- 1Soil and Fertilizer Institute, Sichuan Academy of Agricultural Sciences, Chengdu, China
- 2Department of Microbiology, College of Resources, Sichuan Agricultural University, Chengdu, China
Truffles are ascomycetous ectomycorrhizal fungi that have elevated status in the culinary field due to their unique aroma and taste as well as their nutritional value and potential biological activities. Tuber melanosporum, T. indicum, T. panzhihuanense, T. sinoaestivum, and T. pseudoexcavatum are five commercial truffle species mainly distributed in Europe or China. In this study, an untargeted metabolomics technology based on an ultra-high-performance liquid chromatography-tandem mass spectrometry (UHPLC-MS/MS) method was applied to analyze the metabolic profiles and variations among these five truffle species. In our results, a total of 2376 metabolites were identified under positive ion mode, of which 1282 had significantly differential amounts and covered 110 pathways or metabolisms. Principal component analysis (PCA) and partial least squares-discriminant analysis (PLS-DA) revealed a clear separation from each of these five truffles, indicating a significantly different metabolic profile among them, with the biggest difference between T. melanosporum and the other four truffles. The differential metabolites covered various chemical categories, and a detailed analysis was performed for nine metabolic categories, including amino acids, saccharides and nucleosides, organic acids, alkaloids, flavonoids, carnitines, phenols and alcohols, esters, and sulfur compounds. For each of the nine categories, most of metabolites predominantly accumulated in T. melanosporum compared with the other four truffles. Meanwhile, there were significant differences of the average ion intensity in each category among the five truffles, e.g., higher amounts of amino acids was detected in T. panzhihuanense and T. pseudoexcavatum; T. indicum contained significantly more carnitines, while there were more alkaloids in T. melanosporum. Additionally, some metabolites with biological activities were discussed for each category, such as acetyl-L-carnitine, adenine, neobavaisoflavone, and anandamide. Generally, this study may provide the valuable information regarding the variation of the metabolic composition of these five commercial truffle species, and the biological significance of these metabolites was uncovered to explore the metabolic mechanisms of truffles, which would be helpful for further research on the compounds and potential biological functions in truffles that have not yet been investigated.
Introduction
Tuber spp., belonging to Ascomycota, are an ectomycorrhizal fungus that lives symbiotically with plants and is characterized by hypogeous fruiting bodies (Mello et al., 2006; Benucci and Bonito, 2016). Their fruiting bodies are also called truffles, and are referred to as “underground gold” because they are rare and are highly valued for their culinary and medicinal traits (Tang et al., 2015). At present, there are at least 180–230 truffle species that have been discovered throughout the world (Kues and Martin, 2011). Tuber melanosporum is the most highly appreciated black truffle due to its unique and intense fragrance. It is mainly produced in countries along the Mediterranean coast, such as Italy, France, and Spain, and commands a very expensive price in the edible fungus market (Bertault et al., 1998; Campo et al., 2017). Tuber indicum is also a black truffle species that is phylogenetically and morphologically close or similar to T. melanosporum, and it is one of the major commercial truffles in China (Zhang et al., 2019). In addition to T. indicum, Tuber sinoaestivum, and Tuber pseudoexcavatum are all Chinese black truffles with commercial value, and they have been exported to Europe, the United States, and Australia, although they do not command a price as high as T. melanosporum (García-Montero et al., 2010; Zambonelli, 2012; Ye et al., 2018). Also, the investigations about the T. sinoaestivum and T. pseudoexcavatum were relatively rare compared with T. melanosporum and T. indicum. Tuber panzhihuanense, also called the Chinese white truffle, is a unique truffle species in southwest China discovered in 2011 with a pleasant aroma and flavor, and is also the only white truffle species with great commercial potential found in China (3000–5000 RMB/kg market value) (Deng et al., 2013; Yang et al., 2019).
In addition to the unique and seductive aroma of truffles, the nutrients and bioactive compounds with health benefits in truffles are also the major attraction, leading to a series of investigations to explore the metabolites in their fruiting bodies. It has been found that truffles are rich in protein, fatty acids, carbohydrates, amino acids, and minerals (Wang and Marcone, 2011; El Enshasy et al., 2013). Some important metabolites with biological activities have been isolated and characterized from truffle fruiting bodies, including polysaccharides, androstenol, ceramides, ergosterol, and phenolics, which have been verified to possess multiple healthy attributes (Zhao et al., 2014; Longo et al., 2017; Patel et al., 2017). For example, polysaccharide extracts and ribonuclease from truffle fruiting bodies showed high antioxidant potential, immunomodulatory and antitumor activities (Zhao et al., 2014). In addition, anti-inflammation, antimicrobial, anti-depressant, and menstruation regulation properties in truffles have also been also demonstrated (Wang and Marcone, 2011). Furthermore, the chemical compounds contributing to the aroma of truffles have been studied to explore the secrets of truffles fragrance, which is closely related to the microbiome in truffles, and over 200 volatile organic compounds (VOCs) have been isolated and reported (Zhang et al., 2016). The aroma of truffles is finally determined by the comprehensive action of various compounds, including alcohols, ketones, enols, aldehydes, esters, aromatic compounds, amines, and sulfur compounds (Mannina et al., 2012; Splivallo and Ebeler, 2015). However, the nutrients, bioactive compounds, and VOCs are variable in different truffle species, meaning that there are different metabolite profiles in different truffle species.
Metabolomics is a newly developed technique in the field of systematic biology following genomics and proteomics, and it explores the metabolic mechanism of the entire organism by detecting the changes in metabolites (Fiehn et al., 2000; Zhao et al., 2019). Metabolites provide a functional output of biochemical activity as the downstream of genes, transcripts, and proteins (Patti, 2011). Currently, metabolomics has been utilized in medicine and botany, and for the analysis of food quality, nutrition, and components. An untargeted metabolomics analysis simultaneously detects as many metabolites as possible, to systematically compare the features of metabolites among species, and is also suitable for truffles (Zhao et al., 2019). Many studies have reported the metabolites in different truffle fruiting bodies under different conditions, with the methods of standard wet chemistry analysis, spectrophotometry, gas chromatography-olfactometry (GC/O), gas chromatography-mass spectrometry (GC-MS), NMR spectroscopy, picot-electronic nose, and liquid chromatography-mass spectrometry (LC-MS), to detailly reveal the compositions of the chemical compounds (Wang and Marcone, 2011; Patel et al., 2017). However, many of these previous studies were more concerned about how to extend the shelf life of fresh truffles by detecting some of the metabolite components that contributed to truffle commercial use, or analyzed the aroma of changing VOCs without the entire metabolic profile or the metabolic mechanism of truffle fruiting bodies. For example, Savini et al. (2017) only detected the phenolic profiles and volatile profile of T. melanosporum by high-performance liquid chromatography-high resolution mass spectrometry (HPLC-HRMS) under different packaging conditions. Additionally, these studies focused more on well-known truffle species, such as Tuber magnatum, T. melanosporum, Tuber borchii, and Tuber aestivum, and rarely provided the details regarding Chinese truffle species, which limits the further exploration of the chemical compounds and the potential biological functions of truffles.
In this study, an LC-MS-based metabolomic approach was used to investigate the metabolic composition and variations among five commercial truffle species, including T. melanosporum and four Chinese truffles, aiming to reveal the differences in the metabolic profile and compare their edible values. In addition, the biological significance of these metabolites was uncovered to explore the metabolic mechanisms of truffles, which would be helpful to find out the formation mechanism of truffle ascocarps, which thus far has been unclear. To the best of our knowledge, this is the first study that used metabolomics to enhance the insight into the metabolic variation of these five truffle species.
Materials and Methods
Strategy for Sampling Truffle Fruiting Bodies and Associated Soils
There were five commercial truffle species selected for this study: T. melanosporum, T. indicum, T. panzhihuanense, T. sinoaestivum, and T. pseudoexcavatum (Figure 1). The fruiting bodies from these Tuber spp. were taxonomically identified by morphological method and molecular method based on ITS sequence amplified with primers ITS1F (5′-CTTGGTCATTTAGAGGAAGTAA-3′) and ITS4 (5′-TCTCGGGCTGGAGGTGCGGGTCGAGT-3′). The nucleotides alignment of the obtained sequences was performed based on the National Center for Biotechnology Information (NCBI) database and the phylogenetic analysis of these five truffle species was also carried out by Mega 6.0 (Supplementary Figure S1). The nucleotide sequences in this study have been deposited in NCBI database with GenBank accession numbers MN338092–MN338096.
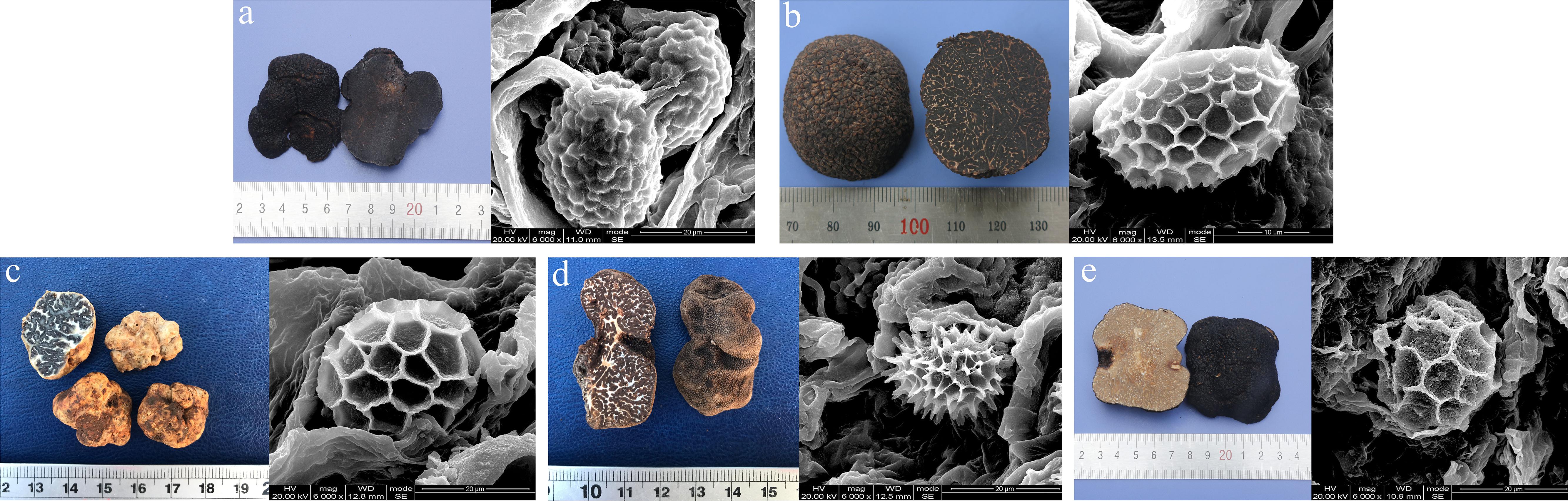
Figure 1. Images of one of the ascocarps and their mature spores of each truffle species used for LC-MS-based metabolomic analysis. (a) T. melanosporum, (b) T. indicum, (c) T. panzhihuanense, (d) T. pseudoexcavatum, and (e) T. sinoaestivum. The spores were observed under the scanning electron microscope (SEM) (Inspect, FEI, United States) and these ascocarps were taxonomically identified by morphological and molecular methods at the Soil and Fertilizer Institute, Sichuan Academy of Agricultural Sciences.
The sampling locations and the corresponding host plants for these different truffle species are shown in Table 1. For each truffle species, a total of thirty ascocarps from close geographical position and at the same maturity were collected. These ascocarps were all at the completely maturity stage and the maturation degrees were judged by the ascospores, peridium, gleba, size and weight according to Le Tacon et al. (2013) and Antony-Babu et al. (2014). When collecting in the field, the ascocarps were removed from the ground by hand. Then, the unbroken ascocarps were selected, wrapped with aluminum foil, and placed in a low-temperature storage box with ice packs, after which they were immediately transported to the laboratory. Meanwhile, the surrounding soils of the ascocarps in five different sampling locations were also collected for soil properties analysis, which were stored at 4°C. There were three biological replicates of associated soil samples from each truffle species.
In the laboratory, the ascocarps were cleaned with small brushes to wipe off the soil, rinsed three times with sterile water, and then surface-disinfected with 75% alcohol. Broke the ascocarps and then carved a piece on their inside with the sterile blade on a clean bench, and their tissues were transferred with sterile tweezers into 5 ml sterile Eppendorf tubes. The tubes were placed into liquid nitrogen for 30 s, and then quickly stored at –80°C until analysis. In order to reduce error, tissues from three ascocarps (the same truffle species) were mixed into one biological sample, so there were ten biological samples obtained from thirty ascocarps of each truffle species, which finally underwent metabolic analysis. No less than 100 mg fruiting bodies per sample were prepared for metabolite extraction. These samples from five Tuber species were assigned as “mel,” “sin,” “ind,” “pan,” and “pse.”
Soil Properties Analysis
The properties of the associated soil samples from five different sampling locations were analyzed according to the previously described method (Li et al., 2016), including pH, organic matter content (OM), total nitrogen (TN), available nitrogen (AN), available phosphorus (AP) and available potassium (AK). Briefly, soil pH was determined with a soil–water (1:5, w/v) slurry using a compound electrode; OM was measured using the Tyurin method; TN was determined by the Kjeldahl method; AN was measured with the alkali solution diffusion method; AP was extracted with NaHCO3 and determined by the ammonium molybdate ascorbic method; and ammonium acetate extraction – flame photometry was used to measure AK.
Metabolite Extraction
The process of metabolite extraction for ultra-high performance liquid chromatography-tandem mass spectrometry (UHPLC-MS/MS) was as follows, according to previous methods (De Vos et al., 2007; Li et al., 2018). Use the frozen samples to ground into fine powders with liquid nitrogen, and then a total of 100 mg powder was weighed in centrifuge tubes. Afterward, 400 μL 80% (v/v) methanol aqueous solution (pre-cooled at –20°C) was added, followed by vortexing and oscillating for 30 s (Vortex Mixer, QL-866). The homogenates were subsequently placed at –20°C for 60 min and centrifuged at 14,000 × g, at 4°C for 20 min (Thermo Fisher Scientific, ST16R). All the supernatant was then transferred into 1.5-mL Eppendorf tubes, frozen, and dried under vacuum (Labogene, Scan Speed 40). The residue was dissolved with 100 μl complex solvents, vortexed and oscillated again, and centrifuged at 14,000 × g, at 4°C for 15 min. Finally, the supernatant was purified by passing through a 0.22-μm membrane filter for LC-MS detection. To monitor and evaluate the system stability throughout the experiment, equal amounts of supernatant from each processed sample were mixed as quality control (QC) samples. Meanwhile, take the blank matrix of the experimental sample as the blank samples, which composed of the reagents used before and were equally treated to the experimental samples. Blank samples also underwent analysis to remove the background ions.
UHPLC-MS/MS Analysis for Untargeted Metabolomics
The chromatographic separation was performed using an Accucore HILIC column (50 mm × 2.1 mm, 2.6 μm, Thermo Fisher ScientificTM, United States) fitted to the Thermo Scientific Vanquish UHPLC system. Metabolites were eluted from the column using a gradient mobile phase that consisted of phase A (0.1% formic acid, 10 mM ammonium acetate, and 95% acetonitrile) and phase B (0.1% formic acid, 10 mM ammonium acetate, and 50% acetonitrile), at a flow rate of 0.3 mL/min. A volume of 5 μL per sample was injected after equilibration. The temperature of the column and auto-sampler was maintained at 40 and 4°C, respectively. The linear gradient elution procedure was set as follows: 2% B for 0–1 min, from 2% B at 1 min to 50% B at 17 min until 17.5 min, then returning to the initial gradient conditions (2% B) at 18 min, followed by maintaining at 2% B for 18–20 min. In order to avoid the influence caused by the detected signal fluctuation of the apparatus, samples were randomly injected. A QC sample followed by a blank sample was injected after every five experimental sample injections.
The electrospray ionization-mass spectrometry (ESI-MS) experiments were carried out using a Thermo Q ExactiveTM HF-X mass spectrometer with a spray voltage of 3.2 kV in both positive and negative modes. The sheath gas and auxiliary gas flow rate were set at 35 and 10 arbitrary units, respectively. The capillary temperature was 320°C. The MS analysis alternated between MS full scans and data-dependent MS/MS scans with dynamic exclusion, and the mass scan range was selected from 100–1500 m/z at a scan rate of 40 Hz.
Data Acquisition and Processing
After the detection and analysis of truffle ascocarps by UHPLC-MS/MS methods, the total ion chromatograms of all the samples were extracted. The acquired raw MS files were processed with the Compound Discoverer (Thermo Fisher Scientific) software for data pretreatments including peak identification, peak alignment, peak feature extraction, and peak area normalization, running separately under positive and negative ionization mode (Ganna et al., 2015; Yu et al., 2018).
Firstly, screening for the retention time (RT), mass-to-charge ratio (m/z) and other parameters, and the peak alignment of different samples was conducted according to the retention time deviation of 0.2 min and the mass deviation of 5 ppm in order to make the identification more accurate. Then, the peak extraction was performed according to the set information and adduct information: mass deviation = 5 ppm, signal strength deviation = 30%, signal-to-noise ratio = 3, minimum signal strength = 100000. Additionally, the peak area was quantified. The mass spectrometry matrix data containing sample names, m/z-retention time pairs, and ion intensity information were generated and exported. The target ions were then integrated to predict the molecular formula and compared against ChemSpider and mzCloud online databases for the identification and confirmation of the compounds. The background ions were removed with blank samples, and the quantitative results were normalized with QC samples. Finally, the identification and quantitative results from the data were obtained and used for subsequent statistical analysis.
Statistical Analysis
Multivariate statistical analysis of metabolites, including principal component analysis (PCA) and partial least squares-discriminant analysis (PLS-DA), were carried out by SIMCA-P software (v13.0, Umetrics, Umeå, Sweden) after Pareto scaling, in order to reveal the differences in the metabolic composition among the ascocarps of the five different Tuber species. The reliability of the PLS-DA model was verified by permutation test, which was used to evaluate whether the model was overfitted. An independent samples Kruskal–Wallis one-way analysis of variance (ANOVA) followed by the Dunn–Bonferroni post hoc method was used for the pairwise multiple comparisons of each metabolite (IBM® SPSS® v22). Additionally, variable importance in the projection (VIP) value of the first principal component in the PLS-DA model combined with fold change (FC) were also computed to screen out the significantly differential metabolites. The threshold was set as: VIP > 1, corrected P < 0.05, and FC > 2.0 or FC < 0.5. The volcano plots made by R package DESeq2 were used to analyze the differential log2-FC- and log10-P-transformed values of each metabolite. In addition, hierarchical clustering analysis (HCA) was performed with the pheatmap-package in R software (v3.3.2) to visualize the metabolite profiles and reveal the relationship between metabolites and samples. Furthermore, the biological significance of metabolites was uncovered through the functional analysis of metabolic pathways using the Kyoto Encyclopedia of Genes and Genomes (KEGG) pathway analysis. The KEGG database provides an excellent integrated metabolic pathway query and is helpful for metabolic analysis and metabolic network research (Kanehisa, 2016). The hypergeometric test was used to determine the significantly enriched KEGG pathways of differential metabolites by comparison with all the identified metabolites.
Statistical analysis of the soil properties’ data was performed by one-way analysis of variance (ANOVA) using SPSS 22.0. Least significant difference (LSD) was used to test if the results between different treatments were significant at P < 0.05.
Results
The Differences of the Soil Properties Associated With Five Different Truffle Species
The properties of the soils from different truffle sampling locations were shown in Table 2, which reflected the significant differences in the basic characteristics of the soils around the five truffle species. The pH was significantly higher in the soil of T. melanosporum (P < 0.05), which presented alkaline, while the soils around the other four truffles were slightly acidic. The soil pH of T. sinoaestivum and T. pseudoexcavatum were significantly higher than that of T. indicum and T. panzhihuanense (P < 0.05). The OM content of the soils around the five truffles is significantly different from each other (P < 0.05), with the highest OM content in the soil of T. sinoaestivum and the lowest in T. panzhihuanense. The soil associated with T. pseudoexcavatum showed the highest TN content, followed by T. indicum (P < 0.05). However, the AN content was highest in the soil of T. melanosporum, also followed by T. indicum (P < 0.05). The content of both TN and AN were the lowest in the soil of T. panzhihuanense. There was significantly more AP in the soil of T. sinoaestivum and significantly more AK in the soil of T. indicum and T. panzhihuanense compared with the other soil samples (P < 0.05).
Untargeted Metabolomic Profiling of Five Truffle Species – Quality Control and Metabolite Quantitative Analysis
The correlation of QC samples indicated that the system stability and the quality of the acquired data were fine (Supplementary Figure S2), and the correlation coefficient (R2) of QC samples in positive ion mode was higher than that in negative ion mode. Additionally, the PCA plots of all samples showed that the distribution of QC samples was clustered together (Supplementary Figure S3), which also indicated satisfactory data quality.
A comparison of the total ion chromatograms of the extracts (Figure 2) showed that the types of metabolites in the ascocarps of five truffle species were similar, but the content of many metabolites was different. From the five Tuber species, a total of 7278 and 2460 metabolites were detected under positive and negative ion mode, respectively, of which 2376 and 843 named metabolites in positive and negative ion mode were finally identified and confirmed, respectively. The total ion intensity of all the identified metabolites in the five different truffle species is shown in a violin plot (Supplementary Figure S4), which reflects the intensity and density distribution of each metabolite in different groups at the overall level. It was found that the metabolite intensity of each truffle species was obviously higher under positive ion mode compared with negative ion mode, and the metabolites of T. melanosporum exhibited greater average ion intensity than other truffle species under positive ion modes. The distribution range of metabolite intensity for each truffle species was similar in positive ion mode.
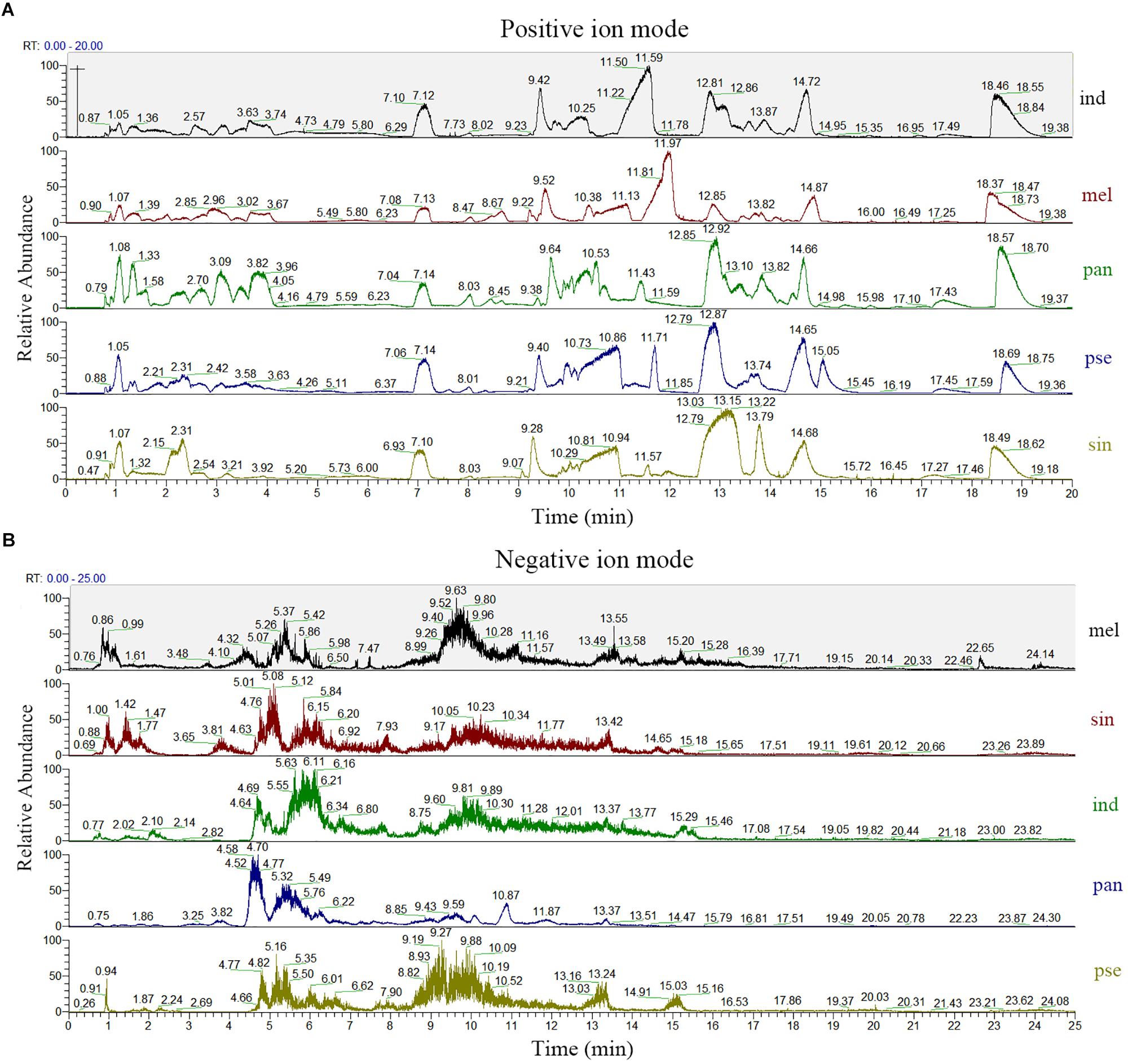
Figure 2. The total ion chromatogram of the five truffle species in both positive (A) and negative (B) ion modes. Abbreviations: ind, Tuber indicum; mel, Tuber melanosporum; pan, Tuber panzhihuanense; sin, Tuber sinoaestivum; pse, Tuber pseudoexcavatum.
According to the results reported above, because more metabolites were identified and data of higher quality were acquired in positive ion mode, the subsequent analysis was carried out under this mode.
The Metabolic Variations Among the Five Truffle Species
The PC analysis of the 2376 metabolites (except for the QC samples) was displayed in Figure 3. The first two principal components of the PCA score plot were responsible for 53.27% (38.88% for PC1 and 14.39% for PC2) of the overall variance of the metabolite profiles, showing a clear separation of these five truffle species. Therefore, the metabolite profiles of the five truffle species were different from each other. There was a great degree of separation between T. melanosporum and the other four truffle species, indicating that the metabolic profiles of T. melanosporum were prominently different from those of the other four truffles. Additionally, T. melanosporum was separated from the other species mainly along PC1. However, the differences in the metabolic profiles among T. indicum, T. panzhihuanense, and T. pseudoexcavatum were relatively small due to the closer distribution of the three truffle species in the PCA plot, and these three truffles were separated from T. sinoaestivum by a great distance along PC2. To further verify the difference in metabolic profiles among these truffles, PLS-DA was carried out, which showed a clear separation for pairwise comparison of the five truffles (Supplementary Figure S5). The validation of the PLS-DA models presented a high interpretation rate (R2Y) and prediction degree (Q2 > 0.5), indicating a higher reliability of these models (Supplementary Figure S6).
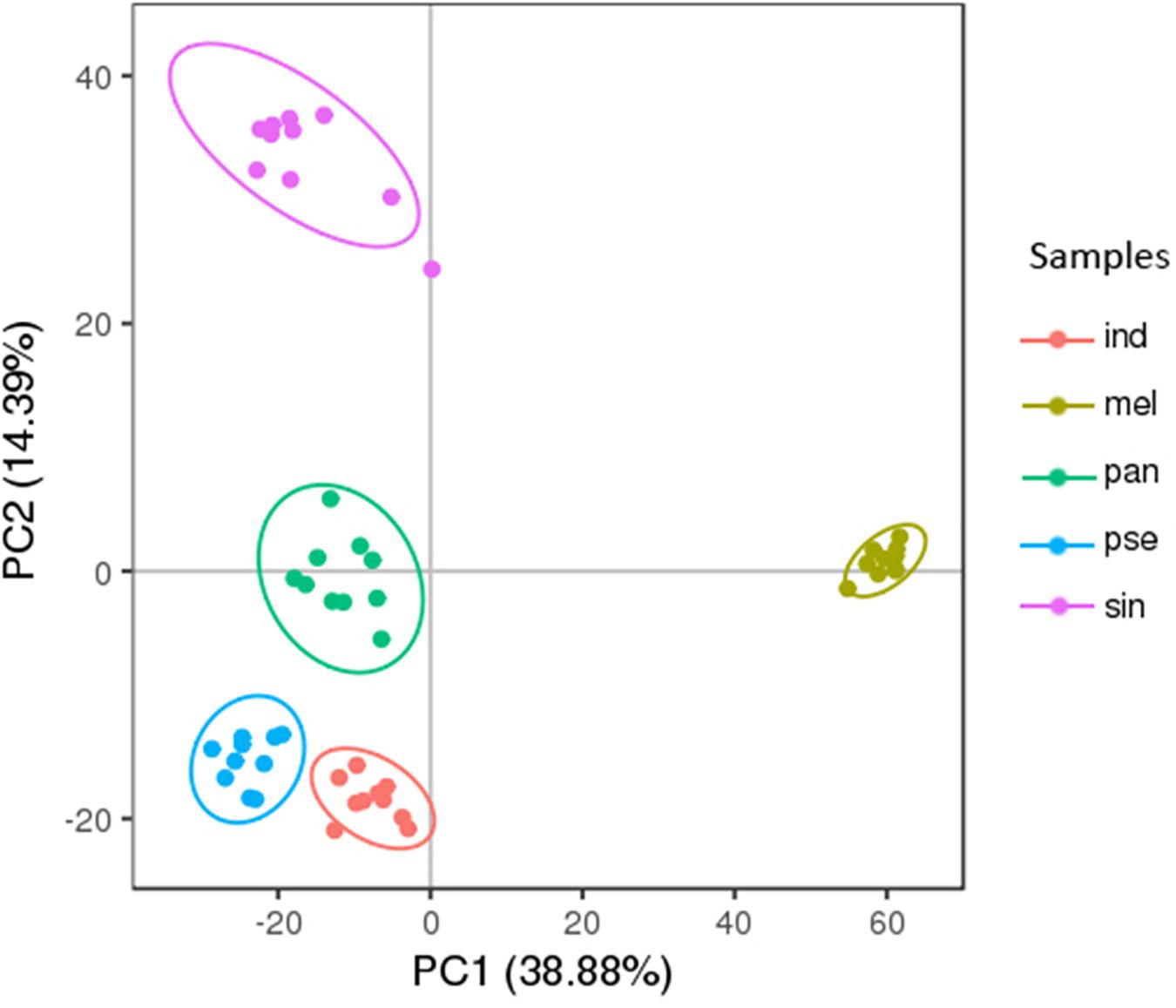
Figure 3. The principal component analysis (PCA) score plots of the five truffle species in positive ion mode. Abbreviations: ind, Tuber indicum; mel, Tuber melanosporum; pan, Tuber panzhihuanense; sin, Tuber sinoaestivum; pse, Tuber pseudoexcavatum.
Screening and Classification of the Differential Metabolites
A total of 1282 potential metabolites with significant differential amounts among the five truffle species were screened by statistical analysis, and the profiling overview of these differential metabolites in pairwise comparison of the five truffle species is shown in the heatmaps and volcano plots in Figure 4. The number of differential metabolites between T. melanosporum and T. sinoaestivum was the greatest, while the number of those between T. panzhihuanense and T. pseudoexcavatum was the smallest. There were clearly more upregulated differential metabolites for T. melanosporum when compared with the other four truffles, but there were more downregulated metabolites in T. pseudoexcavatum when compared with the others.
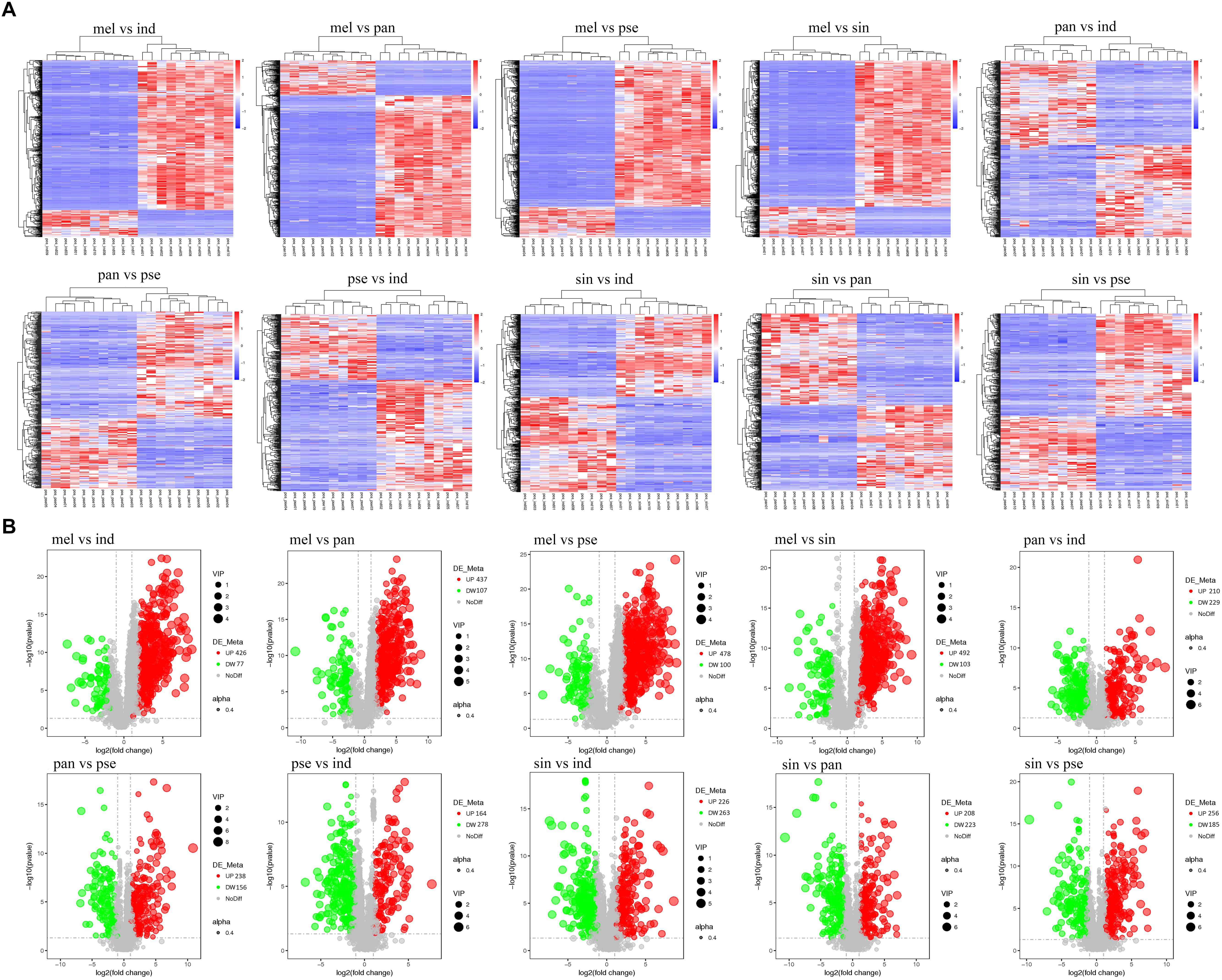
Figure 4. The (A) heatmaps and (B) volcanic plots of differential metabolites in pairwise comparison among the five truffle species. Abbreviations: ind, Tuber indicum; mel, Tuber melanosporum; pan, Tuber panzhihuanense; sin, Tuber sinoaestivum; pse, Tuber pseudoexcavatum.
All the 1282 differential metabolites were assigned to various chemical categories, including amino acids, saccharides, nucleosides and their analogs, organic acids, alkaloids, flavonoids, carnitines, and others. From another classification perspective, these metabolites covered amides, ketones, phenols and alcohols, esters, sulfur compounds, and others. The numbers of the differential metabolites that accumulated with the greatest frequency in each truffle species for some of these chemical categories were calculated (Figure 5A). In the nine selected categories shown in Figure 5A, compared with the other four truffles, the numbers of metabolites that predominantly accumulated in T. melanosporum were the most. Except for T. melanosporum, the numbers of saccharides and nucleosides, organic acids, esters, and sulfur compounds that accumulated with the greatest frequency in T. sinoaestivum were the maximum, and there was a greater number of phenols and alcohols accumulated most in T. indicum compared with the remaining three truffles, with the opposite occurring for amino acids and alkaloids. There were less predominantly accumulated organic acids, saccharides, and nucleosides in T. panzhihuanense and T. pseudoexcavatum.
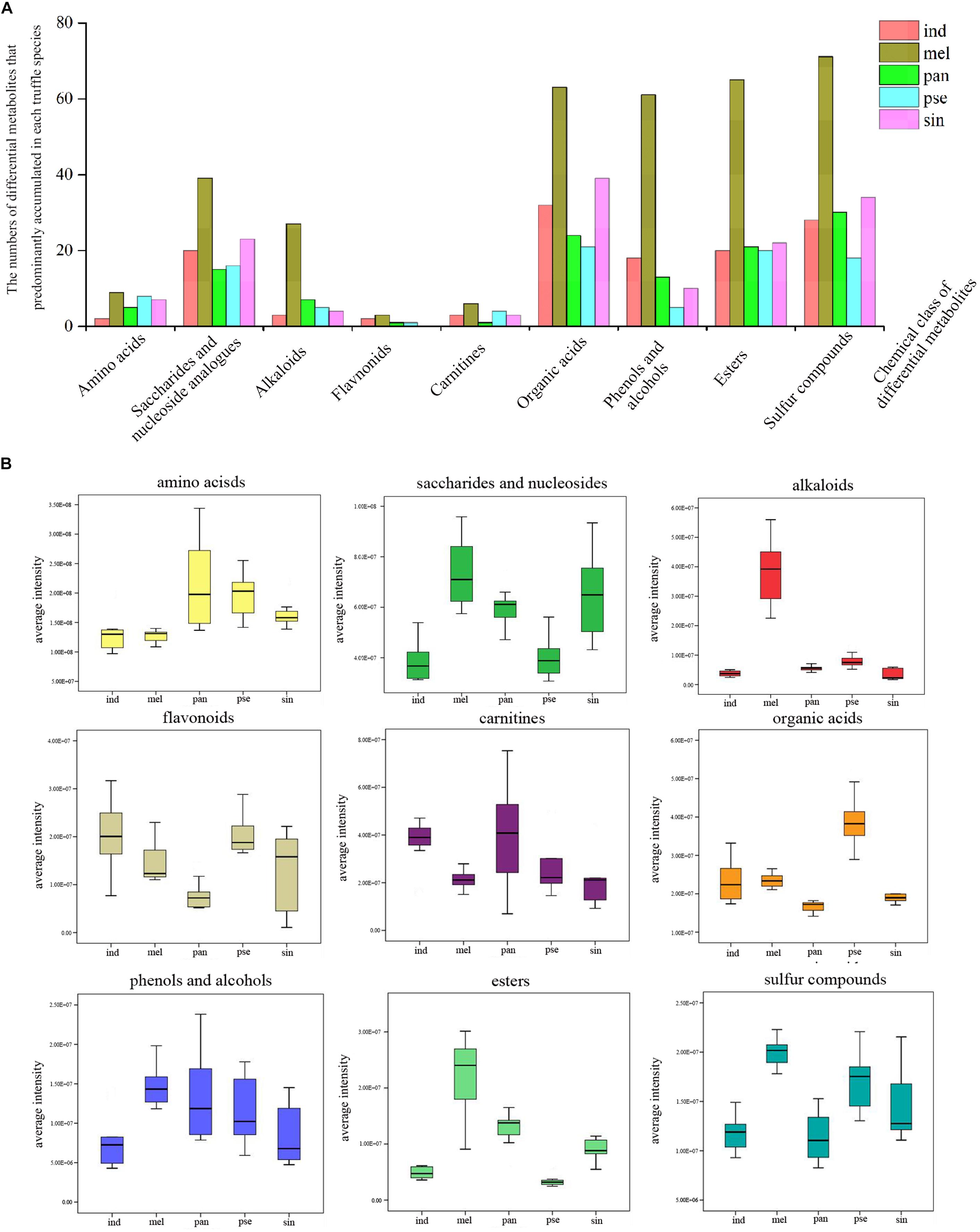
Figure 5. (A) The numbers of the differential metabolites with the greatest amounts in each truffle species compared with the other truffles and (B) the average ion intensity in each chemical class in the five truffle species. Abbreviations: ind, Tuber indicum; mel, Tuber melanosporum; pan, Tuber panzhihuanense; sin, Tuber sinoaestivum; pse, Tuber pseudoexcavatum.
Differential Metabolite Analysis of the Five Truffle Species
The average ion intensities of the differential metabolites belonging to the nine selected categories are displayed in Figure 5B, and each category showed a significant difference among the five truffle species (P < 0.05). The important differential metabolites with pharmacological activities or with more attention before in each category are displayed in Table 3. Amino acids had the strongest intensities in T. panzhihuanense and T. pseudoexcavatum, and were significantly higher than that in T. melanosporum and T. indicum (P < 0.05). Among these differential amino acids, significantly more ornithine, asparagine, isoleucine, cysteine, and citrulline were detected in T. sinoaestivum (P < 0.05). Threonine showed significantly higher amounts in T. pseudoexcavatum (P < 0.05), and T. melanosporum contained more proline and leucine compared with the other truffles. There was more methionine and taurine in T. indicum and more valine in T. panzhihuanense, respectively.
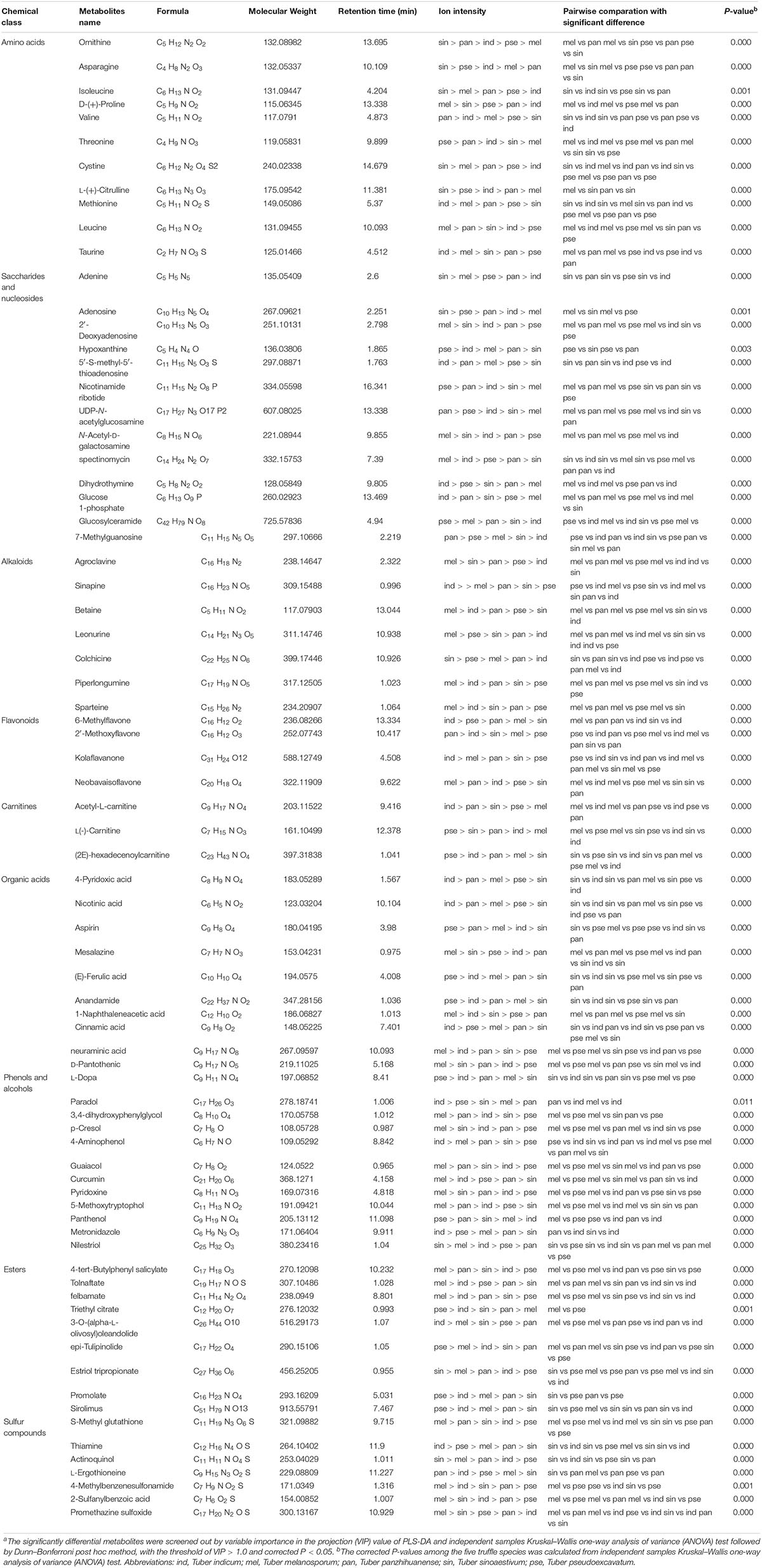
Table 3. Some of the significantly differential metabolites among the five truffle species and their chemical categoriesa.
Saccharides and nucleosides showed higher intensities in T. melanosporum (P < 0.05). Some metabolites, such as 2′-deoxyadenosine, N-acetyl-D-galactosamine, and spectinomycin, had the most amounts in T. melanosporum, while they exhibited significantly lower average ion intensities in T. pseudoexcavatum and T. panzhihuanense (P < 0.05). However, some metabolites such as hypoxanthine, nicotinamide ribotide, UDP-N-acetylglucosamine, 7-methylguanosine, and glucosylceramide were detected in greater amounts in T. panzhihuanense and T. pseudoexcavatum compared with the other truffles. T. sinoaestivum contained significantly greater amounts of adenine, adenosine, and succinyladenosine (P < 0.05).
Alkaloids, esters, sulfur compounds, phenols, and alcohols all showed the most strongly intensities in T. melanosporum (P < 0.05), particularly the alkaloids, including agroclavine, leonurine, betaine, piperlongumine, and sparteine. There was more sinapine and colchicine in T. indicum and T. sinoaestivum, respectively. As for phenols and alcohols, there was greater amounts of 3,4-dihydroxyphenylglycol, p-cresol, guaiacol, curcumin, pyridoxine, and 5-methoxytryptophol in T. melanosporum. However, paradol, 4-aminophenol, and metronidazole were more in T. indicum. The average ion intensity of carnitines was significantly higher in T. indicum than in T. melanosporum (P < 0.05), similar to that of acetyl-L-carnitine and (2E)-hexadecenoyl carnitine.
Organic acids and flavonoids exhibited the highest average ion intensities in T. pseudoexcavatum and T. indicum, with significantly lower amounts in T. panzhihuanense (P < 0.05). In terms of organic acids, there were greater amounts of mesalazine, 1-naphthaleneacetic acid, neuraminic acid, and D-pantothenic acid in T. melanosporum, with greater amounts of 4-pyridoxic acid and nicotinic acid in T. indicum. T. pseudoexcavatum contained more aspirin and (E)-ferulic acid, while T. sinoaestivum had significantly less anandamide and cinnamic acid. Among the few differential flavonoids, 6-methylflavone and kolaflavanone exhibited significantly higher intensity in T. indicum, and neobavaisoflavone was more in T. melanosporum and T. panzhihuanense compared with the other truffles.
Metabolic Pathway Analysis Based on the KEGG Database
There were 235 identified metabolites and 149 differential metabolites annotated by the KEGG database. The differential metabolites among five truffle species covered a total of 110 pathways or metabolisms, including vitamin digestion and absorption, vitamin B6 metabolism, serotonergic synapse, drug metabolism-cytochrome P450, amino sugar and nucleotide sugar metabolism, ABC transporters, sulfur metabolism, microbial metabolism in diverse environments, biosynthesis of secondary metabolites, central carbon metabolism in cancer (Supplementary Table S1), and the 20 most enriched pathway terms are shown in the KEGG enrichment bubble diagrams (Figure 6). The content of the metabolites annotated to vitamin B6 metabolism was relatively less in T. pseudoexcavatum as compared to the other truffles, while the metabolites annotated to fatty acid metabolism were more in T. pseudoexcavatum. The metabolites annotated to the drug metabolism-cytochrome P450 and sulfur metabolism pathways were upregulated in T. indicum (Supplementary Figure S7a). There was higher ion intensity of annotated metabolites from cysteine and methionine metabolism in T. melanosporum and arachidonic acid metabolism in T. panzhihuanense (Supplementary Figure S7b). Many pathways, such as microbial metabolism in diverse environments, biosynthesis of secondary metabolites, mineral absorption, and biosynthesis of amino acids, involve multiple annotated metabolites, but the variation of these metabolites amounts among the five truffle species (upregulation or downregulation) was inconsistent.
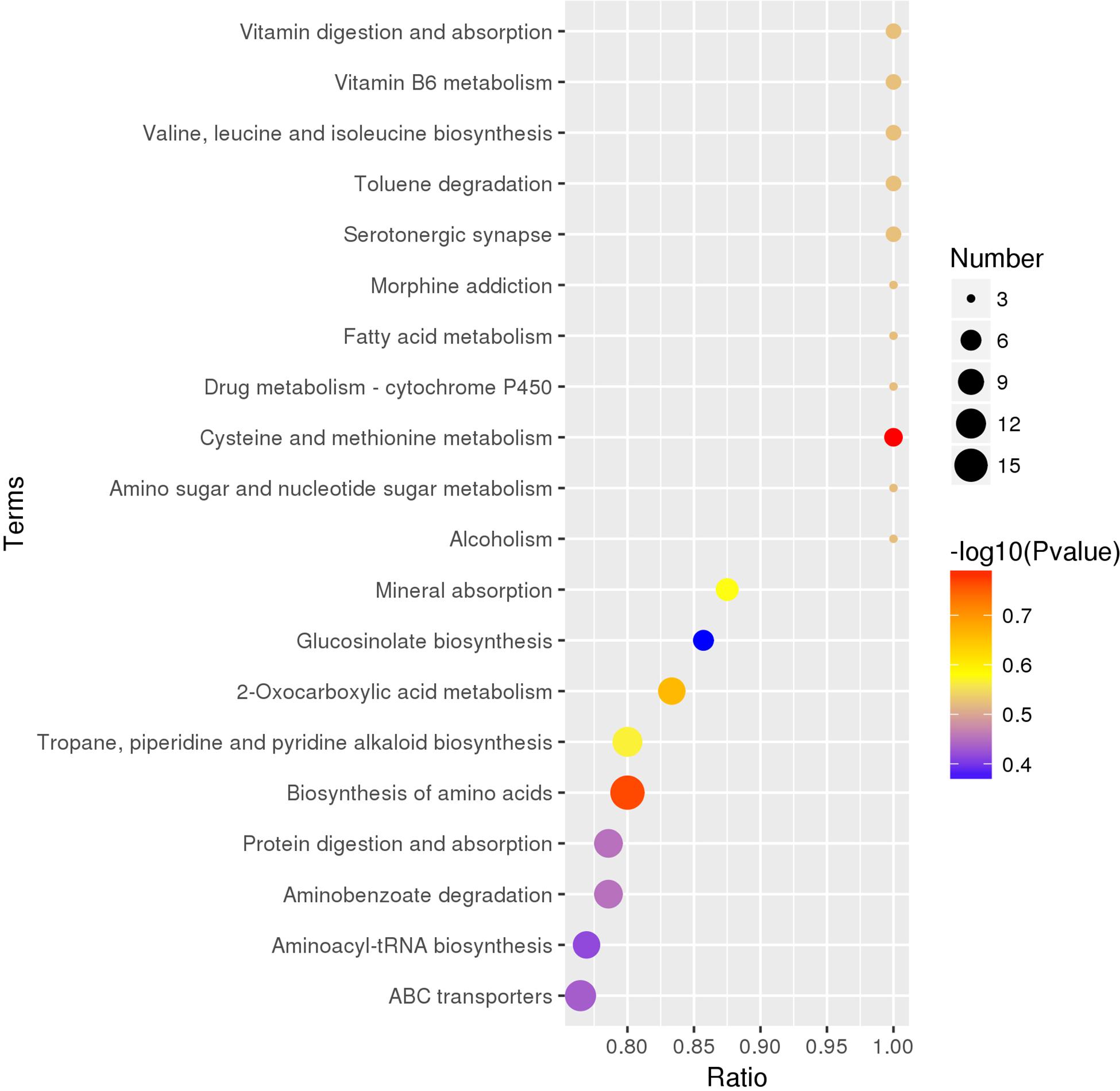
Figure 6. The top 20 enriched KEGG pathway terms covered by differential metabolites. On the abscissa is the ratio of the differential metabolite numbers in the corresponding pathway to the total identified metabolite numbers in this pathway, and the larger the ratio value, the higher the degree of enrichment of differential metabolites in this pathway. The color of the point represents the transformed P-value of the hypergeometric test, and the smaller the value, the greater the reliability and statistical significance of the test. The size of the dots represents the number of differential metabolites in the corresponding pathway, and the larger the size, the greater the number of differential metabolites within the pathway.
Discussion
It is important for the investigations that gather data related to the metabolites of truffle fruiting bodies, which is not only because of the unique flavor of truffles that is desired in various cuisines, but also because the knowledge regarding their nutritional value, biological activity, and the potential therapeutic usages is limited, and is in need of additional exploration (Wang and Marcone, 2011; Tang et al., 2015). With the development of technology, liquid chromatography coupled to mass spectrometry approach can be widely applied in metabolomics studies currently, having a wide detected range and the high specificity and sensitivity (Velez et al., 2007). In the current study, this method was used to analyze the metabolic profiling of truffle fruiting bodies of five species (T. indicum, T. melanosporum, T. panzhihuanense, T. sinoaestivum, and T. pseudoexcavatum), which showed satisfactory data quality. Except for T. melanosporum and T. indicum, previous studies on the metabolites of the other three truffle species have been rare.
The results in this study revealed that there were significant differences in the metabolic characteristics among the five truffle species, especially between T. melanosporum and the other four truffles. The difference between T. indicum and T. pseudoexcavatum was relatively small. Besides the genetic reasons, combined with the different host plants and different soil properties around the ascocarps from these different truffle species, it should be because these truffles have different ecological, nutritional, climatic and geographical needs. In other studies, it was found that the metabolite composition was complex, and the metabolite accumulation was variable because it was greatly affected by genetic and environmental factors (Bertault et al., 1998; Mannina et al., 2012; Splivallo et al., 2012; Tang et al., 2017), such as growth characteristics, development stage, preservation conditions, and geographical origins. In this study, we detected the metabolites in the reproductive stage of truffles, and the mature degree and preservation conditions of truffle fruiting bodies were basically the same, however, the different geographical location led the significant difference of their ecology, like the vegetation (host plants) and soil we had investigated. The host plants of T. melanosporum belongs to Quercus while the other four truffles’ host plants are Pinus; and T. melanosporum grow in the alkaline soil while the other truffles in this study adapted to slightly acidic soils, which showed the obvious differences of the ecological environment between T. melanosporum and the other four truffles. This should be closely related to the significant differences in the metabolic characteristics between T. melanosporum and the other four truffles. In terms of other soil properties which all showed the obvious differences, the basic nutrient status of the soil associated with T. indicum was relatively good overall and the soil nutrients of T. panzhihuanense were poor compared with the soils around the other truffles. These soil characteristics may also related to the variation of metabolites in truffle fruiting bodies, which need further exploration of the specific effects. In previous studies, Diaz et al. (2003) compared the aroma profiles of T. melanosporum and T. aestivum at different geographical zones, and indicated that different truffle aroma metabolites could result from a difference in both truffle species and geographical areas of origin, which had the similarity with our study. From the perspective of phylogenetic analysis of these five truffle species in this study, T. indicum was phylogenetically related to T. melanosporum (Zhang et al., 2019), and T. sinoaestivum had relatively closer phylogenetical relationship with T. melanosporum and T. indicum compared with the other two truffle species, but their metabolic profiling was clearly different from each other. Previous researches said that the diverse ecological environment may cause a high level of genetic variability, which finally led to the obvious difference in metabolites (Bertault et al., 1998). Except for above factors, the effect of microbial communities on the metabolites variation in truffle fruiting bodies could not be ignored, because truffle fruiting bodies harbor a diverse microbes, including bacteria and fungi, etc., and they play an important role in the truffle ascocarps formation as well as their aroma (Antony-Babu et al., 2014; Vahdatzadeh et al., 2015). So the metabolites produced by microbes were also included in truffle fruiting bodies, which could not been seen as a single organism to investigate. Meanwhile, the species of truffle, the soil properties and host plants all had the influence on the microbial communities (Vahdatzadeh et al., 2015; Benucci and Bonito, 2016; Ye et al., 2018), which may finally lead the differences of metabolic profiling in truffle fruiting bodies. So the research on the truffle-associated microbiome and the related metabolites from these microbiome was an important step in future for further exploring the metabolic mechanism of truffles. And the associated microbiome may play an important role of bridge for the correlation between truffle metabolic profiling and the specific ecological environment or growth stage.
As for the detected metabolites, although thetotal ion intensity of all the identified metabolites was different in the five truffles, which was higher in T. melanosporum, the types of these metabolites were similar. A total of 1282 metabolites with significantly differential amounts among the five truffle species were detected, accounting for approximately 54% of the 2376 identified metabolites, which covered various chemical classes including amino acids, saccharides, nucleosides and their analogs, organic acids, alkaloids, flavonoids, carnitines, amides, ketones, phenols and alcohols, esters, sulfur compounds, and others. Although previous studies also detected these chemical categories in other truffle species (Wang and Marcone, 2011; El Enshasy et al., 2013; Patel et al., 2017), few mentioned on the nucleosides and their analogs, alkaloids, carnitines, or flavonoids in the truffle fruiting bodies.
As was reported, nucleosides and their analogs exhibited widely varied biochemical activities, such as antiviral and anticonvulsant activity, and maintaining the immune response (Ballarin et al., 1987; Liu et al., 2011). Adenine, adenosine, 2′-deoxyadenosine, 7-methylguanosine, and 5′-S-methyl-5′-thioadenosine were detected in our study, as well as in previous studies (Liu et al., 2011; Longo et al., 2017), and the average content of saccharides, nucleosides, and their analogs was significantly higher in T. melanosporum, T. sinoaestivum, and T. panzhihuanense. Adenine is an electron-rich substance known to bind to electrical ions and interfere with oxidative protection (Goncçalves et al., 2010), and their amounts were greater in T. sinoaestivum and T. melanosporum.
Alkaloids from herbs exhibited wide biological and pharmacological activities (Fang et al., 2011), and had been identified in these five truffles, with a significantly greater amounts in T. melanosporum. Many of the alkaloids had antioxidant, anti-tumor, anti-inflammatory, or antidepressant-like effects, such as colchicine, sinapine, leonurine, and piperlongumine (Guo et al., 2016; Kumar et al., 2016; Jia et al., 2017; Karki et al., 2017), which were predominant in T. sinoaestivum, T. indicum, and T. melanosporum. The number of differential carnitines and flavonoids was fewer, and in T. indicum, their ion intensity was higher. Acetyl-L-carnitine, a powerful antioxidant that can easily penetrate the blood–brain barrier to the central nervous system, has an obvious protective effect on nerves (Ferreira and McKenna, 2017). There was significantly more of it in T. indicum and T. panzhihuanense. Neobavaisoflavone is also a bioactive compound that was isolated from Psoralea corylifolia, and possesses significant anti-inflammatory and anti-cancer functions (Szliszka et al., 2011). Great amounts of it were found in T. melanosporum and T. panzhihuanense.
As was demonstrated, among the aromatic metabolites, aldehydes and alcohols were the two most abundant aromatic components in truffles, while the sulfur-containing compounds such as dimethyl sulfide and dimethyl disulfide were considered the key components to human sensory interpretation (Splivallo et al., 2007; Splivallo and Ebeler, 2015). Esters, sulfur compounds, phenols, and alcohols are the metabolites involved in truffle aroma which received much attention in previous researches (Vahdatzadeh et al., 2015), and were also detailly described in our study. Consistently, they were all more abundant in T. melanosporum. Many phenolic compounds, such as paradol, p-cresol, 4-aminophenol, guaiacol, and curcumin, exhibit bioactivities, having anti-inflammation, antioxidation, antimicrobial, and antitumoral characteristics (Villares et al., 2012; Lee and Lee, 2014; Gaire et al., 2015), and most of them were found in greater amounts in T. melanosporum and T. indicum. Another study (Patel et al., 2017) showed that T. melanosporum contained anandamide, a fatty acid neurotransmitter that is an immunomodulator of the central nervous system and was found to have the ability to lead in the process of cancer cell apoptosis (Patsos et al., 2010; Patel et al., 2017). In our study, this metabolite existed in the all five truffle species, but with significantly greater amounts in T. sinoaestivum. Wang and Marcone summarized the nutritional content variation in different truffle species, such as T. magnatum, T. borchii, and T. aestivum (Wang and Marcone, 2011), and similarly, the five truffle species in our study also differed in their nutritional content, e.g., amino acids, although the truffle species we used was different from this previous study.
The metabolic pathways of the metabolites were also analyzed according to the KEGG database, which reflected the most important biochemical metabolic pathway and signal transduction pathway involved by metabolites that had differential amounts patterns. Truffles produced metabolic molecules during each stage of their growth for necessary survival, and the metabolites from truffles change during their life cycle and also during the maturity stage of the truffles (Streiblova et al., 2012; Mello et al., 2013). To research their metabolism mechanisms, it would be helpful to roundly explore the formation mechanism of truffle ascocarps. At present, it is found that the truffle formation is the result of the sexual reproduction of the fungi, and the stages of the development of T. melanosporum are described in previous studies, but some parts of their life cycle are also missing (Murat et al., 2013; Le Tacon et al., 2016). A previous report examined the metabolic profiles of the ectomycorrhizae of T. indicum, showing the metabolic pathways of T. indicum ectomycorrhizae, which include linoleic acid metabolism, and dalanine, aspartate, and glutamate metabolism (Li et al., 2018). However, the metabolic pathways of truffle fruiting bodies in our study were obviously different from those. In this study, a total of 110 pathways or metabolisms were covered by the differential metabolites among five truffle species. The most enriched pathway terms were vitamin digestion and absorption, vitamin B6 metabolism, and valine, leucine, and isoleucine biosynthesis. However, most metabolic reactions involved multiple metabolites, and the variation of these metabolites amounts among the five truffle species was inconsistent. Therefore, it cannot be simply said that the expression of some metabolic pathways was increased or decreased in a certain truffle species, which indicates that there were complex changes in the metabolic mechanisms in truffle fruiting bodies that require our further investigation.
In terms of the methods in this study, using untargeted analysis, metabolomics can be used to simultaneously detect and quantify a wide range of compounds that cover various chemical classes, which is ideal for the detection of unexpected changes or unknown information in metabolite levels (Bloszies and Fiehn, 2018; Gomez-Gomez et al., 2019). Also in this study, a lot of amounts of the metabolites were found in different truffle species, which would broaden the knowledge of metabolites of different truffle species in different environments. Meanwhile, the LC-MS approach also contributes to a better result due to its intrinsic selectivity, specificity and sensitivity (Gomez-Gomez et al., 2019). In the future research about truffles, untargeted metabolomics can also be considered to use, such as investigating the metabolic variations in different stage of truffles, under different storage conditions, in a specific environment, or in the fermentation process. Additionally, the genomic and transcriptomics data can be connected to the construction of genome metabolic network of truffles, which would be helpful to systematically understand truffles and realize the truffles artificial cultivation. However, the technical challenges of this approach are also exist, such as filtering procedures improvement for untargeted LC-MS metabolomics data (Schiffman et al., 2019), completing the functional database for metabolomics research.
In conclusion, through the LC-MS-based metabolomics method, the significantly different metabolic profiles of these five commercial truffle species were obtained, with the biggest difference between T. melanosporum and the other four truffles, which should be the results of comprehensive effects of genetic factors and different ecology, including different truffle species, host plants, and soil characteristics. The amounts of many metabolites were different in five truffles and every truffle species had their predominantly accumulated metabolites. The chemical categories of the differential metabolites were rich among the five truffle species, which covered a total of 110 pathways or metabolisms. Each of the nine categories with detailed description in this study all contained some metabolites with bioactivities that are beneficial to human beings, like acetyl-L-carnitine, neobavaisoflavone, anandamide. Generally, compared with the other four truffles, there were more compounds with higher intensity in T. melanosporum, which indicated that T. melanosporum has a higher value for utilization as a drug or food, and that it is reasonable for it to command a higher price. Besides, the maturity, the life cycle, and the associated microbes all had the effects on the metabolic profiles of different truffle species, which is interesting to combine these for the further exploration, aiming to better reveal the metabolic mechanism of truffles and may enhance the insight into the truffle research.
Data Availability Statement
The datasets generated for this study can be found in the NCBI database with GenBank accession numbers MN338092–MN338096.
Author Contributions
XL, XZ, and BZ conceived and designed the experiments. LY, ZK, DJ, and LFY performed the experiments. XZ and XL wrote and revised the manuscript. All authors approved the final version of the manuscript.
Funding
This work was supported by the National Natural Science Foundation of China (No. 31900079), Project of Sichuan Academy of Agricultural Sciences (2018LWJJ-012), Science and Technology Support Project in Sichuan Province (2016NYZ0040), and the Sichuan Mushroom Innovation Team.
Conflict of Interest
The authors declare that the research was conducted in the absence of any commercial or financial relationships that could be construed as a potential conflict of interest.
Acknowledgments
We thank LetPub (www.letpub.com) for its linguistic assistance during the preparation of this manuscript.
Supplementary Material
The Supplementary Material for this article can be found online at: https://www.frontiersin.org/articles/10.3389/fmicb.2019.02227/full#supplementary-material
References
Antony-Babu, S., Deveau, A., Van Nostrand, J., Zhou, J., Le Tacon, F., Robin, C., et al. (2014). Black truffle-associated bacterial communities during the development and maturation of Tuber melanosporum ascocarps and putative functional roles. Environ. Microbiol. 16, 2831–2847. doi: 10.1111/1462-2920.12294
Ballarin, M., Herrera-Marschitz, M., Casas, M., and Ungerstedt, U. (1987). Striatal adenosine levels measured ‘in vivo’ by microdialysis in rats with unilateral dopamine denervation. Neurosci. Lett. 83, 338–344. doi: 10.1016/0304-3940(87)90111-X
Benucci, G. M. N., and Bonito, G. M. (2016). The truffle microbiome: species and geography effects on bacteria associated with fruiting bodies of hypogeous pezizales. Microb. Ecol. 72, 4–8. doi: 10.1007/s00248-016-0755-3
Bertault, G., Raymond, M., Berthomieu, A., Callot, G., and Fernandez, D. (1998). Trifling variation in truffles. Nature 394, 734–734. doi: 10.1038/29428
Bloszies, C. S., and Fiehn, O. (2018). Using untargeted metabolomics for detecting exposome compounds. Curr. Opin. Toxicol. 8, 87–92. doi: 10.1016/j.cotox.2018.03.002
Campo, E., Marco, P., Oria, R., Parmo, D., and Venturini, M. E. (2017). What is the best method for preserving the genuine black truffle (Tuber melanosporum) aroma? An olfactometric and sensory approach. LWT Food Sci. Technol. 80, 84–91. doi: 10.1016/j.lwt.2017.02.009
De Vos, R. C., Moco, S., Lommen, A., Keurentjes, J. J., Bino, R. J., and Hall, R. D. (2007). Untargeted large-scale plant metabolomics using liquid chromatography coupled to mass spectrometry. Nat. Protoc. 2, 778–791. doi: 10.1038/nprot.2007.95
Deng, X. J., Liu, P. G., Liu, C. Y., and Wang, Y. (2013). A new white truffle species, Tuber panzhihuanense from China. Mycol. Prog. 12, 557–561. doi: 10.1007/s11557-012-0862-6
Diaz, P., Ibanez, E., Senorans, F. J., and Reglero, G. (2003). Truffle aroma characterization by headspace solid-phase microextraction. J. Chromatogr. A 1017, 207–214. doi: 10.1016/j.chroma.2003.08.016
El Enshasy, H., Elsayed, E. A., Aziz, R., and Wadaan, M. A. (2013). Mushrooms and truffles: historical biofactories for complementary medicine in Africa and in the middle East. Evid. Based Complement Alternat. Med. 2013:620451. doi: 10.1155/2013/620451
Fang, L., Liu, Y., Yang, B., Wang, X., and Huang, L. (2011). Separation of alkaloids from herbs using high-speed counter-current chromatography. J. Sep. Sci. 34, 2545–2558. doi: 10.1002/jssc.201000857
Ferreira, G. C., and McKenna, M. C. (2017). L-Carnitine and Acetyl-L-carnitine roles and neuroprotection in developing brain. Neurochem. Res. 42, 1661–1675. doi: 10.1007/s11064-017-2288-7
Fiehn, O., Kopka, J., Dormann, P., Altmann, T., Trethewey, R. N., and Willmitzer, L. (2000). Metabolite profiling for plant functional genomics. Nat. Biotechnol. 18, 1157–1161. doi: 10.1038/81137
Gaire, B. P., Kwon, O. W., Park, S. H., Chun, K. H., Kim, S. Y., Shin, D. Y., et al. (2015). Neuroprotective effect of 6-paradol in focal cerebral ischemia involves the attenuation of neuroinflammatory responses in activated microglia. PLoS One 10:e0120203. doi: 10.1371/journal.pone.0120203
Ganna, A., Fall, T., Salihovic, S., Lee, W., Broeckling, C. D., Kumar, J., et al. (2015). Large-scale non-targeted metabolomic profiling in three human population-based studies. Metabolomics 12:4. doi: 10.1007/s11306-015-0893-5
García-Montero, L. G., Díaz, P., Di Massimo, G., and García-Abril, A. J. M. P. (2010). A review of research on Chinese Tuber species. Mycol. Prog. 9, 315–335. doi: 10.1007/s11557-009-0647-8
Gomez-Gomez, A., Soldevila, A., Pizarro, N., Andreu-Fernandez, V., and Pozo, O. J. (2019). Improving liquid chromatography-tandem mass spectrometry determination of polycarboxylic acids in human urine by chemical derivatization. Comparison of o-benzyl hydroxylamine and 2-picolyl amine. J. Pharm. Biomed. Anal. 164, 382–394. doi: 10.1016/j.jpba.2018.10.055
Goncçalves, L. M., Batchelor-McAuley, C., Barros, A. A., and Compton, R. G. (2010). Electrochemical oxidation of adenine: a mixed adsorption and diffusion response on an edge-plane pyrolytic graphite electrode. J. Phys. Chem. 114, 14213–14219. doi: 10.1021/jp1046672
Guo, Y., Ding, Y., Zhang, T., and An, H. (2016). Sinapine reverses multi-drug resistance in MCF-7/dox cancer cells by downregulating FGFR4/FRS2alpha-ERK1/2 pathway-mediated NF-kappaB activation. Phytomedicine 23, 267–273. doi: 10.1016/j.phymed.2015.12.017
Jia, M., Li, C., Zheng, Y., Ding, X., Chen, M., Ding, J., et al. (2017). Leonurine exerts antidepressant-like effects in the chronic mild stress-induced depression model in mice by inhibiting neuroinflammation. Int. J. Neuropsychopharmacol. 20, 886–895. doi: 10.1093/ijnp/pyx062
Kanehisa, M. (2016). “KEGG Bioinformatics Resource for Plant Genomics and Metabolomics,” in Plant Bioinformatics. Methods in Molecular Biology, Vol. 1374, ed. D. Edwards (New York, NY: Humana Press), doi: 10.1007/978-1-4939-3167-5_3
Karki, K., Hedrick, E., Kasiappan, R., Jin, U. H., and Safe, S. (2017). Piperlongumine induces reactive oxygen species (ROS)-dependent downregulation of specificity protein transcription factors. Cancer Prev. Res. 10, 467–477. doi: 10.1158/1940-6207.Capr-17-0053
Kues, U., and Martin, F. (2011). On the road to understanding truffles in the underground. Fungal Genet. Biol. 48, 555–560. doi: 10.1016/j.fgb.2011.02.002
Kumar, A., Sharma, P. R., and Mondhe, D. M. (2016). Potential anticancer role of colchicine-based derivatives: an overview. Anticancer Drugs 28, 250–262. doi: 10.1097/CAD.0000000000000464
Le Tacon, F., Rubini, A., Murat, C., Riccioni, C., Robin, C., Belfiori, B., et al. (2016). Certainties and uncertainties about the life cycle of the Périgord black truffle (Tuber melanosporum Vittad.). Ann. For. Sci. 73, 105–117. doi: 10.1007/s13595-015-0461-1
Le Tacon, F., Zeller, B., Plain, C., Hossann, C., Bréchet, C., and Robin, C. (2013). Carbon transfer from the host to Tuber melanosporum mycorrhizas and ascocarps followed using a 13C pulse-labeling technique. PLoS One 8:e64626. doi: 10.1371/journal.pone.0064626
Lee, W., and Lee, D. G. (2014). An antifungal mechanism of curcumin lies in membrane-targeted action within Candida albicans. IUBMB Life 66, 780–785. doi: 10.1002/iub.1326
Li, Q., Li, X., Chen, C., Li, S., Huang, W., Xiong, C., et al. (2016). Analysis of bacterial diversity and communities associated with Tricholoma matsutake fruiting bodies by barcoded pyrosequencing in sichuan province, Southwest China. J. Microbiol. Biotechnol. 261, 89–98. doi: 10.4014/jmb.1505.05008
Li, Q., Yan, L., Ye, L., Zhou, J., Zhang, B., Peng, W., et al. (2018). Chinese black truffle (Tuber indicum) alters the ectomycorrhizosphere and endoectomycosphere microbiome and metabolic profiles of the host tree Quercus aliena. Front. Microbiol. 9:2202. doi: 10.3389/fmicb.2018.02202
Liu, P., Li, Y. Y., Li, H. M., Wan, D. J., and Tang, Y. J. (2011). Determination of the nucleosides and nucleobases in Tuber samples by dispersive solid-phase extraction combined with liquid chromatography-mass spectrometry. Anal. Chim. Acta. 687, 159–167. doi: 10.1016/j.aca.2010.12.025
Longo, E., Morozova, K., Loizzo, M. R., Tundis, R., Savini, S., Foligni, R., et al. (2017). High resolution mass approach to characterize refrigerated black truffles stored under different storage atmospheres. Food Res. Int. 102, 526–535. doi: 10.1016/j.foodres.2017.09.025
Mannina, L., Sobolev, A. P., and Capitani, D. (2012). Applications of NMR metabolomics to the study of foodstuffs: truffle, kiwifruit, lettuce, and sea bass. Electrophoresis 33, 2290–2313. doi: 10.1002/elps.201100668
Mello, A., Ding, G. C., Piceno, Y. M., Napoli, C., Tom, L. M., DeSantis, T. Z., et al. (2013). Truffle brules have an impact on the diversity of soil bacterial communities. PLoS One 8:e61945. doi: 10.1371/journal.pone.0061945
Mello, A., Murat, C., and Bonfante, P. (2006). Truffles: much more than a prized and local fungal delicacy. FEMS Microbiol. Lett. 260, 1–8. doi: 10.1111/j.1574-6968.2006.00252.x
Murat, C., Rubini, A., Riccioni, C., De la Varga, H., Akroume, E., Belfiori, B., et al. (2013). Fine-scale spatial genetic structure of the black truffle (Tuber melanosporum) investigated with neutral microsatellites and functional mating type genes. New Phytol. 199, 176–187. doi: 10.1111/nph.12264
Patel, S., Rauf, A., Khan, H., Khalid, S., and Mubarak, M. (2017). Potential health benefits of natural products derived from truffles: a review. Trends Food Sci. Technol. 70, 1–8. doi: 10.1016/j.tifs.2017.09.009
Patsos, H. A., Greenhough, A., Hicks, D. J., Al Kharusi, M., Collard, T. J., Lane, J. D., et al. (2010). The endogenous cannabinoid, anandamide, induces COX-2-dependent cell death in apoptosis-resistant colon cancer cells. Int. J. Oncol. 37, 187–193. doi: 10.3892/ijo-00000666
Patti, G. J. (2011). Separation strategies for untargeted metabolomics. J. Sep. Sci. 34, 3460–3469. doi: 10.1002/jssc.201100532
Savini, S., Loizzo, M. R., Tundis, R., Mozzon, M., Foligni, R., Longo, E., et al. (2017). Fresh refrigerated Tuber melanosporum truffle: effect of the storage conditions on the antioxidant profile, antioxidant activity and volatile profile. Eur. Food Res. Technol. 243, 2255–2263. doi: 10.1007/s00217-017-2927-x
Schiffman, C., Petrick, L., Perttula, K., Yano, Y., Carlsson, H., Whitehead, T., et al. (2019). Filtering procedures for untargeted LC-MS metabolomics data. BMC Bioinformatics 20:334. doi: 10.1186/s12859-019-2871-9
Splivallo, R., Bossi, S., Maffei, M., and Bonfante, P. (2007). Discrimination of truffle fruiting body versus mycelial aromas by stir bar sorptive extraction. Phytochemistry 68, 2584–2598. doi: 10.1016/j.phytochem.2007.03.030
Splivallo, R., and Ebeler, S. E. (2015). Sulfur volatiles of microbial origin are key contributors to human-sensed truffle aroma. Appl. Microbiol. Biotechnol. 99, 2583–2592. doi: 10.1007/s00253-014-6360-9
Splivallo, R., Valdez, N., Kirchhoff, N., Ona, M. C., Schmidt, J. P., Feussner, I., et al. (2012). Intraspecific genotypic variability determines concentrations of key truffle volatiles. New Phytol. 194, 823–835. doi: 10.1111/j.1469-8137.2012.04077.x
Streiblova, E., Gryndlerova, H., and Gryndler, M. (2012). Truffle brule: an efficient fungal life strategy. FEMS Microbiol. Ecol. 80, 1–8. doi: 10.1111/j.1574-6941.2011.01283.x
Szliszka, E., Czuba, Z. P., Sedek, L., Paradysz, A., and Krol, W. (2011). Enhanced TRAIL-mediated apoptosis in prostate cancer cells by the bioactive compounds neobavaisoflavone and psoralidin isolated from Psoralea corylifolia. Pharmacol. Rep. 63, 139–148. doi: 10.1016/S1734-1140(11)70408-X
Tang, W., Hazebroek, J., Zhong, C., Harp, T., Vlahakis, C., Baumhover, B., et al. (2017). Effect of genetics, environment, and phenotype on the metabolome of maize hybrids using GC/MS and LC/MS. J. Agric. Food Chem. 65, 5215–5225. doi: 10.1021/acs.jafc.7b00456
Tang, Y., Liu, R., and Li, H. (2015). Current progress on truffle submerged fermentation: a promising alternative to its fruiting bodies. Appl. Microbiol. Biotechnol. 99, 2041–2053. doi: 10.1007/s00253-015-6379-6
Vahdatzadeh, M., Deveau, A., and Splivallo, R. (2015). The role of the microbiome of truffles in aroma formation: a meta-analysis approach. Appl. Environ. Microbiol. 81, 6946–6952. doi: 10.1128/AEM.01098-15
Velez, H., Glassbrook, N. J., and Daub, M. E. (2007). Mannitol metabolism in the phytopathogenic fungus Alternaria alternata. Fungal Genet. Biol. 44, 258–268. doi: 10.1016/j.fgb.2006.09.008
Villares, A., García-Lafuente, A., Guillamón, E., and Ramos, A. (2012). Identification and quantification of ergosterol and phenolic compounds occurring in Tuber spp. truffles. J. Food Compost. Anal. 26, 177–182. doi: 10.1016/j.jfca.2011.12.003
Wang, S., and Marcone, M. (2011). The biochemistry and biological properties of the world’s most expensive underground edible mushroom: truffles. Food Res. Int. 44, 2567–2581. doi: 10.1016/j.foodres.2011.06.008
Yang, M., Zou, J., Liu, C., Xiao, Y., Zhang, X., Yan, L., et al. (2019). Chinese white truffles shape the ectomycorrhizal microbial communities of Corylus avellana. Ann. Microbiol. 69, 553–565. doi: 10.1007/s13213-019-1445-4
Ye, L., Li, Q., Fu, Y., Sourzat, P., Tan, H., Zou, J., et al. (2018). Host species effects on bacterial communities associated with the fruiting bodies of Tuber species from the Sichuan Province in Southwest China. Mycol. Prog. 17, 833–840. doi: 10.1007/s11557-018-1397-2
Yu, C., Luo, X., Zhan, X., Hao, J., Zhang, L., Song, Y. B. L., et al. (2018). Comparative metabolomics reveals the metabolic variations between two endangered Taxus species (T. fuana and T. yunnanensis) in the Himalayas. BMC Plant Biol. 18:197. doi: 10.1186/s12870-018-1412-4
Zambonelli, A. (2012). Chinese Tuber aestivum sensu lato in Europe. Open Mycol. J. 6, 22–26. doi: 10.2174/1874437001206010022
Zhang, N., Chen, H., Sun, B., Mao, X., Zhang, Y., and Zhou, Y. (2016). Comparative analysis of volatile composition in chinese truffles via GC x GC/HR-TOF/MS and electronic nose. Int. J. Mol. Sci. 17:412. doi: 10.3390/ijms17040412
Zhang, X., Ye, L., Kang, Z., Zou, J., Zhang, X., and Li, X. (2019). Mycorrhization of Quercus acutissima with Chinese black truffle significantly altered the host physiology and root-associated microbiomes. PeerJ. 7:e6421. doi: 10.7717/peerj.6421
Zhao, L., Zhang, H., Wang, J., Tian, L., Li, F., Liu, S., et al. (2019). C60 fullerols enhance copper toxicity and alter the leaf metabolite and protein profile in Cucumber. Environ. Sci. Technol. 53, 2171–2180. doi: 10.1021/acs.est.8b06758
Keywords: truffles, metabolomics, LC-MS, bioactivity, edible fungus
Citation: Li X, Zhang X, Ye L, Kang Z, Jia D, Yang L and Zhang B (2019) LC-MS-Based Metabolomic Approach Revealed the Significantly Different Metabolic Profiles of Five Commercial Truffle Species. Front. Microbiol. 10:2227. doi: 10.3389/fmicb.2019.02227
Received: 17 May 2019; Accepted: 11 September 2019;
Published: 25 September 2019.
Edited by:
Haifeng Zhao, South China University of Technology, ChinaReviewed by:
Antonietta Mello, Institute for Sustainable Plant Protection, Italian National Research Council (IPSP-CNR), ItalyHerminia De La Varga, Fertinagro Biotech SL, Spain
Gian Maria Niccolò Benucci, Michigan State University, United States
Copyright © 2019 Li, Zhang, Ye, Kang, Jia, Yang and Zhang. This is an open-access article distributed under the terms of the Creative Commons Attribution License (CC BY). The use, distribution or reproduction in other forums is permitted, provided the original author(s) and the copyright owner(s) are credited and that the original publication in this journal is cited, in accordance with accepted academic practice. No use, distribution or reproduction is permitted which does not comply with these terms.
*Correspondence: Xiaoping Zhang, eGlhb3Bpbmd6aGFuZzk3QDE2My5jb20=; Bo Zhang, NjkwNDkyOTY3QHFxLmNvbQ==
†These authors have contributed equally to this work