- 1Biosciences Division, Oak Ridge National Laboratory, Oak Ridge, TN, United States
- 2BioTechnology Institute, University of Minnesota, St. Paul, MN, United States
- 3Department of Earth Sciences, Minneapolis, MN, United States
- 4Smithsonian Environmental Research Center, Edgewater, MD, United States
The gene pair hgcAB is essential for microbial mercury methylation. Our understanding of its abundance and diversity in nature is rapidly evolving. In this study we developed a new broad-range primer set for hgcAB, plus an expanded hgcAB reference library, and used these to characterize Hg-methylating communities from diverse environments. We applied this new Hg-methylator database to assign taxonomy to hgcA sequences from clone, amplicon, and metagenomic datasets. We evaluated potential biases introduced in primer design, sequence length, and classification, and suggest best practices for studying Hg-methylator diversity. Our study confirms the emerging picture of an expanded diversity of HgcAB-encoding microbes in many types of ecosystems, with abundant putative mercury methylators Nitrospirae and Chloroflexi in several new environments including salt marsh and peat soils. Other common microbes encoding HgcAB included Phycisphaerae, Aminicenantes, Spirochaetes, and Elusimicrobia. Combined with high-throughput amplicon specific sequencing, the new primer set also indentified novel hgcAB sequences similar to Lentisphaerae, Bacteroidetes, Atribacteria, and candidate phyla WOR-3 and KSB1 bacteria. Gene abundance data also corroborate the important role of two “classic” groups of methylators (Deltaproteobacteria and Methanomicrobia) in many environments, but generally show a scarcity of hgcAB+ Firmicutes. The new primer set was developed to specifically target hgcAB sequences found in nature, reducing degeneracy and providing increased sensitivity while maintaining broad diversity capture. We evaluated mock communities to confirm primer improvements, including culture spikes to environmental samples with variable DNA extraction and PCR amplification efficiencies. For select sites, this new workflow was combined with direct high-throughput hgcAB sequencing. The hgcAB diversity generated by direct amplicon sequencing confirmed the potential for novel Hg-methylators previously identified using metagenomic screens. A new phylogenetic analysis using sequences from freshwater, saline, and terrestrial environments showed Deltaproteobacteria HgcA sequences generally clustered among themselves, while metagenome-resolved HgcA sequences in other phyla tended to cluster by environment, suggesting horizontal gene transfer into many clades. HgcA from marine metagenomes often formed distinct subtrees from those sequenced from freshwater ecosystems. Overall the majority of HgcA sequences branch from a cluster of HgcAB fused proteins related to Thermococci, Atribacteria (candidate division OP9), Aminicenantes (OP8), and Chloroflexi. The improved primer set and library, combined with direct amplicon sequencing, provide a significantly improved assessment of the abundance and diversity of hgcAB+ microbes in nature.
Introduction
Environmental microbial methylation of inorganic mercury (Hg) produces the bio-accumulative neurotoxin methylmercury (MeHg) (Hsu-Kim et al., 2013; Chen et al., 2018). This process and the subsequent accumulation of MeHg in food webs increase Hg pollution risks to humans (Debes et al., 2016) and ecosystems (Eagles-Smith et al., 2018). In order to reduce this risk, we seek to better understand which organisms contribute to MeHg production and how we might model and perhaps reduce their activity. Although some of the biogeochemical controls on the activity of Hg methylators are well known and may be managed to reduce risk (Hsu-Kim et al., 2018), the organisms that drive MeHg production in several critical environments remain poorly understood. These include the oceans, source of most MeHg risk to humans globally (UNEP, 2019), and many Hg-contaminated environments (Brooks and Southworth, 2011; Eckley et al., 2020). Additionally, numerical models for net MeHg production are generally poor without cite-specific empirical parameterization. Here we report on an improved approach to identifying Hg-methylators in nature. We hope that this information will advance biogeochemical models of MeHg production and perhaps provide routes for mitigation.
We still know relatively little about the distribution of Hg-methylating organisms in the environment, although the discovery of the hgcAB gene pair conferring Hg-methylation capability has greatly improved their identification (Parks et al., 2013; Bravo and Cosio, 2019). Mercury methylation is a species (and possibly strain)-specific trait that is not well predicted by 16S rRNA taxonomic classification (Ranchou-Peyruse et al., 2009; Gilmour et al., 2013; Yu et al., 2013; Gilmour et al., 2018; Christensen et al., 2019; McDaniel et al., 2020; Peterson et al., 2020). The presence or abundance of groups of microbes that include Hg methylators is not predictive of the abundance of hgcAB+ microbes. Thus, identification of hgcAB sequences is required to evaluate the distribution of Hg-methylators in nature. The known diversity of Hg methylators is expanding as increasing numbers of hgcAB sequences are identified from shotgun metagenomics, metagenome-assembled genomes (MAGS), and hgcAB amplicon-specific sequencing (Jones et al., 2019; Xu et al., 2019; Azaroff et al., 2020; Lin et al., 2020; McDaniel et al., 2020; Peterson et al., 2020).
In addition to the Hg-methylators confirmed in culture, sulfate- and iron-reducing Deltaproteobacteria, acetogenic and syntrophic Firmicutes, and methanogenic Archaea (Compeau and Bartha, 1985; Fleming et al., 2006; Kerin et al., 2006; Ranchou-Peyruse et al., 2009; Gilmour et al., 2013; Yu et al., 2013; Gilmour et al., 2018; Goñi-Urriza et al., 2019)- genomic analyses have identified hgcAB (and thus inferred the ability to produce MeHg) in many other lineages including Aminicenantes (Candidate Phylum OP8), Atribacteria (Candidate Phylum OP9), Bacteroidetes, Chloroflexi, Chrysiogenetes, Elusimicrobia, Marinimicrobia, Nitrospira, Nitrospina, the PVC group, Spirochaetes, and Thermotogae (Podar et al., 2015; Gionfriddo et al., 2016; Liu X. et al., 2018; Bowman et al., 2019; Christensen et al., 2019; Jones et al., 2019; Lin et al., 2020; McDaniel et al., 2020; Peterson et al., 2020). The occurrence of hgcAB in Bacterial and Archaeal genomes is rare but widely dispersed across phyla (Parks et al., 2013; Podar et al., 2015; Jones et al., 2019). Hg-methylating microorganisms often constitute less than 5% of the bulk microbial community (Christensen et al., 2019), yet in these same environments MeHg can comprise up to 10% of the total Hg within a system (Christensen et al., 2019).
PCR primers and reference libraries for hgcAB have improved with the increasing number of hgcAB sequences identified. Early studies relied on sequences from a small number of cultured methylators (Bae et al., 2014; Liu et al., 2014; Schaefer et al., 2014) while more recent work has captured a wider diversity (Christensen et al., 2016, 2019; Bravo and Cosio, 2019; Jones et al., 2019). Improved techniques for capturing hgcAB presence and diversity are necessary for identifying both the major players in environmental MeHg production as well as the evolutionary history of the gene pair.
Here we report an improved PCR primer set, reference library and amplification protocol for hgcAB detection from environments significant to Hg biogeochemical cycling. The new primer set is a modification of the Christensen broad-range hgcAB primer set, which was designed using a database of 84 known and predicted Hg-methylator genomes (Christensen et al., 2016). To improve amplification across clades, we modified the reverse primer to explicitly target only hgcAB sequences found in nature. PCR primer degeneracy is an important tool for capturing genetic diversity while minimizing biases (von Wintzingerode et al., 1997; Sipos et al., 2007), but high levels of degeneracy can reduce amplification of individual primer matches. This change significantly reduced primer degeneracy while maintaining the ability to capture broad hgcAB sequence diversity. Use of this primer set limits non-specific amplification, resulting in increased primer efficiency. To improve detection from complex environments, we also optimized sample volumes and concentrations to mitigate PCR inhibition, using several sample matrices. The updated primer and PCR method were validated using mock communities and culture spikes into sediments and soils. Additionally, an expanded hgcAB reference library was developed based on a larger number of sequences from confirmed hgcAB+ cultures plus sequences from publicly available MAGS for a total of 283 sequences.
Finally, the new primer, the protocol, and the reference library were used to evaluate the community structure of HgcAB-encoding microbes from several novel environments, including salt marsh soils, freshwater sediments and peat soils. Hg methylation genes were evaluated using both clone libraries and high-throughput amplicon-specific sequencing. The use of high-throughput amplicon sequencing eliminates the need to create clone libraries and very significantly increases the efficacy of identifying hgcAB+ organisms. A new phylogenetic analysis of HgcA was constructed using the deep sequencing data from fresh and estuarine sediments of two of the study sites.
Materials and Methods
Expanded Reference Database of hgcAB Sequences
An expanded library was constructed based on confirmed Hg-methylators plus sequences from single cell genomes and MAGs from diverse environments (Supplementary Table S1). The new hgcA library contains 283 hgcA sequences, including 124 Deltaproteobacteria, 33 Firmicutes, 15 Methanomicrobia, and 111 sequences from other clades (Gionfriddo et al., 2019). Of those sequences, 239 had an available hgcB sequence. This custom database of known and predicted Hg-methylators with NCBI taxonomy was compiled on the 16th of October 2018 using Taxtastic (v0.8.51) (Sayers et al., 2009). The compiled Hg-methylator reference database is maintained by the ORNL Mercury SFA and is publicly available through the DOE Data Explorer (Gionfriddo et al., 2019). This database expands the hgcAB sequence library substantially over most libraries used in hgcAB classification to date (Podar et al., 2015; Bravo et al., 2018b; Liu Y. et al., 2018).
The ORNL compiled Hg-methylator database can be used to assign taxonomic classification to HgcA sequences from clone, amplicon, or meta-omic datasets (Gionfriddo et al., 2019). The database contains reference hgcA and hgcB sequences, and two reference packages for identifying and classifying longer (654 nt bp) or shorter (201 nt bp) hgcA sequences. Each reference package contains an HgcA hmm-model and HgcA phylogenetic tree. To construct the hmm-models, nucleotide hgcA sequences were aligned (MUSCLE), trimmed (201 bp or 654 bp), translated into amino acids using Geneious version 10.2, and compiled into an hmm-model using ‘hmmbuild’ (Edgar, 2004b; Johnson et al., 2010; Kearse et al., 2012). A maximum likelihood (ML) HgcA distribution was estimated using the GAMMA model of rate heterogeneity and ML estimate of alpha-parameter in RAxML with 150 bootstrap replicates. The tree was rooted by carbon monoxide dehydrogenases from non-Hg-methylators Candidatus Omnitrophica bacterium CG1-02-41-171 and Thermosulfurimonas dismutans (Stamatakis, 2014). These paralogous sequences with distinct taxonomy from Hg-methylators were included in the database to identify non-HgcA encoding sequences that may have passed previous filtering.
Primer Design
Our goal in creating a revised broad-diversity primer set for hgcAB was to increase the specificity and efficiency of the previously published primer set (Christensen et al., 2016) while retaining the ability to capture the full diversity of the community. The Christensen 2016 primer set (ORNL-HgcAB-uni-F and ORNL-HgcAB-uni-R) was designed based on the hgcAB sequences from 84 strains that were available at that time, including Deltaproteobacteria, Firmicutes, Archaea, and a limited number of other clades. The primers target the highly conserved cap-helix encoding region of hgcA and the highly conserved ‘C(M/I)ECGA’ ferredoxin-type encoding domain of hgcB (Parks et al., 2013), amplifying a ∼950 nt bp product. To capture the diversity of the reference strains, the Christensen primers (especially the reverse primer) are highly (96-fold) degenerate. When used in combination with a diverse reference library for OTU identification, these primers capture a highly diverse community of hgcAB+ organisms, including many groups outside of the three used to construct the primers (Christensen et al., 2019; Jones et al., 2019).
Our strategy was to reduce the overall degeneracy of the reverse (hgcB) primer by eliminating oligonucleotide sequences from the primer mix that are not found in nature. Lower degeneracy should result in more efficient amplification, and thus recover more hgcAB sequences from the environmental samples. Only the reverse primer sequence, not position, was updated.
The 96 reverse primer sequences that make up ORNL-HgcAB-uni-R (Supplementary Table S2) were screened against the expanded hgcAB reference library using Geneious version 10.22 (Kearse et al., 2012). Only 39 of the possible 96 sequences in ORNL-HgcAB-uni-R degenerate primer matched sequences in the expanded reference library. In the reverse primer-binding region, seven of twenty bases show 100% pairwise identity, twelve are fairly conserved (30–100% sequence identity), and one is highly divergent (<30% pairwise identity) across the available hgcB reference sequences (Figure 1). To choose the final primer, degeneracy was reduced at the 5′ end and increased at the 3′ end to combat primer mismatch and binding efficiencies. Mismatches within five base pairs (nt bp) of the 3′ end can have larger effects on primer efficiency than 5′ located mismatches (Stadhouders et al., 2010; Campos and Quesada, 2017). Therefore, increasing the possible primer sequences at the 3′ end increases the likelihood of exact matches, theoretically improving binding specificity (Stadhouders et al., 2010). Changing, from the 5′ end, the third base B = C/T/G to G, the ninth base from R = G/A to ‘G’, and increasing the 15th base degeneracy from ‘C’ to S = C/G decreased the degeneracy from 96 to 32 and also resulted in eliminating rare hgcB reference sequences (Figure 1). To summarize, the reverse primer sequence was changed from ‘CAB GCN CCR CAY TCC ATR CA’ to ‘CAG GCN CCG CAY TCS ATR CA’ (differences shown in bold). The new primer is designated ORNL-HgcAB-uni-32R, signifying the 32-fold degeneracy.
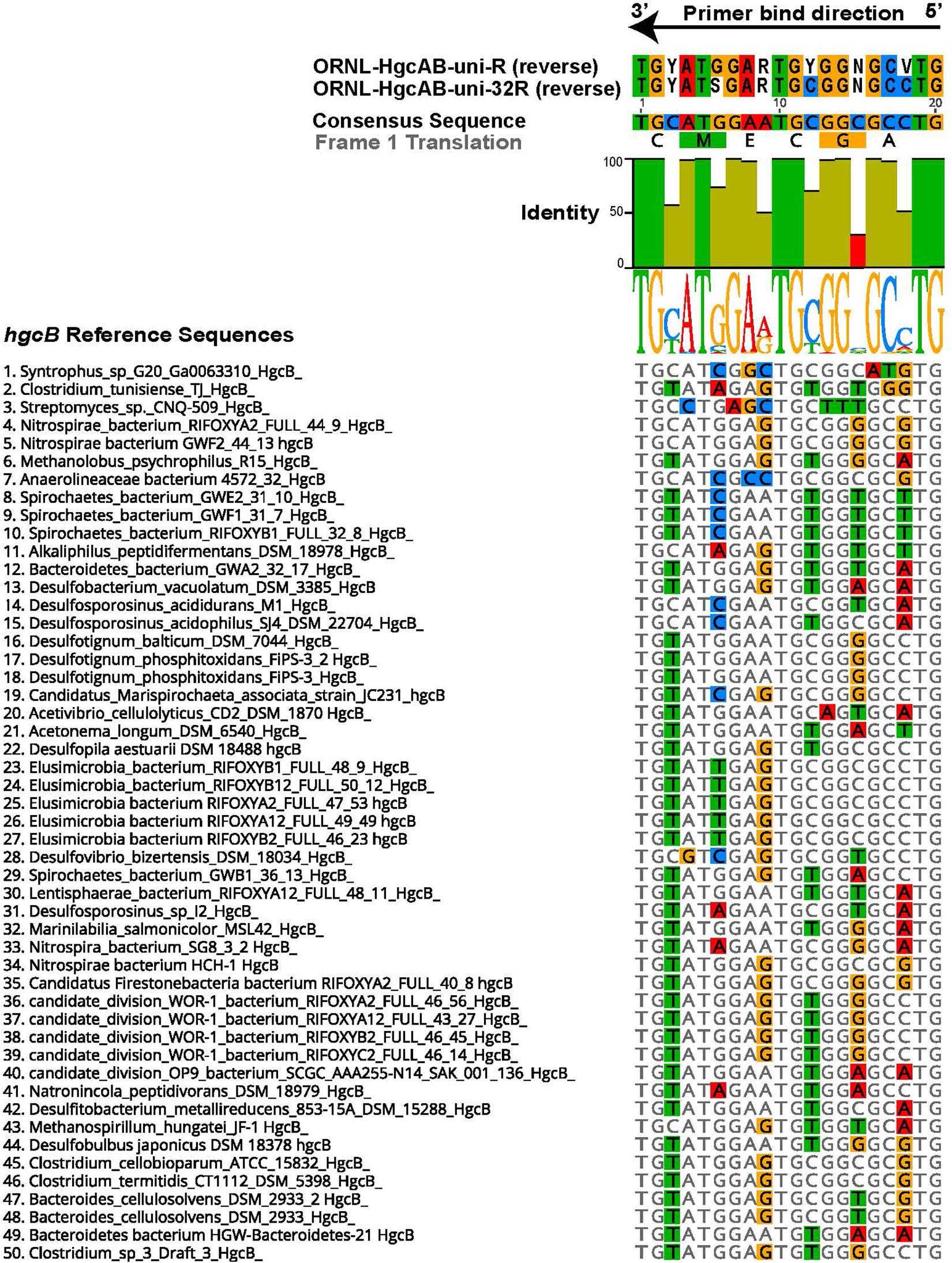
Figure 1. Alignment showing the highly conserved 20 nt bp region of hgcB where the reverse primer binds. Alignment is based on 239 reference hgcB sequences, however only the top 50 sequences most divergent from the consensus sequence are shown. Bases that disagree with consensus sequence are highlighted. Reverse complements of each primer (ORNL-HgcAB-uni-R, ORNL-HgcAB-uni-32R) are included for reference. The bar graph at the top of the image shows mean pairwise identity for each base across all sequences – green is 100%, green-brown is between 30 and 100%, and red is < 30% conserved in 239 sequences. Y = C/T, R = G/A, V = C/G/A, S = C/G, N = A/T/C/G.
In silico primer match efficiency and specificity was examined using the Geneious version 10.2 ‘test primer’ function, allowing zero to two mismatches (see footnote 2) (Kearse et al., 2012). Mismatches within five nucleotides of the 3′ end were not allowed. Efficiency is defined as whether the primer sequence matched HgcB-encoding sequences from known and predicted methylators. Reverse primers were tested for specificity against a reference dataset of publicly available nucleotide sequences encoding 4Fe–4S ferredoxins (i.e., non-HgcB encoding paralogs). The sequences for the ferredoxin dataset were pulled from JGI IMG using a gene search of isolate genomes for 4Fe–4S ferredoxins by Pfam ID (00037) on 12-17-20183. Specificity is defined as whether the primer sequence would theoretically bind to non-HgcB encoding sequences. The reference set contained a total of 14,161 nucleotide sequences from environmental and cultured isolates in the JGI IMG database.
For initial validation, ORNL-HgcAB-uni-32R was tested on genomic DNA (gDNA) from 31 pure culture strains (26 hgcAB + and 5 hgcAB- controls) (Christensen et al., 2016). Premixed and individual oligonucleotides were ordered from Integrated DNA Technologies and the latter were mixed in-house at equimolar concentrations. The annealing temperatures for the sequences in the 96-fold degenerate primer (ORNL-HgcAB-uni-R) ranged from 56.1–68.2°C, compared to 60.2–68.5°C for ORNL-HgcAB-uni-32R (Supplementary Table S2). It is used with the published forward primer (ORNL-HgcAB-uni-F, 5′ AAYGTCTGGTGYGCNGCVGG). Annealing temperatures for the forward primer ranged from 60.9 to 70.7°C.
Sample Sites and DNA Extraction
Environmental samples were obtained from Oak Ridge National Laboratory (ORNL) collaborative sites including peatland sediments from Spruce and Peatland Responses Under Changing Environments (SPRUCE) study plots in the USDA Forest Service Marcell Experimental Forest (MEF) (Grand Rapids, MN, United States), freshwater sediments from Sandy Creek (Durham, NC, United States), mesohaline tidal salt marsh sediments from Rhode River (Edgewater, MD, United States), periphyton and sediment from East Fork Poplar Creek (EFPC) (Oak Ridge, TN, United States), and rice paddy soil from Yanwuping (Guizhou Province, China). Sample collection information, including location and date of collection are summarized in Supplementary Table S3. SPRUCE sediments were collected from treed hummock (2012-10-T-Hum; 2012-6-T-Hum) and hollow (2012-10-T-Hol, 2012-6-T-Hol) sites of the S1-Bog from surface to 200–300 cm (Iversen et al., 2012). Background soil total Hg (HgT) concentrations in the SPRUCE site ranged from roughly 20–200 ng/g, and MeHg concentrations from roughly 0.5–10 ng/g, depending on depth (Pierce et al., 2017). The EFPC sediment and periphyton samples were collected from the New Horizon (NH) study site (35.9662°N, 84.35817°W). Total Hg (HgT) concentrations ranged ∼20–50 mg kg–1 (Southworth et al., 2010). EFPC water ranged from 110–211 ng Hg L–1 (unfiltered) and 15–89 ng L–1 (filterable), respectively (Olsen et al., 2016). Total and filterable methylmercury (MeHg) ranged from 0.15–0.43 ng L–1 and 0.10–0.29 ng L–1, respectively (Olsen et al., 2016). Mesohaline tidal salt marsh sediments were collected from a Phragmites-dominated area of the Global Change Research Wetland on the Rhode River (Edgewater, MD, United States), where soil Hg concentrations averaged 0.17 ± 0.007 mg kg–1 Hg (Mitchell and Gilmour, 2008). Sandy Creek sediments were collected from a freshwater retention pond (Durham, NC, United States) that receives legacy Hg, with sediments averaging 0.05 ± 0.006 mg kg–1 Hg (Ndu et al., 2018). Rice paddy soils were collected during the rice growing season from Yanwuping in Guizhou province, China. Rice paddy fields were 2–14.5 km downstream from a historical Hg mining site, with HgT levels ranging from 0.83 to 990 mg kg–1 and MeHg from 0.0038 to 0.021 mg kg–1 (Vishnivetskaya et al., 2018).
Sediment, soil, and periphyton samples were stored at −80°C prior to DNA extraction. Genomic DNA (gDNA) was isolated from sediments using the MoBio Powersoil kit (now Qiagen DNeasy) with the addition of a 30-min incubation at 65°C following the bead beating step. A standard phenol chloroform extraction was used to isolate gDNA from periphyton biomass. All gDNA was quantified using QubitTM (Thermo Fisher Scientific) and quality was assessed with NanoDropTM One (Thermo Fisher Scientific).
Mock Communities
Pure cultures of known Hg-methylators were spiked into fresh homogenized Rhode River tidal salt marsh soil sample #1064 (∼0.2 g) to form mock community samples. Two mock communities were constructed. For Combo 1, sediment was spiked with 1.00E9 cells Geobacter (G.) sulfurreducens (∼3800 ng DNA), 4.93E8 cells Desulfosporosinus (Ds.) acidophilus (2700 ng DNA), and 1.01E9 cells Methanocorpusculum (Mc.) bavaricum (1900 ng DNA). Combo 2 was spiked with 6.34E8 cells Desulfovibrio (Dv.) desulfuricans ND132 (2700 ng DNA), 1.13E8 cells Desulfitobacterium (Db.) dehalogens (540 ng DNA), and 4.10E8 cells Methanomassiliicoccus (Mm.) luminyensis (1200 ng DNA). DNA was recovered using a modified MoBio Powersoil kit. To estimate DNA recovery, extraction efficiencies were evaluated from individual cultures alone and cultures spiked into the marsh soil. Average extraction efficiencies for cultures spiked into sediment were similar to efficiencies from cultures alone, ∼30%, but with significant variability among cultures. For the mock communities, DNA recoveries for spiked cultures were 10 ± 4.8% and 37 ± 6% respectively from Combo 1 and 2.
PCR Amplification of 16S rRNA and hgcAB
For environmental samples, both 16S rRNA and hgcAB genes were amplified. For 16S rRNA amplification, the 25-μL reaction mixture contained 0.2 μM of 8F (5′ AGA GTT TGA TCC TGG CTC AG) and 1492R (5′ GGT TAC CTT GTT ACG ACT T) (Turner et al., 1999), Apex TaqRed polymerase (Genesee Scientific, San Diego, CA, United States), and a dilution series of gDNA template (10 pg–10 ng) to determine the optimal gDNA concentration and ensure amplification. Amplification was initiated with pre-incubation (2 min at 98°C), 30 cycles of denaturation (15 s at 98°C), annealing (30 s at 54°C), and extension (60 s at 72°C), then a final incubation (3 min at 72°C).
For hgcAB amplification from both pure culture and environmental samples, the use of a polymerase without a 3′ to 5′ exonuclease proofreading activity is important. In our experience, a proofreading polymerase that uses 3′ to 5′ exonuclease, such as a Pfu polymerase, leads to multiple visible gel bands of non-specific PCR product. For hgcAB amplification, 20 and 50-μL reaction mixtures used 0.5–1 μM of ORNL-HgcAB-uni-F and ORNL-HgcAB-uni-32R, Apex TaqRed polymerase (Genesee Scientific, San Diego, CA, United States), and 100 pg to 10 ng of gDNA. A touch-down PCR protocol was used with pre-incubation (2 min at 98°C), then 5 cycles of denaturation (30 s at 98°C), annealing (30 s at 68°C; −1°C/cycle), and extension (30 s at 72°C), then 30 cycles of denaturation (30 s at 98°C), annealing (30 s at 63°C), and extension (60 s at 72°C), then a final incubation step (2 min at 72°C).
Clone Libraries
Clone libraries of hgcAB were constructed from all of the environmental samples listed in Supplementary Table S3. PCR conditions were 0.5 ng/μL (final concentration) gDNA template and 0.5 μM primer in 20 μL reaction volume. Clone libraries were created from both the ORNL-HgcAB-uni-F/ORNL-HgcAB-uni-R and the ORNL-HgcAB-uni-F/ORNL-HgcAB-uni-32R primer pairs for comparison.
The number of clones created for each sampling area ranged from 12 to >100 (Supplementary Table S3). The environmental clone libraries contained 509 hgcAB sequences amplified with the ORNL-HgcAB-uni-F/ORNL-HgcAB-uni-32R primers and 119 with ORNL-HgcAB-uni-F/ORNL-HgcAB-uni-R primers. To create the libraries, hgcAB PCR products (∼950 bp) from each sample were excised from an agarose gel, cleaned with Wizard SV Gel and PCR Clean-Up system (Promega, Madison, WI, United States) and cloned into One Shot TOP10 cells with TOPO-TA cloning kit (Invitrogen, Waltham, MA, United States). Cloned plasmids were screened for the ∼1150 bp insert using 100 pM of M13F (5′ GTAAAACGACGGCCAG) and M13R (5′ CAGGAAACAGCTATGAC) primers with Apex TaqRed polymerase. The insert size was confirmed (on 1% agarose gel), cleaned (Wizard SV Gel and PCR Clean-Up) and sent for Sanger sequencing by Eurofins Genomics (Louisville, KY, United States).
For classification of the clone sequences, the following pipeline was used. All hgcAB sequences from the clones were confirmed by blastx against the NCBI non-redundant database (Altschul et al., 1990) and non-specific products were removed from further analysis. The resulting sequences were aligned, trimmed, and primers annotated using Geneious version 10.2 (see footnote 2) (Kearse et al., 2012). The trimmed hgcAB sequences were aligned using MUSCLE (Edgar, 2004a) to a reference database of 296 hgcA and 200 hgcB sequences from known and predicted Hg-methylators (Supplementary Table S1). While both genes were present in cloned sequences, only the hgcA portion of the sequence was used for downstream analyses. This is due to both the discrepancy in the number of available reference sequences between hgcA and hgcB, as well the difficulty in aligning sequences across the portion of DNA between the protein-coding regions of hgcA and hgcB.
We performed taxonomic classification of clone sequences using our custom in-house short-read HgcA reference package (‘ORNL_HgcA_201.refpkg’) and longer-read HgcA reference package (‘ORNL_HgcA_654_full.refpkg’) (Gionfriddo et al., 2019). In order to compare to the shorter amplicon sequences, clone hgcA sequences were trimmed to 201 nt bp and classified following the same protocol as amplicon sequences. In short, clone hgcA sequences were clustered using ‘cd-hit-est’ on a 90% sequence identity cut-off over a 201 nt bp region of hgcA. Taxonomic classifications were assigned to OTUs based on phylogenetic placement of translated HgcA sequences on HgcA reference tree (in ‘ORNL_HgcA_201.refpkg’) using ‘pplacer’ and ‘guppy classify’ with posterior probability classification cut-off of 90% (Matsen et al., 2010). To compare how our classification pipeline performed on short (201 nt bp) versus longer (654 nt bp) hgcA sequences, we classified the same clone sequences, now trimmed to 654 nt bp, using ‘ORNL_HgcA_654_full.refpkg.’ The longer-read reference package contains reference HgcA sequences encoded by 654 nt bp, corresponding to the 268–955 nt bp region of D. desulfuricans ND132 hgcA (Genbank CP003220.1, 1150554:1151240). The environmental clone hgcA sequences from this study are publicly available under the NCBI GenBank accession numbers MT122211 – MT122744.
High-Throughput Sequencing of hgcAB Amplicons
For environmental samples from the SERC salt marsh and the NH site in EFPC, and for select mock communities (Supplementary Table S4), hgcAB sequence abundance and diversity were also evaluated by high-throughput amplicon-specific sequencing, using Illumina MiSeq 2 × 300 bp chemistry at the University of Minnesota Genomics Facility (UMGC). A two-step PCR amplified hgcAB from environmental and mock community gDNA by adapting published protocols (Gohl et al., 2016; Jones et al., 2017). The modified hgcAB-specific primers included 5’ adaptor sequences (adaptor TCG TCG GCA GCG TCA GAT GTG TAT AAG AGA CAG with ORNL-HgcAB-uni-F; adaptor GTC TCG TGG GCT CGG AGA TGT GTA TAA GAG ACA G with ORNL-HgcAB-uni-R and ORNL-HgcAB-uni-32R). Amplicon libraries were created by amplifying hgcAB with the modified primers for 30 or 35 cycles (Supplementary Table S4) following the PCR protocol for hgcAB cloning described above as in Jones et al. (2017). Illumina sequencing adaptors and dual indices were added at UMGC with a second amplification step (10 cycles, after diluting PCR products 1:100) (Gohl et al., 2016). Libraries were pooled and sequenced on an Illumina MiSeq v3 PE300. Read length was not sufficient to produce an overlap between forward and reverse reads, therefore two separate libraries were produced, the forward reads encompassing approximately the first 300 bp of hgcA starting at the cap-helix and reverse reads encompassing a 300 bp portion of hgcB and hgcA. The presence of both genes (hgcA and hgcB) is needed for PCR amplification. The environmental and mock community hgcAB amplicon raw sequence files are publicly available at the NCBI SRA accession (PRJNA608965).
Raw sequences were filtered and trimmed using Trimmomatic, and only forward hgcA reads were used (Bolger et al., 2014). We adapted OTU clustering methods applied to functional genes from Pelikan et al. (2016) and Bravo et al. (2018a). For this study, hgcA sequences were trimmed (201 nt bp), dereplicated, and singletons removed with USEARCH (Edgar, 2010). Chimeras were removed using de novo and reference filtering with VSEARCH (Rognes et al., 2016). For NH hgcAB, 88.2–91.7% of amplicons from the old primer set and 80.8–90.5% of amplicons from the new primer set passed quality and chimeric filtering. The non-chimeric dereplicated sequences were clustered at 90% identity using cd-hit-est (Huang et al., 2010), and trimmed forward reads were mapped to the centroid database using ‘-usearch_global’ to produce OTU counts. An example annotated workflow for the HgcAB sequence processing and taxonomy assignment approaches used in this study can be found at (https://github.com/caitlingio/Gionfriddo-et-al-hgcAB-amplicon-tutorial) along with a sample data set, the reference library, and example sample output.
The sequencing error rate was calculated for both primer-sets using the quality filtered and trimmed combo 1 and combo 2 mock community datasets using the ‘seq.error’ function in mothur (Schloss et al., 2009). The sequencing error for combo 1 mock community amplified with the new primer set was 1.94%, while with the old published primer set was 2.20%. The sequencing error for combo 2 mock community amplified with the new primer set was 2.05%, while with the old published primer set was 2.27%.
Taxonomic Classification of Amplicon HgcA Sequences
The Taxtastic reference package ‘ORNL_HgcA_201.refpkg’ was used to assign taxonomy to the short (201 nt bp) centroid sequences. The nucleotide sequences are first translated and then aligned to reference sequences using ‘transeq’ and ‘hmmalign,’ respectively (Johnson et al., 2010; Li et al., 2015). Only forward sequences where the first base was the start codon were kept after translation. Prior to alignment, sequences with stop codons were removed, and reads with < 1e-7 inclusion value with the HgcA cap-helix region were filtered using ‘hmmsearch’ (Johnson et al., 2010). Pplacer placed the filtered, translated, and aligned centroid sequences on the ML reference tree. Taxonomic classifications were assigned to each centroid sequence based on the lowest common ancestor (LCA) of the subtree where the sequence was placed using ‘guppy classify’ with a classification cutoff of 90% (Matsen et al., 2010). To avoid misclassifications of distantly related sequences, only placements with a branch length < 1 were classified. OTU assignments, phylogenetic placements of centroid sequences, and taxonomic classifications were analyzed in R using ‘BoSSA’ (v3.44) and ‘phyloseq’ (McMurdie and Holmes, 2013).
In the NH hgcA dataset, 86.0–96.1% of filtered non-chimeric amplicons from the old primers and 69.0–92.7% of amplicons from the new primers could be classified. The top ten OTUs from both primer-sets that did not pass filtering criteria contained sequences with high similarity to hgcA and hgcB outside the 201 nt bp hgcA region as well as non-hgcAB genes that encode 4Fe-4S ferredoxins with reverse primer-binding sites when allowing up to 3 mismatches (NADH:ubiquinone oxidoreductase, xanthine dehydrogenase) and genes that encode proteins that do not appear to contain primer-binding sites even when allowing for mismatches (ATPases, diguanylate cyclase, threonine/serine dehydratase, O-methyltransferase, proteins with unknown function).
For comparison, the 50 most abundant OTUs from NH amplicon libraries were classified based on LCA (cut-off 50%) taxonomy from protein BLAST against NCBI non-redundant protein database using MEGANv6 (Altschul et al., 1990; Huson et al., 2016) (Supplementary Figure S1). The closest BLAST matches were often ‘unclassified’ sequences (Supplementary Figure S1). Therefore, only hgcAB sequences from genomic data with accompanying phylogeny were included in the reference database. As a direct comparison, publicly available rice paddy metagenomic reads were downloaded from the NCBI sequence archive (Accession: PRJNA450451) (Liu Y. et al., 2018). The hgcA genes were identified in sequence reads and aligned to reference sequences using the hmm model from the ‘ORNL_HgcA_201.refpkg’ using ‘hmmsearch’ and ‘hmmalign’ (inclusion value 1E-7). Taxonomic classifications were assigned to hgcA reads using pplacer with ‘ORNL_HgcA_201.refpkg’ with the same parameters as amplicon sequences.
Results
Evaluating the Need for Degeneracy in Broad-Range hgcAB Primers
To evaluate whether the degeneracy of the new primer set was high enough to capture the natural diversity of hgcAB, we queried hgcAB libraries constructed from several environmental matrices (Supplementary Table S3), using Geneious version 10.2 (see footnote 2) (Kearse et al., 2012). The hgcAB products were searched for primer-binding locations to resolve which primer sequences were likely binding and amplifying hgcAB genes from environmental samples. Libraries were constructed using both the original (ORNL-HgcAB-uni-F, ORNL-HgcAB-uni-R) primer set and the new less degenerate primer set (ORNL-HgcAB-uni-F, ORNL-HgcAB-uni-32R) for comparison. We evaluated our clone libraries constructed from a range of environments including boreal peats, freshwater stream sediments, freshwater periphyton mats, and salt marsh and rice paddy soils (Supplementary Table S3). We also evaluated high-throughput hgcAB amplicon libraries for the New Horizon site in EFPC, chosen due to the expected high diversity in Hg-methylators at this site (Supplementary Table S5).
Forward primer binding sites were found in 84% of the clones and 95% of the amplicons searched. Each of the 48 possible primer oligonucleotide sequences were found in the clone and amplicon hgcAB sequence libraries (Supplementary Figure S2). The fraction of hgcAB clones and amplicons that did not amplify with the primers may reflect amplification of ferrodoxins similar to hgcB, or amplification of sequences not captured in the current hgcAB reference library. In some cases, forward primer binding sites may have been trimmed off during quality filtering. The relatively even distribution of percent occurrence among the forward oligonucleotide sequences in environmental sequences indicates that the degeneracy (n = 48) of the forward primer is necessary for capturing hgcAB diversity. The hgcAB clone and amplicon libraries sequenced were searched for reverse oligonucleotide sequences from both ORNL-HgcAB-uni-R and ORNL-HgcAB-uni-32R primers (Figure 2). In amplicon sequence libraries amplified with ORNL-HgcAB-uni-F and ORNL-HgcAB-uni-R, 39.2% of oligonucleotide sequences found in amplicon sequences were shared between primer sets. In amplicon libraries amplified with ORNL-HgcAB-uni-F and ORNL-HgcAB-uni-32R, the percentage of shared oligonucleotide sequences found in amplicon sequences increased to 89.9%. When allowing up to two mismatches, both reverse primers theoretically bind to all available clone and amplicon sequences with reverse primer binding sites from this study. This indicates that theoretical binding efficiencies of both reverse primers to hgcAB genes in environments tested are highly similar. This is supported by the high similarity, based on weighted UniFrac distances, between taxonomic profiles of hgcAB genes amplified from the same sample but with different reverse primers (Figure 3).
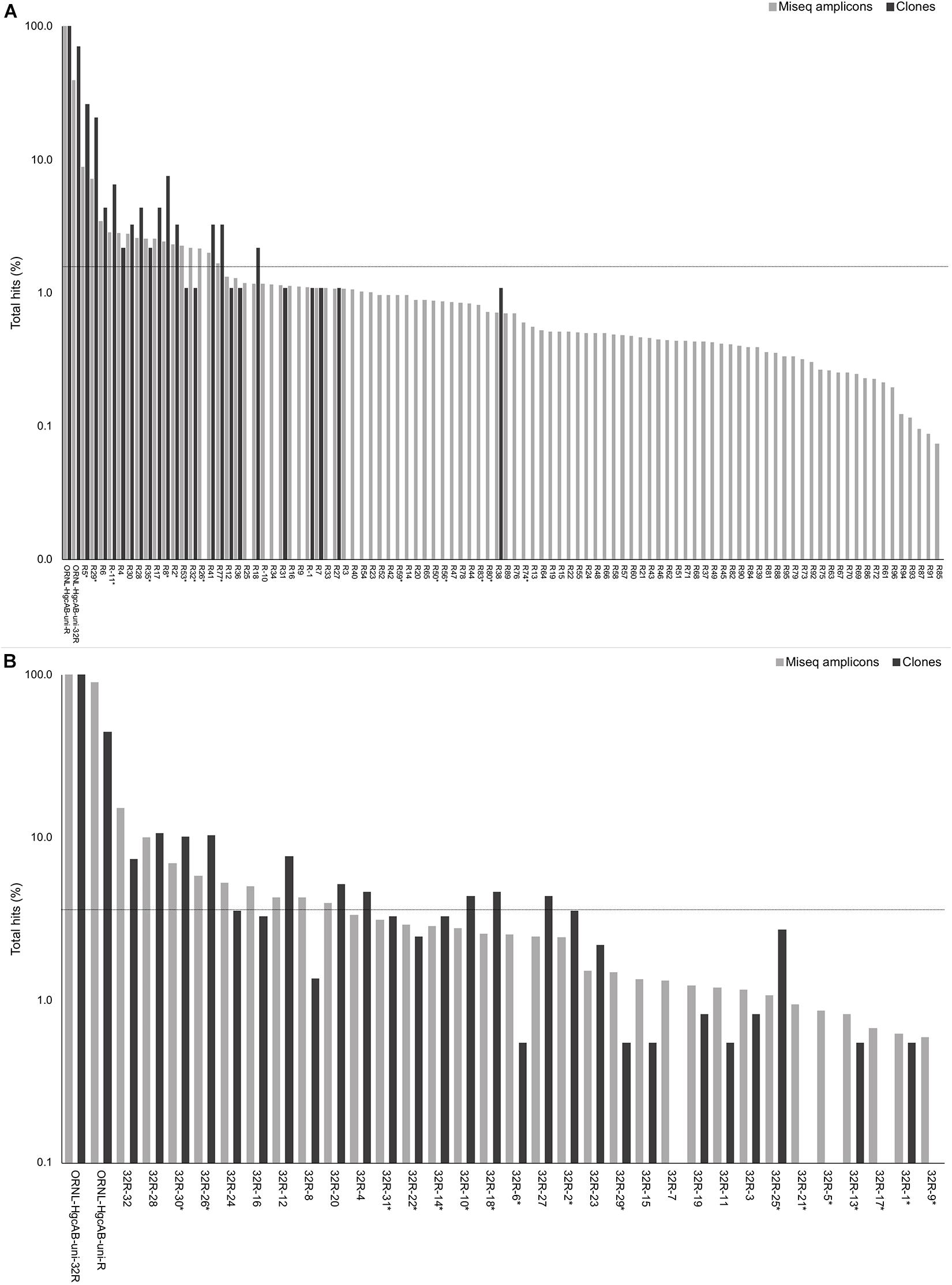
Figure 2. Percent occurrence of each 96-degenerate reverse (top; ORNL-HgcAB-uni-R) and 32-degenerate reverse primer (bottom; ORNL-HgcAB-uni-32R) oligonucleotide sequence in the environmental clone hgcAB sequences and Miseq amplicons. Primers are listed in Supplementary Table S2. Matches to each broad-range reverse primer (ORNL-HgcAB-uni-R, ORNL-HgcAB-uni-32R) are shown for each set of clone and amplicon sequences amplified with the ORNL-HgcAB-uni-R (A) and ORNL-HgcAB-uni-32R (B). Oligonucleotide sequences shared between each reverse primer are indicated with asterisk (∗). Reverse primer sequences were found in 95 clones (A) and 369 clones (B), and 210, 362 (A) and 233, 934 (B) amplicon reads. Data is shown on a log scale. Dashed line indicates equal distribution of reverse primer sequences across amplicon sequences: (A) 1.04%, (B) 3.13%. Ordered by decreasing abundance in Miseq amplicon libraries.
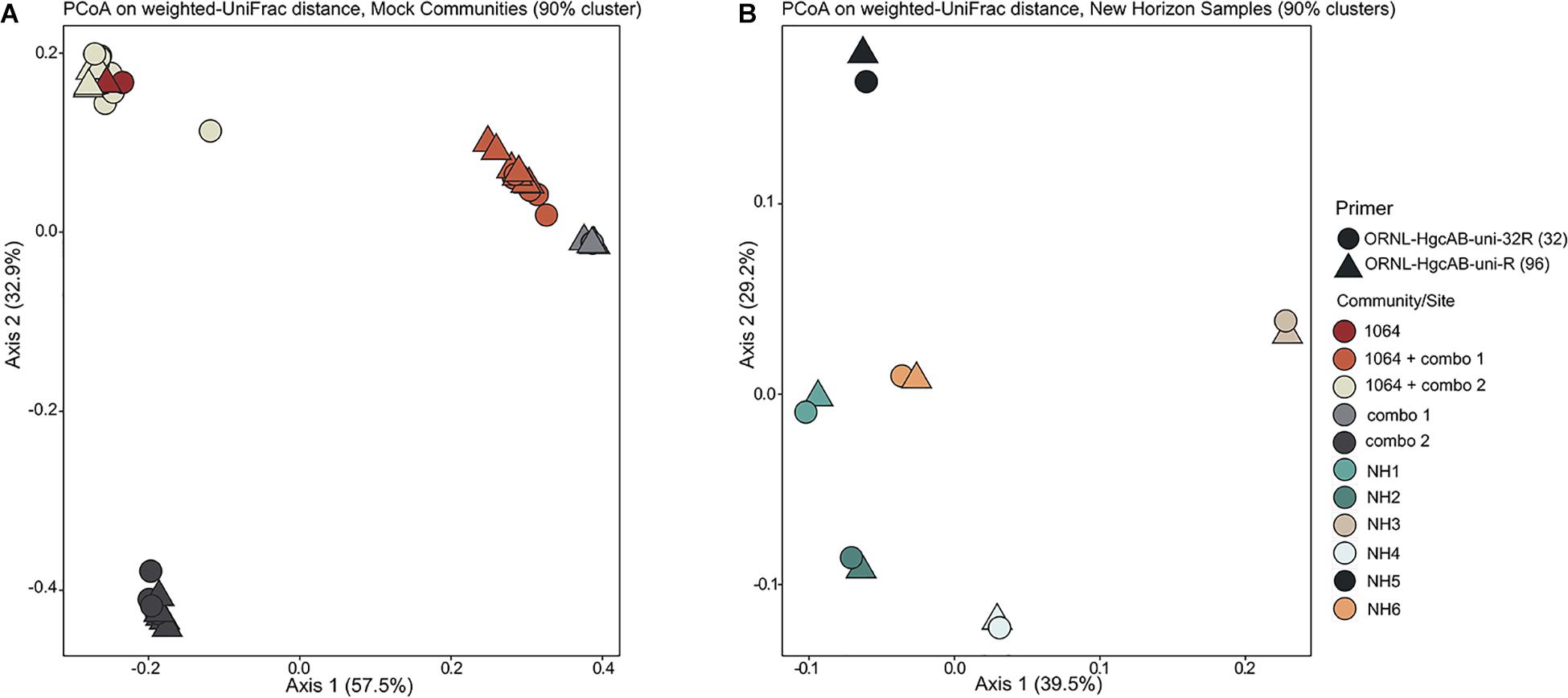
Figure 3. Principle coordinate analyses (PCoA) showing the variance in phylogenetic distances between mock community (A) and New Horizon (NH) sediment (B) hgcAB amplicon libraries. Reverse primer (i.e., ORNL-HgcAB-uni-R, ORNL-HgcAB-uni-32R) is indicated by shape. Weighted UniFrac distances calculated from the taxonomic classifications of clustered (90%) OTUs using the program ‘pplacer’ and the R package ‘phyloseq.’
One of the goals for reducing the degeneracy of the reverse primer from 96 to 32 was the removal of redundant oligonucleotide sequences, i.e., sequences that do not actually bind and amplify template DNA. Removal of the redundant sequences from the primer mix should increase amplification efficiencies by increasing the ratio of each of the remaining oligonucleotide sequences to template DNA (Lee et al., 2012). Decreasing degeneracy also reduced the annealing temperature range of the primer-set (Supplementary Table S2), which can also have an effect on PCR amplification bias (Lee et al., 2012). There are 50 sequences from ORNL-HgcAB-uni-R primer that do not appear in the reference hgcB database (i.e., 52.1% redundant) (Supplementary Table S2). When searching the 210,362 amplicon sequences amplified by ORNL-HgcAB-uni-R, each of the 96 ORNL-HgcAB-uni-R oligonucleotide sequences are found in amplicons. However, 65 oligonucleotide sequences fall below the 1.04% threshold that represents equal distribution of reverse primer sequences across amplicon sequences; 59 of which were not conserved in the primer redesign (Figure 2). Of the 32 possible ORNL-HgcAB-uni-32R sequences, 14 do not appear in the reference hgcB database (i.e., 43.8% redundant) (Supplementary Table S5). The ORNL-HgcAB-uni-32R oligonucleotide sequences were tallied for each clone (n = 369) and amplicon (n = 233,934) amplified by ORNL-HgcAB-uni-32R to determine the frequency of each oligonucleotide to generate a PCR product (Supplementary Table S5). When searching the 233,934 hgcAB amplicon sequences, all 32 ORNL-HgcAB-uni-32R oligonucleotide sequences match at least 0.59% of amplicons. However, 22 oligonucleotide sequences fall below the 3.13% threshold that represents equal distribution of reverse primer sequences across amplicon sequences (Figure 2). This indicates that primer degeneracy could further be reduced if desired.
We identified six sequences that were underrepresented in both clone and amplicon libraries and rarely found in reference hgcAB sequences, ORNL-HgcAB-uni-32R-05, ORNL-HgcAB-uni-32R-09, ORNL-HgcAB-uni-32R-17, ORNL-HgcAB-uni-32R-21, ORNL-HgcAB-uni-32R-15, and ORNL-HgcAB-uni-32R-7 (Supplementary Table S5). These six oligonucleotide sequences were removed during the preparation of equimolar mixtures of the ORNL-HgcAB-uni-32R primer and labeled ‘equimolar 26.’ Equimolar mixtures of the 32 and 26 reverse oligonucleotide sequences were used to amplify hgcAB genes from peatland soil, periphyton, and freshwater sediments (Supplementary Figure S3). Decreasing degeneracy in the reverse primer sequence from 96 to 32, and further to 26, appeared to produce brighter, clearer bands at the target amplicon size (∼950 bp) (Supplementary Figure S3).
In silico Evaluation of ORNL-HgcAB-uni-32R Primer Binding Efficiency and Specificity
We compared the theoretical binding efficiency of ORNL-HgcAB-uni-32R and ORNL-HgcAB-uni-R primers to reference hgcB sequences (Supplementary Table S6), where efficiency is defined as a match with any of the degenerate reverse primer oligosequences. Since mismatches likely occur between primers and template DNA, in silico binding efficiencies were calculated allowing for up to two mismatches. Mismatches within five bases of the 3′ were not allowed. When allowing for mismatches, binding efficiencies of both reverse primers to reference sequences increased to 98% coverage (Supplementary Table S6 and Supplementary Figure S4). Mismatches primarily occurred at the third or fourth nucleotide base from the 5′ binding position of the reverse primer. This correlates with the fairly conserved 5′ end guanines which are (>30% but <100% sequence identity) (Figure 1). Reverse primer degeneracy was decreased by a factor of three by changing the third base of ORNL-HgcAB-uni-R from ‘B’, (C/T/G), to ‘G.’ Mismatches at these sites would likely not decrease binding efficiencies due to the proximity to the 5′ end of the primer-bind region (Campos and Quesada, 2017). When analyzing the ability of each primer to bind in silico to hgcB sequences from various clades, sequences that appear most often in the database were conserved between the primers (Supplementary Figure S4). When allowing for mismatches, there was no loss in primer binding efficiency to hgcB from various clades, despite decreased degeneracy (Supplementary Figure S4 and Supplementary Table S6).
The reverse primer targets the highly conserved ‘C(M/I)ECGA’ motif in HgcB. This triple cysteine (CXXCXXC) motif is well-conserved in other ferredoxins so non-specific reverse primer binding may occur. In silico primer specificity was tested against a reference database of 14,161 ferredoxin-encoding gene sequences. Combined, the reverse primers aligned to 786 sequences (5.6% of the database), with up to two mismatches (Supplementary Table S6). Of those matches, only 88 sequences were predicted to encode HgcB from possible Hg-methylators. Sequences were identified as HgcB by conserved motifs, sequence similarity to known HgcB using blastp, and presence of an HgcA-encoding region upstream of HgcB (if scaffolded sequence was available). Both reverse primers aligned to the 88 hgcB sequences. The additional 698 genes likely encode ferredoxins paralogous to HgcB. Shared sequences of both primers did not align completely with any paralogous sequence but matched 114 sequences when allowing for two mismatches (<1% of total ferredoxin sequences). When allowing mismatches, both reverse primers performed quite similarly in silico. Sequences unique to the less degenerate primer, ORNL-HgcAB-uni-32R matched 424 paralogous ferredoxins (3.0% of total ferredoxin sequences), while sequences unique to ORNL-HgcAB-uni-R matched 344 paralogous sequences (2.4% of total ferredoxin sequences), when allowing up to two mismatches. Overall, the primer sequences appeared unique to hgcB, and false positives occurred only when mismatches were allowed. Decreasing the degeneracy of the reverse primer did not decrease primer binding to genes encoding non-HgcB ferredoxins in silico. However, less degeneracy may increase selective amplification of hgcA and hgcB from environmental samples by limiting false positives from hybridization.
Amplification of hgcAB From Pure Cultures Using ORNL-HgcAB-uni-R32
The new less degenerate reverse primer, in combination with ORNL-HgcAB-uni-F, correctly amplified ∼950 bp hgcAB product from 25 hgcAB+ pure cultures. Species tested included Deltaproteobacteria, Firmicutes, and Archaea (Christensen et al., 2016). The 20 μl PCR mixture contained 1 × 106 copies of gDNA from pure cultures and 1.0 μM of each primer. As with ORNL-HgcAB-uni-R, no amplification was observed from Pyrococcus furiosus Vc1 which has a fused hgcAB gene. No product of the correct size was amplified from five hgcAB– cultures with either reverse primer. However, multiple bands of smaller size, and thus likely not hgcAB, were amplified from most pure cultures. Repeated attempts to purify, ligate, and clone PCR products < 950 bp failed. These extra bands are likely mispriming, possibly to ferredoxin-encoding genes, or primer dimers. Overall, amplification of hgcAB from known methylators using the new reverse primer was consistent with amplification from the more degenerate reverse primer (Christensen et al., 2016).
Optimizing PCR Amplification of hgcAB From Environmental Samples
In addition to improving the reverse primer, other PCR- modifications were tested to improve hgcAB amplification efficiencies from environmental samples. Using DNA from sediment, soil and periphyton samples, and Desulfovibrio desulfuricans ND132, we tested template concentrations between 10 ng to 100 pg, reaction volumes from 20 μl to 50 μl, and primer concentrations from 1.0 μM to 0.5 μM (see Supplementary Table S7). For low template concentrations, increasing the reaction volume to 50 μl was necessary in order to ensure enough PCR product could be gel-excised and cleaned prior to high-throughput sequencing. Both the 96-fold degenerate ORNL-HgcAB-uni-R and 32-fold degenerate ORNL-HgcAB-uni-32R were tested. PCR products were compared visually on 1% agarose gels (Supplementary Figure S3), for brightness of the ∼950 bp band. An important result was that no single PCR protocol was ideal for all samples. Periphyton were particularly difficult to amplify, although we observed improved efficiencies with lower primer to template ratios (Condition B). This was somewhat unexpected, since increased primer to template ratios can reduce PCR inhibitors (Schrader et al., 2012). In contrast, a clear PCR product was produced from freshwater sediment and peatland soil under multiple PCR conditions. We therefore recommend optimizing protocols for each sample matrix, and not assuming a one-size fits all strategy. If community composition is being evaluated, an initial estimate of appropriate template concentration can be made based on 16S rRNA amplification results (Turner et al., 1999). However, template concentrations and reaction volumes should be tested using hgcAB primer sets prior to amplification for sequencing.
Recovery of Mock Communities
The revised reverse primer ORNL-HgcAB-uni-32R recovered hgcAB+ cultures spiked into salt marsh soils in about the same abundance as the more degenerate ORNL-HgcAB-uni-R (Figure 4). Recovery of mock communities was evaluated using high-throughput amplicon specific sequencing. Both primer sets recovered hgcAB from all of the organisms used in the mock communities, however, recovery varied among organisms. For community “Combo 1” (a mix containing 41% G. sulfurreducens, 50% Ds. acidophilus, and 9% Mc. Bavaricum), Ds. acidophilus was substantially under-recovered by both primer sets, even though we found that pure Ds. acidophilus gDNA was amplified by both. One explanation may be the difficulty in isolating high quality gDNA from endospore-forming bacteria such as Ds. acidophilus (Filippidou et al., 2015). From mock community “Combo 2” (59% Dv. desulfuricans ND132, 7% Df. dehalogenans, and 35% Mm. luminyensis), Mm. luminyensis was over-represented and Dv. desulfuricans ND132 was under recovered, while Df. dehalogenans recoveries by ORNL-HgcAB-uni-32R were similar to the spike. The higher than expected recovery of the two Methanomicrobia isolates compared to Deltaproteobacteria and Firmicutes indicates a possible bias toward this clade. However, performance of both primers with the mock communities does not reflect theoretical binding efficiencies to hgcAB from Deltaproteobacteria, Methanomicrobia, and Firmicutes (Supplementary Table S6). Discrepancies between expected and actual DNA recovery could be due to extraction efficiencies (Figure 4).
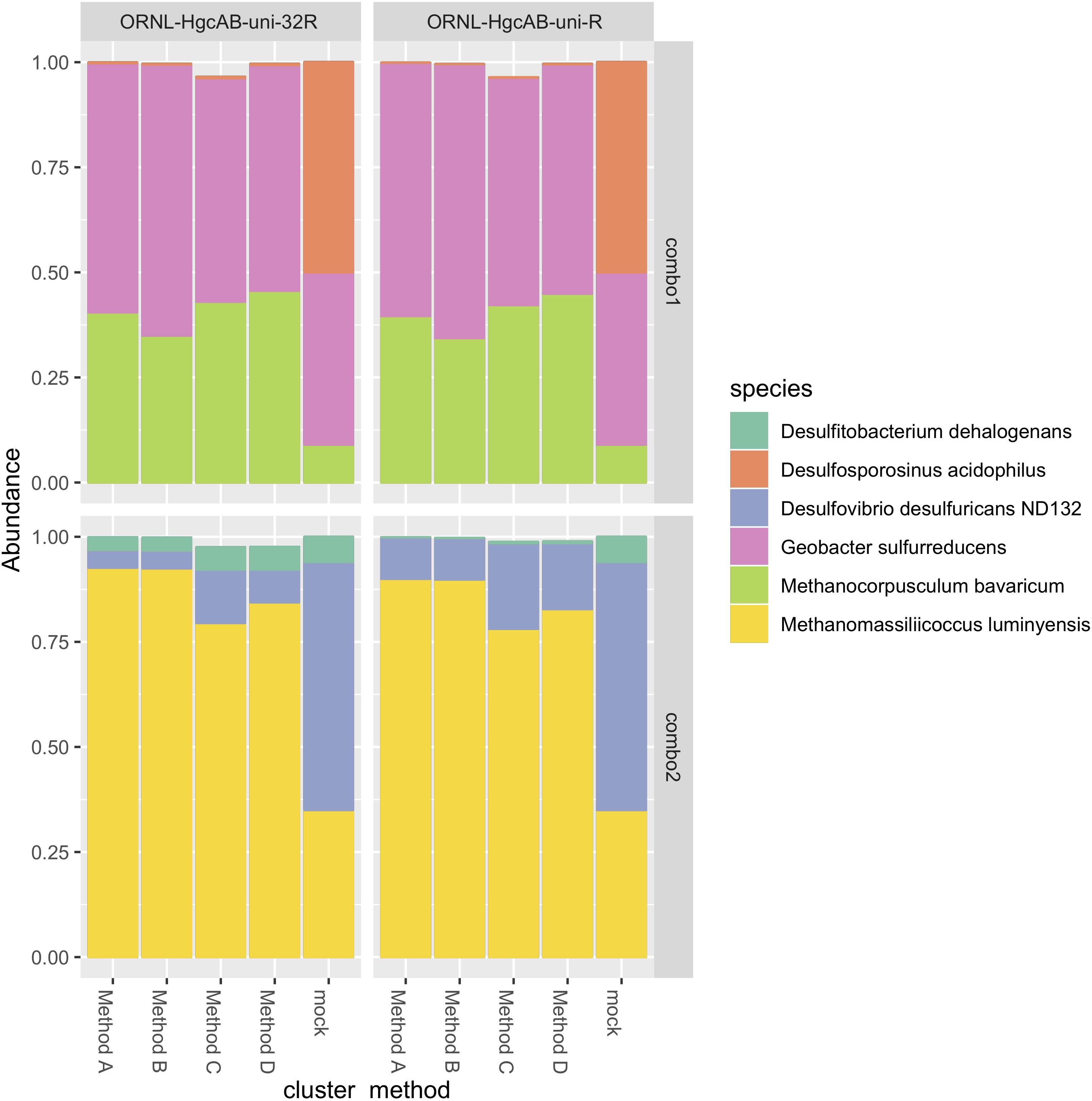
Figure 4. Comparison of expected and actual taxonomic composition of hgcA sequences in recovered mock community amplicon libraries. Top, mock community Combo1; bottom, mock community Combo2. Libraries were constructed using the newer less degenerate hgcAB reverse primer (left) or the Christensen 2016 reverse primer (right). Comparison of various clustering methods: Method A: cluster nucleotide sequences at 90% identity using VSEARCH; Method B: cluster peptide sequences at 90% identity, using USEARCH; Method C: clustering nucleotide sequences at 90% using cd-hit-est; Method D: chimeras removed prior to clustering nucleotide sequences at 90% using cd-hit-est.
Comparing OTU Clustering Methods of hgcA and HgcA Sequences
We investigated variance in nucleotide and translated peptide sequences of reference hgcA genes to determine best sequence identity cut-offs for clustering and classifying environmental clone and amplicon sequences. Clustering hgcA nucleotide sequences and classifying the translated peptide sequences at a sequence identity cut-off of 90% retained genus-level resolution in taxonomic classifications. Sequence divergence of reference hgcA genes appear incongruent to phylogeny. In the 201 nt bp reference hgcA database, 79.0% of the 283 hgcA sequences are unique from one another. Variance in hgcA nucleotide sequences increased over the 654 nt bp region, with the percent of unique sequences increasing to 80.7%. Some hgcA reference sequences were highly variant (<80% sequence identity) to one another even amongst genus-level representatives (e.g., Syntrophus, Desulfovibrio, Desulfobulbus, Desulfitobacterium). Reference hgcA genes from strain-level representatives or from MAGs from the same environment often had 100% sequence identity to one another over these two regions. With the exception of two hgcA genes from bacterial endosymbionts (i.e., Spirochaeta sp. JC202 and Deltaproteobacterium sp. OalgD1a), all other reference hgcA with 100% sequence identity shared common phylogeny. Over the 201 nt bp region of hgcA, 73.0% of reference sequences were unique at a 90% sequence identity cut-off, and 65.5% unique with an 80% sequence identity cut-off. Over the 654 nt bp region, 73.0% of reference hgcA sequences were unique at 90% sequence identity cut-off, and 68.9% were unique at an 80% cut-off. We found that clustering nucleotide sequences at 90% identity over both the 201 nt bp and 654 nt bp region kept genus-level resolution of hgcA phylogeny. With an 80% cut-off, clustering of hgcA from reference genomes of different phyla but the same environment occurred (e.g., Nitrospira bacterium SG-35-1 and Desulfobacterales bacterium SG-35-2).
Clustering of peptide sequences at 80–90% sequence identity also affected the reliability in classifying translated hgcA sequences. Collapsing subtrees of the translated 201 nt bp (67 aa bp) HgcA reference tree at a 90% cut-off reduced the nodes from 283 to 181, combining species-level variants as well as some MAGs and single cell genomes from the same environment and phyla. Collapsing the HgcA reference tree at an 80% cut-off reduced the nodes from 283 to 136 and resulted in a loss of resolution to the order level or higher for some subtrees. For example, the HgcAB fused proteins related to Desulfarculaceae, Kosmotoga, and Methanococcoides are 80% similar across the translated 201 nt bp region. The subtree containing HgcA from Aminicenantes and Atribacteria marine genomes also collapsed with an 80% cut-off. Other subtrees that collapsed included HgcA from Dehalococcoides mccartyi with Clostridium cellulosi and HgcA from Dehalobacter species with HgcA from Bacteroides cellulosolvens, Clostridium cellobioparum and Acetivibrio cellulolyticus, and Acetonema longum. Therefore, we recommend both clustering and classifying environmental samples with a 90% nt and aa sequence identity cut-off. High sequence similarity (>90%) between environmental and reference sequences likely indicates they are close relatives at the species level.
We compared several methods for OTU clustering using the mock community amplicon datasets (Figure 4). We clustered non-chimeric dereplicated nucleotide sequences at 90% with VSEARCH, clustered nucleotide sequences not filtered for chimeras at 90% using cd-hit-est, and clustered translated peptide sequences at 90% similarity with USEARCH (Rognes et al., 2016) (Figure 4). OTU clustering method affected relative abundance distribution of mock community hgcA amplicon sequences. Clustering of nucleotide sequences at 90% identity using cd-hit-est was closest to the expected composition of the mock community. However, biases are likely to occur with any clustering method. Chimera filtering removed 7.9% of sequences from the mock community hgcAB dataset (Figure 4), consistent with detection rates for other high-throughput amplicon libraries (Haas et al., 2011; de Muinck et al., 2017; Sze and Schloss, 2019). Slightly fewer putative chimeric sequences were detected in libraries amplified with 30 cycles than those amplified with 35 cycles (roughly 1–2% fewer). Non-chimeric sequences clustered at 90% using cd-hit-est were used for downstream analyses.
Direct Amplicon Sequencing From Environmental Samples
We validated PCR amplification of hgcAB from environmental matrices by Sanger sequencing the cloned PCR product and directly sequencing PCR amplicons. We observed similar phylogeny in hgcAB clone and amplicon sequences amplified using both primer-sets. The hgcAB genes were amplified from pooled SPRUCE peatland gDNA (SPRUCE1, clone IDs: OP1, NP1; Supplementary Table S3), freshwater periphyton and sediment from NH (NH3, clone IDs: OP3, NP3; Supplementary Table S3) using PCR condition B (Supplementary Table S7 and Supplementary Figure S3). Amplification from periphyton was poor, and only the PCR products from SPRUCE soil and NH sediment that amplified with both primer sets were chosen for cloning (Supplementary Figure S3). The trimmed and quality filtered hgcAB clone libraries for SPRUCE (n = 58, n = 65) and NH (n = 42, n = 61) using the 96- and 32- degenerate primers, respectively, showed similar diversity (Figure 5). The NH hgcAB genes (NH3) amplified from both primer sets were also directly sequenced, resulting in 65,832–106,964 reads per sample (Supplementary Table S4). A direct comparison of the two sequencing strategies revealed a highly similar phylogenetic distribution (Figure 5).
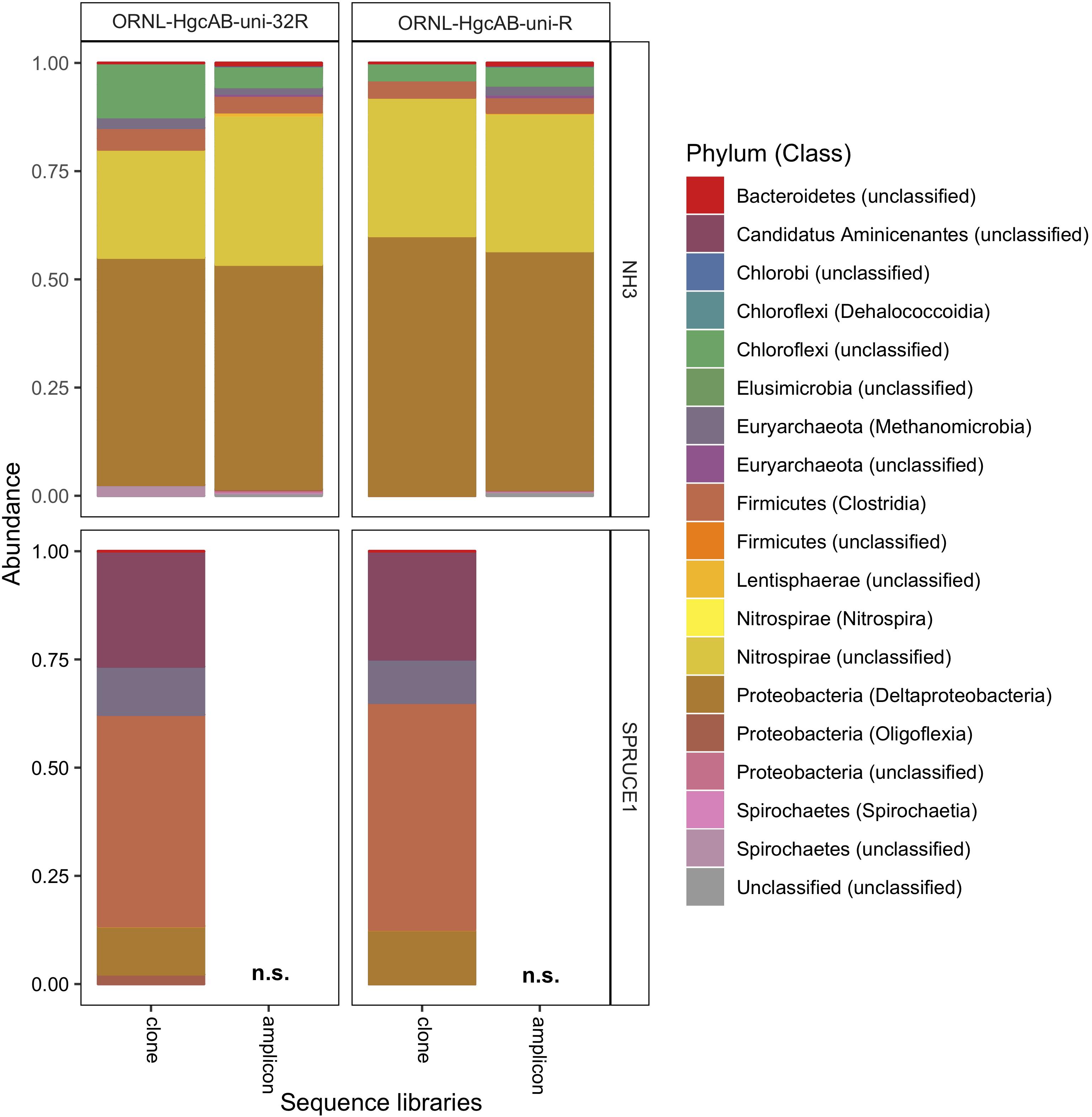
Figure 5. Relative abundance of cloned and amplicon hgcAB sequences by phyla and class. hgcAB genes were amplified from New Horizon sediment (NH3) and SPRUCE soil (SPRUCE1) using ORNL-HgcAB-uni-F with both reverse primers (ORNL-HgcAB-uni-R, ORNL-HgcAB-uni-32R). Taxonomic classifications based on phylogenetic placement of representative sequences on the reference tree (‘ORNL_HgcA_201.ref.pkg’) using program Taxtastic with classification cutoff of 90%. OTUs have been merged at the class-level, with relative abundance values calculated from proportion of total clone of amplicon sequences in each library. Figure produced using R packages ‘phyloseq’ and ‘ggplot2.’ hgcA amplicons were not sampled (n.s.) from SPRUCE soils.
Both the mock and NH communities were used to compare performance of both primer-sets in capturing diverse hgcAB sequences (Figure 3). Principle coordinate analyses (PCoA) of phylogenetic distances between hgcA OTUs indicated that sample-type rather than primer-set accounted for the greatest variance in hgcA diversity (Figure 3). Furthermore, taxonomic composition of hgcA OTUs amplified from the same mock community or NH sample, but using different reverse primers, were highly similar (Figure 6). This indicated that decreased reverse primer degeneracy did not lower the captured hgcAB diversity from the mock communities or environmental samples.
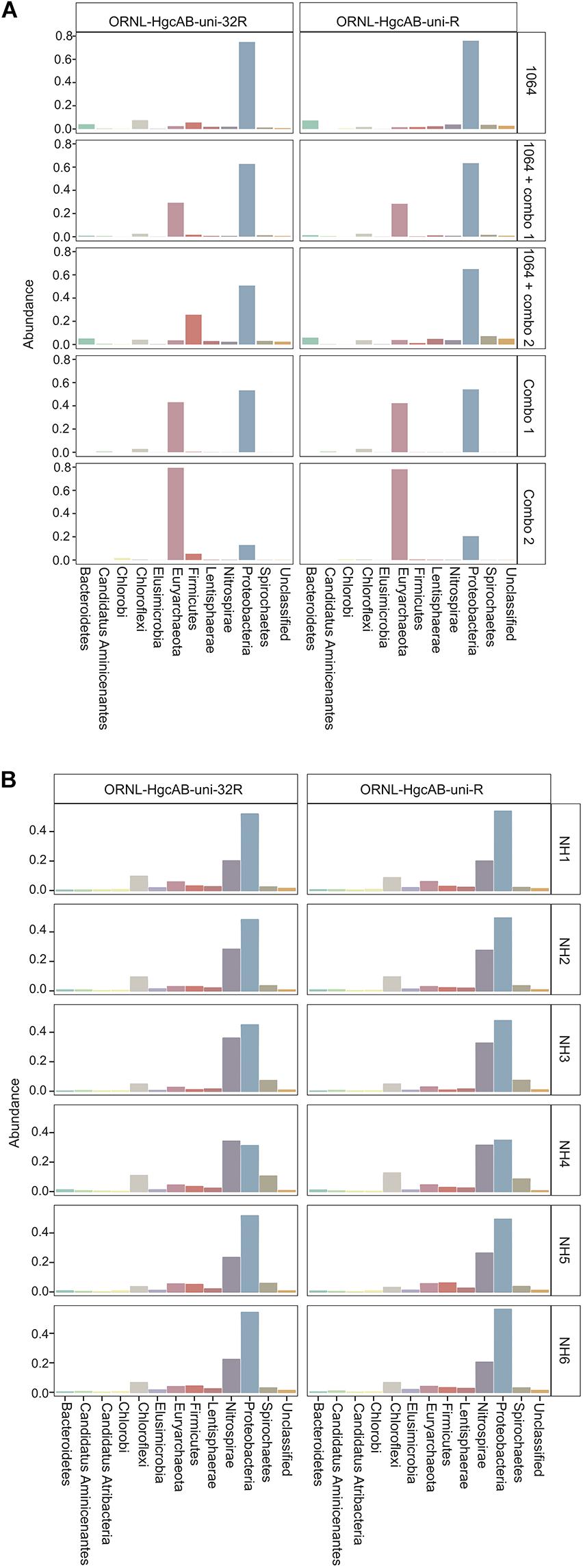
Figure 6. Relative abundance profiles of hgcA amplicons from mock (A) and environmental (B) NH sediment samples at the phyla level. OTUs were clustered at 90% sequence similarity, and taxonomic classifications were assigned based on phylogenetic placement of representative sequences on the ‘ORNL_HgcA_201.ref.pkg’ reference tree using the program ‘pplacer’ with a classification cut-off of 90%. OTUs have been merged at the phyla level.
All hgcAB sequences from NH3 and SPRUCE1 clone and amplicon libraries were compared by trimming to 201 nt bp and clustered on a 90% sequence identity cut-off, resulting in 3,861 unique operational taxonomic units (OTUs). Taxonomic classifications of translated hgcA sequences were similar between two clone libraries produced from the same sample but different primer-sets (Figure 5). The hgcA clones from SPRUCE (SPRUCE1; OP1, NP1) were classified as Methanomicrobia, Deltaproteobacteria, Firmicutes, and Aminicenantes. The hgcA clones from NH (NH3; NP3, OP3) primarily grouped with Chloroflexi, Firmicutes, Methanomicrobia, Nitrospirae, and Deltaproteobacteria, particularly Geobacter hgcA sequences. However, at the class level, several OTUs were unique to clone libraries produced with the new primer including unclassified Spirochaetes and Lentisphaerae, Oligoflexia, and Methanomicrobia (Figure 5). While rarefaction curves of clone libraries did not approach saturation, deeper MiSeq amplicon sequencing of the NH3 hgcA captured these excluded OTUs from each primer set. This indicated that both primer-sets, when evaluated at sufficient sequencing depth, can capture the same hgcAB diversity.
Broad-Range Detection of hgcAB+ Microorganisms in Environmental Samples
The new primer set captured a diverse array of sequences similar to hgcA from cultured isolates as well as environmental MAGs. A phylogenetic analysis of translated hgcA sequences highlights several novel potential Hg-methylators (Figure 7). The hgcA high-throughput amplicon libraries from freshwater EFPC sediment and tidal marsh sediment contained sequences closely related to known and potential Hg-methylators from the three major clades, Deltaproteobacteria, Methanomicrobia, and Firmicutes (Figure 7) as well as novel sequences similar to Nitrospirae, Elusimicrobia, Spirochaetes, Chloroflexi, Lentisphaerae, Bacteroidetes, Atribacteria, and candidate phyla WOR-3 and KSB1 bacteria with many lacking cultured representatives. In SPRUCE peatland clone libraries, both primers captured hgcA sequences closely related to Aminicenantes (Figure 5); however, this clade was not prevalent in freshwater or tidal marsh amplicon libraries (Figure 7). Interestingly, hgcA amplicons and clones closely related to Bacteroidetes and Chloroflexi were prevalent in both tidal marsh and freshwater sediments, while nitrogen-cycling Nitrospirae were prevalent primarily in freshwater sediment (Figures 5, 6). Several Chloroflexi, Bacteroidetes, and Nitrospirae containing hgcA have been sequenced (Baker et al., 2015; Podar et al., 2015; Anantharaman et al., 2016; Jones et al., 2019) but none have been tested for Hg-methylation. Nitrospirae are often found alongside sulfate-reducing bacteria, and can perform sulfate-reduction (Henry et al., 1994; Teske et al., 1994; Zecchin et al., 2018). Further, Nitrospirae and Bacteroidetes possess the reductive acetyl-CoA (Wood-Ljungdahl) pathway for carbon fixation and acetate production, which may be a connected biochemical pathway for HgcAB function (Baker et al., 2015). This expanded phylogeny of potential Hg-methylators includes metabolic clades associated with nitrogen-cycling, fermentation, and chemoheterotrophy. Members of these metabolic clades have previously been connected to Hg-cycling in environmental metagenome studies (Podar et al., 2015; Gionfriddo et al., 2016; Jones et al., 2019).
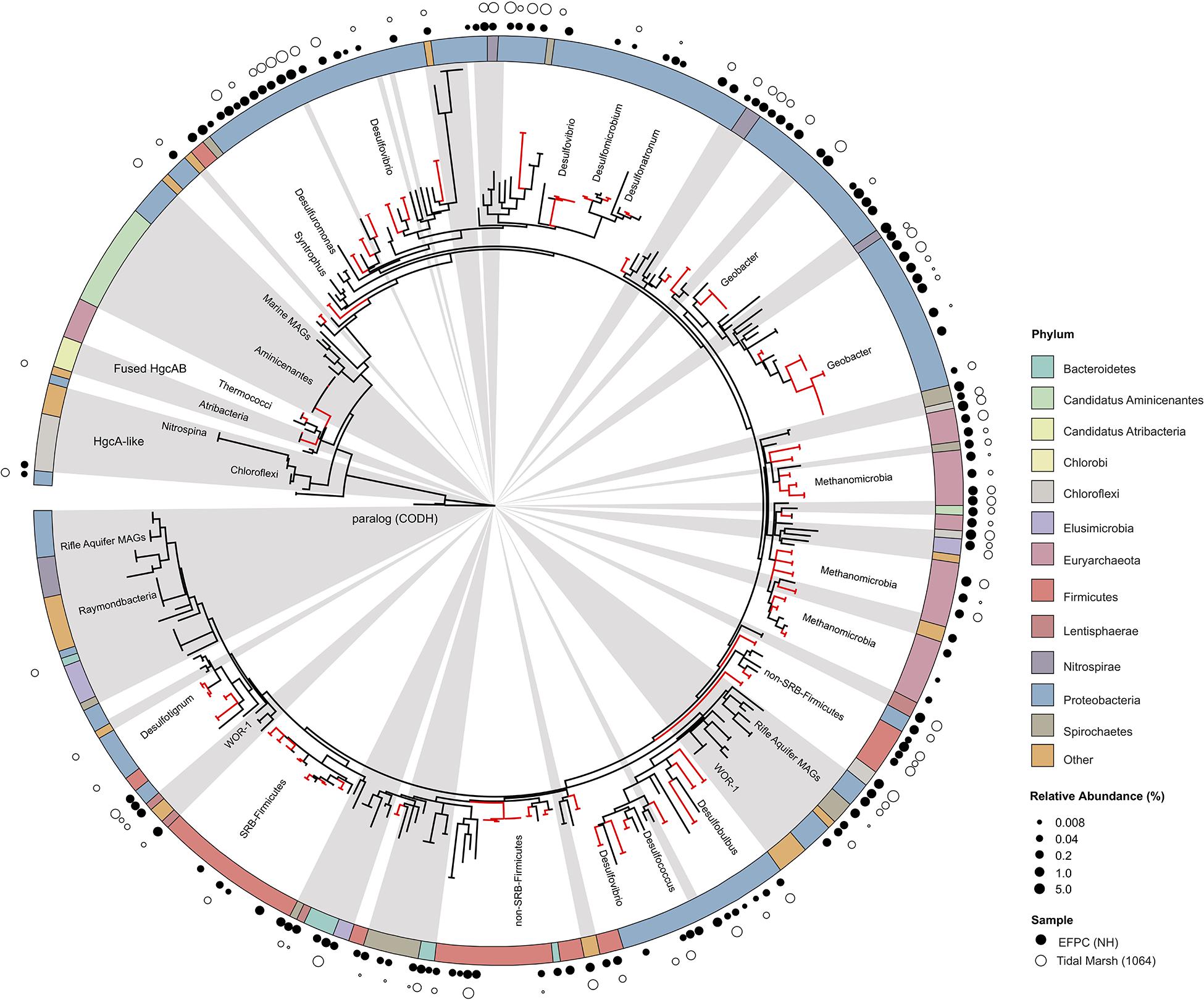
Figure 7. Molecular phylogenetic analysis of HgcA reference sequences by Maximum Likelihood method. Phylogeny was calculated using the GAMMA model of rate heterogeneity and ML estimate of alpha-parameter in RAxML with 150 bootstrap replicates. The tree was rooted by carbon monoxide dehydrogenases from non-Hg-methylators Candidatus Omnitrophica bacterium CG1-02-41-171 and Thermosulfurimonas dismutans. Relative abundance of hgcA amplicons from Tidal Marsh sediment (1064) and East Fork Poplar Creek NH sediments were calculated from OTU clustering at 90%, and the values placed on tree using ‘pplacer.’ Phylum-level classifications of HgcA reference sequences are color-coded, and distinct subtrees are labeled, including metagenome-assembled genomes from Rifle Aquifer Field Research Site and marine datasets. ‘Other’ phyla include phyla with low number of HgcA representatives: Planctomycetes, Nitrospina, and Candidate phyla Raymondbacteria, WOR-1, WOR-3, and Wallibacteria. Gray background indicates reference sequences are from metagenome-assembled genomes (MAGs) or environmental single cell genome center sequencing (SCGC) datasets. Red branches indicate that sequences were included in phylogenetic analysis from Podar et al. (2015). Black branches are sequences that have since been identified.
Directly sequencing hgcA amplicons enables a deeper dive into the diversity of the Hg-methylator community compared to cloning and metagenomic techniques, especially when community composition is complex. We used rarefraction curves to compare the richness of hgcA OTUs in NH sediment captured by cloning, high-throughput amplicon, and metagenomic sequencing techniques (Supplementary Figure S5). The NH3 clone and amplicon hgcA sequences from this study were compared to hgcA sequences pulled from a NH sediment metagenome from a previous study (Christensen et al., 2019). The hgcA sequences from each technique were pooled into one dataset and processed following the OTU-clustering and hgcA classification pipeline described above. The clone and metagenome datasets captured only a small subset of the total number of hgcA OTUs compared to high-throughput amplicon sequencing. The NH3-uni-R clone library captured 25 OTUs from 33 reads, the NH3-uni-32R captured 42 OTUs from 57 reads, and the NH metagenome captured 63 OTUs from 79 hgcA reads (pulled from 6.31 Gbp of metagenomic sequencing). The clone libraries captured the relatively abundant OTUs in the HTS dataset. The NH3-uni-R and NH3-uni-32R clone OTUs contained 25.6 and 33.2% of the total abundance of hgcA reads in the dataset, respectively. The metagenome hgcA OTUs represented only 1.5% of the total number of reads in the dataset. However, this may be because the metagenomic sequencing was performed on a different sample than NH3 and is not directly comparable. Overall it appears that deeper sequencing would be needed to capture the same diversity of hgcA sequences captured by high-throughput amplicon sequencing (Supplementary Figure S5).
The retention of low abundant and unique sequences may over-estimate hgcA diversity in a sample. When singleton sequences were kept in analysis, the amplicon dataset produced using the more degenerate primer-set (NH3-amplicon-uni-R) had a higher richness in OTUs (Supplementary Figure S5). Inclusion of singleton sequences in rarefraction analysis likely overestimates OTU richness because of spurious OTUs resulting from sequencing error (Huse et al., 2010). Indeed, the sequencing error rate calculated using the mock community amplicons produced with ORNL-HgcAB-uni-R were slightly higher (2.20–2.27%) compared to ORNL-HgcAB-uni-32R (1.94–2.05%). We recommend removing singletons prior to OTU-clustering and performing quality filtering steps such as trimming poor-quality bases, chimera filtering, and filtering non-hgcA reads using the hmm-model to remove spurious OTUs. Even following these guidelines, it is important to note that high-throughput amplicon sequencing may still over-estimate hgcA abundance in a sample.
Insights From Applying the Updated Protocol to a Previously Studied Environment
Use of the new primer set, hgcAB reference library and improved PCR protocols expanded the known diversity of methylators in EFPC sediments, including New Horizon (NH), a previously well-studied site (Mosher et al., 2012; Christensen et al., 2016, 2019). Prior studies at EFPC identified the dominant members of the Hg-methylating community including Deltaproteobacteria, methanogenic Archaea, and Firmicutes based on 16S rDNA sequencing, clone-derived hgcAB sequencing, and meta-omic analyses (Mosher et al., 2012; Christensen et al., 2016, 2019). With the improved primer set and analysis pipeline we have recovered hgcA genes from all clades represented in the previous studies as well as novel potential methylators like Nitrospirae and Elusimicrobia, as discussed above. The high-throughput nature of this updated method allowed for comparisons of the hgcA population across the sediment depth profile (Figure 8) showing the relative abundance of Proteobacteria decreasing with depth while Firmicutes and methanogenic Archaea increase in relative abundance. Nitrospirae containing hgcA were abundant in these samples, representing up to 20% of the hgcA population. The updated analysis pipeline provides a more nuanced understanding of the Hg-methylating community than previously available and suggests that novel potential methylators may represent a larger proportion of the Hg-methylating community in the stream than previously realized.
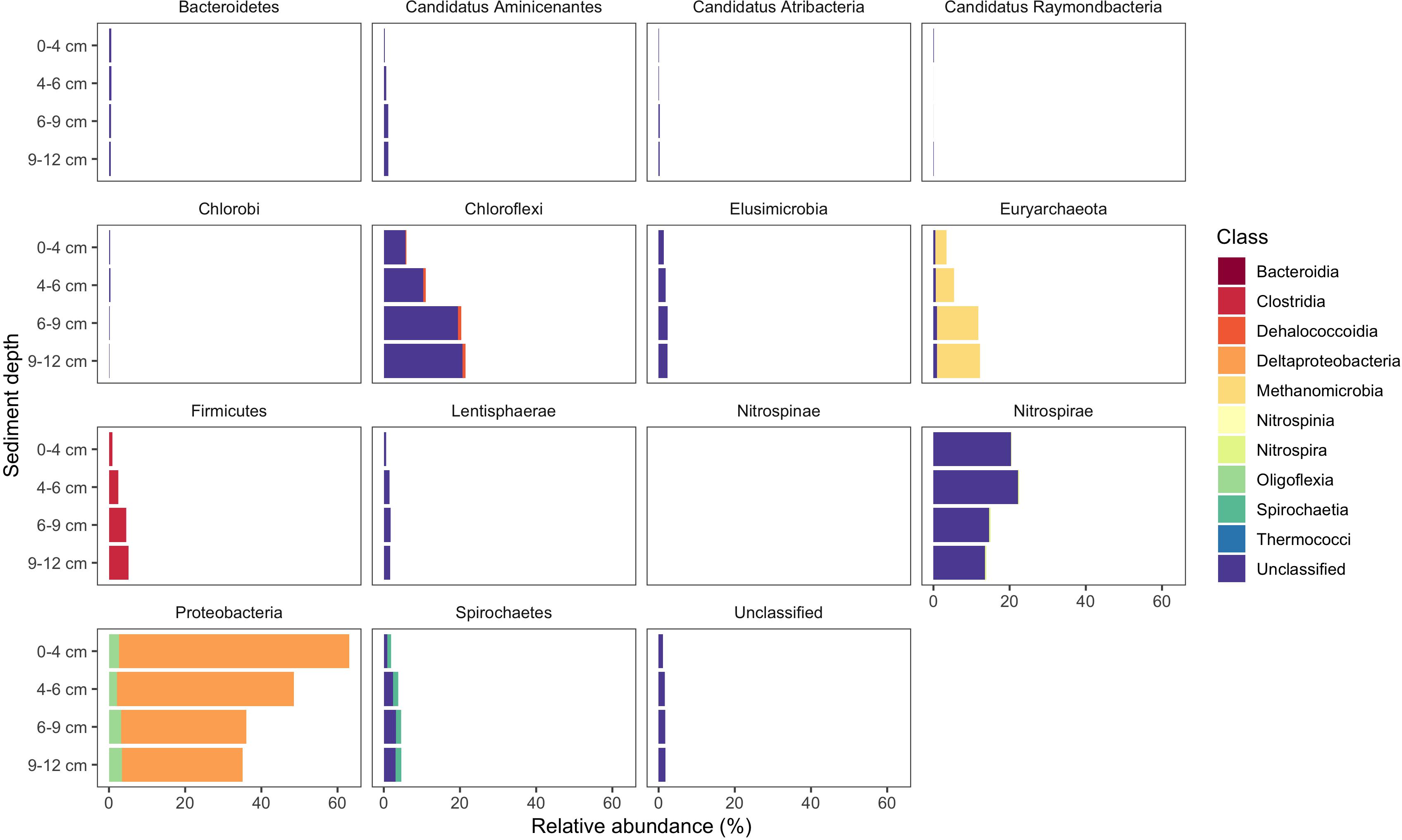
Figure 8. Known and novel methylators in a sediment core from East Fork Poplar Creek (Oak Ridge, TN, United States). Relative abundance of hgcA phyla across a 12 cm sediment core were identified using the new degenerate primers, direct sequencing technique, and the updated hgcA database. Known methylators (Deltaproteobacteria, Firmicutes-Clostridia, and Euryarchaeota-Methanomicrobia) were recovered as well as novel potential methylators.
Discussion
Broad-range PCR primers can measure the presence and diversity of genes in environmental samples. In this study, we provide updated primers and protocols for amplifying genes encoding Hg-methylation to best capture Hg-methylator diversity. While primer degeneracy is necessary to capture hgcAB diversity, a trade-off in specificity and binding efficiencies may occur. This new and improved PCR protocol was modified to enhance amplification efficiencies of hgcAB from environmental samples by decreasing the reverse primer degeneracy from 96 to 32. The reverse primer is paired with the published forward broad-range primer which has a degeneracy value of 48 (Christensen et al., 2016). Since the inception of hgcA and hgcAB primers (Bae et al., 2014; Liu et al., 2014; Schaefer et al., 2014; Christensen et al., 2016), most amplification has been followed by laborious clone library construction and Sanger sequencing. One drawback is the limited number of hgcAB+ organisms that can be identified (50–100 clones/library) as well as inherent protocol bias. Direct amplicon sequencing is desirable given the increased data-output and lower labor (Bravo et al., 2018a; Liu Y. et al., 2018). Here we include direct hgcAB amplicon sequencing which was validated using a mock community and tidal salt marsh soils spiked with the mock community.
Evaluating HgcA Phylogeny
With the growing number of available reference HgcA-encoding sequences, it is apparent that the phylogeny of HgcA sequences is not congruent to species trees. While the majority of HgcA sequences tend to cluster by clade, there are several exceptions (Figure 7). The Deltaproteobacteria HgcA are most similar to one another, with sulfate-reducing, syntrophic, and metal-reducing members forming their own subtrees. The same is true for fermentative and sulfate-reducing Firmicutes, and Methanomicrobia. However, clustering patterns are not as well defined for HgcA representatives from lesser represented taxa, for example Elusimicrobia, Spirochaetes, Phycisphaerae, and Lentisphaerae (Figure 7). Often HgcA sequences extracted from environmental MAGs diverge from pure-culture HgcA from the same phyla. Metagenome-resolved HgcA sequences tend to cluster by environment, with HgcA from marine metagenomes often forming distinct subtrees from those sequenced from freshwater aquifers (Figure 7) (Rinke et al., 2013; Baker et al., 2015; Anantharaman et al., 2016; Bendall et al., 2016; Seitz et al., 2016; Probst et al., 2018; Tully et al., 2018). For example, several Nitrospirae HgcA-encoding genomes sequenced from aquifer groundwater and estuarine sediments either form their own distinct subtree or cluster with Deltaproteobacteria (Baker et al., 2015; Anantharaman et al., 2016). This may be indicative of the close relationship of some Nitrospirae to Deltaproteobacteria based on 16S rRNA gene analyses, as well as their cohabitation of sulfate-reducing environments (Teske et al., 1994).
Overall the majority of HgcA sequences branch from a cluster of HgcAB fused proteins related to Thermococci, Atribacteria (candidate division OP9), Aminicenantes (OP8), and Chloroflexi (Figure 7). The pattern of distribution and diversity amongst hgcAB+ microbes has been attributed to horizontal gene transfer in environments supporting syntrophic interactions, similar to the Woods Ljungdahl (WL) pathway (Podar et al., 2015). Due to the discontinuity between hgcA and organism phylogeny, taxonomic identification from hgcA sequences alone are not conclusive. However, the similarity between clone, amplicon, or metagenomic read and representative hgcA sequences can estimate the prevalence and diversity of Hg-methylators through nearest neighbor analyses.
Classifying Short-Read hgcA Sequences
Environmental samples from Hg-cycling environments (Supplementary Table S3) were amplified with the new primers, cloned, trimmed, and aligned to both our 654 nt bp and 201 nt bp hgcA reference databases (Supplementary Table S1). The reference database was compiled of hgcA genes from cultured, single cell genomes, and MAGs using taxonomy information from the NCBI database (Federhen, 2012). This provided a direct comparison of processing and classifying translated hgcA sequences over different sized portions of the gene. Generally, the predicted HgcA phylogeny from the 201 nt bp sequence agreed with the 654 nt bp classification (Figure 9). The environmental clones were primarily similar to HgcA from Chloroflexi, Euryarchaeota, Firmicutes, Nitrospirae, and Proteobacteria (Figure 9). There were notable disagreements in classification using different sized portions of the gene.
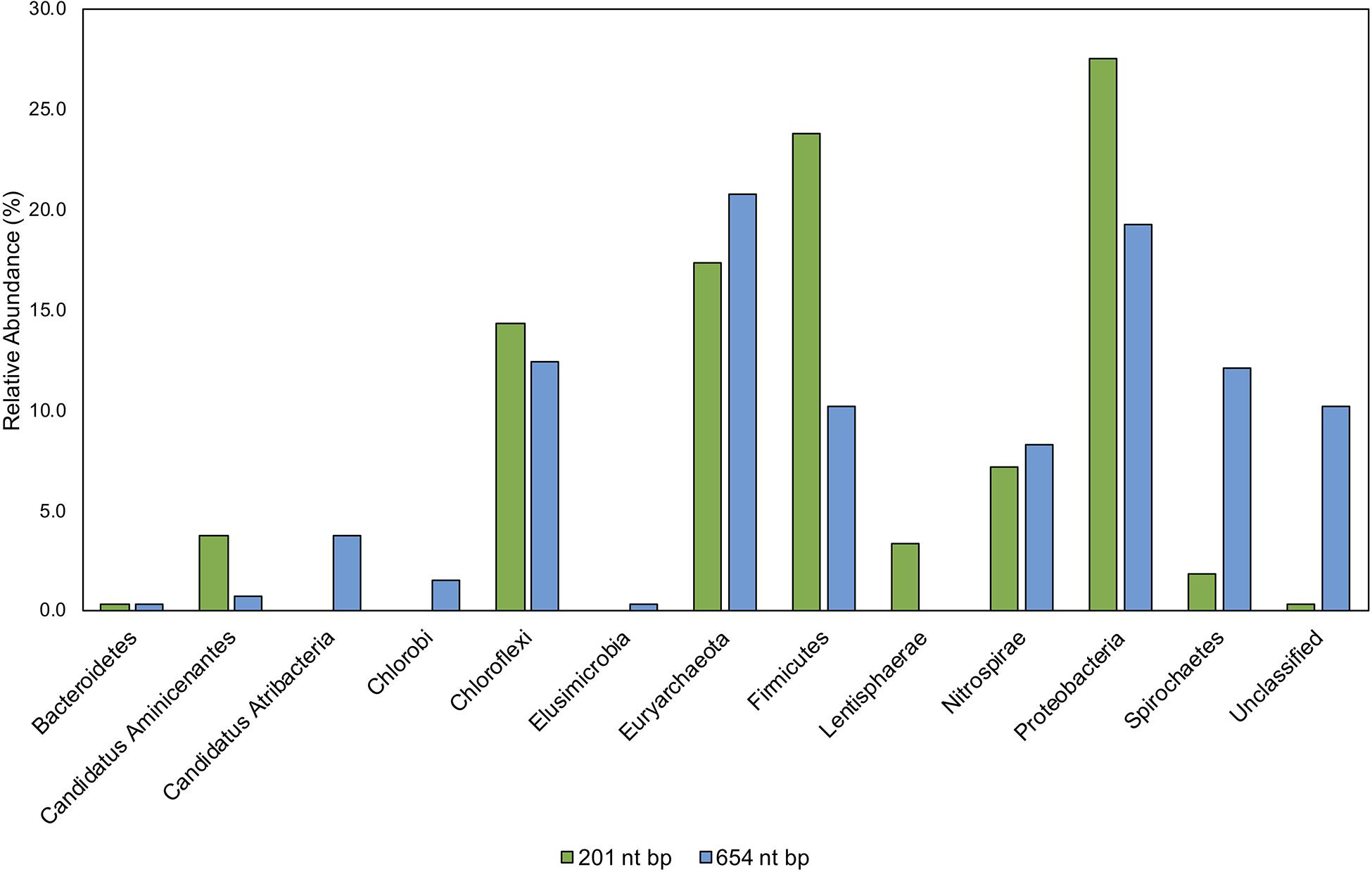
Figure 9. Comparison of taxonomic classifications of environmental hgcA clone sequences (n = 265) trimmed to 201 nt bp or 654 nt bp. Classifications were assigned to the translated hgcA clone sequences using ‘pplacer’ and ‘guppy classify’ with either the ‘HgcA_201.refpkg’ or ‘HgcA_654_full.refpkg.’ Relative abundance of phylum level classifications are reported.
Classifications based on shorter read length may over-predict similarity to reference sequences. This is likely due to a decrease in pairwise identity from 54.3 to 36.1% across the translated 201 nt bp to 654 nt bp region in all included reference HgcA (excluding paralog sequences). Therefore, trimming a sequence from 654 to 201 bp could increase its pairwise identity to reference sequences, inflating confidence values. Approximately 10% of the clone sequences (n = 265) that were assigned taxonomy when only 201 nt bp was included, became unclassified when 654 nt bp was used for analysis. Decreasing the classification cut-off by 30% for the longer reads did not overcome this short-fall.
While the overall structure of the maximum likelihood trees of HgcA in both reference packages are similar, inclusion of a larger portion of the gene helps resolve subtrees that contain mixed phylogeny. For example, over the translated 201 nt bp portion of hgcA, phyla Aminicenantes and Atribacteria are 72% similar. But over the translated 654 nt bp portion of hgcA, similarity drops to 33%. Discrepancies in classification often occurred due to differences in resolution between reference trees. Classification of short sequences likely over estimated sequence similarity to Aminicenantes, Lentisphaerae, Spirochaetes, Proteobacteria, Firmicutes but under-estimated Atribacteria, Chlorobi, and Elusimicrobia (Figure 9).
Potential Biases in Identifying Key Hg-Methylators
Early hgcA primers could be biased toward Deltaproteobacteria, Methanomicrobia, and Firmicutes (Liu et al., 2014; Schaefer et al., 2014), often identifying Deltaproteobacteria and Methanomicrobia as dominant with less representation from Firmicutes, Chloroflexi, and unclassified clades (Liu et al., 2014; Schaefer et al., 2014; Du et al., 2017; Bravo et al., 2018a). While sulfate-reducing bacteria may be significant Hg-methylators (Compeau and Bartha, 1985; Kerin et al., 2006; Gilmour et al., 2018), it is unclear what role confirmation bias has had in their association with Hg cycling. With high-throughput hgcAB sequencing, one bias may be short sequence lengths (i.e., <300 bp), only partially capturing hgcA and hgcB. While platforms (e.g., PacBio, Nanopore) advance toward longer read capability (>1 kbp), error rates are often two orders of magnitude or greater compared to short reads (Ardui et al., 2018). Hence, reliance on these platforms (Liu Y. et al., 2018) should be approached with caution. However, the implicit error rate in PacBio sequencing can be corrected at least in part, via circular consensus sequencing, but the amplicon length must be 1/3-1/4 of the maximum read length in order to achieve higher quality reads (Francis et al., 2018). While low throughput, Sanger sequencing provides high quality sequencing of the entire amplicon and can validate the shorter read platforms, as performed herein but is unfortunately not routinely performed, e.g. (Liu Y. et al., 2018).
Use of the new reference database increased diversity in previously published sequencing studies where classification used a smaller database. Biases may also be introduced during classification of translated hgcA sequences due to HgcA phylogeny being incongruent to species trees and limited reference sequences (Podar et al., 2015; McDaniel et al., 2020; Peterson et al., 2020). Therefore including new hgcAB+ organism discoveries are critical (Parks et al., 2013) since new reference sequences may identify ‘unclassified’ hgcA sequences. Using an outdated database for hgcA classification can underestimate Hg-methylator diversity. A recent study used PacBio hgcAB amplicon and Illumina MiSeq metagenomic sequencing from rice paddies without Sanger sequencing validation or using the circular consensus protocol to correct the PacBio data, and indicated that the Hg-methylating community was dominated by Deltaproteobacteria and Methanomicrobia, with some Firmicutes and Chloroflexi (Liu Y. et al., 2018). Additionally, taxonomic classifications were performed with an outdated (65 hgcAB sequences) database limited to Deltaproteobacteria, Methanomicrobia, Firmicutes, and Chloroflexi (Liu Y. et al., 2018). We applied our 201 nt bp database to the publicly available metagenomic reads, since the amplicon data were not made public, and found that hgcA closely related to Deltaproteobacteria, Methanomicrobia, Chloroflexi, and Firmicutes were present but also present were Aminicenantes, Atribacteria, candidate division WOR-1, Chlorobi, Thermococci, Oligoflexi, Nitrospirae, Spirochaetes, Lentisphaerae, Bacteroidetes and Elusimicrobia and unclassified Bacteria and Euryarchaeota (Figure 10). This represents a 25.4–37.1% increase in diversity. We also found that hgcA were dominated by Deltaproteobacteria (39–49%) compared to their findings (40%). However, Methanomicrobia did not comprise ∼60% of the hgcA community, but rather was 5.1–10.5%, similar to Chloroflexi (7.2–15.1%), Firmicutes (5.4–10.2%), and Nitrospirae (3.1–9.1%). While Geobacter spp. reportedly comprised ∼34% of the communities with low hgcAB+ sulfate-reducer abundances, our analyses showed Geobacter spp. (2.8-12.9%) and sulfate-reducers were equivalent (3.6-6.1%), suggesting that both Fe(III)- and sulfate- reduction are important and both contribute to Hg-methylation. Going forward it is imperative that such biases are recognized since the implications of incorrect data analysis and interpretation can lead to erroneous conclusions. Should these data be used in predictive models, the models would most likely be incorrect, invalidating the model for less than obvious reasons, and likely leading investigators down the wrong path.
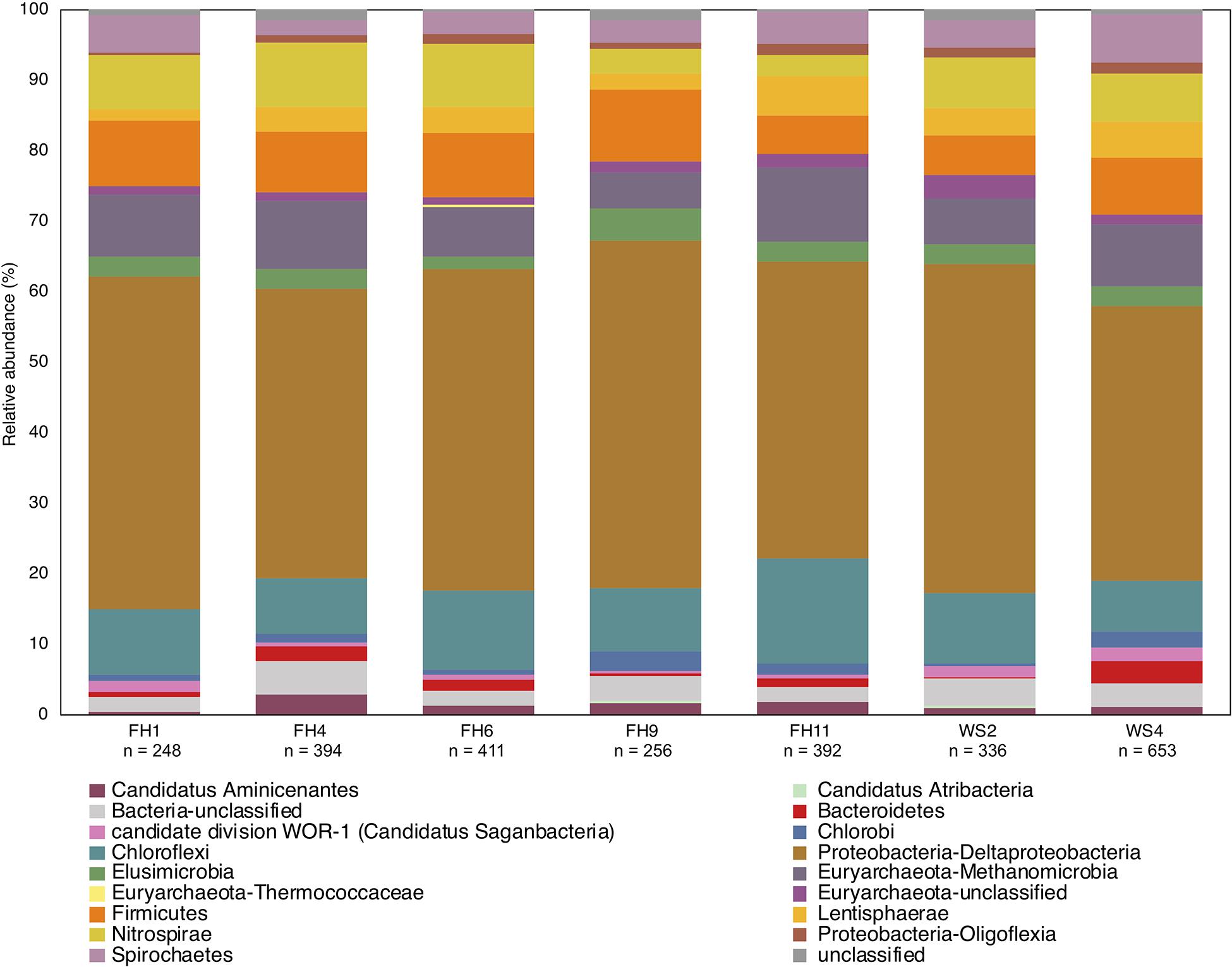
Figure 10. Phylogenetic composition of hgcA genes from published rice paddy metagenomes (Genbank PRJNA450451) at the phylum and class level (Liu Y. et al., 2018). The hgcA genes were identified in raw sequence reads using the hmm profile from the in-house reference package (HgcA_201.refpkg) with an inclusion value 1E-7. Taxonomic classifications were made using the in-house reference package with a confidence cut-off of 90%. Relative abundance of hgcA genes normalized to total hgcA genes (n) that aligned to reference package in each metagenome.
Critically, the revised primer set and protocol outlined herein improves our ability to capture the hgcAB diversity in shotgun metagenomic analysis of environmental samples (Figure 7). Both primer-sets captured hgcA related to the Deltaproteobacteria, Firmicutes, and Methanomicrobia as well as clades representing novel potential Hg-methylators including Nitrospirae, Phycisphaerae, Aminicenantes, Spirochaetes, Chloroflexi, and Elusimicrobia. We conclude that the original reverse primer degeneracy (Christensen et al., 2016) is not necessary. Amplification efficiencies from environmental samples have been improved with the new primers and can now be used directly with high-throughput sequencing to achieve ∼100,000 hgcAB amplicons per sample. The ability to capture and directly sequence highly diverse hgcAB amplicons from environments will advance our understanding of Hg-methylator distribution across phylogeny and environment. Being able to correctly identify a broad-range of Hg-methylators is critical to understanding the key players in MeHg production and accumulation in aquatic ecosystems. Knowing which organisms are most strongly contributing to Hg methylation in a given environment is crucial for constructing an ecosystem-level biogeochemical model for Hg methylation as well as designing strategies for mitigating MeHg production.
Data Availability Statement
The datasets generated for this study can be found in the environmental clone hgcA sequences from this study are publicly available under the NCBI GenBank accession numbers MT122211 – MT122744. The environmental and mock community hgcAB amplicon raw sequence files are publicly available at the NCBI SRA accession (PRJNA608965).
Author Contributions
CMG did the primer design and testing with assistance from AW, DE, and MP, based on modifications to earlier designs by GC, DE, AW, and MP. DJ developed the high-throughput sequencing method. AW, ML, CCG, RW, and CMG contributed to the environmental DNA extraction, PCR, and cloning method development and testing. AS and CCG provided the model communities and DNA extractions. CMG did the primary writing with assistance from RW, DE, AW, and CCG. All authors contributed to the article and approved the submitted version.
Funding
This research was sponsored by the Office of Biological and Environmental Research, Office of Science, US Department of Energy (DOE) as part of the Mercury Science Focus Area at Oak Ridge National Laboratory, which is managed by UT-Battelle LLC for the DOE under contract DE-AC05-00OR22725.
Conflict of Interest
The authors declare that the research was conducted in the absence of any commercial or financial relationships that could be construed as a potential conflict of interest.
Acknowledgments
We wish to thank and acknowledge the staff at the University of Minnesota Genomics Center (UMGC) for advice and assistance with high-throughput sequencing of custom hgcAB amplicon libraries. We also thank Brandy Toner (University of Minnesota), for peat, hollow and hummock samples from SPRUCE, Caroline Pierce for SPRUCE Hg/MeHg data, Helen Hsu-Kim for sediment samples from Sandy Creek, NC, United States, and Baohua Gu for rice paddy soils from Yanwuping, China. This manuscript has been authored by UT-Battelle, LLC under Contract No. DE-AC05-00OR22725 with the U.S. Department of Energy. The United States Government retains and the publisher, by accepting the article for publication, acknowledges that the United States Government retains a non-exclusive, paid-up, irrevocable, world-wide license to publish or reproduce the published form of this manuscript, or allow others to do so, for United States Government purposes. The Department of Energy will provide public access to these results of federally sponsored research in accordance with the DOE Public Access Plan (http://energy.gov/downloads/doe-public-access-plan).
Supplementary Material
The Supplementary Material for this article can be found online at: https://www.frontiersin.org/articles/10.3389/fmicb.2020.541554/full#supplementary-material
Footnotes
- ^ https://github.com/fhcrc/taxtastic
- ^ http://www.geneious.com
- ^ https://img.jgi.doe.gov/
- ^ https://cran.r-project.org/web/packages/BoSSA/
References
Altschul, S. F., Gish, W., Miller, W., Myers, E. W., and Lipman, D. J. (1990). Basic local alignment search tool. J. Mol. Biol. 215, 403–410. doi: 10.1016/S0022-2836(05)80360-2S0022-2836(05)80360-2
Anantharaman, K., Brown, C. T., Hug, L. A., Sharon, I., Castelle, C. J., Probst, A. J., et al. (2016). Thousands of microbial genomes shed light on interconnected biogeochemical processes in an aquifer system. Nat. Commun. 7:13219.
Ardui, S., Ameur, A., Vermeesch, J. R., and Hestand, M. S. (2018). Single molecule real-time (SMRT) sequencing comes of age: applications and utilities for medical diagnostics. Nucleic Acids Res. 46, 2159–2168. doi: 10.1093/nar/gky066
Azaroff, A., Urriza, M. G., Gassie, C., Monperrus, M., and Guyoneaud, R. (2020). Marine mercury-methylating microbial communities from coastal to Capbreton Canyon sediments (North Atlantic Ocean). Environ. Pollut. 262:114333. doi: 10.1016/j.envpol.2020.114333
Bae, H.-S., Dierberg, F. E., and Ogram, A. (2014). Syntrophs dominate sequences associated with the mercury methylation-related gene hgcA in the water conservation areas of the Florida Everglades. Appl. Environ. Microbiol. 80, 6517–6526. doi: 10.1128/aem.01666-14
Baker, B. J., Lazar, C. S., Teske, A. P., and Dick, G. J. (2015). Genomic resolution of linkages in carbon, nitrogen, and sulfur cycling among widespread estuary sediment bacteria. Microbiome 3:14.
Bendall, M. L., Stevens, S. L., Chan, L.-K., Malfatti, S., Schwientek, P., Tremblay, J., et al. (2016). Genome-wide selective sweeps and gene-specific sweeps in natural bacterial populations. ISME J. 10, 1589–1601. doi: 10.1038/ismej.2015.241
Bolger, A. M., Lohse, M., and Usadel, B. (2014). Trimmomatic: a flexible trimmer for Illumina sequence data. Bioinformatics 30, 2114–2120. doi: 10.1093/bioinformatics/btu170
Bowman, K. L., Collins, R. E., Agather, A. M., Lamborg, C. H., Hammerschmidt, C. R., Kaul, D., et al. (2019). Distribution of mercury-cycling genes in the Arctic and equatorial Pacific Oceans and their relationship to mercury speciation. Limnol. Oceanogr. 65, S310–S320.
Bravo, A. G., and Cosio, C. (2019). Biotic formation of methylmercury: a bio–physico–chemical conundrum. Limnol. Oceanogr. 65, 1010–1027. doi: 10.1002/lno.11366
Bravo, A. G., Peura, S., Buck, M., Ahmed, O., Mateos-Rivera, A., Ortega, S. H., et al. (2018a). Methanogens and iron-reducing bacteria: the overlooked members of mercury-methylating microbial communities in boreal lakes. Appl. Environ. Microbiol. 84:e01774-18.
Bravo, A. G., Zopfi, J., Buck, M., Xu, J., Bertilsson, S., Schaefer, J. K., et al. (2018b). Geobacteraceae are important members of mercury-methylating microbial communities of sediments impacted by waste water releases. ISME J. 12, 802–812. doi: 10.1038/s41396-017-0007-7
Brooks, S. C., and Southworth, G. R. (2011). History of mercury use and environmental contamination at the Oak Ridge Y-12 Plant. Environ. Pollut. 159, 219–228. doi: 10.1016/j.envpol.2010.09.009
Campos, M. J., and Quesada, A. (2017). Strategies to Improve Efficiency and Specificity of Degenerate Primers in PCR. Berlin: Springer, 75–85.
Chen, C. Y., Driscoll, C. T., Eagles-Smith, C. A., Eckley, C. S., Gay, D. A., Hsu-Kim, H., et al. (2018). A critical Time for Mercury Science to Inform Global Policy. Washington, DC: ACS Publications.
Christensen, G. A., Gionfriddo, C. M., King, A. J., Moberly, J. G., Miller, C. L., Somenahally, A. C., et al. (2019). Determining the reliability of measuring mercury cycling gene abundance with correlations with mercury and methylmercury concentrations. Environ. Sci. Technol. 53, 8649–8663. doi: 10.1021/acs.est.8b06389
Christensen, G. A., Wymore, A. M., King, A. J., Podar, M., Hurt, R. A., Santillan, E. U., et al. (2016). Development and validation of broad-range qualitative and clade-specific quantitative molecular probes for assessing mercury methylation in the environment. Appl. Environ. Microbiol. 82, 6068–6078. doi: 10.1128/aem.01271-16
Compeau, G., and Bartha, R. (1985). Sulfate-reducing bacteria: principal methylators of mercury in anoxic estuarine sediment. Appl. Environ. Microbiol. 50, 498–502. doi: 10.1128/aem.50.2.498-502.1985
de Muinck, E. J., Trosvik, P., Gilfillan, G. D., Hov, J. R., and Sundaram, A. Y. (2017). A novel ultra high-throughput 16S rRNA gene amplicon sequencing library preparation method for the Illumina HiSeq platform. Microbiome 5, 1–15.
Debes, F., Weihe, P., and Grandjean, P. (2016). Cognitive deficits at age 22 years associated with prenatal exposure to methylmercury. Cortex 74, 358–369. doi: 10.1016/j.cortex.2015.05.017
Du, H., Ma, M., Sun, T., Dai, X., Yang, C., Luo, F., et al. (2017). Mercury-methylating genes dsrB and hgcA in soils/sediments of the three gorges reservoir. Environ. Sci. Pollut. Res. 24, 5001–5011. doi: 10.1007/s11356-016-8213-9
Eagles-Smith, C. A., Silbergeld, E. K., Basu, N., Bustamante, P., Diaz-Barriga, F., Hopkins, W. A., et al. (2018). Modulators of mercury risk to wildlife and humans in the context of rapid global change. Ambio 47, 170–197. doi: 10.1007/s13280-017-1011-x
Eckley, C. S., Gilmour, C. C., Janssen, S., Luxton, T. P., Randall, P. M., Whalin, L., et al. (2020). The assessment and remediation of mercury contaminated sites: a review of current approaches. Sci. Total Environ. 707:136031. doi: 10.1016/j.scitotenv.2019.136031
Edgar, R. C. (2004a). MUSCLE: a multiple sequence alignment method with reduced time and space complexity. BMC Bioinformatics 5:113. doi: 10.1186/1471-2105-5-113
Edgar, R. C. (2004b). MUSCLE: multiple sequence alignment with high accuracy and high throughput. Nucleic Acids Res. 32, 1792–1797. doi: 10.1093/nar/gkh340
Edgar, R. C. (2010). Search and clustering orders of magnitude faster than BLAST. Bioinformatic 26, 2460–2461. doi: 10.1093/bioinformatics/btq461
Filippidou, S., Junier, T., Wunderlin, T., Lo, C.-C., Li, P.-E., Chain, P. S., et al. (2015). Under-detection of endospore-forming Firmicutes in metagenomic data. Comput. Struct. Biotechnol. J. 13, 299–306. doi: 10.1016/j.csbj.2015.04.002
Fleming, E. J., Mack, E. E., Green, P. G., and Nelson, D. C. (2006). Mercury methylation from unexpected sources: molybdate-inhibited freshwater sediments and an iron-reducing bacterium. Appl. Environ. Microbiol. 72, 457–464. doi: 10.1128/aem.72.1.457-464.2006
Francis, F., Dumas, M. D., Davis, S. B., and Wisser, R. J. (2018). Clustering of circular consensus sequences: accurate error correction and assembly of single molecule real-time reads from multiplexed amplicon libraries. BMC Bioinformatics 19:302. doi: 10.1186/s12859-018-2293-0
Gilmour, C. C., Bullock, A. L., McBurney, A., Podar, M., and Elias, D. A. (2018). Robust mercury methylation across diverse methanogenic archaea. mBio 9:e02403-17. doi: 10.1128/mBio.02403-17
Gilmour, C. C., Podar, M., Bullock, A. L., Graham, A. M., Brown, S. D., Somenahally, A. C., et al. (2013). Mercury methylation by novel microorganisms from new environments. Environ. Sci. Technol. 47, 11810–11820. doi: 10.1021/es403075t
Gionfriddo, C., Podar, M., Gilmour, C., Pierce, E., and Elias, D. (2019). ORNL Compiled Mercury Methylator Database. ORNLCIFSFA (Critical Interfaces Science Focus Area). Oak Ridge, TN: Oak Ridge National Lab. (ORNL).
Gionfriddo, C. M., Tate, M. T., Wick, R. R., Schultz, M. B., Zemla, A., Thelen, M. P., et al. (2016). Microbial mercury methylation in Antarctic sea ice. Nat. Microbiol. 1:16127. doi: 10.1038/nmicrobiol.2016.127
Gohl, D. M., Vangay, P., Garbe, J., MacLean, A., Hauge, A., Becker, A., et al. (2016). Systematic improvement of amplicon marker gene methods for increased accuracy in microbiome studies. Nat. Biotechnol. 34, 942–949. doi: 10.1038/nbt.3601
Goñi-Urriza, M., Klopp, C., Ranchou-Peyruse, M., Ranchou-Peyruse, A., Monperrus, M., Khalfaoui-Hassani, B., et al. (2019). Genome insights of mercury methylation among desulfovibrio and pseudodesulfovibrio strains. Res. Microbiol. 171, 3–12.
Haas, B. J., Gevers, D., Earl, A. M., Feldgarden, M., Ward, D. V., Giannoukos, G., et al. (2011). Chimeric 16S rRNA sequence formation and detection in Sanger and 454-pyrosequenced PCR amplicons. Genome Res. 21, 494–504. doi: 10.1101/gr.112730.110
Henry, E., Devereux, R., Maki, J., Gilmour, C., Woese, C., Mandelco, L., et al. (1994). Characterization of a new thermophilic sulfate-reducing bacterium. Arch. Microbiol. 161, 62–69.
Hsu-Kim, H., Eckley, C. S., Achá, D., Feng, X., Gilmour, C. C., Jonsson, S., et al. (2018). Challenges and opportunities for managing aquatic mercury pollution in altered landscapes. Ambio 47, 141–169. doi: 10.1007/s13280-017-1006-7
Hsu-Kim, H., Kucharzyk, K. H., Zhang, T., and Deshusses, M. A. (2013). Mechanisms regulating mercury bioavailability for methylating microorganisms in the aquatic environment: a critical review. Environ. Sci. Technol. 47, 2441–2456. doi: 10.1021/es304370g
Huang, Y., Niu, B., Gao, Y., Fu, L., and Li, W. (2010). CD-HIT Suite: a web server for clustering and comparing biological sequences. Bioinformatics 26, 680–682. doi: 10.1093/bioinformatics/btq003
Huse, S. M., Welch, D. M., Morrison, H. G., and Sogin, M. L. (2010). Ironing out the wrinkles in the rare biosphere through improved OTU clustering. Environ. Microbiol. 12, 1889–1898. doi: 10.1111/j.1462-2920.2010.02193.x
Huson, D. H., Beier, S., Flade, I., Górska, A., El-Hadidi, M., Mitra, S., et al. (2016). MEGAN community edition-interactive exploration and analysis of large-scale microbiome sequencing data. PLoS Comput. Biol. 12:e1004957. doi: 10.1371/journal.pcbi.1004957
Iversen, C., Hanson, P., Brice, D., Phillips, J., McFarlane, K., Hobbie, E., et al. (2012). SPRUCE Peat Physical and Chemical Characteristics From Experimental Plot Cores, 2012. ORNLTESSFA. Oak Ridge, TN: Oak Ridge National Lab’s.
Johnson, L. S., Eddy, S. R., and Portugaly, E. (2010). Hidden Markov model speed heuristic and iterative HMM search procedure. BMC Bioinformatics 11:431. doi: 10.1186/1471-2105-11-431
Jones, D. S., Lapakko, K. A., Wenz, Z. J., Olson, M. C., Roepke, E. W., Sadowsky, M. J., et al. (2017). Novel microbial assemblages dominate weathered sulfide-bearing rock from copper-nickel deposits in the Duluth complex, Minnesota, USA. Appl. Environ. Microbiol. 83:AEM.00909-17.
Jones, D. S., Walker, G. M., Johnson, N. W., Mitchell, C. P., Wasik, J. K. C., and Bailey, J. V. (2019). Molecular evidence for novel mercury methylating microorganisms in sulfate-impacted lakes. ISME J. 13, 1659–1675. doi: 10.1038/s41396-019-0376-1
Kearse, M., Moir, R., Wilson, A., Stones-Havas, S., Cheung, M., Sturrock, S., et al. (2012). Geneious Basic: an integrated and extendable desktop software platform for the organization and analysis of sequence data. Bioinformatics 28, 1647–1649. doi: 10.1093/bioinformatics/bts199
Kerin, E. J., Gilmour, C. C., Roden, E., Suzuki, M., Coates, J., and Mason, R. (2006). Mercury methylation by dissimilatory iron-reducing bacteria. Appl. Environ. Microbiol. 72, 7919–7921. doi: 10.1128/aem.01602-06
Lee, C. K., Herbold, C. W., Polson, S. W., Wommack, K. E., Williamson, S. J., McDonald, I. R., et al. (2012). Groundtruthing next-gen sequencing for microbial ecology–biases and errors in community structure estimates from PCR amplicon pyrosequencing. PLoS One 7:e44224. doi: 10.1371/journal.pone.0044224
Li, W., Cowley, A., Uludag, M., Gur, T., McWilliam, H., Squizzato, S., et al. (2015). The EMBL-EBI bioinformatics web and programmatic tools framework. Nucleic Acids Res. 43, W580–W584.
Lin, H., Ascher, D. B., Myung, Y., Lamborg, C. H., Hallam, S. J., Gionfriddo, C. M., et al. (2020). Mercury methylation by metabolically versatile and cosmopolitan marine bacteria. bioRxiv [Preprint]. doi: 10.1101/2020.06.03.132969
Liu, X., Ma, A., Zhuang, G., and Zhuang, X. (2018). Diversity of microbial communities potentially involved in mercury methylation in rice paddies surrounding typical mercury mining areas in China. MicrobiologyOpen 7:e00577. doi: 10.1002/mbo3.577
Liu, Y., Johs, A., Bi, L., Lu, X., Hu, H., Sun, D., et al. (2018). Unraveling microbial communities associated with methylmercury production in paddy soils. Environ. Sci. Technol. 52, 13110–13118. doi: 10.1021/acs.est.8b03052
Liu, Y.-R., Yu, R.-Q., Zheng, Y.-M., and He, J.-Z. (2014). Analysis of the microbial community structure by monitoring an Hg methylation gene (hgcA) in paddy soils along an Hg gradient. Appl. Environ. Microbiol. 80, 2874–2879. doi: 10.1128/aem.04225-13
Matsen, F. A., Kodner, R. B., and Armbrust, E. V. (2010). pplacer: linear time maximum-likelihood and Bayesian phylogenetic placement of sequences onto a fixed reference tree. BMC Bioinformatics 11:538. doi: 10.1186/1471-2105-11-538
McDaniel, E. A., Peterson, B., Stevens, S. L., Tran, P. Q., Anantharaman, K., and McMahon, K. (2020). Expanded phylogenetic diversity and metabolic flexibility of microbial mercury methylation. bioRxiv [Preprint]. doi: 10.1101/2020.01.16.909358
McMurdie, P. J., and Holmes, S. (2013). phyloseq: an R package for reproducible interactive analysis and graphics of microbiome census data. PLoS One 8:e61217. doi: 10.1371/journal.pone.0061217
Mitchell, C. P., and Gilmour, C. C. (2008). Methylmercury production in a Chesapeake Bay salt marsh. J. Geophys. Res. 113:G00C04.
Mosher, J. J., Vishnivetskaya, T. A., Elias, D. A., Podar, M., Brooks, S. C., Brown, S. D., et al. (2012). Characterization of the Deltaproteobacteria in contaminated and uncontaminated stream sediments and identification of potential mercury methylators. Aquat. Microb. Ecol. 66, 271–282. doi: 10.3354/ame01563
Ndu, U., Christensen, G. A., Rivera, N. A., Gionfriddo, C. M., Deshusses, M. A., Elias, D. A., et al. (2018). Quantification of mercury bioavailability for methylation using diffusive gradient in thin-film samplers. Environ. Sci. Technol. 52, 8521–8529. doi: 10.1021/acs.est.8b00647
Olsen, T. A., Brandt, C. C., and Brooks, S. C. (2016). Periphyton biofilms influence net methylmercury production in an industrially contaminated system. Environ. Sci. Technol. 50, 10843–10850. doi: 10.1021/acs.est.6b01538
Parks, J. M., Johs, A., Podar, M., Bridou, R., Hurt, R. A., Smith, S. D., et al. (2013). The genetic basis for bacterial mercury methylation. Science 339, 1332–1335. doi: 10.1126/science.1230667
Pelikan, C., Herbold, C. W., Hausmann, B., Müller, A. L., Pester, M., and Loy, A. (2016). Diversity analysis of sulfite-and sulfate-reducing microorganisms by multiplex dsrA and dsrB amplicon sequencing using new primers and mock community-optimized bioinformatics. Environ. Microbiol. 18, 2994–3009. doi: 10.1111/1462-2920.13139
Peterson, B. D., McDaniel, E. A., Schmidt, A. G., Lepak, R. F., Tran, P. Q., Marick, R. A., et al. (2020). Mercury methylation trait dispersed across diverse anaerobic microbial guilds in a eutrophic sulfate-enriched lake. bioRxiv [Preprint]. doi: 10.1101/2020.04.01.018762
Pierce, C. E., Psarska, S., Brozowski, J., Sebestyen, S. D., Kolka, R. K., Gutknecht, J. L. M., et al. (2017). SPRUCE Peat Mercury, Methylmercury and Sulfur Concentrations From Experimental Plot Cores, Beginning in 2014. Washington, DC: Department of Energy.
Podar, M., Gilmour, C. C., Brandt, C. C., Soren, A., Brown, S. D., Crable, B. R., et al. (2015). Global prevalence and distribution of genes and microorganisms involved in mercury methylation. Sci Adv. 1:e1500675. doi: 10.1126/sciadv.1500675
Probst, A. J., Ladd, B., Jarett, J. K., Geller-McGrath, D. E., Sieber, C. M., Emerson, J. B., et al. (2018). Differential depth distribution of microbial function and putative symbionts through sediment-hosted aquifers in the deep terrestrial subsurface. Nat. Microbiol. 3, 328–336. doi: 10.1038/s41564-017-0098-y
Ranchou-Peyruse, M., Monperrus, M., Bridou, R., Duran, R., Amouroux, D., Salvado, J., et al. (2009). Overview of mercury methylation capacities among anaerobic bacteria including representatives of the sulphate-reducers: implications for environmental studies. Geomicrobiol. J. 26, 1–8. doi: 10.1080/01490450802599227
Rinke, C., Schwientek, P., Sczyrba, A., Ivanova, N. N., Anderson, I. J., Cheng, J.-F., et al. (2013). Insights into the phylogeny and coding potential of microbial dark matter. Nature 499, 431–437. doi: 10.1038/nature12352
Rognes, T., Flouri, T., Nichols, B., Quince, C., and Mahé, F. (2016). VSEARCH: a versatile open source tool for metagenomics. PeerJ 4:e2584. doi: 10.7717/peerj.2584
Sayers, E. W., Barrett, T., Benson, D. A., Bolton, E., Bryant, S. H., Canese, K., et al. (2009). Database resources of the national center for biotechnology information. Nucleic Acids Res. 38(Suppl._1), D5–D16.
Schaefer, J., Kronberg, R. M., Morel, F., and Skyllberg, U. (2014). Detection of a key Hg methylation gene, hgcA, in wetland soils. Environ. Microbiol. Rep. 6, 441–447. doi: 10.1111/1758-2229.12136
Schloss, P. D., Westcott, S. L., Ryabin, T., Hall, J. R., Hartmann, M., Hollister, E. B., et al. (2009). Introducing mothur: open-source, platform-independent, community-supported software for describing and comparing microbial communities. Appl. Environ. Microbiol. 75, 7537–7541. doi: 10.1128/AEM.01541-09
Schrader, C., Schielke, A., Ellerbroek, L., and Johne, R. (2012). PCR inhibitors–occurrence, properties and removal. J. Appl. Microbiol. 113, 1014–1026. doi: 10.1111/j.1365-2672.2012.05384.x
Seitz, K. W., Lazar, C. S., Hinrichs, K.-U., Teske, A. P., and Baker, B. J. (2016). Genomic reconstruction of a novel, deeply branched sediment archaeal phylum with pathways for acetogenesis and sulfur reduction. ISME J. 10, 1696–1705. doi: 10.1038/ismej.2015.233
Sipos, R., Székely, A. J., Palatinszky, M., Révész, S., Márialigeti, K., and Nikolausz, M. (2007). Effect of primer mismatch, annealing temperature and PCR cycle number on 16S rRNA gene-targetting bacterial community analysis. FEMS Microbiol. Ecol. 60, 341–350. doi: 10.1111/j.1574-6941.2007.00283.x
Southworth, G. R., Greeley, M., Peterson, M., Lowe, K., and Ketelle, R. (2010). Sources of Mercury to East Fork Poplar Creek Downstream From the Y-12 National Security Complex: Inventories and Export Rates. ORNL/TM-2009/231. Ridge, TN: Oak Ridge National Laboratory.
Stadhouders, R., Pas, S. D., Anber, J., Voermans, J., Mes, T. H., and Schutten, M. (2010). The effect of primer-template mismatches on the detection and quantification of nucleic acids using the 5’ nuclease assay. J. Mol. Diagnost. 12, 109–117. doi: 10.2353/jmoldx.2010.090035
Stamatakis, A. (2014). RAxML version 8: a tool for phylogenetic analysis and post-analysis of large phylogenies. Bioinformatics 30, 1312–1313. doi: 10.1093/bioinformatics/btu033
Sze, M. A., and Schloss, P. D. (2019). The impact of DNA polymerase and number of rounds of amplification in PCR on 16S rRNA gene sequence data. Msphere 4:e00163-19. doi: 10.1128/mSphere.00163-19
Teske, A., Alm, E., Regan, J., Toze, S., Rittmann, B., and Stahl, D. (1994). Evolutionary relationships among ammonia-and nitrite-oxidizing bacteria. J. Bacteriol. 176, 6623–6630. doi: 10.1128/jb.176.21.6623-6630.1994
Tully, B. J., Graham, E. D., and Heidelberg, J. F. (2018). The reconstruction of 2,631 draft metagenome-assembled genomes from the global oceans. Scie. Data 5:170203.
Turner, S., Pryer, K. M., Miao, V. P., and Palmer, J. D. (1999). Investigating deep phylogenetic relationships among cyanobacteria and plastids by small subunit rRNA sequence analysis. J. Eukaryot. Microbiol. 46, 327–338. doi: 10.1111/j.1550-7408.1999.tb04612.x
Vishnivetskaya, T. A., Hu, H., Van Nostrand, J. D., Wymore, A. M., Xu, X., Qiu, G., et al. (2018). Microbial community structure with trends in methylation gene diversity and abundance in mercury-contaminated rice paddy soils in Guizhou. China. Environ. Sci. Process. Impacts 20, 673–685. doi: 10.1039/c7em00558j
von Wintzingerode, F., Göbel, U. B., and Stackebrandt, E. (1997). Determination of microbial diversity in environmental samples: pitfalls of PCR-based rRNA analysis. FEMS Microbiol. Rev. 21, 213–229. doi: 10.1111/j.1574-6976.1997.tb00351.x
Xu, J., Buck, M., Eklöf, K., Ahmed, O. O., Schaefer, J. K., Bishop, K., et al. (2019). Mercury methylating microbial communities of boreal forest soils. Sci. Rep. 9:518.
Yu, R.-Q., Reinfelder, J. R., Hines, M. E., and Barkay, T. (2013). Mercury methylation by the methanogen Methanospirillum hungatei. Appl. Environ. Microbiol. 79, 6325–6330. doi: 10.1128/AEM.01556-13
Keywords: amplicon sequencing, hgcAB, primer development, mercury methylation, environmental microbiology, microbial diversity
Citation: Gionfriddo CM, Wymore AM, Jones DS, Wilpiszeski RL, Lynes MM, Christensen GA, Soren A, Gilmour CC, Podar M and Elias DA (2020) An Improved hgcAB Primer Set and Direct High-Throughput Sequencing Expand Hg-Methylator Diversity in Nature. Front. Microbiol. 11:541554. doi: 10.3389/fmicb.2020.541554
Received: 16 March 2020; Accepted: 25 August 2020;
Published: 06 October 2020.
Edited by:
Julia W. Neilson, University of Arizona, United StatesReviewed by:
Stefan Bertilsson, Uppsala University, SwedenAditi Sengupta, Pacific Northwest National Laboratory (DOE), United States
Copyright © 2020 Gionfriddo, Wymore, Jones, Wilpiszeski, Lynes, Christensen, Soren, Gilmour, Podar and Elias. This is an open-access article distributed under the terms of the Creative Commons Attribution License (CC BY). The use, distribution or reproduction in other forums is permitted, provided the original author(s) and the copyright owner(s) are credited and that the original publication in this journal is cited, in accordance with accepted academic practice. No use, distribution or reproduction is permitted which does not comply with these terms.
*Correspondence: Caitlin M. Gionfriddo, Z2lvbmZyaWRkb2NtQG9ybmwuZ292; Dwayne A. Elias, ZWxpYXNkYUBvcm5sLmdvdg==
†Present address: Daniel S. Jones, New Mexico Institute of Mining and Technology, Socorro, NM, United States; Geoff A. Christensen, Department of Bioengineering, University of Illinois at Urbana–Champaign, Champaign, IL, United States