- 1Laboratory of Probiogenomics, Department of Chemistry, Life Sciences and Environmental Sustainability, University of Parma, Parma, Italy
- 2Stress Physiology Laboratory, Department of Chemistry, Life Sciences and Environmental Sustainability, University of Parma, Parma, Italy
- 3GenProbio s.r.l., Parma, Italy
- 4Division of Computational and Systems Medicine, Department of Surgery and Cancer, Imperial College London, London, United Kingdom
- 5Department of Food and Drug, University of Parma, Parma, Italy
- 6APC Microbiome Institute and School of Microbiology, National University of Ireland, Cork, Ireland
The composition of the gut microbiota of mammals is greatly influenced by diet. Therefore, evaluation of different food ingredients that may promote changes in the gut microbiota composition is an attractive approach to treat microbiota disturbances. In this study, three dietary fibers, such as inulin (I, 10%), resistant starch (RS, 10%), and citrus pectin (3%), were employed as supplements to normal chow diet of adult male rats for 2 weeks. Fecal microbiota composition and corresponding metabolite profiles were assessed before and after prebiotics supplementation. A general increase in the Bacteroidetes phylum was detected with a concurrent reduction in Firmicutes, in particular for I and RS experiments, while additional changes in the microbiota composition were evident at lower taxonomic levels for all the three substrates. Such modifications in the microbiota composition were correlated with changes in metabolic profiles of animals, in particular changes in acetate and succinate levels. This study represents a first attempt to modulate selectively the abundance and/or metabolic activity of various members of the gut microbiota by means of dietary fiber.
Introduction
The term “microbiota” is defined as the community of commensal, symbiotic, and pathogenic microorganisms present in a specific environment (Peterson et al., 2009). Since 2009, different metagenomic projects have shed light on the microbiota composition of humans from various geographical locations (Peterson et al., 2009; Cusack et al., 2013) by assessing hundreds of samples from skin, mouth, and gut. Such projects have generated a massive collection of individual data sets that have provided a detailed view of the composition, diversity, and adaptations of the retrieved bacterial DNA.
Definitely, the most interesting human niche is the gastrointestinal tract (GIT) where microbial colonization occurs at differing efficiency from the oral cavity to the rectum, depending on the different environmental conditions. In the large intestine, an estimated 1011–1012 bacterial cells/mL (Turroni et al., 2008) gain energy predominantly by fermentation of indigestible dietary components or molecules secreted by the host (Graf et al., 2015).
It is believed that diet modulates the composition of the gut microbiota (Conlon and Bird, 2014; Cockburn and Koropatkin, 2016). In particular, dietary carbohydrates that transit through the GIT are able to influence the composition and stability of the gut microbiota (Fava et al., 2013; Xu and Knight, 2015). Furthermore, disturbing the balance between “protective” versus “harmful” intestinal bacteria may result in a so-called “dysbiosis state” (Tamboli et al., 2004; Milani et al., 2016), where an overgrowth of “pathobionts,” i.e., potentially pathogenic symbionts of the microbiota (Chow et al., 2011), could negatively affect important functions of the microbiota (i.e., maturation and regulation of host immunity and gut functions) (Lee, 2013).
The term “prebiotic” refers to compounds, and in particular to indigestible (i.e., non-digestible by the host) carbohydrates, that are able to stimulate growth and/or metabolism of (selected) beneficial gut bacteria, such as members of the genera Bifidobacterium and Lactobacillus (Tanaka et al., 1983; Gibson and Roberfroid, 1995; Gibson et al., 2004; Rastall and Gibson, 2015). Such dietary compounds, through their ability to modulate the gut microbiota, are purported to reduce the prevalence and duration of infectious and antibiotic-associated diarrhea and the inflammation and symptoms associated with inflammatory bowel disease, exert protective effects to prevent colon cancer, enhance the bioavailability and uptake of minerals, reduce risk factors for cardiovascular disease, and prevent obesity (Slavin, 2013; Gibson et al., 2017). Thus, the use of prebiotics is considered an important approach to manipulate the gut microbiota in order to prevent or treat unbalanced GIT conditions (Dahiya et al., 2017). Prebiotic compounds, such as the human milk-, galacto-, fructo-, xylo-, and pectin-oligosaccharides and lactulose, are known to selectively stimulate bifidobacterial growth and metabolism (Roberfroid et al., 2010; Cockburn and Koropatkin, 2016; Wilson and Whelan, 2017). However, much less is known about the effects of dietary fibers on the gut microbiota, and it has not been established a direct effect of the supplementation of these compounds on the re-establishment of specific gut commensals. For these reasons, we investigated in this study the effects of various dietary substrates, including inulin (I), resistant starch (RS), and citrus pectin (CP), on the gut microbiota of adult wild-type Groningen rats in a time-limited intervention study employing 16S rRNA microbial profiling and metabolomic analyses.
Materials and Methods
Ethics Statement
All experimental procedures and protocols involving animals were approved by the Veterinarian Animal Care and Use Committee of Parma University, and conducted in accordance with the European Community Council Directives dated 22 September 2010 (2010/63/UE).
Animal Housing
Experiments involved 5/4-month-old male wild-type Groningen rats (Rattus norvegicus), originally obtained from the University of Groningen (Netherlands), and bred in animal facilities under standard conditions. This outbred strain displays a large inter-individual variability, as observed in humans (Carnevali et al., 2014). After weaning, rats were housed in same sex sibling groups in rooms under humidity-(50 ± 10%) and temperature-(22 ± 2°C) controlled conditions, a 12-h light–dark cycle (lights on at 7 a.m.), and with food and water available ad libitum.
Diet and Experimental Design
Figure 1A displays the timeline of all procedures. From the initiation of the experiments, rats were housed individually in polymethyl methacrylate (Plexiglas®) cages (39 cm × 23 cm × 15 cm). The first week acts as an acclimatization period, where rats do not change in their habits and continue to follow a normal chow diet. In this way, rats could represent a negative control of themselves, acting as the baseline for microbiota and metabolomics analyses (Turroni et al., 2016). Following this first acclimatization week, rats (n = 18) were randomized to standard chow diet with added I (n = 6), RS (n = 6), or CP (n = 6) for 14 days (Table 1). Following these 2 weeks, all animals returned to the standard chow diet without added substances, for a 1-week wash-out period before the end of the experiment.
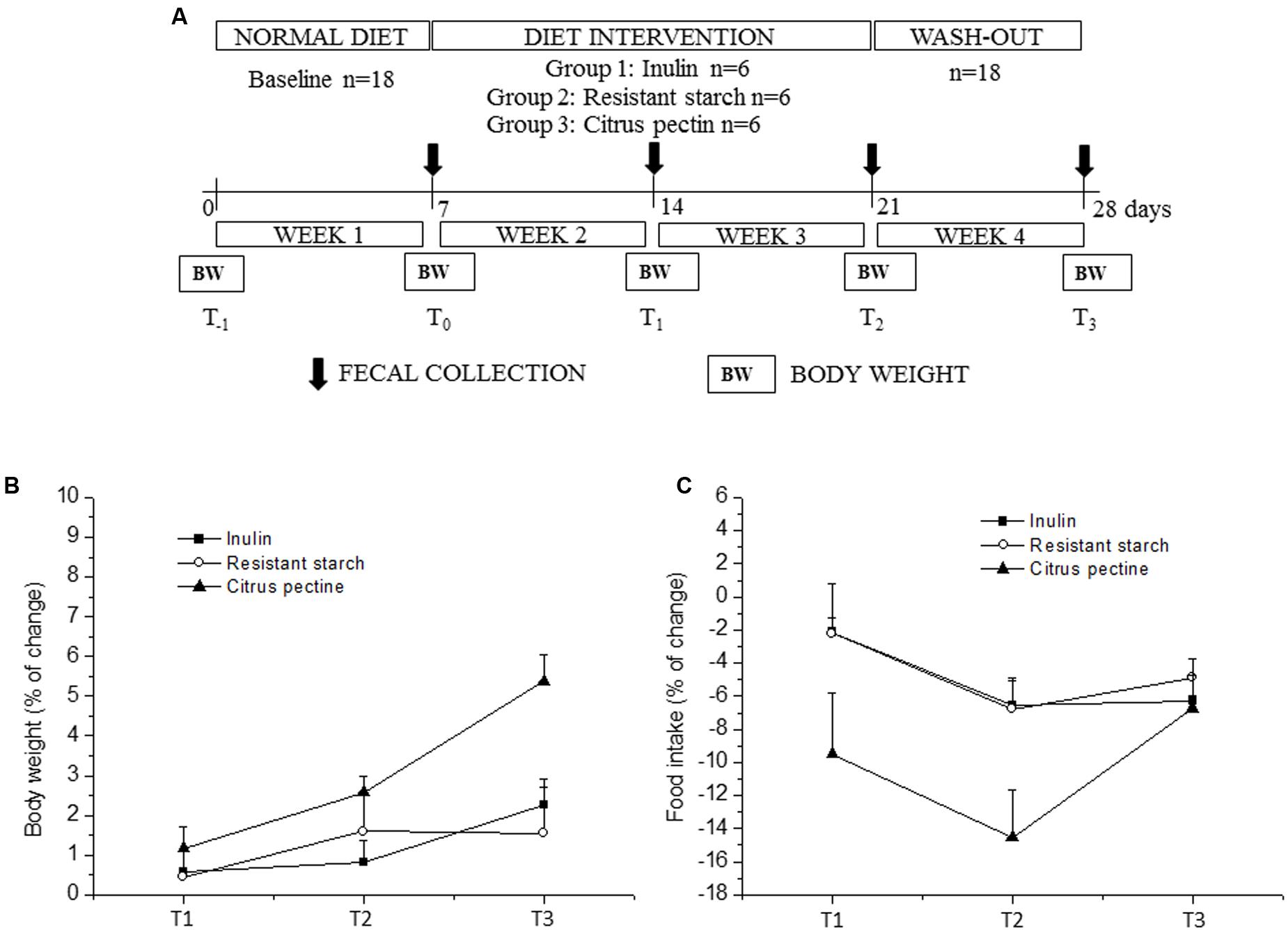
FIGURE 1. Timeline and results of the experimental procedure. (A) shows the schedule of the experimental procedures in wild-type Groningen rats that were fed with different carbohydrate supplements: I (10%), RS (10%), and CP (3%). (B,C) Display the body weight and food intake percentage changes relative to the respective “time 0” values, during the experimental procedure, respectively. Values are expressed as means ± SEM. Statistical results are reported in the “Results” section.
Standard diet consisted of 54.61% nitrogen-free extract (mainly represented by starch and hemicellulose), 5.54% fibers, 19.42% protein, 11.09% water, 2.58% lipids, and 6.76% ash (non-organic mineral matter) (3.9 kcal/g; 4RF21, Mucedola, Italy). The percentage of substrate supplementation to standard chow diet was determined based on published literature: 10% (w/w) I (Van den Abbeele et al., 2011; Pattananandecha et al., 2016), 10% (w/w) RS (Koh et al., 2016), and 3% (w/w) CP (Tian et al., 2016).
Food intake (FI) and body weight (BW) were measured daily and weekly, respectively (Figure 1A). BW changes were calculated as the percentage differences between BW values at the end of every week and the respective baseline value (T0). Food consumption was calculated as the percentage changes in FI adjusted for BW (FI/BW) relative to the respective T0 value. The sawdust bedding was replaced at the end of every week and before fecal collection. Consequently, fresh fecal samples were collected manually from a clean sawdust bedding for each animal, at most 1 h after deposition and stored at -20°C until analysis.
Fecal Bacterial DNA Extraction, 16S rRNA Gene PCR Amplification, and Sequencing
Rats samples were subjected to DNA extraction using the QIAamp DNA Stool Mini Kit following the manufacturer’s instructions (Qiagen). Partial 16S rRNA gene sequences were amplified from extracted DNA using primer pair Probio_Uni and/Probio_Rev, targeting the V3 region of the 16S rRNA gene sequence (Milani et al., 2013). Illumina adapter overhang nucleotide sequences were added to the partial 16S rRNA gene-specific amplicons, which were further, processed employing the 16S Metagenomic Sequencing Library Preparation Protocol (Part #15044223 Rev. B – Illumina). Amplifications were carried out using a Veriti Thermo Cycler (Applied Biosystems). The integrity of the PCR amplicons was analyzed by electrophoresis on a 2200 TapeStation Instrument (Agilent Technologies, United States). DNA products obtained following PCR-mediated amplification of the 16S rRNA gene sequences were purified by a magnetic purification step involving the Agencourt AMPure XP DNA purification beads (Beckman Coulter Genomics GmbH, Bernried, Germany) in order to remove primer dimers. DNA concentration of the amplified sequence library was determined by a fluorometric Qubit quantification system (Life Technologies, United States). Amplicons were diluted to a concentration of 4 nM, and 5 μL quantities of each diluted DNA amplicon sample were mixed to prepare the pooled final Library. Sequencing was performed using an Illumina MiSeq sequencer with MiSeq Reagent Kit v3 chemicals.
Bioinformatic Analysis
The fastq files were processed using QIIME (Caporaso et al., 2010) as previously described (Milani et al., 2013). Paired-end reads were merged and sequences that had passed quality control were kept with a length between 140 and 400 bp, mean sequence quality score >25, and with truncation of a sequence at the first base if a low quality rolling 10 bp window was found. Sequences with mismatched forward and/or reverse primers were removed.
In order to calculate downstream diversity measures (alpha and beta diversity indices, UniFrac analysis), 16S rRNA Operational Taxonomic Units (OTUs) were defined at 97% sequence homology using uclust (Edgar, 2010) and identified OTUs that were represented by less than 10 sequences were removed. All reads were classified to the lowest possible taxonomic rank using QIIME (Caporaso et al., 2010) and a reference dataset from the SILVA database version 123 (Quast et al., 2013). Biodiversity of the samples (alpha-diversity) was calculated with Chao1 and Shannon indexes. For alpha-diversity analysis, samples with less than 20,000 reads were omitted (see Supplementary Table S1 for details). Similarities between samples (beta-diversity) were calculated by weighted UniFrac (Lozupone and Knight, 2005). Similarity scores were calculated as values between 0 and 1. Principal coordinate analysis (PCoA) representations of beta-diversity were performed using QIIME (Caporaso et al., 2010).
Metabolomics Analyses
For short-chain fatty acid (SCFA) detection, fecal samples were treated as previously reported (Turroni et al., 2016). Briefly, fecal samples (0.1 g) were dissolved in 800 μL of water and lysed by mechanical treatment with 0.5 g of glass beads (diameter, 106 μm; Carlo Erba, Italy) on a Precellys homogenizer (Bertin, France) at 4°C for 2 min (maximum setting). Supernatant was collected and freeze-dried. Dried fecal extract samples were dissolved in 600 μL of 99.9% D2O (Sigma), vortexed for 1 min, and then centrifuged at 18,000 ×g for 10 min at 4°C. Supernatant was transferred into a 1.5 mL tube containing 60 μL of 1.5 M potassium phosphate buffer in D2O, pH = 7.4, 0.1% 3-(trimethylsilyl)-[2,2,3,3-2H4]propionic acid sodium salt (TSP) and 2 mM sodium azide. The resulting mixture was then vortexed, and 580 μL was transferred to an NMR tube with an outer diameter of 5 mm. 1H NMR spectra were acquired using a Bruker 600 MHz spectrometer (Bruker, Rheinstetten, Germany) at the operating 1H frequency of 600.13 MHz at a temperature of 300 K. 1H one-dimensional NMR profiling was acquired using a presaturation pulse sequence with the form RD-90°-t-90°-tm-90°-ACQ, where RD is the relaxation delay (4 s) during which a low power pulse is applied to saturate the water magnetization, t is a short delay typically of about 3 μs, 90° represents a 90° RF pulse, tm is the mixing time (10 ms) where a second presaturation sequence and gradients are used to filter out the water signal and ACQ is the data acquisition period (2.7 s). A total of 32 scans were collected into 64 k data points with a spectral width of 20 ppm. Automatic phasing, baseline correction, and reference to TSP signal at (0.00 ppm) were performed in automation using TopSpin 3.6 (Bruker BioSpin, Germany). The processed NMR spectral data were imported into MATLAB (R2016a, 7.14.0.739; MathWorks) and the region containing signals 0.25–10 ppm was digitized into approximately 20 k data points with a resolution of 0.0005 ppm. The remaining water peak region 4.7–4.9 ppm was removed. Probabilistic quotient normalization was applied to the remaining spectral data. Principal component analysis (PCA) and orthogonal partial least squares (OPLS) models were carried out with the unit variance scaling method in SIMCA (P+14.1). OPLS models were built to identify the metabolites with biggest changes in concentration in the fecal water due to the diet intervention. The concentration of these metabolites was calculated when possible due to overlap by integrating the area under the curve of the corresponding signals.
Statistical Analyses
Two-way ANOVA for repeated measures with “group” as between-subject factor (three levels: I, RS, and CP) was performed for: (i) BW changes, with “time” as within-subject factor (three levels: T1, T2, and T3); (ii) FI-to-BW ratio, with “time” as within-subject factor (four levels: week1, week2, week3, and week4). Follow up analysis was conducted using Student’s “t”-test, with a Bonferroni correction for multiple comparisons.
For alpha- and beta-diversity data, PERMANOVA and ANOVA analyses were used, using Tuckey’s HSD post hoc test. Linear discriminant analysis (Lefse) was performed for microbial modulation by dietary fibers. Kendall’s tau and significance (two-tailed test) were calculated for co-occurrence analysis. All statistical analyses were performed with SPSS software1.
Data Deposition
The 16S rRNA microbial profiling data sets achieved in this study were deposited in SRA under accession number PRJNA369513.
Results and Discussion
Food Consumption and Animal Growth
All dietary supplements examined in this study, i.e., I, RS, and CP, were selected based on previous scientific publications on effectiveness of these compounds in modulating certain gut microbiota members. In fact, I is a well-established prebiotic with stimulating effect on various saccharolytic bacteria (Ramirez-Farias et al., 2009). In addition, RS is the starch fraction that reaches the intestine in an intact form and provides a substantial energy supply for colonic bacteria (Birt et al., 2013). Furthermore, pectin is present in the primary cell walls of dicotyledonous plants, consists of a complex polymer of 1,4-linked-α-D-galacturonic acid units, and can be metabolized by gut bacteria (Martens et al., 2011; Lopez-Siles et al., 2012; Kaya et al., 2014). BW and FI changes are depicted in Figures 1B,C, respectively.
Two-way ANOVA for repeated measures yielded a significant effect of time and a time × group interaction for BW changes (time: p-value < 0.001; time × group: p-value < 0.01) and FI/BW (time: p-value < 0.001; time × group: p-value < 0.05). BW increment was significantly higher in CP compared to RS group at the end of the experimental protocol (T3). No significant differences among the three groups were found in FI/BW ratio. However, this parameter was significantly reduced in the CP group during the first (FI/BW: 0.0562, p-value < 0.01) and second week of diet intervention (FI/BW: 0.0531, p-value < 0.001) compared to the relative baseline value (week1, FI/BW: 0.0623).
Microbial Diversity in Rat Feces after Dietary Fiber Administration
In order to investigate possible gut microbiota modulation exerted by the three different substrates, we assessed the microbiota composition of fecal samples collected from all animals enrolled in this study (a total of 72 fecal samples) based on partial 16S rRNA gene-sequencing analysis as described previously (Milani et al., 2013). The 16S rRNA microbial sequencing of 72 fecal samples produced a total of 4,035,869 sequencing reads with an average of 56,053 reads per sample (Supplementary Table S1). Quality and chimera filtering produced a total of 3,634,488 filtered reads with an average of 50,479 filtered reads per sample (ranging from 1132 to 224,186 reads per sample, Supplementary Table S1).
Assessment of rarefaction curves based on the Shannon and Chao1 biodiversity indexes calculated for 10 subsampling of sequenced read pools indicated that Shannon and Chao1 curves, for all three interventions and at all experimental times, tend to reach a plateau (Supplementary Figures S1a,b). In this case, only 65 samples, those encompassing more than 20,000 reads were considered (see Supplementary Table S1 for details).
Therefore, in all considered cases the obtained sequencing data were deemed adequate to cover the large majority of biodiversity contained within the samples. Moreover, for RS and I experiments, rarefaction curves at time point 0 (aggregate T0, Supplementary Figures S1a,b) were shown to exhibit a significantly (p-value < 0.05) higher level of complexity than that observed after fiber interventions (aggregate T1 and T2, Supplementary Figures S1a,b). After the wash-out period, observed differences were shown to be not statistically significant (p-value > 0.05). When the analysis was restricted to phylum level, no significant differences were detected for CP intervention (p-value > 0.05), comparing rat microbiota before and at the end of the treatment (respectively, aggregate T0 and T2, Supplementary Figures S1a,b).
Microbiota Modulation by Dietary Fiber Supplementation
In order to evaluate if and how different substrates influence the microbiota composition, we explored the beta-diversity based on weighted UniFrac for RS, I, and CP interventions using the data obtained from samples at the four different time points, after which the UniFrac distance matrix was represented through PCoA (Figure 2). Notably, the inter-individual variability of the fecal microbiota assessed by PCoA analysis at T0 revealed a significant uniformity between the various animals enrolled in the study (Supplementary Figure S2a). Moreover, inspection of the predicted taxonomic profiles at phylum level for all samples showed that Bacteroidetes (53.9%) represents the dominant phylum, outnumbering the Firmicutes (39.8%) and Proteobacteria (4%) phyla (Supplementary Figure S2b). Actinobacteria, unclassified members (U.m.) of Saccharibacteria phylum, Cyanobacteria and Tenericutes together represent about 2% of the microbiota, while Verrucomicrobia, Spirochaetae, Fusobacteria, and Elusimicrobia phyla were determined to exhibit a low level presence (≤0.1%).
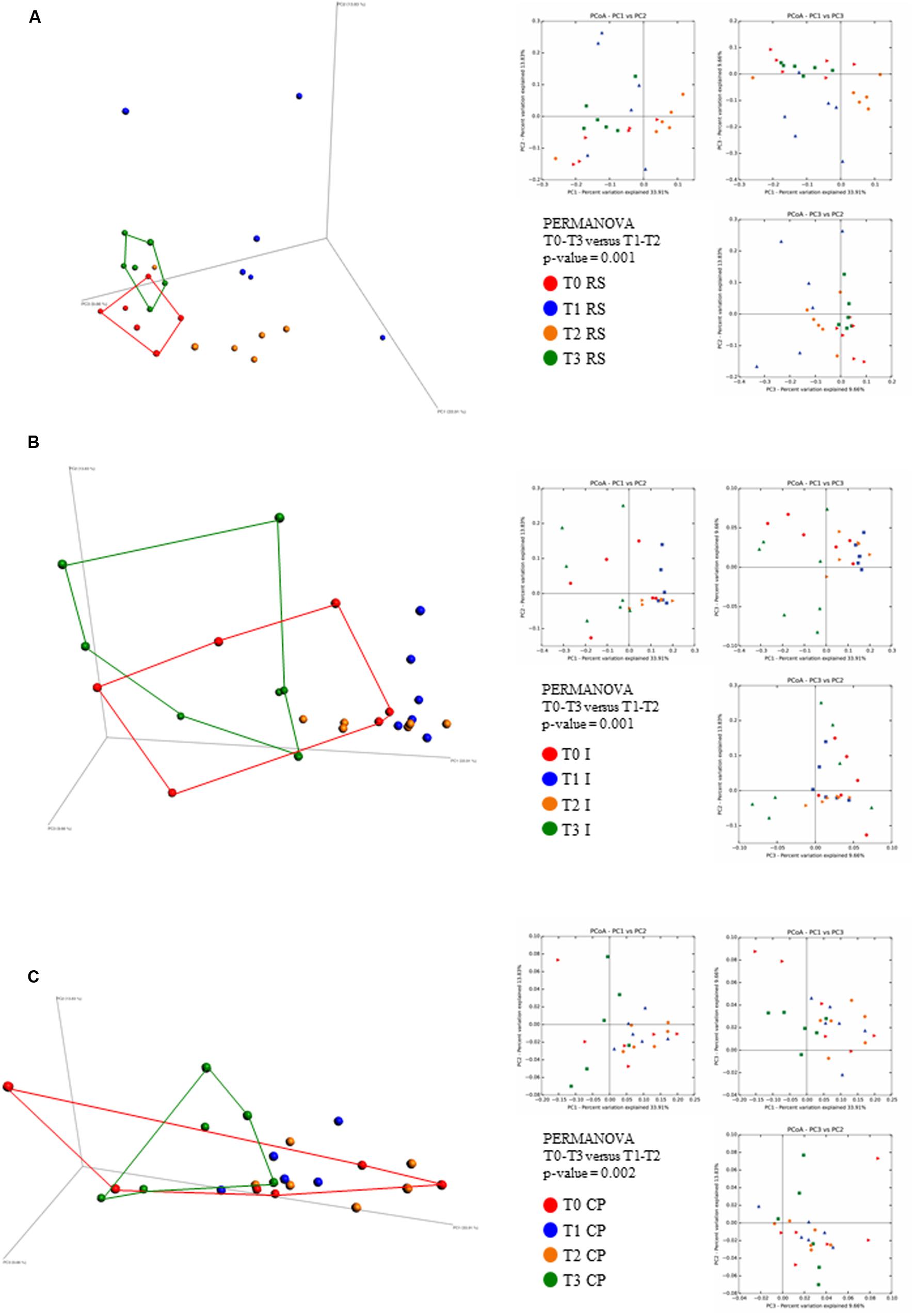
FIGURE 2. Evaluation of beta-diversity of the three sample sets. The predicted PCoA plots represent weighted UniFrac beta-diversity measures, encompassing all 72 fecal samples, are reported via three-dimensional images as well as two-dimensional sections. The three panels report the compositional analyses obtained for the three dietary fibers supplements (RS, A; I, B; and CP, C) at four different time points and are colored in red for time point 0 (T0), in blue for time point 1 (T1), in yellow for time point 2 (T2), and in green for time point 3 (T3).
Interestingly, for all three substrates, after 1 week of intervention, the samples grouped separately, thus suggesting different effects of these dietary ingredients on the various members of the fecal microbiota (Figure 2). The second week of dietary fiber supplementation maintained this trend, with a mitigation of most increased/reduced taxa for I and RS, while a consolidation of taxa that were increased/reduced during the first week of intervention was detected for CP (Figures 2A–C).
The wash-out period, consisted of 7 days during which rats were given an unsupplemented chow diet (Warden and Fisler, 2008). Analysis of the corresponding fecal samples indicated a reversion back to the T0 (i.e., baseline) fecal microbial composition (Figures 2A–C), thereby abolishing the effects of the administered prebiotics.
Such findings were confirmed by the obtained p-value of PERMANOVA statistical analysis (being 0.001 for RS and I and 0.002 for CP experiments), when the two groups data sets (T0 and T3 versus T1 and T2) are compared and excluding samples with less than 20,000 reads (Supplementary Table S1).
Inulin Selected Bacteroidetes – Responsive Taxa
Analyses of the microbiota composition following 2 weeks of I intervention, revealed an enrichment in bacterial genera belonging to the Bacteroidetes phylum (Figure 3A) (from 53 to 65%, not statistically significant) as well as of the Proteobacteria phylum (from 3 to 9%, p-value = 0.006), with a concomitant decrease of Firmicutes (from 41 to 23%, not statistically significant). A similar gut microbiota modulation by I has been described previously (Van den Abbeele et al., 2013; Reygner et al., 2016).
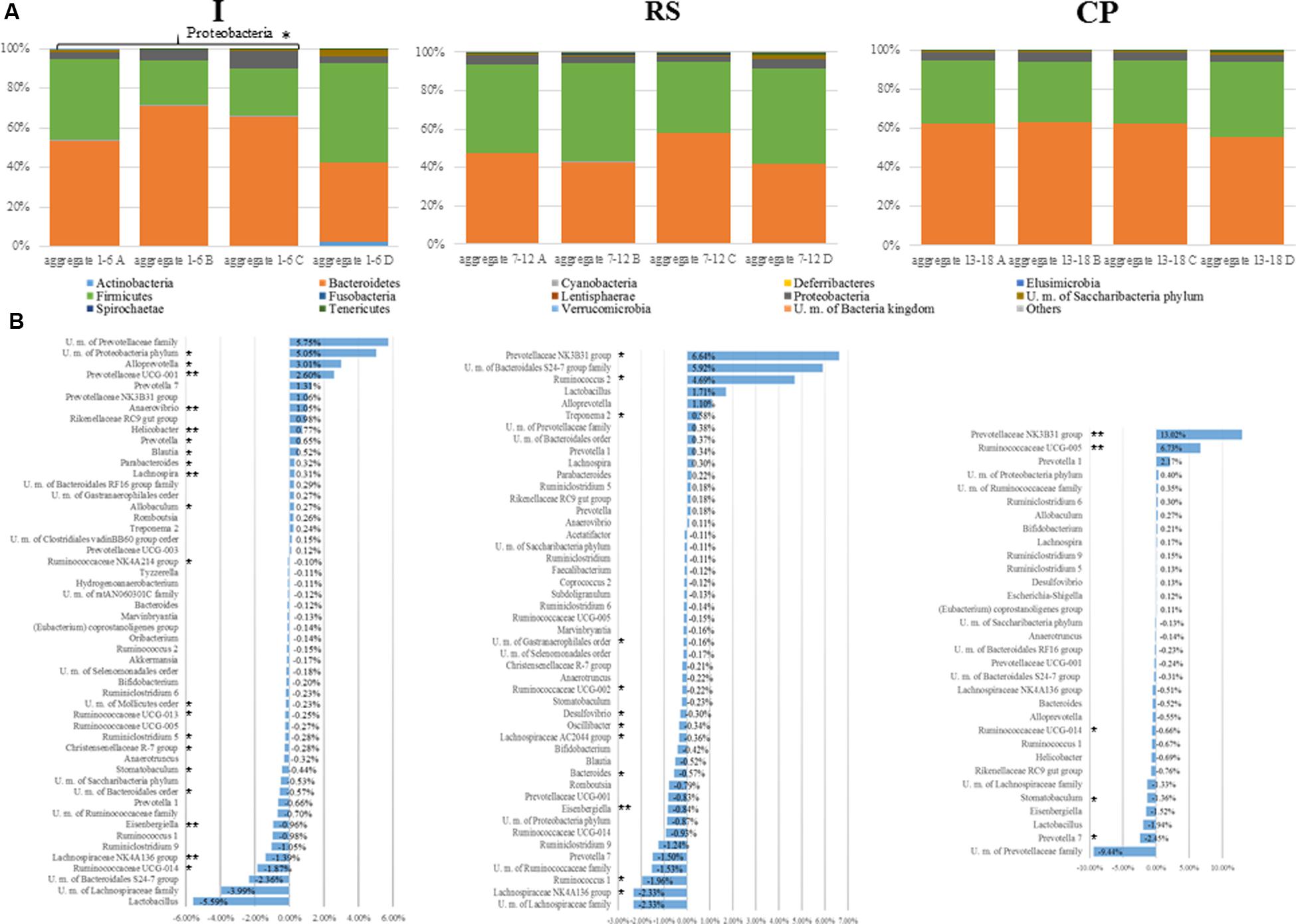
FIGURE 3. Exploration of microbiota diversity associated with RS, I, and CP interventions at different time points. (A) Represents bar plots of the identified bacterial phyla in the 72 analyzed samples, 24 samples per substrate, six for each time point. The legend reports the average relative abundance of each phyla for each substrate tested. (B) Depicts the variation in terms of relative abundance >0.1% and <–0.1% of the bacterial taxa, for the three substrates (respectively, I, RS, and CP) at the end of the prebiotic supplementation (T2) in comparison with the initial microbiota composition (T0). Statistical significance of microbial modulation has been calculated using Lefse (https://huttenhower.sph.harvard.edu/galaxy/) and indicated with asterisks as follow: ∗p-value < 0.05, ∗∗p-value < 0.005, and ∗∗∗p-value < 0.001.
Assessment of modulation of the Bacteroidetes phylum members at finer taxonomic levels (Figure 3B) revealed an increase in the saccharolytic (Rajilic-Stojanovic and de Vos, 2014) Prevotellaceae family members (+13.5%, p-value < 0.05) and in Parabacteroides spp. (+0.32%, p-value = 0.01), while a modest reduction in members of the Bacteroidales order was detected (p-value < 0.05). When a similar analysis was performed for detected Firmicutes phylum members a reduction in Lachnospiraceae family members (Eisengerghiella spp. – 1% with p-value 0.0039, U.m. Lachnospiraceae – 4% with p-value > 0.05 and Stomatobaculum spp. – 0.04% with p-value 0.025), Clostridiales order members (Ruminoclostridium spp. – 1.5% with p-value 0.0373 and members of Ruminococcaceae family +4.3% with p-values < 0.05), and Christensenellaceae R-7 group were observed (p-value < 0.05) (Figure 3B). Interestingly, in accordance with the current exiting literature, the level of Allobaculum spp., belonging to the Erysipelotrichaceae family, was increased by I treatment (+0.27%, p-value = 0.0104) (Catry et al., 2017).
Resistant Starch Stimulated Ruminococcus 2 Group and Lactobacillus spp. Growth
Resistant starch supplementation produced a lower modification in the composition of the fecal microbiota at phylum level, as compared to I intervention. In fact, an increase in Bacteroidetes was detected (an approximate 10% increase in relative abundance, p-value > 0.05), whose main contributors were Prevotellaceae family members (+7.5%, p-value < 0.05), Alloprevotella spp., and Parabacteroides spp. (respectively, +1.16 and +0.22%, p-values > 0.05). Such findings confirmed previous studies showing that some Bacteroides spp. and Prevotella spp. can degrade complex plant polysaccharides including starch (Sakamoto and Ohkuma, 2012).
A less evident decrease in members of Firmicutes phylum (-10%, p-value > 0.05) was reported in accordance with previous studies (Young et al., 2012). Nevertheless, a reduction of Lachnospiraceae family members (-5%, p-value < 0.05) and Clostridiales members (-5.6%, p-value < 0.05) was observed as previously reported (Ordiz et al., 2015). Against this trend, Lactobacillus spp. and Ruminococcus 2 group were increased by RS supplementation (respectively, +1.71 and +4.7%, p-values > 0.05 and 0.0065), probably due to the characteristic amylolytic activity of these genera (Abell et al., 2008; Goldsmith et al., 2017). Notably, Ruminococcus spp. has been previously shown to play an important role in RS degradation (Walker et al., 2011; Umu et al., 2015). Here, despite an abundant decrease of 2.2% of Ruminococcus 1 and Ruminococcaceae UCG_002 (p-values 0.0249 and 0.0065, respectively, Figure 3B), these observations were confirmed (Figure 3B).
Citrus Pectin Selected Specific Taxa, Without Changing the General Microbiota Composition
Citrus pectin intervention was shown to result in weak or undetectable effects on the microbiota composition at phylum level (p-value > 0.05). However, when the analysis was performed at lower taxonomic ranks it was possible to identify significant changes in a small number of taxa. Principal variations in rodent microbiota fed with CP were characterized by an increase in members of the Prevotellaceae NK3B31 group (+13%, p-value = 0.004) and Ruminococcaceae UCG-005 (+6.7%, p-value = 0.004). Members of the Prevotella genus were previously described as active pectin degraders (Nograsek et al., 2015), producing metabolic end-products such as succinate and acetate. Despite this, different OTUs related to the Prevotella genus showed different behaviors (Figure 3B). Moreover, a significant decrease was observed for Stomatobaculum spp. belonging to the Lachnospiraceae family (-1.4%, p-value = 0.037).
In contrast to the body of data about I and RS, relatively few information are public available for microbiota modulation exerted by pectin. In this context, it has been reported a decrease in the level of Bacteroidetes phylum and an increase in Firmicutes phylum (Jiang et al., 2016; Tian et al., 2016). In contrast, our experiments revealed no considerable changes in the gut microbiota composition of the rats following CP treatment.
Effects of Dietary Fiber on Metabolomics Profiles
Short-chain fatty acids and other metabolites derived from degradation of the fibers supplemented to normal chow diet of rats were investigated through NMR analysis and the orthogonal projections to latent structures for discriminant analysis (OPLS-DA) were used to identify discriminative metabolite profiles from samples pre- and post-prebiotic treatment (respectively, T0 and T2 time points). PCA was used to observe the separation trend between the 1H NMR metabolic profiles of the fecal water samples for the three groups of animals before and after the diet intervention.
Notably, the metabolic profiles show that rats after supplementation with I, RS, and CP clustered separately from the rat gut-metabolomes prior the treatment (Figures 4A–C), suggesting an effects of these dietary ingredients on the metabolomes. This finding is likely a reflection of the specific effects on microbiota changes elicited by each of the substrates, and suggests that the modulated bacterial species impact on the associated metabolome (Tang, 2011).
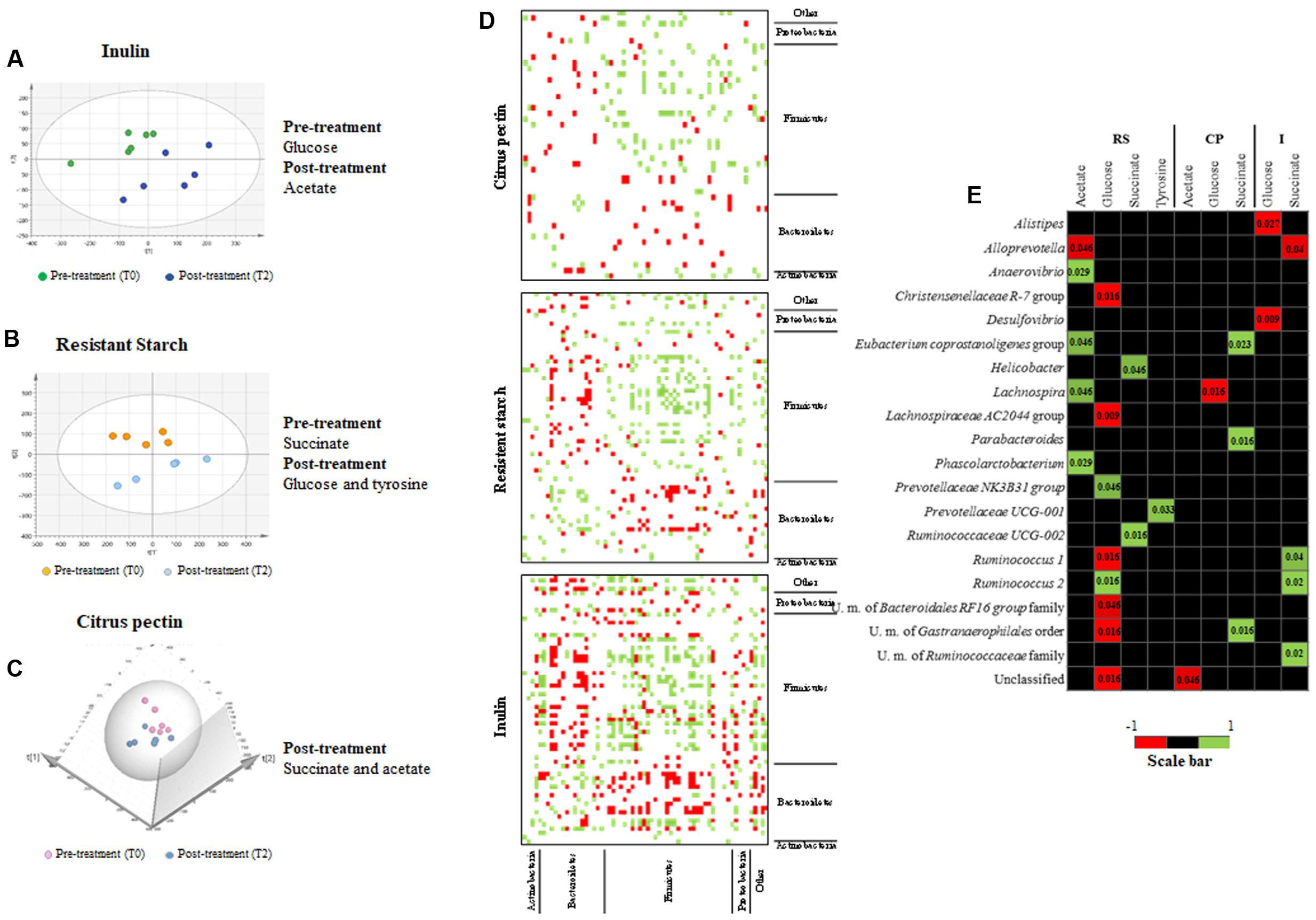
FIGURE 4. Metabolomics profile of dietary fiber intervention on rats. (A–C) Show the PCA score plots of rat fecal samples, respectively, for I (R2(cum) = 0.57, Qy(cum)2 = 0.33), RS (R2(cum) = 0.65, Qy(cum)2 = 0.414), and CP (R2(cum) = 0.70, Qy(cum)2 = 0.408). PCA score plots were obtained considering all metabolites obtained from NMR analysis. The discriminant metabolites between pre- and post-prebiotic intake are reported on the right. Pre-treatment (T0) and post-treatment (T2) samples were considered for the analysis. (D) Shows the correlation based on a co-variance analysis depicting the relationship between fecal microbiota members, detected through 16S rRNA profiling analysis. (E) Depicts a heat map representation illustrating the co-variance between the bacterial species abundance resulting from 16S rRNA gene-based microbial profiling and the abundance of metabolites resulting from NMR analyses of rat fecal samples. p-Values are reported inside each significant square.
It should be noted that only a relatively small number of metabolites were detected by NMR analysis of the assessed fecal samples, including acetate, succinate, glucose, tyrosine, and N-phenylacetylglycine (Supplementary Table S2). However, certain metabolites, such as lactate, butyrate, propionate, valerate, and caproate, which are typically found to be produced by the gut microbiota, appeared to be absent or present at a concentration that was below our detection limit (Sugahara et al., 2015).
The analysis allowed to clearly identify particular metabolites that were characteristic of the pre- or post-treatment groups (Figures 4A–C). In this context, a high production of acetate was detected and shown to be discriminative, i.e., able to differentiate two or more sample groups (Weimer and Slupsky, 2013), after supplementation with I and CP (Figures 4A,C,E). In contrast, in RS treatment, succinate was the distinctive metabolite present in pre-treatment samples (Figure 4B, time point T2). Succinate may be fermented by bacteria enhanced by RS diet, such as those belonging to the Bacteroidetes phylum, to produce propionate using the succinate pathway (Reichardt et al., 2014; Rios-Covian et al., 2016).
Moreover, glucose was the second most abundant metabolite and resulted discriminative only in RS post-treatment group (Student’s t-test, p-value < 0.05) (Supplementary Table S2), probably due to the release of glucose following extracellular RS degradation (Ze et al., 2012). Regarding CP, succinate was the discriminative metabolite of the post-treatment group (Figure 4C). Notably, this metabolite may be derived from pectin degradation by Prevotellaceae family members (Nograsek et al., 2015).
Correlations between Microbiota and Metabolite Modulations
In order to assess if a correlation exists between the changes observed in gut microbiota composition following dietary fiber supplementation and the identified metabolite profiles co-variance analyses were performed. Initial OTU-based cluster analysis indicated the existence of co-occurrence/co-exclusion of microbial taxa when a dietary fiber was added to the rodent diet. Therefore, in order to evaluate taxa coexistence, we calculated the Kendall tau rank correlation between the principal genera found in the samples considering only genera showing relative abundance >1% (Turroni et al., 2016; Figure 4D). Interestingly, a negative correlation between the genera belonging to the Prevotella – Porphyromonadaceae group and Lachnospiraceae and Ruminococcaceae families were detected when rats were fed with the three substrates (p-value < 0.05). This association is one of the main detected in the gut microbiota (Faust et al., 2012). In contrast, in our results, Bacteroides spp. showed a positive correlation with Lachnospiraceae and Ruminococcaceae families in RS and I treatments (p-value < 0.05).
In order to identify further relationships between metabolite abundances and specific microbe populations, a co-correlation plot was generated, which revealed a variety of significant microbe–metabolite associations (Figure 4E). The results highlight a positive covariance between the presence of members of the Clostridiales order and acetate production in RS experiments (p-values < 0.05, Figure 4E). As reported above, RS treatment reduces the presence of genera belonging to the Clostridiales family (such as Anaerovibrio spp., Eubacterium spp., Lachnospira spp., and Phascolarctobacterium spp.). Consequently, a reduction in this bacterial group may in turn cause a decreased acetate production. Indeed, this metabolite was not represented as discriminative after RS treatment (Figure 4B). A positive correlation was detected for Ruminococcus 2 and glucose (p-value = 0.016), after RS treatment, further confirming the well-known ability of this bacterial genus to degrade starch (Ze et al., 2012). Furthermore, Prevotellaceae NK3B31 group showed the identical positive correlation (p-value = 0.046). A negative correlation was detected between Alloprevotella spp., increased by RS, and acetate (p-value = 0.046).
Regarding succinate, a positive correlation was detected for members of the Ruminococcaceae family following RS and I supplementations (p-values < 0.05, Figure 4E). These bacteria were previously described to decrease following supplementation of these fibers (Young et al., 2012), and this finding correlates with the higher presence of succinate in pre-supplementation fecal samples. A negative correlation was detected for Alloprevotella spp. and succinate, in I treated rats (p-value = 0.04). In CP fecal samples, positive correlations were identified between succinate and Eubacterium spp. (p-value = 0.023), Parabacteroides spp. (p-value = 0.016), and U.m. of Gastranaerophilales order (p-value = 0.016), but these taxa were only modestly modified by this glycan supplementation.
Conclusion
The disruption of the microbiome equilibrium, which may lead to the so-called dysbiosis state, has been associated with intestinal disorders such as inflammatory bowel disease, irritable bowel syndrome, and celiac disease. There are different strategies proposed to prevent/remedy dysbiosis, one of these being the re-establishment of the microbiota homeostasis through the use of probiotic microorganisms that increase the portion of beneficial bacteria. Unfortunately, a large part of the human gut microbiota is represented so far by unculturable microorganisms, including bacteria that are highly sensitive to oxygen and that are thus very difficult to exploit as probiotic bacteria (Lagier et al., 2016).
An alternative strategy is to selectively increase the number or metabolic activity of specific members of the microbiota by certain food ingredients or prebiotics. In this study, a murine model was fed with food ingredients including non-digestible polysaccharides. Selective and specific modulation of the rat gut microbiota was observed, with enhancement and/or reduction of various gut commensals during treatments with these molecules.
No dysbiosis simulation was performed on the murine model, but the results obtained indicate that dietary fiber, in particular I and RS, could act as valuable prebiotic ingredients for selected microbiota members. The approach described in this study, despite the low number of substrates tested, may represent a valuable procedure in order to assess the microbiota modulation properties of defined food ingredients.
Author Contributions
CF, RS, AS, and FT designed and performed experiments and wrote the manuscript; LM, GL, and CM performed bioinformatic analyses; LC, SD, MM, AV, BJ, SL, GA, and MD performed experiments; AS, DDR, MV, and FT commented the manuscript; MV, FT, and DvS conceived the study, revised, and approved the manuscript; and all authors reviewed the manuscript.
Funding
This work was funded by the EU Joint Programming Initiative – A Healthy Diet for a Healthy Life (JPI HDHL, http://www.healthydietforhealthylife.eu/) and MIUR to MV. We thank GenProbio s.r.l. for financial support of the Laboratory of Probiogenomics. LM is supported by Fondazione Cariparma, Parma, Italy. SD is supported by Fondazione Caritro, Trento, Italy. DvS is a member of the APC Microbiome Institute funded by Science Foundation Ireland (SFI), through the Irish Government’s National Development Plan (Grant number SFI/12/RC/2273). Part of this research is conducted using the High Performance Computing (HPC) facility of the University of Parma.
Conflict of Interest Statement
The authors declare that the research was conducted in the absence of any commercial or financial relationships that could be construed as a potential conflict of interest.
Supplementary Material
The Supplementary Material for this article can be found online at: http://journal.frontiersin.org/article/10.3389/fmicb.2017.01749/full#supplementary-material
Footnotes
References
Abell, G. C. J., Cooke, C. M., Bennett, C. N., Conlon, M. A., and McOrist, A. L. (2008). Phylotypes related to Ruminococcus bromii are abundant in the large bowel of humans and increase in response to a diet high in resistant starch. FEMS Microbiol. Ecol. 66, 505–515. doi: 10.1111/j.1574-6941.2008.00527.x
Birt, D. F., Boylston, T., Hendrich, S., Jane, J. L., Hollis, J., Li, L., et al. (2013). Resistant starch: promise for improving human health. Adv. Nutr. 4, 587–601. doi: 10.3945/an.113.004325
Caporaso, J. G., Kuczynski, J., Stombaugh, J., Bittinger, K., Bushman, F. D., Costello, E. K., et al. (2010). QIIME allows analysis of high-throughput community sequencing data. Nat. Methods 7, 335–336. doi: 10.1038/nmeth.f.303
Carnevali, L., Nalivaiko, E., and Sgoifo, A. (2014). Respiratory patterns reflect different levels of aggressiveness and emotionality in Wild-type Groningen rats. Respir. Physiol. Neurobiol. 204, 28–35. doi: 10.1016/j.resp.2014.07.003
Catry, E., Bindels, L. B., Tailleux, A., Lestavel, S., Neyrinck, A. M., Goossens, J. F., et al. (2017). Targeting the gut microbiota with inulin-type fructans: preclinical demonstration of a novel approach in the management of endothelial dysfunction. Gut doi: 10.1136/gutjnl-2016-313316 [Epub ahead of print].
Chow, J., Tang, H., and Mazmanian, S. K. (2011). Pathobionts of the gastrointestinal microbiota and inflammatory disease. Curr. Opin. Immunol. 23, 473–480. doi: 10.1016/j.coi.2011.07.010
Cockburn, D. W., and Koropatkin, N. M. (2016). Polysaccharide degradation by the intestinal microbiota and its influence on human health and disease. J. Mol. Biol. 428, 3230–3252. doi: 10.1016/j.jmb.2016.06.021
Conlon, M. A., and Bird, A. R. (2014). The impact of diet and lifestyle on gut microbiota and human health. Nutrients 7, 17–44. doi: 10.3390/nu7010017
Cusack, S., O’Toole, P. W., and Eldermet consortium (2013). Challenges and implications for biomedical research and intervention studies in older populations: insights from the ELDERMET study. Gerontology 59, 114–121. doi: 10.1159/000343158
Dahiya, D. K., Renuka, Puniya, M., Shandilya, U. K., Dhewa, T., Kumar, N., et al. (2017). Gut microbiota modulation and its relationship with obesity using prebiotic fibers and probiotics: a review. Front. Microbiol. 8:563. doi: 10.3389/fmicb.2017.00563
Edgar, R. C. (2010). Search and clustering orders of magnitude faster than BLAST. Bioinformatics 26, 2460–2461. doi: 10.1093/bioinformatics/btq461
Faust, K., Sathirapongsasuti, J. F., Izard, J., Segata, N., Gevers, D., Raes, J., et al. (2012). Microbial co-occurrence relationships in the human microbiome. PLOS Comput. Biol. 8:e1002606. doi: 10.1371/journal.pcbi.1002606
Fava, F., Gitau, R., Griffin, B. A., Gibson, G. R., Tuohy, K. M., and Lovegrove, J. A. (2013). The type and quantity of dietary fat and carbohydrate alter faecal microbiome and short-chain fatty acid excretion in a metabolic syndrome ‘at-risk’ population. Int. J. Obes. 37, 216–223. doi: 10.1038/ijo.2012.33
Gibson, G. R., Hutkins, R., Sanders, M. E., Prescott, S. L., Reimer, R. A., Salminen, S. J., et al. (2017). Expert consensus document: The International Scientific Association for Probiotics and Prebiotics (ISAPP) consensus statement on the definition and scope of prebiotics. Nat. Rev. Gastroenterol. Hepatol. 14, 491–502. doi: 10.1038/nrgastro.2017.75
Gibson, G. R., Probert, H. M., Loo, J. V., Rastall, R. A., and Roberfroid, M. B. (2004). Dietary modulation of the human colonic microbiota: updating the concept of prebiotics. Nutr. Res. Rev. 17, 259–275. doi: 10.1079/NRR200479
Gibson, G. R., and Roberfroid, M. B. (1995). Dietary modulation of the human colonic microbiota: introducing the concept of prebiotics. J. Nutr. 125, 1401–1412.
Goldsmith, F., Guice, J., Page, R., Welsh, D. A., Taylor, C. M., Blanchard, E. E., et al. (2017). Obese ZDF rats fermented resistant starch with effects on gut microbiota but no reduction in abdominal fat. Mol. Nutr. Food Res. 61:1501025 doi: 10.1002/mnfr.201501025
Graf, D., Di Cagno, R., Fak, F., Flint, H. J., Nyman, M., Saarela, M., et al. (2015). Contribution of diet to the composition of the human gut microbiota. Microb. Ecol. Health Dis. 26:26164. doi: 10.3402/mehd.v26.26164
Jiang, T., Gao, X., Wu, C., Tian, F., Lei, Q., Bi, J., et al. (2016). Apple-derived pectin modulates gut microbiota, improves gut barrier function, and attenuates metabolic endotoxemia in rats with diet-induced obesity. Nutrients 8:126. doi: 10.3390/nu8030126
Kaya, M., Sousa, A. G., Crepeau, M. J., Sorensen, S. O., and Ralet, M. C. (2014). Characterization of citrus pectin samples extracted under different conditions: influence of acid type and pH of extraction. Ann. Bot. 114, 1319–1326. doi: 10.1093/aob/mcu150
Koh, G. Y., Rowling, M. J., Schalinske, K. L., Grapentine, K., and Loo, Y. T. (2016). Consumption of dietary resistant starch partially corrected the growth pattern despite hyperglycemia and compromised kidney function in streptozotocin-induced diabetic rats. J. Agric. Food Chem. 64, 7540–7545. doi: 10.1021/acs.jafc.6b03808
Lagier, J. C., Khelaifia, S., Alou, M. T., Ndongo, S., Dione, N., Hugon, P., et al. (2016). Culture of previously uncultured members of the human gut microbiota by culturomics. Nat. Microbiol. 1, 16203. doi: 10.1038/Nmicrobiol.2016.203
Lee, Y. K. (2013). Effects of diet on gut microbiota profile and the implications for health and disease. Biosci. Microbiota Food Health 32, 1–12. doi: 10.12938/bmfh.32.1
Lopez-Siles, M., Khan, T. M., Duncan, S. H., Harmsen, H. J. M., Garcia-Gil, L. J., and Flint, H. J. (2012). Cultured representatives of two major phylogroups of human colonic Faecalibacterium prausnitzii can utilize pectin, uronic acids, and host-derived substrates for growth. Appl. Environ. Microbiol. 78, 420–428. doi: 10.1128/Aem.06858-11
Lozupone, C., and Knight, R. (2005). UniFrac: a new phylogenetic method for comparing microbial communities. Appl. Environ. Microbiol. 71, 8228–8235. doi: 10.1128/Aem.71.12.8228-8235.2005
Martens, E. C., Lowe, E. C., Chiang, H., Pudlo, N. A., Wu, M., McNulty, N. P., et al. (2011). Recognition and degradation of plant cell wall polysaccharides by two human gut symbionts. PLOS Biol. 9:e1001221. doi: 10.1371/journal.pbio.1001221
Milani, C., Ferrario, C., Turroni, F., Duranti, S., Mangifesta, M., van Sinderen, D., et al. (2016). The human gut microbiota and its interactive connections to diet. J. Hum. Nutr. Diet. 29, 539–546. doi: 10.1111/jhn.12371
Milani, C., Hevia, A., Foroni, E., Duranti, S., Turroni, F., Lugli, G. A., et al. (2013). Assessing the fecal microbiota: an optimized ion torrent 16S rRNA gene-based analysis protocol. PLOS ONE 8:e68739. doi: 10.1371/journal.pone.0068739
Nograsek, B., Accetto, T., Fanedl, L., and Avgutin, G. (2015). Description of a novel pectin-degrading bacterial species Prevotella pectinovora sp nov., based on its phenotypic and genomic traits. J. Microbiol. 53, 503–510. doi: 10.1007/s12275-015-5142-0
Ordiz, M. I., May, T. D., Mihindukulasuriya, K., Martin, J., Crowley, J., Tarr, P. I., et al. (2015). The effect of dietary resistant starch type 2 on the microbiota and markers of gut inflammation in rural Malawi children. Microbiome 3, 37. doi: 10.1186/s40168-015-0102-9
Pattananandecha, T., Sirilun, S., Duangjitcharoen, Y., Sivamaruthi, B. S., Suwannalert, P., Peerajan, S., et al. (2016). Hydrolysed inulin alleviates the azoxymethane-induced preneoplastic aberrant crypt foci by altering selected intestinal microbiota in Sprague-Dawley rats. Pharm. Biol. 54, 1596–1605. doi: 10.3109/13880209.2015.1110597
Peterson, J., Garges, S., Giovanni, M., McInnes, P., Wang, L., Schloss, J. A., et al. (2009). The NIH human microbiome project. Genome Res. 19, 2317–2323. doi: 10.1101/gr.096651.109
Quast, C., Pruesse, E., Yilmaz, P., Gerken, J., Schweer, T., Yarza, P., et al. (2013). The SILVA ribosomal RNA gene database project: improved data processing and web-based tools. Nucleic Acids Res. 41, D590–D596. doi: 10.1093/nar/gks1219
Rajilic-Stojanovic, M., and de Vos, W. M. (2014). The first 1000 cultured species of the human gastrointestinal microbiota. FEMS Microbiol. Rev. 38, 996–1047. doi: 10.1111/1574-6976.12075
Ramirez-Farias, C., Slezak, K., Fuller, Z., Duncan, A., Holtrop, G., and Louis, P. (2009). Effect of inulin on the human gut microbiota: stimulation of Bifidobacterium adolescentis and Faecalibacterium prausnitzii. Br. J. Nutr. 101, 541–550. doi: 10.1017/S0007114508019880
Rastall, R. A., and Gibson, G. R. (2015). Recent developments in prebiotics to selectively impact beneficial microbes and promote intestinal health. Curr. Opin. Biotechnol. 32, 42–46. doi: 10.1016/j.copbio.2014.11.002
Reichardt, N., Duncan, S. H., Young, P., Belenguer, A., Leitch, C. M., Scott, K. P., et al. (2014). Phylogenetic distribution of three pathways for propionate production within the human gut microbiota. ISME J. 8, 1323–1335. doi: 10.1038/ismej.2014.14
Reygner, J., Lichtenberger, L., Elmhiri, G., Dou, S., Bahi-Jaber, N., Rhazi, L., et al. (2016). Inulin supplementation lowered the metabolic defects of prolonged exposure to chlorpyrifos from gestation to young adult stage in offspring rats. PLOS ONE 11:e0164614. doi: 10.1371/journal.pone.0164614
Rios-Covian, D., Ruas-Madiedo, P., Margolles, A., Gueimonde, M., de los Reyes-Gavilan, C. G., and Salazar, N. (2016). Intestinal short chain fatty acids and their link with diet and human health. Front. Microbiol. 7:185. doi: 10.3389/Fmicb.2016.00185
Roberfroid, M., Gibson, G. R., Hoyles, L., McCartney, A. L., Rastall, R., Rowland, I., et al. (2010). Prebiotic effects: metabolic and health benefits. Br. J. Nutr. 104, S1–63. doi: 10.1017/S0007114510003363
Sakamoto, M., and Ohkuma, M. (2012). Bacteroides sartorii is an earlier heterotypic synonym of Bacteroides chinchillae and has priority. Int. J. Syst. Evol. Microbiol. 62, 1241–1244. doi: 10.1099/ijs.0.035659-0
Slavin, J. (2013). Fiber and prebiotics: mechanisms and health benefits. Nutrients 5, 1417–1435. doi: 10.3390/nu5041417
Sugahara, H., Odamaki, T., Fukuda, S., Kato, T., Xiao, J. Z., Abe, F., et al. (2015). Probiotic Bifidobacterium longum alters gut luminal metabolism through modification of the gut microbial community. Sci. Rep. 5:13548. doi: 10.1038/Srep13548
Tamboli, C. P., Neut, C., Desreumaux, P., and Colombel, J. F. (2004). Dysbiosis as a prerequisite for IBD. Gut 53, 1057–1057.
Tanaka, R., Takayama, H., Morotomi, M., Kuroshima, T., Ueyama, T., Matsumoto, K., et al. (1983). Effects of administration of TOS and Bifidobacterium breve 4006 on the human faecal flora. Bifidobacteria Microflora 2, 17–24.
Tang, J. (2011). Microbial metabolomics. Curr. Genomics 12, 391–403. doi: 10.2174/138920211797248619
Tian, L., Scholte, J., Borewicz, K., van den Bogert, B., Smidt, H., Scheurink, A. J., et al. (2016). Effects of pectin supplementation on the fermentation patterns of different structural carbohydrates in rats. Mol. Nutr. Food Res. 60, 2256–2266. doi: 10.1002/mnfr.201600149
Turroni, F., Milani, C., Duranti, S., Mancabelli, L., Mangifesta, M., Viappiani, A., et al. (2016). Deciphering bifidobacterial-mediated metabolic interactions and their impact on gut microbiota by a multi-omics approach. ISME J. 10, 1656–1668. doi: 10.1038/ismej.2015.236
Turroni, F., Ribbera, A., Foroni, E., van Sinderen, D., and Ventura, M. (2008). Human gut microbiota and bifidobacteria: from composition to functionality. Antonie Van Leeuwenhoek 94, 35–50. doi: 10.1007/s10482-008-9232-4
Umu, O. C., Frank, J. A., Fangel, J. U., Oostindjer, M., da Silva, C. S., Bolhuis, E. J., et al. (2015). Resistant starch diet induces change in the swine microbiome and a predominance of beneficial bacterial populations. Microbiome 3, 16. doi: 10.1186/s40168-015-0078-5
Van den Abbeele, P., Gerard, P., Rabot, S., Bruneau, A., El Aidy, S., Derrien, M., et al. (2011). Arabinoxylans and inulin differentially modulate the mucosal and luminal gut microbiota and mucin-degradation in humanized rats. Environ. Microbiol. 13, 2667–2680. doi: 10.1111/j.1462-2920.2011.02533.x
Van den Abbeele, P., Venema, K., Van de Wiele, T., Verstraete, W., and Possemiers, S. (2013). Different human gut models reveal the distinct fermentation patterns of Arabinoxylan versus inulin. J. Agric. Food Chem. 61, 9819–9827. doi: 10.1021/jf4021784
Walker, A. W., Ince, J., Duncan, S. H., Webster, L. M., Holtrop, G., Ze, X., et al. (2011). Dominant and diet-responsive groups of bacteria within the human colonic microbiota. ISME J. 5, 220–230. doi: 10.1038/ismej.2010.118
Warden, C. H., and Fisler, J. S. (2008). Comparisons of diets used in animal models of high-fat feeding. Cell Metab. 7, 277–277. doi: 10.1016/j.cmet.2008.03.014
Weimer, B. C., and Slupsky, C. (eds). (2013). Metabolomics in Food and Nutrition. Cambridge: Woodhead Publishing.
Wilson, B., and Whelan, K. (2017). Prebiotic inulin-type fructans and galacto-oligosaccharides: definition, specificity, function, and application in gastrointestinal disorders. J. Gastroenterol. Hepatol. 32(Suppl. 1), 64–68. doi: 10.1111/jgh.13700
Xu, Z. J., and Knight, R. (2015). Dietary effects on human gut microbiome diversity. Br. J. Nutr. 113, S1–S5. doi: 10.1017/S0007114514004127
Young, W., Roy, N. C., Lee, J., Lawley, B., Otter, D., Henderson, G., et al. (2012). Changes in bowel microbiota induced by feeding weanlings resistant starch stimulate transcriptomic and physiological responses. Appl. Environ. Microbiol. 78, 6656–6664. doi: 10.1128/Aem.01536-12
Keywords: diet, microbiota, dietary fibers, rat model, acetate, succinate
Citation: Ferrario C, Statello R, Carnevali L, Mancabelli L, Milani C, Mangifesta M, Duranti S, Lugli GA, Jimenez B, Lodge S, Viappiani A, Alessandri G, Dall’Asta M, Del Rio D, Sgoifo A, van Sinderen D, Ventura M and Turroni F (2017) How to Feed the Mammalian Gut Microbiota: Bacterial and Metabolic Modulation by Dietary Fibers. Front. Microbiol. 8:1749. doi: 10.3389/fmicb.2017.01749
Received: 08 May 2017; Accepted: 28 August 2017;
Published: 12 September 2017.
Edited by:
Suhelen Egan, University of New South Wales, AustraliaReviewed by:
Hongxiang Hui, Southern Medical University, ChinaM. Pilar Francino, Fundación para el Fomento de la Investigación Sanitaria y Biomédica de la Comunitat Valenciana (FISABIO), Universitat de València, Spain
Copyright © 2017 Ferrario, Statello, Carnevali, Mancabelli, Milani, Mangifesta, Duranti, Lugli, Jimenez, Lodge, Viappiani, Alessandri, Dall’Asta, Del Rio, Sgoifo, van Sinderen, Ventura and Turroni. This is an open-access article distributed under the terms of the Creative Commons Attribution License (CC BY). The use, distribution or reproduction in other forums is permitted, provided the original author(s) or licensor are credited and that the original publication in this journal is cited, in accordance with accepted academic practice. No use, distribution or reproduction is permitted which does not comply with these terms.
*Correspondence: Francesca Turroni, ZnJhbmNlc2NhLnR1cnJvbmlAdW5pcHIuaXQ=