- 1Department of Ecology and Environmental Science, Umeå University, Umeå, Sweden
- 2Umeå Marine Sciences Centre, Umeå University, Hörnefors, Sweden
Bacteria are major consumers of dissolved organic matter (DOM) in aquatic systems. In coastal zones, bacteria are exposed to a variety of DOM types originating from land and open sea. Climate change is expected to cause increased inflows of freshwater to the northern coastal zones, which may lead either to eutrophication or to increased inputs of refractory terrestrial compounds. The compositional and functional response of bacterial communities to such changes is not well understood. We performed a 2-day microcosm experiment in two bays in the coastal northern Baltic Sea, where we added plankton extract to simulate eutrophication and soil extract to simulate increased inputs of refractory terrestrial compounds. Our results showed that the bacterial communities responded differently to the two types of food substrates but responded in a similar compositional and functional way in both bays. Plankton extract addition induced a change of bacterial community composition, while no significant changes occurred in soil extract treatments. Gammaproteobacteria were promoted by plankton extract, while Alphaproteobacteria dominated in soil extract addition and in the non-amended controls. Carbohydrate metabolism genes, such as aminoglycan and chitin degradation, were enriched by plankton extract, but not soil extract. In conclusion, the coastal bacterial communities rapidly responded to highly bioavailable substrates, while terrestrial matter had minor influence and degraded slowly. Thus, in the northern Baltic Sea, if climate change leads to eutrophication, large changes of the bacterial community composition and function can be expected, while if climate change leads to increased inflow of refractory terrestrial organic matter the bacterial communities will not show fast compositional and functional changes. Degradation of terrestrial organic matter may instead occur over longer periods of time, e.g. years. These findings help to better understand the ability of bacterial communities to utilize different carbon sources and their role in the ecosystem.
Introduction
Climate change has different impacts in varying regions on Earth, and in northern Europe both warming and increased precipitation are expected (Meier et al., 2012). In the Baltic Sea, climate change has consequences including eutrophication in certain areas and potential oligotrophication in others (e.g. Andersson et al., 2015). More frequent phytoplankton blooms and increasing production of autochthonous dissolved organic matter (AtDOM) are expected in the southern Baltic Sea, while in the north increased inflow of coloured refractory terrestrial dissolved organic matter (tDOM) may instead nurture heterotrophic bacteria and the “microbial loop” (Andersson et al., 2015; Meier et al., 2022). While tDOM reaching coastal areas is composed of refractory high molecular weight molecules, such as lignin, cellulose, pectin, xylan and humic substances (Pettersson et al., 1997; Akita et al., 2016; Zhao et al., 2021), AtDOM is constituted by plankton derived labile carbon sources, for example chitin, starch and glycogen (e.g. Bertilsson and Jones, 2003).
Dissolved organic matter (DOM) constitute the main energy source for heterotrophic bacteria in surface waters (Jiao et al., 2011). During the bacterial degradation of DOM, complex organic carbon compounds can be broken down into smaller compounds, inorganic nutrients could be released, which can be utilized by other organisms, such as phytoplankton. In turn, phytoplankton excrete organic matter back into the water column, which is degraded by bacteria. Thus, these bacterial communities play an important role in regulating the availability of nutrients and the overall carbon cycle (Azam and Malfatti, 2007; Jiao et al., 2011).
Different DOM types can regulate bacterial community structure due to the occurrence of taxa-specific metabolic capabilities (Logue et al., 2016). In aquatic systems primary producers release large amounts of photosynthetic products, which are food resources for heterotrophic bacteria (Mühlenbruch et al., 2018). The bacterial community composition associated with phytoplankton blooms is often dominated by Gammaproteobacteria, Flavobacteriia and Roseobacter, which is linked to their capability of producing specific exo-enzymes degrading phytoplankton-derived polysaccharides (Teeling et al., 2012). In the Baltic Sea, Gammaprotebacteria have been observed to be common in highly productive areas (Lindh et al., 2015a; Herlemann et al., 2016; Mühlenbruch et al., 2018). Further, this group is known to quickly exploit new environments upon disturbances (Lindh et al., 2015b; Andersson et al., 2018).
Terrestrial DOM, drives communities towards proliferation of Bacteroidetes, Gemmatimonadetes, Planctomycetes, and Alpha- and Betaproteobacteria (Traving et al., 2017; Broman et al., 2019). In coastal areas of the northern Baltic Sea, Burkholderiales dominate the community during the spring river flush period, when tDOM concentrations are elevated (Figueroa et al., 2021). The underlying mechanism may be that Burkholderiales have the capacity for lignin degradation by harbouring a large diversity of catabolic enzymes that can degrade recalcitrant aromatic compounds in a variety of environmental conditions (Morya et al., 2020). Since the transport of tDOM to subarctic coastal zones is huge (Cole et al., 2007; Drake et al., 2018), it can be expected that bacteria with capacity to degrade tDOM are selected in coastal areas receiving freshwater in the northern Baltic Sea.
Previous studies indicate a link between DOM properties and bacterial diversities in aquatic environments, such as glacial systems (Smith et al., 2018), streams (D’Andrilli et al., 2019), river estuaries (Figueroa et al., 2021) and coastal areas (Teira et al., 2009; Traving et al., 2017; Bruhn et al., 2021). The northern Baltic Sea is a particularly interesting region for studying the effects of shifts in DOM properties on bacterial communities, as it is a semi-enclosed brackish basin that receives inputs from both marine and terrestrial sources. Several studies have investigated the effects of various environmental factors on bacterial community composition and diversity in the Baltic Sea (e.g. Andersson et al., 2010; Figueroa et al., 2021). However, the effects of climate change induced DOM shift on bacterial community composition and functional profiles in the northern Baltic Sea are still poorly understood. Therefore, further investigation of the responses of bacterial communities to these changes in the northern Baltic Sea is needed to better understand the potential impacts of climate change on the functioning of this ecosystem. The bacterial community has an important role in biogeochemical cycling and ecological functions in the Baltic Sea. By examining changes in both the taxonomic and functional profiles of bacterial communities, it is possible to gain a more complete understanding of how shifts in DOM properties can affect the ecological and biogeochemical functions of bacterial communities in the Baltic Sea. Metagenomics sequencing techniques can be used to identify genes involved in the degradation of different types of organic matter, such as complex carbon compounds (Lindh and Pinhassi, 2018).
Heterotrophic bacteria play a vital role in the environment by utilizing and breaking down various carbon-rich compounds through the production of exoenzymes, these enzymes facilitate the degradation and utilization of different types of carbon compounds present in the environment (Beier and Bertilsson, 2013; Mühlenbruch et al., 2018). The Carbohydrate-Active enZymes (CAZyme) database describes the genes and functions of relevant enzymes involved in the biosynthesis and breakdown of complex carbohydrates (Drula et al., 2022). By comparing the functional profiles of bacterial communities under different environmental conditions, it is possible to gain insights into the specific enzymatic pathways that are involved in the degradation of different types of organic matter in the Baltic Sea.
To gain insight into how bacterial communities in the northern Baltic Sea may respond to future changes in the dissolved organic matter (DOM) pool resulting from climate change, we conducted a field experiment in a coastal area of the northern Baltic Sea. The study investigated the effects of two types of DOM on bacterioplankton, including labile plankton-derived DOM (plankton extract) and relatively refractory soil-derived DOM (soil extract).
We hypothesized that the varying DOM types would select for bacterial communities with different diversity, community composition and carbohydrate metabolism-related gene profiles. More specifically, that labile plankton-derived DOM amendment would promote Gammaproteobacteria and cause decreased diversity, whereas addition of soil-derived DOM would favour taxa known to be common in terrestrial systems. Bacterial taxa promoted by the addition of plankton-derived DOM were expected to harbour genes for degradation of autochthonous DOM, such as chitin, alginate, xylan, starch and glycogen. Bacterial taxa favoured by terrestrial DOM were expected to harbour genes for degradation of refractory DOM, such as lignin and cellulose.
To test our hypotheses, we employed 16S rRNA metabarcoding and shotgun metagenomic sequencing to explore the structure of the bacterial communities and their potential metabolic functions. We examined the associations between bacterial taxa and environmental conditions to understand how labile versus refractory DOM impacts the bacterial community assembly. Our overall aim was to investigate the potential shifts of bacterial communities and their functions under climate change scenarios in a coastal region of northern Baltic Sea.
Material and methods
Substrate extraction
To obtain the labile substrate, plankton samples were collected using a WP2 plankton net with a 90 µm mesh at a coastal station (about 20 meters depth) 2 weeks before we performed the experiment, 2018. After combining several vertical net hauls, the collected plankton samples were lysed with a tissue lyser, then the mixture was centrifuged and the supernatant was filtered through a syringe filter with 0.2 µm supor membrane non-polygenic (Pall Corporation). The plankton extract was mainly based on lysates of filamentous cyanobacteria (mainly Aphanizomenon sp.) and zooplankton (mainly Eurytemora sp., Acartia sp. and Bosmina sp.), and consisted of high molecular weight (HMW) organic compounds, such as polysaccharides (Zhao et al., 2021), but also inorganic substances. The molar ratio of carbon to nitrogen to phosphorus in the plankton extract was 44:9:1, calculated using the concentrations of dissolved organic carbon (DOC), total dissolved nitrogen (TDN), and total dissolved phosphorus (TDP).
To obtain the refractory terrestrial extract, humic rich river bank soil was collected from a 5-10 cm layer above the sand layer near the Öre River. The soil sample was homogenized and sieved through 4-6 mm sieve nets, then, a 9.8 kg collected soil sample was strongly mixed with 65 l MQ-water for 2 days of vigorous mechanical mixing with a large pump. After that, the mixture was manually pushed through 100 µm nylon net and subsequently through 15 µm nylon net and centrifuged at 10 000 rpm. Then the mixture was stepwise filtered through a filtration system compiled of 25, 5, 3, 1 μm (IFAB product Nr. PPE 025-10, PPE 005-10, SRL 030 250L CZ and SLS 010-250L EF, respectively) by pump suction at high flow rate (~200-400 ml.min-1). Approximately 1.7 l extract was then pushed at low flow rate and high pressure through 0.22 µm filter (250L-CSS-002SFA) before use. The C: N: P molar ratio of the soil extract was 569:36:1, calculated based on the ratio of DOC: TDN: TDP.
Experimental set-up
The experiment aimed to mimic climate change impacts on inputs of terrestrial substances associated with river runoff increasing and on phytoplankton derived substances associated with eutrophication. Two bays situated in the northern Baltic Sea (Figure 1) were selected to conduct the field microcosm experiment (18-20 September 2018), (a) Ängerån (AN) bay (63°32′21″ N, 19.46′29″ E), which receives humic rich river water from the Änger River, (b) Kalvarskatan (KA) bay (63°35′54″N, 19°53′4″ E), which does not have any immediate freshwater inflow (Table 1). The temperature of the water in both bays was 12.7°C. We collected seawater at a depth of 0.5 meters using a Ruttner water sampler (NormecTec, Johanneshov) at each bay, and then filled nine transparent polyethylene cubitainers (10 liters each) with 7.8 liters of seawater filtered through a 200 μm nylon mesh.
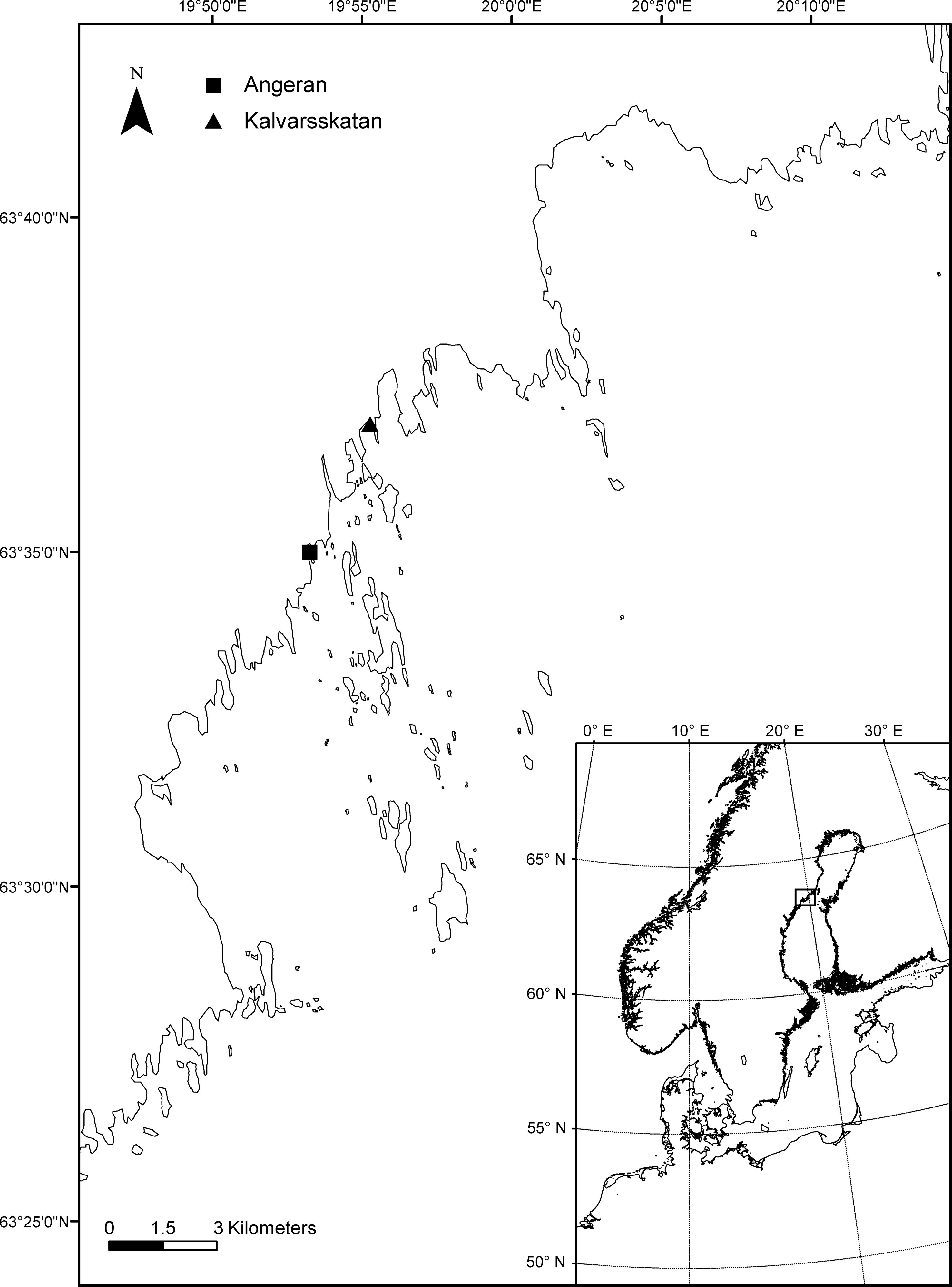
Figure 1 Map of experimental sites in coastal northern Baltic Sea. Solid triangle indicates site Kalvarskatan bay receiving no river inflow, and solid square indicates site Angeran bay receiving river inflow.
In each bay, we incubated seawater samples in triplicate with three different treatments for 2 days. One group received plankton extract addition, resulting in initial concentrations
of ~435 DOC μmol l-1, 2 TDP μmol l-1 and 35 TDN μmol l-1, while another group received soil extract addition, resulting in an initial concentration of ~435 DOC μmol l-1, 0.3 TDP μmol l-1 and 22 TDN μmol l-1, both of the amendments resulted in approximately a 23-24% increase in carbon concentration. The third group (control) did not receive any addition. We thoroughly mixed the cubitainers and incubated them in situ at 0.5 m depth at each bay. We tested the bacterial community response to different treatments by measuring bacterial production and bacterial abundance, inorganic nutrients and dissolved organic carbon (DOC) at the beginning and the end of the experiment. For DNA samples, 1 liter water was filtered on the 47-mm, 0.2 μm PES Supor (Pall Corporation, Ann Arbor) membrane filters, stored at -80°C until DNA extraction.
Bacterial production and bacterial abundance
Bacterial production (BP) was measured using the 3H-thymidine incorporation technique as described in (Berglund et al., 2007). One milliliter of seawater was added to four Eppendorf tubes, one control and triplicate samples. Bacteria in the control were pre-killed by adding 100 μl ice-cold 50% trichloro acetic acid (TCA) and incubating at 2°C for 5 min. After that, 2 μl [3H]-thymidine (83.3 Ci mmol−1; PerkinElmer, Massachusetts, USA) were added to each tube to a final concentration of 24 nM. 100 µl of 50% ice-cold TCA were added to the samples after 1 h to terminate the incubations. Samples were analyzed using a Perkin Elmer Tri-Carb 2910TR scintillation counter. The incorporated thymidine was converted to cell production using the conversion factor of 1.4 × 1018 cells mol−1 (Fuhrman and Azam, 1982).
Samples for bacterial counts were pre-filtered through a 50 µm mesh, preserved in 0.1% glutaraldehyde (final concentration), frozen at -80°C and analyzed with a BD FACSVerse™ flow cytometer (BD Biosciences). Frozen samples were quickly thawed in a 30°C water bath. Samples were stained with SYBR Green I (Invitrogen) at a final concentration of 1:10 000. As internal standard, 1 μm microspheres (Fluoresbrite plain YG, Polysciences) were added to each sample (Marie et al., 2005).
Chemical analyses
Dissolved organic carbon (DOC) was analyzed in water filtered through a 0.22 µm Supor Membrane Syringe Filter (non-pyrogenic; Acrodisc®) and acidified to 18 mM HCl, final concentration. Samples were analyzed with a high-temperature combustion Shimadzu (Kyoto) TOC-5000 analyzer. Total dissolved phosphorus (TDP) and total dissolved nitrogen (TDN) were measured in 0.22 µm filtered samples, as described for DOC, using a Seal (Norderstedt) QuAAtro39 autoanalyzer after an oxidation step using peroxodisulfate, according to standard analytical methods (Grasshoff et al., 1999).
The concentration of humic substances (HS) was measured from unfiltered water samples using a Perkin Elmer LS 30 fluorometer at 350/450 nm excitation/emission wavelengths, calibrated with a serial dilution of quinine sulfate solution (Hoge et al., 1993).
Picophytoplankton
Samples were pre-filtered through a 50 µm mesh, preserved in 0.1% glutaraldehyde (final concentration) and frozen at −80°C (Marie et al., 2005) for later counts using a BD FACSVerse™ flow cytometer (BD Biosciences) equipped with a 488 nm laser (20 mW output) and a 640 mn laser (output 40 mW). Frozen samples were quickly thawed in a 30°C water bath. Picophytoplankton samples were run with 3 µm microspheres (Fluoresbrite ® plain YG, Polysciences) as internal standard. Picophytoplankton abundance was converted to biomass using carbon conversion factors: 120 fgC cell−1 for picocyanobacteria and 829 fgC cell−1 for picoeukaryotic phytoplankton, based on microscopic measurements of cell sizes and use of conversion factors (see above).
Nano- and microphytoplankton, heterotrophic nanoflagellates and ciliates
Samples were preserved with 2% acidic Lugol’s solution and stored in darkness at 4°C until analysis. For the analysis of nano- and microplankton, 10-50 ml were settled for 12-48 hours in sedimentation chambers and cells were counted on an inverted microscope (Nikon Eclipse Ti) at 100-400x magnification using phase contrast settings (Utermöhl, 1958). Cells were grouped into three functional groups (AU: autotrophs, HT: heterotrophs, MX: mixotrophs), based on the feeding mode (Olenina et al., 2006). Nutritional characteristics of plankton were identified based on described trophy (Tikkanen and Wille'n, 1992; Hallfors, 2004; Olenina et al., 2006). Further, the coloration of the smallest cells was used to support the trophy classification as Lugol’s solution stains Chl a in brown. Plankton biomass was calculated from the geometric shape of cells following Olenina et al. (2006) and cell carbon content was calculated according to Menden-Deuer and Lessard (2000).
DNA extraction
DNA was extracted from filter samples using DNeasy Power Water kit (Qiagen, Sweden) according to a modified DNeasy PowerWater protocol (e.g. extended lysis time, lower elution volume). Further details of the modified protocol can be provided upon request. Two milli-Q water extraction controls and two filter extraction controls were used during DNA extraction, these are samples of Milli-Q water or empty filters that are processed in the same way as the environmental samples, all the extraction controls were amplified and sequenced in parallel to the samples to monitor possible contamination. The extracted DNA was measured by gel electrophoresis and Qubit fluorometer.
16S rRNA sequencing and data analysis
Prokaryote 16S V4-V5 rRNA gene sequences were generated by PCR amplification using tagged primers 563F (5’-AYTGGGYDTAAAGNG) and 907R (5’-CCGTCAATTCMTTTGAGTTT) (Hugoni et al., 2017). The primers contained sample tags of 8 bp and 4 leading Ns to improve sequence diversity. Q5 High-Fidelity DNA Polymerase was used for the PCR reactions, which included three non-template PCR controls that produced very few reads. Each sample was amplified in triplicate, and the amplicon was purified using magnetic beads. The amplicons were mixed in equimolar proportions and used to construct a 16S library. The amplicons were then mixed in equimolar proportions, and used to construct a 16S library and sequenced on Illumina Hiseq Platform 2500, generated 250 bp paired end reads (Novogene, Beijing, China).
The Obitools package (Boyer et al., 2016) was employed to perform read filtering, primer trimming, merging of paired end reads and demultiplexing. Briefly, the forward and reverse reads were assembled using the illuminapairedend command with a minimum alignment score of 40. Any unpaired reads were eliminated with the obigrep command. Demultiplexing was then carried out using the ngsfilter command (Boyer et al., 2016). The reads were further filtered (maxN = 0, maxEE = 2), and the learnErrors command was applied, and then the reads were denoised using DADA2 version 1.22 (Callahan et al., 2016) to generate amplicon sequence variants (ASVs). Chimers (method = “consensus”) and any ASV with a length less than 300 bp were removed. The ASV table was further filtered to exclude potential contaminations identified by the prevalence method of the ‘decontam’ package (Davis et al., 2018). Taxonomy was assigned using the assignTaxonomy command (minBoot = 80) against the SILVA database v138.1 by Naïve Bayes classifier (Quast et al., 2013). The table was then filtered to exclude the ASVs assigned to mitochondria and chloroplast. Rare taxa with a read number of less than 10 and were prevalent in less than 20% of samples were excluded. Normalization was done by the mirlyn R package with 1000 iterations of rarefaction (without replacement) at a threshold of 49402 reads. Initial analyses of multiple rarefied tables produced similar results, so one representative table from the data set (set.seed =123) was used for the further analyses (Table S1). The ASV table was analyzed and graphically displayed using the R package phyloseq (McMurdie and Holmes, 2013) and ggplot2 (Hadley, 2016). Phyloseq was also used to measure the diversity of bacterial taxa in our samples. Alpha diversity was assessed based on rarefied counts using Shannon-Wiener index, Observed, Chao1, InvSimpson, ACE, Simpson, and Fisher indices were included in Table S2. To compare the microbiota composition between samples, we calculated Bray-Curtis dissimilarity metrics using Phyloseq and visualized the results in a non-metric multidimensional scaling (NMDS) plot. The significant differences between the treatments were determined by Kruskal–Wallis tests from the agricolae R package (de Mendiburu, 2021). We used the DESeq2 package (Love et al., 2014) in R to perform differential abundance testing at the ASV level, comparing addition treatments to control using the contrast function and the Wald test. To account for multiple testing, we applied the Benjamini and Hochberg correction to adjust p-values. ASVs with an adjusted p-value < 0.05 were considered differentially abundant.
In order to estimate the contribution of environmental data to variations in community compositions, we employed a series of redundancy analyses (RDA). We used the Hellinger-transformed ASV matrix created in the R package vegan (Oksanen et al., 2022) as the dependent matrix and the scaled environmental variables as independent matrices. To avoid overfitting the model, only variables with correlation coefficients less than or equal to 0.7 were used in the RDA analysis.
Metagenome sequencing and data analysis
For metagenomics, the DNA extracts from the three replicates of each treatment were pooled, resulting in six samples that were denoted as KAC, KAP, KAS, ANC, ANP and ANS, plus 2 initial samples from day 0, KA0 and AN0. For each sample, 1μg DNA was used for PCR-free library construction with NEBNext® Ultra™ II DNA Library Prep Kit (Cat No. E7645, NEB, USA) according to the instructions of the manufacturer. The library was first quantified using Qubit and real-time PCR, and the size distribution was checked using a bioanalyzer. Quantified libraries were pooled and paired ends (2 × 150 bp) sequenced on a Novaseq 6000 platform.
The basic sequence read quality was checked with FastQC (https://www.bioinformatics.babraham.ac.uk/projects/fastqc/). Adapter sequences and low-quality bases were removed using fastp with default parameters (Chen et al., 2018). The trimmed reads were mapped with PhiX genome to remove potential PhiX reads. Then, the clean reads from the 8 samples were co-assembled using SPAdes v3.13.0 (metaspades model, kmers were 21, 33, and 55). Protein-coding genes were predicted by prodigal (v2.6.3). Predicted genes with a length less than 100 were removed. The remaining gene sequences were clustered by CD-HIT-EST with a minimum of 95% identity and 95% read coverage on amino acid level. Taxonomic assignment up to species level was performed using Kaiju v1.8.2 (Menzel et al., 2016), with an E-vale threshold of 0.00001. Salmon v1.8.0 (Patro et al., 2017) was used to quantify the abundance of coding sequences (CDS) abundance, while eggNOG-mapper v2.1.7 (Huerta-Cepas et al., 2019) was used for functional annotation based on precomputed orthology assignments. Differential abundance analysis of CDS under different treatments was performed using EdgeR (Robinson et al., 2010), samples from the same treatment were used as replicates. Genes meeting the criteria of p < 0.001 and |log2FC| > 5 were identified as significant differentially abundant genes (DAGs) in both the comparison of plankton extract treatment versus the control and soil extract treatment versus the control (data can be provided upon request). To investigate whether the differences in food substrates might lead to functional differences, Gene ontology (GO) enrichment analysis of significant DAGs was performed using R package clusterProfiler (Wu et al., 2021). For enrichment analysis, the SQLite-based custom annotation package named as “org. Bpro.org.db” was prepared in R using the AnnotationForge package (Carlson and Pagès, 2023). Using clusterProfiler, significant GO term associated DAGs were identified using enrichGO function. GO terms with both p value and FDR < 0.05 were considered significant and were represented, no significant Gene Ontology (GO) terms were found in the comparison between soil extract treatment and control. To achieve a more precise annotation of carbohydrate-active enzymes (CAZymes), we used the dbCAN2 web server to identify potential CAZymes in CDS (Zhang et al., 2018). To enhance annotation accuracy, we only kept CAZymes that were confirmed by at least two of the following tools: HMMER, DIAMOND and eCAMI (Table S3). We selected genes of interest, such as those encoding for chitinase and glycosidase CAZymes for further discussion. To confirm their function, we conducted a BLAST search against the Uniprot database (https://www.uniprot.org/blast).
Nucleotide sequence accession numbers
All sequencing data is archived in the NCBI SRA database under BioProject accession number PRJNA915349.
Results
Physicochemical and biological characterization of the bay water
Both the Ängerån and Kalvarskatan bays are brackish water systems, but the Ängerån bay is more influenced by freshwater than Kalvarskatan. Consequently, the salinity was slight lower and the concentrations of DOC and humic substances higher in Ängerån (Table 1). The total dissolved nitrogen (TDN) and phosphorus (TDP) were relatively similar in the two bays. The bacterial abundance and bacterial production were also higher in Ängerån than in Kalvarskatan (Table 1).
Carbon biomass of phytoplankton and heterotrophic protozoa
The addition of plankton extract had a stronger effect on primary production because it was rich in inorganic nutrients which promoted both heterotrophic bacterial production and phytoplankton primary production.
The carbon biomass of phytoplankton and heterotrophic protozoa increased 2-3 times in all treatments during the two-day incubation (Figure S1). Approximately 60% of the biomass was constituted by autotrophic pro- and eukaryotic picoplankton. Heterotrophic protozoa, composed of heterotrophic nanoflagellates (HNF) and ciliates, constituted ~10% of the biomass. Their biomass was relatively similar in all samples. At large, the plankton responses were similar in all treatments in the two bays. However, dinoflagellates showed a greater increase in KA than in AN, and therefore the total plankton biomass was higher in KA.
DOC consumption, bacterial abundance and production
The DOC concentration at the start of the experiment was ~350 µmolC.l-1 in the controls and ~435 µmolC.l-1 in the amendment microcosms. Plankton extract was more bioavailable than the soil extract (Table 2). The DOC net consumption in plankton extract treatment was about 18%~20% while below 5% in soil extract treatment (Table 2). The total dissolved phosphorus (TDP) consumption was also high in the plankton extract treatment compared to the control and soil extract treatments.
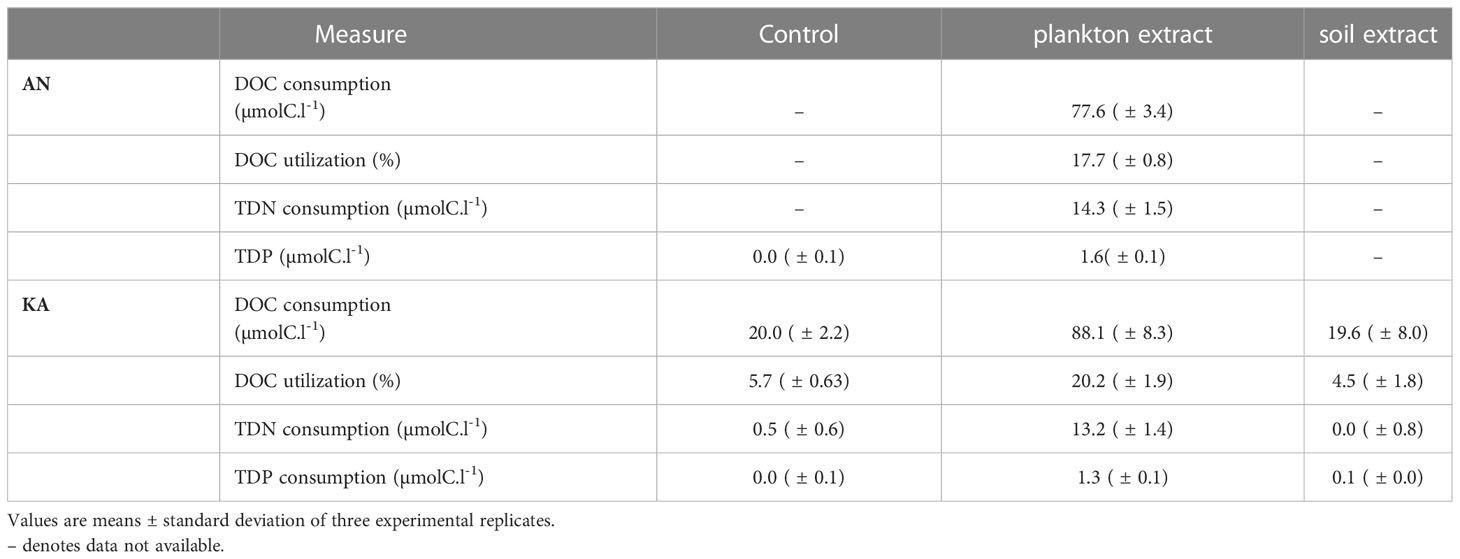
Table 2 Comparison of the bacterial DOC, TDN and TDP consumption and percentage DOC utilization in different treatments.
The bacterial growth in the two bays showed similar trends. The abundance in the KA bay increased from the initial 3.9 × 106 cell.ml-1 to 5.3 × 106 cell.ml-1 in the control, 5.9 × 106 cell.ml-1 in soil extract treatment and 18.0 × 106 cell.ml-1 in plankton extract treatment (Figure 2). In AN, the bacterial abundance also showed a similar increase, from initially 4.4× 106 to 6.4 × 106 in the control, 7.0 × 106 in soil extract treatment and 17.8 × 106 cell.ml-1 in plankton extract treatment (Figure 2). Bacterial production (BP) was stimulated by the amendments of both soil extract and plankton extract; it was enhanced 50-123% by soil extract and 1500-3300% by plankton extract relative to the control (Figure 2).
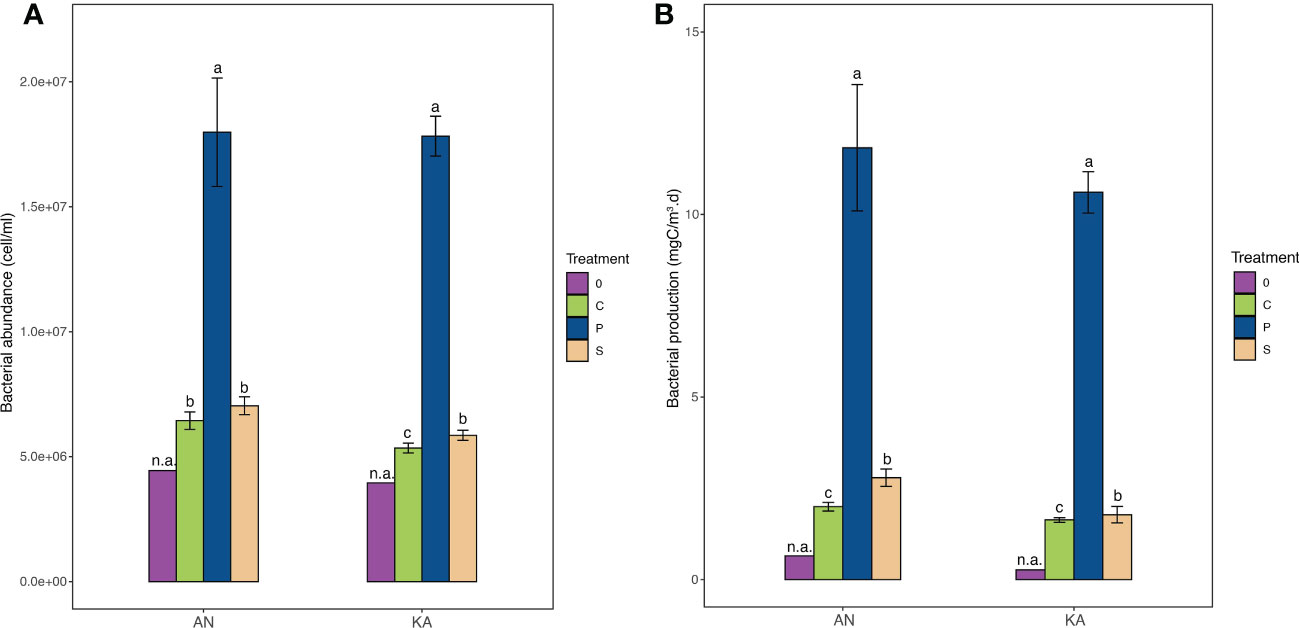
Figure 2 Bacterial abundance (A) and bacterial production (B) in different treatments measured in Kalvarsskatan (KA) and Ängerån (AN) bay at the start (and the end (day 2) of the experiment. The colours in the graph represent different treatments, with purple representing day 0, green representing control, blue representing plankton extract treatment, and brown representing soil extract treatment, day 2. Values are means of 3 replicates ± standard deviation. 0: day 0 (before DOM amendments). Error bars represent the standard deviation. The letter s “a” “b” and “c” placed above each bar indicate significant differences (α = 0.05) between the treatments (excluding day 0), as determined by Kruskal–Wallis tests.
Bacterial diversity and community composition
A total number of 462 ASVs were recovered by 16S rRNA amplicon sequencing of the bacterial communities incubated with the two different substrates in the two bays. Rarefaction curves started to plateau indicating that the diversity of the whole bacterial community was sampled (Figure S2). We used the Shannon-Wiener index as indicator of bacterial diversity. After the 2-day in situ incubation, the plankton extract treatment exhibited a significant decrease in bacterial diversity in KA, whereas the soil extract treatment and control group showed only a slight decrease in diversity compared to the initial condition in both bays (Figure 3). The beta-diversity analysis, using nMDS based on Bray–Curtis dissimilarity calculated with ASV relative abundance, indicated that the bacterial structure changed in all treatments compared to the initial condition at Day 0, with no variation between soil treatment and control (Figure 4).
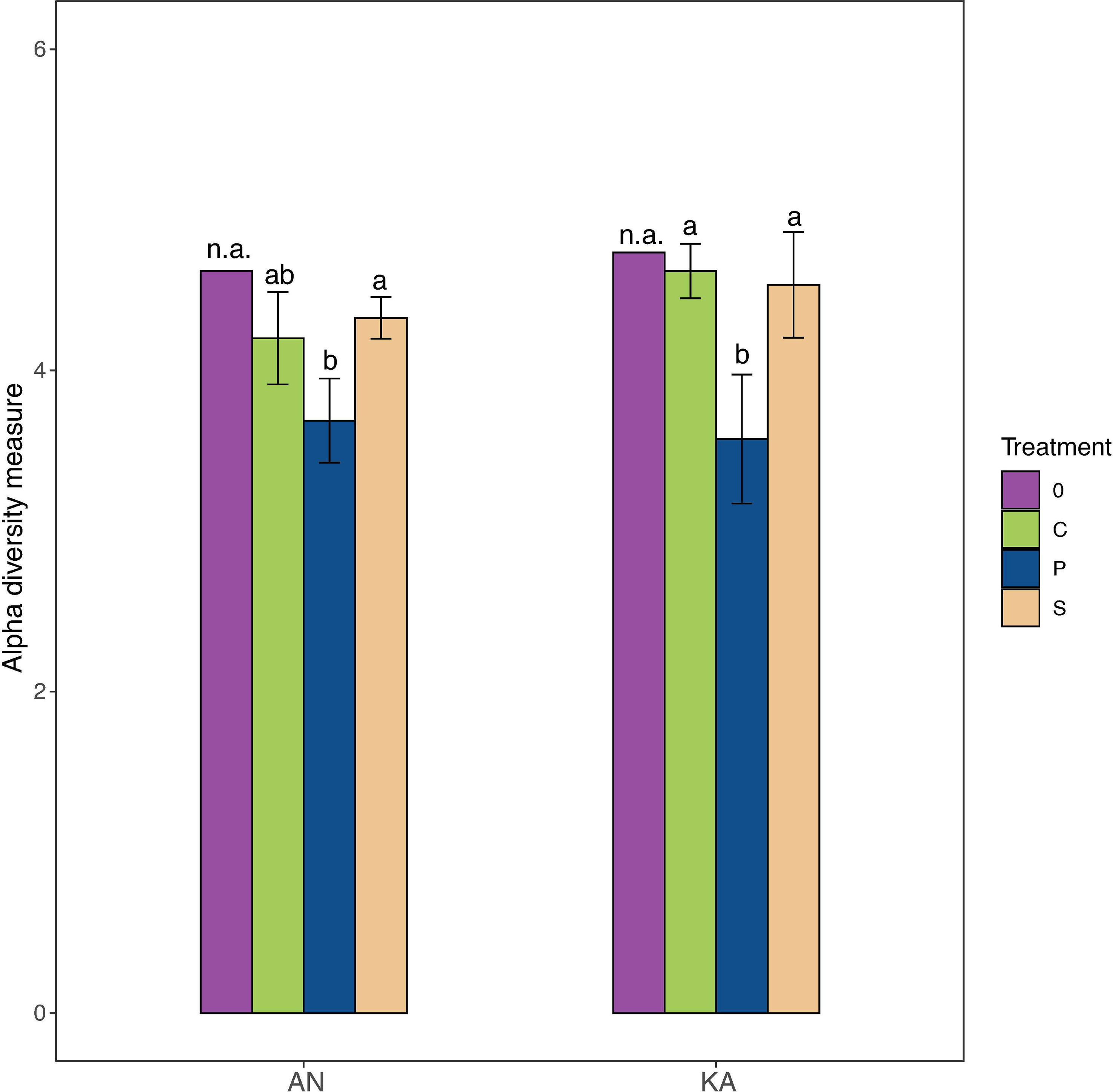
Figure 3 Alpha diversity (Shannon–Wiener diversity index) of samples in different treatment for Kalvarsskatan (KA) and Ängerån (AN) at the start (day 0, before DOM amendments) and end of the experiment (day 2). The colours in the graph represent different treatments, with purple representing day 0, green representing control, blue representing plankton extract treatment, and brown representing soil extract treatment, day 2. Error bars represent the standard deviation. The letter s “a” and “b” placed above each bar indicate significant differences (α = 0.05) between the treatments (excluding day 0), as determined by Kruskal–Wallis tests.
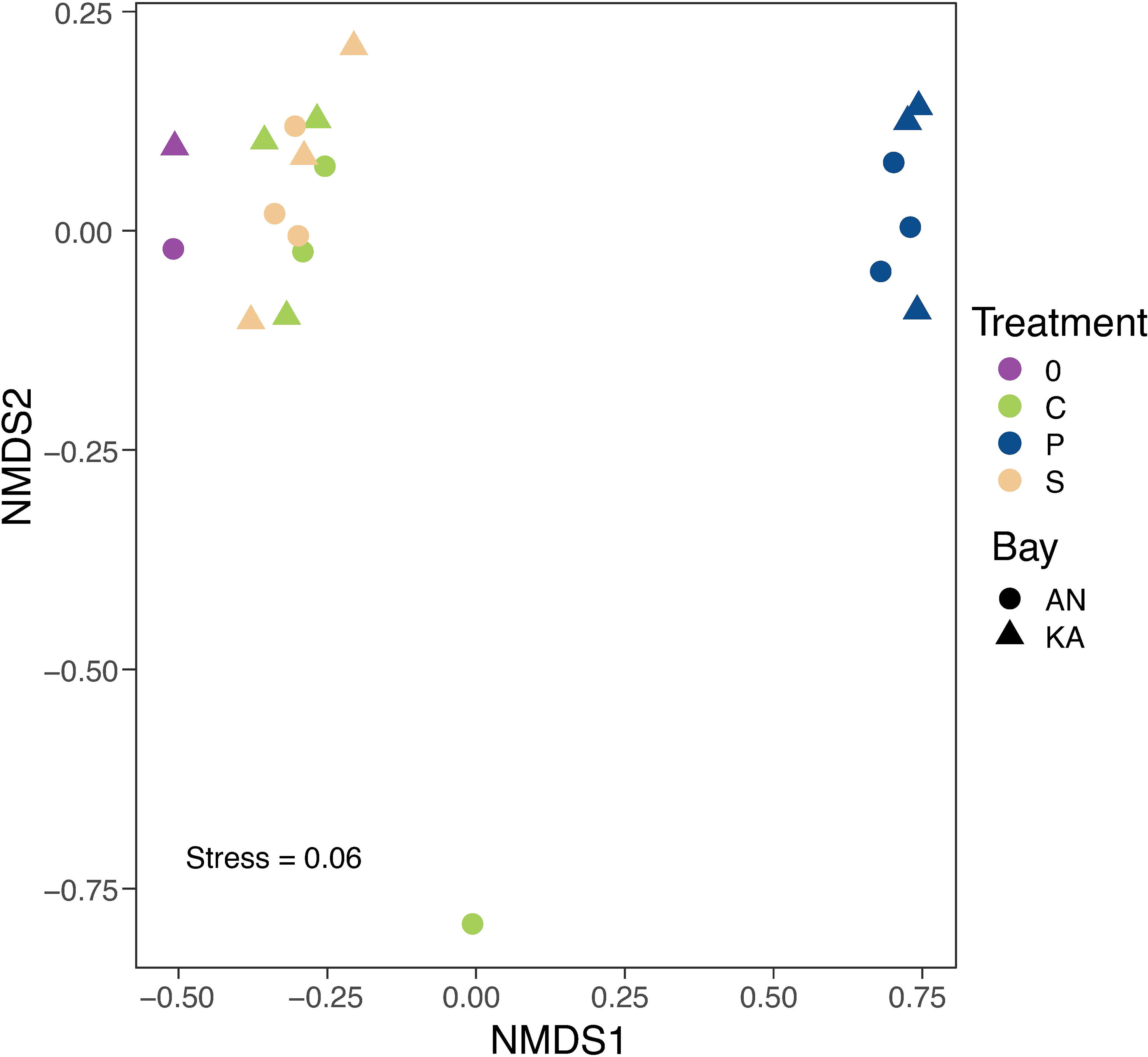
Figure 4 NMDS plot based on Bray-Curtis dissimilarities of ASVs relative abundance. The colours in the graph represent different treatments, with purple representing day 0, green representing control, blue representing plankton extract treatment, and brown representing soil extract treatment, day 2. The shapes represent different bays, with triangles representing KA and circles representing AN. The stress value is 0.06 indicates that the NMDS plot provides a highly accurate representation in reduced dimensions.
The community composition at the class level was quite similar in both bays, with the Gammaproteobacteria and Alphaproteobacteria being the major class observed, followed by Verricucomicrobiae (Figure 5). However, we observed differences in the relative abundance of these sub-phyla between the treatments. Specifically, the Gammaproteobacteria showed the highest percentages in the plankton extract treatment compared to the soil extract and control treatments. On the other hand, the Alphaproteobacteria did not show much difference between the treatments (Figure 5). These observations suggest that the different treatments may have a selective effect on the abundance of certain bacterial taxa. Gammaproteobacteria were in general promoted by plankton extract (Figure S3), the differential abundance analysis between plankton extract treatment and control showed that ~85% of the increased abundance ASVs were Gammaproteobacteria (58 out of 67 in KA and 39 out of 47 in AN) (Table S4). More specially, the bacterial community in the plankton extract treatment had higher relative abundance of ASVs from genera Aeromonas, Shewanella and Motilimonas. There was a significant increase in the abundance of some taxa in both bays, for example genera Aeromonas (ASV3, ASV14), Shewanella (ASV8) and Motilimonas (ASV17) increased over 100-fold, and from rare taxa in the initial community became dominant taxa after 2 days incubation (Figure 6). The bacterial community in the soil extract treatment had higher relative abundance of ASVs from class Actinobacteria and Verrucomicrobiae, but we identified no ASV as significantly different when comparing the soil extract treatment to the control.
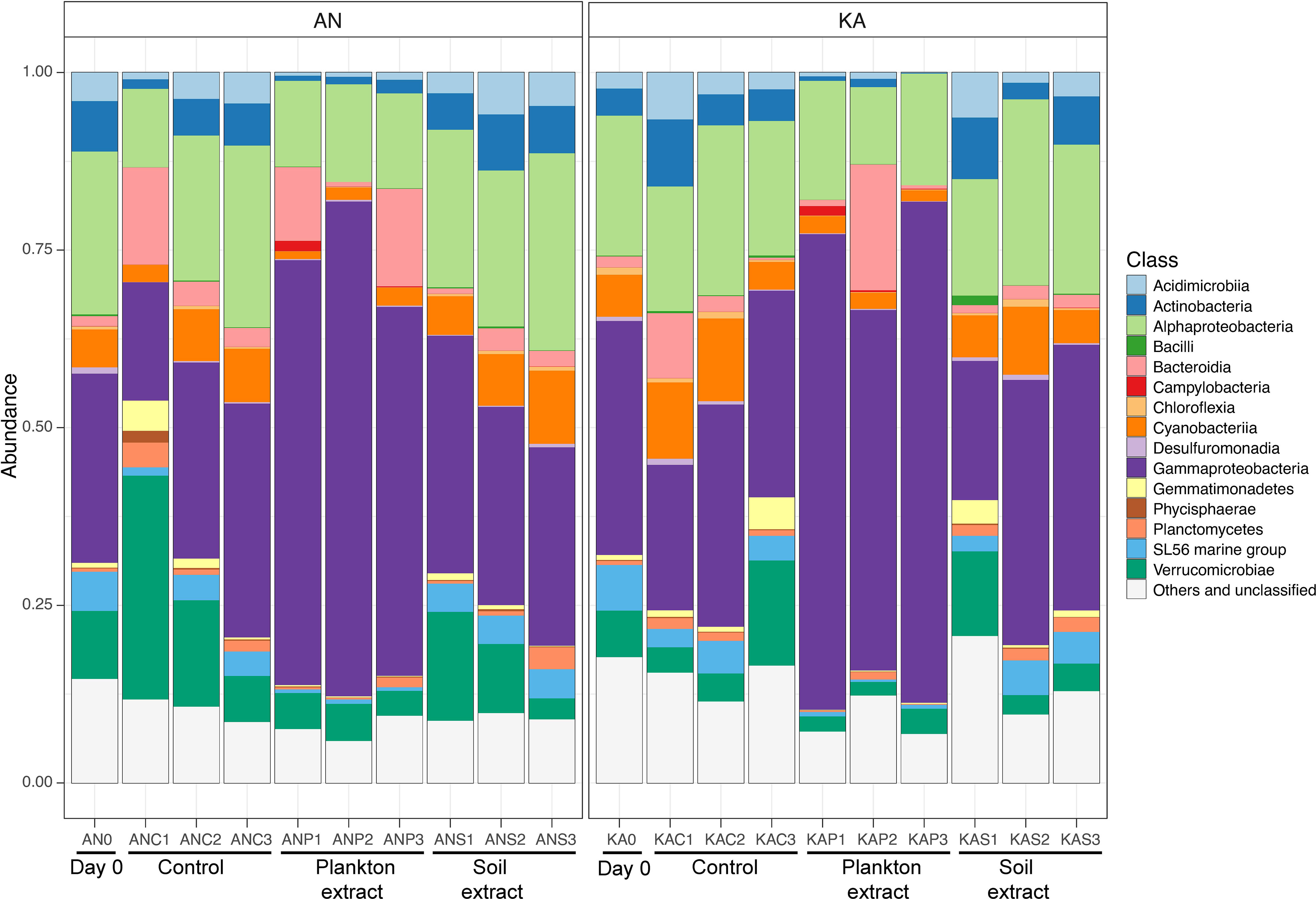
Figure 5 Relative abundance of major bacterial taxa at the class level at the start (day 0, before amendments) and end (day 2) of the experiment, in Kalvarsskatan (KA) and Ängerån (AN). AN0 indicates the start sample in Ängerån, and ANC1, ANC2, and ANC3 indicate triplicate samples of the control taken on day 2 in Ängerån. ANP1, ANP2, and ANP3 indicate triplicate samples of the plankton extract treatment taken on day 2 in Ängerån, and ANS1, ANS2, and ANS3 indicate triplicate samples of the soil extract treatment taken on day 2 in Ängerån. KA0 indicates the start sample in Kalvarsskatan, and KAC1, KAC2, and KAC3 indicate triplicate samples of the control taken on day 2 in Kalvarsskatan. KAP1, KAP2, and KAP3 indicate triplicate samples of the plankton extract treatment taken on day 2 in Kalvarsskatan, and KAS1, KAS2, and KAS3 indicate triplicate samples of the soil extract treatment taken on day 2 in Kalvarsskatan. All unidentified and low-abundance ASVs (<0.1%) as “Others and unclassified”.
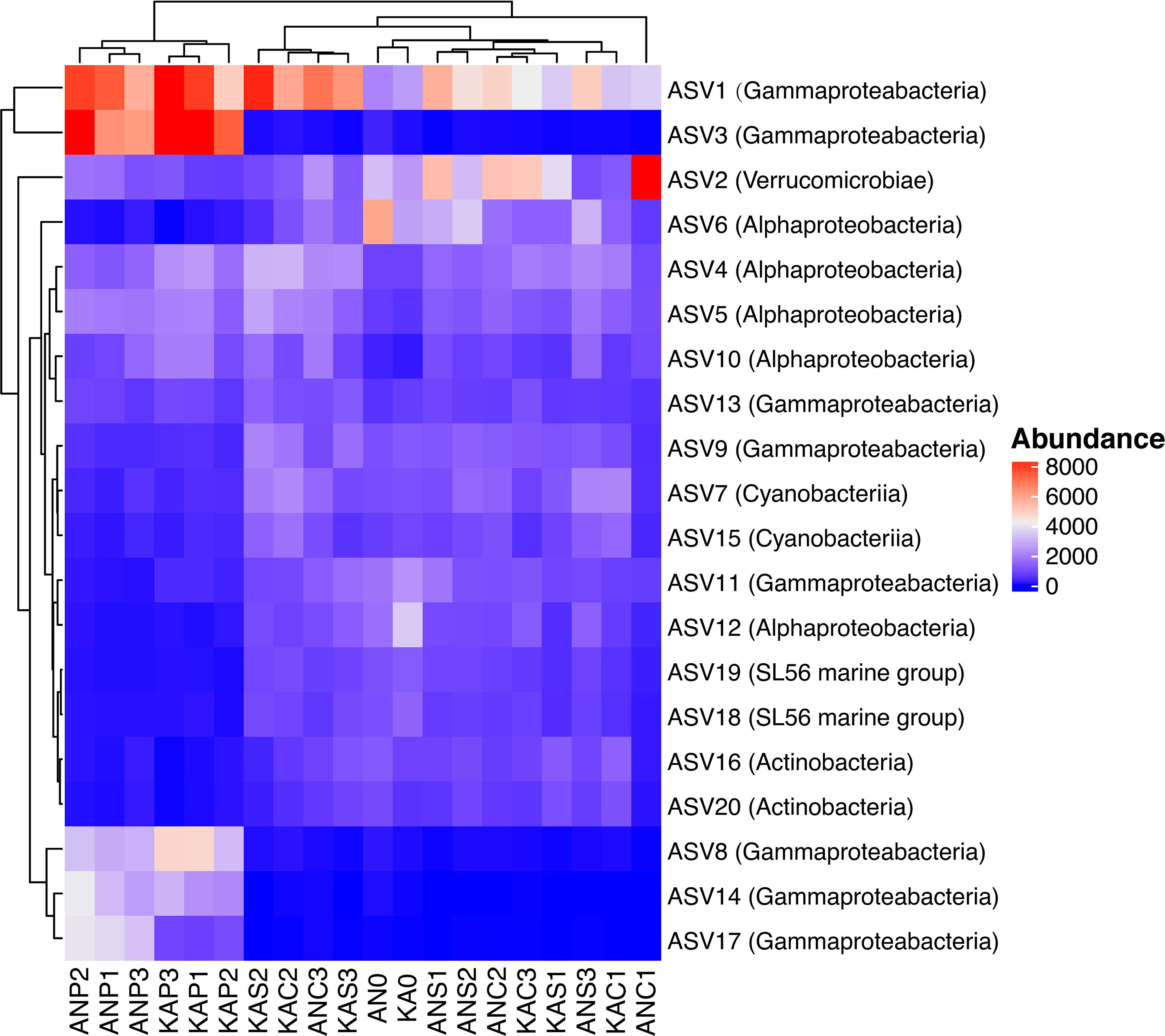
Figure 6 Heatmap of the 20 most abundant ASVs in different treatment, each column in the heatmap is an individual sample, each row is an ASV, with the corresponding taxonomic classification indicated at the class level in the parentheses. Clusters based on ASV abundance values. 0: day 0, C: control day 2, P: plankton extract treatment and S: soil extract treatment day 2." to "AN0 indicates the start sample in Ängerån, and ANC1, ANC2, and ANC3 indicate triplicate samples of the control taken on day 2 in Ängerån. ANP1, ANP2, and ANP3 indicate triplicate samples of the plankton extract treatment taken on day 2 in Ängerån, and ANS1, ANS2, and ANS3 indicate triplicate samples of the soil extract treatment taken on day 2 in Ängerån. KA0 indicates the start sample in Kalvarsskatan, and KAC1, KAC2, and KAC3 indicate triplicate samples of the control taken on day 2 in Kalvarsskatan. KAP1, KAP2, and KAP3 indicate triplicate samples of the plankton extract treatment taken on day 2 in Kalvarsskatan, and KAS1, KAS2, and KAS3 indicate triplicate samples of the soil extract treatment taken on day 2 in Kalvarsskatan.
Carbon metabolism-associated genes and relevant taxa
Gene Ontology Annotations of the most differentially abundant genes (DAGs) between plankton extract treatment and control, showed enrichment of genes involved in aminoglycan catabolic processes, peptidoglycan catabolic process and chitin catabolic process (Figure 7). Accordingly, we focused on the analysis of genes related to carbohydrate degradation. Our gene profile analysis revealed the presence of DAGs encoding for the carbohydrate-active enzymes glycoside hydrolases (GHs), polysaccharide lyases (PLs), carbohydrate esterases (CEs), and auxiliary activities (AAs) (Table S5). Based on literature survey, we selected the most enriched CAZyme-coding genes which actively responded to the addition of plankton extract and soil extract (Table 3).
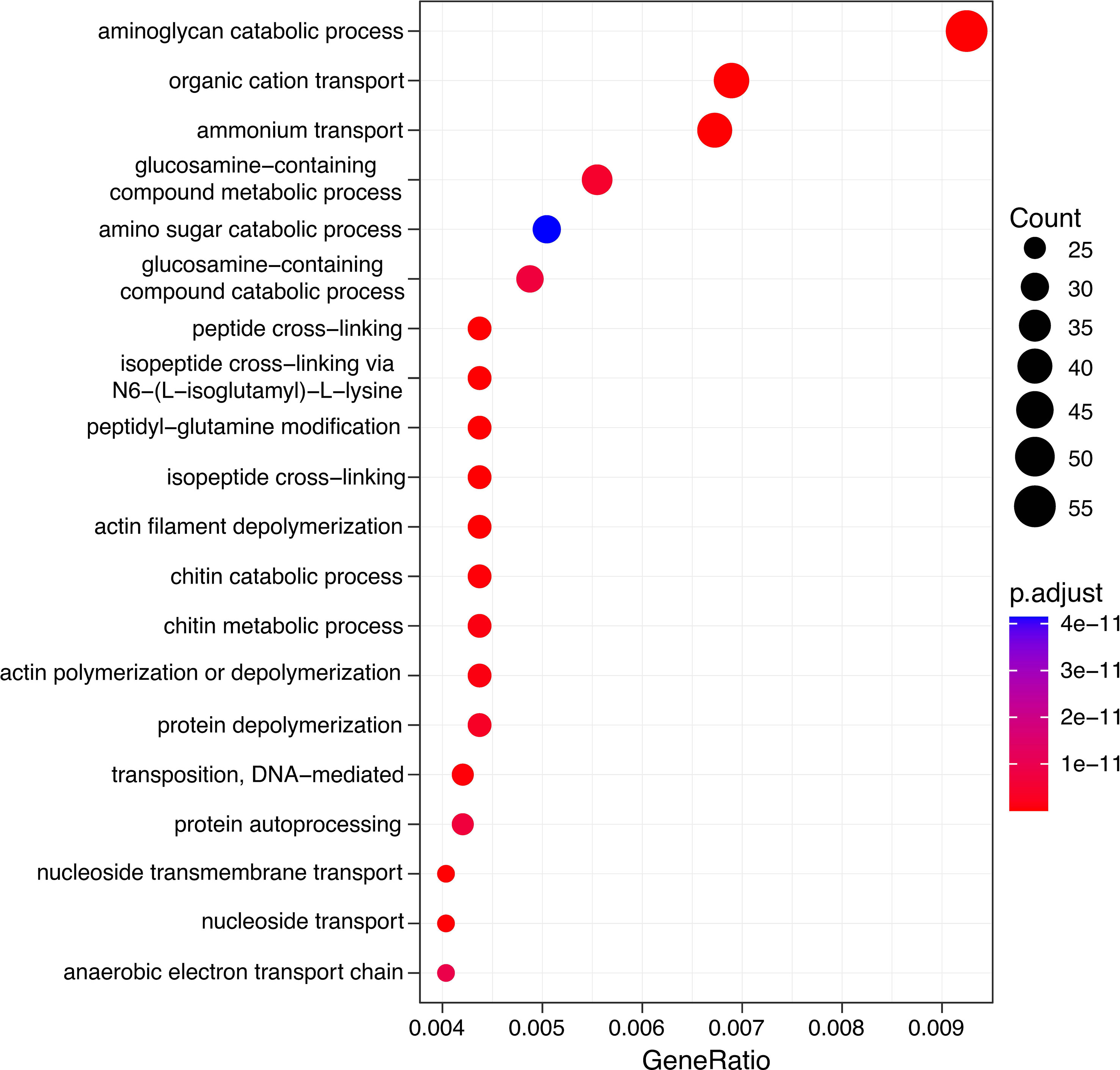
Figure 7 Gene ontology (GO) analysis of differentially abundant genes in plankton extract treatment compared to control.
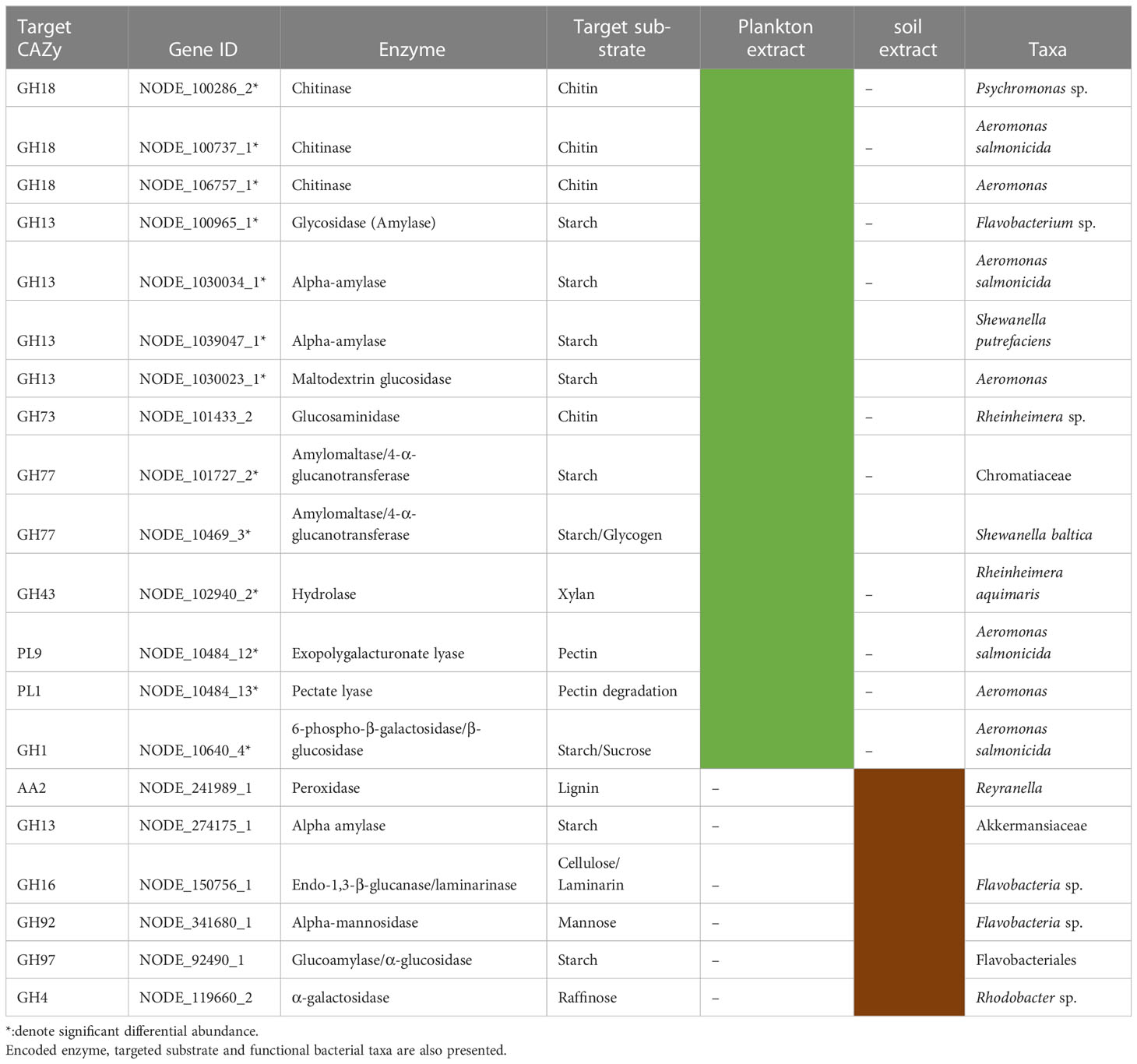
Table 3 List of selected enriched genes coding for carbohydrate active enzymes (CAZymes) induced by addition of plankton extract and soil extract.
The most abundant CAZy families predicted under the plankton extract treatment belonged to GHs, and among them, Chitinase (GH18), alpha-amylase and maltodextrin glucosidase (GH13), glucosaminidase (GH73), and amylomaltase/4-α-glucanotransferase (GH77) were responsible for the possible degradation of chitin, starch, and glycogen (Table 3). In contrast, under the soil extract treatment, we observed a higher abundance of genes encoding endo-1,3-β-glucanase/ laminarinase (GH16) by Flavobacteria, and a gene encoding peroxidase (AA2) by the genus Reyranella, which may have a function in the degradation of cellulose and lignin, respectively (Table 3). The heatmap of the selected genes encoding for carbohydrate active enzymes (CAZymes) showed their different abundance across different treatments (Figure S4).
Discussion
The results of this study showed that the addition of different DOM types had varying effects on the composition, diversity and carbohydrate metabolism-related gene profiles of the bacterial communities. In general, the addition of autochthonous, plankton derived DOM, induced much larger effects on the bacterial community than the addition of allochthonous, terrestrial DOM. Bacterial production was significantly higher in response to the plankton extract treatment compared to the control in both bays, while the soil extract treatment did not result in such big increase. The high bacterial production in the plankton extract microcosms can be explained by higher bioavailability of the added carbon and higher concentrations of phosphorous compared to the soil extract incubation. The DOC consumption, bacterial abundance, and bacterial production showed that the bacterial community responded rapidly in the plankton extract treatment (Table 2, Figure 2). Our findings suggest that the bacterial community exhibited similar responses to various types of DOM in both bays, indicating a general pattern of community-level response in the northern Baltic Sea.
Alpha-diversity – fast response of the bacterial community to plankton-derived DOM while lack of response to soil-derived DOM
The DOM composition in marine systems has been shown to be highly dynamic and complex (Benner and Amon, 2015), potentially selecting for bacterial communities with high species richness/diversity and large functional redundancy. However, our study found that the addition of plankton-derived DOM to microcosms resulted in a decrease in species richness, as measured by Shannon-Wiener diversity, while the addition of allochthonous, terrestrial DOM did not significantly affect the diversity compared to the control (Figure 3).
This decrease in species richness in response to plankton-derived DOM could be due to competition for resources. Plankton-derived DOM may contain high-quality organic matter that supports fast-growing, competitive bacterial taxa, leading to the outcompeting of less-competitive taxa and a reduction in diversity (Teeling et al., 2012). Additionally, plankton-derived DOM may select for specific bacterial taxa that are better adapted to utilize this type of organic matter, resulting in a shift in community composition towards more specialized groups, further reducing diversity (Lindh and Pinhassi, 2018).
One possible explanation for the lack of response to soil extract inputs could be related to the lability of the DOM. While previous studies have demonstrated the importance of tDOM for marine bacterial communities and highlighted their positive response to tDOM inputs (Figueroa et al., 2021), it is possible that the soil extract used in our study was not as labile as the tDOM in previous studies. Moreover, the nutrient content could also influence the response of bacterial communities to DOM inputs, while the nutrient in the soil extract was quite low in our study. Furthermore, our study was conducted over a short time frame (2 days), which may not be sufficient to capture the functional response of the bacterial community to tDOM inputs. Longer-term experiments and metatranscriptomic or metaproteomic approaches would be needed to better understand the functional response of the bacterial community to soil-derived DOM inputs.
Understanding how different types of organic matter affect bacterial communities can help predict how coastal ecosystems may respond to changes in DOM inputs, such as those resulting from climate change (Andersson et al., 2015). Additionally, understanding the mechanisms behind how specific bacterial taxa utilize different types of organic matter can enhance our comprehension of carbon cycling processes. To achieve this, the potential role of specific bacterial taxa in DOM utilization should be investigated.
Labile plankton-derived DOM induces fast shifts of the bacterial community composition
In both bays, Gammaproteobacteria were promoted when plankton extract was added to the microcosms. Their relative abundance increased from ca. 25 to 75%, thus dominating the bacterial community in this treatment (Figure 5). For example, the data in this study showed that the abundance of the fish pathogen Aeromonas salmonicida (Gammaproteobacteria) (McCarthy, 1977) increased in plankton extract treatment. A previous study showed that a representative for coastal Gammaproteobacteria, Shewanella baltica, harbors genes for degradation of labile DOM (Zhao et al., 2021). The gene profile analysis in this study however showed that Gammaproteobacteria have diverse metabolic functions potentially enabling them to utilize both refractory and labile substrates. Nevertheless, the differential gene abundance analysis showed that, compared to the control, the addition of plankton extract led to increased abundance of genes coding for carbohydrate-active enzymes degrading labile food substrates. The metabolic specialization of Gammaproteobacteria thus gave them a competitive advantage in the exploitation of labile source, while other bacterial groups were outcompeted in this short time experiment.
In contrast, when soil extract was added as food resource, the bacterial community composition did not change too much compared to the control (Figure 3). This indicates that the initial conditions of the coastal environment were similar refractive to the soil extract, which contains high molecular weight terrestrial DOM (Pettersson et al., 1997; Skoog et al., 2011). We performed a short-time experiment, but the bacterial community will be exposed to increased terrestrial DOM during longer periods of time according to a climate change scenario (Meier et al., 2022). The water turnover time in the Gulf of Bothnia is 5-6 years (Snoeijs-Leijonmalm et al., 2017). Slow growing bacteria did not show any change in the experiment, but in a longer run, we expect the composition of the coastal bacterial community to change towards an increased importance of Actinobacteria and Bacteroidetes, as they have been shown to be dominant in the northern Baltic Sea coast during the spring river flush of terrestrial matter (Figueroa et al., 2021), and in particularly Bacteroidetes which has been reported to responsive to elevated terrestrial DOM (Traving et al., 2017).
Our results conform to previous studies suggesting that the DOM composition in land-sea transitional areas, seasonal cycles and phytoplankton blooms affect the bacterial community composition and function (Lindh et al., 2015b; Mühlenbruch et al., 2018; Broman et al., 2019). However, Langenheder et al. (2005) conducted a study to investigate the relationship between bacterial community composition and functioning in freshwater lakes from mid Sweden. They analyzed the bacterial community composition and the metabolic activity of the bacteria and found that, although there was some correlation between bacterial community composition and function, the relationship was weak. Likely, a certain degree of change of the DOM composition is needed in order to change the bacterial community composition, and the DOM composition in the soil extract and the original coastal water were too similar to induce any major change of the bacterial community composition and function at a short time-scale.
Autochthonous DOM enriches genes encoding for enzymes degrading both labile and refractory carbohydrate substrates
Heterotrophic bacteria utilise DOM based on the molecular weight i.e. low-molecular-weight (LMW) (oligosaccharides, amino acids) and high molecular weight (HMW) polysaccharides. Both the soil and plankton extracts were assumed to at least partly consist of hydrolysable high molecular weight compounds (Pettersson et al., 1997; Akita et al., 2016; Mühlenbruch et al., 2018; Zhao et al., 2021). Bacterial exo-enzymes with hydrolytic properties, for example chitinases, would enable degradation of the DOM compounds, and assist in the assimilation of the organic carbon into the bacterial food web (Mühlenbruch et al., 2018).
Our study found that the addition of plankton-derived dissolved organic matter (DOM) resulted in a more than 100-fold increase in the abundance of genes coding for enzymes that are involved in carbohydrate degradation pathways and degrade various polysaccharides, including chitin, starch, glycogen, xylan, and pectin, compared to the control group (Figure 7, Table S5). Chitin is a major component of the exoskeleton of zooplankton, such as copepods (Jeuniaux et al., 1989), while glycogen and starch are the storage compounds of cyanobacteria and other autotrophic plankton (Mühlenbruch et al., 2018). The plankton we collected for our study was dominated by filamentous cyanobacteria and mesozooplankton, suggesting that the plankton extract we used was enriched with chitin, glycogen, and starch, all of which are labile carbon substrates for bacterial utilization. Bacteria, especially Gammaproteobacteria are the major degrader of chitin in aquatic systems, and their chitinases are mostly found in family 18 of glycoside hydrolase families, but are also annotated to other families (Adrangi and Faramarzi, 2013; Beier and Bertilsson, 2013; Wang et al., 2019; Jiang et al., 2022). According to the CAZy database, the α-amylases which hydrolyze starch and glycogen, were categorized in glycoside hydrolase family 13 (GH13) (Drula et al., 2022). Thus, our observed increase in the abundance of genes coding for chitinases and α-amylases in response to the addition of plankton extract might be due to the presence of the GH18 and GH13 families in the identified taxa.
Surprisingly, genes for enzymatic degradation of xylan, pectin and cellulose were also enriched by plankton extract addition, even though these compounds originate mostly from terrestrial systems (Pettersson et al., 1997; Akita et al., 2016; Zhao et al., 2021). Multiple genes with the same function could occur in a single organism (e.g. Fuchs et al., 1986; Colatriano et al., 2018), which may repeat similar sequences of genes by gene duplication or acquisition of genes from other organisms by lateral gene transfer (Marri et al., 2006; Colatriano et al., 2018). Multiple enzymes of similar function within an individual bacterium could increase the metabolic potential to utilize carbon and enhance the substrate utilization efficiency compared to other organisms. Probably such metabolic capacity could make specific bacteria groups more successful in the bacterial community under certain environmental conditions (Beier and Bertilsson, 2013; Colatriano et al., 2018).
In our study, the enriched genes coding for polysaccharide degradation enzymes were mostly assigned to Gammaproteobacteria (eg. Aeromonas salmonicida; Shewanella baltica) and Bacteroidetes (eg. Flavobacterium sp.) in the plankton extract addition treatment (Table 3). Aeromonas salmonicida harbours various genes simultaneously coding for chitin, starch, pectin and cellulose degradation, Shewanella baltica for starch/glycogen degradation. These observations agree with increasing relative abundance of the taxa from Gammaproteobacteria, for example, ASV3 (Aeromonas), ASV14 (Aeromonas) and ASV8 (Shewanella) (Figure 6, Table S1). Xylan is a relatively refractory organic compound, originating from terrestrial systems, which was expected to be enriched in soil extract amended microcosms together with pectin, cellulose and lignin. Interestingly, we observed genes coding for xylan decomposition to be enriched as well in the plankton extract treatment, which was assigned to taxa Rheinheimera aquimaris. One of the possible explanations can be a “priming effect”, where degradation of labile organic matter stimulates the decomposition of soil extract compounds following the supply of the plankton extract (Bianchi, 2011), but this could also be due to metabolic plasticity of Rheinheimera, which support diverse metabolic processes (Lindh et al., 2015b). From our study, we can draw the conclusion that Flavobacterium sp. also has metabolic plasticity, as it harbours genes for cellulose, starch and mannose degradation. We observed increased gene abundances under both plankton extract addition and soil extract addition compared to the control. Nevertheless, our study indicated that genes encoding for lignin degradation enzymes from Reyranella had higher abundance in soil extract addition than in the control, even though significant difference could not be proven.
Taken together, our study complies with earlier studies showing that autochthonous DOM select for the opportunistic bacteria especially Gammaproteobacteria and Flavobacteriia (Beier and Bertilsson, 2013). However, in soil extract addition we only found signs of increased abundances of genes encoding for lignin degradation. Probably long-term studies are needed to get evidence for soil extract being such a driver.
Conclusion and outlook
Our study demonstrates how coastal bacteria can respond compositionally and functionally to climate-induced shifts in dissolved organic matter. The addition of plankton extract induced a change of the bacterial community composition and reduced the species richness, while no significant changes occurred with soil extract. Various genes related to carbohydrate metabolism, such as the degradation of chitin, were enriched by plankton extract amendment, while soil extract only showed slight signs of upregulating genes for lignin degradation. Taken together, coastal bacterial communities showed fast responses to inputs of highly bioavailable autochthonous substrates, while terrestrial matter was not quickly degraded and has minor influence on the bacterial community. We acknowledge the limitations of our short-term study and recognize the need for more comprehensive investigations to better understand the bacterial degradation of terrestrial dissolved organic matter (DOM) in the land-sea transitional zone. Long-term experiments or field studies, in combination with metatranscriptomic or metaproteomic approaches, could provide valuable insights into the functions of slow-growing bacteria and the gradual degradation processes. Such studies would enhance our understanding of how the bacterial community contributes to the overall degradation of terrestrial DOM in the land-sea transitional zone. This information is critical for assessing the impacts of climate change on coastal ecosystems and developing protective strategies.
Data availability statement
The datasets presented in this study can be found in online repositories. The names of the repository/repositories and accession number(s) can be found below: https://www.ncbi.nlm.nih.gov/, PRJNA915349.
Author contributions
LZ, SB and AA conceived the study. LZ and SB carried out the microcosm experiment. SB performed microbial analyses and compiled the environmental data. LZ performed the molecular work and bioinformatics. KR performed the gene analysis related to carbohydrate metabolism. LZ wrote the paper together with all authors. All authors contributed to the article and approved the submitted version.
Funding
This project was financed by the Swedish research council FORMAS (FR-2019/0007), Umeå Marine Sciences Centre, and the Swedish research environment EcoChange.
Acknowledgments
We thank the staff at Umeå Marine Sciences Center and Björn Karlsson for excellent support during performance of the field experiment and for chemical analyses. We thank Eric Cope for the supply of the 16S primers. The bioinformatic data analysis was enabled by resources provided by the Swedish National Infrastructure for Computing (SNIC) at High Performance Computing Center North (HPC2N) partially funded by the Swedish Research Council through grant agreement no. 2018-05973.
Conflict of interest
The authors declare that the research was conducted in the absence of any commercial or financial relationships that could be construed as a potential conflict of interest.
Publisher’s note
All claims expressed in this article are solely those of the authors and do not necessarily represent those of their affiliated organizations, or those of the publisher, the editors and the reviewers. Any product that may be evaluated in this article, or claim that may be made by its manufacturer, is not guaranteed or endorsed by the publisher.
Supplementary material
The Supplementary Material for this article can be found online at: https://www.frontiersin.org/articles/10.3389/fmars.2023.1130855/full#supplementary-material
Supplementary Table 1 | The table presents an overview of the sequence count and taxonomic annotation per ASV obtained from 16S rRNA sequencing.
Supplementary Table 2 | Alpha diversity metrics assessed by rarefied count.
Supplementary Table 3 | The table presents an overview of the potential carbohydrate active enzymes (CAZymes) encoding genes.
Supplementary Table 4 | The table showing the significant differential abundant ASVs and their taxonomic annotation between plankton extract treatment to control.
Supplementary Table 5 | The table showing the differential abundant genes and their taxonomic annotation between plankton extract treatment to control or between soil extract treatment to control.
References
Adrangi S., Faramarzi M. A. (2013). From bacteria to human: a journey into the world of chitinases. Biotechnol. Adv. 31, 1786–1795. doi: 10.1016/j.biotechadv.2013.09.012
Akita H., Kimura Z., Mohd Yusoff M. Z., Nakashima N., Hoshino T. (2016). Isolation and characterization of burkholderia sp. strain CCA53 exhibiting ligninolytic potential. Springerplus 5, 596. doi: 10.1186/s40064-016-2237-y
Andersson A., Ahlinder J., Mathisen P., Hägglund M., Bäckman S., Nilsson E., et al. (2018). Predators and nutrient availability favor protozoa-resisting bacteria in aquatic systems. Sci. Rep. 8, 8415. doi: 10.1038/s41598-018-26422-4
Andersson A., Meier H. E., Ripszam M., Rowe O., Wikner J., Haglund P., et al. (2015). Projected future climate change and Baltic Sea ecosystem management. Ambio 44 (Suppl 3), 345–356. doi: 10.1007/s13280-015-0654-8
Andersson A. F., Riemann L., Bertilsson S. (2010). Pyrosequencing reveals contrasting seasonal dynamics of taxa within Baltic Sea bacterioplankton communities. ISME J. 4, 171–181. doi: 10.1038/ismej.2009.108
Azam F., Malfatti F. (2007). Microbial structuring of marine ecosystems. Nat. Rev. Microbiol. 5, 782–791. doi: 10.1038/nrmicro1747
Beier S., Bertilsson S. (2013). Bacterial chitin degradation-mechanisms and ecophysiological strategies. Front. Microbiol. 4, 149. doi: 10.3389/fmicb.2013.00149
Benner R., Amon R. M. (2015). The size-reactivity continuum of major bioelements in the ocean. Ann. Rev. Mar. Sci. 7, 185–205. doi: 10.1146/annurev-marine-010213-135126
Berglund J., Müren U., Båmstedt U., Andersson A. (2007). Efficiency of a phytoplankton-based and a bacterial-based food web in a pelagic marine system. Limnol. Oceanogr. 52, 121–131. doi: 10.4319/lo.2007.52.1.0121
Bertilsson S., Jones J. B. (2003). “Supply of dissolved organic matter to aquatic ecosystems: autochthonous sources,” in Aquatic ecosystems. Eds. Findlay S. E. G., Sinsabaugheds R. L. (Burlington: Academic Press), 3–24.
Bianchi T. S. (2011). The role of terrestrially derived organic carbon in the coastal ocean: a changing paradigm and the priming effect. Proc. Natl. Acad. Sci. U.S.A. 108, 19473–19481. doi: 10.1073/pnas.1017982108
Boyer F., Mercier C., Bonin A., Le Bras Y., Taberlet P., Coissac E. (2016). Obitools: a unix-inspired software package for DNA metabarcoding. Mol. Ecol. Resour. 16, 176–182. doi: 10.1111/1755-0998.12428
Broman E., Asmala E., Carstensen J., Pinhassi J., Dopson M. (2019). Distinct coastal microbiome populations associated with autochthonous-and allochthonous-like dissolved organic matter. Front. Microbiol. 10, 2579. doi: 10.3389/fmicb.2019.02579
Bruhn A. D., Stedmon C. A., Comte J., Matsuoka A., Speetjens N. J., Tanski G., et al. (2021). Terrestrial dissolved organic matter mobilized from eroding permafrost controls microbial community composition and growth in Arctic coastal zones. Front. Earth Sci. 9, 640580. doi: 10.3389/feart.2021.640580
Callahan B. J., McMurdie P. J., Rosen M. J., Han A. W., Johnson A. J., Holmes S. P. (2016). DADA2: high-resolution sample inference from illumina amplicon data. Nat. Methods 13, 581–583. doi: 10.1038/nmeth.3869
Carlson M., Pagès H. (2023) AnnotationForge: tools for building SQLite-based annotation data packages. Available at: https://bioconductor.org/packages/AnnotationForge.
Chen S., Zhou Y., Chen Y., Gu J. (2018). Fastp: an ultra-fast all-in-one FASTQ preprocessor. Bioinformatics 34, i884–i890. doi: 10.1093/bioinformatics/bty560
Colatriano D., Tran P. Q., Guéguen C., Williams W. J., Lovejoy C., Walsh D. A. (2018). Genomic evidence for the degradation of terrestrial organic matter by pelagic Arctic ocean chloroflexi bacteria. Commun. Biol. 1, 90. doi: 10.1038/s42003-018-0086-7
Cole J. J., Prairie Y. T., Caraco N. F., McDowell W. H., Tranvik L. J., Striegl R. G., et al. (2007). Plumbing the global carbon cycle: integrating inland waters into the terrestrial carbon budget. Ecosystems 10, 172–185. doi: 10.1007/s10021-006-9013-8
D’Andrilli J., Junker J. R., Smith H. J., Scholl E. A., Foreman C. M. (2019). DOM composition alters ecosystem function during microbial processing of isolated sources. Biogeochemistry 142, 281–298. doi: 10.1007/s10533-018-00534-5
Davis N. M., Proctor D. M., Holmes S. P., Relman D. A., Callahan B. J. (2018). Simple statistical identification and removal of contaminant sequences in marker-gene and metagenomics data. Microbiome 6, 226. doi: 10.1186/s40168-018-0605-2
de Mendiburu F. (2021) Agricolae: statistical procedures for agricultural research. Available at: https://CRAN.R-project.org/package=agricolae.
Drake T. W., Raymond P. A., Spencer R. G. M. (2018). Terrestrial carbon inputs to inland waters: a current synthesis of estimates and uncertainty. Limnol. Oceanogr. 3, 132–142. doi: 10.1002/lol2.10055
Drula E., Garron M. L., Dogan S., Lombard V., Henrissat B., Terrapon N. (2022). The carbohydrate-active enzyme database: functions and literature. Nucleic Acids Res. 50, D571–D577. doi: 10.1093/nar/gkab1045
Figueroa D., Capo E., Lindh M. V., Rowe O. F., Paczkowska J., Pinhassi J., et al. (2021). Terrestrial dissolved organic matter inflow drives temporal dynamics of the bacterial community of a subarctic estuary (northern Baltic Sea). Environ. Microbiol. 23, 4200–4213. doi: 10.1111/1462-2920.15597
Fuchs R. L., McPherson S. A., Drahos D. J. (1986). Cloning of a Serratia marcescens gene encoding chitinase. Appl. Environ. Microbiol. 51, 504–509. doi: 10.1128/aem.51.3.504-509.1986
Fuhrman J. A., Azam F. (1982). Thymidine incorporation as a measure of heterotrophic bacterioplankton production in marine surface waters: evaluation and field results. Mar. Biol. 66, 109–120. doi: 10.1007/BF00397184
Grasshoff K., Kremling K., Ehrhardt M. (1999). Methods of seawater analysis. 3rd Edition (Weinheim, Germany: Wiley-VCH).
Hadley W. (2016). ggplot2: elegant graphics for data analysis-Hadley wickham (USA: Springer-Verlag New York).
Hallfors G. (2004). “Checklist of Baltic Sea phytoplankton species (including some heterotrophic protistan groups),” in HELCOM, balt. Sea environ. proc (Helsinki, Finland: Helsinki Commission).
Herlemann D. P., Lundin D., Andersson A. F., Labrenz M., Jürgens K. (2016). Phylogenetic signals of salinity and season in bacterial community composition across the salinity gradient of the Baltic Sea. Front. Microbiol. 7, 1883. doi: 10.3389/fmicb.2016.01883
Hoge F. E., Vodacek A., Blough N. V. (1993). Inherent optical properties of the ocean: retrieval of the absorption coefficient of chromophoric dissolved organic matter from fluorescence measurements. Limnol. Oceanogr. 38, 1394–1402. doi: 10.4319/lo.1993.38.7.1394
Huerta-Cepas J., Szklarczyk D., Heller D., Hernández-Plaza A., Forslund S. K., Cook H., et al. (2019). eggNOG 5.0: a hierarchical, functionally and phylogenetically annotated orthology resource based on 5090 organisms and 2502 viruses. Nucleic Acids Res. 47, D309–D314. doi: 10.1093/nar/gky1085
Hugoni M., Vellet A., Debroas D. (2017). Unique and highly variable bacterial communities inhabiting the surface microlayer of an oligotrophic lake. Aquat. Microb. Ecol. 79, 115–125. doi: 10.3354/ame01825
Jeuniaux C., Voss-Foucart M. F., Poulicek M., Bussers J. C. (1989). “Sources of chitin, estimated from new data on chitin biomass and production,” in Chitin and chitosan. Eds. Skjak-Braek G., Antonsen T., Sandfordeds P. A. (London and New York: Elsevier Applied Science), 3–11.
Jiang W. X., Li P. Y., Chen X. L., Zhang Y. S., Wang J. P., Wang Y. J., et al. (2022). A pathway for chitin oxidation in marine bacteria. Nat. Commun. 13, 5899. doi: 10.1038/s41467-022-33566-5
Jiao N., Herndl G. J., Hansell D. A., Benner R., Kattner G., Wilhelm S. W., et al. (2011). The microbial carbon pump and the oceanic recalcitrant dissolved organic matter pool. Nat. Rev. Microbiol. 9, 555–555. doi: 10.1038/nrmicro2386-c5
Langenheder S., Lindström E. S., Tranvik L. J. (2005). Weak coupling between community composition and functioning of aquatic bacteria. Limnol. Oceanogr. 50, 957–967. doi: 10.4319/lo.2005.50.3.0957
Lindh M. V., Figueroa D., Sjöstedt J., Baltar F., Lundin D., Andersson A., et al. (2015a). Transplant experiments uncover Baltic Sea basin-specific responses in bacterioplankton community composition and metabolic activities. Front. Microbiol. 6, 223. doi: 10.3389/fmicb.2015.00223
Lindh M. V., Pinhassi J. (2018). Sensitivity of bacterioplankton to environmental disturbance: a review of Baltic Sea field studies and experiments. Front. Mar. Sci. 5, 361. doi: 10.3389/fmars.2018.00361
Lindh M. V., Sjöstedt J., Andersson A. F., Baltar F., Hugerth L. W., Lundin D., et al. (2015b). Disentangling seasonal bacterioplankton population dynamics by high-frequency sampling. Environ. Microbiol. 17, 2459–2476. doi: 10.1111/1462-2920.12720
Logue J. B., Stedmon C. A., Kellerman A. M., Nielsen N. J., Andersson A. F., Laudon H., et al. (2016). Experimental insights into the importance of aquatic bacterial community composition to the degradation of dissolved organic matter. ISME J. 10, 533–545. doi: 10.1038/ismej.2015.131
Love M. I., Huber W., Anders S. (2014). Moderated estimation of fold change and dispersion for RNA-seq data with DESeq2. Genome Biol. 15, 550. doi: 10.1186/s13059-014-0550-8
Marie D., Simon N., Vaulot D. (2005). “Phytoplankton cell counting by flow cytometry,” in Algal culturing techniques. Ed. Andersen R. A. (San Diego: Academic Press), 253–267.
Marri P. R., Bannantine J. P., Golding G. B. (2006). Comparative genomics of metabolic pathways in Mycobacterium species: gene duplication, gene decay and lateral gene transfer. FEMS Microbiol. Rev. 30, 906–925. doi: 10.1111/j.1574-6976.2006.00041.x
McCarthy D. H. (1977). Some ecological aspects of the bacterial fish pathogen - Aeromonas salmonicida. Aquat. Microb. 6, 299–324.
McMurdie P. J., Holmes S. (2013). Phyloseq: an r package for reproducible interactive analysis and graphics of microbiome census data. PloS One 8, e61217. doi: 10.1371/journal.pone.0061217
Meier H. E. M., Kniebusch M., Dieterich C., Gröger M., Zorita E., Elmgren R., et al. (2022). Climate change in the Baltic Sea region: a summary. Earth Syst. Dynam. 13, 457–593. doi: 10.5194/esd-13-457-2022
Meier H. E., Müller-Karulis B., Andersson H. C., Dieterich C., Eilola K., Gustafsson B. G., et al. (2012). Impact of climate change on ecological quality indicators and biogeochemical fluxes in the Baltic sea: a multi-model ensemble study. Ambio 41, 558–573. doi: 10.1007/s13280-012-0320-3
Menden-Deuer S., Lessard E. J. (2000). Carbon to volume relationships for dinoflagellates, diatoms, and other protist plankton. Limnol. Oceanogr. 45, 569–579. doi: 10.4319/lo.2000.45.3.0569
Menzel P., Ng K. L., Krogh A. (2016). Fast and sensitive taxonomic classification for metagenomics with kaiju. Nat. Commun. 7, 11257. doi: 10.1038/ncomms11257
Morya R., Salvachúa D., Thakur I. S. (2020). Burkholderia: an untapped but promising bacterial genus for the conversion of aromatic compounds. Trends Biotechnol. 38, 963–975. doi: 10.1016/j.tibtech.2020.02.008
Mühlenbruch M., Grossart H. P., Eigemann F., Voss M. (2018). Mini-review: phytoplankton-derived polysaccharides in the marine environment and their interactions with heterotrophic bacteria. Environ. Microbiol. 20, 2671–2685. doi: 10.1111/1462-2920.14302
Oksanen J., Simpson G. L., Blanchet F. G., Kindt R., Legendre P., Minchin P. R., et al. (2022) Vegan: community ecology package. Available at: https://CRAN.R-project.org/package=vegan.
Olenina I., Hajdu S., Edler L., Andersson A., Wasmund N., Busch S., et al. (2006). “Biovolumes and size-classes of phytoplankton in the Baltic Sea,” in HELCOM, balt. Sea environ. proc (Helsinki, Finland: Helsinki Commission), 1–144.
Patro R., Duggal G., Love M. I., Irizarry R. A., Kingsford C. (2017). Salmon provides fast and bias-aware quantification of transcript expression. Nat. Methods 14, 417–419. doi: 10.1038/nmeth.4197
Pettersson C., Allard B., Borén H. (1997). River discharge of humic substances and humic-bound metals to the gulf of bothnia. Estuar. Coast. Shelf Sci. 44, 533–541. doi: 10.1006/ecss.1996.0159
Quast C., Pruesse E., Yilmaz P., Gerken J., Schweer T., Yarza P., et al. (2013). The SILVA ribosomal RNA gene database project: improved data processing and web-based tools. Nucleic Acids Res. 41, D590–D596. doi: 10.1093/nar/gks1219
Robinson M. D., McCarthy D. J., Smyth G. K. (2010). edgeR: a bioconductor package for differential expression analysis of digital gene expression data. Bioinformatics 26, 139–140. doi: 10.1093/bioinformatics/btp616
Skoog A., Wedborg M., Fogelqvist E. (2011). Decoupling of total organic carbon concentrations and humic substance fluorescence in a an extended temperate estuary. Mar. Chem. 124, 68–77. doi: 10.1016/j.marchem.2010.12.003
Smith H. J., Dieser M., McKnight D. M., SanClements M. D., Foreman C. M. (2018). Relationship between dissolved organic matter quality and microbial community composition across polar glacial environments. FEMS Microbiol. Ecol. 94, 1–10. doi: 10.1093/femsec/fiy090
Snoeijs-Leijonmalm P., Schubert H., Radziejewska T. (2017). Biological oceanography of the Baltic Sea (Dordrecht, Netherland: Springer Science & Business Media).
Teeling H., Fuchs B. M., Becher D., Klockow C., Gardebrecht A., Bennke C. M., et al. (2012). Substrate-controlled succession of marine bacterioplankton populations induced by a phytoplankton bloom. Science 336, 608–611. doi: 10.1126/science.1218344
Teira E., Nieto-Cid M., Álvarez-Salgado X. A. (2009). Bacterial community composition and colored dissolved organic matter in a coastal upwelling ecosystem. Aquat. Microb. Ecol. 55, 131–142. doi: 10.3354/ame01290
Tikkanen T., Wille'n T. (1992). “Phytoplankton flora,” (Solna, Sweden: The Swedish Environmental Protection Agency).
Traving S. J., Rowe O., Jakobsen N. M., Sørensen H., Dinasquet J., Stedmon C. A., et al. (2017). The effect of increased loads of dissolved organic matter on estuarine microbial community composition and function. Front. Microbiol. 8, 351. doi: 10.3389/fmicb.2017.00351
Utermöhl H. (1958). Zur vervolkommung der quantitativen phytoplankton-methodik. Mitt. Int. Verein. Theor. Angew. Limnol. 9, 1–38.
Wang Y. J., Jiang W. X., Zhang Y. S., Cao H. Y., Zhang Y., Chen X. L., et al. (2019). Structural insight into chitin degradation and thermostability of a novel endochitinase from the glycoside hydrolase family 18. Front. Microbiol. 10, 2457. doi: 10.3389/fmicb.2019.02457
Wu T., Hu E., Xu S., Chen M., Guo P., Dai Z., et al. (2021). clusterProfiler 4.0: a universal enrichment tool for interpreting omics data. Innovation 2, 100141. doi: 10.1016/j.xinn.2021.100141
Zhang H., Yohe T., Huang L., Entwistle S., Wu P., Yang Z., et al. (2018). dbCAN2: a meta server for automated carbohydrate-active enzyme annotation. Nucleic Acids Res. 46, W95–W101. doi: 10.1093/nar/gky418
Keywords: Microcosm experiment, coastal bacteria, dissolved organic matter, Baltic Sea, metagenome
Citation: Zhao L, Brugel S, Ramasamy KP and Andersson A (2023) Bacterial community responses to planktonic and terrestrial substrates in coastal northern Baltic Sea. Front. Mar. Sci. 10:1130855. doi: 10.3389/fmars.2023.1130855
Received: 23 December 2022; Accepted: 04 April 2023;
Published: 19 April 2023.
Edited by:
Songlin Liu, South China Sea Institute of Oceanology (CAS), ChinaReviewed by:
Raju Sekar, Xi’an Jiaotong-Liverpool University, ChinaBenjamin Misson, Université de Toulon, France
Copyright © 2023 Zhao, Brugel, Ramasamy and Andersson. This is an open-access article distributed under the terms of the Creative Commons Attribution License (CC BY). The use, distribution or reproduction in other forums is permitted, provided the original author(s) and the copyright owner(s) are credited and that the original publication in this journal is cited, in accordance with accepted academic practice. No use, distribution or reproduction is permitted which does not comply with these terms.
*Correspondence: Li Zhao, bGkuemhhb0B1bXUuc2U=