- 1Department of Food Science and Agricultural Chemistry, McGill University, Ste Anne de Bellevue, QC, Canada
- 2Department of Microbiology and Immunology, Faculty of Pharmacy, Mansoura University, Mansoura, Egypt
- 3Ottawa Laboratory Fallowfield, Canadian Food Inspection Agency, Ottawa, ON, Canada
- 4Laboratoire de Santé Publique du Québec, Institut National de Santé Publique due Québec, Ste Anne de Bellevue, QC, Canada
- 5Institut de Biologie Intégrative et des Systèmes, Université Laval, Québec City, QC, Canada
- 6Health Canada, Bureau of Microbial Hazards, Ottawa, ON, Canada
- 7Institute of Parasitology, McGill University, Montreal, QC, Canada
- 8Centre for Infectious Disease Control, National Institute for Public Health and the Environment, Bilthoven, Netherlands
- 9National Microbiology Laboratory, Public Health Agency of Canada, Guelph, ON, Canada
- 10Department of Biology, Wilfrid Laurier University, Waterloo, ON, Canada
- 11Department of Microbiology and Immunology, McGill University, Montreal, QC, Canada
- 12Department of Food Science, Cornell University, Ithaca, NY, United States
- 13Département de Microbiologie, Infectiologie et Immunologie, Université de Montréal, Montreal, QC, Canada
- 14Département de Biochimie, de Microbiologie et de Bioinformatique, Université Laval, Québec City, QC, Canada
Non-typhoidal Salmonella is a leading cause of foodborne illness worldwide. Prompt and accurate identification of the sources of Salmonella responsible for disease outbreaks is crucial to minimize infections and eliminate ongoing sources of contamination. Current subtyping tools including single nucleotide polymorphism (SNP) typing may be inadequate, in some instances, to provide the required discrimination among epidemiologically unrelated Salmonella strains. Prophage genes represent the majority of the accessory genes in bacteria genomes and have potential to be used as high discrimination markers in Salmonella. In this study, the prophage sequence diversity in different Salmonella serovars and genetically related strains was investigated. Using whole genome sequences of 1,760 isolates of S. enterica representing 151 Salmonella serovars and 66 closely related bacteria, prophage sequences were identified from assembled contigs using PHASTER. We detected 154 different prophages in S. enterica genomes. Prophage sequences were highly variable among S. enterica serovars with a median ± interquartile range (IQR) of 5 ± 3 prophage regions per genome. While some prophage sequences were highly conserved among the strains of specific serovars, few regions were lineage specific. Therefore, strains belonging to each serovar could be clustered separately based on their prophage content. Analysis of S. Enteritidis isolates from seven outbreaks generated distinct prophage profiles for each outbreak. Taken altogether, the diversity of the prophage sequences correlates with genome diversity. Prophage repertoires provide an additional marker for differentiating S. enterica subtypes during foodborne outbreaks.
Introduction
Salmonella is a genus of Gram-negative bacteria composed of two species: Salmonella bongori and Salmonella enterica. The latter is divided into six subspecies and one of these subspecies, S. enterica subsp. enterica is divided into more than 2,500 serovars based on the surface antigens (Grimont and Weill, 2007). S. enterica includes all Salmonella of medical importance; these are divided into typhoidal serovars (Typhi and Paratyphi A, B, and C) and thousands of non-typhoidal serovars (NTS). Human infection with non-typhoidal Salmonella strains typically results in a self-limiting enterocolitis known as salmonellosis. The World Health Organization (WHO) estimated that approximately 180 million cases of salmonellosis occur in people every year with 298,000 deaths (WHO, 2016).
Salmonellosis typically results from consumption of contaminated food or water.
High discrimination subtyping of isolates is essential to link outbreak cases and for source tracking, so that intervention to prevent further illnesses may be made. This can be challenging for S. enterica, for though it is serologically diverse it is a relatively clonal pathogen and the majority of reported cases of salmonellosis can be attributed to a small group of serovars. In Canada, 75% of reported cases of salmonellosis are attributed to 10 serovars, with 57% of cases attributed to only 3 serovars: S. Enteritidis (30%), S. Heidelberg (15%), and S. Typhimurium (12%) (Public Health Agency of Canada [PHAC], 2002). Consequently, conventional Salmonella sub-typing schemes, including pulsed field gel electrophoresis (PFGE), phage typing and Multiple Locus Variable Number Tandem Repeat Analysis (MLVA) may lack the level of discrimination required to support effective public health decision making (Cooke et al., 2007; Feasey et al., 2012). Current whole genome sequencing (WGS)-based technologies can provide accurate and effective tools for the rapid subtyping of foodborne Salmonella (Brüssow et al., 2004; Ashton et al., 2017). However, in cases where a clearer distinction is needed among many closely related strains, e.g., delineating different outbreak strains or separating outbreak and sporadic strains, current WGS methodologies do not consistently meet the required need. Therefore, developing a parallel WGS-based subtyping tool may improve the differentiation among highly clonal Salmonella isolates.
The pan-genome of a bacterial species consists of a core genome which is the collection of coding sequences present in all members of the species and an accessory genome made up of sequences that are not consistently present among all members. The core genome defines the common attributes of the species whereas the accessory genome affect strain fitness by encoding proteins that influence virulence, antigenic structure, antimicrobial resistance, and metabolic characteristics. The accessory genome can represent up to 10% of the typical S. enterica genome (Jacobsen et al., 2011). These sequences include mobile genetic elements such as prophages, plasmids, transposons, and insertion elements (Thomson et al., 2008). Prophage sequences are common in the genome of Salmonella and their potential as molecular markers of genomic diversity has been previously investigated (Brüssow et al., 2004; Cooke et al., 2007; Feasey et al., 2012). For example, prophage sequences were used to discriminate between different field isolates of S. Typhimurium (Cooke et al., 2007). Moreover, two African and two global epidemic clades of S. Enteritidis were recently discriminated by their prophage repertoire (Feasey et al., 2016). Others have used a single prophage gene, the prophage integrase, to demonstrate genomic diversity in S. enterica (Colavecchio et al., 2017a). However, no single gene is likely to provide a comprehensive enough information for taxonomic analyses (Rohwer and Edwards, 2002) and is likely applicable only within a narrow spectrum of diversity such as within the same serovar of Salmonella (Colavecchio et al., 2017a).
Prophage sequences have been shown to encode virulence factors, toxins, and antimicrobial resistance genes (Brüssow et al., 2004; Colavecchio et al., 2017b) all of which contribute to diversity of strains. Therefore, we postulated that prophage features may be one of the principal drivers of diversification among and within serovars of Salmonella. To that end, we aimed to conduct a comprehensive characterization of prophage diversity in 151 serovars of S. enterica and to test the performance of the whole prophage repertoire as a measure of the host organism diversity. We tested the discriminative ability of this approach among S. enterica serovars, closely related isolates of the same serovar from different outbreaks and finally between predominant S. enterica serovars and other closely related members of the family Enterobacteriaceae including Proteus, Hafnia, and Citrobacter, which are sometimes misidentified as Salmonella isolates.
Materials and Methods
Bacterial Strains and Whole Genome Sequencing
A set of 1,826 bacterial strains was used in the current study. These strains included S. enterica isolates (n = 1,760) from 151 serovars (Table 1), S. bongori isolates (n = 2), Citrobacter amalonaticus (n = 6), C. freundii (n = 14), C. braakii (n = 5), C. koseri (n = 2), C. farmeri (n = 1), C. werkmanii (n = 1), other Citrobacter spp. (n = 9), Hafnia alvei (n = 11), Proteus mirabilis (n = 14), and P. vulgaris (n = 1). The strains were isolated from different sources: 527 from humans; 217 from domestic and wild animals; 117 from poultry; 267 from environmental samples; 48 from fish; 7 from dairies; 35 from fruits and vegetables; 17 from nuts or seeds, 15 from animal feed, and 576 from undetermined or unspecified food sources (Supplementary Table 1). The isolates originated from 27 countries and the Middle East. Although the majority of isolates came from Canada (n = 877) and the United States (n = 551), sizeable numbers were sourced from the Netherlands (n = 53), Jamaica (n = 40), France (n = 32), Switzerland (n = 13), and Scotland (n = 12). Fewer than 10 isolates came from each of the remaining 20 countries and the Middle East as shown in Supplementary Table 1. All S. enterica isolates were typed serologically by the classical Kauffman-White procedure in use by Salmonella reference laboratories around the world. Genomes of these isolates were sequenced at the EcoGenomics Analysis Platform of the Institute for Integrative and Systems Biology (IBIS; Université Laval) as previously described (Emond-Rheault et al., 2017). Briefly, 300 bp paired-end reads were generated on an Illumina MiSeq from large insert libraries to a sequencing depth of approximately 45X coverage on average per strain. The raw reads, in addition to the genomic and metadata, were submitted to the Salmonella Foodborne Syst-OMICS database (SalFoS; https://salfos.ibis.ulaval.ca/; Emond-Rheault et al., 2017).
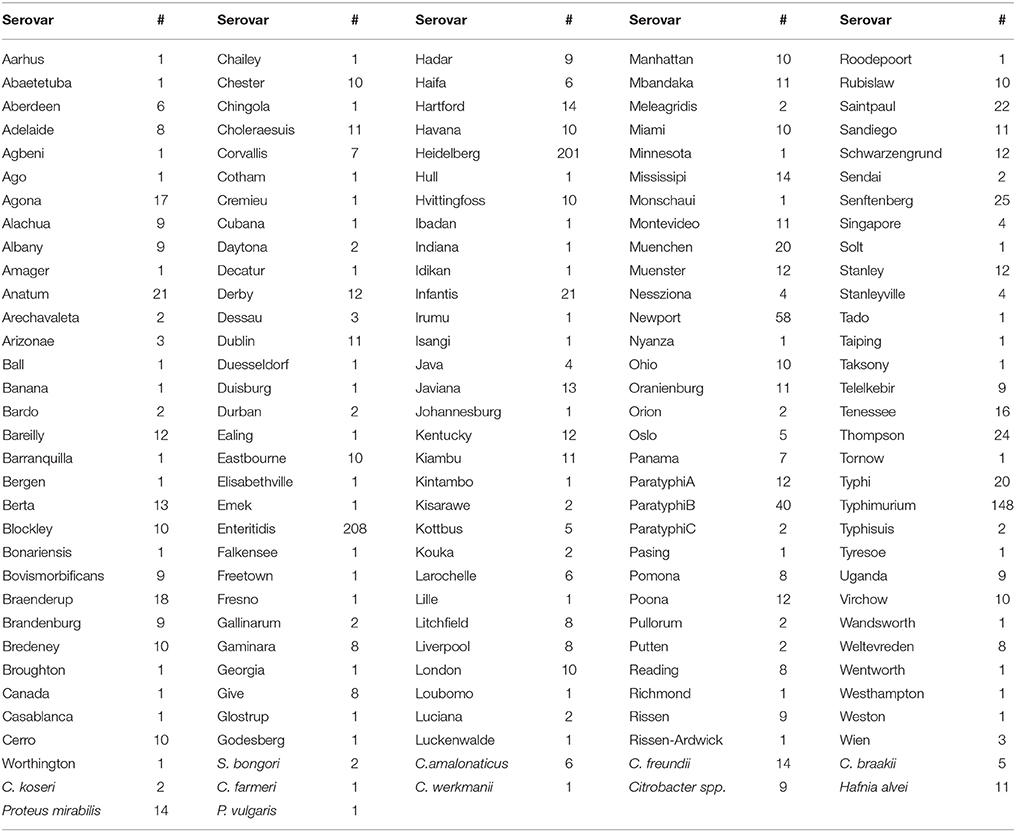
Table 1. Salmonella enterica serovars and non-Salmonella species with the corresponding number of genomes investigated in the current study.
Prophage Sequence Detection
Even though the Salmonella genomes used in this study were assembled previously using A5 (Tritt et al., 2012; Coil et al., 2015) at a high level of quality (Emond-Rheault et al., 2017), we decided to reassemble the raw data using SPAdes genome assembler version 3.10.1 with updates available at: http://bioinf.spbau.ru/en/spades (Bankevich et al., 2012). The raw paired-end Illumina reads were trimmed to a minimum PHRED quality score of Q10 from the 3′ end using the BBDuk tool (http://jgi.doe.gov/data-and-tools/bbtools/). Trimmed reads shorter than 64 bases were discarded and the remainders were merged using BBMerge (http://jgi.doe.gov/data-and-tools/bbtools/). Both paired and unpaired reads were kept for de novo assembly, which was performed using SPAdes genome assembler 3.10.1 algorithm (Bankevich et al., 2012). The quality of each assembled genome was assessed following analysis with the QUAST software (Gurevich et al., 2013) and all were satisfactory based on the number of contigs, size of the largest contig, number of bases in the assembly, and NG50 (data not shown). Prophage sequences within the assembled contigs of each genome were identified with the PHAge Search Tool Enhanced Release (PHASTER; Zhou et al., 2011; Arndt et al., 2016). All contigs <2 Kb were discarded before submitting the assembly to PHASTER, as PHASTER processes only contigs of length >= 2 kb.
Prophage Sequence Typing
We developed a prophage sequence typing procedure by clustering the identified prophages sequences present in the genomes. The prophage regions identified by PHASTER were extracted from each genome and every sequence was renamed by adding the sample name to the sequence header. All identified prophage regions from the strains analyzed were clustered using CD–HIT-EST (Fu et al., 2012) based on 90% identity and 90% prophage sequence length coverage. Increasing the stringency to identity and sequence coverage of 95, 99, and 100% did not improve the clustering efficiency at the serovar level (data not shown), hence the 90% threshold was selected. Due to the high genomic clonality of S. Enteritidis isolates we used 100% sequence identity and alignment coverage for comparing the prophage profiles of S. Enteritidis outbreak isolates. The representative sequence of each cluster generated by CD–HIT-EST was used to determine the prophage identity of that cluster by local alignment of the sequences against the PHASTER prophage virus database using BLASTX (Altschul et al., 1990). A prophage cluster matrix table was then constructed from the CD–HIT-EST and BLASTX results showing the prophage cluster number, the length and the identity of each prophage sequence detected in every analyzed genome (Supplementary Table 2).
Prophage Diversity and Composition Analyses
The prophage matrix table was fed to Quantitative Insights Into Microbial Ecology (QIIME) analytical platform (Caporaso et al., 2010) to determine the diversity of prophage repertoire within (alpha diversity) and among (beta diversity) the different strains in addition to prophage taxonomy summarization. To test the variability of prophage profiles between two or more groups of samples, the diversity of the identified prophage clusters was used to calculate the Bray-Curtis distances among different strains and the generated distances were employed in Unweighted Pair Group Method with Arithmetic mean (UPGMA) clustering. The generated UPGMA tree files were exported to the Interactive Tree of Life (iTOL) software (Letunic and Bork, 2011) for viewing and editing. The identified RE-2010 sequences in S. Enteritidis genomes from known outbreaks (n = 111) were extracted from PHASTER results and aligned with Multiple Alignment using Fast Fourier Transform (MAFFT; Katoh and Standley, 2013) tool from Galaxy platform (https://usegalaxy.org) and the alignment tree file was uploaded to iTOL platform for editing. We conducted an analysis of similarity (ANOSIM) on the prophage sequence profiles using QIIME where the p-value was calculated using 999 permutations; p-value <0.05 was considered significant. ANOSIM generates R-value that lies between 0 and +1 where the closer the value to +1 the higher the dissimilarity of the prophage profiles among the compared groups of isolates (i.e., among different serovars, outbreaks, or species) (Mottawea et al., 2016).
Furthermore, a supervised learning serovar classification using QIIME was conducted by training the supervised random forests classifier with the prophage clusters as predictors and the serovar name as the class labels. This supervised learning analysis generated a ranking of prophage clusters by importance, estimated the classifier out-of-bag error, and predicted the serovar label in addition to the probabilities of classification.
Results
Prophage Sequence Diversity in S. enterica Serovars
The prophage sequence diversity was characterized among the whole genome sequences of 1,760 S. enterica isolates with 86% or 1,508 isolates belonging to 151 serovars, while the serovar designations of 14% or 252 isolates were unknown. Overall, 11,297 discrete prophage sequences were identified among all the total genomes, with a median of 5 and interquartile range (IQR) of three prophage sequences per isolate. These sequences were grouped into 3,126 clusters based on 90% sequence identity and 90% long sequence coverage. No prophage sequence was detected in 3 S. enterica strains belonging to serovars Braenderup, Brandenburg and Typhimurium, despite a genome sequence coverage of 16, 22, and 19X, respectively. We identified only one prophage sequence in 12 strains (4 Havana, 3 Bareilly, and 1 strain from each of Gallinarum, Infantis, Liverpool, Putten, and Stanleyville serovars). In contrast, the highest number of prophage sequences in one genome (n = 15) was detected in a strain belonging to serovar Kisarawe. Prophage sequences were most prevalent in S. Typhimurium (148 strains) (Median ± IQR of 9 ± 2 prophages per isolate). The genomes of the other two common serovars; S. Enteritidis (208 strains) and S. Heidelberg (201 strains) carried 5 ± 1 and 6 ± 2 prophage sequences per isolate, respectively. S. Havana serovar (10 strains) had the fewest prophages (2 ± 1) (Figure 1 and Supplementary Table 3).
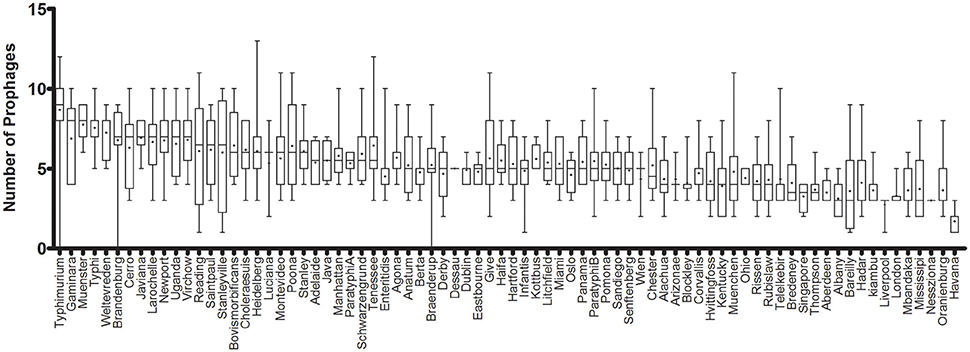
Figure 1. Prophage sequence diversity among different Salmonella enterica serovars. Only serovars with more than two isolates are represented. The columns are sorted in a decreasing order of the median number of prophages per serovar. The middle line represents the median and + represents the mean prophage number per genome, while the bar indicates the range of prophage numbers.
Prophage Sequence Profiles in S. enterica Genomes
In order to assign a prophage identity to every prophage sequence detected, the CD–HIT-identified reference sequence from each prophage cluster (see under Prophage sequence clustering, above) was aligned against the PHASTER prophages/virus database (last updated on Feb 9, 2017), using the BLASTX tool. Among the 151 serovars (n = 1,508 isolates), we detected 154 different prophages (Figure 2 and Supplementary Table 3). The most prevalent prophages identified among our cohort of S. enterica serovars were Escherichia phage MG1655 which was present in 82 out of 151 serovars, PHAGE Salmonella phage SPN3UB in 63 serovars, Phage Gifsy 2 in 61 serovars as well as Phage Gifsy 1 and Burkholderia phage BcepMu in 60 serovars (Figure 2 and Supplementary Table 3). We identified 41 different prophages in S. Typhimurium isolates (n = 144) which included highly prevalent prophages such as Burkholderia phage BcepMu (99.3% of the isolates), Gifsy-2 (95.8%), Gifsy-1 (91.7%), Salmonella phage SJ46 (70.1%), Enterobacteria phage SfV (65.3%), Escherichia phage EB49 (CFT073; 61.8%), Escherichia phage MG1655 (59.7%), and Salmonella phage ST64B (50.7%). In the case of S. Enteritidis (n = 208), we identified 21 different prophages, while the serovar Heidelberg (n = 196) harbored 26 different prophages. The predominant prophages in S. Enteritidis were Erwinia phage vB EamP S6 (96.2%), Gifsy-2 (95.8%), Salmonella phage SJ46 (88.5%), Salmonella phage RE-2010 (81.3%), and Salmonella phage ST64B (61.5%), while seven prophages were the most common among Heidelberg isolates: Burkholderia phage BcepMu (100%), Gifsy-2 (97.4%), Salmonella phage 118970 sal4 (92.3%), Salmonella phage 118970 sal3 (79.6%), Escherichia phage MG1655 (64.8%), Enterobacteria phage UAB Phi20 (63.3%), and Escherichia phage EB49 (53.6%).
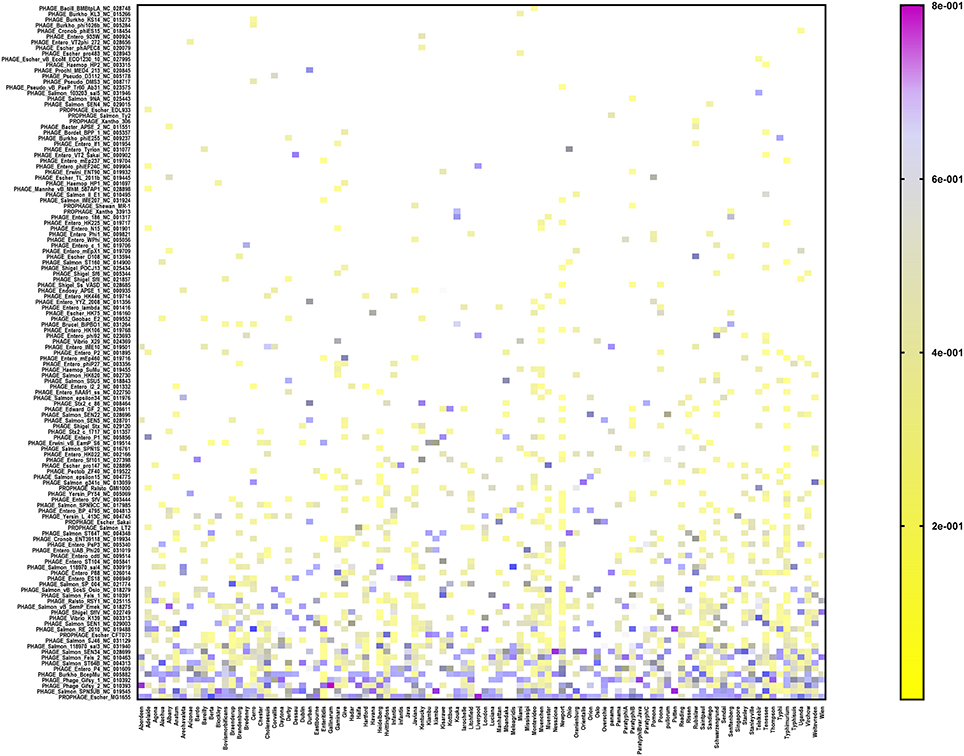
Figure 2. Heat map showing the prophage repertoire in different Salmonella enterica serovars. The color scale denotes the relative length of each prophage compared to the collective prophage sequences detected in each serovar with the violet color denoting maximum contribution of one prophage (i.e., up to 80%). Prophages are sorted according to their prevalence among different serovars in a decreasing order from the bottom to the top of the figure.
Exploiting Prophage Sequence Diversity for Salmonella enterica Subtyping
In order to assess the performance of genomic prophage sequence diversity as a discriminatory marker for the different Salmonella serovars, we calculated Bray-Curtis distance among the prophage profiles of different strains and the generated distance matrix was used to construct a phylogenetic tree of the strains. In order to reduce the bias generated by low isolate number and prophages that may be detected due to analytical errors, this analysis included those serovars that had eight or more Salmonella strains (n = 1,350 isolates) and by using only prophage clusters detected in at least four strains. We found that strains within each serovar clustered separately from strains of other serovars as indicated by the constructed phylogenetic tree (Figure 3). Clustering of serovars based on prophages was found to be statistically significant following ANOSIM evaluation (R = 0.88, p = 0.001). In order to test the ability to predict the S. enterica serovar from the prophage sequences, we also conducted a supervised machine learning algorithm using random forests classifier and out-of-the-bag prediction to estimate the generalization error. The estimated generalization error was 0.15, while the baseline error (for random guessing) was 0.85 and the ratio of baseline error to the observed error was 5.38. The model confusion matrix, cv_probabilities and mislabelling matrix are detailed in Supplementary Tables 4–6. In general, these results indicate a significant correlation between the identified prophage profiles and the genome diversity of different S. enterica serovars.
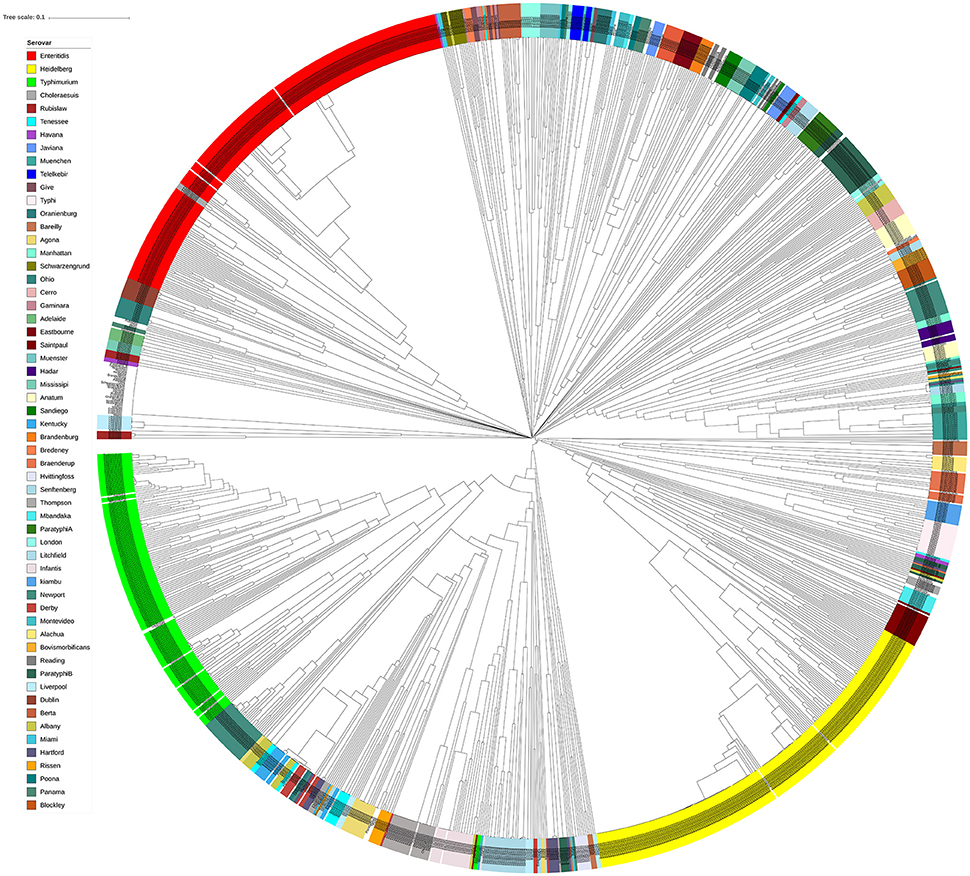
Figure 3. Isolates of different Salmonella serovars clustered separately based on their prophage sequence diversity. Bray-Curtis distances among 1,427 Salmonella isolates were calculated based on genomic prophage sequences diversity and were applied in Unweighted Pair Group Method with arithmetic mean (UPGMA) hierarchical clustering using Quantitative Insights Into Microbial Ecology (QIIME) pipeline. Analysis of Similarity (ANOSIM) R = 0.884; p = 0.001.
Prophage Sequence Diversity Differentiates Salmonella enterica Serovar Enteritidis Outbreak Isolates
Salmonella enterica serovars are characterized by a high genetic clonality. For this reason, conventional typing methods such as PFGE cannot reliably differentiate between closely related strains (Bekal et al., 2016). Although typing by SNPs has been successfully used to differentiate strains of highly clonal serovars, such as S. Enteritidis (Ogunremi et al., 2014; Ashton et al., 2016) the high resolution does not always result in a separation of outbreak and sporadic strains. For these reasons, development of an accurate, parallel subtyping method should provide additional tools to discriminate bacterial strains. To this end, we investigated the correlation between prophage sequence profiles and the genome diversity among 111 S. Enteritidis strains isolated during seven outbreaks. A total of 607 prophage sequences was detected in the genomes of these strains. The identified prophage sequences were clustered based on 100% identity and sequence coverage. A UPGMA clustering tree was generated based on Bray-Curtis distances among the prophage profiles of these strains. We identified 43 prophage sequence clusters that belong to five different prophages in our dataset. The UPGMA clustering resulted in a complete separation of these strains into seven clusters with each cluster specific to an outbreak, except only one strain isolated from outbreak 7 that clustered with outbreak 4 (Figure 4; ANOSIM R = 0.98; p = 0.001). The large Gifsy-2 prophage fragment was consistently detected in all isolates (100%, fragment length = 31,114–31,120 bases). The small Gifsy-2 prophage fragment (8,541 bases) was also very prevalent (93%) but was missing in a few isolates. Similarly, prophage Erwinia phage vB was present in almost all the isolates (99%) either as a small (13,087 bases), or large (25,792 bases) fragment. On the other hand, four different sequence variants of prophage ST64B were detected in our Enteritidis dataset (Image 1): one variant was specific to outbreaks 2 and 3 (length = 34,766 bases), while the other three variants were distributed among the isolates of outbreaks 1 and 5 (lengths = 50,483, 54,013, and 64,334 bases). We did not detect phage ST64B sequence in strains from outbreaks 4, 6, and 7. Interestingly, the prophage RE2010 was detected as six different clusters (Figure 5): one specific for each of the outbreaks 2, 3, 4, 5, 6, and 7, whereas this prophage was not detected in outbreak 1 (Figure 4). Finally, we did not detect any sequence corresponding to the prophage SJ46 in outbreak 6 (Figure 4), but it was identified in other outbreaks with a length of 8,109 bases. These findings indicate that prophage sequence profiles distinguished among the different S. Enteritidis subtypes responsible for distinct foodborne outbreaks.
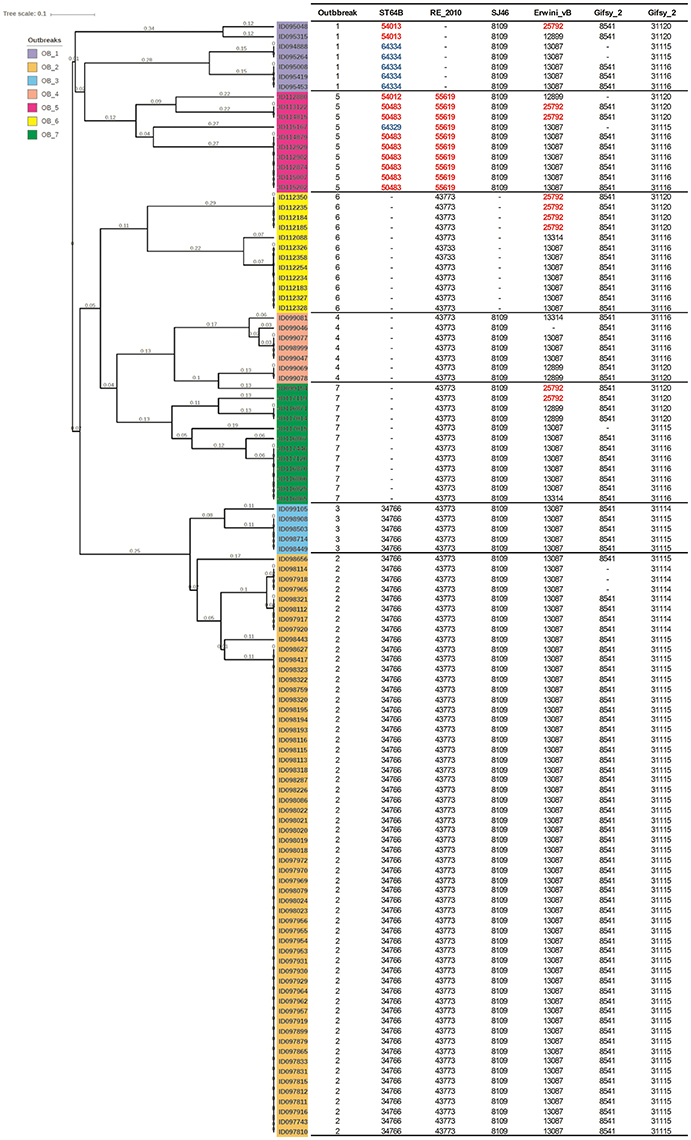
Figure 4. Prophage sequence profiles discriminated among Salmonella Enteritidis isolates from seven outbreaks. Bray-Curtis distances among S. Enteritidis strains obtained from seven outbreaks (n = 111) were calculated based on prophage sequence diversity and were applied in Unweighted Pair Group Method with Arithmatic Mean (UPGMA) hierarchical clustering using Quantitative Insights Into Microbial Ecology (QIIME) pipeline. The numbers in the table refer to the length of the prophage region detected, while the red and blue colors indicate different length variants of the same prophage. Numbers on the tree branches refer to the branch length. Analysis of Similarity (ANOSIM) R = 0.98; p = 0.001.
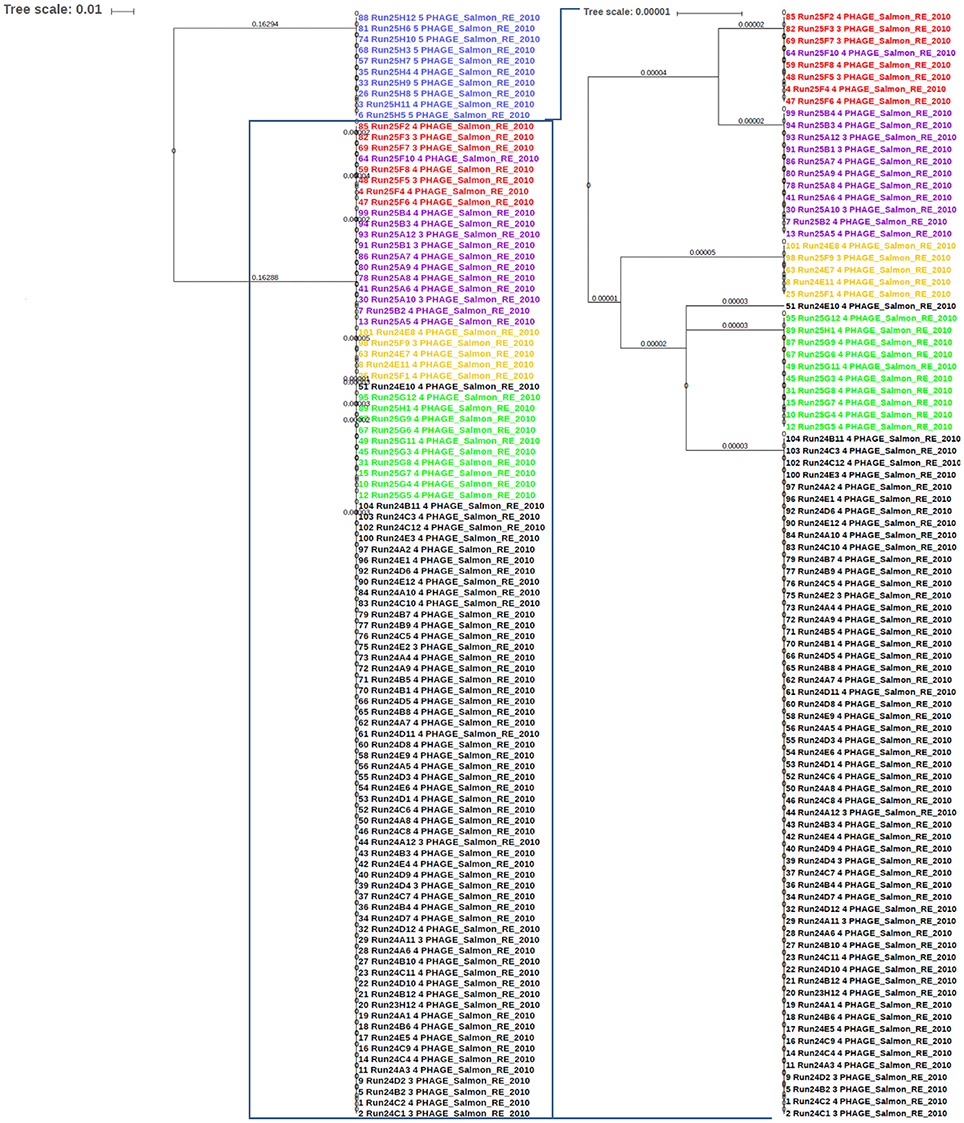
Figure 5. The sequence of phage RE-2010 distinguished among Salmonella Enteritidis isolates from different outbreaks. The identified RE-2010 sequences in S. Enteritidis genomes involved in known outbreaks (n = 111) were extracted from PHASTER results and aligned with Multiple Alignment using Fast Fourier Transform (MAFFT) tool from Galaxy platform (https://usegalaxy.org). The alignment tree was exported to the Interactive Tree of Life (iTOL) software for viewing and editing. Numbers on branches represent the corresponding branch length. Blue color represents strains of outbreak 6; Red for outbreak 4; Purple for outbreak 8, Orange for outbreak 3, Green for outbreak 7, and Black for outbreak 2.
Non-typhoidal Salmonella Isolates Harbour Prophage Sequence Profiles Distinct From Other Closely Related Enterobacteriaceae
The bacterial set in this study included organisms commonly misidentified as S. enterica strains such as Citrobacter, Proteus, and Hafnia, as well as S. bongori. To investigate the genomic prophage sequence profiles in these pathogens as compared to S. enterica, we extracted the prophage clusters identified in these bacteria along with those identified in S. Enteritidis, S. Heidelberg and S. Typhimurium isolates, as these serovars represent the majority of our S. enterica set. UPGMA clustering showed that these organisms which can cause false positives clustered separately from S. Enteritidis, S. Heidelberg and S. Typhimurium isolates (Figure 6). ANOSIM R-value was 0.86 with p-value of 0.001. In contrast to Salmonella isolates, we observed that the prophage profiles of these bacteria were highly variable among strains analyzed with the exception of P. mirabilis, which were clustered together. This conservation among P. mirabilis isolates mainly arises from one prophage cluster identified as Shewanella phage 1/41 NC 025458 which was present in 13 out of the 14 isolates (Supplementary Table 7). These results revealed that the prophage sequences integrated in the genome of S. enterica isolates are distinct from those harbored by the genomes of other closely related bacteria of the family Enterobacteriaceae.
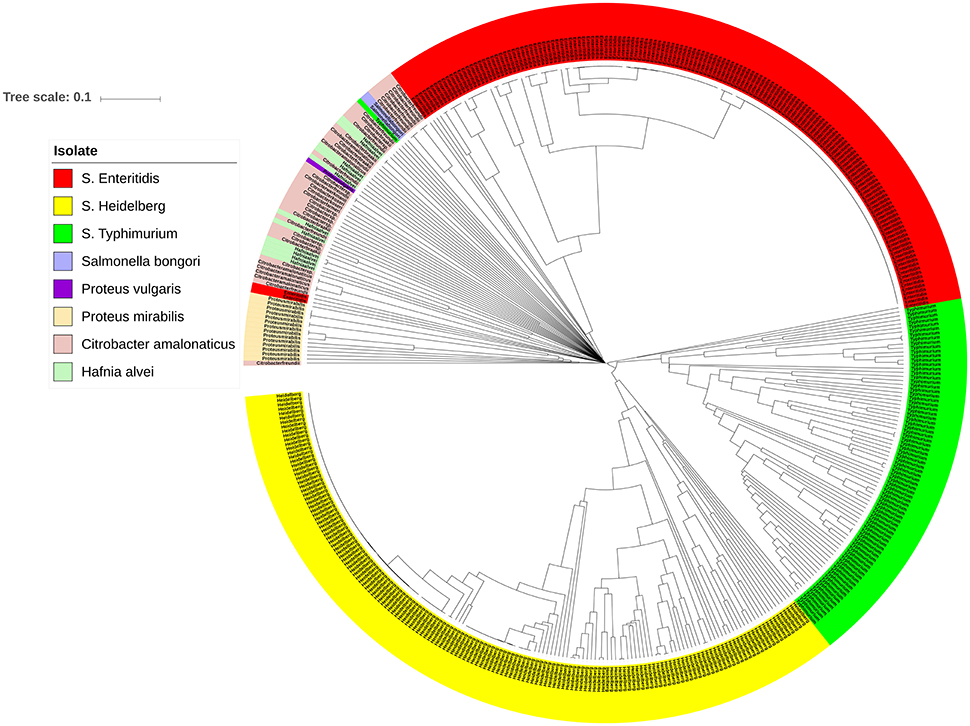
Figure 6. Prophage sequence profiles distinguished between Salmonella enterica and other related bacteria. Bray-Curtis distances among Salmonella and non-Salmonella isolates (n = 453) were calculated based on genomic prophage sequences diversity and were applied in Unweighted Pair Group Method with arithmetic mean (UPGMA) hierarchical clustering using Quantitative Insights Into Microbial Ecology (QIIME) pipeline. Analysis of Similarity (ANOSIM) R = 0.86; p = 0.001.
Discussion
Bacteriophages appear to be significant drivers of the evolution of pathogenic bacteria including S. enterica (Figueroa-Bossi and Bossi, 1999; Figueroa-Bossi et al., 2001; Davies et al., 2016; Diard et al., 2017). Accordingly, prophage sequences constitute a significant component of Salmonella accessory genomes (Ogunremi et al., 2014; Owen et al., 2017) and have been reported to enable discrimination among closely related Salmonella lineages (Kingsley et al., 2009; Okoro et al., 2015; Colavecchio et al., 2017a; Owen et al., 2017). This discriminatory power of prophage sequences has been demonstrated also for two different serovars of Streptococcus pyogenes (Brüssow et al., 2004).
The current study reports a pipeline for the rapid identification of genomic prophage sequences in Salmonella and to use them to discriminate between different epidemiological categories and serovars. Previous efforts to characterize bacterial strains and distinguish one from another based on their prophage content have followed different approaches. Some studies have relied on descriptive methods that compare the number of prophages and their identity among a certain number of bacterial strains within specific taxonomic groups (Okoro et al., 2015; Zheng et al., 2017). In general, these studies focussed on prevalent and intact prophage regions while neglecting less predominant and incomplete ones. Some prophages exhibit sequence variants that are differentially integrated among distinct host organisms and serovars (Mmolawa et al., 2003; Bobay et al., 2013; Hiley et al., 2014; Zheng et al., 2017). For example, Bobay and coauthors have detected different prophage sequences in the whole genome of 20 strains of S. enterica and classified them based on their sequence similarity in order to understand prophage integration patterns and their genetic adaptation to host genomes (Bobay et al., 2013). These sequence variants may arise from modular exchange with other prophage DNA, mutation, adaptational shift, and/or drift (Brüssow et al., 2004; Boyd et al., 2012; Omer et al., 2017) and these changes can be expected to have evolutionary implications (Diard et al., 2017). To that end, prophage diversity within bacterial strains does not only rely on the presence or absence of a certain prophage, but also on the conservation of the prophage sequences. Few studies have targeted these sequence variations in certain phage genes to assess the diversity of both phages and their host genomes (Adriaenssens and Cowan, 2014; Colavecchio et al., 2017a). Since there is no universal phage gene (Rohwer and Edwards, 2002), relying on one gene will narrow the spectrum of the discriminative approach to phages that have this gene. Additionally, each prophage sequence feature represents a specific evolutionary event in the bacterial genome (Brüssow et al., 2004).
Here, we analyzed every sequence detected by PHASTER (Arndt et al., 2016) as a distinct operational unit that has its own discriminative weight among Salmonella strains. Moreover, we identified prophage sequence clusters based on the similarity and coverage lengths so that sequence variants are analyzed as distinct operational units. It should be noted that the performance of the current pipeline is limited by the sensitivity and the accuracy of the incorporated assembly and prophage detection tools. For example, we may lose some prophage regions because of improper sequence assembly. Furthermore, PHASTER appears to have the same sensitivity index (85.4%) as its predecessor, PHAST (Zhou et al., 2011). Therefore, the performance errors of the genome assembly and prophage detection tools involved in our pipeline could be reflected on the sensitivity and the prediction accuracy of our approach. Despite this potential limitation, the use of PHASTER proved to be adequately sensitive and the procedure outlined highly discriminative of epidemiologically unrelated strains of Enteritidis.
Salmonella populations are characterized by highly variable prophage profiles (Figueroa-Bossi et al., 2001; Brüssow et al., 2004; Owen et al., 2017). This is largely consistent with our finding that the prophage repertoires identified in this study are highly variable among different Salmonella serovars. The number of prophage sequences detected ranged from 1 to 15 regions per genome. Even within the same serovar, there is a high variability in prophage combinations between strains. For example, we detected a median of 9 ± 2 (IQR) prophages per genome in S. Typhimurium isolates. Eight of these prophages (Burkholderia phage BcepMu, Gifsy-2, Gifsy-1, Salmonella phage SJ46, Enterobacteria phage SfV, Escherichia phage EB49, Escherichia phage MG1655, Salmonella phage ST64B) are prevalent among S. Typhimurium genomes. Gifsy-2, Gifsy-1, and ST64B are known to be common among different S. Typhimurium lineages (Kingsley et al., 2009; Okoro et al., 2015; Owen et al., 2017). Certain prophages, such as Gifsy-2, are highly prevalent among different Salmonella serovars. We detected Gifsy-2 in 61 serovars of S. enterica and it is present in more than 95% of S. Enteritidis, S. Heidelberg and S. Typhimurium isolates. Still, Gifsy-2 exhibits three different sequence variants among the three serovars (Image 2). This result is consistent with Colavecchio et al. who reported that one gene of this prophage was sufficient to differentiate between S. Enteritidis and S. Heidelberg isolates (Colavecchio et al., 2017a). In addition to the genetic variants of common prophages, newly acquired prophages or lineage-specific prophages may contribute to strain divergence as shown recently with S. Newport (Zheng et al., 2017). In general, this variability in prophage sequence types illustrates the correlation between prophage repertoires and the genome diversity of different Salmonella serovars.
Foodborne Salmonellosis is an important concern for public health (Ziebell et al., 2017). To reduce the impact of outbreaks caused by foodborne pathogens, timely subtyping of isolates to incriminate sources of contamination is crucial. Conventional Salmonella typing methods such as PFGE, phage typing (PT), MLVA, and multiple amplification of phage locus typing (MAPLT) do not always provide the required level of discrimination (Assis et al., 2017; Ziebell et al., 2017). It was proposed previously that prophage composition could differentiate between S. Enteritidis subtypes during foodborne outbreaks (Ogunremi, 2013). Although prophage sequences are highly variable among Salmonella and can sometimes be transient and mobile (Owen et al., 2017), herein we have established that these repertoires correlate with outbreak strains of Salmonella with these results illustrate how prophage sequences can accurately distinguish between epidemiologically unrelated isolates. While Gifsy-2 prophage sequence is common among all the isolates from different outbreaks as noted by others (Colavecchio et al., 2017a), three other prophages (RE2010, ST64B, and SJ46) exhibit outbreak-specific sequences and profiles. Interestingly, a single prophage sequence (phage RE2010) was enough to differentiate between the investigated seven outbreaks. This finding supports a previous report on the contribution of RE2010 to separate S. Enteritidis isolates with the same PFGE pattern (Allard et al., 2013) but considerably expanded on previous observations by showing a common prophage profile among isolates recovered from each outbreak of S. Enteritidis, which distinguished the different outbreaks. Furthermore, prophages ST64B and SJ46 have been shown previously to be present as multiple variants among closely related S. enterica genotypes of the same serovar (Mmolawa et al., 2003; Hiley et al., 2014; Zheng et al., 2017). Therefore, we conclude that prophage diversity can serve to differentiate epidemiologically unrelated subtypes of S. Enteritidis. In addition, considering that single nucleotide polymorphism (SNP) in a 60-loci test can differentiate between unrelated S. Enteritidis (Ogunremi et al., 2014), detecting SNPs within the sequence of universally distributed prophages, such as Gifsy-2 or RE2010, may provide a synergistic mean of differentiating S. Enteritidis subtypes during foodborne outbreaks.
One difficulty in the detection of foodborne Salmonella is the misidentification of strains of Citrobacter, Hafnia, and Proteus spp. as Salmonella (Muldoon et al., 2007; Fang et al., 2009; Margot et al., 2013). The results of this study revealed that the prophage profiles in these false positive strains are completely different from those of S. enterica isolates. In contrast to Salmonella prophages, which are characterized by high degree of conservation within the same serovar, the prophage profiles of Citrobacter and Hafnia spp. are characterized by high degree of variability among strains. For P. mirabilis, only one prophage remnant sequence is common among its isolates, while the other sequences are highly variable. Therefore, it is clear that prophage sequences could provide specific S. enterica targets for development of inclusive and exclusive detection tools of foodborne Salmonella pathogens.
In conclusion, this study reports a new pipeline for the detection of prophage sequence diversity in S. enterica. The diversity of the prophage sequences described here was found to be sufficient in differentiating among S. enterica serovars, between genetically related isolates from different epidemiological events and between Salmonella and Citrobacter, Hafnia, and Proteus. Our data reveal a high diversity among S. enterica prophages which is herein applied to develop a novel subtyping tool for identifying S. enterica clusters.
Author Contributions
WM developed the prophage sequence analyses pipeline, conducted the data analyses, prepared the figures and tables and drafted the manuscript; M-OD wrote the pipeline script for prophage sequence analyses; AD, VU, and HH contributed to data analyses; JJ, LF, J-GE-R, JH, IK-I, BB, and RL developed the whole genome sequences of all the bacterial isolates, developed, curated, and maintained the SalFos database; AG, EB, EF, GA, JTW, SG, MW, FD, SM, SB, and LG provided bacterial isolates for whole genome sequencing and provided metadata; SB, RL, and LG were involved in the study design, data analysis and review; DO conceived the study and participated in data analysis, development of manuscript and review. All the authors revised and approved the manuscript.
Funding
This study was funded by Genome Canada grant.
Conflict of Interest Statement
The authors declare that the research was conducted in the absence of any commercial or financial relationships that could be construed as a potential conflict of interest.
Acknowledgments
We would like to greatly thank the members of the genomics analysis and bioinformatics platforms at IBIS. SM holds a Tier 1 Canada Research Chair in Bacteriophages. WM is funded by Genome Canada. DO's contribution was funded by the Canadian Food Inspection Agency. We would like to express our special thanks to Sandeep Tamber, Danielle Malo, Ann Perets, Kenneth E. Sanderson, and Roger Stephan for providing bacterial strains.
Supplementary Material
The Supplementary Material for this article can be found online at: https://www.frontiersin.org/articles/10.3389/fmicb.2018.00836/full#supplementary-material
References
Adriaenssens, E. M., and Cowan, D. A. (2014). Using signature genes as tools to assess environmental viral ecology and diversity. Appl. Environ. Microbiol. 80, 4470–4480. doi: 10.1128/AEM.00878-14
Allard, M. W., Luo, Y., Strain, E., Pettengill, J., Timme, R., Wang, C., et al. (2013). On the evolutionary history, population genetics and diversity among isolates of Salmonella Enteritidis PFGE pattern JEGX01.0004. PLoS ONE 8:e55254. doi: 10.1371/journal.pone.0055254
Altschul, S. F., Gish, W., Miller, W., Myers, E. W., and Lipman, D. J. (1990). Basic local alignment search tool. J. Mol. Biol. 215, 403–410. doi: 10.1016/S0022-2836(05)80360-2
Arndt, D., Grant, J. R., Marcu, A., Sajed, T., Pon, A., Liang, Y., et al. (2016). PHASTER: a better, faster version of the PHAST phage search tool. Nucleic Acids Res. 44, W16–W21. doi: 10.1093/nar/gkw387
Ashton, P. M., Nair, S., Peters, T. M., Bale, J. A., Powell, D. G., Painset, A., et al. (2016). Identification of Salmonella for public health surveillance using whole genome sequencing. PeerJ 4:e1752. doi: 10.7717/peerj.1752
Ashton, P. M., Owen, S. V., Kaindama, L., Rowe, W. P. M., Lane, C. R., Larkin, L., et al. (2017). Public health surveillance in the UK revolutionises our understanding of the invasive Salmonella Typhimurium epidemic in Africa. Genome Med. 9:92. doi: 10.1186/s13073-017-0480-7
Assis, F. E. A., Dallagassa, C. B., Farah, S. M. S. S., Souza, E. M., Pedrosa, F. O., Chubatsu, L. S., et al. (2017). Molecular characterisation of Salmonella strains isolated from outbreaks and sporadic cases of diarrhoea occurred in Parana State, South of Brazil. Epidemiol. Infect 145, 1953–1960. doi: 10.1017/S0950268817000619
Bankevich, A., Nurk, S., Antipov, D., Gurevich, A. A., Dvorkin, M., Kulikov, A. S., et al. (2012). SPAdes: a new genome assembly algorithm and its applications to single-cell sequencing. J. Comput. Biol. 19, 455–477. doi: 10.1089/cmb.2012.0021
Bekal, S., Berry, C., Reimer, A. R., Van Domselaar, G., Beaudry, G., Fournier, E., et al. (2016). Usefulness of high-quality core genome single-nucleotide variant analysis for subtyping the highly clonal and the most prevalent Salmonella enterica serovar Heidelberg clone in the context of outbreak investigations. J. Clin. Microbiol. 54, 289–295. doi: 10.1128/JCM.02200-15
Bobay, L. M., Rocha, E. P., and Touchon, M. (2013). The adaptation of temperate bacteriophages to their host genomes. Mol. Biol. Evol. 30, 737–751. doi: 10.1093/molbev/mss279
Boyd, E. F., Carpenter, M. R., and Chowdhury, N. (2012). Mobile effector proteins on phage genomes. Bacteriophage 2, 139–148. doi: 10.4161/bact.21658
Brüssow, H., Canchaya, C., and Hardt, W. D. (2004). Phages and the evolution of bacterial pathogens: from genomic rearrangements to lysogenic conversion. Microbiol. Mol. Biol. Rev. 68, 560–602. doi: 10.1128/MMBR.68.3.560-602.2004
Caporaso, J. G., Kuczynski, J., Stombaugh, J., Bittinger, K., Bushman, F. D., Costello, E. K., et al. (2010). QIIME allows analysis of high-throughput community sequencing data. Nat. Methods 7, 335–336. doi: 10.1038/nmeth.f.303
Coil, D., Jospin, G., and Darling, A. E. (2015). A5-miseq: an updated pipeline to assemble microbial genomes from Illumina MiSeq data. Bioinformatics 31, 587–589. doi: 10.1093/bioinformatics/btu661
Colavecchio, A., Cadieux, B., Lo, A., and Goodridge, L. D. (2017b). Bacteriophages contribute to the spread of antibiotic resistance genes among foodborne pathogens of the enterobacteriaceae family - A review. Front. Microbiol. 8:1108. doi: 10.3389/fmicb.2017.01108
Colavecchio, A., D'Souza, Y., Tompkins, E., Jeukens, J., Freschi, L., Emond-Rheault, J. G., et al. (2017a). Prophage integrase typing is a useful indicator of genomic diversity in Salmonella enterica. Front. Microbiol. 8:1283. doi: 10.3389/fmicb.2017.01283
Cooke, F. J., Wain, J., Fookes, M., Ivens, A., Thomson, N., Brown, D. J., et al. (2007). Prophage sequences defining hot spots of genome variation in Salmonella enterica serovar Typhimurium can be used to discriminate between field isolates. J. Clin. Microbiol. 45, 2590–2598. doi: 10.1128/JCM.00729-07
Davies, E. V., Winstanley, C., Fothergill, J. L., and James, C. E. (2016). The role of temperate bacteriophages in bacterial infection. FEMS Microbiol. Lett. 363:fnw015. doi: 10.1093/femsle/fnw015
Diard, M., Bakkeren, E., Cornuault, J. K., Moor, K., Hausmann, A., Sellin, M. E., et al. (2017). Inflammation boosts bacteriophage transfer between Salmonella spp. Science 355, 1211–1215. doi: 10.1126/science.aaf8451
Emond-Rheault, J. G., Jeukens, J., Freschi, L., Kukavica-Ibrulj, I., Boyle, B., Dupont, M. J., et al. (2017). A Syst-OMICS approach to ensuring food safety and reducing the economic burden of salmonellosis. Front. Microbiol. 8:996. doi: 10.3389/fmicb.2017.00996
Fang, S. B., Tseng, W. Y., Lee, H. C., Tsai, C. K., Huang, J. T., and Hou, S. Y. (2009). Identification of Salmonella using colony-print and detection with antibody-coated gold nanoparticles. J. Microbiol. Methods 77, 225–228. doi: 10.1016/j.mimet.2009.02.008
Feasey, N. A., Dougan, G., Kingsley, R. A., Heyderman, R. S., and Gordon, M. A. (2012). Invasive non-typhoidal salmonella disease: an emerging and neglected tropical disease in Africa. Lancet 379, 2489–2499. doi: 10.1016/S0140-6736(11)61752-2
Feasey, N. A., Hadfield, J., Keddy, K. H., Dallman, T. J., Jacobs, J., Deng, X., et al. (2016). Distinct Salmonella Enteritidis lineages associated with enterocolitis in high-income settings and invasive disease in low-income settings. Nat. Genet. 48, 1211–1217 doi: 10.1038/ng.3644
Figueroa-Bossi, N., and Bossi, L. (1999). Inducible prophages contribute to Salmonella virulence in mice. Mol. Microbiol. 33, 167–176. doi: 10.1046/j.1365-2958.1999.01461.x
Figueroa-Bossi, N., Uzzau, S., Maloriol, D., and Bossi, L. (2001). Variable assortment of prophages provides a transferable repertoire of pathogenic determinants in Salmonella. Mol. Microbiol. 39, 260–271. doi: 10.1046/j.1365-2958.2001.02234.x
Fu, L., Niu, B., Zhu, Z., Wu, S., and Li, W. (2012). CD-HIT: accelerated for clustering the next-generation sequencing data. Bioinformatics 28, 3150–3152. doi: 10.1093/bioinformatics/bts565
Grimont, P., and Weill, F. X. (2007). Antigenic Formulae of the Salmonella Serovars, 9th Edn. Geneva: WHO Collaborating Centre for Reference and Research on Salmonella. Available online at: https://www.pasteur.fr/sites/default/files/veng_0.pdf
Gurevich, A., Saveliev, V., Vyahhi, N., and Tesler, G. (2013). QUAST: quality assessment tool for genome assemblies. Bioinformatics 29:1072–1075. doi: 10.1093/bioinformatics/btt086
Hiley, L., Fang, N. X., Micalizzi, G. R., and Bates, J. (2014). Distribution of Gifsy-3 and of variants of ST64B and Gifsy-1 prophages amongst Salmonella enterica Serovar Typhimurium isolates: evidence that combinations of prophages promote clonality. PLoS ONE 9:e86203. doi: 10.1371/journal.pone.0086203
Jacobsen, A., Hendriksen, R. S., Aaresturp, F. M., Ussery, D. W., and Friis, C. (2011). The Salmonella enterica pan-genome. Microb. Ecol. 62, 487–504. doi: 10.1007/s00248-011-9880-1
Katoh, K., and Standley, D. M. (2013). MAFFT multiple sequence alignment software version 7, Improvements in performance and usability. Mol. Biol. Evol. 30, 772–780. doi: 10.1093/molbev/mst010
Kingsley, R. A., Msefula, C. L., Thomson, N. R., Kariuki, S., Holt, K. E., Gordon, M. A., et al. (2009). Epidemic multiple drug resistant Salmonella Typhimurium causing invasive disease in sub-Saharan Africa have a distinct genotype. Genome Res. 19, 2279–2287. doi: 10.1101/gr.091017.109
Letunic, I., and Bork, P. (2011). Interactive tree of life v2: online annotation and display of phylogenetic trees made easy. Nucleic Acids Res. 39, W475–W478. doi: 10.1093/nar/gkr201
Margot, H., Stephan, R., Guarino, S., Jagadeesan, B., Chilton, D., O'Mahony, E., et al. (2013). Inclusivity, exclusivity and limit of detection of commercially available real-time PCR assays for the detection of Salmonella. Int. J. Food Microbiol. 165, 221–226. doi: 10.1016/j.ijfoodmicro.2013.05.012
Mmolawa, P. T., Schmieger, H., and Heuzenroeder, M. W. (2003). Bacteriophage ST64B, a genetic mosaic of genes from diverse sources isolated from Salmonella enterica serovar typhimurium DT 64. J. Bacteriol. 185, 6481–6485. doi: 10.1128/JB.185.21.6481-6485.2003
Mottawea, W., Chiang, C. K., Mühlbauer, M., Starr, A. E., Butcher, J., Abujamel, T., et al. (2016). Altered intestinal microbiota-host mitochondria crosstalk in new onset Crohn's disease. Nat. Commun. 7:13419. doi: 10.1038/ncomms13419
Muldoon, M. T., Teaney, G., Li, J., Onisk, D. V., and Stave, J. W. (2007). Bacteriophage-based enrichment coupled to immunochromatographic strip-based detection for the determination of Salmonella in meat and poultry. J. Food Prot. 70, 2235–2242. doi: 10.4315/0362-028X-70.10.2235
Ogunremi, D. (2013). “Strategy for developing a molecular subtyping tool for a foodborne bacterial pathogen using a whole genome analysis: the case of Salmonella Enteritidis,” in 63rd Annual Conference of the Canadian Society of Microbiologists (Ottawa, ON), 199.
Ogunremi, D., Devenish, J., Amoako, K., Kelly, H., Dupras, A. A., Belanger, S., et al. (2014). High resolution assembly and characterization of genomes of Canadian isolates of Salmonella Enteritidis. BMC Genomics 15:713. doi: 10.1186/1471-2164-15-713
Ogunremi, D., Kelly, H., Dupras, A. A., Belanger, S., and Devenish, J. (2014). Development of a new molecular subtyping tool for Salmonella enterica serovar Enteritidis based on single nucleotide polymorphism genotyping using PCR. J. Clin. Microbiol. 52, 4275–4285. doi: 10.1128/JCM.01410-14
Okoro, C. K., Barquist, L., Connor, T. R., Harris, S. R., Clare, S., Stevens, M. P., et al. (2015). Signatures of adaptation in human invasive Salmonella Typhimurium ST313 populations from sub-Saharan Africa. PLoS Negl. Trop. Dis. 9:e0003611. doi: 10.1371/journal.pntd.0003611
Omer, S., Harlow, T. J., and Gogarten, J. P. (2017). Does sequence conservation provide evidence for biological function? Trends Microbiol. 25, 11–18. doi: 10.1016/j.tim.2016.09.010
Owen, S. V., Wenner, N., Canals, R., Makumi, A., Hammarlöf, D. L., Gordon, M. A., et al. (2017). Characterization of the prophage repertoire of African Salmonella Typhimurium ST313 reveals high levels of spontaneous induction of novel phage BTP1. Front. Microbiol. 8:235. doi: 10.3389/fmicb.2017.00235
Public Health Agency of Canada [PHAC] (2012). In: National Enteric Surveillance Program - Annual Summary. Available online at: http://publications.gc.ca/collections/collection_2014/aspc-phac/HP37-15-2012-eng.pdf
Rohwer, F., and Edwards, R. (2002). The phage proteomic tree: a genome-based taxonomy for phage. J. Bacteriol. 184, 4529–4535. doi: 10.1128/JB.184.16.4529-4535.2002
Thomson, N. R., Clayton, D. J., Windhorst, D., Vernikos, G., Davidson, S., Churcher, C., et al. (2008). Comparative genome analysis of Salmonella Enteritidis PT4 and Salmonella Gallinarum 287/91 provides insights into evolutionary and host adaptation pathways. Genome Res. 18, 1624–1637. doi: 10.1101/gr.077404.108
Tritt, A., Eisen, J. A., Facciotti, M. T., and Darling, A. E. (2012). An integrated pipeline for de novo assembly of microbial genomes. PLoS ONE 7:e42304. doi: 10.1371/journal.pone.0042304
WHO (2016) WHO Estimates of the Global Burden of Foodborne Diseases. Foodborne Disease Burden Epidemiology Reference Group 2007-2015. Available online at: http://www.who.int/foodsafety/areas_work/foodborne-diseases/ferg/en/
Zheng, J., Luo, Y., Reed, E., Bell, R., Brown, E. W., and Hoffmann, M. (2017). Whole-genome comparative analysis of Salmonella enterica serovar Newport strains reveals lineage-specific divergence. Genome Biol. Evol. 94, 1047–1050. doi: 10.1093/gbe/evx065
Zhou, Y., Liang, Y., Lynch, K. H., Dennis, J. J., and Wishart, D. S. (2011). PHAST: a fast phage search tool. Nucleic Acids Res. 39, W347–W352. doi: 10.1093/nar/gkr485
Ziebell, K., Chui, L., King, R., Johnson, S., Boerlin, P., and Johnson, R. P. (2017). Subtyping of Canadian isolates of Salmonella Enteritidis using Multiple Locus Variable Number Tandem Repeat Analysis (MLVA) alone and in combination with Pulsed-Field Gel Electrophoresis (PFGE) and phage typing. J. Microbiol. Methods 139, 29–36. doi: 10.1016/j.mimet.2017.04.012
Keywords: Salmonella, prophage sequence typing, genome diversity, outbreaks, Enteritidis
Citation: Mottawea W, Duceppe M-O, Dupras AA, Usongo V, Jeukens J, Freschi L, Emond-Rheault J-G, Hamel J, Kukavica-Ibrulj I, Boyle B, Gill A, Burnett E, Franz E, Arya G, Weadge JT, Gruenheid S, Wiedmann M, Huang H, Daigle F, Moineau S, Bekal S, Levesque RC, Goodridge LD and Ogunremi D (2018) Salmonella enterica Prophage Sequence Profiles Reflect Genome Diversity and Can Be Used for High Discrimination Subtyping. Front. Microbiol. 9:836. doi: 10.3389/fmicb.2018.00836
Received: 12 December 2017; Accepted: 12 April 2018;
Published: 04 May 2018.
Edited by:
Ludmila Chistoserdova, University of Washington, United StatesReviewed by:
Francisco Rodriguez-Valera, Universidad Miguel Hernández de Elche, SpainEric Altermann, AgResearch, New Zealand
Copyright © 2018 Mottawea, Duceppe, Dupras, Usongo, Jeukens, Freschi, Emond-Rheault, Hamel, Kukavica-Ibrulj, Boyle, Gill, Burnett, Franz, Arya, Weadge, Gruenheid, Wiedmann, Huang, Daigle, Moineau, Bekal, Levesque, Goodridge and Ogunremi. This is an open-access article distributed under the terms of the Creative Commons Attribution License (CC BY). The use, distribution or reproduction in other forums is permitted, provided the original author(s) and the copyright owner are credited and that the original publication in this journal is cited, in accordance with accepted academic practice. No use, distribution or reproduction is permitted which does not comply with these terms.
*Correspondence: Dele Ogunremi, dele.ogunremi@inspection.gc.ca