sea through the Danish Strait. Sea level variation of the basin has rather complex dynamics due to various factors, e.g., water exchange with the North Sea (Ekman and Mäkinen, 1996; Gustafsson, 1997; Passaro et al., 2015), the influence of the North Atlantic Oscillation (Andersson, 2002; Yan et al., 2004; Hünicke et al., 2015), the north-south gradient because of the salinity difference (Meier and Kauker, 2003; Kniebusch et al., 2019), presence of seasonal sea ice in the Gulf of Bothnia (Omstedt et al., 2004; Kudryavtseva and Soomere, 2016; Kudryavtseva and Soomere, 2017), and substantial land uplift due to Glacial Isostatic Adjustment (GIA; Richter et al., 2012; Madsen et al., 2019; Vestøl et al., 2019). The fact that a major part of the Baltic Sea coasts is safe from sea level rise, thanks to land uplift, does not obviate the need for an in-depth analysis of the contributors to sea level change in the basin. As such, various investigations have been carried out to understand the dynamics and shed light on the role of different drivers in sea level variation of the Baltic Sea (all references hitherto and Hünicke and Zorita, 2006; Karabil et al., 2017; Karabil et al., 2018; Gräwe et al., 2019).
North Atlantic Oscillation (NAO) is a major driver of the sea level variation in the Baltic Sea. Several studies (Andersson, 2002; Yan et al., 2004; Hünicke et al., 2015; Xu et al., 2015; Karabil et al., 2018; Passaro et al., 2021) have demonstrated its impact on different time scales. Dangendorf et al. (2014) and Gräwe et al. (2019) report that the influence of NAO on sea level variations of the Baltic Sea is through weakening/strengthening westerly winds which leads to outflow/inflow to/from the North Sea. Passaro et al. (2021) show that NAO in the Baltic Sea is responsible for 1) the general variability of the sea level on the full-basin scale excluding the area close to the Danish Strait, and 2) the sea level gradient between the south-west and the north of the basin. They demonstrate that this is mainly due to winter conditions, where the strength and direction of the dominant wind pattern are driven by the NAO phase. Additionally, they report that, on a timescale of a couple of decades, such a pattern also translates into different sea level trends depending on the sub-basin. Hünicke and Zorita (2006; 2008) and Lehmann et al. (2011) indicate that NAO controls the precipitation regime in the basin and impacts freshwater input from surrounding catchments.
The contributions to sea level change are generally divided into three categories of atmospheric surface pressure, ocean bottom pressure, and steric elements (Dangendorf et al., 2014). Here, we do not discuss the role of GIA; its impact is removed from the datasets. Elaborate investigations about GIA can be found in Tamisiea and Mitrovica (2011). When analysing the contribution of bottom pressure, we assume that it is completely due to the barystatic sea level variations (i.e., the contribution of seabed deformation is negligible). Even though the GIA causes changes in the seabed close to the coastal area, this change is linear in the time frame of this study. Thus, it does not affect the results of this study since the linear trend is not within the scope of this study.
The datasets of Gravity Recovery And Climate Experiment (GRACE; Tapley et al., 2004) from 2002 to 2017 and its follow-on mission (GRACE-FO; Landerer et al., 2020) since mid-2018 are used. The average depth of the basin is 55m; this shallowness leads to the dominance of barystatic sea level in the basin as shown in Virtanen et al. (2010) and Dangendorf et al. (2021). Salinity and temperature profiles have been collected in the Baltic Sea since the 1970s but only sporadically until 1995. Since then, these in-situ observations are measured almost regularly. Satellite altimetry data is employed to quantify the total sea level. Unlike tide gauge data, satellite altimetry data are not prone to any vertical land movement and they have the advantage of better spatial coverage compared to locally collected sea level measurements by tide gauges. The satellite altimetry measurements of the basin are meticulously reprocessed (Passaro et al., 2021). The need for reprocessing stems from the rugged coasts, numerous small islands, and the presence of sea ice in the northern part of the basin in the winter seasons.
There has been only one attempt to discretise the components of sea level to steric and barystatic in the Baltic Sea. Virtanen et al. (2010) analysed 5-year data of GRACE, tide gauges, and two hydrodynamic models for the basin. They concluded that 110 mm and 140 mm of residual root mean square (RMS) for two GRACE datasets and around 70% of observed variance by tide gauge could be explained by these datasets. The role of the steric sea level is neglected in this study because of its small amplitude. They did not extend their results to the linear trend as it is not possible to render a meaningful result considering the basin size and shape as well as the versatility of the GRACE signal in such a basin. Royston et al. (2020) concluded that the sea level trend budget closure cannot be established on regional scales with the current GRACE dataset.
We used satellite altimetry, satellite gravimetry, and in situ temperature and salinity profiles to examine the role of steric and barystatic sea levels on seasonal, interannual, and decadal time scales. Here, we seek answers to two questions: 1) what is the role of steric and barystatic contributors to sea level change in the basin on different temporal scales? and 2) How do these changes relate to the sea level variation in the North Sea and to the NAO index? The accuracy of the barystatic datasets is also investigated by comparing them to the total sea level provided by satellite altimetry.
Data and steric sea level estimation
Datasets for total, barystatic, and steric sea levels are acquired through three different methods of observation. Satellite altimetry uses a pointwise approach to measure the range between the satellite and the sea surface. GRACE/GRACE-FO consisted of tandem satellites in which the distance between them is used to estimate the surface mass change in the Earth system with an effective spatial resolution of ~300 km and monthly sampling. Salinity and temperature profiles collected to a certain depth compose the dataset to estimate the density variations and hence, the steric sea level in the basin. The first two datasets are ready-to-use, however, the latter one needs processing tailored to the basin. All time series are subtracted by their means from 2004 to 2010.
Two salinity and temperature datasets are evaluated for steric sea level estimations, in-situ observations from three stations in the Baltic Proper, and profiles from a reanalysis model over the whole basin. Detailed information regarding the estimation process and results using two datasets are provided in Supplementary Materials. Even though both datasets lead to similar results in the analyses of the next section, the steric sea level estimated by observations shows slightly better compatibility with total sea level when it is added to the barystatic sea level. Thus, we used it in the rest of the study (Figure 1B).
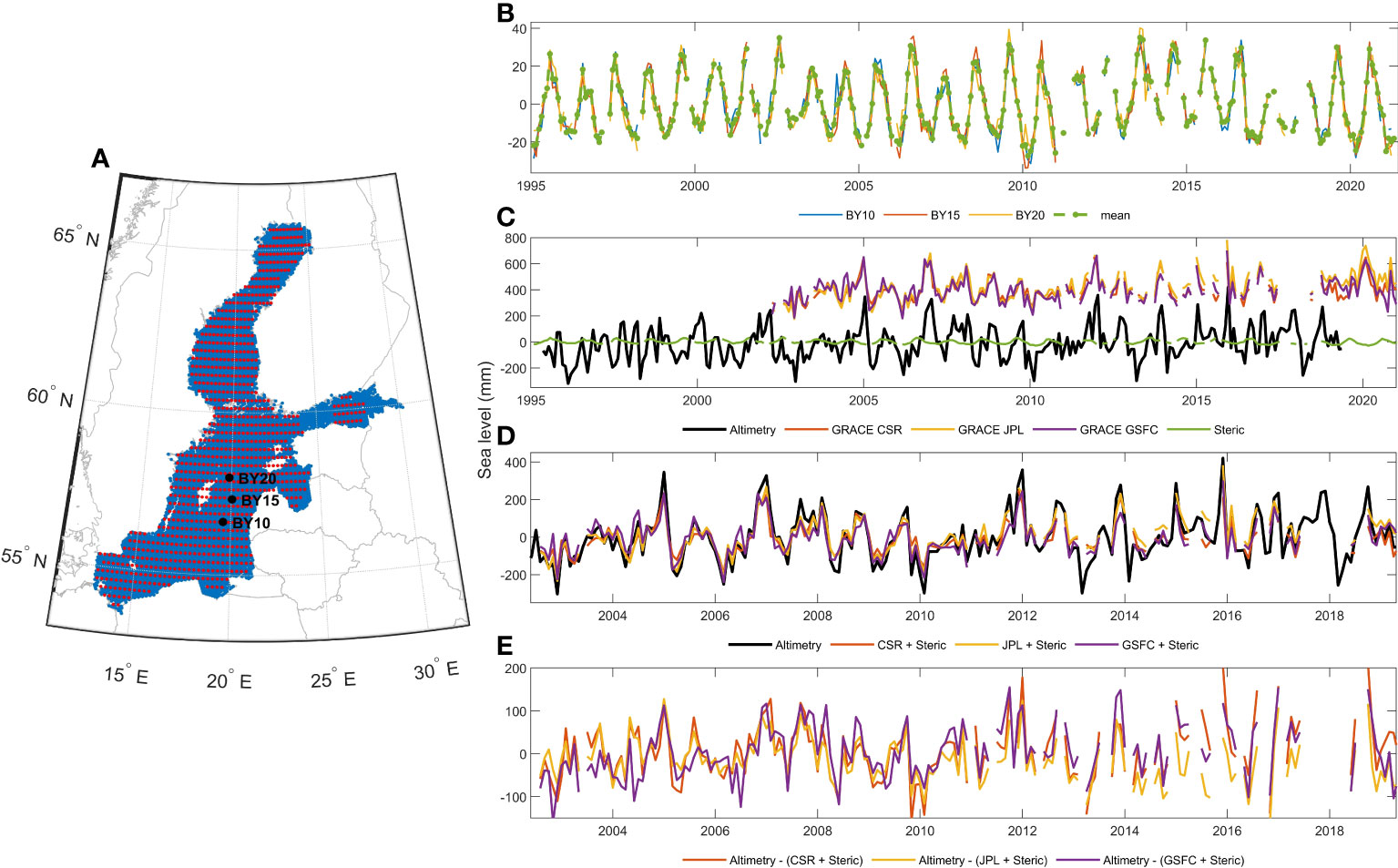
Figure 1 Datasets used in the study: (A) The extent of altimetry (blue), GRACE CSR (red), and location of the stations for subsurface salinity and temperature data. (B) Estimated steric sea level from three stations. (C) Total sea level (altimetry), barystatic (GRACE mascons from CSR, JPL, GSFC), and steric sea level. The barystatic data is shifted by arbitrary value for visual presentation. (D) Comparison of total sea level (altimetry) to sum of contributors (Eq. 1) and, (E) Residuals (ϵ) of Eq. 1.
The satellite altimetry data for the basin are acquired from the output of the Baltic SEAL project (Passaro et al., 2021). This project aimed at reprocessing the altimetry data collected in the basin from 1995 to 2019. Measurements from nine satellite altimetry missions (Topex/Poseidon, Jason-1, Jason-2, ERS-2, Envisat, SARAL, Cryosat-2, Sentinel-3A, Sentinel-3B) are retracked, corrected by geophysical and standard corrections, and gridded for the basin. This dataset was extensively validated against tide gauges and showed the best performances in terms of the agreement in trend estimation compared to other global datasets such as the Copernicus Marine Environment Monitoring Service (CMEMS) service and the European Space Agency’s Sea Level Climate Change Initiative (Passaro et al., 2021, Figure 2 of the reference). Compared to the global dataset, Baltic SEAL has the advantage of a large number of additional sea level estimations retrieved close to the coast and from leads among sea ice, which are typically absent or not correctly retrieved in standard altimetry products (Benveniste et al., 2019; Quartly et al., 2019). Since this study is focused on basin-scale variations, the gridded time series are averaged to form a single time series (Figures 1A, C). The satellite altimetry products of a similar project conducted over the North Sea are also used for investigating the water exchange between basins (not shown). Similar methods to the Baltic SEAL are used for this project (Dettmering et al., 2021). The time series for the North Sea is multiplied by 2.5 to scale the water volume with the Baltic Sea since we use the average time series for each basin.
Three mass concentration (mascon) solutions of GRACE/GRACE-FO data processed by CSR – University of Texas at Austin, NASA Jet Propulsion Laboratory (JPL), and NASA Goddard Space Flight Center (GSFC) are used to study the barystatic sea level variations in the Baltic Sea. The mascon solutions use spatial constraints to reduce the noise in the data which makes it unnecessary to apply a posteriori filtering/smoothing like that used in spherical harmonic solutions. Moreover, the spatial constraints used in mascon solutions minimize the leakage between land and sea/ocean mass change signal, which results in resolving the mass-driven sea level changes with higher accuracy compared to the spherical harmonic solutions. From herein, the solutions for different centres will be denoted as CSR (Save et al., 2016; Save, 2020), JPL (Watkins et al., 2015; Landerer et al., 2020), and GSFC (Loomis et al., 2019). The data points within the basin are separated and averaged to create the mean time series for each dataset (Figures 1A, D). Unfortunately, there is a gap between GRACE and GRACE-FO missions, causing a ~ 1-year discontinuity in the time series from mid-2017 to mid-2018.
The coarse spatial resolution and leakage effect (between sea and land signals) are two of the well-known limitations of the GRACE data for regional studies. The recent advancement in GRACE data processing (e.g., Watkins et al., 2015) has improved the accuracy and spatial resolution of GRACE data and reduced the leakage effect, enabling GRACE to be used for monitoring the mass change in relatively small seas. For instance, Loomis and Luthcke (2017) and Feng et al. (2014) used GRACE data for the Red Sea which is as narrow as the Baltic Sea. In another example, the Mediterranean Sea has been the subject of some studies (e.g., Fenoglio et al., 2012; Loomis and Luthcke, 2017). The Adriatic Sea of the Mediterranean Sea is also a narrow and semi-enclosed basin (in a similar size and width to the Baltic Sea). Additionally, Virtanen et al. (2010) also investigated the Baltic Sea level by GRACE as it was stated in the previous section.
In addition to the datasets above, the North Atlantic Oscillation index is used to interpret the patterns of the sea level on different time scales (Hurrell and Deser, 2009). The dataset is acquired through the Climate Data Guide initiative (Schneider et al., 2013).
Total, barystatic, and steric sea level variations
Theoretically, the sum of steric and barystatic components needs to be equal to the total sea level (Leuliette and Miller, 2009):
where SLT, SLS, SLB are total, steric and barystatic sea level at time t and ε represents the accumulative error stem from all datasets. On the global scale, Eq. 1 yields promising results (WCRP Global Sea Level Budget Group, 2018), but on regional scales, ε is considerable due to limitations of the measurements, mainly in the barystatic datasets (Figure 1D). The sum of contributors captures the variations of total sea level successfully by a correlation coefficient of ~ 0.9 (Table 1). There are considerable misclosures in the amplitudes of two signals as they are reflected in terms of residual RMS. Among the barystatic time series of different centers, the JPL dataset shows the smallest residual RMS when they are subtracted from the total sea level. Adding the steric sea level to barystatic component reduces these RMS values further by 4.5%, on average, although the share of GSFC is rather smaller compared to the other datasets. The sea level variation in the Baltic Sea is dominated by the barystatic component. This has already been shown by Virtanen et al. (2010) who showed that the steric signal is only 10% of the total sea level when peak to peak amplitude is considered. In our study, a similar conclusion can be reached as the contribution of the steric sea level is one order lower than the barystatic signal. If we use the standard deviation of the time series as a measure of their signal variability, the figures for total, barystatic, and steric sea levels are 130, 95, and 15 mm, respectively.
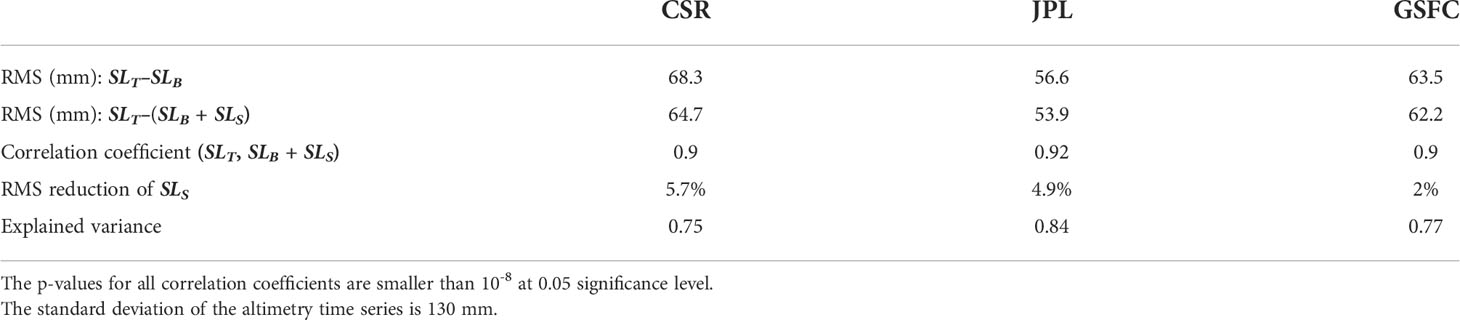
Table 1 Correlation and discrepancies between total sea level and the barystatic datasets and the sum of contributors in terms of RMS.
It is worth mentioning that when the weighting is not applied to the steric time series at different depths, the residual RMS (ε) exceeds SLT– SLB figures. Thus, it can be argued weighting the steric sea level is, in fact, a better estimation for the basin than the regular integral over different depths.
Hydrological leakage (i.e., leakage of larger hydrologic mass change into smaller sea level change) is the main downside of the barystatic time series provided by GRACE in small basins with irregular shapes (Loomis and Luthcke, 2017). Since the technique is not measuring pointwise observations, separation of the mass change signal around water-land transition zones is hard to achieve. Despite the assiduous efforts by researchers to minimize this effect, a part of the true mass signal either leaks in or out in the transition zones. In the case of this study, the basin is shaped in the form of different bifurcations such as the Gulf of Riga and Gulf of Finland which exposes the barystatic data to severe leakage effect. There are also small basins nearby, (e.g., Lake Ladoga, to the east of the Gulf of Finland), which aggravate the accuracy of the barystatic dataset. The residuals of Eq.1 (Figure 1E) do not demonstrate any substantial correlation with barystatic data. It is respectively 0.48, 0.40, 0.38 for CSR, JPL, and GSFC. This shows that the main problem is not amplitude but out of phase variations. This can be linked to the phase difference between the hydrological and barystatic sea level signals (Virtanen et al., 2010). Moreover, the estimated steric sea level does not reflect the accurate density related changes basin-wide either. Despite the fact that the Gulf of Bothnia is not very deep compared to the Baltic Proper, the lack of dataset in this part of the basin may underestimate the role of freshwater and water temperature in the estimated steric sea level. Even if the steric sea level from the reanalysis model is used, the estimation is impacted by the uncertainties raising from ocean model, atmospheric forcing dataset, and other factors (Storto et al., 2019). Such deficiencies in the datasets, as well as the satellite altimetry errors, are responsible for the mismatch between the sum of contributors and total sea level variations. Compared to Virtanen et al. (2010), the only similar study in the Baltic Sea, there is a significant improvement in terms of RMS. The minimum RMS they could reach was 115 mm, while it is maximum 65 mm in our analysis. They also used “explained variance (1–var(SLT–(SLs + SLB))/var(SLT))” to evaluate their results and the highest figure they reached was 0.70. This parameter in this study yields 0.75, 0.84, and 0.77 for CSR, JPL, and GSFC solutions, respectively. Despite the deficiencies in the datasets, the sum of barystatic and steric components explains up to 84% of the total sea level variations.
Since the amplitudes of high frequency variations are masking the long-term changes in satellite altimetry and GRACE data, we applied a signal decomposition method to the datasets to analyse seasonal, internannual, and decadal components. This will help on eliciting information on the role of total sea level and its contributors on different time scales. To this end, we opt for the multiresolution analysis based on the maximal overlap discrete wavelet transform (MODWTMRA). In MODWTMRA (Percival and Walden, 2000; Whitcher et al., 2000), the signal is decomposed into different levels and a residual. The number of levels can be between 2 to n, n< ln(N) where N is the size of the dataset. Each level covers a range of frequencies. In this study, all signals are partitioned into six levels in which the first two levels render the high frequency variability with periods shorter than the seasonal signal. Since the study aims at variations over longer periods, we did not use these two levels. Level three (6 months to 1.3 years) corresponds to seasonal variability and levels four to six cover the internannual changes (1.5 years to 9 years). The residual will represent the decadal variation of each time series. The NAO index is similarly decomposed into different time scales. The transformation requires evenly sampled data with no gaps. To fill the gaps in steric and barystatic sea level, linear interpolation was carried out.
Figure 2 (left panels) shows the result of decomposition for the Baltic Sea and Table 2 reports the correlation coefficients between total sea level variations and the contributors at different time scales. The barystatic datasets are coherent with total sea level variations on the seasonal time scale (correlation coefficient > 0.83), while the amplitudes are not completely compatible. The discrepancies are expectedly more pronounced over the gap period between GRACE and GRACE-FO. Note that the time-variable seasonal cycle (from one year to the next) in altimetry data, which agrees with that of GRACE/GRACE-FO data, is clearly extracted by the decomposition method employed here. The conventional Fourier approach fails to capture such variability in the seasonal signal. The seasonal steric sea level has around 3-4 months lag w.r.t barystatic and total sea level with substantially smaller but more consistent amplitude in the study period. The peaks for barystatic and total sea level are in November or December. On the interannual scale, the steric sea level has a negligible contribution to the sea level variation in the basin. The coherency between the total and barystatic sea level changes is substantial on this time scale (correlation coefficient > 0.80), disregarding the data over the gap period. The high correlation between sea level and NAO indicates that the interannual sea level variability is governed by this pattern in the basin (Andersson, 2002; Yan et al., 2004; Hünicke et al., 2015). The mass signals in the Baltic Sea agree with the sea level signal in the North Sea and NAO on interannual signal. This indicates that NAO likely affects the Baltic Sea level by inflow/outflow from/to the North Sea on interannual time scale. This happens by strengthening/weakening the currents and westerly winds as reported by Passaro et al. (2021). To support this argument, Gräwe et al. (2019) show that a substantial amount of sea level variation in the basin is caused by external factors (see Figure 4 of the reference). Despite all these factors, a deeper investigation is required for understanding the role of NAO on sea level variation of the Baltic Sea.
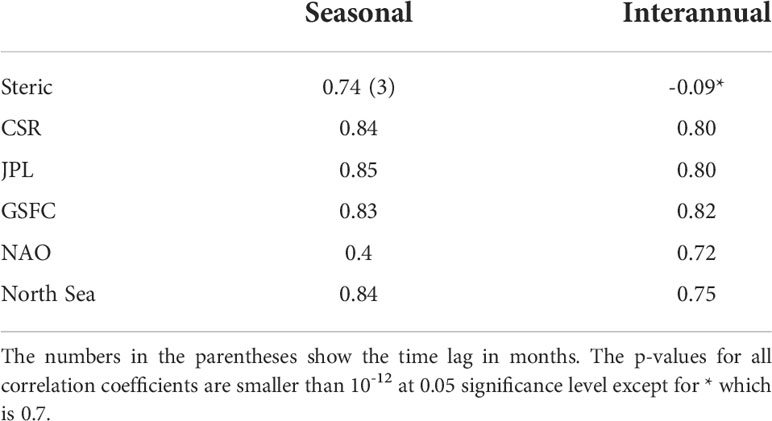
Table 2 The correlation coefficients between total sea level in the Baltic Sea and the data indicated on the first column.
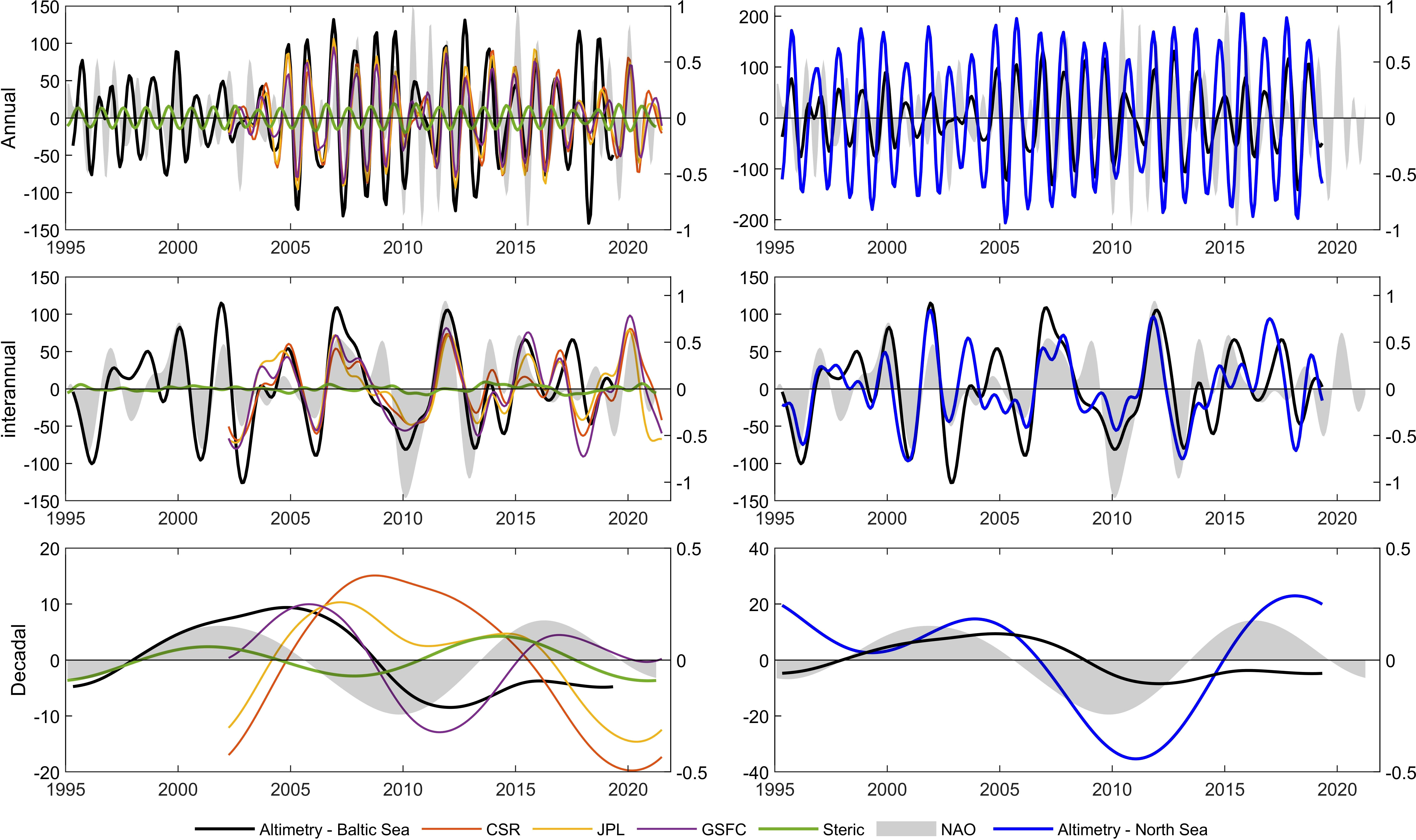
Figure 2 Left panels: decomposition of total (altimetry), barystatic (CSR, JPL, and GSFC), and steric sea level in the Baltic Sea on different time scales. Right panels: comparison of sea level variations in the North Sea, Baltic Sea. The shaded area and right axes represent the NAO. Left axes show sea level variations in mm.
In contrast to seasonal and interannual time scales, the barystatic datasets do not show high agreement on the decadal scale, likely due to limited data. Among them, the GSFC dataset shows a relatively coherent variation (0.78 correlation coefficient) when it is compared with the total sea level. At the decadal time scale, the most remarkable point is the relatively large amplitude of steric sea level changes compared to total seal level variations. Despite its insignificant contribution on the interannual scale, the peak-to-peak amplitude of the steric sea level in the decadal signal is 40% of the total sea level. However, the steric sea level variations are out-of-phase w.r.t total sea level, similar to the case of seasonal changes.
From a GRACE data perspective, the data from different centres have good agreement with each other on seasonal and interannual time scales. They also agree with the satellite altimetry data on these time scales. On decadal time scales, GRACE data are not in agreement with the altimetry data due to the short length of the data and leakage effect.
The decomposition method is also applied to the mean sea level of the North Sea to investigate the similarities with the Baltic Sea (see the right panel in Figure 2). The amplitude of the seasonal signal in the North Sea is slightly greater than in the Baltic Sea while both have similar amplitudes on the interannual scale. The correlations between them are also substantial on these time scales despite differences in interannual signals from 2003 to 2006 which can be attributed to local drivers. On the decadal scale, the sea level in the North Sea falls substantially from 2004 to 2011. A similar decrease, but with lower amplitude, can be seen in the Baltic Sea with a few years lag. This could imply an outflow from the Baltic to the North Sea. However, after this period, the North Sea level recovers while the Baltic Sea continues to hover below average. This might be linked to the decadal variation of the steric sea level since it follows a downward trend since 2014.
Data availability statement
The original contributions presented in the study are included in the article/Supplementary Material. Further inquiries can be directed to the corresponding author.
Author contributions
AK carried out conception, design, and analyses. AK, KG-F, and MP contributed to manuscript writing, revision, read, and approved the submitted version.
Acknowledgments
We would like to thank the Climate Data Guide initiative, Baltic SEAL, CSR, JPL, GSFC, and Copernicus Marine Service to provide us with high quality datasets which made this study possible.
Conflict of interest
The authors declare that the research was conducted in the absence of any commercial or financial relationships that could be construed as a potential conflict of interest.
Publisher’s note
All claims expressed in this article are solely those of the authors and do not necessarily represent those of their affiliated organizations, or those of the publisher, the editors and the reviewers. Any product that may be evaluated in this article, or claim that may be made by its manufacturer, is not guaranteed or endorsed by the publisher.
Supplementary material
The Supplementary Material for this article can be found online at: https://www.frontiersin.org/articles/10.3389/fmars.2022.963564/full#supplementary-material
References
Andersson H. C. (2002). Influence of long-term regional and large-scale atmospheric circulation on the Baltic sea level. Tellus A: Dynamic Meteorol. Oceanogr. 54, 76–88. doi: 10.3402/tellusa.v54i1.12125
Barnoud A., Pfeffer J., Guérou A., Frery M.-L., Siméon M., Cazenave A., et al. (2021). Contributions of altimetry and argo to non-closure of the global mean Sea level budget since 2016. Geophys. Res. Lett. 48, e2021GL092824. doi: 10.1029/2021GL092824
Benveniste J., Cazenave A., Vignudelli S., Fenoglio-Marc L., Shah R., Almar R., et al. (2019). Requirements for a coastal hazards observing system. Front. Mar. Sci. 6. doi: 10.3389/fmars.2019.00348
Dangendorf S., Calafat F. M., Arns A., Wahl T., Haigh I. D., Jensen J. (2014). Mean sea level variability in the north Sea: Processes and implications. J. Geophys. Res.: Oceans 119, 6820–6841. doi: 10.1002/2014JC009901
Dangendorf S., Frederikse T., Chafik L., Klinck J. M., Ezer T., Hamlington B. D. (2021). Data-driven reconstruction reveals large-scale ocean circulation control on coastal sea level. Nat. Climate Change 11, 514–520. doi: 10.1038/s41558-021-01046-1
Dettmering D., Müller F. L., Oelsmann J., Passaro M., Schwatke C., Restano M., et al. (2021). North SEAL: A new dataset of sea level changes in the north Sea from satellite altimetry. Earth Syst. Sci. Data 13, 3733–3753. doi: 10.5194/essd-13-3733-2021
Dobslaw H., Dill R., Bagge M., Klemann V., Boergens E., Thomas M., et al. (2020). Gravitationally consistent mean barystatic Sea level rise from leakage-corrected monthly GRACE data. J. Geophys. Res.: Solid Earth 125 (11), e2020JB020923. doi: 10.1029/2020JB020923
Ekman M., Mäkinen J. (1996). Mean sea surface topography in the Baltic Sea and its transition area to the north Sea: A geodetic solution and comparisons with oceanographic models. J. Geophys. Res.: Oceans 101, 11993–11999. doi: 10.1029/96JC00318
Feng W., Lemoine J. M., Zhong M., Hsu H. T. (2014). Mass-induced sea level variations in the red Sea from GRACE, steric-corrected altimetry, in situ bottom pressure records, and hydrographic observations. J. Geodynam. 78, 1–7. doi: 10.1016/j.jog.2014.04.008
Fenoglio-Marc L., Rietbroek R., Grayek S., Becker M., Kusche J., Stanev E. (2012). Water mass variation in the Mediterranean and black seas. J. Geodynam. 59, 168–182. doi: 10.1016/j.jog.2012.04.001
Gräwe U., Klingbeil K., Kelln J., Dangendorf S. (2019). Decomposing mean Sea level rise in a semi-enclosed basin, the Baltic Sea. J. Climate 32, 3089–3108. doi: 10.1175/JCLI-D-18-0174.1
Gregory J. M., Griffies S. M., Hughes C. W., Lowe J. A., Church J. A., Fukimori I., et al. (2019). Concepts and terminology for sea level: Mean, variability and change, both local and global. Surveys Geophys. 40 (6), 1251–1289. doi: 10.1007/s10712-019-09525-z
Gustafsson B. (1997). Interaction between Baltic Sea and north Sea. Dtsch. Hydrografische Z. 49, 165–183. doi: 10.1007/BF02764031
Hünicke B., Zorita E. (2006). Influence of temperature and precipitation on decadal Baltic Sea level variations in the 20th century. Tellus A: Dynamic Meteorol. Oceanogr. 58, 141–153. doi: 10.1111/j.1600-0870.2006.00157.x
Hünicke B., Zorita E. (2008). Trends in the amplitude of Baltic Sea level annual cycle. Tellus A: Dynamic Meteorol. Oceanogr. 60, 154–164. doi: 10.1111/j.1600-0870.2007.00277.x
Hünicke B., Zorita E., Soomere T., Madsen K. S., Johansson M., Suursaar Ü., et al. (2015). "Recent change–Sea level and wind waves," in Second assessment of climate change for the Baltic Sea basin (Cham: Springer International Publishing), 155–185.
Hurrell J. W., Deser C. (2009). North Atlantic climate variability: The role of the north Atlantic oscillation. J. Mar. Syst. 78, 28–41. doi: 10.1016/j.jmarsys.2008.11.026
Karabil S., Zorita E., Hünicke B. (2017). Mechanisms of variability in decadal sea-level trends in the Baltic Sea over the 20th century. Earth Syst. Dynam. 8, 1031–1046. doi: 10.5194/esd-8-1031-2017
Karabil S., Zorita E., Hünicke B. (2018). Contribution of atmospheric circulation to recent off-shore sea-level variations in the Baltic Sea and the north Sea. Earth Syst. Dynam. 9, 69–90. doi: 10.5194/esd-9-69-2018
Kniebusch M., Meier H. E. M., Radtke H. (2019). ). changing salinity gradients in the Baltic Sea as a consequence of altered freshwater budgets. Geophys. Res. Lett. 46, 9739–9747. doi: 10.1029/2019GL083902
Kudryavtseva N. A., Soomere T. (2016). Validation of the multimission altimeter data for the Baltic Sea region. Estonian J. Earth Sci. 65, 161–175. doi: 10.3176/earth.2016.13
Kudryavtseva N., Soomere T. (2017). Satellite altimetry reveals spatial patterns of variations in the Baltic Sea wave climate. Earth Syst. Dynam. 8, 697–706. doi: 10.5194/esd-8-697-2017
Landerer F. W., Flechtner F. M., Save H., Webb F. H., Bandikova T., Bertiger W. I., et al. (2020). Extending the global mass change data record: GRACE follow-on instrument and science data performance. Geophys. Res. Lett. 47, e2020GL088306. doi: 10.1029/2020GL088306
Lehmann A., Getzlaff K., Harlaß J. (2011). Detailed assessment of climate variability in the Baltic Sea area for the period 1958 to 2009. Climate Res. 46, 185–196. doi: 10.3354/cr00876
Leuliette E. W., Miller L. (2009). Closing the sea level rise budget with altimetry, argo, and GRACE. Geophys. Res. Lett. 36. doi: 10.1029/2008GL036010
Loomis B. D., Luthcke S. B. (2017). Mass evolution of Mediterranean, black, red, and Caspian seas from GRACE and altimetry: Accuracy assessment and solution calibration. J. Geodesy 91, 195–206. doi: 10.1007/s00190-016-0952-3
Loomis B. D., Luthcke S. B., Sabaka T. J. (2019). Regularization and error characterization of GRACE mascons. J. Geodesy 93, 1381–1398. doi: 10.1007/s00190-019-01252-y
Madsen K. S., Høyer J. L., Suursaar Ü., She J., Knudsen P. (2019). Sea Level trends and variability of the Baltic Sea from 2D statistical reconstruction and altimetry. Front. Earth Sci. 7. doi: 10.3389/feart.2019.00243
Meier H. E. M., Kauker F. (2003). Modeling decadal variability of the Baltic Sea: 2. role of freshwater inflow and large-scale atmospheric circulation for salinity. J. Geophys. Res.: Oceans 108. doi: 10.1029/2003JC001797
Omstedt A., Pettersen C., Rodhe J., Winsor P. (2004). Baltic Sea Climate: 200 yr of data on air temperature, sea level variation, ice cover, and atmospheric circulation. Climate Res. 25, 205–216. doi: 10.3354/cr025205
Passaro M., Cipollini P., Benveniste J. (2015). Annual sea level variability of the coastal ocean: The Baltic Sea-north Sea transition zone. J. Geophys. Res.: Oceans 120, 3061–3078. doi: 10.1002/2014JC010510
Passaro M., Müller F. L., Oelsmann J., Rautiainen L., Dettmering D., Hart-Davis M. G., et al. (2021). Absolute Baltic Sea level trends in the satellite altimetry era: A revisit. Front. Mar. Sci. 8. doi: 10.3389/fmars.2021.647607
Percival D. B., Walden A. T. (2000). Wavelet methods for time series analysis (Cambridge university press).
Quartly G. D., Rinne E., Passaro M., Andersen O. B., Dinardo S., Fleury S., et al. (2019). Retrieving Sea level and freeboard in the Arctic: A review of current radar altimetry methodologies and future perspectives. Remote Sens. 11 (7), 881. doi: 10.3390/rs11070881
Richter A., Groh A., Dietrich R. (2012). Geodetic observation of sea-level change and crustal deformation in the Baltic Sea region. Phys. Chem. Earth Parts A/B/C 53-54, 43–53. doi: 10.1016/j.pce.2011.04.011
Royston S., Dutt Vishwakarma B., Westaway R., Rougier J., Sha Z., Bamber J. (2020). Can we resolve the basin-scale Sea level trend budget from GRACE ocean mass? J. Geophys. Res.: Oceans 125, e2019JC015535. doi: 10.1029/2019JC015535
Save H. (2020). Csr grace and grace-fo rl06 mascon solutions v02. Mascon Solut. 12, 24. doi: 10.15781/cgq9-nh24
Save H., Bettadpur S., Tapley B. D. (2016). High-resolution CSR GRACE RL05 mascons. J. Geophys. Res.: Solid Earth 121, 7547–7569. doi: 10.1002/2016JB013007
Schneider D. P., Deser C., Fasullo J., Trenberth K. E. (2013). Climate data guide spurs discovery and understanding. Eos Trans. Am. Geophys. Union 94, 121–122. doi: 10.1002/2013EO130001
Storto A., Masina S., Simoncelli S., Iovino D., Cipollone A., Drevillon M., et al. (2019). The added value of the multi-system spread information for ocean heat content and steric sea level investigations in the CMEMS GREP ensemble reanalysis product. Climate Dynam. 53 (1), 287–312. doi: 10.1007/s00382-018-4585-5
Tamisiea M. E., Mitrovica J. X. (2011). The moving boundaries of Sea level change: Understanding the origins of geographic variability. Oceanography 24, 24–39. doi: 10.5670/oceanog.2011.25
Tapley B. D., Bettadpur S., Ries J. C., Thompson P. F., Watkins M. M. (2004). GRACE measurements of mass variability in the earth system. science 305, 503–505. doi: 10.1126/science.1099192
Vestøl O., Ågren J., Steffen H., Kierulf H., Tarasov L. (2019). NKG2016LU: A new land uplift model for fennoscandia and the Baltic region. J. Geodesy 93, 1759–1779. doi: 10.1007/s00190-019-01280-8
Virtanen J., Mäkinen J., Bilker-Koivula M., Virtanen H., Nordman M., Kangas A., et al. (2010). “"Baltic sea mass variations from GRACE: Comparison with in situ and modelled sea level heights,",” in Gravity, geoid and earth observation (Springer), 571–577.
Watkins M. M., Wiese D. N., Yuan D. N., Boening C., Landerer F. W. (2015). Improved methods for observing earth's time variable mass distribution with GRACE using spherical cap mascons. J. Geophys. Res.: Solid Earth 120, 2648–2671. doi: 10.1002/2014JB011547
WCRP Global Sea Level Budget Group (2018). Global sea-level budget 1993–present. Earth Syst. Sci. Data 10, 1551–1590. doi: 10.5194/essd-10-1551-2018
Whitcher B., Guttorp P., Percival D. B. (2000). Wavelet analysis of covariance with application to atmospheric time series. J. Geophys. Res.: Atmospheres 105, 14941–14962. doi: 10.1029/2000JD900110
Xu Q., Cheng Y., Plag H.-P., Zhang B. (2015). Investigation of sea level variability in the Baltic Sea from tide gauge, satellite altimeter data, and model reanalysis. Int. J. Remote Sens. 36, 2548–2568. doi: 10.1080/01431161.2015.1043405
Keywords: baltic sea level, satellite altimetry, grace, steric sea level, barystatic sea level
Citation: Karimi AA, Ghobadi-Far K and Passaro M (2022) Barystatic and steric sea level variations in the Baltic Sea and implications of water exchange with the North Sea in the satellite era. Front. Mar. Sci. 9:963564. doi: 10.3389/fmars.2022.963564
Received: 07 June 2022; Accepted: 28 July 2022;
Published: 22 August 2022.
Edited by:
Shoshiro Minobe, Hokkaido University, JapanReviewed by:
Hu Yang, Alfred Wegener Institute Helmholtz Centre for Polar and Marine Research (AWI), GermanyJun She, Danish Meteorological Institute (DMI), Denmark
Copyright © 2022 Karimi, Ghobadi-Far and Passaro. This is an open-access article distributed under the terms of the Creative Commons Attribution License (CC BY). The use, distribution or reproduction in other forums is permitted, provided the original author(s) and the copyright owner(s) are credited and that the original publication in this journal is cited, in accordance with accepted academic practice. No use, distribution or reproduction is permitted which does not comply with these terms.
*Correspondence: Armin Agha Karimi, YXJtaW5rYXJAa3RoLnNl