- 1Institute for Integrative and Systems Biology, Université Laval, Québec City, QC, Canada
- 2McGill University, Montréal, QC, Canada
- 3National Microbiology Laboratory, Public Health Agency of Canada, Ottawa, ON, Canada
- 4Laboratoire de Santé Publique du Québec, Sainte-Anne-de-Bellevue, QC, Canada
- 5Université Laval, Québec City, QC, Canada
- 6Institute of Food and Agricultural Sciences, University of Florida, Gainesville, FL, United States
- 7Département de Microbiologie, Infectiologie et Immunologie, Université de Montréal, Montréal, QC, Canada
- 8Agriculture and Agri-Food Canada, Summerland, BC, Canada
- 9Génome Québec Innovation Center, Montréal, QC, Canada
- 10Food Safety Engineering, Faculty of Land and Food Systems, University of British Columbia, Vancouver, BC, Canada
- 11National Institute for Public Health and the Environment, Bilthoven, Netherlands
- 12Canadian Food Inspection Agency, Halifax, NS, Canada
- 13Bureau of Microbial Hazards, Health Canada, Ottawa, ON, Canada
- 14UC Davis Food Science and Technology, Davis, CA, United States
- 15UC Davis School of Veterinary Medicine, Davis, CA, United States
- 16Canadian Food Inspection Agency, Ottawa, ON, Canada
- 17Food Science, University of Guelph, Guelph, ON, Canada
- 18Department of Microbiology and Immunology, Faculty of Pharmacy, Mansoura University, Mansoura, Egypt
- 19Department of Microbiology and Immunology, Dalhousie University, Halifax, NS, Canada
- 20Department of Biological Sciences, University of Calgary, Calgary, AB, Canada
- 21Institute for Food Safety and Hygiene, University of Zurich, Zurich, Switzerland
- 22Biological and Chemical Sciences, Wilfrid Laurier University, Waterloo, ON, Canada
- 23Department of Food Science, Cornell University, Ithaca, NY, United States
- 24College of Veterinary Medicine, The Ohio State University, Columbus, OH, United States
The Salmonella Syst-OMICS consortium is sequencing 4,500 Salmonella genomes and building an analysis pipeline for the study of Salmonella genome evolution, antibiotic resistance and virulence genes. Metadata, including phenotypic as well as genomic data, for isolates of the collection are provided through the Salmonella Foodborne Syst-OMICS database (SalFoS), at https://salfos.ibis.ulaval.ca/. Here, we present our strategy and the analysis of the first 3,377 genomes. Our data will be used to draw potential links between strains found in fresh produce, humans, animals and the environment. The ultimate goals are to understand how Salmonella evolves over time, improve the accuracy of diagnostic methods, develop control methods in the field, and identify prognostic markers for evidence-based decisions in epidemiology and surveillance.
Importance of Foodborne Salmonella as a Model in Large-Scale Bacterial Genomics
Salmonella enterica is a foodborne bacterial pathogen having at least 2,600 serotypes (Gal-Mor et al., 2014)1 that contaminates a diversity of foods and is a leading cause of foodborne illnesses and mortality globally. In fact, there are an estimated 93.3 million cases of gastroenteritis due to non-typhoidal Salmonella infections each year, resulting in approximately 155,000 deaths (Majowicz et al., 2010). In Canada, non-typhoidal salmonellosis accounts for more than 88,000 cases of foodborne illness each year, and has among the highest incidence rate of any bacterial foodborne pathogen (Thomas et al., 2015). S. enterica is responsible for more than 50% of fresh produce-borne outbreaks, the highest number of foodborne outbreaks of any inspected food commodity in North America (Kozak et al., 2013). Because of its remarkable genomic diversity, Salmonella is found in complex environmental and ecological niches and survives in harsh environments for long periods (Podolak et al., 2010; Fatica and Schneider, 2011). Several research groups have identified relationships between some of the 2,557 S. enterica serotypes and specific foods, which suggests, that some food commodities act as reservoirs for particular serotypes (Kim, 2010; Jackson et al., 2013; Nuesch-Inderbinen et al., 2015).
Salmonella outbreaks are monitored with support from the PulseNet surveillance system in 86 countries2 (Ribot and Hise, 2016; Scharff et al., 2016). PulseNet Canada3 is a national surveillance system used to quickly identify and respond to foodborne disease outbreaks, centralized at the National Microbiology Laboratory in Winnipeg, MB, and working in close collaboration with a network of federal and provincial public health laboratories and epidemiologists. Still, despite the availability of thousands of sequenced genomes, knowledge of genome evolution integrated with transmission and epidemiology is limited for produce-related outbreaks.
Studies of S. enterica population structure in humans, animals, food and the environment are central to understand the biodiversity, evolution, ecology and epidemiology of this pathogen. However, studies describing the genetic structure of Salmonella populations are commonly based on isolates drawn overwhelmingly from clinical collections (Hoffmann et al., 2014). This approach has resulted in a limited view of Salmonella’s evolutionary history (D’costa et al., 2006; Perry and Wright, 2014). In Salmonella as in many other bacterial pathogens, there is limited knowledge on how genome content, rearrangements and the complement of genes including those acquired by horizontal gene transfer (HGT) contribute to strain-specific phenotypes, including virulence (Casadevall, 2017). Various studies have sought to resolve the population structure of Salmonella using complementary subtyping methods including pulsed-field gel electrophoresis (PFGE), multiple loci VNTR analysis (MLVA), 7-gene housekeeping schemes, whole-genome multi-locus sequence typing (wgMLST) profiles, pan- and core genome studies, and CRISPR analysis to define molecular signatures, pathogen subtypes and the potential for pathogenicity (Shariat and Dudley, 2014; Rouli et al., 2015; Liu et al., 2016). Next-generation sequencing (NGS) coupled with whole-genome comparison is well-positioned to become the gold standard subtyping method, as it offers previously unmatched resolution for phylogenetic analysis and rapid subtyping during investigation of food contamination and outbreaks (Ashton et al., 2016; Bekal et al., 2016).
The Syst-OMICS Strategy
The application of genomics to infectious pathogens via WGS is transforming the practice of Salmonella diagnostics, epidemiology and surveillance. Genomic data are increasingly used to understand infectious disease epidemiology (Didelot et al., 2017). With rapidly falling costs and turnaround time, microbial WGS and analysis is becoming a viable strategy to identify the geographic origin of bacterial pathogens (Weedmark et al., 2015; Hoffmann et al., 2016). The objective of the Canadian-based international Syst-OMICS consortium is to sequence a minimum of 4,500 genomes, include the data in the Salmonella Foodborne Syst-OMICS database (SalFoS) at https://SalFoS.ibis.ulaval.ca/, share this information plus available metadata with Canadian federal and provincial regulators and the food industry, and develop pipelines to study these genomes. Genomics data will support molecular epidemiology and source attribution of outbreaks and has the potential for future genotypic antimicrobial susceptibility testing, as well as the identification of novel therapeutic targets and prognostic markers. Moreover, the large-scale genomics and evolutionary biology tools developed may lead to new strategies for countering not only Salmonella infections, but other pathogens as well (Little et al., 2012).
The Syst-OMICS project is based upon a systems approach (flowchart and screening method available in Supplementary File 1). First, the genome diversity of 4,500 isolates will be assessed using high-quality WGS, assembly, annotation and phylogeny. This data will be used for in silico serotyping (Yoshida et al., 2016), as well as analysis of virulence (Chen et al., 2012), antibiotic resistance (Jia et al., 2016) and mobilome gene content (Lanza et al., 2014). Based on this genomic data, a funnel-type model will be applied such that 300 isolates will be selected for in vitro high-throughput screening (HTS) in cell lines to determine attachment, adhesion, invasion and replication of each isolate (protocol adapted to 96-well plates from Forest et al., 2007). From the results, isolates will be categorized as being of high, medium, or low virulence. A limited number of those isolates will then be selected for further screening in vivo using a mouse model (Roy et al., 2007) and in vitro using gastrointestinal fermenter models (Kheadr et al., 2010; Le Blay et al., 2012). These data will identify isolates to represent the different levels of virulence that will be used to develop novel diagnostic and control tools. We propose to enhance food safety and lower the economic burden of salmonellosis through a farm-to-table systematic approach to control Salmonella, with a focus on new control methods in agricultural production, more specific diagnostics and improved bacterial subtyping methods to support investigation of foodborne outbreaks, as no single intervention is likely to produce meaningful and lasting effects.
The Salmonella Foodborne Syst-OMICS Database (SalFoS)
Salmonella Foodborne Syst-OMICS database is an online web application that relies on a Mysql 5 database. It was designed not only to store data for the Salmonella strain collection but also to provide access to each isolate’s phenotypic, genomic, virulence, serotype, mobilome and epidemiological data. Different levels of access may be granted, but data modification is strictly reserved to the curators. It includes isolate identification, host, provider, date of isolation, geographical origin, phenotypic data, DNA extraction details, NGS information and genome assembly statistics. SalFoS currently contains NGS data and unpublished draft genomes from produce, human, animal and environmental isolates. Upon publication, draft genomes of SalFoS will become available at NCBI and EnteroBase4.
The SalFoS collection currently contains 2,498 entries for Salmonella, as well as for Citrobacter, Hafnia and Proteus, three genera often identified as false-positives by a number of Salmonella detection schemes. It includes previously described collections such as the unique Salmonella Genetic Stock Centre strains, described at http://people.ucalgary.ca/tilde{}kesander/. This collection was assembled with the aim of representing maximal genomic diversity.
Sequencing 4,500 Salmonella Genomes: Objectives and Strategy
Our working hypothesis is that a very high-quality, large-scale bacterial genome database available through a user-friendly pipeline will have a major impact for epidemiology, diagnosis, prevention and treatment. By generating a comprehensive genome sequence database truly representative of the foodborne Salmonella population, we will: (1) assemble a large and representative strain collection, with associated genome data, useful for antimicrobial testing, identification of resistance markers, data mining for new therapeutic targets and development of machine learning strategies; (2) develop platforms and pipelines to manage and analyze this information, which will allow identification of prognostic markers, fast epidemiological tracking and reduction of socio-economic costs. We seek to develop user-friendly tools that will enable epidemiologists, microbiologists, clinicians and others to interpret genomic data, thus leading to informed decisions in cases of food contamination and outbreaks. The contamination of fresh produce by Salmonella will be addressed through the development of natural solutions to control the presence of Salmonella on fruits and vegetables as they are growing in the fields. New tests will also be developed so that fresh produce can be quickly and efficiently tested for the presence of Salmonella before being sold to consumers. In the context of outbreak investigation, the genomic data will be used to assess high-quality SNPs and core/whole genome MLST for their usefulness in genetic discrimination in addition to other emerging methods such as CRISPR and prophage sequence typing. As for outbreak investigation software, the National Microbiology Laboratory-Public Health Agency of Canada group has implemented the Integrated Rapid Infectious Disease Analysis project (IRIDA)5 and developed the SNVPhyl phylogenomics pipeline that is in use by PulseNet Canada for microbial genomic epidemiology (Petkau et al., 2016). A complementary system called the Metagenomics Computation and Analytics Workbench (MCAW) is being implemented as a computing service for food safety (Edlund et al., 2016; Weimer et al., 2016).
Sequencing for this project is performed on an Illumina MiSeq instrument (at the Plateforme d’Analyses Génomiques of the IBIS, Université Laval, Quebec City, QC, Canada), at a rate of 120 genomes per week, using 300 bp paired-end libraries, and with a median coverage of 45×. In order to perform core genome phylogenetic analysis, the pan-genome, i.e., the complete repertoire of genes of a species, is determined using a recently developed software capable of handling high-quality NGS data from thousands of genomes: Saturn V version 1.06 (Jeukens et al., 2017). Additional analyses focus on genes implicated in virulence using comparative genomics predictions of confirmed and predicted virulence factors (Yang et al., 2008), and resistome identification based on the comprehensive antibiotic resistance database (CARD) (Mcarthur et al., 2013; Jia et al., 2016). A set of new reference Salmonella genomes representing maximal genomic diversity among foodborne pathogens will then be selected for PacBio Sequel sequencing to become fully assembled and annotated as a single circular chromosome.
The Ibis Bioinformatics Pipeline for Genome Assembly
When working with hundreds or thousands of genomes, analysis software for assembly, annotation, statistics for quality control and selection of additional reference genomes is required to extract relevant information in an automated and reliable fashion with minimal human intervention. Ideally, this software should be platform independent and able to analyze sequence data directly without being tied to proprietary data formats. This insures maximal flexibility and reduces lag time to a minimum. We are currently using an integrated pipeline for de novo assembly of microbial genomes based on the A5 pipeline (Tritt et al., 2012). It was parallelized on a Silicon Graphics UV 300 using up to 120 cores to accommodate raw data from 120 genomes and provide assembly statistics as well as reference genome alignment metrics in as little as 2 h. This automated approach currently results in a median of 35 scaffolds per genome (median N50 = 462 kb).
Phylogeny of Salmonella
Once isolates from a given outbreak are sequenced, patterns of shared variations can be used to infer which isolates within the outbreak are most closely related to each other (e.g., Didelot et al., 2017). As a future strategy for the Syst-OMICS project, this could be applied to partially sampled and on-going Salmonella outbreaks. Here, as a first step in the study of S. enterica diversity and epidemiology, we used 3,377 genomes; 1,627 were from a collaboration with UC Davis (Bart C. Weimer), and 1,750 were part of SalFoS. All genomes with >100 scaffolds were eliminated; this filter typically removes the vast majority of low coverage (i.e., low quality) assemblies and mixed cultures. As our assembly pipeline also includes alignment on a suite of reference genomes, it is also possible to ensure that genomes used belong to S. enterica. The core (conserved) genome was identified with Saturn V, and consisted of 839 genes, which were used for phylogenetic analysis. This number of core genes, which seems small compared to other studies (2,882 core genes for 73 genomes from 2 subspecies, Leekitcharoenphon et al., 2012), is due to both the extensive diversity and the high number of genomes used. As depicted in Figure 1, this population of S. enterica strains could be divided into seven major groups. They correspond to S. enterica subspecies enterica clades A and B and a collection of branching subspecies previously defined as salamae, arizonae, diarizonae, houtenae and indica. The significant number of strains (3,377) included in our analysis and their wide-ranging sources (including environmental, human, animal and food) is essential to understand the diversity of Salmonella as a foodborne pathogen and in defining levels of virulence. The remarkable genomic diversity exhibited in Figure 1 is thought to enable the colonization of a wide range of ecological niches. The Salmonella Syst-OMICS consortium will provide fine-scale analysis of this diversity via virulence factors, antibiotic resistance genes as well as complete core and accessory genomes.
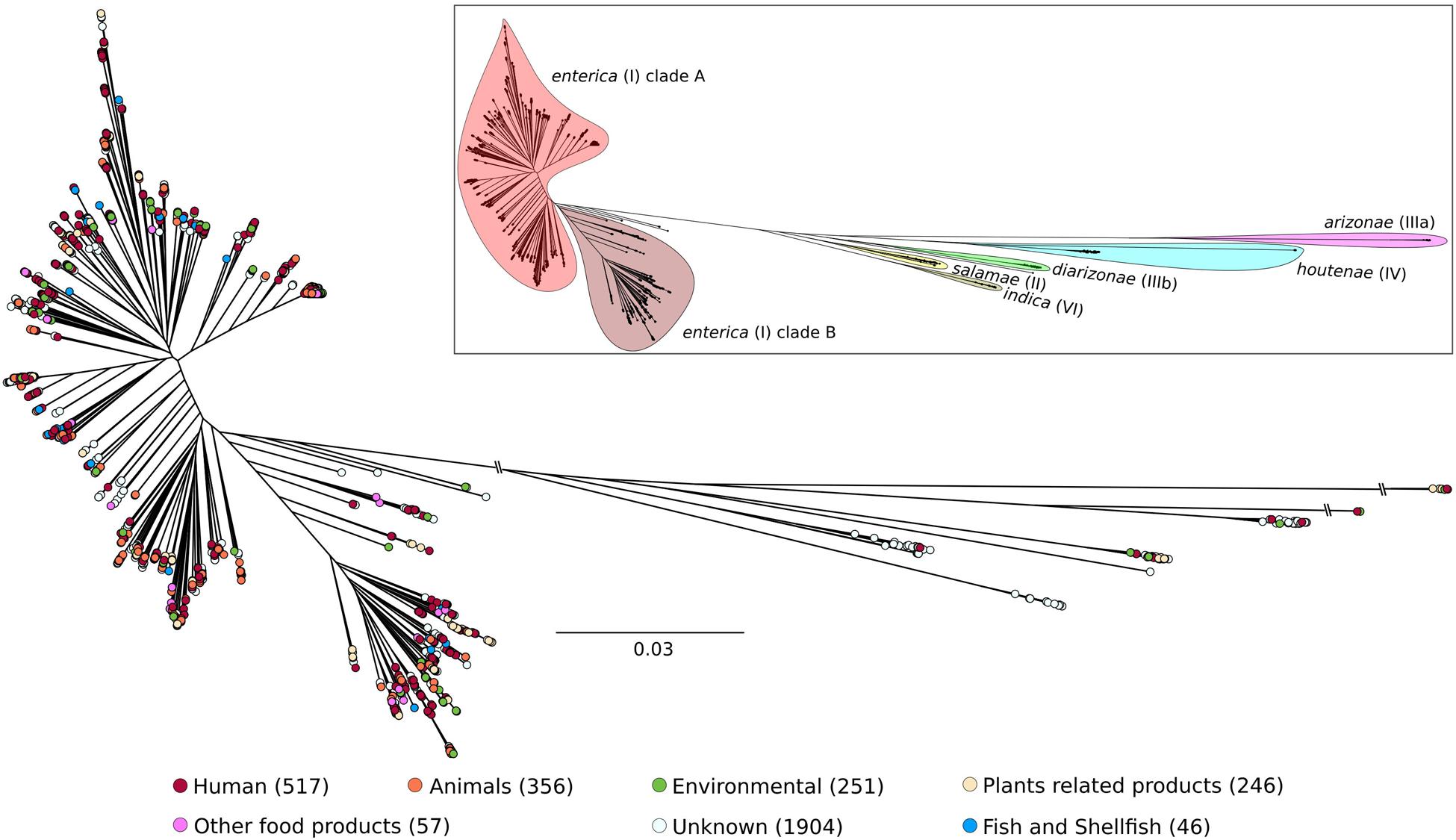
FIGURE 1. Unrooted maximum likelihood tree of 3,377 Salmonella enterica genomes based on 196,774 SNPs using FastTree 2.1.9 (1000 bootstraps). The six S. enterica subspecies names and specific epithets are indicated on the upper right tree. S. enterica subspecies enterica is split into two major lineages, clade A and clade B, as proposed by Timme et al. (2013). The two S. bongori (V) isolates contained in SalFoS were not included in the phylogenetic tree because they unnecessarily decrease resolution within the S. enterica subspecies. Number of genomes within each S. enterica subspecies are 3,235 enterica (2,648 in clade A and 587 in clade B), 51 houtenae, 32 diarizonae, 28 salamae, 17 indica, 8 arizonae and 6 with unknown subspecies. Tree tips were colored based on the source of each isolate. The number of isolates represented for each source category is shown between parentheses.
Linking SalFoS with the Comprehensive Antibiotic Resistance Database
The SalFoS database is intended to become an established platform for searching and comparing multiple genome sequences for Salmonella isolates. The database will also incorporate genome annotation and serotype prediction based on SISTR (Yoshida et al., 2016). Close attention to the links between specific genomic islands and patterns of SNPs in the core genome will help identify diagnostic sequences and SNP combinations for the development of new Salmonella subtyping methods with the highest resolution to date. This will be done using de novo island prediction with IslandViewer (Langille and Brinkman, 2009; Dhillon et al., 2015) as well as with gene presence-absence from SaturnV.
As an additional feature, we routinely determine the resistome of the genomes in SalFoS, i.e., the genes and variants likely involved in antibiotic resistance. This is done using the Resistance Gene Identifier (RGI) available with the CARD (Mcarthur et al., 2013; Jia et al., 2016), at http://arpcard.mcmaster.ca/. Figure 2 summarizes the resistomes of 3,377 genomes. In fact, the original dataset contained 1,003 unique resistomes, composed of various combinations of 195 different genes and variants. Despite this impressive diversity, the most striking feature shown in Figure 2 is that the two most frequently observed resistomes, which are extremely similar, account for 23% of the strains. They are therefore highly conserved and warrant further investigation. These results will be exploited to study and understand the pool of resistance genes present in Salmonella strains, with a focus on strains found in fresh produce, to understand the links between foodborne Salmonella and environmental strains with respect to resistance genes.
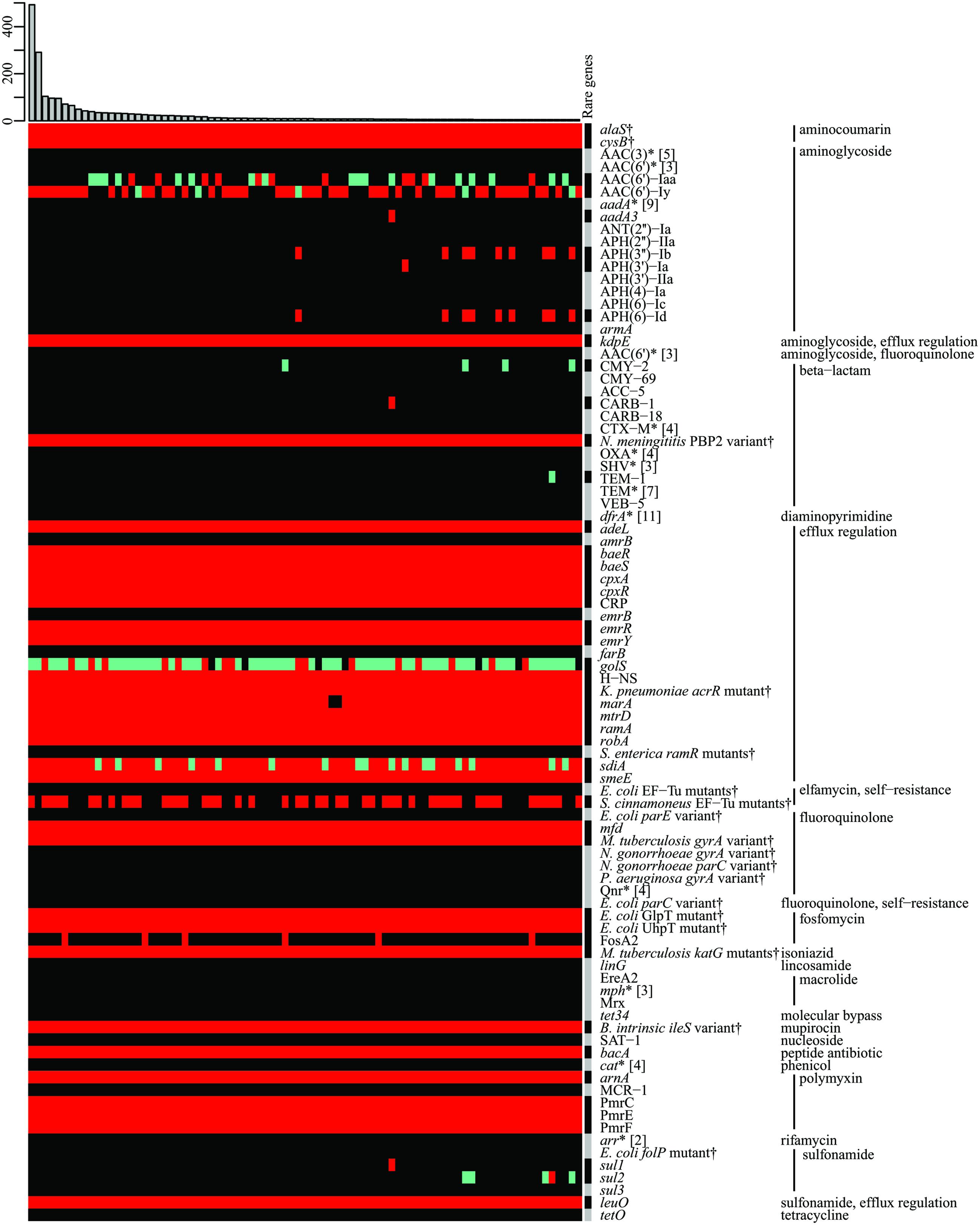
FIGURE 2. The resistome of 3,377 Salmonella strains. Gene, protein or variant presence were determined using the RGI-CARD (Mcarthur et al., 2013). Of 1,003 unique resistomes, only those present in at least five strains are shown; the histogram at the top represents absolute frequency. Other resistomes are condensed in the “Rare genes” column. AMR genes and variants are grouped by antibiotic family or function. Genes encoding efflux pumps, which are generally conserved, have been removed for figure clarity. Green: perfect match to a gene in the CARD, red: similar to a gene in the CARD, according to curated homology cut-offs, gray: perfect match and/or similar to a gene in the CARD, black: no match in the CARD, ∗(wildcard) represents multiple forms (exact number between square brackets) of the same gene or protein, †specific variants conferring resistance (protein variant models).
Linking Genomic and Clinical Data
It will be essential to match phenotypic, epidemiological and available clinical Salmonella data (antibiotic resistance, virulence, and anonymized clinical observations) to the genomic data produced. We will categorize metadata in SalFoS so that isolates can be sorted by phenotype, allowing rapid identification of linked genomic signatures and the development of prognostic approaches for diagnostic, epidemiology and surveillance. We will develop tools to rapidly collate data for a given strain type and produce a concise phenotypic and clinical profile that provides users with an evidence-based decision-making platform. The Canadian Food Inspection Agency, Health Canada, Agriculture Canada, provincial public health laboratories and the National Microbiology Laboratory-Public Health Agency of Canada group are expected to be end-users of the projects outcomes.
Future Genomic and Biological Studies of Salmonella
We will continuously improve SalFoS by adding Salmonella strains, NGS data and analysis as well as experimental results. Another aim of the Syst-OMICS consortium is to avoid duplication of efforts in Salmonella genomics and enhance interest from researchers having common goals. Additional members are welcome to join in and expand on our original Genome Canada project. We also intend to seek collaboration with other groups to connect our database with those developed for other Salmonella genomes. Finally, the Salmonella Syst-OMICS project could be a model for other groups interested in the bacterial genomics of infectious diseases, a strategy that we are also pursuing for Pseudomonas aeruginosa (Freschi et al., 2015).
Author Contributions
J-GER, JJ, LF, IK-I and RL collected strains, performed the analyses and drafted the manuscript. BB provided support for sequencing and analysis. MD contributed to the development of SalFoS. All other authors handled strains and collected metadata. All authors revised the manuscript.
Conflict of Interest Statement
The handling Editor declared a shared affiliation, though no other collaboration, with the authors ST and AG, and the handling Editor states that the process met the standards of a fair and objective review.
The other authors declare that the research was conducted in the absence of any commercial or financial relationships that could be construed as a potential conflict of interest.
Acknowledgments
We express our gratitude to members of the genomics analysis and bioinformatics platforms at IBIS. We also acknowledge Betty Wilkie, Ketna Mistry, Robert Holtslander and Shaun Kenaghan from the NML Salmonella reference laboratory for their assistance with serotyping. RL, LG, AG, ST, PD, DM, SG, SB, FD, SW, SM, GL, INF, YJ, PT, CN, RG, JoR, JW are funded by Genome Canada, provincial genome centers Génome Québec and Genome BC, and the Ontario Ministry of Research and Innovation. SM holds a Tier 1 Canada Research Chair in Bacteriophages.
Supplementary Material
The Supplementary Material for this article can be found online at: http://journal.frontiersin.org/article/10.3389/fmicb.2017.00996/full#supplementary-material
Footnotes
- ^ https://www.cdc.gov/salmonella/reportspubs/salmonella-atlas/serotypesnapshots.html
- ^ http://www.pulsenetinternational.org/networks/usa/
- ^ https://www.nml-lnm.gc.ca/Pulsenet/index-eng.htm
- ^ http://enterobase.warwick.ac.uk/
- ^ http://dev.irida.ca/
- ^ https://github.com/ejfresch/saturnV
References
Ashton, P. M., Nair, S., Peters, T. M., Bale, J. A., Powell, D. G., Painset, A., et al. (2016). Identification of Salmonella for public health surveillance using whole genome sequencing. PeerJ 4:e1752. doi: 10.7717/peerj.1752
Bekal, S., Berry, C., Reimer, A. R., Van Domselaar, G., Beaudry, G., Fournier, E., et al. (2016). Usefulness of high-quality core genome single-nucleotide variant analysis for subtyping the highly clonal and the most prevalent Salmonella enterica serovar heidelberg clone in the context of outbreak investigations. J. Clin. Microbiol. 54, 289–295. doi: 10.1128/JCM.02200-15
Casadevall, A. (2017). The pathogenic potential of a microbe. mSphere 2:e00015-17. doi: 10.1128/mSphere.00015-17
Chen, L., Xiong, Z., Sun, L., Yang, J., and Jin, Q. (2012). VFDB 2012 update: toward the genetic diversity and molecular evolution of bacterial virulence factors. Nucleic Acids Res. 40, D641–D645. doi: 10.1093/nar/gkr989
D’costa, V. M., Mcgrann, K. M., Hughes, D. W., and Wright, G. D. (2006). Sampling the antibiotic resistome. Science 311, 374–377. doi: 10.1126/science.1120800
Dhillon, B. K., Laird, M. R., Shay, J. A., Winsor, G. L., Lo, R., Nizam, F., et al. (2015). IslandViewer 3: more flexible, interactive genomic island discovery, visualization and analysis. Nucleic Acids Res. 43, 27. doi: 10.1093/nar/gkv401
Didelot, X., Fraser, C., Gardy, J., and Colijn, C. (2017). Genomic infectious disease epidemiology in partially sampled and ongoing outbreaks. Mol. Biol. Evol. 34, 997–1007. doi: 10.1093/molbev/msw275
Edlund, S. B., Beck, K. L., Haiminen, N., Parida, L. P., Storey, D. B., Weimer, B. C., et al. (2016). Design of the MCAW compute service for food safety bioinformatics. IBM J. Res. Dev. 60:12. doi: 10.1147/JRD.2016.2584798
Fatica, M. K., and Schneider, K. R. (2011). Salmonella and produce: survival in the plant environment and implications in food safety. Virulence 2, 573–579. doi: 10.4161/viru.2.6.17880
Forest, C., Faucher, S. P., Poirier, K., Houle, S., Dozois, C. M., and Daigle, F. (2007). Contribution of the stg fimbrial operon of Salmonella enterica serovar typhi during interaction with human cells. Infect. Immun. 75, 5264–5271. doi: 10.1128/iai.00674-07
Freschi, L., Jeukens, J., Kukavica-Ibrulj, I., Boyle, B., Dupont, M. J., Laroche, J., et al. (2015). Clinical utilization of genomics data produced by the international Pseudomonas aeruginosa consortium. Front. Microbiol. 6:1036. doi: 10.3389/fmicb.2015.01036
Gal-Mor, O., Boyle, E. C., and Grassl, G. A. (2014). Same species, different diseases: how and why typhoidal and non-typhoidal Salmonella enterica serovars differ. Front. Microbiol. 5:391. doi: 10.3389/fmicb.2014.00391
Hoffmann, M., Luo, Y., Monday, S. R., Gonzalez-Escalona, N., Ottesen, A. R., Muruvanda, T., et al. (2016). Tracing origins of the Salmonella bareilly strain causing a food-borne outbreak in the United States. J. Infect. Dis. 213, 502–508. doi: 10.1093/infdis/jiv297
Hoffmann, M., Zhao, S., Pettengill, J., Luo, Y., Monday, S. R., Abbott, J., et al. (2014). Comparative genomic analysis and virulence differences in closely related Salmonella enterica serotype Heidelberg isolates from humans, retail meats, and animals. Genome Biol. Evol. 6, 1046–1068. doi: 10.1093/gbe/evu079
Jackson, B. R., Griffin, P. M., Cole, D., Walsh, K. A., and Chai, S. J. (2013). Outbreak-associated Salmonella enterica serotypes and food commodities, United States, 1998-2008. Emerg. Infect. Dis. 19, 1239–1244. doi: 10.3201/eid1908.121511
Jeukens, J., Freschi, L., Vincent, A. T., Emond-Rheault, J. G., Kukavica-Ibrulj, I., Charette, S. J., et al. (2017). A pan-genomic approach to understand the basis of host adaptation in Achromobacter. Genome Biol. Evol. doi: 10.1093/gbe/evx061 [Epub ahead of print].
Jia, B., Raphenya, A. R., Alcock, B., Waglechner, N., Guo, P., Tsang, K. K., et al. (2016). CARD 2017: expansion and model-centric curation of the comprehensive antibiotic resistance database. Nucleic Acids Res. 45, D566–D573. doi: 10.1093/nar/gkw1004
Kheadr, E., Zihler, A., Dabour, N., Lacroix, C., Le Blay, G., and Fliss, I. (2010). Study of the physicochemical and biological stability of pediocin PA-1 in the upper gastrointestinal tract conditions using a dynamic in vitro model. J. Appl. Microbiol. 109, 54–64. doi: 10.1111/j.1365-2672.2009.04644.x
Kim, S. (2010). Salmonella serovars from foodborne and waterborne diseases in korea, 1998-2007: total isolates decreasing versus rare serovars emerging. J. Kor. Med. Sci. 25, 1693–1699. doi: 10.3346/jkms.2010.25.12.1693
Kozak, G. K., Macdonald, D., Landry, L., and Farber, J. M. (2013). Foodborne outbreaks in Canada linked to produce: 2001 through 2009. J. Food Prot. 76, 173–183. doi: 10.4315/0362-028X.JFP-12-126
Langille, M. G. I., and Brinkman, F. S. L. (2009). IslandViewer: an integrated interface for computational identification and visualization of genomic islands. Bioinformatics 25, 664–665. doi: 10.1093/bioinformatics/btp030
Lanza, V. F., De Toro, M., Garcillan-Barcia, M. P., Mora, A., Blanco, J., Coque, T. M., et al. (2014). Plasmid flux in Escherichia coli ST131 sublineages, analyzed by plasmid constellation network (PLACNET), a new method for plasmid reconstruction from whole genome sequences. PLoS Genet. 10:e1004766. doi: 10.1371/journal.pgen.1004766
Le Blay, G., Hammami, R., Lacroix, C., and Fliss, I. (2012). Stability and inhibitory activity of pediocin PA-1 against Listeria sp. in simulated physiological conditions of the human terminal ileum. Probiotics Antimicrob. Proteins 4, 250–258. doi: 10.1007/s12602-012-9111-1
Leekitcharoenphon, P., Lukjancenko, O., Friis, C., Aarestrup, F. M., and Ussery, D. W. (2012). Genomic variation in Salmonella enterica core genes for epidemiological typing. BMC Genomics 13:88. doi: 10.1186/1471-2164-13-88
Little, T. J., Allen, J. E., Babayan, S. A., Matthews, K. R., and Colegrave, N. (2012). Harnessing evolutionary biology to combat infectious disease. Nat. Med. 18, 217–220. doi: 10.1038/nm.2572
Liu, Y. Y., Chen, C. C., and Chiou, C. S. (2016). Construction of a pan-genome allele database of Salmonella enterica serovar enteritidis for molecular subtyping and disease cluster identification. Front. Microbiol. 7:2010. doi: 10.3389/fmicb.2016.02010
Majowicz, S. E., Musto, J., Scallan, E., Angulo, F. J., Kirk, M., O’brien, S. J., et al. (2010). The global burden of nontyphoidal Salmonella gastroenteritis. Clin. Infect. Dis. 50, 882–889. doi: 10.1086/650733
Mcarthur, A. G., Waglechner, N., Nizam, F., Yan, A., Azad, M. A., Baylay, A. J., et al. (2013). The comprehensive antibiotic resistance database. Antimicrob. Agents Chemother. 57, 3348–3357. doi: 10.1128/AAC.00419-13
Nuesch-Inderbinen, M., Cernela, N., Althaus, D., Hachler, H., and Stephan, R. (2015). Salmonella enterica serovar szentes, a rare serotype causing a 9-month outbreak in 2013 and 2014 in switzerland. Foodborne Pathog. Dis. 12, 887–890. doi: 10.1089/fpd.2015.1996
Perry, J. A., and Wright, G. D. (2014). Forces shaping the antibiotic resistome. Bioessays 36, 1179–1184. doi: 10.1002/bies.201400128
Petkau, A., Mabon, P., Sieffert, C., Knox, N., Cabral, J., Iskander, M., et al. (2016). SNVPhyl: a single nucleotide variant phylogenomics pipeline for microbial genomic epidemiology. bioRxiv 092940. doi: 10.1101/092940
Podolak, R., Enache, E., Stone, W., Black, D. G., and Elliott, P. H. (2010). Sources and risk factors for contamination, survival, persistence, and heat resistance of Salmonella in low-moisture foods. J. Food Prot. 73, 1919–1936.
Ribot, E. M., and Hise, K. B. (2016). Future challenges for tracking foodborne diseases: pulseNet, a 20-year-old US surveillance system for foodborne diseases, is expanding both globally and technologically. EMBO Rep. 17, 1499–1505. doi: 10.15252/embr.201643128
Rouli, L., Merhej, V., Fournier, P. E., and Raoult, D. (2015). The bacterial pangenome as a new tool for analysing pathogenic bacteria. New Microbes New Infect 7, 72–85. doi: 10.1016/j.nmni.2015.06.005
Roy, M.-F., Riendeau, N., Bédard, C., Hélie, P., Min-Oo, G., Turcotte, K., et al. (2007). Pyruvate kinase deficiency confers susceptibility to Salmonella Typhimurium infection in mice. J. Exp. Med. 204, 2949–2961. doi: 10.1084/jem.20062606
Scharff, R. L., Besser, J., Sharp, D. J., Jones, T. F., Peter, G.-S., and Hedberg, C. W. (2016). An Economic Evaluation of PulseNet. Am. J. Prev. Med. 50, S66–S73. doi: 10.1016/j.amepre.2015.09.018
Shariat, N., and Dudley, E. G. (2014). CRISPRs: molecular signatures used for pathogen subtyping. Appl. Environ. Microbiol. 80, 430–439. doi: 10.1128/AEM.02790-13
Thomas, M. K., Murray, R., Flockhart, L., Pintar, K., Fazil, A., Nesbitt, A., et al. (2015). Estimates of foodborne illness–related hospitalizations and deaths in Canada for 30 specified pathogens and unspecified agents. Foodborne Pathog. Dis. 12, 820–827. doi: 10.1089/fpd.2015.1966
Timme, R. E., Pettengill, J. B., Allard, M. W., Strain, E., Barrangou, R., Wehnes, C., et al. (2013). Phylogenetic diversity of the enteric pathogen Salmonella enterica subsp. enterica inferred from genome-wide reference-free SNP characters. Genome Biol. Evol. 5, 2109–2123. doi: 10.1093/gbe/evt159
Tritt, A., Eisen, J. A., Facciotti, M. T., and Darling, A. E. (2012). An integrated pipeline for de novo assembly of microbial genomes. PLoS ONE 7:e42304. doi: 10.1371/journal.pone.0042304
Weedmark, K. A., Mabon, P., Hayden, K. L., Lambert, D., Van Domselaar, G., Austin, J. W., et al. (2015). Clostridium botulinum Group II isolate phylogenomic profiling using whole-genome sequence data. Appl. Environ. Microbiol. 81, 5938–5948. doi: 10.1128/aem.01155-15
Weimer, B. C., Storey, D. B., Elkins, C. A., Baker, R. C., Markwell, P., Chambliss, D. D., et al. (2016). Defining the food microbiome for authentication, safety, and process management. IBM J. Res. Dev. 60:13. doi: 10.1147/JRD.2016.2582598
Yang, J., Chen, L., Sun, L., Yu, J., and Jin, Q. (2008). VFDB 2008 release: an enhanced web-based resource for comparative pathogenomics. Nucleic Acids Res. 36, D539–D542. doi: 10.1093/nar/gkm951
Yoshida, C. E., Kruczkiewicz, P., Laing, C. R., Lingohr, E. J., Gannon, V. P., Nash, J. H., et al. (2016). The Salmonella in silico typing resource (SISTR): an open web-accessible tool for rapidly typing and subtyping draft Salmonella genome assemblies. PLoS ONE 11:e0147101. doi: 10.1371/journal.pone.0147101
Keywords: Salmonella, foodborne pathogen, next-generation sequencing, bacterial genomics, phylogeny, antibiotic resistance, database
Citation: Emond-Rheault J-G, Jeukens J, Freschi L, Kukavica-Ibrulj I, Boyle B, Dupont M-J, Colavecchio A, Barrere V, Cadieux B, Arya G, Bekal S, Berry C, Burnett E, Cavestri C, Chapin TK, Crouse A, Daigle F, Danyluk MD, Delaquis P, Dewar K, Doualla-Bell F, Fliss I, Fong K, Fournier E, Franz E, Garduno R, Gill A, Gruenheid S, Harris L, Huang CB, Huang H, Johnson R, Joly Y, Kerhoas M, Kong N, Lapointe G, Larivière L, Loignon S, Malo D, Moineau S, Mottawea W, Mukhopadhyay K, Nadon C, Nash J, Ngueng Feze I, Ogunremi D, Perets A, Pilar AV, Reimer AR, Robertson J, Rohde J, Sanderson KE, Song L, Stephan R, Tamber S, Thomassin P, Tremblay D, Usongo V, Vincent C, Wang S, Weadge JT, Wiedmann M, Wijnands L, Wilson ED, Wittum T, Yoshida C, Youfsi K, Zhu L, Weimer BC, Goodridge L and Levesque RC (2017) A Syst-OMICS Approach to Ensuring Food Safety and Reducing the Economic Burden of Salmonellosis. Front. Microbiol. 8:996. doi: 10.3389/fmicb.2017.00996
Received: 29 March 2017; Accepted: 17 May 2017;
Published: 02 June 2017.
Edited by:
Sabah Bidawid, Health Canada, CanadaReviewed by:
Young Min Kwon, University of Arkansas, United StatesSheng Chen, Hong Kong Polytechnic University, Hong Kong
Copyright © 2017 Emond-Rheault, Jeukens, Freschi, Kukavica-Ibrulj, Boyle, Dupont, Colavecchio, Barrere, Cadieux, Arya, Bekal, Berry, Burnett, Cavestri, Chapin, Crouse, Daigle, Danyluk, Delaquis, Dewar, Doualla-Bell, Fliss, Fong, Fournier, Franz, Garduno, Gill, Gruenheid, Harris, Huang, Huang, Johnson, Joly, Kerhoas, Kong, Lapointe, Larivière, Loignon, Malo, Moineau, Mottawea, Mukhopadhyay, Nadon, Nash, Ngueng Feze, Ogunremi, Perets, Pilar, Reimer, Robertson, Rohde, Sanderson, Song, Stephan, Tamber, Thomassin, Tremblay, Usongo, Vincent, Wang, Weadge, Wiedmann, Wijnands, Wilson, Wittum, Yoshida, Youfsi, Zhu, Weimer, Goodridge and Levesque. This is an open-access article distributed under the terms of the Creative Commons Attribution License (CC BY). The use, distribution or reproduction in other forums is permitted, provided the original author(s) or licensor are credited and that the original publication in this journal is cited, in accordance with accepted academic practice. No use, distribution or reproduction is permitted which does not comply with these terms.
*Correspondence: Roger C. Levesque, rclevesq@ibis.ulaval.ca Lawrence Goodridge, lawrence.goodridge@mcgill.ca
†These authors have contributed equally to this work.