- 1Research Group Systems Biology and Bioinformatics, Leibniz Institute for Natural Product Research and Infection Biology – Hans Knoell Institute, Jena, Germany
- 2Computational Systems Biology Group, Department of Bioengineering, Gebze Technical University, Kocaeli, Turkey
- 3Department of Computer Engineering, Gebze Technical University, Kocaeli, Turkey
In the emerging field of systems biology of fungal infection, one of the central roles belongs to the modeling of gene regulatory networks (GRNs). Utilizing omics-data, GRNs can be predicted by mathematical modeling. Here, we review current advances of data-based reconstruction of both small-scale and large-scale GRNs for human pathogenic fungi. The advantage of large-scale genome-wide modeling is the possibility to predict central (hub) genes and thereby indicate potential biomarkers and drug targets. In contrast, small-scale GRN models provide hypotheses on the mode of gene regulatory interactions, which have to be validated experimentally. Due to the lack of sufficient quantity and quality of both experimental data and prior knowledge about regulator–target gene relations, the genome-wide modeling still remains problematic for fungal pathogens. While a first genome-wide GRN model has already been published for Candida albicans, the feasibility of such modeling for Aspergillus fumigatus is evaluated in the present article. Based on this evaluation, opinions are drawn on future directions of GRN modeling of fungal pathogens. The crucial point of genome-wide GRN modeling is the experimental evidence, both used for inferring the networks (omics ‘first-hand’ data as well as literature data used as prior knowledge) and for validation and evaluation of the inferred network models.
Introduction
While most of fungal species are harmless for human, some can cause infections (mucoses) with very high mortality rates. The number of fungal infections has increased over the last decades due to the rising number of immunocompromised patients (Pfaller and Diekema, 2010). Mucoses are associated with excessive morbidity and mortality because of difficulties with diagnosis and antifungal therapy. Fungal pathogens have evolved diverse strategies for colonisation, adhesion, invasion, translocation, nutrient acquisition, interaction with immune cells of the host, and (secondary) metabolite synthesis (Brakhage, 2013). About 600 fungal species have been reported to infect human (Brown et al., 2012a). Over 90% of fungal infections resulting in death are caused by Candida, Cryptococcus, Aspergillus, and Pneumocystis. These species cause over two million life-threatening infections each year (Brown et al., 2012b). Species belonging to the genera Candida and Aspergillus are widely recognized as the most important human fungal pathogens (Moran et al., 2011).
A key feature of fungal infections is the interaction between different morphotypes of fungal cells (colonizing, persisting, or invading) and all kinds of tissues and immune effector cells of the host. Because of their complexity, the elucidation of invasive fungal infections requires a systems biological approach for modelling the complex interactions between a large number of genes, proteins, metabolites, cells, and tissues from both the pathogen(s) and the host (Forst, 2006). For reviews of the systems biology of infections caused by the human pathogenic fungi Candida albicans and Aspergillus fumigatus, (see e.g., Albrecht et al., 2008, 2011; Rizzetto and Cavalieri, 2011; Horn et al., 2012, 2014).
The dynamics of host-pathogen interactions has to be analyzed and modeled in temporal and spatial resolution on different scales. Recently, we reviewed the emerging multi-scale modeling of bacterial and microbial infections (Schleicher et al., 2016). Dühring et al. (2015) reviewed interaction mechanisms between the human innate immune system and C. albicans focussing on the mathematical modeling of immune evasion strategies. These molecular interactions can be modelled by networks composed of nodes (molecules) connected by edges (interactions). Network models of host–pathogen interactions, such as protein–protein interactions, signaling, metabolic, and gene regulatory networks (GRNs) can be computationally reconstructed by exploiting molecular databases, literature mining and model fit to experimental data (Durmuş et al., 2015).
Focussing on the transcriptomic scale, here we review fungal GRN models, where nodes represent genes and edges describe causal regulator–target gene relations. GRN reconstruction is mainly based on transcriptome data supported by prior knowledge interactions derived from literature. Additionally, a set of experimentally verified interactions is needed as ‘gold standard’ for validation of the inferred model (Hecker et al., 2009; Linde et al., 2015). In the present article, we compare the feasibility of genome-wide vs. small-scale modelling under the current state of (i) species-specific interactome knowledge, (ii) prior knowledge retrieved via orthologous genes in other fungal species, (iii) transcriptome data for model fit, and (iv) confirmed knowledge for model validation.
Here, we discuss GRN prediction for A. fumigatus – one of the most important causes of life-threatening invasive mycoses. While a genome-wide network model for for C. albicans has been published based on a compendium of microarray data and knowledge extracted by molecular database search and literature mining (Altwasser et al., 2012), we here show that genome-wide GRN modeling with sufficient quality is currently not feasible for A. fumigatus. We found that this is due to a larger genome compared with C. albicans in conjunction with a lower number of known interactions and publicly available transcriptome data sets. Finally, systems biology of fungal infection requires modeling GRNs not only in the pathogen but also in the host and modeling of molecular interactions between them. This was demonstrated so far only for C. albicans inferring a small-scale model (Tierney et al., 2012).
Species-Specific Genomic Knowledge About the Fungal Pathogens
The current knowledge about molecular interactions for C. albicans and A. fumigatus is relatively moderate compared with the comprehensive knowledge about the model eukaryote Saccharomyces cerevisiae that is represented in more than 100,000 scientific publications. Moreover, the knowledge about A. fumigatus is scarcer than that for C. albicans as shown in Table 1. For C. albicans with 6,218 genes (ORFs) there are more than 33,000 journal papers whereas for A. fumigatus, despite a larger genome size, there are less than 10,000 articles referenced in PubMed.
Curated databases have been developed to provide improved computational access to the biologically important information. Basic genomic knowledge about C. albicans and A. fumigatus is provided by the Candida Genome Database (CGD; Skrzypek et al., 2016) and Aspergillus Genome Databas (AspDB; Cerqueira et al., 2014), respectively. Additionally, functional categorisation of fungal genes and proteins can be extracted for 298 fungal strains by the Web tool FungiFun2 (Priebe et al., 2014).
In general, manually curated databases have high quality. However, they cannot be up-to-date as it takes time before new discoveries are included (Baumgartner et al., 2007). Therefore, the data presented in databases, such as CGD, AspDB, and TRANSFAC (Wingender, 2008), does not fully represent the current state of rapidly growing knowledge. Obviously, this is the case for TRANSFAC that does not provide yet any information about TFs in A. fumigatus (Table 1). The model fungus S. cerevisiae has an estimated number of 140 to 250 TFs (Costanzo et al., 2014) and about 600 proteins may be involved in the transcriptional regulation of approximately 6,000 protein-coding genes (Cherry et al., 2012). The number of TFs varies according to the selection criteria. Some studies count proteins with predicted DNA-binding domains; others include only proteins shown to bind DNA directly; still others also include non-DNA binding subunits of TF complexes. The regulatory proteins can be subdivided into the basal machinery, co-regulators, chromatin-remodeling and modifying factors, as well as DNA-binding TFs. We estimate for A. fumigatus a number of about 600 TFs (unpublished results). Compared to these estimated high number of TFs, the number of experimentally verified TFs that are included in TRANSFAC is very small. However, the list of predicted (putative) TFs is increasing. For instance, based on the first complete genome sequence for A. fumigatus Af293, a set of 28 transcriptional regulators (22 TFs) were predicted (Nierman et al., 2005). The AspDB currently assigns 273 gene loci to a TF.
Prior Knowledge From Other Databases Via Orthologous Genes
An approach to fill the gap of missing species-specific knowledge is to make use of known interactions in closely related species via the mapping of orthologs taking into account the problem that the regulation of a gene might be different between phylogenetically close fungal species. Linde et al. (2011) and Altwasser et al. (2012) mapped orthologous genes of several fungal species including S. cerevisiae, A. nidulans, C. glabrata to C. albicans and screened four databases for transcriptional interactions: (i) TRANSFAC, (ii) MPact (Güldener et al., 2006), (iii) the transcriptional regulatory network for yeast by Balaji et al. (2006), and (iv) BIND (Bader et al., 2003). In this article, we present primary results from genome-wide network inference for A. fumigatus. Therefore, we exploited three of these [TRANSFAC, MPact and Balaji et al. (2006)] and additionally used the STRING database (Szklarczyk et al., 2015) and BioGRID (Chatr-Aryamontri et al., 2015). The numbers of gene regulatory interactions and genes involved are shown in Table 1.
Fungal Transcriptome Data
To identify and validate unknown putative gene regulatory interactions, a compendium of experimental gene expression data should be collected and analyzed. Ihmels et al. (2005) published a collection of microarray-based transcriptome data for 6,167 genes of C. albicans under 198 experimental conditions. A search for C. albicans in the Gene Expression Omnibus database (GEO; Barrett et al., 2013) resulted in transcriptome data sets composed of 1,846 samples at 2016/01/06. Among them, 1,467 samples were analyzed by microarrays and 379 samples were analyzed by high-throughput sequencing (HTS; 263 of them using Illumina HiSeq 2500).
For A. fumigatus, Nierman et al. (2005) published the first gene expression data set. At 2016/01/06 GEO contained transcriptome data from 101 samples analyzed by HTS of which we used 81 (GEO: GSE55743, GSE55663, GSE55943, and GSE30579). In addition, we considered unpublished HTS data of 79 samples from A. fumigatus. To avoid incompatibility problems between HTS and microarray data, we restricted the data analysis for A. fumigatus to the HTS data from the total of 160 samples. The HTS data was preprocessed as described by Schulze et al. (2015).
Knowledge Extracted By Text Mining for Model Validation
To provisionally fill the gap of knowledge between the high number of expected and the low number of verified TFs and their target genes, articles downloaded from PubMed (abstracts) or PMC (full text) were computationally scanned using a software developed by us (Durmuş et al., 2015). The articles were preprocessed to obtain relevant sentences which were parsed afterward using natural language processing.
For C. albicans more than 20,000 articles were scanned for interactions comprising the following words (and its derivatives): bind, regulate, promote, suppress, in/activate, enhance, overexpress, attenuate, induce, block, inhibit, repress. A number of 4,625 unique interactions (including 1,388 interactions added from CGD) between 372 regulators and 1,484 target genes were found (Table 1). In a previous study using a text mining tool (JRex), we scanned about 9,000 articles on C. albicans and found 1,016 interactions between 509 genes (Altwasser et al., 2012).
In contrast, for A. fumigatus we scanned 1,580 open-access full-text articles and 6,420 abstracts of non-open-access articles. Automatic identification of 136 regulatory interactions between 28 regulators and 104 target genes complemented by manually curated interactions resulted in 31 regulators (including 15 TFs, Supplementary Table S1) and a total of 153 regulatory interactions. Based on the aforementioned knowledge for A. fumigatus, the well-known TFs SrbA, SreA, LaeA, BrlA, and Yap1 were identified as hubs (>7 regulator-target gene interactions). After addition of further interactions retrieved from AspGD, we obtained for A. fumigatus a set of 321 gene regulatory interactions between 273 genes, which was used as ‘gold standard’ for GRN model validation as described in the next section. This shows that despite the larger size of the genome, the numbers of known interactions are significantly smaller for A. fumigatus than for C. albicans.
Large-Scale Modeling of GRN in Pathogenic Fungi
There exists an impressive corpus of studies in the field of mathematical modeling and identification of GRN. For reviews see Hartemink (2005), Hecker et al. (2009), Huang et al. (2009), Goldenberg et al. (2010), and Linde et al. (2015). Genome-scale network models arose shortly after the first genome sequencing, starting with constraint-based metabolic network models (Bordbar et al., 2014). Most of the published genome-wide models are knowledge-based. Protein–protein interaction network models of C. albicans and A. fumigatus were published (Remmele et al., 2015) based on sequence similarity of known interaction and supported by GO enrichment analysis. As the available knowledge for the fungi, in particular for A. fumigatus, is scarce and far from complete, novel interactions have to be predicted by the integration of experimental data into the process of model construction. Due to the availability of data from only less than 200 samples compared to about 10.000 genes (see Table 1), low complex models were fitted, e.g., by regression of linear models. This was performed for C. albicans (Altwasser et al., 2012) using the workflow depicted in Figure 1, but to the best of our knowledge not yet for A. fumigatus and other human pathogenic fungi. Altwasser et al. (2012) inferred a linear regression model describing the interactions between regulators (expression intensity xj) and target genes (xi) of N (6,167) genes for different experimental conditions (samples m) with penalty defined by the prior knowledge:
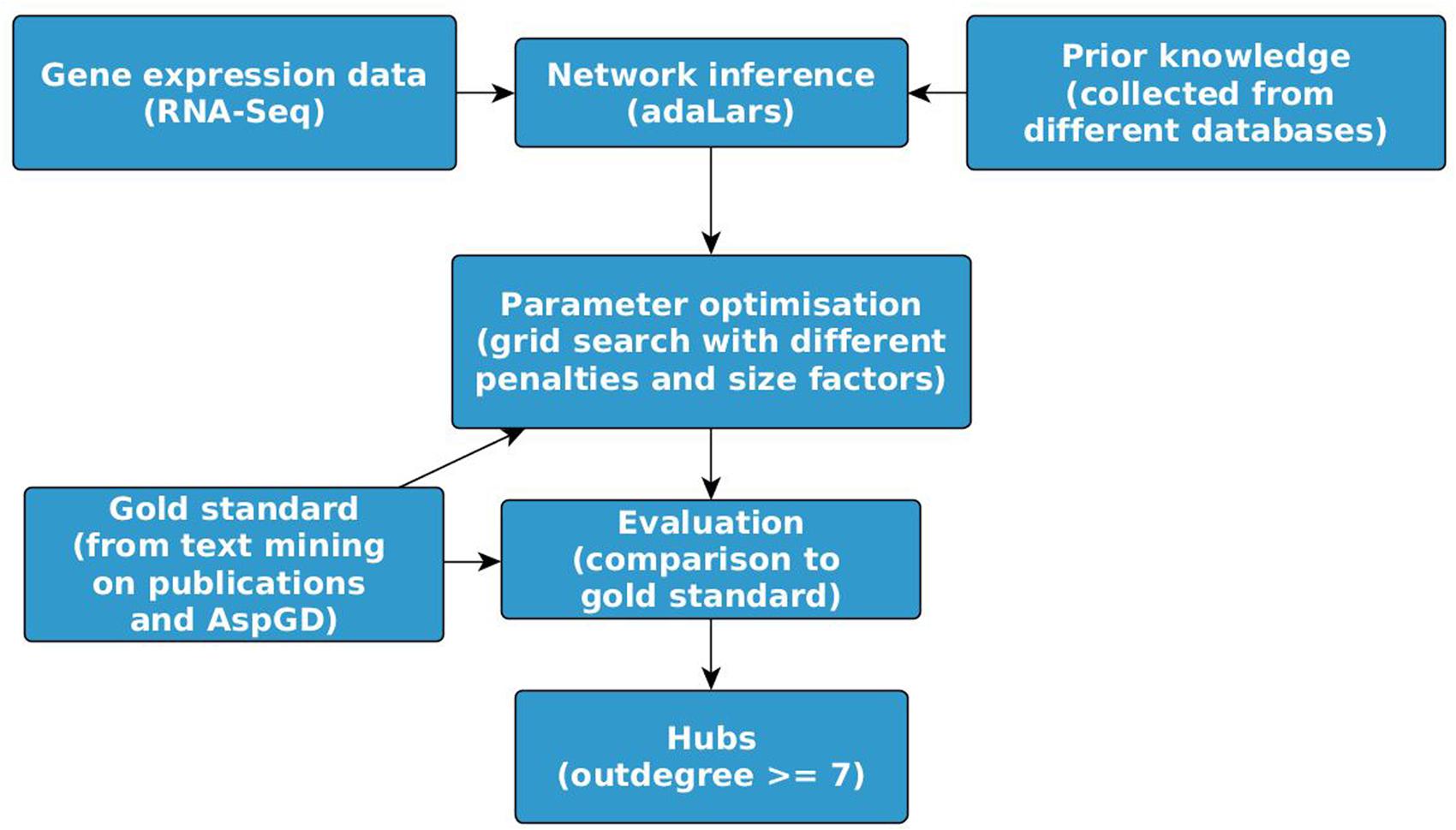
FIGURE 1. Workflow for large-scale GRN inference. For the example GRN for Aspergillus fumigatus, the network was inferred using the software package ‘adaLARS,’ an adaptive LASSO algorithm (Zou, 2006) combined with ridge regression as used by Altwasser et al. (2012). The prior knowledge was collected for this example from different databases as listed in Table 1 under ‘Prior knowledge from other databases via orthologs.’ The Gold standard was extracted as described in section ‘Knowledge extracted by text mining for model validation.’ The hubs were identified using the tool igraph (Csardi and Nepusz, 2006).
The identification of the parameters βi,j was performed using an adaptive LASSO (Least Absolute Shrinkage and Selection Operator) combined with ridge regression.
As the ‘gold standard’ for model validation in Altwasser et al. (2012) comprised only 503 genes, the refined GRN inference was restricted to this subset genes. The model was fitted to the transcription data collected by Ihmels et al. (2005) with 198 samples.
Here, we present preliminary results of genome-wide modeling for A. fumigatus based on HTS data from 160 samples and the knowledge (prior knowledge and ‘gold standard’) surveyed in Table 1. The workflow is depicted in Figure 1 and the inferred network with highlighted hubs in the Supplementary Figure S1. The precision and sensitivity of the model quantified by the F-measure of inferred genome-wide GRN is low due to several reasons: the number of samples (160) is very small compared with the number of genes (almost 10,000). This is called the dimensionality problem (‘curse of dimensionality’). In general, this problem can be reduced by integration of prior knowledge. However, for both C. albicans and A. fumigatus, the overlap of the prior knowledge extracted from different databases and the ‘gold standard’ (obtained by text mining) is very small (as shown for C. albicans by Altwasser et al., 2012). For A. fumigatus, the overlap between the 47,230 interactions used as prior knowledge (including non-species-specific data) and the 321 interactions used as ‘gold standard’ (species-specific knowledge) is only 25 – indicating the high diversity of fungal species with respect to gene regulation. Nevertheless, despite the modest quality of the inferred large-scale network model for A. fumigatus, the identified hubs were found to be independent of the parameters of the inference algorithm.
Small-Scale Modeling of GRN in Pathogenic Fungi
While the quality of genome-wide models for pathogenic fungi is low due to (i) the moderate amount of publicly available transcriptome data and (ii) the sparse quality and quantity of experimentally verified knowledge, there are some examples for the inference of small-scale modeling of GRNs for C. albicans and A. fumigatus with sufficient reliability. Using the NetGenerator algorithm (Guthke et al., 2005; Weber et al., 2013), a first model comprising four genes (Guthke et al., 2007) was presented for A. fumigatus based on transcriptome data published by Nierman et al. (2005). Later, small-scale networks for A. fumigatus with 12 and 26 genes were published by Linde et al. (2012) and Altwasser et al. (2015), respectively. Small-scale GRN models for C. albicans were also inferred using the NetGenerator algorithm and presented by Linde et al. (2010), Tierney et al. (2012), Ramachandra et al. (2014), Schulze et al. (2015), and Böhringer et al. (2016), whereas the articles on small-scale GRN inference used the top–down approach and simulated the expression profiles of selected genes using ordinary differential equations, Brandon et al. (2015) applied a bottom up approach to investigate the iron acquisition and oxidative stress response in A. fumigatus by discrete dynamic simulation of Boolean network models.
In all cases, the critical challenge in modeling of small-scale GRNs is the selection of genes. Mostly, the feature selection is supported by both prior knowledge and unsupervised learning algorithms, e.g., clustering or module identification.
An advantage and benefit of small-scale modeling is the opportunity to predict a small set of hypotheses about previously unknown gene regulatory interactions, that needs to be verified experimentally as done for the models by Linde et al. (2012), Tierney et al. (2012), Altwasser et al. (2015) and Böhringer et al. (2016). In these cases, small-scale GRN models supported the experimental design for the follow-up investigation of transcriptomic regulation in pathogenic fungi.
Future Research
The molecular understanding of fungal pathogens will become more important as there are an ever-growing number of drug-resistant strains of human pathogenic fungi and an increasing number of immunocompromised individuals. Most certainly, the modeling of omics data will pave the way for predictive diagnostics and personalized treatment of fungal infections (Oliveira-Coelho et al., 2015). In future this modeling approach will be extended from the molecular to the cellular, organism, and population scale (Schleicher et al., 2016).
The modeling of GRNs is crucial to predict potential drug targets for improved treatment of fungal infections. One of the aims of GRN modeling is to identify ‘hubs,’ i.e., important transcriptional regulators, which are highly connected. Therefore, it is necessary to reconstruct the topology of the GRNs as precise as possible. Currently, only small-scale GRN models were inferred with sufficient reliability, i.e., their in silico predictions could be experimentally verified. The disadvantage of small-scale models is the need of feature selection, i.e., the selection of a number of genes of interest. Such investigation is hypothesis-driven and does make sense only in connection with a certain focussed scientific question. In contrast, systems biology claims a holistic perspective, which requires large-scale GRN modeling. We reviewed here the feasibility for large-scale GRN modeling for the two most important human pathogenic fungi C. albicans and A. fumigatus. For C. albicans such a model was previously published, but it could now be improved based on (i) the increased amount of transcriptional data, (ii) enhanced set of prior knowledge, and (iii) extended set of knowledge extracted by text mining for model validation as shown in Table 1.
In contrast, for A. fumigatus the quantity and quality of data and knowledge have to be improved before the time will be ripe for genome-wide GRN modeling. These requirements will be important for efficient genome-wide GRN modelling with high reliability also for other fungi: (i) curation of a more comprehensive database of TFs and TF-binding sites of human pathogenic fungi, (ii) more standardized reporting of results in the scientific literature using ontologies and controlled vocabulary, (iii) improved text mining algorithms to extract knowledge about gene regulatory interactions to reduce the effort for manual curation of the databases, (iv) increase in the number of gene expression data sets covering more experimental conditions of fungi in public repositories, (v) investigation on comparative genomics with respect to gene regulation pattern of different fungi to improve a more careful inclusion or exclusion of non-species-specific prior knowledge into the inference of large-scale GRN models. Currently, reliable genome-wide GRN modeling with some thousands of genes is impossible due to the scarce knowledge for model validation, i.e., there is no confirmed ‘gold standard.’ Today, the size of network models has to be adapted to the number of some dozens or hundreds of genes with sufficient knowledge about gene regulatory interactions.
Author Contributions
RG and JL conceived and drafted the manuscript. SG collected and processed the transcriptome data for Candida albicans and Aspergillus fumigatus and performed the network inference for A. fumigatus. TC and SV contritubed to the network inference algorithms. SD, TC and FS performed the text mining. ES contributed to the transcription factors. All approved the final manuscript.
Conflict of Interest Statement
The authors declare that the research was conducted in the absence of any commercial or financial relationships that could be construed as a potential conflict of interest.
Acknowledgments
This work was supported by the Deutsche Forschungsgemeinschaft CRC/Transregio 124 ‘Pathogenic fungi and their human host: Networks of interaction’ sub-projects B3 to RG and ES and INF to JL, the Jena School for Microbial Communication to TC, and the German Federal Ministry of Education and Research (BMBF, ‘InfectControl’, sub-project FINAR) to SV.
Supplementary Material
The Supplementary Material for this article can be found online at: http://journal.frontiersin.org/article/10.3389/fmicb.2016.00570
References
Albrecht, D., Guthke, R., Kniemeyer, O., and Brakhage, A. A. (2008). “Systems biology of human-pathogenic fungi,” in Handbook of Research on Systems Biology Applications in Medicine, Vol. 1, ed. A. Daskalaki (Hershey: IGI Global), 400–418.
Albrecht, D., Kniemeyer, O., Mech, F., Gunzer, M., Brakhage, A. A., and Guthke, R. (2011). On the way toward systems biology of Aspergillus fumigatus infection. Int. J. Med. Microbiol. 301, 453–459. doi: 10.1016/j.ijmm.2011.04.014
Altwasser, R., Baldin, C., Weber, J., Guthke, R., Kniemeyer, O., Brakhage, A. A., et al. (2015). Network modeling reveals cross talk of MAP kinases during adaptation to caspofungin stress in Aspergillus fumigatus. PLoS ONE 10:e0136932. doi: 10.1371/journal.pone.0136932
Altwasser, R., Linde, J., Buyko, E., Hahn, U., and Guthke, R. (2012). Genome-wide scale-free network inference for Candida albicans. Front. Microbiol. 3:51. doi: 10.3389/fmicb.2012.00051
Aspergillus Genome Database [ASPGD] (2016). A Aspergillus fumigatus Af293 Genome Snapshot Overview. Available at: http://www.aspergillusgenome.org/ [accessed on January 26, 2016].
Bader, G. D., Betel, D., and Hogue, C. W. (2003). BIND: the biomolecular interaction network database. Nucleic Acids Res. 31, 248–250. doi: 10.1093/nar/gkg056
Balaji, S., Babu, M. M., Iyer, L. M., Luscombe, N. M., and Aravind, L. (2006). Comprehensive analysis of combinatorial regulation using the transcriptional regulatory network of yeast. J. Mol. Biol. 360, 213–227. doi: 10.1016/j.jmb.2006.04.029
Barrett, T., Wilhite, S. E., Ledoux, P., Evangelista, C., Kim, I. F., Tomashevsky, M., et al. (2013). NCBI GEO: archive for functional genomics data sets — update. Nucleic Acids Res. 41, D991–D995. doi: 10.1093/nar/gks1193
Baumgartner, W. A., Cohen, K. B., Fox, L. M., Acquaah-Mensah, G., and Hunter, L. (2007). Manual curation is not suffcient for annotation of genomic databases. Bioinformatics 23, i41–i48. doi: 10.1093/bioinformatics/btm229
Böhringer, M., Pohlers, S., Schulze, S., Albrecht-Eckardt, D., Piegsa, J., Weber, M., et al. (2016). Candida albicans infection leads to barrier breakdown and a MAPK/NF-κB mediated stress response in the intestinal epithelial cell line C2BBe1. Cell Microbiol. doi: 10.1111/cmi.12566 [Epub ahead of print].
Bordbar, A., Monk, J. M., King, Z. A., and Palsson, B. O. (2014). Constraint-based models predict metabolic and associated cellular functions. Nat. Rev. Genet. 15, 107–120. doi: 10.1038/nrg3643
Brakhage, A. A. (2013). Regulation of fungal secondary metabolism. Nat. Rev. Microbiol. 11, 21–32. doi: 10.1038/nrmicro2916
Brandon, M., Howard, B., Lawrence, C., and Laubenbacher, R. (2015). Iron acquisition and oxidative stress response in Aspergillus fumigatus. BMC Syst. Biol. 9:19. doi: 10.1186/s12918-015-0163-1
Brown, G. D., Denning, D. W., and Levitz, S. M. (2012a). Tackling human fungal infections. Science 336:647. doi: 10.1126/science.1222236
Brown, G. D., Denning, D. W., Gow, N. A., Levitz, S. M., Netea, M. G., and White, T. C. (2012b). Hidden killers: human fungal infections. Sci. Transl. Med. 4, 165rv13. doi: 10.1126/scitranslmed.3004404
Candida Genome Database [CGD] (2016). Candida albicans SC5314 Genome Snapshot Overview. Available at: http://www.candidagenome.org [accessed on January 26, 2016].
Cerqueira, G. C., Arnaud, M. B., Inglis, D. O., Skrzypek, M. S., Binkley, G., Simison, M., et al. (2014). The Aspergillus genome database: multispecies curation and incorporation of RNA-Seq data to improve structural gene annotations. Nucleic Acids Res. 42, D705–D710. doi: 10.1093/nar/gkt1029
Chatr-Aryamontri, A., Breitkreutz, B. J., Oughtred, R., Boucher, L., Heinicke, S., Chen, D., et al. (2015). The BioGRID interaction database: 2015 update. Nucleic Acids Res. 43, D470–D478. doi: 10.1093/nar/gku1204
Cherry, J. M., Hong, E. L., Amundsen, C., Balakrishnan, R., Binkley, G., Chan, E. T., et al. (2012). Saccharomyces Genome Database: the genomics resource of budding yeast. Nucleic Acids Res. 40, D700–D705. doi: 10.1093/nar/gkr1029
Costanzo, M. C., Engel, S. R., Wong, E. D., Lloyd, P., Karra, K., Chan, E. T., et al. (2014). Saccharomyces genome database provides new regulation data. Nucleic Acids Res. 42, D717–D725. doi: 10.1093/nar/gkt1158
Csardi, G., and Nepusz, T. (2006). The igraph software package for complex network research. Int. J. Complex Syst. 1695, 1–9.
Dühring, S., Germerodt, S., Skerka, C., Zipfel, P. F., Dandekar, T., and Schuster, S. (2015). Host-pathogen interactions between the human innate immune system and Candida albicans - understanding and modeling defense and evasion strategies. Front. Microbiol. 6:625. doi: 10.3389/fmicb.2015.00625
Durmuş, S., Cakir, T., Ozgur, A., and Guthke, R. (2015). A review on computational systems biology of pathogen-host interactions. Front. Microbiol. 6:235. doi: 10.3389/fmicb.2015.00235
Forst, C. V. (2006). Host–pathogen systems biology. Drug Discov. Today 11, 220–227. doi: 10.1016/S1359-6446(05)03735-9
Goldenberg, A., Zheng, A. X., Fienberg, S. E., and Airoldi, E. M. (2010). A survey of statistical network models. Foundat. Trends Machine Learn. 2, 129–133. doi: 10.1561/2200000005
Güldener, U., Münsterkötter, M., Oesterheld, M., Pagel, P., Ruepp, A., Mewes, H. W., et al. (2006). MPact: the MIPS protein interaction resource on yeast. Nucleic Acids Res. 34, D436–D441. doi: 10.1093/nar/gkj003
Guthke, R., Kniemeyer, O., Albrecht, D., Brakhage, A. A., and Moeller, U. (2007). Discovery of gene regulatory networks in Aspergillus fumigatus. Lect. Notes Bioinf. 4366, 22–41.
Guthke, R., Möller, U., Hoffmann, M., Thies, F., and Töpfer, S. (2005). Dynamic network reconstruction from gene expression data applied to immune response during bacterial infection. Bioinformatics 21, 1626–1634. doi: 10.1093/bioinformatics/bti226
Hartemink, A. J. (2005). Reverse engineering gene regulatory networks. Nat. Biotechnol. 23, 554–555. doi: 10.1038/nbt0505-554
Hecker, M., Lambeck, S., Toepfer, S., van Someren, E., and Guthke, R. (2009). Gene regulatory network inference: data integration in dynamic models-a review. Biosystems 96, 86–103. doi: 10.1016/j.biosystems.2008.12.004
Horn, F., Heinekamp, T., Kniemeyer, O., Pollmächer, J., Valiante, V., and Brakhage, A. A. (2012). Systems biology of fungal infection. Front. Microbiol. 3:108. doi: 10.3389/fmicb.2012.00108
Horn, F., Valiante, V., Guthke, R., and Brakhage, A. A. (2014). “Data-driven systems biology of fungal infections,” in Human Pathogenic Fungi: Molecular Biology and Pathogenic Mechanisms, eds D. Sullivan and G. P. Moran (Norfolk: Caister Academic Press).
Huang, Y., Tienda-Luna, I. M., and Wang, Y. (2009). A survey of statistical models for reverse engineering gene regulatory networks. IEEE Signal Process. Mag. 26, 76–97. doi: 10.1109/MSP.2008.930647
Ihmels, J., Bergmann, S., Berman, J., and Barkai, N. (2005). Comparative gene expression analysis by a differential clustering approach: application to the Candida albicans transcription program. PLoS Genet. 1:e39. doi: 10.1371/journal.pgen.0010039
Linde, J., Buyko, E., Altwasser, R., Hahn, U., and Guthke, R. (2011). “Full-genomic network inference for non-modell organisms: a case study for the fungal pathogen Candida albicans,” in Proceeding of the International Conference on Bioinformatics, Computational Biology and Biomedical Engineering, ICBCBBE2011, Marseille, 430–434. [accessed August 24–26, 2011].
Linde, J., Hortschansky, P., Fazius, E., Brakhage, A. A., Guthke, R., and Haas, H. (2012). Regulatory interactions for iron homeostasis in Aspergillus fumigatus inferred by a Systems Biology approach. BMC Syst. Biol. 6:6. doi: 10.1186/1752-0509-6-6
Linde, J., Schulze, S., Henkel, S. G., and Guthke, R. (2015). Data- and knowledge-based modeling of gene regulatory networks: an update EXCLI. J. Exp. Clin. Sci. 14, 346–378. doi: 10.17179/excli2015-168
Linde, J., Wilson, D., Hube, B., and Guthke, R. (2010). Regulatory network modelling of iron acquisition by a fungal pathogen in contact with epithelial cells. BMC Syst. Biol. 4:148. doi: 10.1186/1752-0509-4-148
Moran, G. P., Coleman, D. C., and Sullivan, D. J. (2011). Comparative genomics and the evolution of pathogenicity in human pathogenic fungi. Eukaryot. Cell 10, 34–42. doi: 10.1128/EC.00242-10
Nierman, W. C., Pain, A., Anderson, M. J., Wortman, J. R., Kim, H. S., Arroyo, J., et al. (2005). Genomic sequence of the pathogenic and allergenic filamentous fungus Aspergillus fumigatus. Nature 438, 1151–1156. doi: 10.1038/nature04332
Oliveira-Coelho, A., Rodrigues, F., Campos, A., Lacerda, J. F., Carvalho, A., and Cunha, C. (2015). Paving the way for predictive diagnostics and personalized treatment of invasive aspergillosis. Front. Microbiol. 6:411. doi: 10.3389/fmicb.2015.00411
Pfaller, M. A., and Diekema, D. J. (2010). Epidemiology of invasive mycoses in North America. Crit. Rev. Microbiol. 36, 1–53. doi: 10.3109/10408410903241444
Priebe, S., Kreisel, C., Horn, F., Guthke, R., and Linde, J. (2014). FungiFun2: a comprehensive online resource for systematic analysis of gene lists from fungal species. Bioinformatics 31, 445–446. doi: 10.1093/bioinformatics/btu627
Ramachandra, S., Linde, J., Brock, M., Guthke, R., Hube, B., and Brunke, S. (2014). Regulatory networks controlling nitrogen sensing and uptake in Candida albicans. PLoS ONE 9:e92734. doi: 10.1371/journal.pone.0092734
Remmele, C. W., Luther, C. H., Balkenhol, J., Dandekar, T., Müller, T., and Dittrich, M. T. (2015). Integrated inference and evaluation of host-fungi interaction networks. Front. Microbiol. 6:764. doi: 10.3389/fmicb.2015.00764
Rizzetto, L., and Cavalieri, D. (2011). Friend or foe: using systems biology to elucidate interactions between fungi and their hosts. Trends Microbiol. 19, 509–515. doi: 10.1016/j.tim.2011.07.007
Schleicher, J., Conrad, T., Gustafsson, M., Cedersund, G., Guthke, R., and Linde, J. (2016). Facing the challenges of multiscale modelling of bacterial and fungal pathogen-host interactions. Briefings Funct. Genomics 2016, 1–13. doi: 10.1093/bfgp/elv064
Schulze, S., Henkel, S. G., Driesch, D., Guthke, R., and Linde, J. (2015). Computational prediction of molecular pathogen-host interactions based on dual transcriptome data. Front. Microbiol. 6:65. doi: 10.3389/fmicb.2015.00065
Skrzypek, M. S., Binkley, J., and Sherlock, G. (2016). How to use the candida genome database. Methods Mol. Biol. 1356, 3–15. doi: 10.1007/978-1-4939-3052-4_1
Szklarczyk, D., Franceschini, A., Wyder, S., Forslund, K., Heller, D., Huerta-Cepas, J., et al. (2015). STRING v10: protein-protein interaction networks, integrated over the tree of life. Nucleic Acids Res. 43, D447–D452. doi: 10.1093/nar/gku1003
Tierney, L., Linde, J., Müller, S., Brunke, S., Molina, J. C., Hube, B., et al. (2012). An interspecies regulatory network inferred from simultaneous RNA-seq of Candida albicans invading innate immune cells. Front. Microbiol. 3:85. doi: 10.3389/fmicb.2012.00085
Weber, M., Henkel, S. G., Vlaic, S., Guthke, R., van Zoelen, E. J., and Driesch, D. (2013). Inference of dynamical gene-regulatory networks based on time-resolved multi-stimuli multi-experiment data applying NetGenerator V2.0. BMC Syst. Biol. 7:1. doi: 10.1186/1752-0509-7-1
Wingender, E. (2008). The TRANSFAC project as an example of framework technology that supports the analysis of genomic regulation. Brief. Bioinform. 9, 326–332. doi: 10.1093/bib/bbn016
Keywords: Candida albicans, Aspergillus fumigatus, reverse engineering, text mining, transcription factor, genome-wide modeling
Citation: Guthke R, Gerber S, Conrad T, Vlaic S, Durmuş S, Çakır T, Sevilgen FE, Shelest E and Linde J (2016) Data-based Reconstruction of Gene Regulatory Networks of Fungal Pathogens. Front. Microbiol. 7:570. doi: 10.3389/fmicb.2016.00570
Received: 26 February 2016; Accepted: 05 April 2016;
Published: 22 April 2016.
Edited by:
Dominique Sanglard, University of Lausanne and University Hospital Center, SwitzerlandReviewed by:
Paolo Tieri, Consiglio Nazionale delle Ricerche, ItalyRonald Leonard Westra, Maastricht University, Netherlands
Copyright © 2016 Guthke, Gerber, Conrad, Vlaic, Durmuş, Çakır, Sevilgen, Shelest and Linde. This is an open-access article distributed under the terms of the Creative Commons Attribution License (CC BY). The use, distribution or reproduction in other forums is permitted, provided the original author(s) or licensor are credited and that the original publication in this journal is cited, in accordance with accepted academic practice. No use, distribution or reproduction is permitted which does not comply with these terms.
*Correspondence: Reinhard Guthke, cmVpbmhhcmQuZ3V0aGtlQGhraS1qZW5hLmRl