- 1Department of Dairy Science, South Dakota State University, Brookings, SD, USA
- 2Department of Dairy Microbiology, Anand Agricultural University, Anand, India
- 3Department of Animal Biotechnology, Anand Agricultural University, Anand, India
- 4Department of Community Medicine, H. M Patel Center for Medical Care and Education, Karamsad, India
- 5Department of Microbiology, H. M Patel Center for Medical Care and Education, Karamsad, India
- 6Department of Medicine, H. M Patel Center for Medical Care and Education, Karamsad, India
- 7Central Research Services, Charutar Arogya Mandal, Karamsad, India
Scope: Probiotic interventions are known to have been shown to influence the composition of the intestinal microbiota in geriatrics. The growing concern is the apparent variation in response to identical strain dosage among human volunteers. One factor that governs this variation is the host gut microbiome. In this study, we attempted to define a core gut metagenome, which could act as a predisposition signature marker of inherent bacterial community that can help predict the success of a probiotic intervention.
Methods and results: To characterize the geriatric gut microbiome, we designed primers targeting the 16S rRNA hypervariable region V2–V3 followed by semiconductor sequencing using Ion Torrent PGM. Among respondents and non-respondents, the chief genera of phylum Firmicutes that showed significant differences are Lactobacillus, Clostridium, Eubacterium, and Blautia (q < 0.002), while in the genera of phylum Proteobacteria included Shigella, Escherichia, Burkholderia and Camphylobacter (q < 0.002).
Conclusion: We have identified potential microbial biomarkers and taxonomic patterns that correlate with a positive response to probiotic intervention in geriatric volunteers. Future work with larger cohorts of geriatrics with diverse dietary influences could reveal the potential of the signature patterns of microbiota for personalized nutrition.
Introduction
An integrative study of the host and its surrounding environment is imperative to comprehend the complex biological system of the human body. As a part of the environment, the human host more than 100 trillion bacteria forming the “in-vironment” (de Wouters et al., 2000) made up of millions of microbial genes in the intestine (Lederberg, 2000). The indigenous microbial community plays an integral role in regulating the host’s physiological, nutritional, and immunological processes (Hooper et al., 2001). The study of the diversity of the indigenous microbial community can explain the host microbe interaction (Gerritsen et al., 2011). The gut microbiota composition changes with age due to physiological reasons and increased use of medications (Bartosch et al., 2004; Mueller et al., 2006; Mariat et al., 2009; Zwielehner et al., 2009). The microbiota of elderly people showed a higher Bacteroidetes/Firmicutes ratio along with a high inter-individual variation in microbiota composition at the phylum level when compared with young adults (Claesson et al., 2011). Studies of the geriatric gut have shown a decline in the count and diversity among Bacteroidetes (Hopkins and Macfarlane, 2002; Woodmansey et al., 2004; Guigoz et al., 2008). Proteolytic bacteria increase on aging in the large bowel, leading to putrefaction (Hopkins et al., 2001). Such changes in intestinal microbiome cause prolonged intestinal transit time and fecal retention among geriatrics (Tiihonen et al., 2009). An understanding of the changes in the microbiome of the elderly has led to the possibility of correcting the dysbiosis by administering probiotics. Probiotics have been successful in increasing the levels of health-promoting bacteria in the fecal microbiota of elderly (Ahmed et al., 2007; Lahtinen et al., 2009; Matsumoto et al., 2009), improving the frequency of bowel movements (Pitkala et al., 2007), Clostridium difficile-associated diarrhea incidence (Ouwehand et al., 2009), and frequency of defecation (An et al., 2010).
The translation of the above-mentioned benefits of probiotics to the host cannot be guaranteed. This could be due to the individual differences with respect to diet, the structure and operations of the gut microbiota, nutrient and energy harvest, variations in human environmental exposures, microbial ecology, and genotype (Turnbaugh et al., 2009). In order to assure uniform outcomes of therapy among subjects, the International Scientific Association for Probiotics and Prebiotics (ISAPP) had come up with recommendations for conducting a well-defined trial (Reid et al., 2010). Briefly, they include (1) clearly define the end goal, (2) design the study by identifying precise parameters and defining the level of response that will be tested, (3) base the selection of the intervention on scientific investigations, and (4) carefully select the study cohort. Inter-individual diversity in responses toward probiotics could also be due to core gut microbiome patterns. Recently, role of microbial biomarkers for determining dietary responsiveness were identified in obese individuals (Korpela et al., 2014) and metabolic diseases (McOrist et al., 2011; Walker et al., 2011; Louis, 2012; Lampe et al., 2013), paving the way for personalized nutrition. This study takes up the challenge to identify the factors that differentiate a respondent from a non-respondent and utilize the findings to define the precise dose and response prognosis. This finding can help design probiotic supplements catering to a niche market defined by age, location, or disease state.
From an Indian perspective, gut metagenomics have been studied in malnourished children (Gupta et al., 2011), obese individuals (Patil et al., 2012), and children of varying nutritional status (Ghosh et al., 2014). It was for the first time in India that the present study was conducted to investigate the elderly gut metagenome to identify microbial biomarkers determining responsiveness of the host to a probiotic therapy. We hypothesized that by studying the baseline gut microbiota diversity of elderly subjects, we could identify a core gut microbiome signature pattern that is likely to positively influence the response of an individual to the probiotic strain. The strain under study, Lactobacillus helveticus MTCC 5463 is an indigenous potential probiotic with in vitro, in vivo, and in silico studies providing suggestive evidences of the strain’s robustness in the gut and transit, adhesion, autoaggregation, colonization, antibacterial property, hypocholesterolemic, and immunomodulatory properties (Senan et al., 2015). The outcome of this study paves the way forward for tailored probiotic therapy.
Materials and Methods
Origin and Maintenance of Bacterial Strains
The indigenous probiotic strain L. helveticus MTCC 5463 (Prajapati et al., 2011) and starter culture Streptococcus thermophilus MTCC 5460 (Prajapati et al., 2013) were maintained by the Department of Dairy Microbiology, Anand Agricultural University, India at −80°C as 15% glycerol stocks and were routinely cultured in de Man, Rogosa, Sharpe (MRS) and M 17 medium, respectively (HiMedia India Ltd., India).
Product Preparation
The test product was a fermented probiotic drink (Lassi) with double toned milk fermented with culture containing S. thermophilus MTCC 5460 and L. helveticus MTCC 5463. The cultures were added at 0.1% each and incubated aerobically till an acidity of 0.8–0.9% lactic acid was obtained. Both the test and placebo products contained sugar and prebiotic honey in a standardized ratio. The control product was made in a similar manner without the addition of MTCC 5463. The shelf life of the fermented drink was 28 days at 4°C, corresponding to the lower level (109 CFU/ml) of strain MTCC 5463.
Participant Selection
Individuals ranging from 64 to 74 years were recruited. Initially, 112 subjects were enrolled in the trial, 36 had to withdraw because of antibiotic consumption. Volunteers were asked to sign the consent form before recruitment. Exclusion criteria included lactose intolerance, recent antibiotic treatment, frequent gastrointestinal disorders, or metabolic diseases. Participants included in the trial had no known allergies or intolerance to dairy foods. The trial had 80% power at a 5% 2-sided significance level to detect a >50% change in the primary outcome among subjects. No antibiotics or laxatives were taken 2 months before or during the study.
Intervention
Sixteen participants showing diversity in lactobacilli count and cholesterol levels were involved in the double-blind, crossover, placebo-controlled, and randomized-feeding trial. The trial was divided into five consecutive periods: a pre-feeding period (2 weeks), followed by a feeding period (4 weeks), a washout period (4 weeks), a second-feeding period (4 weeks), and a final washout period (2 weeks).
Collection and Analysis of the Blood Samples
Blood samples were taken from each volunteer immediately before and after each treatment period using EDTA-containing vacutainers. Total cholesterol (TC) was measured using enzyme-spectrophotometry kits and IgG, IgM, TNF-alpha, INF-gamma, and IL-2 by ELISA capture assay (Siemens Medical Solutions Diagnostics Ltd., India). All the tests were done at the Central Diagnostic Laboratory, Shri Krishna Medical College Karamsad, Anand, Gujarat, an NABL accredited and ISO 15189:2003 laboratory.
Selection of Respondents and Non-Respondents
A respondent was defined as a subject having an improvement in the levels of L. helveticus MTCC 5463 strain count in feces and a reduction in cholesterol levels. Similarly, a non-responder was defined as a subject who displayed an absence of decrease in cholesterol levels and increase in viability of the bacterial strain. Based on these criteria, we identified eight subjects each in respondents and non-respondents category.
Fecal Sample Collection
Single fecal samples were collected at the end of every 2 weeks. Participants were given 60 ml sterile stool container with a sterile plastic spoon (Polylab Plasticware, India) and were asked to fill the tube to the 30 ml mark with feces from the midstream defecation period. During the second-feeding period, there was a crossover of the feeding design. For every collection, the stool samples were immediately frozen at −20°C.
DNA Extraction from Stool Samples
DNA was extracted from feces using a QIAamp MiniPrep DNA extraction kit following the manufacturer’s instructions. The DNA was stored at −20°C. Quality and purity of the isolated genomic DNA were confirmed by agarose gel electrophoresis and spectrophotometry on the NanoDrop 2000 device (Fisher Scientific, Schwerte, Germany). DNA concentration was estimated with the Qubit 2.0 instruments applying the Qubit dsDNA HS Assay (Life Technologies, Invitrogen division, Darmstadt, Germany).
Quantification of Lactobacilli in Stool
The subjects voided their feces into a 60-ml sterile stool container with a sterile plastic spoon (Polylab Plasticware, India) from the midstream defecation period. Within an hour of sample procurement, samples were diluted and homogenized to give a 10-fold dilution (wet weight/volume). Stool Lactobacilli content was determined by plating aliquots (1 ml) of each dilution on freshly prepared de Mann Rogosa Sharp agar (Himedia, India), incubated for 24–48 h at 37°C under anaerobic conditions. Stool L. helveticus MTCC 5463 content was determined by quantitative PCR (qPCR) using StepOne Real-Time PCR System (ABI/Thermo Fisher Scientific, Bangalore, India). Primers and 3′ minor groove binder (MGB) probes for accurate detection and quantification of L. helveticus MTCC 5463 in human fecal samples were developed with Primer Express v3.0 [Thermo Fisher Scientific (earlier Applied Biosystems), Bangalore, India]. The temperature profile of the qPCR consisted 2 min at 50°C, 10 min at 95°C, followed by 45 cycles of 15 s at 95°C, and 1 min at 60°C. Species specific primers and probe targeted on the bile salt hydrolase gene of L. helveticus MTCC 5463 (Accession number AEYL01000315; locus tag AAULH_13111 2049 bp). BLAST1 and EMBL2 database were used to ensure the specificity of the primers. Genomic DNA standards prepared with six different serial dilutions (2.68 × 106, 2.68 × 105, 2.68 × 104, 2.68 × 103, 2.68 × 102, and 2.68 × 10) being equivalent to ranges from 106 to 101 CFU/ml of target genome (MTCC 5463). The cycle threshold (CT) was evaluated to create the standard curve. The amplification efficiencies were determined using the formula E = [10(−1/slope) − 1].
16S Primers and Amplicon Library Generation
PCR amplification of the 16S rRNA hypervariable region V2–V3 was performed with a pool of 32 degenerated forward and 1 degenerated reverse primer targeting bacteria as described by Schmalenberger et al. (2001) with barcode sequences at the 5′ end of the primers. Primers were assessed for specificity using the SILVA 108 SSU Reference 16S rRNA gene database and BLASTN matches with corresponding 16S rRNA gene sequences. The addition of the barcodes to the primers resulted in an amplicon approximately 430 nt in length. The primers were diluted and pooled to equimolar quantities. For amplicon library preparation, 4 ng of each genomic DNA, 5 mM dNTPs, 2 mM MgCl2 (Roche Diagnostics, USA), 1 U Platinum Taq DNA polymerase High Fidelity, and 10 pmol primer-mix were used per 25 μl amplification reaction. The PCR conditions were as follows: 95°C for 5 min, followed by 30 cycles of 94°C for 15 s, 60°C for 45 s, 70°C for 30 s, and a final elongation step of 72°C for 10 min. Amplicon product purification was performed via gel electrophoresis on a 1.5% Tris Borat EDTA agarose gel-stained with ethidium bromide (EtBr) (Life Technologies). All positive PCR reactions were electrophoresized in agarose gels and products with the expected size were cut and purified with Qiagen Gel extraction kit (Qiagen, Düsseldorf, Germany). The exact fragment sizes were determined using HT DNA High Sensitivity LabChip Kit (Caliper Life Sciences GmbH, Mainz, Germany). Amplicon library concentration was estimated with the Qubit 2.0 instrument using the Qubit dsDNA HS assay (Life Technologies).
Emulsion PCR and Sequencing
The emulsion PCR was carried out applying the Ion XPress Template kit V2.0 (Life Technologies) as described in the appropriate user Guide (Part No. 4469004 Rev. B 07/2011) provided by the manufacturer. Quality and quantity of the enriched spheres were checked on the Guava easyCyte5 system (Millipore GmbH, Schwalbach am Taunus, Germany) as described in the appendix of the Ion Xpress Template Kit User Guide (Part Number 4467389 Rev. B, 05/2011). Sequencing of the amplicon libraries was carried out on the Ion Torrent Personal Genome Machine (PGM) system using the Ion Sequencing 200 kit (all Life Technologies) following the corresponding protocol (Part No. 4471999 Rev B, October 13, 2011). Quality check passed libraries were subjected to emulsion PCR using the Ion PGM 200 Xpress Template Kit (Life Technologies). After bead enrichment, beads were loaded onto Ion 316 chips and sequenced using an Ion Torrent PGM.
Sequence Analysis
The sequence data sets obtained were uploaded to the Metagenome Rapid Annotation using Subsystem Technology (MG-RAST) server3 and subsequently checked for low-quality reads. The sequence reads that passed the quality filtering step were then subjected to further analysis of the taxonomic annotation of the fecal DNA sequences using QIIME pipeline (Caporaso et al., 2010). To investigate the species diversity, we used rarefaction curves, and richness estimators like Chao1 in QIIME. Statistic comparison of samples organized into respondents and non-respondents for the deferentially abundant microbial diversity was studied using an alignment platform, STAMP.4
Results
We conducted a comparative analysis of respondents and non-respondents fecal microbiome to reveal differences and identified biomarkers that differentiate them.
Quantification of Lactobacilli Count Using Traditional Plating and qPCR
Traditional plate counts of lactobacilli at genus level on selective medium ranged from a baseline reading of 8.6 log CFU/g of wet fecal matter, which rose to 9.3 log CFU/g at the end of feeding period and a gradual decrease to 8.7 log CFU/g at the end of the placebo feeding. The qPCR primers targeted the bile salt hydrolase gene of MTCC 5463, which made the gene copy count a fraction of the plate count. On the other hand, the precise L. helveticus MTCC 5463 strain count from real-time PCR showed a complete absence of the strain before feeding. At the end of 30 days, the strain appeared in the feces of all subjects in the treated group, reaching a level as high as 8.32 to the lowest amount of 6.17 log gene copies/g fecal matter at end of feeding period.
Summary of Sequence Processing Data
Primers targeting the 16S rRNA gene V2–V3 region (Schmalenberger et al., 2001) precisely generated amplicons from members of the domain bacteria and did not hybridize to sequences of the domains Archaea and Eucarya. By using the ARB SILVA 108 SSU database, a high match of 84.5% at a maximum number of four mismatches was observed. The primer pair theoretically targeted all 16S rRNA gene sequences of the gut microbiota bacterial orders and generated a single amplicon. All 32 amplicons from the pool of test and placebo groups were mixed together at an equimolar ratio. After pooling and elution of the amplicons from the gel, we got one band for the probiotic fed group of 520 bp having a concentration of 1912.09 pg/μl and molarity of 5562.5 pmol/l. The placebo fed group gave an amplicon of 513 bp having a concentration of 1435.87 pg/μl and 4237.7 pmol/l molarity. The data sets for 16 subjects before probiotic feeding had reads ranging from 13,061 to 980,628 with a read length ranging from 201 to 251 bp and a total amount of 42,52,62,470 bases.
Inter-Individual Differences in Shifts in Phyla Abundance (%) before and after Probiotic Feeding
The relative abundance of major genera in the elderly gut metagenome and high-level of inter-individual variation is shown in Figure 1. We presume that the inter-individual differences are indicative of a highly personal fecal microbiota profile, which determines the response of the host to probiotics. Host factors probably play a major effect in shaping the intestinal microbial ecosystem during an intervention. In the present study, we attempted to understand the core microbiome of respondents and non-respondents to probiotics.
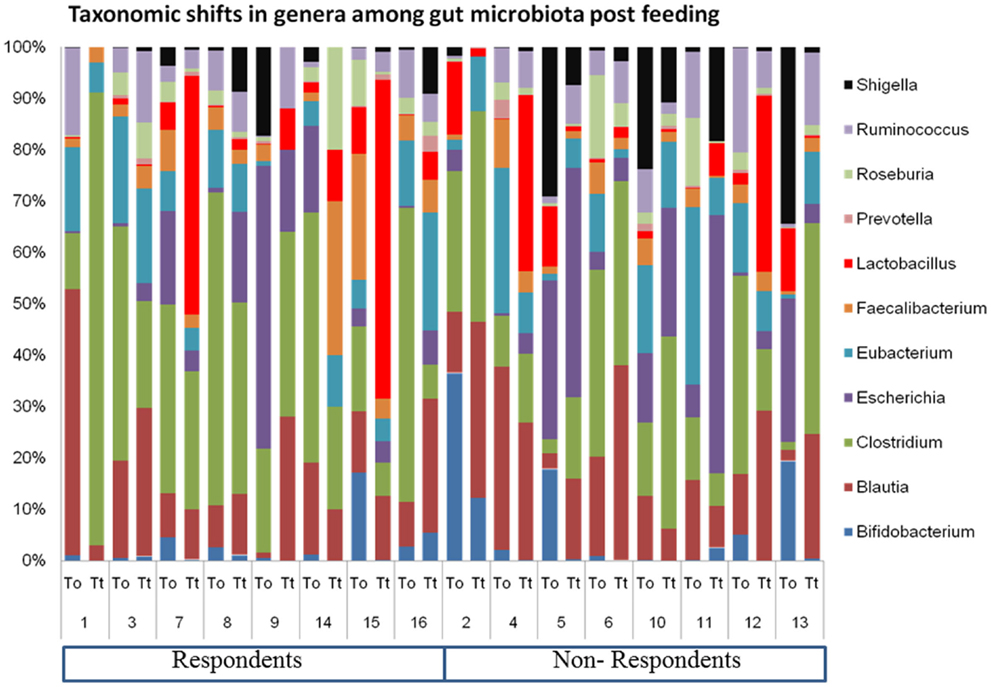
Figure 1. Inter-individual variation in the geriatric gut microbiome pre- and post-probiotic feeding.
Probiotic Feeding and Effect on Lipid Profile and Immunologic Parameters
In addition to TC (primary outcome), in this study, we also investigated the effect of probiotic intervention on lipid profile, beta glucouronidase activity, and immunological parameters. The mean β-glucuronidase activity was reduced in test group from 1.40 to 0.73 (microgram/min/mg of protein), while in case of placebo group, no effect on enzyme activity was observed. A significant immunomodulatory effect on the TNF-α and IL-2 levels in subjects among probiotic group compared to placebo group was observed. There was however no significant beneficiary effect found on IFN-γ, IgG, or IgM levels. Paired t-test showed that there were statistically significant differences in serum cholesterol, VLDL, TC/HDL, LDL/HDL in placebo group and in LDL, TC/HDL, and LDL/HDL in probiotic group. A significant (p = 0.01) decrease in the LDL value was seen in the probiotic group at the end of 30 days of feeding (Table 1).
Participant Diversification into Respondents and Non-Respondents
The primary outcome of this trial was a reduction in TC after 4 weeks of feeding probiotic MTCC 5463. We defined non-respondents as those subjects who experienced elevations in TC of ≥2.509 mg/dL, whereas respondents were the ones who showed no change in TC or <1.72 mg/dL TC in response to the probiotic intervention of 4 weeks. Among the 59 subjects who could complete the study, we classified a total of 16 subjects into respondents (n = 8) and non-respondents (n = 8) based on cholesterol levels and lactobacilli counts (Figure 2). There were no significant differences in the baseline characteristics of the two groups. This eliminates the influence of gender, weight, and age in influencing the response toward probiotic intervention. The abundance of L. helveticus MTCC 5463 was significant (p < 0.05) higher in the individuals with an increase in cholesterol levels, as compared to those with a decrease. The decrease in cholesterol levels among respondents was a maximum 14.19% with a 23.66% increase in lactobacilli count in feces. Among non-respondents, a maximum increase of 34.13% in cholesterol with a 9.31% decrease in lactobacilli count was observed. The increase in lactobacilli counts with a decrease in cholesterol in case of respondents indicated that the observed hypocholesterolemic effect of the strain was dependent on the number of lactobacilli in the gut.
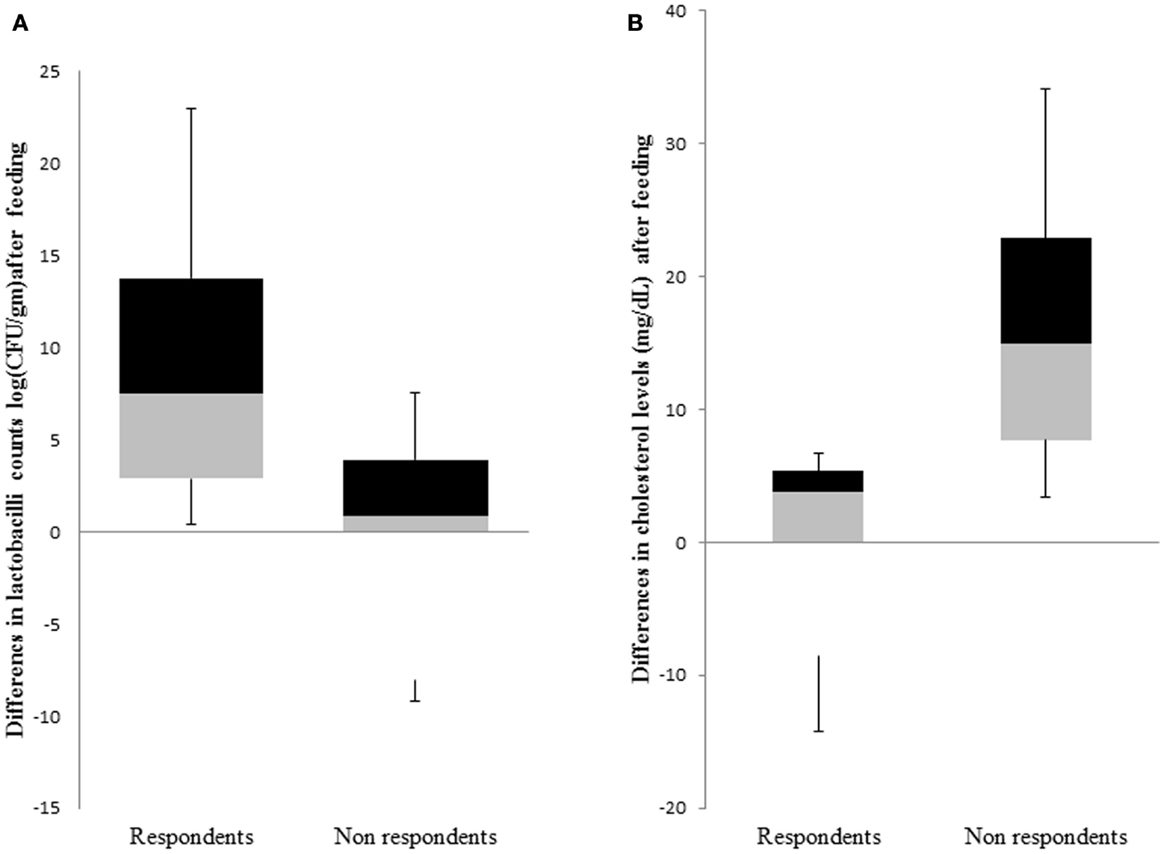
Figure 2. Comparison of response groups with respect to (A) lactobacilli counts and (B) cholesterol levels. The middle line represents the median, the box represents the interquartile range, and the whiskers represent the total range.
Microbiome Diversity Estimates Associated with Respondents and Non-Respondents
The alpha diversity of the respondent group before probiotic feeding (30.8 ± 4.8) and after feeding (26.6 ± 3.6) was higher than non-respondents’ measures for before (25.6 ± 4.5) and after probiotic feeding (24.6 ± 5.8). This indicates that bacterial richness is a factor that promotes responsiveness toward beneficial strains in the gut. To investigate differences in rarefaction measures, we rarified each sample at 33,000 reads and performed the two-sample t-test on the two groups. Respondents had significantly greater alpha diversity indices like phylogenetic distance (p = 0.022), Chao1 (p = 0.019), and Shannon index (p = 0.00058) than the non-respondents (Figure 3). A non-significant increase in observed species in case of non-respondents (p = 0.27) could be due to presence of distinct commensals that reflect the host’s dietary and geographical differences. There was a non-significant abundance of Clostridium, Shigella, and Listeria among rural respondents. Poor sanitation and hygiene maintained in the rural households could have led to the distinct differences in gut microbiota.
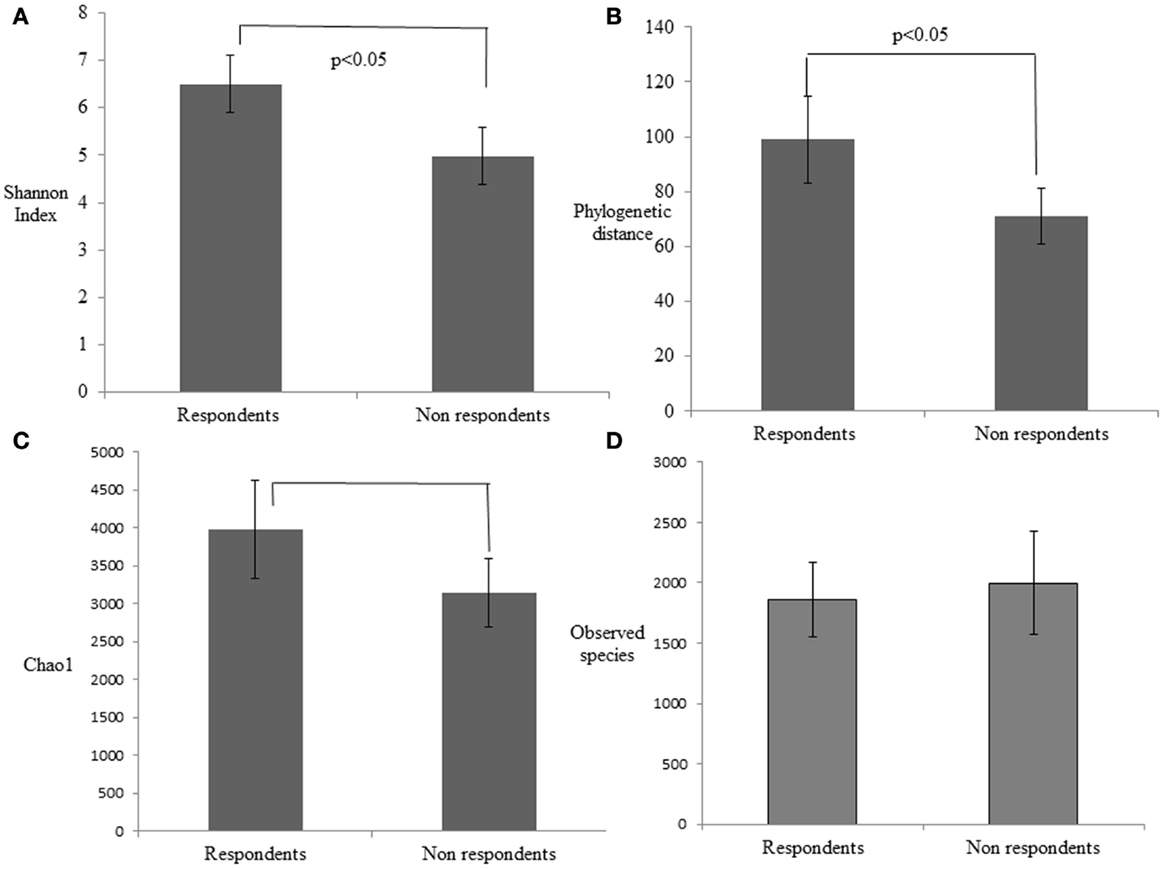
Figure 3. Estimation of the phylogenetic diversity of the gut microbiota in the respondent and non-respondent groups using the (A) Shannon index, (B) phylogenetic distance, (C) Chao1, and (D) observed species. The values are means, and error bars indicate the 95% confidence intervals.
Bacterial Taxa Populations Associated with Respondents and Non-Respondents
We performed a comparison of the microbiota between respondents and non-respondents to find specialized bacterial members within the abundant phyla Firmicutes and Proteobacteria. Respondents carried a lower proportion of Clostridium and a higher proportion of Eubacterium compared to the non-respondents (Figure 4). Surprisingly, although the non-respondents had a higher proportion of gut lactobacilli (31%) compared to 28% in respondents, a favorable reduction in cholesterol corresponding to the increase in strain MTCC 5463 was not observed. This could be due to competitive exclusion by a higher proportion of Clostridium (24%) in the gut on non-respondents compared to respondents (6%). The presence of Listeria in the non-respondents further emphasizes the need to investigate the association of gut microbiota, especially pathobionts with probiotic strain. Comparing the abundance in the genera of Proteobacteria group, it can be observed (Figure 5) that respondents carried a higher amount of Burkholderia (63%) and a lower amount of Shigella (7%) compared to non-respondents, who harbored lower count of Burkholderia (36%) and a higher amount of Shigella (31%), which must have affected the colonization of the probiotic strain. Non-respondents carried a higher amount of Escherichia and Brucella in the gut. Shigella seemed to have a symbiont asymptomatic existence in the host, showing no discomfort to the subjects. The higher amount of Escherichia and Camphylobacter could be the deciding biomarkers of non-responsiveness toward probiotic intervention.
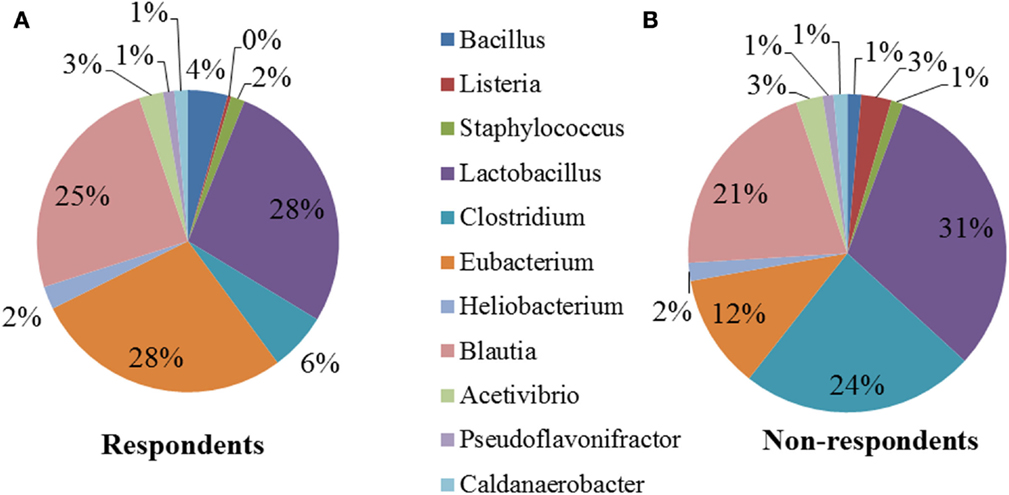
Figure 4. Relative abundances of the dominant genera (Firmicutes) in (A) respondents and (B) non-respondents.
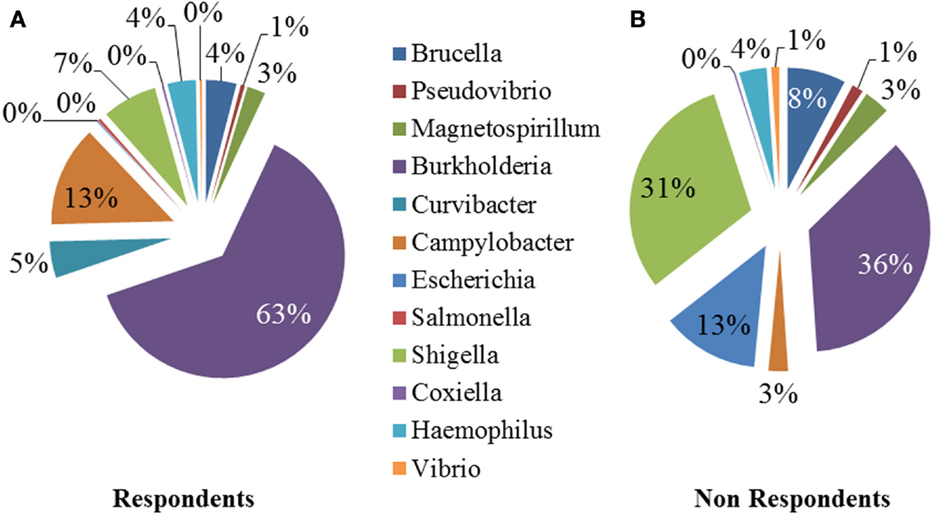
Figure 5. Relative abundances of the dominant genera (Proteobacteria) in (A) respondents and (B) non-respondents.
Statistical Analysis of Metagenomic Data
A remarkable significant difference among the chief genera of Proteobacteria including Shigella, Escherichia, Burkholderia, and Camphylobacter (q < 0.002) was observed. The chief genera of Firmicutes that showed remarkable significant difference were Lactobacillus, Clostridium, Eubacterium, and Blautia (q < 0.002) (Figure 6). Although non-respondents carried a higher proportion of Lactobacilli, a favorable physiological function may not be translated to the host possibly due to an increase in Clostidum, Shigella, and Eschericihia with a decrease in Blautia and Burkholderia. We would like to add that the results of population wide samples taken at one time point for a study might not be able to display the entire variation that exists in that population over time and place.
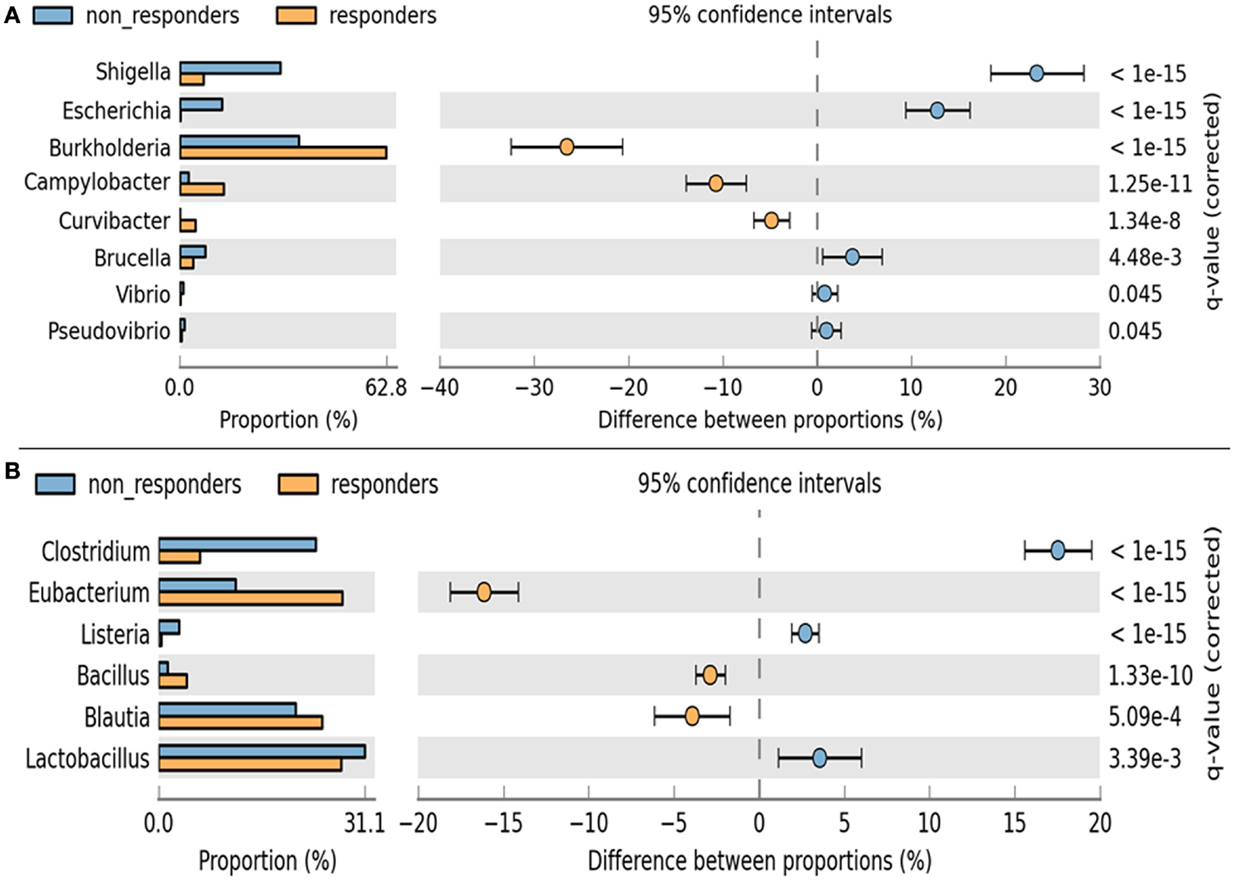
Figure 6. Analysis of significance in abundance of (A) Firmicutes and (B) Proteobacteria among respondents and non-respondents using two-sample Fischer’s exact test.
Discussion
A primary beneficial effect of consuming a bile-salt-hydrolyzing L. helveticus MTCC 5463 strain is a reduction in serum cholesterol levels. In the clinical trials carried out to prove the hypocholesterolemic effect of the strain (Ashar and Prajapati, 2001; Prajapati et al., 2012) we could observe participants responding differently to the same treatment. Similar cases of inter-individual variability in response to probiotics (van Baarlen et al., 2011; Grzeskowiak et al., 2012; Arboleya et al., 2013) have been published. Previously, subjects had been classified as respondents and non-respondents based on a greater than or less than 10% change in cholesterol (Cox et al., 2014) but this classification was criticized as being impractical (Ding and Schloss, 2014). Classification using a fecal biomarker (Coen et al., 2009) or biomarkers of the host’s basic metabolism (Naruszewicz et al., 2002; Herron et al., 2003; Ibrahim et al., 2010) has been suggested. We conducted a comparative analysis of fecal microbiomes of respondents and non-respondents to identify bacterial biomarkers. Results of such microbial profiling may serve as a clinically useful biomarker in geriatric care (Kostic et al., 2013).
Primer usage is one of the most critical factors affecting 16S rDNA analysis (Armougom and Raoult, 2009). There exists a possibility that amplification efficacy of the primers could have led to the underestimation of bacterial richness, in this study. We chose the Ion Torrent PGM platform due to its inherent low-cost per sequencing run that allowed us to perform the next-generation sequencing on site at the Veterinary faculty at AAU.
There were no significant differences in the age or weight of respondents and non-respondents, though respondents had a tendency to be older. An effect size of 0.8 among the groups further eliminated the influence of gender, weight, and age toward the expected response. Grouping of similar sequences as operational taxonomic units (OTUs) and quantifying the number of OTUs gave an approximation of species diversity in a sample (Sun et al., 2009; Schloss et al., 2014). Species diversity has been reported as a characteristic feature determining state of health or disease. Reduced colonic microbial diversity of dysbiosis is reported in Crohn disease (Ott et al., 2004), ulcerative colitis (McLaughlin et al., 2010), antibiotic-associated diarrhea (Chang et al., 2008), and Clostridium difficile infection (Seto et al., 2014). A higher alpha diversity of gut bacteria among respondents compared to non-respondents seemed to support microbial integrity. Bacterial richness and evenness play an integral role in the success of a probiotic therapy as earlier observed in colitis (Kennedy et al., 2000).
A higher Chao’s estimator and the Shannon index among respondents indicate higher saturation and unevenness in taxa abundance within samples. A non-significant increase in observed species in case of non-respondents suggested the presence of species that may have prevented the probiotic to establish and exert the functionality. We could identify distinct microbial diversity in rural subjects compared to the urban dwellers especially in the presence of Clostridium, Shigella, and Listeria, similar to Russian rural communities (Tyakht et al., 2013). Gram-negative bacteria were more abundant than Gram-positive bacteria in the rural population. Shigella and Escherichia were significantly under-represented in rural African children than urban European children (De Filippo et al., 2010). In the present study, we observed Shigella and Escherichia higher in geriatric rural dwellers, which reflected the age and geography-induced diversities in human gut microbiome.
Phyla associated with a healthy state in Indian geriatrics as suggested in this study are Firmicutes accounting for at least 50%, followed by Actinobacteria (20%) and Proteobacteria (10%). At the phylum level, the majority of the intestinal bacteria are known to belong to Bacteroidetes and Firmicutes (Eckburg et al., 2005). Surprisingly, none of the sequences from the present study were assigned to the Bacteroidetes. An under representation of Bacteroidetes could be due to inter-subject variability (Ley et al., 2006), variation due to adiposity (Frank et al., 2007; Wu et al., 2011), or suppression due to inflammatory bowel disease (Lazarevic et al., 2009). We could not ignore the possibility of loss of this phylum in fecal samples when stored for longer periods and MG-RAST-based classifications’ sensitivity for Proteobacteria (Korpela et al., 2014). Antibiotic usage in elderly can again cause a decline in commensal anaerobes like Bacteroides, Lactobacillus, and Bifidobacterium (Macfarlane, 2014).
Responses of the host to individual bacterial strains are influenced by the baseline composition of the gut microbiota (Rajilić-Stojanović et al., 2015). The respondents showed a lower percentage of Firmicutes and non-respondents showed a comparative lower amount of Proteobacteria. Among Firmicutes, Clostridia were higher in case of non-respondents (24% compared to 6% in respondents). Although the volunteers were not consuming antibiotics during the trial, prior usage of antibiotics could have diminished the population of total and commensal bacteria (Biagi et al., 2010) leading to an overgrowth of Clostridium (Round and Mazmanian, 2009) in non-respondents. Antibiotics are readily available over the counter at pharmacies in India and inconsistent hospital standards toward antibiotic usage could have led to higher proportion of Clostridia in the gut. Respondents carried a higher proportion of Eubacterium (28% compared to 12% in non-respondents), which reflected a healthy state. An increased diversity of Eubacteria has been observed in the elderly (Hopkins and Macfarlane, 2002). Decrease in Eubacterium lead to decreased levels of SCFA, facilitating easier entry of Enterobacteriaceae into the intestinal mucosa due to an impaired secretion of mucins by the intestinal epithelial cells (Garrett et al., 2010). The outgrowth of anaerobic Enterobacteriaceae must have led to a competitive exclusion of aerotolerant MTCC 55463 strains in the host intestine.
Proteobacteria, recently defined as “pathobionts” (Morgan et al., 2004) are considered to be minor and opportunistic components of the human gut ecosystem. The majority of the sequences assigned to the Proteobacteria were Burkholderiales. A higher proportion of Burkholderia is a signature of good health as earlier observed in the healthy Indian child data set (Schultz et al., 2004). Non-respondents had a higher proportion of Proteobacteria, especially Escherichia/Shigella (indistinguishable as a 16S-based phylotype), previously implicated in intestinal inflammation (Guslandi et al., 2004). Although the volunteers were seemingly healthy with no complaints of gastrointestinal disturbances, a core structural and functional dysbiosis caused by an overgrowth of Escherichia/Shigella (Malchow, 1997) could have led to a lack of translation of functionality of MTCC 5463 to the host in spite of being present at a higher proportion in the non-respondents gut.
In this study, it was shown that consumption of probiotic yogurt did not significantly reduce TC levels, the intervention significantly reduced serum levels of LDL, TC/HDL ratio, and LDL/HDL ratio in geriatric volunteers. Many studies in literature support the beneficiary effect of probiotics on lipid profiles of subjects. There also exist some contradictory reports where eating probiotic yogurt did not change lipid profiles (Hatakka et al., 2008; Sadrzadeh-Yeganeh et al., 2010). This indicates that apart from the probiotic strain, the host gut microbiome has a big role to play on the response of the host to the probiotic strain.
STAMP analysis revealed proportions of distinct microbial biomarkers like Shigella, Escherichia, Burkholderia, Camphylobacter, Lactobacillus, Clostridium, Eubacterium, and Blautia that can help tailor a probiotic therapy to a niche population. The authors would like to strike an analogy to feeding probiotics to a host with imbalanced consortia in the gut to the likes of pouring water to a filled pitcher. Like the water flows out, the probiotic strains are lost in the feces and fail to colonize and translate the functionality to the host. Metatranscriptomic studies could furnish further information for comprehending the molecular basis of responsiveness toward a probiotic therapy because gene expression profiles are more individualized than DNA-level profiles and less variable than microbial composition. Geriatric care is critical in the aging global population and the role of gut metagenomics cannot be overstated in understanding its role in health and disease for the future development of personalized nutrition.
Conclusion
Globally today, the elderly populations are looking for natural means of sustaining digestive health. Compared to the growing awareness and market penetration of probiotics, there is a dearth of scientific evidence on how probiotics affect the composition of gut microbiota. The well-documented probiotic L. helveticus MTCC 5463 was administered to geriatrics in a clinical trial, and a deep sequencing technology was employed to study the changes in the resident microbes over the duration of probiotic consumption. We could find that chiefly Shigella, Escherichia, Burkholderia, Camphylobacter, Lactobacillus, Clostridium, Eubacterium, and Blautia define the response of the host to the probiotic strain. Moreover, we observed a shift in the gut profile of the non-respondents towards a respondent’s signature gut profile after consuming the probiotic, which proves the importance of precise personalized selection of dosage for an effective tailored probiotic therapy.
Ethics
Approval for the study design was obtained from the Institutional Ethics Committee (IEC) of Shri Krishna Medical College Karamsad, Anand, Gujarat (HMPCMCE:HREC/FCT/41/01) and Anand Agricultural University (AAU), Anand (AAU/DR/RES/DM/IEC/659/2011). The trial was registered at ICMR Clinical Trial Registry (REF/2012/10/004135).
Author Contributions
Conceived and designed the experiments: JP, CJ, and HP; performed metagenomic analysis and manuscript writing: SS; product development: SV; clinical recruitment of participants: MG and US; clinical investigations and data interpretation: ST and RP; literature search and critical review of the manuscript: HAP; statistical data analysis: AP.
Conflict of Interest Statement
The authors declare that the research was conducted in the absence of any commercial or financial relationships that could be construed as a potential conflict of interest.
Acknowledgments
We are indebted to the technical staff of Ome Reserch Facility, Department of Animal Biotechnology, AAU, India whose names were not included in the author list, but who contributed to sequencing and data analysis of this work. The authors kindly acknowledge the funding support received from DBT, India, through Project BT/PR-14950/FNS/20/490/2010. Partial results of the study were presented in the Symposium on “Gut Microbiota, Probiotics and Their Impact throughout the Lifespan,” conducted at Harvard Medical School, Boston, MA, USA. September 22–23, 2014.
Abbreviations
μl, microliter; AAU, Anand Agricultural University; CFU, colony forming unit; dL, deciliter; dNTP, deoxynucleotide; ICMR, Indian Council of Medical Research; ISAPP, International Scientific Association for Probiotics and Prebiotics; mg, milligram; ml, milliliter; mM, millimolar; MTCC, microbial type culture collection; nt, nucleotide; PGM, Personal Genome Machine; pmol, picomol; QIIME, Quantitative Insights into Microbial Ecology; rRNA, ribosomal RNA; s, seconds; S, Svedberg unit; U, unit.
Footnotes
References
Ahmed, S., Macfarlane, G. T., Fite, A., McBain, A. J., Gilbert, P., Macfarlane, S., et al. (2007). Mucosa-associated bacterial diversity in relation to human terminal ileum and colonic biopsy samples. Appl. Environ. Microbiol. 73, 7435–7442. doi: 10.1128/AEM.01143-07
An, H. M., Baek, E. H., Jang, S., Lee, D. K., Kim, M. J., Kim, J. R., et al. (2010). Efficacy of lactic acid bacteria (LAB) supplement in management of constipation among nursing home residents. Nutr. J. 9, 5. doi:10.1186/1475-2891-9-5
Arboleya, S., Salazar, N., Solís, G., Fernández, N., Gueimonde, M., and de los Reyes-Gavilán, C. G. (2013). In vitro evaluation of the impact of human background microbiota on the response to Bifidobacterium strains and fructo-oligosaccharides. Br. J. Nutr. 110, 2030–2036. doi:10.1017/S0007114513001487
Armougom, F., and Raoult, D. (2009). Exploring microbial diversity using 16S rRNA high-throughput methods. J. Comput. Sci. Syst. Biol. 2, 74–92. doi:10.4172/jcsb.1000019
Ashar, M. N., and Prajapati, J. B. (2001). Serum cholesterol level in humans fed acidophilus milk. Indian J. Microbiol. 41, 257–263.
Bartosch, S., Fite, A., Macfarlane, G. T., and McMurdo, M. E. (2004). Characterization of bacterial communities in feces from healthy elderly volunteers and hospitalized elderly patients by using real-time PCR and effects of antibiotic treatment on the fecal microbiota. Appl. Environ. Microbiol. 70, 3575–3581. doi:10.1128/AEM.70.6.3575-3581.2004
Biagi, E., Nylund, L., Candela, M., Ostan, R., Bucci, L., Pini, E., et al. (2010). Through ageing, and beyond: gut microbiota and inflammatory status in seniors and centenarians. PLoS ONE 5:e10667. doi:10.1371/journal.pone.0010667
Caporaso, J. G., Kuczynski, J., Stombaugh, J., Bittinger, K., Bushman, F. D., Costello, E. K., et al. (2010). QIIME allows analysis of high-throughput community sequencing data. Nat. Methods 7, 335–336. doi:10.1038/nmeth.f.303
Chang, J. Y., Antonopoulos, D. A., Kalra, A., Tonelli, A., Khalife, W. T., Schmidt, T. M., et al. (2008). Decreased diversity of the fecal Microbiome in recurrent Clostridium difficile-associated diarrhea. J. Infect. Dis. 197, 435–438. doi:10.1086/525047
Claesson, M. J., Cusack, S., O’Sullivan, O., Greene-Diniz, R., de Weerd, H., Flannery, E., et al. (2011). Composition, variability and temporal stability of the intestinal microbiota of the elderly. Proc. Natl Acad. Sci. U.S.A. 108, 4586–4591. doi:10.1073/pnas.1000097107
Coen, M., Want, E. J., Clayton, T. A., Rhode, C. M., Hong, Y. S., Keun, H. C., et al. (2009). Mechanistic aspects and novel biomarkers of responder and non-responder phenotypes in galactosamine-induced hepatitis. J. Proteome Res. 8, 5175–5187. doi:10.1021/pr9005266
Cox, L. M., Yamanishi, S., Sohn, J., Alekseyenko, A. V., Leung, J. M., Cho, I., et al. (2014). Altering the intestinal microbiota during a critical developmental window has lasting metabolic consequences. Cell 158, 705–721. doi:10.1016/j.cell.2014.05.052
De Filippo, C., Cavalieri, D., Di Paola, M., Ramazzotti, M., Poullet, J. B., Massart, S., et al. (2010). Impact of diet in shaping gut microbiota revealed by a comparative study in children from Europe and rural Africa. Proc. Natl Acad. Sci. U.S.A. 107, 14691–14696. doi:10.1073/pnas.1005963107
de Wouters, T., Doré, J., and Lepage, P. (2000). Does our food (environment) change our gut microbiome (‘In-Vironment’): a potential role for inflammatory bowel disease. Dig. Dis. 30, 33–39. doi:10.1159/000342595
Ding, T., and Schloss, P. D. (2014). Dynamics and associations of microbial community types across the human body. Nature 509, 357–360. doi:10.1038/nature13178
Eckburg, P. B., Bik, E. M., Bernstein, C. N., Purdom, E., Dethlefsen, L., Sargent, M., et al. (2005). Diversity of the human intestinal microbial flora. Science 308, 1635–1638. doi:10.1126/science.1110591
Frank, D. N., St Amand, A. L., Feldman, R. A., Boedeker, E. C., Harpaz, N., and Pace, N. R. (2007). Molecular-phylogenetic characterization of microbial community imbalances in human inflammatory bowel diseases. Proc. Natl Acad. Sci. U.S.A. 104, 13780–13785. doi:10.1073/pnas.0706625104
Garrett, W. S., Gallini, C. A., Yatsunenko, T., Michaud, M., DuBois, A., Delaney, M. L., et al. (2010). Enterobacteriaceae act in concert with the gut microbiota to induce spontaneous and maternally transmitted colitis. Cell Host Microbe 8, 292–300. doi:10.1016/j.chom.2010.08.004
Gerritsen, J., Smidt, H., Rijkers, G. T., and de Vos, W. M. (2011). Intestinal microbiota in human health and disease: the impact of probiotics. Genes Nutr. 6, 209–240. doi:10.1007/s12263-011-0229-7
Ghosh, T. S., Sen Gupta, S., Bhattacharya, T., Yadav, D., Barik, A., Chowdhury, A., et al. (2014). Gut microbiomes of Indian children of varying nutritional status. PLoS ONE 9:e95547. doi:10.1371/journal.pone.0095547
Grzeskowiak, L., Collado, M. C., Mangani, C., Maleta, K., Laitinen, K., Ashorn, P., et al. (2012). Distinct gut microbiota in southeastern African and northern European infants. J. Pediatr. Gastroenterol. Nutr. 54, 812–816. doi:10.1097/MPG.0b013e318249039c
Guigoz, Y., Doré, J., and Schiffrin, E. J. (2008). The inflammatory status of old age can be nurtured from the intestinal environment. Curr. Opin. Clin. Nutr. Metab. Care 11, 13–20. doi:10.1097/MCO.0b013e3282f2bfdf
Gupta, S. S., Mohammed, M. H., Ghosh, T. S., Kanungo, S., Nair, G. B., and Mande, S. S. (2011). Metagenome of the gut of a malnourished child. Gut Pathog. 3, 7. doi:10.1186/1757-4749-3-7
Guslandi, M., Mezzi, G., Sorghi, M., and Testoni, P. A. (2004). Saccharomyces boulardii in maintenance treatment of Crohn’s disease. Dig. Dis. Sci. 45, 1462–1466. doi:10.1023/A:1005588911207
Hatakka, K., Mutanen, M., Holma, R., Saxelin, M., and Korpela, R. (2008). Lactobacillus rhamnosus LC705 together with Propionibacterium freudenreichii ssp shermanii JS administered in capsules is ineffective in lowering serum lipids. J. Am. Coll. Nutr. 27, 441–447. doi:10.1080/07315724.2008.10719723
Herron, K. L., Vega-Lopez, S., Conde, K., Ramjiganesh, T., Shachter, N. S., and Fernandez, M. L. (2003). Men classified as hypo- or hyper respondents to dietary cholesterol feeding exhibit differences in lipoprotein metabolism. J. Nutr. 133, 1036–1042.
Hooper, L. V., Wong, M. H., Thelin, A., Hansson, L., Falk, P. G., and Gordon, J. I. (2001). Molecular analysis of commensal host-microbial relationships in the intestine. Science 291, 881–884. doi:10.1126/science.291.5505.881
Hopkins, M. J., and Macfarlane, G. T. (2002). Changes in predominant bacterial populations in human faeces with age and with Clostridium difficile infection. J. Med. Microbiol. 51, 448–454. doi:10.1099/0022-1317-51-5-448
Hopkins, M. J., Sharp, R., and Macfarlane, G. T. (2001). Age and disease related changes in intestinal bacterial populations assessed by cell culture, 16S rRNA abundance and community cellular fatty acid profiles. Gut 48, 198–205. doi:10.1136/gut.48.2.198
Ibrahim, F., Ruvio, S., Granlund, L., Salminen, S., Viitanen, M., and Ouwehand, A. C. (2010). Probiotics and immunosenescence: cheese as a carrier. FEMS Immunol. Med. Microbiol. 59, 53–59. doi:10.1111/j.1574-695X.2010.00658.x
Kennedy, R. J., Hoper, M., Deodhar, K., Kirk, S. J., and Gardiner, K. R. (2000). Probiotic therapy fails to improve gut permeability in a hapten model of colitis. Scand. J. Gastroenterol. 35, 1266–1271. doi:10.1080/003655200453601
Korpela, K., Flint, H. J., Johnstone, A. M., Lappi, J., Poutanen, K., Dewulf, E., et al. (2014). Gut microbiota signatures predict host and microbiota responses to dietary interventions in obese Individuals. PLoS ONE 9:e90702. doi:10.1371/journal.pone.0090702
Kostic, A. D., Chun, E., Meyerson, M., and Garrett, W. S. (2013). Microbes and inflammation in colorectal cancer. Cancer Immunol. Res. 3, 150–157. doi:10.1158/2326-6066.CIR-13-0101
Lahtinen, S. J., Tammela, L., Korpela, J., Parhiala, R., Ahokoski, H., Mykkänen, H., et al. (2009). Probiotics modulate the Bifidobacterium microbiota of elderly nursing home residents. Age (Dordr) 31, 59–66. doi:10.1007/s11357-008-9081-0
Lampe, J. W., Navarro, S. L., Hullar, M. A. J., and Shojaie, A. (2013). Inter-individual differences in response to dietary intervention: integrating omics platforms towards personalised dietary recommendations. Proc. Nutr. Soc. 72, 207–218. doi:10.1017/S0029665113000025
Lazarevic, V., Whiteson, K., Huse, S., Hernandez, D., Farinelli, L., Osterås, M., et al. (2009). Metagenomic study of the oral microbiota by Illumina high-throughput sequencing. J. Microbiol. Methods 79, 266–271. doi:10.1016/j.mimet.2009.09.012
Ley, R. E., Turnbaugh, P. J., Klein, S., and Gordon, J. I. (2006). Microbial ecology: human gut microbes associated with obesity. Nature 444, 1022–1023. doi:10.1038/4441022a
Louis, P. (2012). Dietary modulation of the human gut microbiota. Agro Food Industry Hi-Tech 23, 26–28.
Macfarlane, S. (2014). Antibiotic treatments and microbes in the gut. Environ. Microbiol. 16, 919–924. doi:10.1111/1462-2920.12399
Malchow, H. A. (1997). Crohn’s disease and Escherichia coli. A new approach in therapy to maintain remission of colonic Crohn’s disease. J. Clin. Gastroenterol. 25, 653–658. doi:10.1097/00004836-199712000-00021
Mariat, D., Firmesse, O., Levenez, F., Guimaraes, V., Sokol, H., Doré, J., et al. (2009). The Firmicutes/Bacteroidetes ratio of the human microbiota changes with age. BMC Microbiol. 9:123. doi:10.1186/1471-2180-9-123
Matsumoto, M., Sakamoto, M., and Benno, Y. (2009). Dynamics of fecal microbiota in hospitalized elderly fed probiotic LKM512 yogurt. Microbiol. Immunol. 53, 421–432. doi:10.1111/j.1348-0421.2009.00140.x
McLaughlin, S. D., Clark, S. K., Tekkis, P. P., Nicholls, R. J., and Ciclitira, P. J. (2010). The bacterial pathogenesis and treatment of pouchitis. Therap. Adv. Gastroenterol. 3, 335–348. doi:10.1177/1756283X10370611
McOrist, A. L., Miller, R. B., Bird, A. R., Keogh, J. B., Noakes, M., Topping, D. L., et al. (2011). Fecal butyrate levels vary widely among individuals but are usually increased by a diet high in resistant starch. J. Nutr. 141, 883–889. doi:10.3945/jn.110.128504
Morgan, X. C., Tickle, T. L., Sokol, H., Gevers, D., Devaney, K. L., Ward, D. V., et al. (2004). Dysfunction of the intestinal microbiome in inflammatory bowel disease and treatment. Genome Biol. 13, R79. doi:10.1186/gb-2012-13-9-r79
Mueller, S., Saunier, K., Hanisch, C., Norin, E., Alm, L., Midtvedt, T., et al. (2006). Differences in fecal microbiota in different European study populations in relation to age, gender, and country: a cross-sectional study. Appl. Environ. Microbiol. 72, 1027–1033. doi:10.1128/AEM.72.2.1027-1033.2006
Naruszewicz, M., Johansson, M. L., Zapolska-Downar, D., and Bukowska, H. (2002). Effect of Lactobacillus plantarum 299v on cardiovascular disease risk factors in smokers. Am. J. Clin. Nutr. 76, 1249–1255.
Ott, S. J., Musfeldt, M., Wenderoth, D. F., Hampe, J., Brant, O., Fölsch, U. R., et al. (2004). Reduction in diversity of the colonic mucosa associated bacterial microflora in patients with active inflammatory bowel disease. Gut 53, 685–693. doi:10.1136/gut.2003.025403
Ouwehand, A. C., Tiihonen, K., Saarinen, M., Putaala, H., and Rautonen, N. (2009). Influence of a combination of Lactobacillus acidophilus NCFM and lactitol on healthy elderly: intestinal and immune parameters. Br. J. Nutr. 101, 367–375. doi:10.1017/S0007114508003097
Patil, D. P., Dhotre, D. P., Chavan, S. G., Sultan, A., Jain, D. S., Lanjekar, V. B., et al. (2012). Molecular analysis of gut microbiota in obesity among Indian individuals. J. Biosci. 37, 647–657. doi:10.1007/s12038-012-9244-0
Pitkala, K. H., Strandberg, T. E., FinneSoveri, U. H., Ouwehand, A. C., Poussa, T., and Salminen, S. (2007). Fermented cereal with specific bifidobacteria normalizes bowel movements in elderly nursing home residents. A randomized controlled trial. J. Nutr. Health Aging 2007, 305–311.
Prajapati, J. B., Khedkar, C. D., Chitra, J., Senan, S., Mishra, V., Sreeja, V., et al. (2011). Whole genome shotgun sequencing of an Indian-origin Lactobacillus helveticus strain MTCC 5463 with probiotic potential. J. Bacteriol. 193, 4282–4283. doi:10.1128/JB.05449-11
Prajapati, J. B., Nathani, N., Patel, A. K., Senan, S., and Joshi, C. G. (2013). Genomic analysis of dairy starter culture Streptococcus thermophilus MTCC 5461. J. Microbiol. Biotechnol. 23, 459–466. doi:10.4014/jmb.1210.10030
Prajapati, J. B., Senan, S., Momin, J. K., Damor, R., and Kamalia, K. B. (2012). A randomised double blind placebo controlled trial of potential probiotic strain Lactobacillus helveticus MTCC 5463: assessment of its safety, tolerance and influence on intestinal wellbeing and humoral immune response in healthy human volunteers. Int. J. Health Pharm. Sci. 1, 92–99.
Rajilić-Stojanović, M., Jonkers, D. M., Salonen, A., Hanevik, K., Raes, J., Jalanka, J., et al. (2015). Intestinal microbiota and diet in IBS: causes, consequences, or epiphenomena. Am. J. Gastroenterol. 110, 278–287. doi:10.1038/ajg.2014.427
Reid, G., Gaudier, E., Guarner, F., Huffnagle, G. B., Macklaim, J. M., Munoz, A. M., et al. (2010). Respondents and non-respondents to probiotic interventions: how can we improve the odds? Gut Microbes 1, 200–204. doi:10.4161/gmic.1.3.12013
Round, J. L., and Mazmanian, S. K. (2009). The gut microbiota shapes intestinal immune responses during health and disease. Nat. Rev. Immunol. 9, 313–323. doi:10.1038/nri2515
Sadrzadeh-Yeganeh, H., Elmadfa, I., Djazayery, A., Jalali, M., Heshmat, R., and Chamary, M. (2010). The effects of probiotic and conventional yoghurt on lipid profile in women. Br. J. Nutr. 103, 1778–1783. doi:10.1017/S0007114509993801
Schloss, P. D., Iverson, K. D., Petrosino, J. F., and Schloss, S. J. (2014). The dynamics of a family’s gut microbiota reveal variations on a theme. Microbiome 2, 25. doi:10.1186/2049-2618-2-25
Schmalenberger, A., Schwieger, F., and Tebbe, C. C. (2001). Effect of primers hybridizing to different evolutionarily conserved regions of the small-subunit rRNA gene in PCR-based microbial community analyses and genetic profiling. Appl. Environ. Microbiol. 67, 3557–3563. doi:10.1128/AEM.67.8.3557-3563.2001
Schultz, M., Timmer, A., Herfarth, H. H., Sartor, R. B., Vanderhoof, J. A., and Rath, H. C. (2004). Lactobacillus GG in inducing and maintaining remission of Crohn’s disease. BMC Gastroenterol. 4:5. doi:10.1186/1471-230X-4-5
Senan, S., Prajapati, J. B., and Joshi, C. G. (2015). Whole-genome based validation of the adaptive properties of Indian origin probiotic Lactobacillus helveticus MTCC 5463. J. Sci. Food Agric. 95, 321–328. doi:10.1002/jsfa.6721
Seto, C. T., Jeraldo, P., Orenstein, R., Chia, N., and DiBaise, J. K. (2014). Prolonged use of a proton pump inhibitor reduces microbial diversity: implications for Clostridium difficile susceptibility. Microbiome 2, 42. doi:10.1186/2049-2618-2-42
Sun, Y., Cai, Y., Liu, L., Yu, F., Farrell, M. L., McKendree, W., et al. (2009). ESPRIT: estimating species richness using large collections of 16S rRNA pyrosequences. Nucleic Acids Res. 37, e76. doi:10.1093/nar/gkp285
Tiihonen, J., Lonnqvist, J., Wahlbeck, K., Klaukka, T., Niskanen, L., Tanskanen, A., et al. (2009). 11-year follow-up of mortality in patients with schizophrenia: a population-based cohort study (FIN11 study). Lancet 374, 620–627. doi:10.1016/S0140-6736(09)60742-X
Turnbaugh, P. J., Ridaura, K., Faith, J. J., Rey, F. E., Knight, R., and Gordon, J. I. (2009). The effect of diet on the human gut microbiome: a metagenomic analysis in humanized gnotobiotic mice. Sci. Transl. Med. 1, 6ra14. doi:10.1126/scitranslmed.3000322
Tyakht, A. V., Kostryukova, E. S., Popenko, A. S., Belenikin, M. S., Pavlenko, A. V., Larin, A. K., et al. (2013). Human gut microbiota community structures in urban and rural populations in Russia. Nat. Commun. 4, 2469. doi:10.1038/ncomms3469
van Baarlen, P., Troost, F., van der Meer, C., Hooiveld, G., Boekschoten, M., Brummer, R. J., et al. (2011). Human mucosal in vivo transcriptome responses to three lactobacilli indicate how probiotics may modulate human cellular pathways. Proc. Natl Acad. Sci. U.S.A. 108, 4562–4569. doi:10.1073/pnas.1000079107
Walker, A. W., Ince, J., Duncan, S. H., Webster, L. M., Holtrop, G., Ze, X., et al. (2011). Dominant and diet-responsive groups of bacteria within the human colonic microbiota. ISME J. 5, 220–230. doi:10.1038/ismej.2010.118
Woodmansey, E. J., McMurdo, M. E., Macfarlane, G. T., and Macfarlane, S. (2004). Comparison of compositions and metabolic activities of fecal microbiotas in young adults and in antibiotic-treated and non-antibiotic-treated elderly subjects. Appl. Environ. Microbiol. 70, 6113–6122. doi:10.1128/AEM.70.10.6113-6122.2004
Wu, G. D., Chen, J., Hoffmann, C., Bittinger, K., Chen, Y. Y., Keilbaugh, S. A., et al. (2011). Linking long-term dietary patterns with gut microbial enterotypes. Science 334, 105–108. doi:10.1126/science.1208344
Zwielehner, J., Liszt, K., Handschur, M., Lassl, C., Lapin, A., and Haslberger, A. G. (2009). Combined PCR-DGGE fingerprinting and quantitative-PCR indicates shifts in fecal population sizes and diversity of Bacteroides, Bifidobacteria and Clostridium cluster IV in institutionalized elderly. Exp. Gerontol. 44, 440–446. doi:10.1016/j.exger.2009.04.002
Keywords: geriatric, gut, metagenome, probiotics, MTCC 5463
Citation: Senan S, Prajapati JB, Joshi CG, Sreeja V, Gohel MK, Trivedi S, Patel RM, Pandya H, Singh US, Phatak A and Patel HA (2015) Geriatric respondents and non-respondents to probiotic intervention can be differentiated by inherent gut microbiome composition. Front. Microbiol. 6:944. doi: 10.3389/fmicb.2015.00944
Received: 12 February 2015; Accepted: 14 August 2015;
Published: 08 September 2015
Edited by:
Kate Howell, University of Melbourne, AustraliaReviewed by:
Stella Maris Reginensi Rivera, Universidad de la República Oriental del Uruguay, UruguayAmit Kumar Tyagi, The University of Texas MD Anderson Cancer Center, USA
Copyright: © 2015 Senan, Prajapati, Joshi, Sreeja, Gohel, Trivedi, Patel, Pandya, Singh, Phatak and Patel. This is an open-access article distributed under the terms of the Creative Commons Attribution License (CC BY). The use, distribution or reproduction in other forums is permitted, provided the original author(s) or licensor are credited and that the original publication in this journal is cited, in accordance with accepted academic practice. No use, distribution or reproduction is permitted which does not comply with these terms.
*Correspondence: Jashbhai B. Prajapati, Department of Dairy Microbiology, SMC College of Dairy Science, Anand Agricultural University, Anand, Gujarat 388 110, India,cHJhamFwYXRpamFzaGJoYWlAeWFob28uY29tLA==amJwcmFqYXBhdGlAYWF1Lmlu