- 1Laboratory of Synthetic Microbiology, School of Chemical Engineering and Technology, Tianjin University, Tianjin, China
- 2Key Laboratory of Systems Bioengineering – Ministry of Education, Tianjin University, Tianjin, China
- 3SynBio Research Platform, Collaborative Innovation Center of Chemical Science and Engineering, Tianjin, China
- 4Center for Biosafety Research and Strategy, Tianjin University, Tianjin, China
Functions of transcriptional regulators (TRs) are still poorly understood in the model cyanobacterium Synechocystis sp. PCC 6803. To address the issue, we constructed knockout mutants for 32 putative TR-encoding genes of Synechocystis, and comparatively analyzed their phenotypes under autotrophic growth condition and metabolic profiles using liquid chromatography-mass spectrometry-based metabolomics. The results showed that only four mutants of TR genes, sll1872 (lytR), slr0741 (phoU), slr0395 (ntcB), and slr1871 (pirR), showed differential growth patterns in BG11 medium when compared with the wild type; however, in spite of no growth difference observed for the remaining TR mutants, metabolomic profiling showed that they were different at the metabolite level, suggesting significant functional diversity of TRs in Synechocystis. In addition, an integrative metabolomic and gene families’ analysis of all TR mutants led to the identification of five pairs of TR genes that each shared close relationship in both gene families and metabolomic clustering trees, suggesting possible conserved functions of these TRs during evolution. Moreover, more than a dozen pairs of TR genes with different origin and evolution were found with similar metabolomic profiles, suggesting a possible functional convergence of the TRs during genome evolution. Finally, a protein–protein network analysis was performed to predict regulatory targets of TRs, allowing inference of possible regulatory gene targets for 4 out of five pairs of TRs. This study provided new insights into the regulatory functions and evolution of TR genes in Synechocystis.
Introduction
Cyanobacteria contribute significantly to global photosynthetic productivity. It is estimated that more than half of the total primary production essential for life on earth is produced by cyanobacteria. In addition, early studies have found that cyanobacteria were able to establish competitive growth in almost any environment, at least temporarily, liquid water and sunlight, due to their strong abilities of withstanding challenges of environmental perturbations (Badger et al., 2006). Moreover, cyanobacteria have recently attracted significant interest because of their ability to function as a “chassis” to produce renewable carbon neutral biofuels or bioproducts (Atsumi et al., 2009). In spite of their important ecological, environmental, and biotechnological applications, many aspects of cyanobacterial physiology remain poorly understood.
To survive the diversities of environments, abundant and dedicated regulatory systems have evolved in cyanobacteria to achieve precise controls of functional gene expression. In the model cyanobacterium Synechocystis sp. PCC 6803 (hereafter Synechocystis), a significant number of regulatory genes of various types have been identified, among which at least 40 genes were annotated as putative transcriptional regulators (TRs) (Kaneko et al., 2003). So far only a dozen of TRs have ever been functionally characterized in Synechocystis, and the results showed that they were involved in the regulation of a wide range of physiological functions, such as nitrite tolerance (Aichi et al., 2001), iron limitation (Michel and Pistorius, 2004), acid tolerance (Ohta et al., 2005), cadmium tolerance (Houot et al., 2007). However, a majority of TRs in the Synechocystis genome are still functionally unknown, which presents significant challenges not only to the basic sciences of Synechocystis but also to the biotechnological application of Synechocystis as a chassis in producing biofuels and chemicals (Atsumi et al., 2009).
To decipher regulatory function of TRs, various approaches have been previously applied. For example, sequence analysis-based identification and evolutionary analysis of DNA-binding proteins, construction of transcriptional networks including TRs and their target genes and analysis of structure and evolution of these networks (Babu et al., 2004), can be used for functional inference of TRs. Metabolomics is a method to define the diversity of low weight molecules in the cell and to display differences in small molecule abundance. When applied for analysis of cellular responses to genetic or physiological changes, it shows many advantages because metabolites are the functional entities within the cells and their concentration levels vary as a consequence of environmental changes (Zhang et al., 2010). In our previous studies, metabolomic analysis has been applied to the functional characterization of response regulators involved in acid and butanol tolerance (Ren et al., 2014; Niu et al., 2015), and TRs involved in ethanol tolerance in Synechocystis (Zhu et al., 2015), and the results demonstrated that it could be a powerful tool in revealing functional clues for functionally unknown regulatory genes. Towards an ultimate goal of deciphering regulatory functions of TRs in Synechocystis, in this study, we applied a liquid chromatography-mass spectrometry (LC-MS) based metabolomics to a comparative analysis of knockout mutants for 32 putative Synechocystis TR-encoding genes (Zhu et al., 2015). The results showed significant functional diversity of TRs at the metabolic level in Synechocystis, as well as functional diversity of TRs based on their differential clustering patterns in relationship trees resulted from TR families and metabolomic clustering analysis. This study provided interesting information on the regulatory functions and evolution of TR genes in Synechocystis.
Materials and Methods
Bacterial Growth Conditions
Synechocystis sp. PCC 6803 was obtained from American Type Culture Collection (ATCC), and used as a wild type to construct single-gene knockout mutants of TR genes. A total of 32 knockout mutants of putative TR-coding genes were constructed, confirmed and described previously (Zhu et al., 2015). Briefly, for the gene target selected, three sets of primers were designed to amplify a linear DNA fragment containing the chloramphenicol resistance cassette (amplified from a plasmid pACYC184) with two flanking arms of DNA upstream and downstream of the targeted gene. The linear fused PCR amplicon was used directly for transformation into Synechocystis by natural transformation. The chloramphenicol resistant transformants were obtained and passed several times on fresh BG11 plates supplemented with 10 μg mL-1 chloramphenicol to achieve full chromosome segregation (confirmed by PCR). The mutants and the wild type were grown in the BG11 medium (pH 7.5) in 100-mL flasks each with 25 mL medium, the light intensity was approximately 50 μmol photons m-2s-1 and the illuminating incubator was 130 rpm, the temperature was controlled at 30°C (HNY-211B Illuminating Shaker, Honour, China). All mutants were first cultivated in BG11 culture with 10 μg/ml chloromycetin for 48 h and then inoculated into BG11 culture without chloromycetin. The growth was determined by cell density measured at OD630 on a UV-1750 spectrophotometer (Shimadzu, Japan) every 12 h. For each mutant, three biological replicates were established independently, and each sample was measured in triplicates (Zhu et al., 2015). To confirm the growth patterns, growth experiment of every knockout mutant was repeated at least three times independently, then the growth rates of all mutants were calculated (Supplementary Table S1). Only the growth rates between different RR mutants and wild type with p-value <0.005 by t-test were considered a significant growth difference.
LC-MS Based Metabolomics Analysis
Liquid chromatography-mass spectrometry based targeted metabolomics was performed according to the protocol described previously (Wang et al., 2014). All chemicals used for LC-MS metabolomics analysis were obtained from Sigma–Aldrich (Taufkirchen, Germany). For metabolomic analysis, the wild type and the mutant cells were collected at 48 and 72 h, respectively, and each sample was prepared with three biological replicates. Due to the large amount of cultivation needed to finish the comparative experiments of 32 TRs, the samples had to be cultivated, prepared, and analyzed in five batches. A separate cultivation and analysis of the wild type as control was conducted for every batch to minimize possible batch difference. Briefly, the cells were collected by centrifugation at 7500 × g for 8 min at 4°C (Eppendorf 5430R, Hamburg, Germany), quenched, and extracted rapidly with 900 μL of 80:20 MeOH/H2O (-80°C) and then frozen in liquid nitrogen. The samples were then frozen-thawed three times to release metabolites from the cells. The supernatant was collected after centrifugation at 15,000 × g for 5 min at -4°C and then stored at -80°C. The remaining cell pellets were re-suspended in 500 μL of 80:20 MeOH/H2O (-80°C), and then the above extraction process was repeated. The supernatant from the second extraction was pooled with that from the first extraction and stored at -80°C until the LC-MS analysis was conducted. LC-MS analysis was conducted on an Agilent 1260 series binary HPLC system (Agilent Technologies, Waldbronn, Germany) using a Synergi Hydro-RP (C18) 150 mm × 2.0 mm ID, 4-μm 80-Å particle column (Phenomenex, Torrance, CA, USA), coupled to an Agilent 6410 triple quadrupole mass analyzer equipped with an electrospray ionization (ESI) source. Data was acquired using Agilent Mass Hunter workstation LC/QQQ acquisition software (version B.04.01), and chromatographic peaks were subsequently integrated via Agilent Qualitative Analysis software (version B.04.00). A total of 24 metabolites were selected for LC-MS-based targeted metabolite analysis in this study. All data of metabolomic profiling was first normalized by the internal control and the cell numbers of the samples.
The 24 targeted metabolites include acetyl coenzyme A (AcCOA), adenosine 5′-diphosphate (ADP), adenosine-5′-diphosphoglucose (ADP-GCS), α-ketoglutaric acid (AKG), adenosine 5′-monophosphate (AMP), adenosine 5′-triphosphate (ATP), coenzyme A hydrate (CoA), dihydroxyacetone phosphate (DHAP), D-fructose 1,6-bisphosphate (FBP), D-fructose 6-phosphate (F6P), sodium fumarate dibasic (FUM), DL-glyceraldehyde 3-phosphate (GAP), D-glucose 6-phosphate (G6P), L-glutamic acid (GLU), α-nicotinamide adenine dinucleotide (NAD), reduced α-nicotinamide adenine dinucleotide (NADH), nicotinamide adenine dinucleotide phosphate (NADP), reduced nicotinamide adenine dinucleotide phosphate (NADPH), uridine 5′-diphosphoglucose (UDP-GCS), oxaloacetic acid (OXA), phosphor (enol)pyruvic acid (PEP), D-(-)-3-phosphoglyceric acid (3PG), D-ribose 5-phosphate (R5P), and D-ribulose1,5-bisphosphate (UDP-GCS), uridine 5′-diphosphoglucose (RiBP).
Statistical Analysis
The metabolomic profiles were further normalized by comparing relative values of the mutants to the wild type, and then log2 transformed. The data were subjected to Principal Component Analysis (PCA) using software SIMCA-P 11.5 (Laiakis et al., 2010). The PCA analysis is a statistical method to find outliers in the whole set of data. Samples with p-value < 0.05 by hotelling t2 statistic were considered significantly different. For Euclidean distance calculation, we used the dist function in R software after data normalization. Only the distances larger than the upper quartile could be considered as the most affected mutants. Hierarchical clustering analysis was conducted using a R software (Deu-Pons et al., 2014).
TR Family Analysis
Protein sequences of all the 32 TR genes were downloaded from NCBI1. To define potential TR families, we used BLAST software for homology identification, only those TR with 80% aligned coverage with E-value < 1e-20 were consider as same families.
Protein–Protein Interaction (PPI) Network Analysis
A protein–protein interaction (PPI) dataset of Synechocystis was downloaded from the STRING database (http://www.string-db.org/) (Jensen et al., 2009). STRING aggregates data and predictions stemming from a wide spectrum of cell types and environmental conditions, and aims to represent the union of all possible protein–protein links. In the STRING database, several types of evidence for the association, including genomic context, high-throughput experiments, conserved co-expression and previous biological knowledge were used to calculate a single combined score for each gene in the genome. In this study, only those experimentally validated were applied to construct the PPI network to cover potential protein-protein connections, and the notes of all the proteins in this study were renamed using gene IDs (Szklarczyk et al., 2011).
Results and Discussion
Comparative Growth Analysis of TR Mutants
Although bioinformatics analysis of TRs in Synechocystis based on the sequence similarity has been conducted previously (Huffman and Brennan, 2002; Los et al., 2010), their functional classification using experimental approaches is still insufficient. A library of single deletion mutants for 32 TR-encoding genes in Synechocystis was constructed and confirmed previously in our laboratory (Zhu et al., 2015), and the majority of them have not been functionally characterized. To seek more functional information for these Synechocystis TRs, we first measured differential growth of all TR mutants in normal BG11 medium in flask cultivation, in parallel with the wild type Synechocystis. While most of the mutants grew equally well as the wild type (Supplementary Figure S2), the comparative analysis showed that four TR mutants, Δslr0395, Δslr1871, Δslr0741, and Δsll1872, grew poorly in the BG11 medium when compared with the wild type (Figure 1 and Supplementary Table S1), suggesting that the function of these four TRs might be related to key metabolism necessary for normal growth in the BG11 autotrophic growth medium. Among them, deletion of slr0395 (ntcB) caused the most significant growth arrest, with approximately 40% of the growth compared to the wild type after cultivation of 72 h, while deletion of slr1871 (pirR) resulted in the least growth defect, with only 15% of the growth compared to the wild type after 72 h cultivation.
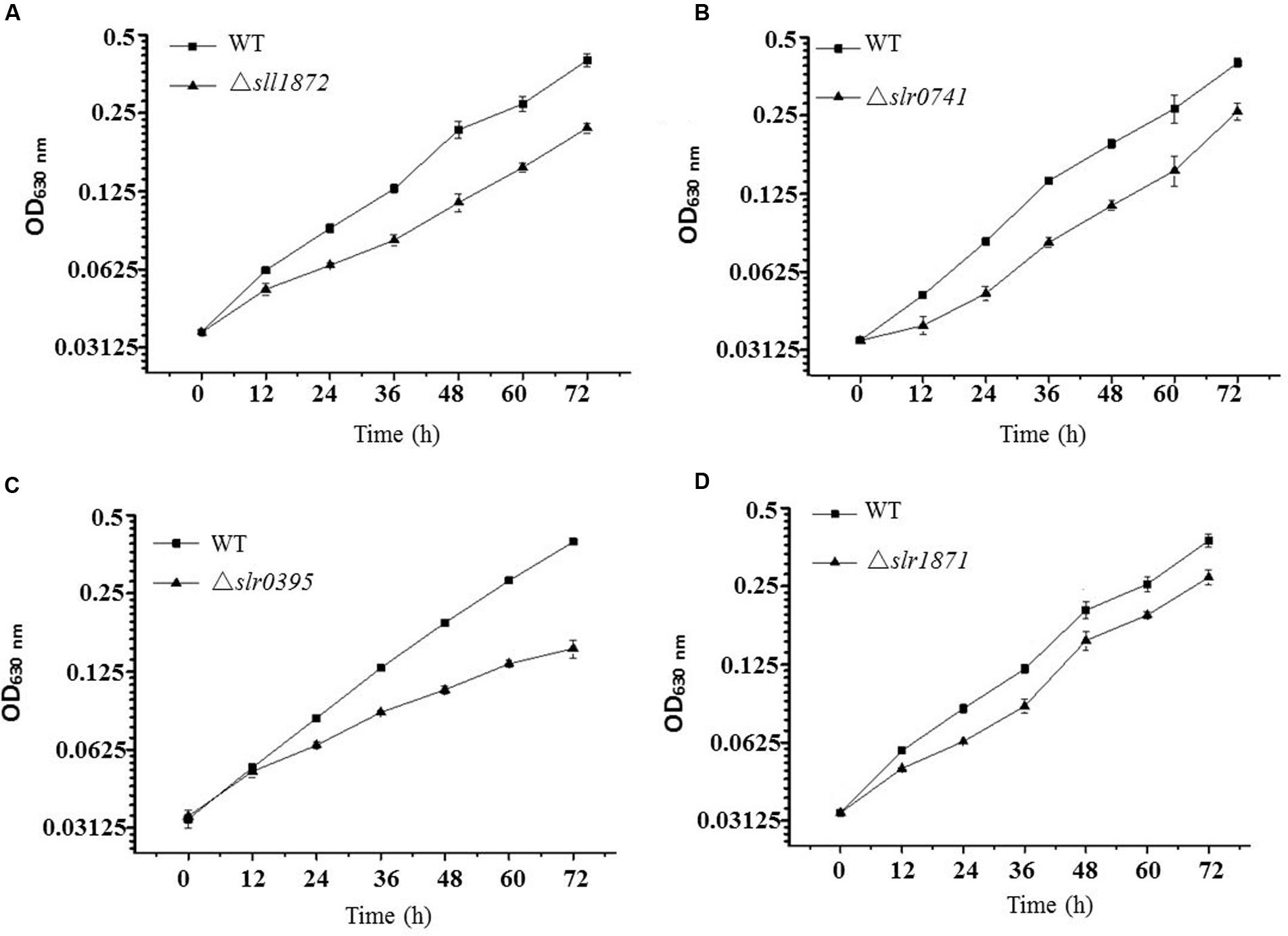
FIGURE 1. Growth time courses of the wild type and the mutants in BG11 media. (A) The wild type and the Δsll1872 mutant. The block represents the wild type and the triangle represents the Δsll1872 mutant. (B) The wild type and the Δslr0741 mutant. The block represents the wild type and the triangle represents the Δslr0741 mutant. (C) The wild type and the Δslr0395 mutant. The block represents the wild type and the triangle represents the Δslr0395 mutant. (D) The wild type and the Δslr1871 mutant. The block represents the wild type and the triangle represents the Δslr1871 mutant.
Slr0395 has previously been annotated as nitrite-responsive transcriptional enhancer NtcB in Synechocystis, on the basis of the inability of the Δslr0395 mutant to rapidly accumulate the transcripts of the nitrate assimilation genes upon induction and to respond to nitrite. In the ntcB mutant, activities of the nitrate assimilation enzymes were 40 to 50% of the wild type level, and the cells grew on nitrate at a rate approximately threefold lower than that of the wild type (Aichi et al., 2001).
Slr1871 was previously annotated as PirR of a LysR family, whose encoding gene is located immediately upstream of pirAB encoding an ortholog of pirin in the divergent direction, and DNA microarray analysis indicated that PirR repressed expression of closely located ORFs, slr1870 and mutS (sll1772), in addition to pirAB and pirR itself (Hihara et al., 2004). Slr0741 was previously found to encode a negative regulator of the Pi regulon and its insertional inactivation in Synechocystis led to increase of the intracellular polyP level (Morohoshi et al., 2002); Slr0741 was also found involved in transduction of the phosphate-limitation signal in Synechocystis (Juntarajumnong et al., 2007), and it was also up-regulated upon ethanol stress as revealed by RNA-seq analysis (Wang et al., 2012). Currently no functional information is available for Sll1872.
Metabolomic Analysis Reveals Functional Diversity of TRs
Liquid chromatography-mass spectrometry-based metabolomics analysis has been recently used to investigate cyanobacterial metabolism due to its advantages toward chemically unstable metabolites, such as the hydrolytically unstable nucleotides (i.e., ATP, GTP, cAMP, and PEP) and the redox active nucleotides (i.e., NADPH, NADP) whose determination could be important in deciphering metabolic responses to genetic or physiological changes. Using a protocol optimized in our previous studies (Wang et al., 2014), a LC-MS-based comparative metabolomics analysis was conducted on all TR mutants and the wild type, with 24 key metabolites involved in central carbon metabolism, cellular energy charge and redox monitored in all samples at 48 and 72 h. The cell samples of 32 TR mutants and the wild type used for LC-MS-based metabolomic analysis were cultivated in BG11 media under autotrophic growth condition and collected at both 48 and 72 h, which were corresponding to earlier and latter exponential phases of cell growth. Each sample was prepared with three biological replicates. As for some, metabolite levels might change during the 8 min centrifugation procedure, a faster centrifugation method may be considered. To reduce the sampling time and maintain the metabolites as much as possible, a higher rotation rate and a lower temperature should be considered. However, with higher rotation rate, more severe damages may occur to cells. A protection agent may be considered in the future. When the rotation rate was higher than 7500 × g, the cells of Synechocystis would be easily broken, leading to less metabolites being preserved.
Several patterns were observed in the PCA plots of the metabolomic data (Figure 2): (i) As a large number of cultivation was needed to finish the comparative experiments of 32 TRs, the samples had to be cultivated, prepared and analyzed in five batches. To minimize possible batch difference, a separate cultivation and analysis of the wild type as control was conducted for every batch. In the PCA plots, the big dots of five different colors representing the controls of five batches were found clustered together after data normalization, demonstrating the systematic errors resulting from the experimental design and different cultivation batches were not significant (Figure 2); (ii) metabolic profiles of TR mutants were in general well separated at both time points, demonstrating that the LC-MS-based methodology we utilized in this study is sensitive enough to investigate possible differences between controls and all the TR mutants; (iii) except for the four mutants that were grew poorer than the wild type, almost no growth difference was observed between the remaining 28 TR mutants and the wild type when cultivated in the BG11 medium; however, PCA analysis of metabolic profiles showed that these TR mutants were well separated from the wild type in the plots, suggesting that the deletion of these TR-encoding genes has caused significant changes to the cells at the metabolite level. At 48 h, seven TR mutants with the most significant metabolic changes from the wild type control were Δslr1871, Δsll1392, Δsll0690, Δslr1666, Δsll1872, Δsll0782, and Δsll0792 (Figure 2A, with two components proportions of 15.94 and 11.23%); while at 72 h, six TR mutants, Δslr1937, Δsll1594, Δslr1529, Δsll0792, Δslr1871, and Δslr0449 (Figure 2B, with two components proportions of 14.70 and 13.10%), displayed the most significant metabolic changes, suggesting a significant functional diversity of TRs in Synechocystis, as revealed by the metabolic profiling of selected metabolites related to central carbohydrate metabolism; (iv) only two TR mutants, Δsll0792 andΔslr1871, were found significantly regulated at both 48 and 72 h at the metabolite level, suggesting that time- or growth phase-dependent regulation may be involved for most of the responsive TRs; (v) a close examination of the mutant Δslr1871 showed that almost all the metabolites involved in central carbohydrate metabolism were down-regulated when compared with the wild type, consistent with the previous results that the slr1871 (pirR) gene had a reduced transcript level during a light-limited linear growth when compared to the exponential growth (Foster et al., 2007). Although it has been reported that the sll0792 gene encodes ZiaR, a Zn2+-responsive repressor of ziaA encoding a polypeptide with sequence features of heavy metal transporting P-type ATPases in Synechocystis (Thelwell et al., 1998), its regulatory function on cellar metabolism has not yet been established.
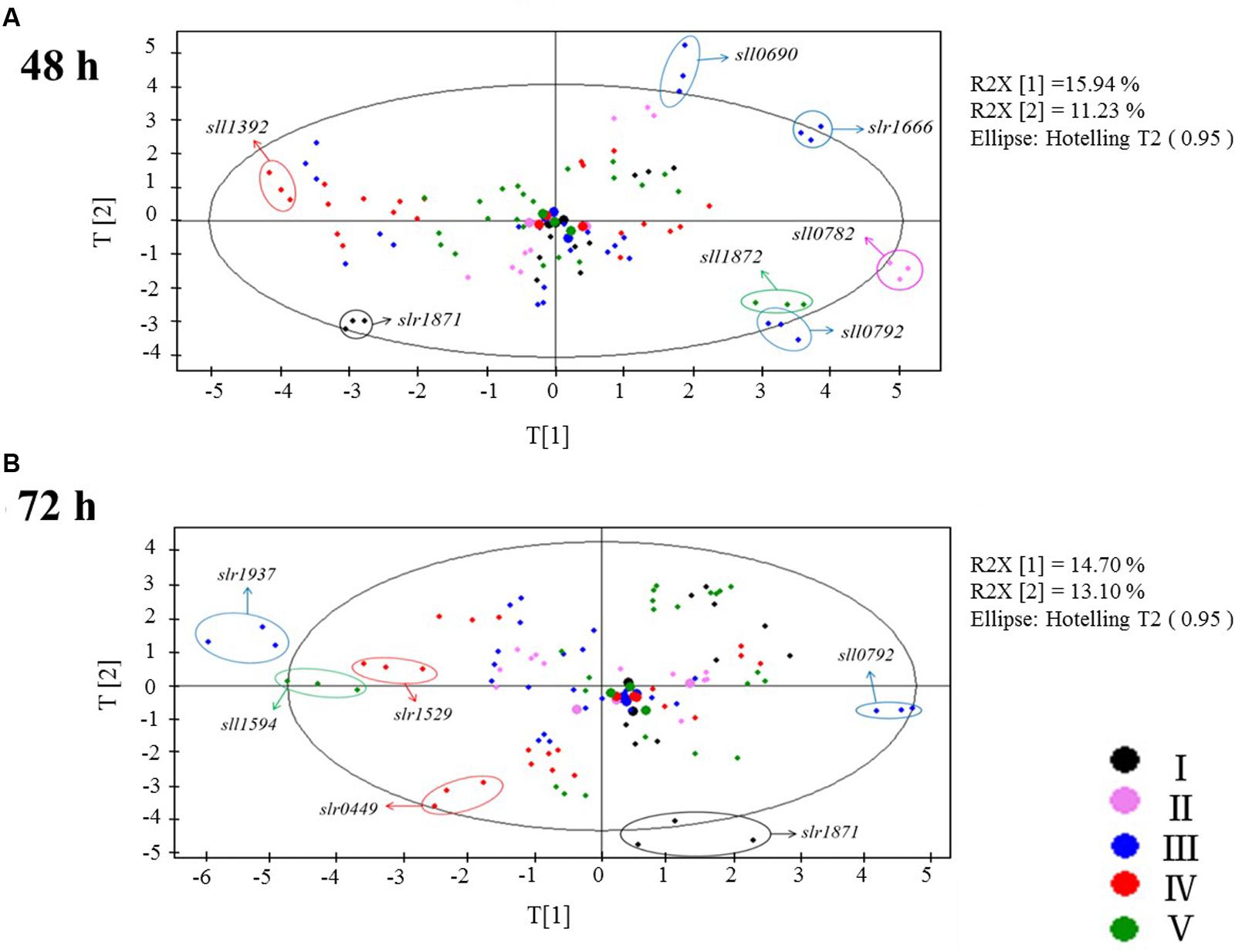
FIGURE 2. Targeted LC-MS metabolomic analysis. (A) PCA plots of the LC-MS metabolomic profiles at 48 h. (B) PCA plots of the LC-MS metabolomic profiles at 72 h. I, II, III, IV, and V are experiments conducted in five separate times with different colors represent for each batch: black, purple, blue, red, green were for batch I, II, III, IV, V separately.
To confirm the analysis with PCA, another approach, the Euclidean distances calculated based on the different metabolite profiles between each mutant and its control (the wild type), were also determined. In Supplementary Figure S1, the TR mutants were ordered based on the degree of metabolic changes when compared with the wild type in descending order. The analysis was conducted separately with metabolomic profiling data of two time points (i.e., 48 and 72 h), and showed that the top changed mutants were Δsll0690, Δslr1871, Δsll1670, Δslr1666, Δslr1245, Δsll1957, Δsll1594, and Δsll1872 at 48 h; Δslr0449, Δslr1871, Δssl0564, Δsll1937, Δslr1666, Δslr0115, Δslr0724, and Δsll0690 at 72 h, respectively. The results were consistent with those of the PCA analysis.
Metabolomic Basis for the Differential Growth in Four TR Mutants
Comparative growth analysis showed that four TR mutants were grown poorly in BG11 medium when compared with the wild type. A detailed analysis of the metabolite abundance of the 24 metabolites in these mutants was then conducted (Figure 3). Slr0395 is involved in regulation of nitrate assimilation gene (ntcB) (Burnap et al., 2015), so it is expected that the deletion of the slr0395 (ntcB) gene would decrease nitrogen metabolism. Accordingly, the metabolomics analysis showed Glu was up-regulated by 24.2, and 11.7% at 48 h and 72 h, respectively. In addition, abundance of CoA in the Δslr0395 was found increased.
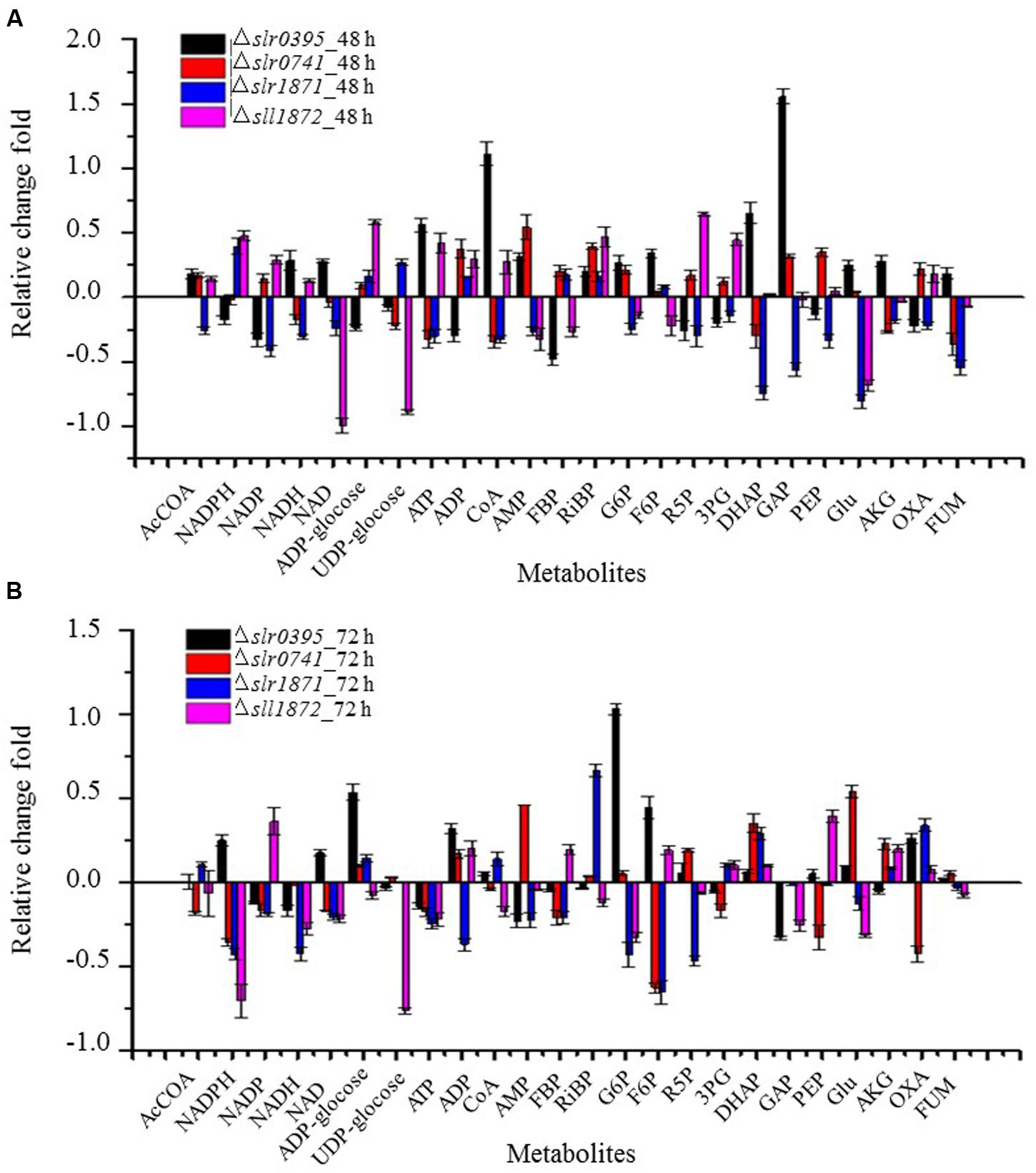
FIGURE 3. A detailed analysis of the metabolite abundance in four mutants with differential growth. (A) calculated based on the metabolite profiles of 48 h. (B) calculated based on the metabolite profiles of 72 h.
Slr0395 and Slr1871 are LysR-type transcriptional regulator protein (LTTRs) that have been found important for regulation of the carbon concentration mechanism (CCM) in cyanobacteria (Daley et al., 2012). In Calvin–Benson cycle, F6P and G3P were converted to GAP, R5P, and then Ru5P and RiBP for CO2 fixation (Wang et al., 2011). The metabolomic analysis showed that metabolite RiBP, F6P, GAP, and R5P associated with RiBP were down-regulated in the Δslr0395 and Δslr1871 mutants (Figure 3), which could be responsible for the slow growth in the mutants.
Regulatory function of gene sll1872 (lytR) has not been determined previously. According to our metabolomic analysis, several metabolites related to energy metabolism, including NADP, NADH, NAD and ATP were all down-regulated at both sampling time points, suggesting that the absence of the sll1872 (lytR) may negatively affect the energy metabolism and then reduced the growth of Δsll1872 mutant (Figure 3).
Interestingly, although the growth of Δslr0741 was clearly slower than the wild type, the metabolomic analysis showed no obvious difference at the metabolite level between the Δslr0741 mutants and the wild type, implying that its regulatory function may be not directly related to the central carbohydrate metabolism.
Functional Conservation of TRs
The finding that a range of metabolic changes occurred in single-deletion TR mutants led to several immediate questions. First, whether the TR mutants with similar metabolic profiles have a close relationship on the evolutionary tree for their encoding genes? Second, whether the TR-encoding genes with a close evolutionary relationship have similar metabolic changes to the gene deletion? Answers to these questions could provide clues to possible function, evolution and origin of the TR genes. To seek answers to the questions, a gene families’ analysis was conducted using full protein sequences of 32 TR genes. The homology analysis showed that 32 TR genes were classified into several different families, indicating different origin during the evolution of TR genes (Figure 4 and Supplementary Table S3). Meanwhile, several pairs of TRs were found in the same gene family, suggesting possible events of gene duplication in recent evolutionary courses. Interestingly, while comparing the trees resulted from the hierarchical clustering analysis of metabolomic data and the gene families, we found that several pairs of TRs were clustered together in same gene family and the relationship tree generated using metabolomic profiles, suggesting possible functional conservation of the genes during genome evolution (Figure 4). At 48 h, one pair of TR genes, slr1489 (pchR) and sll1408 (pcrR), with similar function and evolution was identified; however, when metabolic profiles of 72 h were used, five pairs of TRs were identified. The pairs were slr0895 (prqR) and sll1286. slr1489 (pchR) and sll1408 (pcrR); sll1712 and sll1670 (hrcA); slr0115 (rpaA) and slr0449 (dnr); slr1871 (pirR) and slr1245. Only one pair of TRs, slr1489 (pchR) and sll1408 (pcrR), was identified when using metabolomic profiling data of both time points, suggesting a very conserved role that they might be playing. The difference between 48 and 72 h was probably due to the phase-dependent regulation of TRs in Synechocystis, which has been commonly reported in various microbes (Zhu et al., 2015).
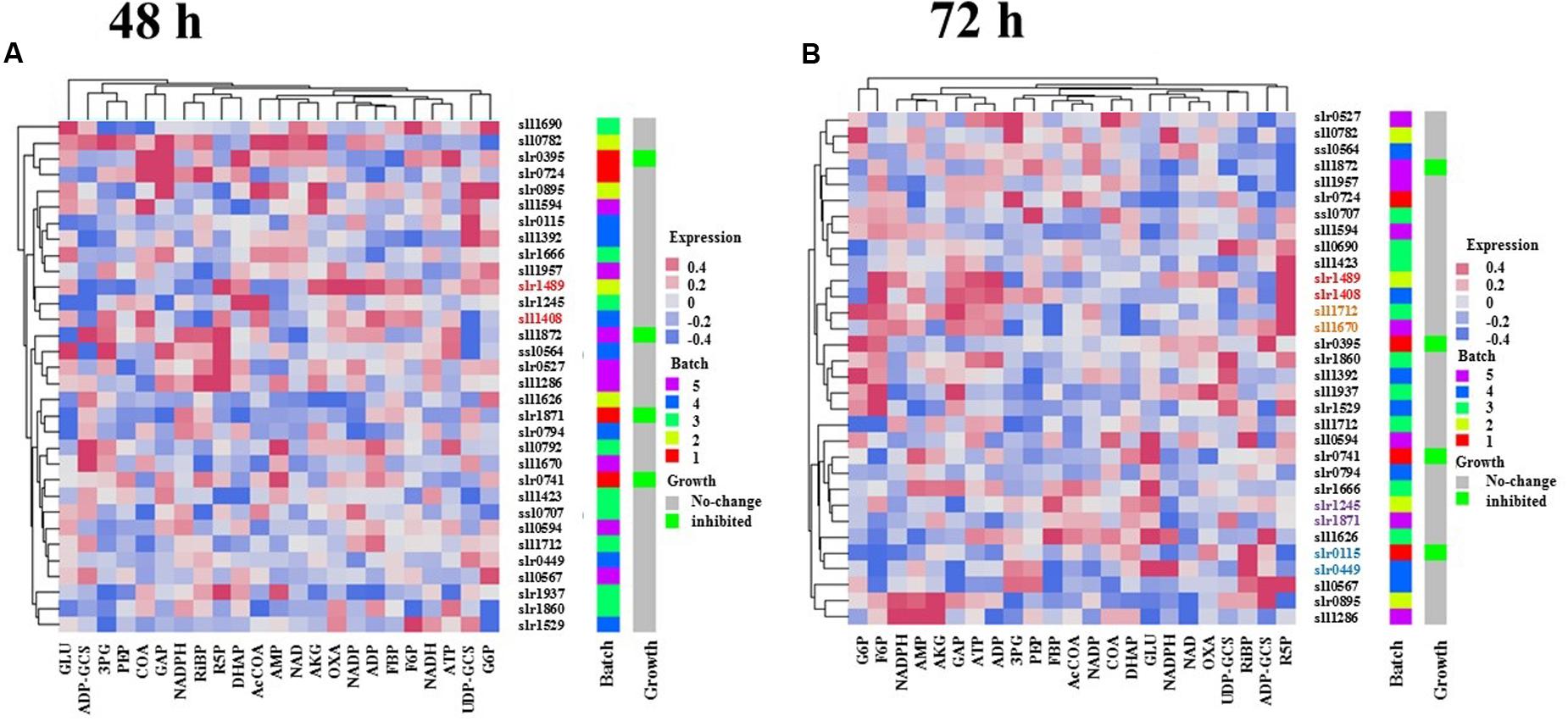
FIGURE 4. Comparison of hierarchical clustering trees of metabolomic data. (A) Hierarchical clustering tree based on the metabolite profiles of 48 h. (B) Hierarchical clustering tree based on the metabolite profiles of 72 h. The same colored text for some of the gene names represents they were clustered in metabolic level and shared common target proteins.
Meanwhile, the results also showed a total of 17 pairs of TRs that were clustered together in the metabolomic trees but did not locate in the same gene family (Figure 4). Although experimental measurements of more metabolites are still necessary, the preliminary analysis pointed to the possibility of functional convergence of TR genes during the genome evolution, as the results showed that deletion of TR genes of different evolutionary origins caused similar metabolic responses in the mutant cells. For example, the slr0395 (ntcB) and slr0724 (sohA) genes were clustered together in the tree resulted from metabolic profiles of 48 h, deletion of these two TR genes caused CoA and GAP were down-regulation while ADP and ADP-glucose were up-regulated, although they belong to different gene families.
Functional Inference of TRs
Experimental and computational data from genome-scale PPI analysis has contributed significantly to the understanding of the gene function (Marcotte et al., 1999; Ikeuchi and Tabata, 2001; Sato et al., 2007). In this study, an attempt was also made to apply PPI network of Synechocystis to determine possible gene targets of the five pairs of TRs identified above with similar clustering patterns in metabolomic profiles and matching gene family membership. As TRs of each pair have similar evolutionary and metabolomics patterns, it was expected that they might function through the same targets. Based on this hypothesis, we then implemented a strategy to first determine regulatory targets of each TR using PPI network analysis, and then identify the common target genes for every pair of TRs. The analysis allowed inference of possible regulatory gene targets for four out of five pairs of TRs, although no common target gene was identified for the slr1871 (pirR) and slr1245 pair (Supplementary Table S2).
(i) sll1408 (pcrR) and slr1489 (pchR): sll1408 is a gene encoding a regulator protein PcrR and repressed by cold sensor Hik33 in Synechocystis (Suzuki et al., 2001). A previous study found that sll1408 and slr1489 were regulated by iron (and cadmium) and hydrogen peroxide stresses under control of the Slr1738 regulator (Houot et al., 2007). The two mutants did not show growth difference. Our analysis showed that Sll1408 and Slr1489 had four common possible target proteins: Slr0707, Slr0664, Sll0043, and Slr0594, among which Slr0707 is DNA polymerase I involved in the biological process of DNA replication (Sato et al., 2003), slr0664 encodes a toxin–antitoxin (TA) system belonging to rel family (Ning et al., 2011), sll0043 encodes a protein related to phototaxis (Shin et al., 2008), and slr0594 was a putative membrane-spanning component of ABC transporters (Wipat et al., 1998), respectively.
(ii) slr0895 (prqR) and sll1286: Previous studies showed that the gene sll1286 was down-regulated by acid stress and sorbitol stress (Ohta et al., 2005; Shapiguzov et al., 2005), while slr0895 (prqR) was found to be an auto-repressor regulating the adaptive responses to the oxidative stress (Cheng and He, 2014). In addition, sll1286 and slr0895 (prqR) were both encoding regulators of TetR family. As one TetR family regulator protein PfsR has been found as a key regulator of iron homeostasis in Synechocystis, and the PrqR (Slr0895) was an auto-repressor regulating the adaptive responses to oxidative stress (Cheng and He, 2014). No growth difference was found between these two mutants. Our analysis showed that Slr0895 and Sll1286 had two common possible target proteins: Slr1705 and Sll0782. slr1705 (aspA) encodes aspartoacylase (Osanai et al., 2014), while sll0782 is annotated as a helix–turn–helix DNA binding motif without any known function (Zhang et al., 1998). Based on COG and KEGG annotation, Slr1705 may be participating in amino acid transport and metabolism, such as alanine, aspartate and glutamate, which is consistent with the down-regulation of Glu at 72 h in the deletion mutants of slr0895 (prqR) and sll1286.
(iii) sll1670 (hrcA) and sll1712. sll1670 gene encodes an ortholog of HrcA, a negative regulator of heat stress genes (Zorina et al., 2011). Sll1712 is a DNA binding protein (Chen et al., 2014) that was positively regulated by cadmium (Houot et al., 2007). The two mutants did not show growth difference. Our analysis showed that Sll1670 and Sll1712 had two common target proteins: Slr0701 and Sll0794. Slr0701 is a mercuric resistance operon regulator (Hirosawa et al., 1997), sll0794 (corR) encoding a sensor gene involved in Ni2+, Co2+, and Zn2+ sensing and tolerance (García-Domínguez et al., 2000; Mehta et al., 2014), and tolerance to ethanol (Huertas et al., 2014).
(iv) slr0115 (rpaA) and slr0449 (dnr): slr0449 (dnr) encodes a TR belonging to the Crp/Fnr family, which has been found regulated by AbrB2 (Leplat et al., 2013). Slr0115 is related to energy transfer from phycobilisomes to photosystems (Hanke et al., 2011), and deletion of slr0115 (rpaA) resulted in increased efficiency of energy transfer from phycobilisomes to photosystem II relative to photosystem I (Ashby and Mullineaux, 1999). No growth difference was found between these two mutants. Our analysis showed that Slr0115 and Slr0449 had four common target proteins: Sll1196, Sll0745, Slr0884, and Sll1342, among which sll1196 (pfkA) and sll0745 (pfkA) encode two phosphofructokinases (Osanai et al., 2005), and participate in carbohydrate transport and metabolism, slr0884 (gap1) and sll1196 (pfkA) showed similar enhancement of expression through overexpression of rre37 (sll1330) (Okada et al., 2015), while sll1342 (gap2) encodes glyceraldehyde-3-phosphate dehydrogenase whose pathway involves in F6P, GAP, and R5P (Rowland et al., 2011; Lee et al., 2015). As a phosphofructokinase encoding gene, the expression of sll1196 (pfkA) could affect the accumulation of F6P (Tabei et al., 2007), while the components GAP and FBP that were related to F6P phosphorylation were also changed.
In this study, 32 knockout mutants for putative TR-encoding genes of Synechocystis were constructed and comparatively analyzed via LC-MS-based metabolomics. Four mutants, sll1872 (lytR). slr0741 (phoU). slr0395 (ntcB), and slr1871 (pirR), showed differential growth patterns in BG11 medium when compared with the wild type. In the remaining TR mutants that did not show growth difference compared with the wild type, metabolomic profiling showed that they were clearly different at the metabolite level, suggesting significant functional diversity of TRs in Synechocystis. Finally, protein-protein interaction network analysis predicted possible regulatory targets of TRs.
Author Contributions
LC and WZ conceived and designed the study. MS and XZ performed the experiments. MS, XZ, GP, LC, and WZ analyzed the data and wrote the manuscript. All authors read and approved the manuscript.
Funding
The research was supported by grants from the Natural Science Foundation of China (No. 31470217 and No. 21621004), National Basic Research Program of China (National “973” program, project No. 2014CB745101), and the Tianjin Municipal Science and Technology Commission (No. 15JCZDJC32500).
Conflict of Interest Statement
The authors declare that the research was conducted in the absence of any commercial or financial relationships that could be construed as a potential conflict of interest.
Acknowledgment
The authors would also like to thank Mingyang Zhang and Siqiang Huang of our laboratory for their helps with constrution ofthe TR mutants.
Supplementary Material
The Supplementary Material for this article can be found online at: http://journal.frontiersin.org/article/10.3389/fmicb.2017.00280/full#supplementary-material
FIGURE S1 | Euclidean distances between each mutant and its control. (A) Calculated based on the metabolite profiles of 48 h; (B) Calculated based on the metabolite profiles of 72 h.
FIGURE S2 | Growth curves of 28 mutants without different growth with the wild type. The black block represents for the wild type and the red dot represents for corresponding mutant.
Footnotes
References
Aichi, M., Takatani, N., and Omata, T. (2001). Role of NtcB in activation of nitrate assimilation genes in the cyanobacterium Synechocystis sp. strain PCC 6803. J. Bacteriol. 183, 5840–5847. doi: 10.1128/JB.183.20.5840-5847.2001
Ashby, M. K., and Mullineaux, C. W. (1999). Cyanobacterial ycf27 gene products regulate energy transfer from phycobilisomes to photosystems I and II. FEMS Microbiol. Lett. 181, 253–260. doi: 10.1111/j.1574-6968.1999.tb08852.x
Atsumi, S., Higashide, W., and Liao, J. C. (2009). Direct photosynthetic recycling of carbon dioxide to isobutyraldehyde. Nat. Biotechnol. 27, 1177–1180. doi: 10.1038/nbt.1586
Babu, M. M., Luscombe, N. M., Aravind, L., Gerstein, M., and Teichmann, S. A. (2004). Structure and evolution of transcriptional regulatory networks. Curr. Opin. Struct. Biol. 14, 283–291. doi: 10.1016/j.sbi.2004.05.004
Badger, M. R., Price, G. D., Long, B. M., and Woodger, F. J. (2006). The environmental plasticity and ecological genomics of the cyanobacterial CO2 concentrating mechanism. J. Exp. Bot. 57, 249–265. doi: 10.1093/jxb/eri286
Burnap, R. L., Hagemann, M., and Kaplan, A. (2015). Regulation of CO2 concentrating mechanism in cyanobacteria. Life (Basel) 5, 348–371. doi: 10.3390/life5010348
Chen, L., Zhu, Y., Song, Z., Wang, J., and Zhang, W. (2014). An orphan response regulator Sll0649 involved in cadmium tolerance and metal homeostasis in photosynthetic Synechocystis sp. PCC 6803. J. Proteom. 103, 87–102. doi: 10.1016/j.jprot.2014.03.029
Cheng, D., and He, Q. (2014). PfsR is a key regulator of iron homeostasis in Synechocystis PCC 6803. PLoS ONE 9:e101743. doi: 10.1371/journal.pone.0101743
Daley, S. M., Kappell, A. D., Carrick, M. J., and Burnap, R. L. (2012). Regulation of the cyanobacterial CO2-concentrating mechanism involves internal sensing of NADP+ and α-ketogutarate levels by transcription factor CcmR. PLoS ONE 7:e41286. doi: 10.1371/journal.pone.0041286
Deu-Pons, J., Schroeder, M. P., and Lopez-Bigas, N. (2014). jHeatmap: an interactive heatmap viewer for the web. Bioinformatics 30, 1757–1758. doi: 10.1093/bioinformatics/btu094
Foster, J. S., Singh, A. K., Rothschild, L. J., and Sherman, L. A. (2007). Growth-phase dependent differential gene expression in Synechocystis sp. strain PCC 6803 and regulation by a group 2 sigma factor. Arch. Microbiol. 187, 265–279. doi: 10.1007/s00203-006-0193-6
García-Domínguez, M., Lopez-Maury, L., Florencio, F. J., and Reyes, J. C. (2000). A gene cluster involved in metal homeostasis in the cyanobacterium Synechocystis sp. strain PCC 6803. J. Bacteriol. 182, 1507–1514.
Hanke, G. T., Satomi, Y., Shinmura, K., Takao, T., and Hase, T. (2011). A screen for potential ferredoxin electron transfer partners uncovers new, redox dependent interactions. Biochim. Biophys. Acta 1814, 366–374. doi: 10.1016/j.bbapap.2010.09.011
Hihara, Y., Muramatsu, M., Nakamura, K., and Sonoike, K. (2004). A cyanobacterial gene encoding an ortholog of Pirin is induced under stress conditions. FEBS Lett. 574, 101–105. doi: 10.1016/j.febslet.2004.06.102
Hirosawa, M., Isono, K., Hayes, W. S., and Borodovsky, M. (1997). Gene identification and classification in the Synechocystis genomic sequence by recursive gene mark analysis. DNA Seq. 8, 17–29. doi: 10.3109/10425179709020881
Houot, L., Floutier, M., Marteyn, B., Michaut, M., Picciocchi, A., Legrain, P., et al. (2007). Cadmium triggers an integrated reprogramming of the metabolism of Synechocystis PCC6803, under the control of the Slr1738 regulator. BMC Genomics 8:350. doi: 10.1186/1471-2164-8-350
Huertas, M. J., Lopez-Maury, L., Giner-Lamia, J., Sanchez-Riego, A. M., and Florencio, F. J. (2014). Metals in cyanobacteria: analysis of the copper, nickel, cobalt and arsenic homeostasis mechanisms. Life 4, 865–886. doi: 10.3390/life4040865
Huffman, J. L., and Brennan, R. G. (2002). Prokaryotic transcription regulators: more than just the helix-turn-helix motif. Curr. Opin. Struct. Biol. 12, 98–106. doi: 10.1016/S0959-440X(02)00295-6
Ikeuchi, M., and Tabata, S. (2001). Synechocystis sp. PCC 6803—a useful tool in the study of the genetics of cyanobacteria. Photosynth. Res. 70, 73–83. doi: 10.1023/A:1013887908680
Jensen, L. J., Kuhn, M., Stark, M., Chaffron, S., Creevey, C., Muller, J., et al. (2009). STRING 8–a global view on proteins and their functional interactions in 630 organisms. Nucleic Acids Res. 37, D412–D416. doi: 10.1093/nar/gkn760
Juntarajumnong, W., Hirani, T. A., Simpson, J. M., Incharoensakdi, A., and Eaton-Rye, J. J. (2007). Phosphate sensing in Synechocystis sp. PCC 6803: SphU and the SphS-SphR two-component regulatory system. Arch. Microbiol. 188, 389–402. doi: 10.1007/s00203-007-0259-0
Kaneko, T., Nakamura, Y., Sasamoto, S., Watanabe, A., Kohara, M., Matsumoto, M., et al. (2003). Structural analysis of four large plasmids harboring in a unicellular cyanobacterium, Synechocystis sp. PCC 6803. DNA Res. 10, 221–228.
Laiakis, E. C., Morris, G. A., Fornace, A. J. Jr., and Howie, S. R. (2010). Metabolomic analysis in severe childhood pneumonia in the Gambia, West Africa: findings from a pilot study. PLoS ONE 5:e12655. doi: 10.1371/journal.pone.0012655
Lee, D.-G., Kwon, J., Eom, C.-Y., Kang, Y.-M., Roh, S. W., Lee, K.-B., et al. (2015). Directed analysis of cyanobacterial membrane phosphoproteome using stained phosphoproteins and titanium-enriched phosphopeptides. J. Microbiol. 53, 279–287. doi: 10.1007/s12275-015-5021-8
Leplat, C., Champeimont, R., Saenkham, P., Cassier-Chauvat, C., Jean-Christophe, A., and Chauvat, F. (2013). Genome-wide transcriptome analysis of hydrogen production in the cyanobacterium Synechocystis: towards the identification of new players. Int. J. Hydrogen Energy 38, 1866–1872. doi: 10.1016/j.ijhydene.2012.11.118
Los, D. A., Zorina, A., Sinetova, M., Kryazhov, S., Mironov, K., and Zinchenko, V. V. (2010). Stress sensors and signal transducers in cyanobacteria. Sensors 10, 2386–2415. doi: 10.3390/s100302386
Marcotte, E. M., Pellegrini, M., Ng, H.-L., Rice, D. W., Yeates, T. O., and Eisenberg, D. (1999). Detecting protein function and protein-protein interactions from genome sequences. Science 285, 751–753. doi: 10.1126/science.285.5428.751
Mehta, A., Lopez-Maury, L., and Florencio, F. J. (2014). Proteomic pattern alterations of the cyanobacterium Synechocystis sp. PCC 6803 in response to cadmium, nickel and cobalt. J. Proteomics 102, 98–112. doi: 10.1016/j.jprot.2014.03.002
Michel, K. P., and Pistorius, E. K. (2004). Adaptation of the photosynthetic electron transport chain in cyanobacteria to iron deficiency: the function of IdiA and IsiA. Physiol. Plant. 120, 36–50. doi: 10.1111/j.0031-9317.2004.0229.x
Morohoshi, T., Maruo, T., Shirai, Y., Kato, J., Ikeda, T., Takiguchi, N., et al. (2002). Accumulation of inorganic polyphosphate in phoU mutants of Escherichia coli and Synechocystis sp. Strain PCC6803. Appl. Environ. Microbiol. 68, 4107–4210. doi: 10.1128/AEM.68.8.4107-4110.2002
Ning, D., Ye, S., Liu, B., and Chang, J. (2011). The proteolytic activation of the relNEs (ssr1114/slr0664) toxin–antitoxin system by both proteases Lons and ClpP2s/Xs of Synechocystis sp. PCC 6803. Curr. Microbiol. 63, 496–502. doi: 10.1007/s00284-011-0011-5
Niu, X., Zhu, Y., Pei, G., Wu, L., Chen, L., and Zhang, W. (2015). Elucidating butanol tolerance mediated by a response regulator Sll0039 in Synechocystis sp. PCC 6803 using a metabolomic approach. Appl. Microbiol. Biotechnol. 99, 1845–1857. doi: 10.1007/s00253-015-6374-y
Ohta, H., Shibata, Y., Haseyama, Y., Yoshino, Y., Suzuki, T., Kagasawa, T., et al. (2005). Identification of genes expressed in response to acid stress in Synechocystis sp. PCC 6803 using DNA microarrays. Photosynth. Res. 84, 225–230. doi: 10.1007/s11120-004-7761-x
Okada, K., Horii, E., Nagashima, Y., Mitsui, M., Matsuura, H., Fujiwara, S., et al. (2015). Genes for a series of proteins that are involved in glucose catabolism are upregulated by the Hik8-cascade in Synechocystis sp. PCC 6803. Planta 241, 1453–1462. doi: 10.1007/s00425-015-2270-z
Osanai, T., Kanesaki, Y., Nakano, T., Takahashi, H., Asayama, M., Shirai, M., et al. (2005). Positive regulation of sugar catabolic pathways in the cyanobacterium Synechocystis sp. PCC 6803 by the group 2 sigma factor sigE. J. Biol. Chem. 280, 30653–30659. doi: 10.1074/jbc.M505043200
Osanai, T., Oikawa, A., Iijima, H., Kuwahara, A., Asayama, M., Tanaka, K., et al. (2014). Metabolomic analysis reveals rewiring of Synechocystis sp. PCC 6803 primary metabolism by ntcA overexpression. Environ. Microbiol. 16, 3304–3317. doi: 10.1111/1462-2920.12554
Ren, Q., Shi, M., Chen, L., Wang, J., and Zhang, W. (2014). Integrated proteomic and metabolomic characterization of a novel two-component response regulator Slr1909 involved in acid tolerance in Synechocystis sp. PCC 6803. J. Proteomics 109, 76–89. doi: 10.1016/j.jprot.2014.06.021
Rowland, J. G., Simon, W. J., Prakash, J. S., and Slabas, A. R. (2011). Proteomics reveals a role for the RNA helicase crhR in the modulation of multiple metabolic pathways during cold acclimation of Synechocystis sp. PCC6803. J. Proteome Res. 10, 3674–3689. doi: 10.1021/pr200299t
Sato, N., Terasawa, K., Miyajima, K., and Kabeya, Y. (2003). Organization, developmental dynamics, and evolution of plastid nucleoids. Int. Rev. Cytol. 232, 217–262. doi: 10.1016/S0074-7696(03)32006-6
Sato, S., Shimoda, Y., Muraki, A., Kohara, M., Nakamura, Y., and Tabata, S. (2007). A large-scale protein–protein interaction analysis in Synechocystis sp. PCC6803. DNA Res. 14, 207–216. doi: 10.1093/dnares/dsm021
Shapiguzov, A., Lyukevich, A. A., Allakhverdiev, S. I., Sergeyenko, T. V., Suzuki, I., Murata, N., et al. (2005). Osmotic shrinkage of cells of Synechocystis sp. PCC 6803 by water efflux via aquaporins regulates osmostress-inducible gene expression. Microbiology 151(Pt 2), 447–455. doi: 10.1099/mic.0.27530-0
Shin, B.-J., Oh, J., Kang, S., Chung, Y.-H., Park, Y. M., Kim, Y. H., et al. (2008). Cyanobacterial hybrid kinase Sll0043 regulates phototaxis by suppressing pilin and twitching motility protein. J. Microbiol. 46, 300–308. doi: 10.1007/s12275-007-0212-6
Suzuki, I., Kanesaki, Y., Mikami, K., Kanehisa, M., and Murata, N. (2001). Cold-regulated genes under control of the cold sensor Hik33 in Synechocystis. Mol. Microbiol. 40, 235–244. doi: 10.1046/j.1365-2958.2001.02379.x
Szklarczyk, D., Franceschini, A., Kuhn, M., Simonovic, M., Roth, A., Minguez, P., et al. (2011). The STRING database in 2011: functional interaction networks of proteins, globally integrated and scored. Nucleic Acids Res. 39, D561–D568. doi: 10.1093/nar/gkq973
Tabei, Y., Okada, K., and Tsuzuki, M. (2007). Sll1330 controls the expression of glycolytic genes in Synechocystis sp. PCC 6803. Biochem. Biophys. Res. Commun. 355, 1045–1050. doi: 10.1016/j.bbrc.2007.02.065
Thelwell, C., Robinson, N. J., and Turner-Cavet, J. S. (1998). An SmtB-like repressor from Synechocystis PCC 6803 regulates a zinc exporter. Proc. Natl. Acad. Sci. U.S.A. 95, 10728–10733. doi: 10.1073/pnas.95.18.10728
Wang, D., Zhang, Y., Pohlmann, E. L., Li, J., and Roberts, G. P. (2011). The poor growth of Rhodospirillum rubrum mutants lacking RubisCO is due to the accumulation of ribulose-1, 5-bisphosphate. J. Bacteriol. 193, 3293–3303. doi: 10.1128/JB.00265-11
Wang, J., Chen, L., Huang, S., Liu, J., Ren, X., Tian, X., et al. (2012). RNA-seq based identification and mutant validation of gene targets related to ethanol resistance in cyanobacterial Synechocystis sp. PCC 6803. Biotechnol. Biofuels 5:89. doi: 10.1186/1754-6834-5-89
Wang, Y., Shi, M., Niu, X., Zhang, X., Gao, L., Chen, L., et al. (2014). Metabolomic basis of laboratory evolution of butanol tolerance in photosynthetic Synechocystis sp. PCC 6803. Microb. Cell Fact. 13, 151. doi: 10.1186/s12934-014-0151-y
Wipat, A., Brignell, S. C., Guy, B. J., Rose, M., Emmerson, P. T., and Harwood, C. R. (1998). The yvsA-yvqA (293-289) region of the Bacillus subtilis chromosome containing genes involved in metal ion uptake and a putative sigma factor. Microbiology 144, 1593–1600. doi: 10.1099/00221287-144-6-1593
Zhang, C.-C., Gonzalez, L., and Phalip, V. (1998). Survey, analysis and genetic organization of genes encoding eukaryotic-like signaling proteins on a cyanobacterial genome. Nucleic Acids Res. 26, 3619–3625. doi: 10.1093/nar/26.16.3619
Zhang, W., Li, F., and Nie, L. (2010). Integrating multiple ‘omics’ analysis for microbial biology: application and methodologies. Microbiology 156, 287–301. doi: 10.1099/mic.0.034793-0
Zhu, Y., Pei, G., Niu, X., Shi, M., Zhang, M., Chen, L., et al. (2015). Metabolomic analysis reveals functional overlapping of three signal transduction proteins in regulating ethanol tolerance in cyanobacterium Synechocystis sp. PCC 6803. Mol. Biosyst. 11, 770–782. doi: 10.1039/c4mb00651h
Keywords: function, metabolomics, Synechocystis, transcriptional regulators, LC-MS
Citation: Shi M, Zhang X, Pei G, Chen L and Zhang W (2017) Functional Diversity of Transcriptional Regulators in the Cyanobacterium Synechocystis sp. PCC 6803. Front. Microbiol. 8:280. doi: 10.3389/fmicb.2017.00280
Received: 14 December 2016; Accepted: 09 February 2017;
Published: 21 February 2017.
Edited by:
Diana Elizabeth Marco, Consejo Nacional de Investigaciones Cientificas y Tecnicas (CONICET), ArgentinaReviewed by:
Takashi Osanai, Meiji University, JapanPaul Hudson, Royal Institute of Technology, Sweden
Johannes Asplund-Samuelsson, Royal Institute of Technology, Sweden
Copyright © 2017 Shi, Zhang, Pei, Chen and Zhang. This is an open-access article distributed under the terms of the Creative Commons Attribution License (CC BY). The use, distribution or reproduction in other forums is permitted, provided the original author(s) or licensor are credited and that the original publication in this journal is cited, in accordance with accepted academic practice. No use, distribution or reproduction is permitted which does not comply with these terms.
*Correspondence: Lei Chen, bGNoZW5AdGp1LmVkdS5jbg==