- 1Computational Biology Institute, Milken Institute School of Public Health, George Washington University, Washington, DC, United States
- 2Department of Medicine, Georgetown University, Washington, DC, United States
- 3HIV Dynamics and Replication Program, Host-Virus Interaction Branch, Center for Cancer Research, National Cancer Institute, National Institutes of Health, Bethesda, MD, United States
- 4Sackler Faculty of Medicine, School of Public Health, Tel Aviv University, Tel Aviv, Israel
- 5CIBIO-InBIO, Centro de Investigação em Biodiversidade e Recursos Genéticos, Universidade do Porto, Vairão, Portugal
- 6Department of Epidemiology and Biostatistics, Milken Institute School of Public Health, George Washington University, Washington, DC, United States
Washington, DC consistently has one of the highest annual rates of new HIV-1 diagnoses in the United States over the last 10 years. To guide intervention and prevention strategies to combat DC HIV infection, it is helpful to understand HIV transmission dynamics in a historical context. Toward this aim, we conducted a retrospective study (years 1987–2015) of 3,349 HIV pol sequences (1,026 bp) from 1,995 individuals living in the DC area belonging to three different cohorts. We coupled HIV sequence data with clinical information (sex, risk factor, race/ethnicity, viral load, subtype, anti-retroviral regimen) to identify circulating drug resistant mutations (DRM) and transmission clusters and assess their persistence over time. Of the transmission clusters identified in the DC area, 78.0 and 31.7% involved MSM and heterosexuals, respectively. The longest spread of time for a single cluster was 5 years (2007–2012) using a distance-based network inference approach and 27 years (1987–2014) using a maximum likelihood phylogenetic approach. We found eight subtypes and nine recombinants. Genetic diversity increased steadily over time with a slight peak in 2009 and remained constant thereafter until 2015. Nucleotide diversity also increased over time while relative genetic diversity (BEAST) remained relatively steady over the last 28 years with slight increases since 2000 in subtypes B and C. Sequences from individuals on drug therapy contained the highest total number of DRMs (1,104–1,600) and unique DRMs (63–97) and the highest proportion (>20%) of resistant individuals. Heterosexuals (43.94%), MSM (40.13%), and unknown (44.26%) risk factors showed similar prevalence of DRMs, while injection drug users had a lower prevalence (33.33%). Finally, there was a 60% spike in the number of codons with DRMs between 2007 and 2010. Past patterns of HIV transmission and DRM accumulation over time described here will help to predict future efficacy of ART drugs based on DRMs persisting over time and identify risk groups of interest for prevention and intervention efforts within the DC population. Our results show how longitudinal data can help to understand the temporal dynamics of HIV-1 at the local level.
Introduction
The prevention and treatment of HIV in Washington, DC is a public health priority both locally and nationally, as more diagnoses per unit population occur annually in the District than in any of the 50 states (Centers for Disease Control and Prevention, 2017). According to the most recent report by the DC Department of Health (DOH), over 1,900 per 100,000 residents are living with HIV in DC as of 2017 (District of Columbia Department of Health, HIV/AIDS, Hepatitis, STD and TB Administration (HAHSTA), 2018). This surpasses the WHO's threshold for a generalized epidemic (1%) (District of Columbia Department of Health, HIV/AIDS, Hepatitis, STD and TB Administration (HAHSTA), 2017). Additionally, the epidemic disproportionately affects black men and women, who comprise 75% of HIV cases but only 46.7% of residents in DC (District of Columbia Department of Health, HIV/AIDS, Hepatitis, STD and TB Administration (HAHSTA), 2017). Seven of the eight wards in DC have an HIV prevalence greater than 1%, indicating that the epidemic is not entirely geographically localized (Pérez-Losada et al., 2017). Similar to the Chicago HIV epidemic (Morgan et al., 2017), it has been proposed that the severity of the DC epidemic may be due to the high proportion of residents who belong to high risk groups and the city's considerable economic stratification (Greenberg et al., 2009).
The United States HIV epidemic is composed of predominantly subtype B variants (Vermund and Leigh-Brown, 2012), although several studies report that non-B subtypes are becoming more prominent over time both in DC (Grossman et al., 2018), the US (Germer et al., 2015) and globally (Ivanov et al., 2013; Neogi et al., 2014; UK Collaborative Group on HIV Drug Resistance, 2014; Esbjörnsson et al., 2016). Notably, of the individuals living with HIV in DC, more than 10% were born outside of the United States, thus leading to a potentially high diversity of HIV in the region (Grossman et al., 2018). Additionally, with a variety of subtypes and recombinant forms circulating in Washington, DC, the presence of drug resistant mutations (DRM) becomes a relevant clinical issue that needs to be addressed in order to provide efficient and effective treatment. Drug resistance is one of the most pressing issues in HIV treatment today, as resistance to preventative and treatment drugs affects the ability of the virus to evade the immune system and persist in the infected individual. Drug resistant mutations may occur at the time of transmission, so treatment-naïve individuals may also be resistant at the time of diagnosis. Currently, there are 97 known codons affected by DRMs in the Stanford HIV database (https://hivdb.stanford.edu), all either conferring resistance to or under surveillance (SDRM) for one or more of the current 22 antiretroviral (ART) drugs. Resistance to antiretroviral drugs and ensuing viremia can result in immunosuppression leading to morbidity, and, if new DRMs arise, then new drugs are needed to combat the ever-evolving virus. In 2016, Kassaye et al. found a high prevalence of drug resistance mutations in persons living with HIV in DC. Thus, studying the presence and spread of DRMs in Washington, DC can provide a temporal and spatial context to study the dynamics of HIV drug resistance. Furthermore, identifying DRMs present and spreading between risk groups will provide public health officials with information to implement more efficient targeted education and prevention efforts and programs.
According to the DC DOH, men who have sex with men (MSM) and heterosexuals (HRH) are the primary modes of HIV transmission in DC, while transmission via injection drug use (IDU) has decreased by 95% from 2007 to 2017 (District of Columbia Department of Health, HIV/AIDS, Hepatitis, STD and TB Administration (HAHSTA), 2017). About 27% of people living with HIV are black MSM, while the second and third most prominent HIV risk groups are heterosexual black women and white MSM (District of Columbia Department of Health, HIV/AIDS, Hepatitis, STD and TB Administration (HAHSTA), 2017). A cross-sectional study in 2009 identified heterosexual transmission as an emerging epidemic in non-Hispanic black men and women in DC, demonstrating that this risk is based on transmission networks rather than individual risk (Magnus et al., 2009). Similarly, a study of transmission clusters in Sweden, Denmark, and Finland suggested MSM-heterosexual transmission played a significant role in HIV infection since the majority of the mixed-transmission clusters involved individuals from those two risk groups (Esbjörnsson et al., 2016).
To address the current HIV epidemic in Washington, DC—and to better recognize how it will evolve in the future—we must first understand the evolutionary dynamics of the local epidemic. Toward this aim, we conducted a retrospective study (years 1987–2015) of 1,995 HIV-infected individuals whose 3,349 sequences were collected from three separate datasets. Our analysis focused specifically on the polymerase (pol) gene, the most frequently sequenced gene in HIV-1 clinical studies of DRMs. We identified transmission clusters with associations to demographic information and mapped epidemiological variables on estimated transmission clusters. Lastly, we identified drug resistance mutations and assessed their variation over time, as well as estimated sequence diversity over time.
Materials and Methods
Datasets
A total of 3,349 HIV sequences (after removing duplicates) from the metropolitan DC area (including Washington, DC, northern Virginia, and northern and southern Maryland) were combined from three independently collected datasets: Pérez-Losada et al. (2017) with 1,659 sequences one each from that same number of individuals, Maldarelli et al. (2013) with 1,387 sequences collected from 33 individuals, and new HIV data presented here representing 303 sequences, one per individual (Table 1). All duplicate sequences in Pérez-Losada et al.'s dataset were removed, but all sequences in Maldarelli et al.'s dataset were used as it included known intra-patient variation and reflected the evolution of the virus over time. All studies performed DNA Sanger sequencing. Collectively, we have a total of 1,995 patients with sequence samples obtained between 1987 and 2015. Demographic variables considered were: sex, gender, race/ethnicity, age, country of birth, state of residence, risk factor, viral load, duration of infection, CD4+T lymphocyte count, HIV-1 subtype, and antiretroviral regimen type. These characteristics were paired with their respective HIV-1 sequence(s) (Table 1).
Briefly, sequences in the Pérez-Losada et al. (2017) dataset were collected through the DC Cohort, a longitudinal study conducted by the DC Department of Health at 13 DC area clinics (Milken Institute School of Public Health, 2018). Over 1,700 patients were enrolled in this study between 2011 and 2015. RNA from plasma samples was sequenced by LabCorp as part of routine clinical care with a focus on the pol genes. RT-PCR and Sanger sequencing were used to generate reads, which were analyzed with Sequencher DNA Sequence Analysis Software. Sequences for PR/RT sequences had a length of 1,496 bp. This dataset included additional integrase sequences (864 bp), which were not included in our analysis.
The dataset originally published in Maldarelli et al. (2013) was comprised of 33 patients treated at the NIH Clinical Center in Bethesda, Maryland. HIV sequences were obtained via single genome Sanger sequencing from plasma samples; limiting dilution was completed on each plasma sample. These patients were sampled longitudinally. Amplicons covering protease and reverse transcriptase (297 and 700–1200 bp, respectively) were used to obtain PR/RT sequences.
Data collection and sequencing of new HIV data was completed with the same single genome sequencing procedure as in Maldarelli et al. (2013). However, longitudinal sampling of these patients was not completed. A single plasma sample was taken from each patient at time of sampling and only a single limiting dilution was completed. These patients were also from the NIH Clinical Center in Bethesda, MD.
Phylodynamic Analyses
We aligned all sequences to the HXB2 reference pol sequence using MAFFT (Katoh et al., 2002). Aligned sequences were trimmed to a 1,026 bp region (2,253–3,279 bp relative to HXB2; GenBank accession K03455), which covered all of PR and a region of RT (55%). Any nucleotides outside this gene region were trimmed to ensure consistency among datasets. We used jModelTest (Posada, 2008) to estimate the best-fit model of molecular evolution along with model parameter values used in phylogenetic inference (Posada and Crandall, 2001). We constructed a maximum-likelihood (ML) phylogenetic tree using RAxML v. 8.2.9 (Stamatakis, 2014).
We assessed transmission clusters using both phylogenetic analyses and the network method HIV-TRACE (Kosakovsky Pond et al., 2018). HIV-TRACE identifies transmission clusters by analyzing genetic distance between pairs of sequences. For HIV-TRACE, we used a genetic distance threshold of 0.01 substitutions/site (Wertheim et al., 2017; as in Pérez-Losada et al., 2017) to account for the genetic diversity of pol. Additionally, we handled the ambiguities in the sequences using an average to account for all possible resolutions and avoid biases and false positive transmission clusters between the different datasets and sequencing strategies. Default settings were used for the remaining parameters. For the ML phylogenetic method, a transmission cluster was defined as a node with ≥70 bootstrap support (Felsenstein, 1985).
Watterson's genetic diversity (θ), nucleotide diversity (π), haplotype diversity (h), and the number of polymorphic sites (S) were estimated separately for HIV subtypes B and C and recombinant B and patient subsets (e.g., risk types, sex, race/ethnicity, date, and dataset) in DnaSP v. 6.11.01 (Rozas et al., 2017). Relative genetic diversity over time was inferred separately for subtypes B and C and recombinant B in BEAST2 (Bouckaert et al., 2014) using the GMRF Bayesian Skyride model (Minin et al., 2008) and the date of the HIV-1 sample as calibration and a normal prior with mean = 0.001 and SD = 0.0005 for ucld.mean. Additionally, we used the HKY substitution model with gamma-distributed among-site rate heterogeneity, a relaxed clock (log-normal) model of rate of substitution and partitioned into three codon positions. We performed two runs 5 × 108 generations each with sampling every 5,000 generations. Parameter uncertainty was summarized in the 95% highest posterior density (HPD) intervals. Results generated by BEAST were visualized in Tracer (Rambaut et al., 2018) with a 10% burn-in.
Drug Resistance Mutations and Subtype Analyses
We identified drug resistance mutations using the HIVdb program (Liu and Shafer, 2006) from the Stanford University HIV Drug Resistance Database (https://hivdb.stanford.edu). HIVdb identifies known resistance and surveillance variants (SDRMs) associated with 22 approved antiretroviral therapies including Protease Inhibitors (PI), Nucleoside RT Inhibitors (NRTI), Non-Nucleoside RT Inhibitors (NNRTI), and Integrase Inhibitors (INSTI), as well as treatment-selected mutations (TSMs). In order to compare DRMs over time, the number of affected codons for sequences from a single year was normalized by the number of sequences from that year in the dataset. We identified HIV-1 subtypes and recombinants phylogenetically using 170 subtype and recombinant reference sequences from the Los Alamos HIV database (http://www.hiv.lanl.gov/) and ML tree reconstruction, as well as using the REGA subtyping tool v. 3.0 provided by Stanford University (Pineda-Peña et al., 2013). Although subtyping was already reported for the Pérez-Losada et al. dataset, we included these sequences again for confirmation and comparison to the additional datasets from Washington, DC.
Results
The number of sequences included in our analyses varied between 0 and 540 sequences per year, with seven years missing between 1987 and 2015. The cohort was comprised primarily of men who have sex with men (MSM; 38.8%) and high-risk heterosexuals (HRH; 35.6%) (Table 1). The median age at the time of sequencing was 44.8 years, with lower and upper bounds of 10.0 and 82.0 years old, respectively. Antiretroviral exposure information was only available for Pérez-Losada et al.'s dataset, and the majority of those patients were on multiple-class drug regimens (57.93%). REGA and phylogenetic subtyping methods identified eight unique HIV-1 subtypes and nine recombinants in our combined dataset, of which 10 were neither B or B recombinants (BD, CRF12_BF, DB, CRF24_BG, BF, and BA). Those subtypes were B (94.95%), C (1.43%), and A, D, G, F1, F2, J, (<1%) and those recombinants were BD (1.37%), and CRF02_AG, CRF12_BF, DB, CRF18_cpx, CRF24_BG, BF, BA, and AK (<1%).
Overall, Washington, DC was found to have a high genetic diversity (mean Watterson's θ = 0.082, Table 2), with B recombinants having a slightly higher genetic diversity (θ = 0.089) compared to subtype B (θ = 0.079) and subtype C (θ = 0.079) (Table 2). For all subtypes, males had a higher genetic diversity than females, and for subtype B, all races/ethnicities had similar genetic diversity. However, for B recombinants and subtype C, blacks had a higher genetic diversity than the remaining races/ethnicities. For subtype B, there was an overall high genetic diversity for all risk groups, with general sexual contact (SEX) risk having the lowest genetic diversity (θ = 0.079). This is not unexpected given that all 1,387 HIV sequences from patients with general sexual contact risk belong to Maldarelli et al.'s dataset and are only from 33 patients. Furthermore, subtype B injection drug users (IDU) had the lowest nucleotide diversity (π = 0.046), while MSM and HRH had similar nucleotide diversity estimates. Moreover, genetic diversity in subtype B increased steadily over time, with a slight decrease in diversity in 2003 and 2004 (Table 2). Nucleotide diversity increased over time with a peak in 2005 and 2006. No steady trends of diversity were estimated in B recombinants and subtype C. Additionally, the past demographic analyses of subtype B, B recombinants, and subtype C in BEAST (Figure 1) indicated that HIV relative genetic diversity remained stable over the last 35 years, with subtype B showing more variability.
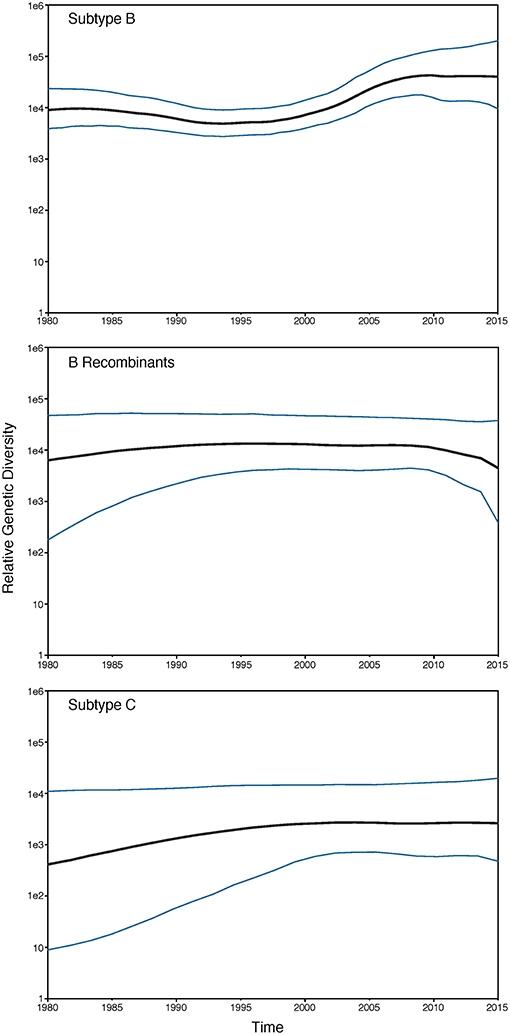
Figure 1. GMRF Bayesian Skyride plot of HIV-1 subtypes past population dynamics. The 95% high posterior density limits of the relative genetic diversity over time are shown in blue. Black lines show the median estimate of the relative genetic diversity over time.
Evolutionary relationships amongst the HIV sequences were represented by a star phylogeny, indicating equal dispersal around the DC area (Figure 2). We annotated the phylogenetic tree with four demographic characteristics (dataset, sex, subtype, and risk behavior) and found near even dispersal for all of them. Sequences from each of the 33 Maldarelli et al.'s patients clustered together both in RAxML with high support and in HIV-TRACE, as expected; however, some of the intra-patient sequences clustered into more than one cluster. Clusters that were comprised of sequences from a single Maldarelli et al.'s patient were not included in the total cluster number count (RAxML = 27, HIV-TRACE = 30). A total of 1,798 sequences (53.7%) were grouped into 215 clusters in the RAxML tree, with 16 of them belonging to groups with >5 individuals (Figure 2). Of the clusters that were comprised of Maldarelli et al.'s patients, 12 clusters contained at least one sequence from a different dataset (most often the new data). MSM comprised 59.7 and 85.7% of all clusters and clusters with ≥5 individuals, respectively (Table 3). A total of 40 clusters were comprised of MSM and HRH samples, while only 8 contained an IDU sample (Table 3). HIV-TRACE transmission networks were labeled by combinations of three phenotypic characteristics: race, risk factor, and sex (Figure 3, Table 3). A total of 314 (9.4%) sequences were grouped into 41 clusters comprised of two or three individuals. Of these clusters, 78.0% included at least one MSM individual and 31.7% included at least one HRH individual. A third (27.9–31.7%) of the clusters predicted from either method included ≥2 individuals from different datasets. The longest spread of time for a single cluster found in HIV-TRACE and RAxML was 5 years (2007–2012) and 27 years (1987–2014), respectively. The majority of sequences in clusters found for HIV-TRACE methods were from the same year (61%), unlike RAxML, where only a quarter (27.4%) of the sequences in the transmission clusters were from the same year. Overall, RAxML included more individuals in more transmission clusters compared to those predicted by HIV-TRACE.
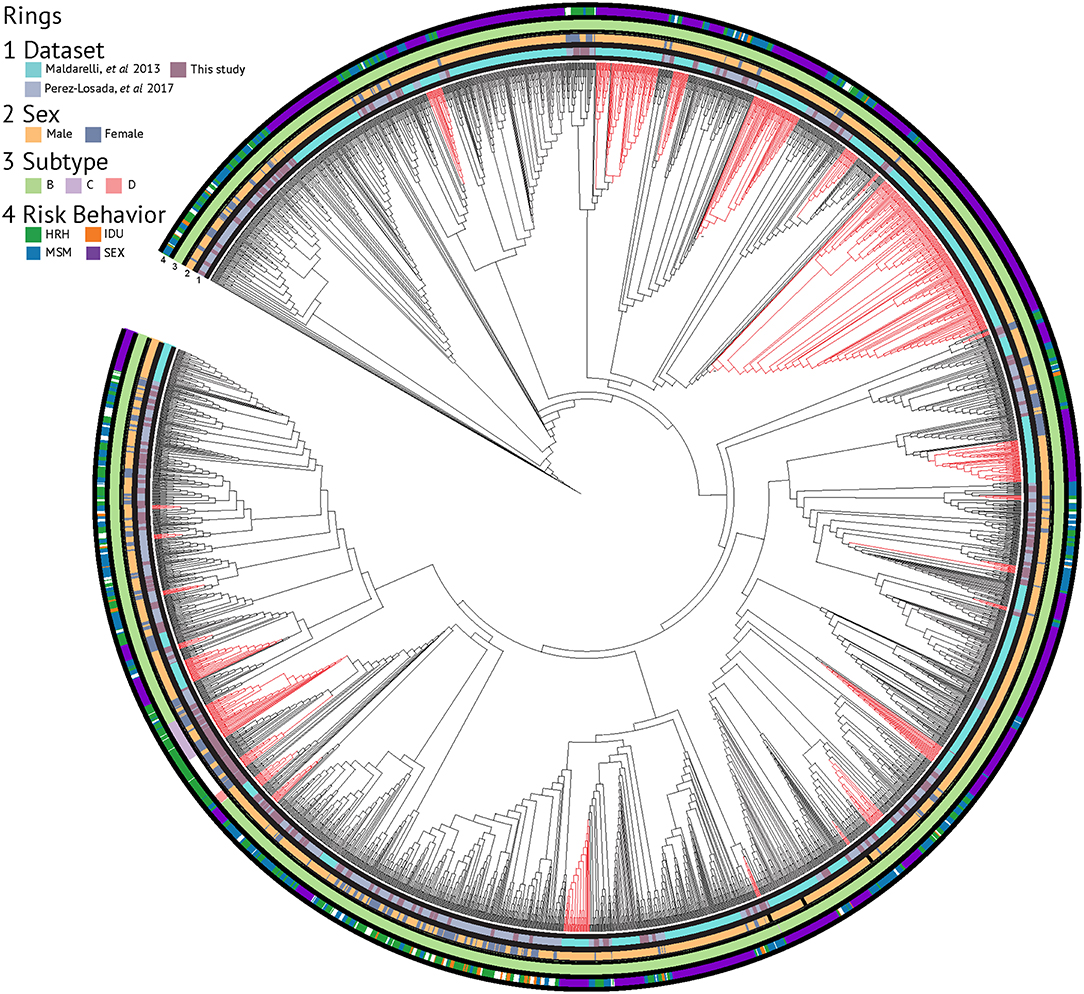
Figure 2. RAxML phylogenetic tree annotated with demographic characteristics. Clusters supported by ≥70% bootstrap values and including ≥5 sequence members are highlighted in red.
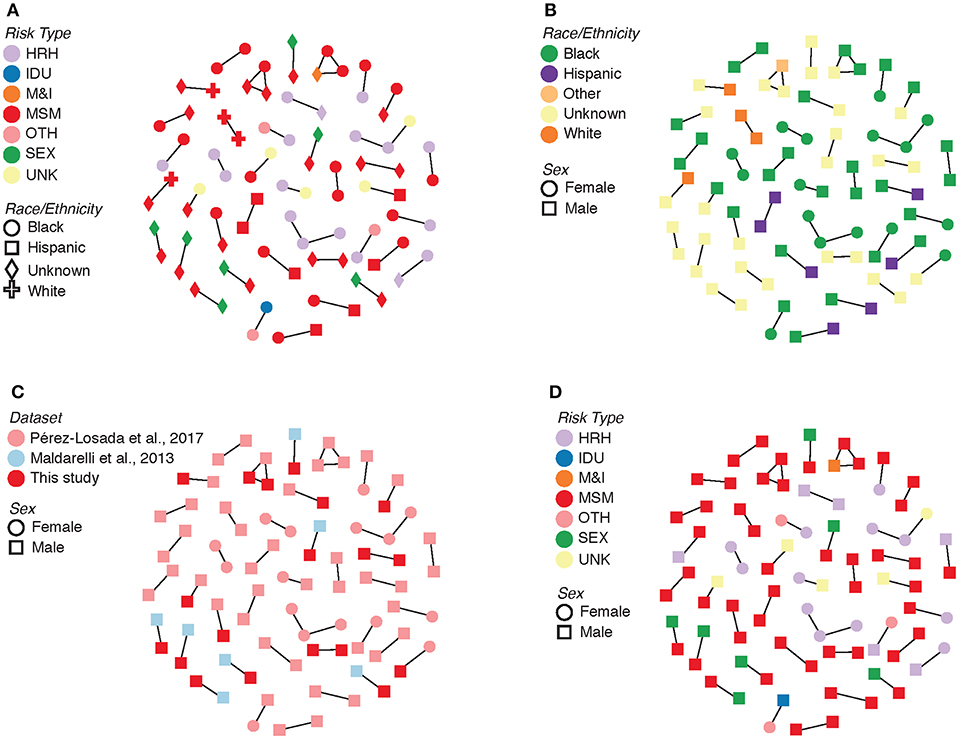
Figure 3. Transmission clusters with associated demographic information. (A) Risk type by race/ethnicity. (B) Race/ethnicity by sex. (C) Dataset by sex. (D) Risk type by sex. HRH, high risk heterosexual; IDU, injection drug user; MSM, man who has sex with men; SEX, sexual activity (general); MSM&IDU, MSM and IDU; OTH, other; UNK, unknown.
Drug resistance mutations (DRMs) were analyzed for subtype B sequences only (95% of the total sequence data). Across the combined dataset comprising all three studies, 24 amino acids in PR and 61 in RT were affected by drug resistance mutations. The types of antiretroviral drugs with the highest DRM prevalence were nucleoside reverse transcriptase inhibitors (NRTI), non-nucleoside reverse transcriptase inhibitors (NNRTI), and reverse transcriptase surveillance drug resistance mutations (RT SDRMs); these drugs were found to have between 64 and 97 unique DRMs present in our dataset (Figure 4, Table 4), with unique DRMs referring to a specific DRM observed at a codon position in one or more sequences. Therefore, if a mutation was detected more than once it was not double counted. Additionally, more than 20% of the individuals in the combined dataset displayed at least one DRM for one or more of these antiretroviral (ART) drugs. The risk group with the highest prevalence of DRMs was blood transfusion/perinatal (58.33%), but this is likely due to the low number of blood transfusion/perinatal individuals in our dataset (44 individuals, 1.3%). Additionally, these individuals often begin ART regimens almost immediately upon birth or acquisition of HIV, thus providing a longer time for DRMs to develop and persist in their viral population. Heterosexuals and MSM were found to have similar DRM prevalence at 43.94 and 40.13%, respectively. Those individuals with unknown risk factors had a DRM prevalence of 44.26%, and a lower prevalence was seen in injection drug users at 33.33% (Figure 4). Notably, a 60% increase in the number of codons affected by DRMs occurred between 2006 and 2010 (Figure 5). The amount of DRMs for PR Major (DRMs that make a major contribution to reduced susceptibility to protease inhibitors), PR Accessory (DRMs that contribute to reduced susceptibility in combination with PR Major DRMs), PR SDRMs, NRTI, NNRTI, RT SDRMs, and protease inhibitor treatment-selected mutations (PI TSMs) increased notably in the more recent years (2011–2015); however, this is likely due to the high number of sequences included in those years (Table 4). NRTI and RT SDRMs consistently had higher number of sequences that contained ≥1 DRM, total mutations, and unique mutations from 1996 onward.
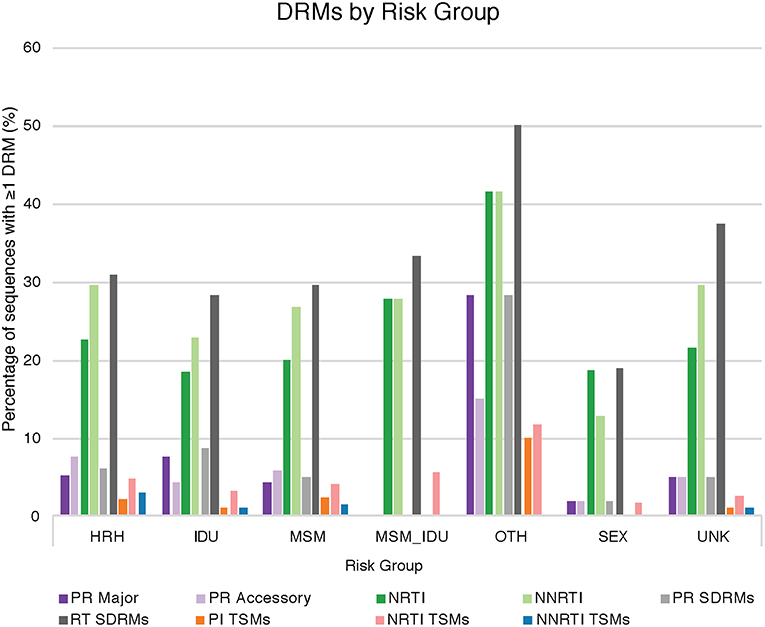
Figure 4. Drug resistance mutations by risk group. The percentage of sequences with at least one drug resistance mutation in a given ART drug category provided by Stanford HIVdb, separated by HIV risk group. HRH, high risk heterosexual; IDU, injection drug user; MSM, man who has sex with men; SEX, sexual activity (general); MSM_IDU, MSM and IDU; OTH, other; UNK, unknown; PR Major, DRMs that make a major contribution to reduced susceptibility to protease inhibitors; PR Accessory, DRMs that contribute to reduced susceptibility in combination with PR Major DRMs; NRTI, nucleoside reverse transcriptase inhibitors; NNRTI, non-nucleoside reverse transcriptase inhibitors; PR SDRMs, protease surveillance drug resistant mutations; RT SDRMs, reverse transcriptase surveillance drug resistant mutations; PI TSMs, protease inhibitor treatment-selected mutations; NRTI TSMs, nucleoside reverse transcriptase inhibitors treatment-selected mutations; NNRTI TSMs, non-nucleoside reverse transcriptase inhibitors treatment-selected mutations.
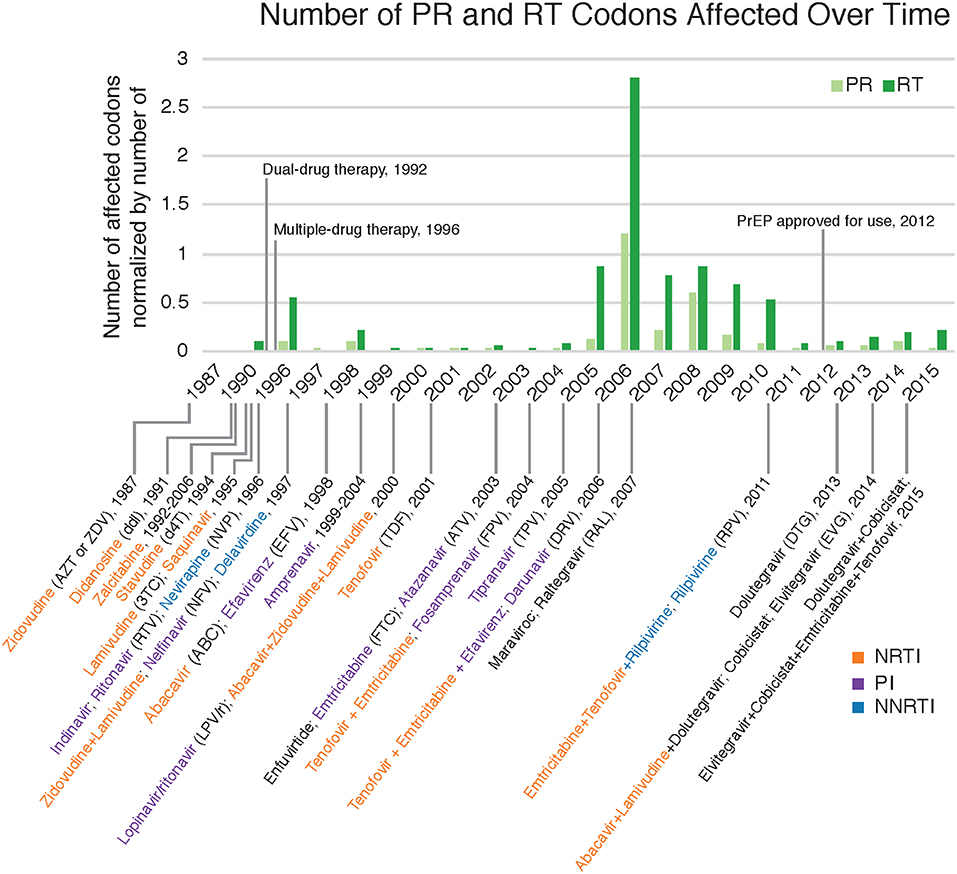
Figure 5. Drug resistant mutations annotated with relevant ART dates. Count of DRM-affected codons observed in each year normalized by the total number of sequences collected in that year. Dates with relevant drug therapy and introductions were included (Arts et al., 2012; Barré-Sinoussi et al., 2013; U.S. Food Drug Administration, 2018). NRTI, nucleoside reverse transcriptase inhibitors; PI, protease inhibitors; NNRTI, non-nucleoside reverse transcriptase inhibitors.
Fewer unique mutations were found to be at higher frequencies in the 1990s, whereas more unique mutations were found to be at a lower frequency in the 2000s (Table 4, Figure 6). This trend can be explained by the number of sequences per year, where there were lower total sequences in the 1990s and early 2000s (Table 4). However, despite there being a lower number of total sequences between 2005 and 2010, there were more distinct mutations in that time range (Figure 6) as well as a peak in the number of affected codons (Figure 5), as seen in a recent study of treatment-naïve individuals in the US (Ross et al., 2018). Notably, in 1996 there were eight DRMs found at high frequencies (PR: D30N, N88D; RT: D67N, K70R, Y181C, M184V, K219E, H22IY); all eight DRMs belonged to a single patient from Maldarelli et al.'s dataset (patient 27), in which one blood sample from a single appointment was obtained and 22 serial dilutions resulted in 22 separate sequences (Maldarelli et al., 2013). Two mutations in RT, K103N and M184V, persisted from around 2005 to 2015 at a lower frequency (average 12.4 and 8.8%, respectively). K103N is a Major NNRTI resistant mutation, whereas M184V is a Major NRTI resistant mutation.
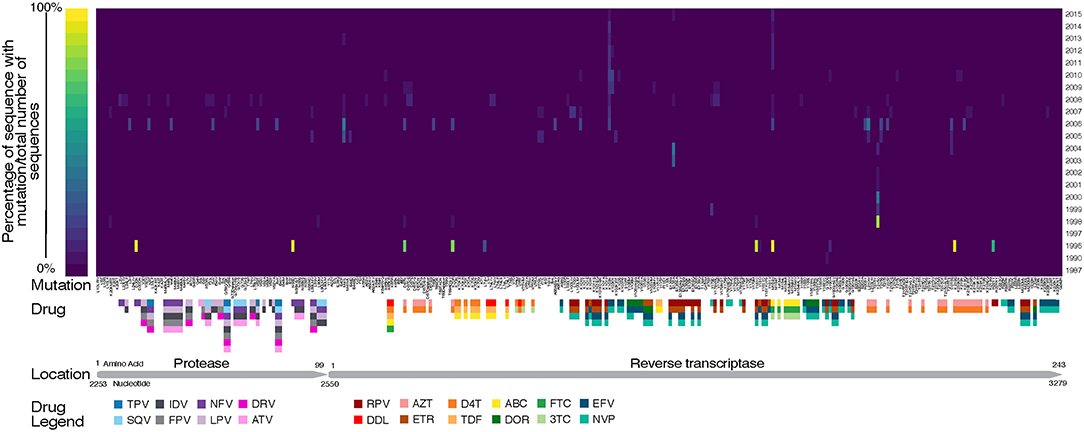
Figure 6. Heat map of drug resistant mutations over time. Annotated with genome location, relative to HXB2 reference, and known drugs that the mutations are selected by. TPV, Tipranavir; SQV, Saquinavir; NFV, Nelfinavir; LPV, Lopinavir; IDV, Indinavir; DRV, Darunavir; ATV, Atazanavir; RPV, Rilpivirine; DDL, Didanosine; AZT, Zidovudine; ETR, Etravirine; D4T, Stavudine; TDF, Tenofovir Disoproxil Fumarate; ABC, Abacavir; DOR, Doravirine; FTC, Emtricitabine; 3TC, Lamivudine; EFV, Efavirenz; NVP, Nevirapine.
Discussion
Transmission Networks
Multiple studies have identified transmission networks as playing a significant role in the spread of HIV in the United States and DC (Magnus et al., 2009; Pérez-Losada et al., 2010, 2017; Esbjörnsson et al., 2016; Kassaye et al., 2016; Morgan et al., 2017; Wertheim et al., 2017). We identified in our dataset 41 and 76 unique transmission clusters using HIV-TRACE and a ML phylogenetic method (RAxML), respectively, most of which included members of the MSM and HRH risk groups. Transmission within these high-risk groups is expected given that both groups are reported as continuing sources of HIV infection in Washington, DC (District of Columbia Department of Health, HIV/AIDS, Hepatitis, STD and TB Administration (HAHSTA), 2017, 2018). A majority of clusters included at least one non-Hispanic black individual, which is consistent with public health surveillance data as well (District of Columbia Department of Health, HIV/AIDS, Hepatitis, STD and TB Administration (HAHSTA), 2018). Like Kassaye et al. (2016), the majority of our identified transmission clusters were comprised of only two or three patients (81.9–100%). The ML and HIV-TRACE results included several transmission clusters containing individuals from different datasets (27.9 and 31.7%, respectively), suggesting that many of those networks are unique to this study. By combining sequencing and demographic information across a 28-year time window, we found that individuals in transmission networks predicted by HIV-TRACE had sequences obtained from different years ranging between 1 and 5 years apart, with a mean of 2.5 year spread in sequence time. However, the majority of the clusters for HIV-TRACE contained sequences from the same year (61.0%). For the RAxML, on the other hand, the longest amount of time between sequences in a transmission cluster was 27 years; although there was a mean of 2.4 year spread in sequence time. These results indicate that viral sequences do not often persist over many years, but when they do, distance-based cluster analyses might have a more difficult time identifying such transmission networks compared to evolutionary phylogenetic approaches. Our results show how insights from longitudinal studies can be used to understand viral dynamics over time in a local epidemic as well as how methodological choices can impact final inferences in such studies.
Temporal Diversity of HIV in DC
HIV diversity, as indicated by the number of unique subtypes and recombinants, and genetic diversity increased in the latter years (most recent sampling) of our study. Subtype B (94.95%) and B recombinants (2.9%) dominated our HIV dataset, followed by subtype C (1.43%). As suggested by Grossman et al. (2018), subtype C has been in DC since the late 1980s; however, our sampling of those same years did not include subtype C sequences. Similarly to a recent study of the San Francisco area over a 14-year time period, we also found over 4% non-subtype B HIV-1 variants in our combined dataset (Dalai et al., 2018). Focusing on subtype B, genetic diversity was similar across race/ethnicity but was higher in HRH and MSM relative to IDU risk factors. Genetic diversity increased steadily over time with a slight peak in 2009 and remained constant thereafter until 2015 (θ = ~0.086). Additionally, π, which is indicative of current genetic diversity (Crandall et al., 1999), increased over time as well, further suggesting that variants are accumulating in the DC population. The number of haplotypes generally increased until 2005 followed by a sharp reduction of haplotypes but a consistent genetic diversity. This trend could be a consequence of the small sample sizes in years 2005–2010, but relatively low numbers of haplotypes remained in years 2011–2013 despite high sample sizes. Our phylodynamic analyses of the three dominant subtypes in our dataset (B, B recombinants, and C) showed that the relative genetic diversity of DC's HIV population has remained relatively steady over the last 35 years, with subtype C showing an increase in relative genetic diversity and subtype B showing an increase in diversity since 2000. These results coupled with the phylogenetic tree being star-like further bolsters the conclusion that the HIV epidemic in DC is mature and individuals with varying infection durations and risk factor have been intermingling for years (Kassaye et al., 2016).
Evolution of Drug Resistant Mutations in DC
Our study found a higher percentage of patients with DRMs (37.9%) than a previous study (Kassaye et al., 2016) of the DC epidemic (22.5%). This is expected since our study also included a higher proportion of treatment-experienced individuals, while Kassaye et al. involved only ART-naïve patients. The DRM classes designated by the Stanford University HIV Drug Resistance Database (NRTI, NNRTI, and RT SDRMs) were notably more prominent in our data. There was little variation between the number of DRM-affected codons between risk groups.
We found an increase in the number of codons affected by DRMs between 2005 and 2008. It is possible that the smaller number of sequences from the years 2005 through 2010 relative to other years may have contributed to an artificial inflation of the number of DRMs during that time period. Additionally, all sequences from 2005 through 2010 came from the new dataset and were all treatment-naïve. A similar trend and spike in DRM prevalence was found in treatment-naïve patients in the U.S. between 2000 and 2009 (Ross et al., 2018), which correlates with the increase in diversity in subtype B. Such a trend may have been caused by changes in ART recommendations that allowed treatment to begin more quickly after diagnosis, the approval of additional therapies, mainly integrase inhibitors and specifically Maraviroc (MVC) and Raltegravir (RAL) introduced in 2007 (see Figure 5), and the increased use of genotypic screening for drug resistance prior to treatment (Ross et al., 2018). Kassaye et al. (2016) also observed a downward trend in DRMs over time between 1997–2006 and 2007–2013. Furthermore, medication guidelines recommended by physicians to patients have changed over time, which could result in the variation of number of DRMs and number of codon positions affected by mutation. Moreover, for the treatment-experienced individuals, HIV sequencing was likely requested because of concerns about their adherence and presumably because they are still viremic while being on medication, contributing to the increase in these numbers as well.
Although there was a decline in the number of DRM-affected codons around 2008, the number of unique drug resistant mutations increased throughout the 2000s. All of these DRMs were found at low frequencies, but a similar upward trend was also seen in genetic and nucleotide diversity. With increased diversity and increased DRMs, combating HIV replication and spread becomes more cumbersome, thus requiring researchers and doctors to stay one step ahead of the epidemic by developing new drugs that are not already impaired by the current DRMs before the current drugs are no longer effective. This is partially corrected already by patients in dual- or multiple-class ART regimens. Two mutations, K103N and M184V both in RT, persisted for 10 years in this combined dataset. K103N is selected for by usage of nevirapine (NVP) and efavirenz (EFV) (Bacheler et al., 2000; Gulick et al., 2004; Margot et al., 2006); M184V is selected by lamivudine (3TC) and emtricitabine (FTC) (Keulen et al., 1996; Frost et al., 2000). These four drugs are commonly prescribed as part of treatment recommendations (Geneva: World Health Organization, 2016, 2017).
Limitations
This study has a few limitations. Sampling across years is inconsistent and heterogeneous with more recent years containing more individuals and HIV sequences than older years. Additionally, initial years of the DC HIV epidemic (1980s) are missing. This uneven sampling could shorten the duration of the observed transmission clusters. This is further compounded when using HIV-TRACE, because a genetic distance cut-off is used, limiting the transmission clusters predicted by HIV-TRACE to more similar sequences. We supplemented the transmission clusters predicted by HIV-TRACE with transmission clusters predicted by phylogenetic methods, where genetic distance is not a cut-off (only branch support is), thus allowing for more distantly related viruses to be included in predicted transmission clusters. Each dataset was sequenced in different laboratories, leading to potential biases in ambiguity codes and consensus sequence resolution that could affect diversity estimates and potentially produce false positive or negative transmission clusters. Moreover, the direction of HIV virus transmission in a transmission cluster was not determined.
Conclusions
Like several other studies in the US (Latkin et al., 2011; DiNenno et al., 2012; Raymond et al., 2017) and in DC (Kassaye et al., 2016), our study supports the DC DOH's observation that HRH and MSM risk groups are primarily contributing to transmission of HIV (District of Columbia Department of Health, HIV/AIDS, Hepatitis, STD and TB Administration (HAHSTA), 2017, 2018). As in our study, the majority of identified transmission clusters include at least one MSM and one HRH individual and often involve sequences obtained from the same year. Additionally, insights from DRM prevalence and genetic diversity trends over time will allow treatment measures to be more effective. Specifically, we found that both genetic diversity and the number of unique DRMs in circulation increased in subtype B sequences from more recent years. As such, our findings suggest that new surveillance studies of HIV subtypes should be conducted to better understand the current transmission dynamics of HIV in Washington, DC, especially in treatment-naïve individuals where our study and others (Kassaye et al., 2016; Ross et al., 2018) saw a spike between 2005 and 2008 in DRM.
Data Availability
The new HIV sequence data associated with this study have been deposited in GenBank under accession numbers MK270625 - MK270927.
Ethics Statement
Individuals represented in the new HIV data participated in clinical protocols (00-I-0110, 97-I-0082, and 95-I-0072), which took place at the NIH Clinical Center in Bethesda, Maryland. These protocols were approved by the NIAID Institutional Review Board (FWA00005897). All participants provided written informed consent.
Author Contributions
KC, MP-L, ZG, FM, and SK designed the project. SK, ZG, and FM collected data. KG and MS analyzed the data and performed all bioinformatic analyses. MP-L and KC reviewed all analyses. KG, MS, MP-L, and KC prepared original draft of manuscript, and all authors read, revised and approved the final manuscript.
Funding
This study was partially supported by a Metropolitan Washington WIHS (Seble Kassaye, PI) U10-A1-034994 award, DC D-CFAR pilot award, USA NIH CCNS214443F and NIH UL1TR000075 awards from the NIH National Center for Advancing Translational Sciences, the DC Cohort Study (UO1 AI69503- 03S2), a supplement from the Women's Interagency Study for HIV (410722_GR410708), and a 2015 HIV Phylodynamics Supplement award from the District of Columbia Center for AIDS Research, an NIH funded program (P30AI117970), which is supported by the following NIH Co- Funding and Participating Institutes and Centers: NIAID, NCI, NICHD, NHLBI, NIDA, NIMH, NIA, FIC, NIGMS, NIDDK, and OAR. Its contents are solely the responsibility of the authors and do not necessarily represent the official views of the National Center for Advancing Translational Sciences or the National Institutes of Health.
Conflict of Interest Statement
The authors declare that the research was conducted in the absence of any commercial or financial relationships that could be construed as a potential conflict of interest.
Acknowledgments
We thank all the authors from the two manuscripts whose data was incorporated into this project (Maldarelli et al., 2013; Pérez-Losada et al., 2017) for their work on those respective projects, as their efforts were vital to the inclusion of those sequencing data.
References
Arts, E. J., Hazuda, D. J., Bushman, E. F. D., Nabel, G. J., and Swanstrom, R. (2012). HIV-1 antiretroviral drug therapy basic principles of antiretroviral. Cold Spring Harb. Perspect. Med. 2, 1–23. doi: 10.1101/cshperspect.a007161
Bacheler, L. T., Anton, E. D., Kudish, P., Baker, D., Bunville, J., Krakowski, K., et al. (2000). Human immunodeficiency virus type 1 mutations selected in patients failing efavirenz combination therapy. Antimicrob. Agents Chemother. 44, 2475–2484. doi: 10.1128/AAC.44.9.2475-2484.2000
Barré-Sinoussi, F., Ross, A. L., and Delfraissy, J. F. (2013). Past, present and future: 30 years of HIV research. Nat. Rev. Microbiol. 11, 877–883. doi: 10.1038/nrmicro3132
Bouckaert, R., Heled, J., Kühnert, D., Vaughan, T., Wu, C. H., Xie, D., et al. (2014). BEAST 2: a software platform for bayesian evolutionary analysis. PLoS Comput. Biol. 10, 1–6. doi: 10.1371/journal.pcbi.1003537
Centers for Disease Control Prevention (2017). HIV in the United States by Geography. Available online at: https://www.cdc.gov/hiv/pdf/statistics/cdc-hiv-geographic-distribution.pdf.
Crandall, K. A., Posada, D., and Vasco, D. (1999). Effective population sizes: missing measures and missing concepts. Anim. Conserv. 2, 317–319. doi: 10.1111/j.1469-1795.1999.tb00078.x
Dalai, S. C., Junqueira, D. M., Wilkinson, E., Mehra, R., Kosakovsky Pond, S. L., Levy, V., et al. (2018). Combining phylogenetic and network approaches to identify hiv-1 transmission links in san mateo county, California. Front. Microbiol. 9, 1–8. doi: 10.3389/fmicb.2018.02799
DiNenno, E. A., Oster, A. M., Sionean, C., Denning, P., and Lansky, A. (2012). Piloting a system for behavioral surveillance among heterosexuals at increased risk of HIV in the United States. Open AIDS J. 6, 169–176. doi: 10.2174/1874613601206010169
District of Columbia Department of Health, HIV/AIDS, Hepatitis, STD TB Administration (HAHSTA),. (2017). Annual Epidemiology and Surveillance Report: Data Through December 2016. Washignton, DC Available online at: www.doh.dc.gov/hahsta
District of Columbia Department of Health, HIV/AIDS, Hepatitis, STD TB Administration (HAHSTA),. (2018). Annual Epidemiology and Surveillance Report: Data Through December 2017 District. Available online at: www.doh.dc.gov/hahsta
Esbjörnsson, J., Mild, M., Audelin, A., Fonager, J., Skar, H., Bruun Jørgensen, L., et al. (2016). HIV-1 transmission between MSM and heterosexuals, and increasing proportions of circulating recombinant forms in the Nordic Countries. Virus Evol. 2:vew010. doi: 10.1093/ve/vew010
Felsenstein, J. (1985). Confidence limits on phylogenies: an approach using the bootstrap. Evolution (N. Y) 39, 783–791.
Frost, S. D., Nijhuis, M., Schuurman, R., Boucher, C. A. B., and Brown, A. J. L. (2000). Evolution of lamivudine resistance in human immunodeficiency virus type 1-infected individuals: the relative roles of drift and selection. J. Virol. 74, 6262–6268. doi: 10.1128/JVI.74.14.6262-6268.2000
Geneva: World Health Organization (2016). Consolidated Guidelines on the Use of Antiretroviral Drugs for Treating and Preventing HIV Infection. Recommendations for a Public Health Approach. Second Edition. Available online at: https://www.who.int/hiv/pub/arv/arv-2016/en/.
Germer, J. J., Wu, P., Soderberg, J. D., Mandrekar, J. N., and Yao, J. D. (2015). HIV-1 subtype diversity among clinical specimens submitted for routine antiviral drug resistance testing in the United States. Diagn. Microbiol. Infect. Dis. 83, 257–260. doi: 10.1016/j.diagmicrobio.2015.07.014
Greenberg, A. E., Hader, S. L., Masur, H., Young, A. T., Skillicorn, J., and Dieffenbach, C. W. (2009). Fighting HIV/AIDS in Washington, D.C. Health Aff. 28, 1677–1687. doi: 10.1377/hlthaff.28.6.1677
Grossman, Z., Rico, S. V., Cone, K., Shao, W., Rehm, C., Jones, S., et al. (2018). Early presence of HIV-1 subtype C in Washington, D.C. AIDS Res. Hum. Retroviruses 34, 680–684. doi: 10.1089/aid.2018.0041
Gulick, R. M., Ribaudo, H. J., Shikuma, C. M., Lustgarten, S., Squires, K. E., Meyer, W. A., et al. (2004). Triple-nucleoside regimens versus efavirenz-containing regimens for the initial treatment of HIV-1 infection. N. Engl. J. Med. 350, 1850–1861. doi: 10.1056/NEJMoa031772
Ivanov, I. A., Beshkov, D., Shankar, A., Hanson, D. L., Paraskevis, D., Georgieva, V., et al. (2013). Detailed molecular epidemiologic characterization of hiv-1 infection in bulgaria reveals broad diversity and evolving phylodynamics. PLoS ONE 8:e59666. doi: 10.1371/journal.pone.0059666
Kassaye, S. G., Grossman, Z., Balamane, M., Johnston-White, B., Liu, C., Kumar, P., et al. (2016). Transmitted HIV drug resistance is high and longstanding in metropolitan Washington, DC. Clin. Infect. Dis. 63, 836–843. doi: 10.1093/cid/ciw382
Katoh, K., Misawa, K., Kuma, K., and Miyata, T. (2002). MAFFT: a novel method for rapid multiple sequence alignment based on fast fourier transform. Nucleic Acids Res. 30, 3059–3066. doi: 10.1093/nar/gkf436
Keulen, W., Boucher, C., and Berkhout, B. (1996). Nucleotide substitution patterns can predict the requirements for drug-resistance of HIV-1 proteins. Antivir. Res. 31, 45–57. doi: 10.1016/0166-3542(96)00944-8
Kosakovsky Pond, S. L., Weaver, S., Leigh Brown, A. J., and Wertheim, J. O. (2018). HIV-TRACE (Transmission Cluster Engine): a tool for large scale molecular epidemiology of HIV-1 and other rapidly evolving pathogens. Mol. Biol. Evol. 35, 1–16. doi: 10.1093/molbev/msy016
Latkin, C., Yang, C., Tobin, K., Penniman, T., Patterson, J., and Spikes, P. (2011). Differences in the social networks of African American men who have sex with men only and those who have sex with men and women. Am. J. Public Health 101, 18–23. doi: 10.2105/AJPH.2011.300281
Liu, T. F., and Shafer, R. W. (2006). Web resources for HIV type 1 genotypic-resistance test interpretation. Clin. Infect. Dis. 42, 1608–1618. doi: 10.1086/503914
Magnus, M., Kuo, I., Shelley, K., Rawls, A., Peterson, J., Montanez, L., et al. (2009). Risk factors driving the emergence of a generalized heterosexual HIV epidemic in Washington, district of Columbia networks at risk. Aids 23, 1277–1284. doi: 10.1097/QAD.0b013e32832b51da
Maldarelli, F., Kearney, M., Palmer, S., Stephens, R., Mican, J., Polis, M. A., et al. (2013). HIV populations are large and accumulate high genetic diversity in a nonlinear fashion. J. Virol. 87, 10313–10323. doi: 10.1128/JVI.01225-12
Margot, N. A., Lu, B., Cheng, A., and Miller, M. D. (2006). Resistance development over 144 weeks in treatment-naive patients receiving tenofovir disoproxil fumarate or stavudine with lamivudine and efavirenz in Study 903. HIV Med. 7, 442–450. doi: 10.1111/j.1468-1293.2006.00404.x
Milken Institute School of Public Health (2018). D.C. Cohort Longitudinal HIV Study. Available online at: http://go.gwu.edu/dccohort.
Minin, V. N., Bloomquist, E. W., and Suchard, M. A. (2008). Smooth skyride through a rough skyline: bayesian coalescent-based inference of population dynamics. Mol. Biol. Evol. 25, 1459–1471. doi: 10.1093/molbev/msn090
Morgan, E., Oster, A. M., Townsell, S., Peace, D., Benbow, N., and Schneider, J. A. (2017). HIV-1 infection and transmission networks of younger people in Chicago, Illinois, 2005-2011. Public Health Rep. 132, 48–55. doi: 10.1177/0033354916679988
Neogi, U., Häggblom, A., Santacatterina, M., Bratt, G., Gisslén, M., Albert, J., et al. (2014). Temporal trends in the Swedish HIV-1 epidemic: increase in non-B subtypes and recombinant forms over three decades. PLoS ONE 9:e99390. doi: 10.1371/journal.pone.0099390
Pérez-Losada, M., Castel, A. D., Lewis, B., Kharfen, M., Cartwright, C. P., Huang, B., et al. (2017). Characterization of HIV diversity, phylodynamics and drug resistance in Washington, DC. PLoS ONE 12:0185644. doi: 10.1371/journal.pone.0185644
Pérez-Losada, M., Jobes, D. V., Sinangil, F., Crandall, K. A., Posada, D., and Berman, P. W. (2010). Phylodynamics of HIV-1 from a phase-III AIDS vaccine trial in North America. Mol. Biol. Evol. 27, 417–425. doi: 10.1093/molbev/msp254
Pineda-Peña, A. C., Faria, N. R., Imbrechts, S., Libin, P., Abecasis, A. B., Deforche, K., et al. (2013). Automated subtyping of HIV-1 genetic sequences for clinical and surveillance purposes: Performance evaluation of the new REGA version 3 and seven other tools. Infect. Genet. Evol. 19, 337–348. doi: 10.1016/j.meegid.2013.04.032
Posada, D. (2008). jModelTest: Phylogenetic model averaging. Mol. Biol. Evol. 25, 1253–1256. doi: 10.1093/molbev/msn083
Posada, D., and Crandall, K. (2001). Selecting models of nucleotide substitution: an application to human immunodeficiency virus 1 (HIV-1). Mol. Biol. Evol. 18, 897–906. doi: 10.1093/oxfordjournals.molbev.a003890
Rambaut, A., Suchard, M., Xie, D., and Drummond, A. (2018). Tracer v1.6. Available online at: http://beast.bio.ed.ac.uk/%0A24.
Raymond, H. F., Al-Tayyib, A., Neaigus, A., Reilly, K. H., Braunstein, S., Brady, K. A., et al. (2017). HIV among MSM and heterosexual women in the United States: an ecologic analysis. J. Acquir. Immune Defic. Syndr. 75, S276–S280. doi: 10.1097/QAI.0000000000001422
Ross, L. L., Shortino, D., and Shaefer, M. S. (2018). Changes from 2000 to 2009 in the prevalence of HIV-1 containing drug resistance-associated mutations from antiretroviral therapy-naive, HIV-1-infected patients in the United States. AIDS Res. Hum. Retroviruses 34, 672–679. doi: 10.1089/aid.2017.0295
Rozas, J., Ferrer-Mata, A., Sanchez-DelBarrio, J. C., Guirao-Rico, S., Librado, P., Ramos-Onsins, S. E., et al. (2017). DnaSP 6: DNA sequence polymorphism analysis of large data sets. Mol. Biol. Evol. 34, 3299–3302. doi: 10.1093/molbev/msx248
Stamatakis, A. (2014). RAxML version 8: A tool for phylogenetic analysis and post-analysis of large phylogenies. Bioinformatics 30, 1312–1313. doi: 10.1093/bioinformatics/btu,033
U.S. Food and Drug Administration (2018). HIV/AIDS Historical Time Line 2010–2017. Silver Spring, MD.
UK Collaborative Group on HIV Drug Resistance (2014). The increasing genetic diversity of HIV-1 in the UK, 2002-2010. AIDS 28, 773–780. doi: 10.1097/QAD.0000000000000119
Vermund, S. H., and Leigh-Brown, A. J. (2012). The HIV epidemic: high-income countries. Cold Spring Harb. Perspect. Med. 2, 1–24. doi: 10.1101/cshperspect.a007195
Keywords: Washington, DC, phylodynamics, HIV-1, drug resistance mutations, transmission networks
Citation: Gibson KM, Steiner MC, Kassaye S, Maldarelli F, Grossman Z, Pérez-Losada M and Crandall KA (2019) A 28-Year History of HIV-1 Drug Resistance and Transmission in Washington, DC. Front. Microbiol. 10:369. doi: 10.3389/fmicb.2019.00369
Received: 03 December 2018; Accepted: 12 February 2019;
Published: 08 March 2019.
Edited by:
Kok Keng Tee, University of Malaya, MalaysiaReviewed by:
Teiichiro Shiino, National Institute of Infectious Diseases (NIID), JapanBin Su, Beijing Youan Hospital, Capital Medical University, China
Copyright © 2019 Gibson, Steiner, Kassaye, Maldarelli, Grossman, Pérez-Losada and Crandall. This is an open-access article distributed under the terms of the Creative Commons Attribution License (CC BY). The use, distribution or reproduction in other forums is permitted, provided the original author(s) and the copyright owner(s) are credited and that the original publication in this journal is cited, in accordance with accepted academic practice. No use, distribution or reproduction is permitted which does not comply with these terms.
*Correspondence: Keylie M. Gibson, a21naWJzb25AZ3d1LmVkdQ==