- 1School of Agriculture, Policy and Development, Department of Animal Sciences, University of Reading, Animal, Dairy and Food Chain Sciences, Reading, United Kingdom
- 2Beef and Sheep Research Centre, Scotland's Rural College (SRUC), Roslin Institute Building, Edinburgh, United Kingdom
- 3The Roslin Institute, University of Edinburgh, Edinburgh, United Kingdom
- 4Dairy Research and Innovation Centre, Scotland's Rural College (SRUC), Dumfries, United Kingdom
- 5Centre for Dairy Research, University of Reading, Reading, United Kingdom
Milk products are an important component of human diets, with beneficial effects for human health, but also one of the major sources of nutritionally undesirable saturated fatty acids (SFA). Recent discoveries showing the importance of the rumen microbiome on dairy cattle health, metabolism and performance highlight that milk composition, and potentially milk SFA content, may also be associated with microorganisms, their genes and their activities. Understanding these mechanisms can be used for the development of cost-effective strategies for the production of milk with less SFA. This work aimed to compare the rumen microbiome between cows producing milk with contrasting FA profile and identify potentially responsible metabolic-related microbial mechanisms. Forty eight Holstein dairy cows were fed the same total mixed ration under the same housing conditions. Milk and rumen fluid samples were collected from all cows for the analysis of fatty acid profiles (by gas chromatography), the abundances of rumen microbiome communities and genes (by whole-genome-shotgun metagenomics), and rumen metabolome (using 500 MHz nuclear magnetic resonance). The following groups: (i) 24 High-SFA (66.9–74.4% total FA) vs. 24 Low-SFA (60.2–66.6%% total FA) cows, and (ii) 8 extreme High-SFA (69.9–74.4% total FA) vs. 8 extreme Low-SFA (60.2–64.0% total FA) were compared. Rumen of cows producing milk with more SFA were characterized by higher abundances of the lactic acid bacteria Lactobacillus, Leuconostoc, and Weissella, the acetogenic Proteobacteria Acetobacter and Kozakia, Mycobacterium, two fungi (Cutaneotrichosporon and Cyphellophora), and at a lesser extent Methanobrevibacter and the protist Nannochloropsis. Cows carrying genes correlated with milk FA also had higher concentrations of butyrate, propionate and tyrosine and lower concentrations of xanthine and hypoxanthine in the rumen. Abundances of rumen microbial genes were able to explain between 76 and 94% on the variation of the most abundant milk FA. Metagenomics and metabolomics analyses highlighted that cows producing milk with contrasting FA profile under the same diet, also differ in their rumen metabolic activities in relation to adaptation to reduced rumen pH, carbohydrate fermentation, and protein synthesis and metabolism.
Introduction
In Western industrialized countries, human diets are generally characterized by saturated fatty acid (SFA) intakes which exceed nutritional recommendations, with milk and dairy products providing from 27 to 57% of all SFA intake in the UK (Givens, 2010). Excessive consumption of SFA has been associated with increased risk of cardiovascular diseases (CVD) in humans (Givens, 2010) whilst the source of fatty acids (FA) also has an effect on CVD risk [World Health Organization (WHO), 2003; Givens, 2010]. However, in addition to SFA, milk and dairy products provide a range of monounsatured FA (MUFA) and polyunsaturated FA (PUFA) that are beneficial for human health (Haug et al., 2007; Barcelo-Goblijn and Murphy, 2009; Swanson et al., 2012). Therefore, health organizations (FAO, 2008; EFSA Panel on Dietetic Products, 2010; Bates et al., 2014; SACN, 2018) and recent studies (Kliem and Shingfield, 2016; Nettleton et al., 2017) have urgently recommended the substitution of SFA in human diets, with cis-monounsaturated FA (cis-MUFA) and cis-polyunsaturated FA (cis-PUFA), in order to reduce the mortality from, and risk of, CVD (Givens, 2008). Given that milk fat is the largest single contributor of SFA in the UK (Bates et al., 2014), producing milk (a product widely consumed by all age groups) where part of its SFA has been replaced with cis-MUFA and/or cis-PUFA might be an effective means of contributing to the dietary targets, without requiring changes in consumer eating habits. Cow diets influence milk FA profile and provide opportunities to reduce milk SFA content, but proposed dietary interventions for the reduction of milk SFA content increase production costs [e.g., oil or oilseed supplementation (Stergiadis et al., 2014; Kliem and Shingfield, 2016)] or risk productivity of high-yielding cows [e.g., increased pasture intake (Chilliard et al., 2007; Ferris, 2007; Glasser et al., 2008; Stergiadis et al., 2012, 2015)]. As a result, these strategies are scarcely used in UK dairy systems and the % SFA in total FA in UK retail milk has changed little since 2006 [68.9% in 2016–2017 (Stergiadis et al., 2019); 70.7% in 2006–2007 (Butler et al., 2011)].
Milk fatty acid profiles generally present much higher concentrations of SFA and lower concentrations of PUFA than the FA profile in ruminant diets. This is partly due to the fat synthesis in the mammary gland, which produces a substantial amount of SFA with 4–16 atoms of carbon (Harfoot and Hazlewood, 1988). In addition, the presence of specific microbial species within the rumen microbiome perform lipolysis and subsequent biohydrogenation of the FA in the rumen (Lourenco et al., 2010); a process that result in C18:0 as the finite end product whilst producing a large amount of new unsaturated FA as intermediates (Jenkins et al., 2008; Ferlay et al., 2017). The rumen microbiome is a complex ecosystem, mainly composed of anaerobic bacteria and completed by archaea, ciliate protozoa and anaerobic fungi (Huws et al., 2013). These communities play different, but also interacting roles, which are essential for their maintenance and cooperative metabolic activities such as plant fiber degradation, carbohydrate fermentation, protein digestion, and synthesis of volatile fatty acids, amino acids, microbial protein and ammonia (Huws et al., 2013; Jami et al., 2014). The rumen microbiome is recognized for having an impact on animal performance traits such as health, methane emissions and feed efficiency (Russell, 1998; Jami et al., 2014; Auffret et al., 2017). In addition, there is large variation in phenotypic responses within ruminant breed and diet groups (Henderson et al., 2015; Roehe et al., 2016) but information explaining variation in milk FA profile, as well as the microbial communities and mechanisms behind such variation, are still, to our knowledge, limited. Buitenhuis et al. (2019) demonstrated the beneficial effect of adding rumen bacterial microbiome information to host genetic data for breeding purposes, and the impact on milk FA composition, but discussion on the potential mechanistic functions was not developed.
The overall aim of our work was to (i) compare the rumen microbiome between Holstein cows which, under the same diet, produce milk with contrasting FA profile, (ii) identify the rumen microbial taxa and genes impacting on individual FA and FA groups which are associated to human health, and (iii) identify metabolic-related microbial mechanisms controlling milk FA content. We hypothesized that differing microbial communities in the rumen of cows fed the same diet will influence the extent of rumen biohydrogenation and so potentially affect the milk FA profile. Given the overarching importance of diet in shaping rumen microbiome composition and its activity (Karisa et al., 2013; Petri et al., 2013; Rooke et al., 2014), we considered that combining metagenomics and metabolomics for studying the rumen fluid ecosystem provides an opportunity to identify microbial mechanisms associated with animals producing extreme contents of milk SFA (e.g., representing the lowest and the highest range). Both omic methodologies are considered complementary to better understand biological interaction networks and biological mechanisms associated with the variation in important traits in livestock production (Karisa et al., 2013; Pitta et al., 2016).
Materials and Methods
Ethics Approval
The animal experiment was conducted at the Centre for Dairy Research (CEDAR), University of Reading, UK; all experimental procedures used were licensed, regulated, and inspected by the UK Home Office under the Animals (Scientific Procedures) Act, 1996.
Animals, Experimental Design and, Samples Collection
Four hundred and fifty two Holstein lactating cows were housed in a purpose built cubicle yard with sand as bedding, and water supplied throughout the day via self-filling drinking troughs. Cows were in mid-lactation between days 90 and 210 in milk. They received the same total mixed ratio (at forage:concentrate ratio of 63:37 on a dry matter basis), throughout the study. Forage was comprised of 71% maize silage and 29% grass silage (on a dry matter basis). Concentrate feed was made of commercial pellet (blend), sodagrain (sodium hydroxide-treated wheat), Megalac (rumen-protected fat supplement; Volac Wilmar Feed Ingredients, Hertfordshire, UK) and salt. Nutrient composition, digestibility, metabolisable energy (ME) content, volatile compounds and fatty acid profiles are presented in Supplementary Table 1. Dry matter intake (DMI) was back-calculated from ME requirements (Agricultural Food Research Council, 1993; Thomas, 2004) and ME content of the diet. Milk yield was automatically recorded in the milking parlor. Initially, the whole herd was screened for milk composition and FA profiles by Fourier Transform InfraRed spectroscopy (FTIR; MilkoScanTM 7RM; FOSS, Denmark), at the National Milk Laboratories (Wolverhampton, UK); using a composite morning and afternoon milk sample per cow. Based on the FTIR results, two groups of cows were created to represent the lowest range (low-SFA, n = 24 cows, 60.2–66.6% total FA) and highest range (high-SFA, n = 24 cows, 66.9–74.4% total FA) of milk SFA contents within this population. After 5 days, milk samples were collected from these cows (n = 48) and sent for immediate analysis of fat and protein content by FTIR, whilst an aliquot was stored in −20°C until FA analysis by gas chromatography (GC). Simultaneously, ~50 mL of rumen liquid were taken from the same 48 cows by inserting a stomach tube (16 × 2,700 mm Equivet Stomach Tube, Jørgen Kruuse A/S, Langeskov, Denmark) nasally and aspirating manually. This liquid was filtered through two layers of muslin and 5 mL strained rumen fluid still containing small particles were mixed with 10 ml phosphate buffered saline containing glycerol (30% v/v). These samples were stored at −80°C between collection and analysis. This technique is a practical means to sample a large number of cows for subsequent microbial community profiling, gene abundance analysis, and metabolite profiling; as well as previously presented sample preparation and storage techniques (Claus et al., 2008; Wallace et al., 2014; Firkins and Yu, 2015; Snelling et al., 2019). The technique has shown high microbiome similarity between rumen fluid samples collected using stomach tube and rumen digesta collected at the abattoir (Wallace et al., 2014; Snelling et al., 2019). Results from the FA analysis by GC were used to classify animals in two ways for subsequent data analysis (i) 24 cows with the lowest SFA concentrations vs. 24 cows with the highest SFA concentrations (Supplementary Table 2) and (ii) 8 cows with the extreme-low SFA concentrations (60.2–64.0% total FA) vs. 8 cows with the extreme-high SFA concentrations (69.9–74.4% total FA) (Supplementary Table 3).
Milk Fatty Acid Profiles Analysis
Milk FA profiling was performed using GC coupled with flame ionization detection (Bruker 350 GC, Bruker, Germany) according to previously described methods of esterification and methylation (Viant, 2007), and techniques of peak identification and quantification (Chilliard et al., 2009; Kliem et al., 2013). A combined correction factor, to account for carbon deficiency in the response of flame ionization detector for FA methyl esters with 4–10 atoms of carbon was used (Ulberth et al., 1999). Milk FA were expressed as proportion of individual FA or FA group in total FA. In order to confirm that both experimental groups (24 vs. 24 and 8 vs. 8 separately) had significant differences for FA profile and potentially for other milk characteristics, one-way ANOVA with adjustment for multiple comparisons using the Bonferroni correction (SPSS Statistics 22, IBM, USA) applied.
Genomic Analysis
DNA was extracted from the rumen fluid samples; all 48 samples were prepared for sequencing. Illumina TruSeq libraries were prepared from genomic DNA and sequenced on a NovaSeq 6000 instrument by Edinburgh Genomics (Edinburgh, UK). Paired-end reads (2 × 150 bp) were generated, resulting in between 10 and 24 GB per sample (between 33 and 80 million paired reads). A metadata file indicating the correspondence between animal and metagenomics IDs as well as grouping information based on milk SFA is summarized in Supplementary Table 4. Analysis of the functional content of the metagenomic data by comparing to the KEGG database (http://www.kegg.jp) followed the same procedure as previously described in Wallace et al. (2015) and Roehe et al. (2016). Statistical analysis of the metagenomics samples was based on the complete sample profiles, as expressed by the pattern of metagenomic reads classified within KEGG ortholog groups with >90% similarity and belonging to a single KEGG ortholog (KO) groups and the relative abundance (percentage) of individual KO group in each profile to normalize the data between animals. The alignment of the reads generated by whole metagenomic sequencing to the KEGG genes database resulted in identification of 4,660 microbial genes for each animal. Microbial genes with a relative abundance >0.001% (n = 1,630) were carried forward for downstream analysis.
For taxonomic annotation, we constructed a custom database using Kraken (v.0.10.5) (Wood and Salzberg, 2014). The database consisted of 7,318 complete bacterial genomes, 229 fungal genomes, 585 archaeal genomes and 75 protozoan genomes (all from RefSeq; 09/2018) augmented with 410 genomes from the Hungate collection and recently published 913 RUG genomes (Stewart et al., 2018). This database was used to assign reads to entries in the NCBI taxonomy database at the level of Kingdom, Phylum, Family, and Genus. Results with zero counts in 3 or more of the 48 animals were removed from the analysis to avoid statistical limitations due to interferences in the study of correlations. Following this step, 1,630 genes, 42 phyla, and 849 genera were selected for the statistical analysis.
Statistical analysis of the metagenomic data was based on the complete sample profiles as expressed by the pattern of metagenomic variables (taxonomic level or genes) and the relative abundance (percentage) of individual variable in each profile. Unless otherwise stated, all parameters used were the default.
Taxonomic and gene abundances were compared individually or within a functional group (e.g., all genes associated with low SFA) using a general linear model with adjustment for multiple comparisons using the Bonferroni correction (SPSS Statistics 22, IBM, USA).
In order to identify the rumen microbial species and genes on individual FA and FA groups which are associated to human health (and in particular SFA), we used partial least squares (PLS) analysis (Version 9.1 for Windows, SAS Institute Inc., Cary, NC, USA) to identify the most important genes associated with the milk SFA content. For the metagenomics analysis, the PLS analysis accounts for multiple testing and the correlation between taxonomic levels or microbial genes as variables. The model selections were based on the variable importance for projection (VIP) criterion (Wold, 1995), whereby variables with a VIP <0.8 contribute little to the prediction. Importance of a particular taxon or gene identified by PLS analysis within 8 extreme-low or 8 extreme-high animals to explain variation in a specific milk fatty acid was also determined by heatmaps. In order to identify the microbial origin of the genes that influence the concentrations of milk SFA, we used partial least squares (PLS) analysis (Version 9.1 for Windows, SAS Institute Inc., Cary, NC, USA) to identify microbial genera mostly correlated with each specific gene.
Metabolomics Analysis of Rumen Fluids
A Bruker Avance HD 700 MHz (Bruker Biopsin, Rheinstetten, Germany) was used to acquire the NMR spectra from the ruminal biofluids (Saleem et al., 2013). The NMR was equipped with a TCI CryoProbes from the same manufacturer and was operating at 7,000 Hz. 1H NMR spectra was obtained using a standard 1D noesypr1d pulse sequences with a total acquisition time of 3.34 s with noesypr1d 90° pulse length of 7.7 μs (Meiboom and Gill, 1958; Aue et al., 1975). Water pre-saturation was applied for 3 s. The mixing time was set up at 50 or 100 ms. In total 64 scans per sample were accumulated into 64 K data points.
For the metabolomics analysis, all spectra were pre-processed in MestReNova version 11.0.2–18,153 (Mestrelab Research S.L., Spain) where it was phased and baseline corrected. The algorithm used for the baseline correction was Whittaker smoother; multipoint baseline correction was used if necessary (Dieterle et al., 2006). TSP (3-(trimethylsilyl)-propionic acid-d4; δ 0.00) was used for the calibration of all the samples. Residual water (δ 4.70–5.10) and noise regions (spectra before δ 0.5 and after δ 9.5) were removed in MestReNova before importing the data into MATLAB. Version R2015b of MATLAB (Mathworks, UK) equipped with Korrigan Toolbox version 0.1 (Korrigan Sciences Ltd., U.K.) was used to carry out the data analysis. Relationships between milk FA in the rumen and milk were evaluated from the loading plots of Principal Component Analysis (PCA) using Gen-Stat 16th edition (VSN International Ltd., UK). In order to correlate microbial genes with specific rumen metabolites and explain their metabolic mechanisms that have a positive effect on milk FA profile, the statistical approach started with a preliminary unsupervised Principal Component Analysis to evaluate the variability of the data. This was followed by a supervised pairwise Orthogonal Projection to Latent Structures Discriminant Analysis (O-PLS DA) (Cloarec et al., 2005; Bylesjö et al., 2006). This algorithm allowed the identification of specific modulations produced by metabolic changes associated with the relative abundance of specific genes previously identified as important to explain a trait. Models were evaluated for coefficient of determination (R2Y value) and goodness of prediction (Q2Y value, calculated using 7-fold cross-validation). The best models (R2 close to 1, Q2 positive and small overfit between R2 and Q2) were included in a second step of the pipeline where a selection of the samples with the lowest and highest concentration of the gene (n = 16) were used to identify metabolomics changes associated with the gene, used as predictor in each case. Metabolomic annotation was carried out using publicly available literature (Saleem et al., 2013), Chenomx NMR Suite 8.2 from Chenomx Inc (Edmonton, Canada) and online publicly available databases such as the Biological Magnetic Resonance data bank (BMRB, http://www.bmrb.wisc.edu) and the Bovine Metabolome Database (http://www.cowmetdb.ca/cgi-bin/browse.cgi).
Results
Variation in Animal Performance and Milk Fatty Acids
Dry matter intake was slightly higher (ANOVA, P = 0.042) for High-SFA cows than Low-SFA cows (Supplementary Figure 1A; 24 vs. 24 cows). This result was more pronounced with the 8 vs. 8 extreme cows (P = 0.013; results not shown).
The total SFA content in milk, produced animals in the 24 v 24 dataset, was 66.9 ± 3.06% whilst cis-MUFA and cis-PUFA represented 25.5 ± 2.30% and 3.0 ± 0.64%, respectively (Supplementary Table 2). The total SFA content in milk, produced by the 16 animals in the 8 vs. 8 dataset, was 66.9 ± 4.92% whilst cis-MUFA and cis-PUFA represented 25.5 ± 3.64% and 2.8 ± 0.87%, respectively (Supplementary Table 3). The cis n-3, which were represented by 72% α-linoleic acid (ALNA, C18:3 c9, c12, c15) across the data, were 0.63 ± 0.08% of total FA for the 48 animals dataset and 0.62 ± 0.09% of total FA for the extremes 16 animals dataset (Supplementary Tables 2, 3, respectively). A principal component analysis to elucidate metabolic relationships between rumen and milk fatty acids (Supplementary Figure 2, Supplementary Tables 2, 5) showed in general a similar grouping of each fatty acid groups except for cis-MUFA. This difference was mainly explained by the first axis explaining >99% of variability. The most abundant individual SFA (C12:0, C14:0, and C16:0) were found in higher concentrations (P < 0.01) in milk from the High-SFA than from Low-SFA cows (Figure 1). Unsaturated fatty acids (UFA) were more abundant for Low-SFA than High-SFA cows (P < 0.01). ALNA and n-3 were both higher for Low-SFA milk whilst the relative difference in n-3 was smaller (P < 0.05). The transfer of linoleic acids (LA, C18:2 c9, c12), but not ALNA, from diet to milk was 1.19-fold higher in Low-SFA compared with High-SFA cows (Supplementary Figure 1B). This transfer difference was stronger in extreme animals with the transfer of dietary LA 1.49-fold higher in Low-SFA compared with High-SFA cows. There was no significant difference in parity or milk yield between the Low-SFA and High-SFA groups (Supplementary Figures 1A,C) or when comparing the extreme cows. Furthermore, there was no significant difference in stage of lactation between the Low-SFA (166 ± 37 days) and High-SFA (153 ± 34 days) groups.
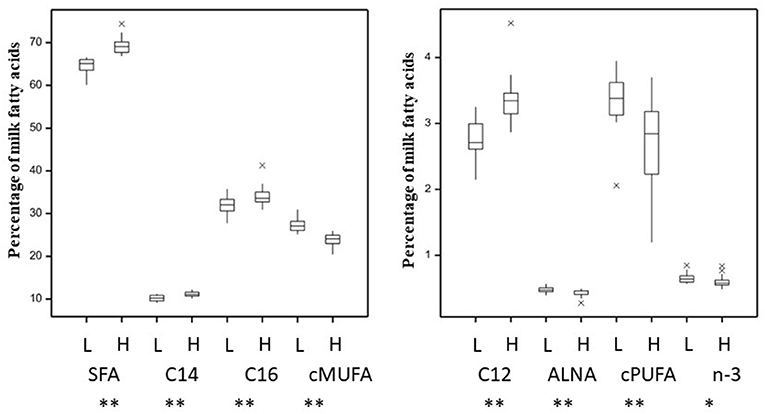
Figure 1. Variation in milk saturated (SFA, C12, C14, C16) and unsaturated (cis-MUFA, cis-PUFA, ALNA, and n-3) fatty acids (as a percentage of total fatty acids) for 48 animals grouped between Low-(L) and High-(H) milk SFA. *P < 0.05, **P < 0.01.
Variation in the Abundance of Rumen Microbial Communities in Cows With Contrasting Milk SFA Content
The rumen microbial communities were mainly composed of Firmicutes, Bacteroidetes and Proteobacteria corresponding on average to 61, 22, and 4%, respectively. Euryarchaeota, mostly composed of methanogens, represented about 3% of the total community. At the phylum level, no significant differences were observed between Low-SFA and High-SFA groups for the 48 animals (Supplementary Figure 3A) even when the comparison was performed in the extreme SFA groups (Supplementary Figure 3B). The Firmicutes-to-Bacteroidetes ratio was not correlated (P > 0.1) with milk FA content.
Comparison of microbial population relative abundances differed (ANOVA, P < 0.05) between SFA groups using the 48 (n = 37) and extreme animals with 37 and 18 genera, respectively, and showing a limited number of shared genera (n = 5) mostly within Actinobacteria (4/5), Denitrobacterium and the Firmicutes Jeotgalibacillus sp. (Table 1). Genera that were more abundant (P < 0.05) in High-SFA animals, within the 48 animals, included low abundant Actinobacteria (<0.01%; n = 12) and other bacteria and archaea. In addition, three methanogens (Methanobrevibacter, Methanobacterium and Methanothermus sp.), and two highly abundant genera (>0.01%), the protist Nannochloropsis and the Proteobacteria Burkholderia showed higher abundance (P < 0.05) in High-SFA animals (Table 1). Furthermore, 13 genera were more abundant (P < 0.05) in High-SFA animals, but only when the 16 extreme cows were compared, and were composed of 3 Actinobacteria including Mycobacterium, 3 lactic acid bacteria (LAB) within Firmicutes (Lactobacillus, Leuconostoc and Weissella), 3 Proteobacteria (Kozakia, Hafnia and Komagataeibacter) and fungi (5/13) such as Cutaneotrichosporon and Cyphellophora (Table 1). Megasphaera and Selenomas genera known to contain lactic acid-degrading species were not significantly different in any of the comparisons.
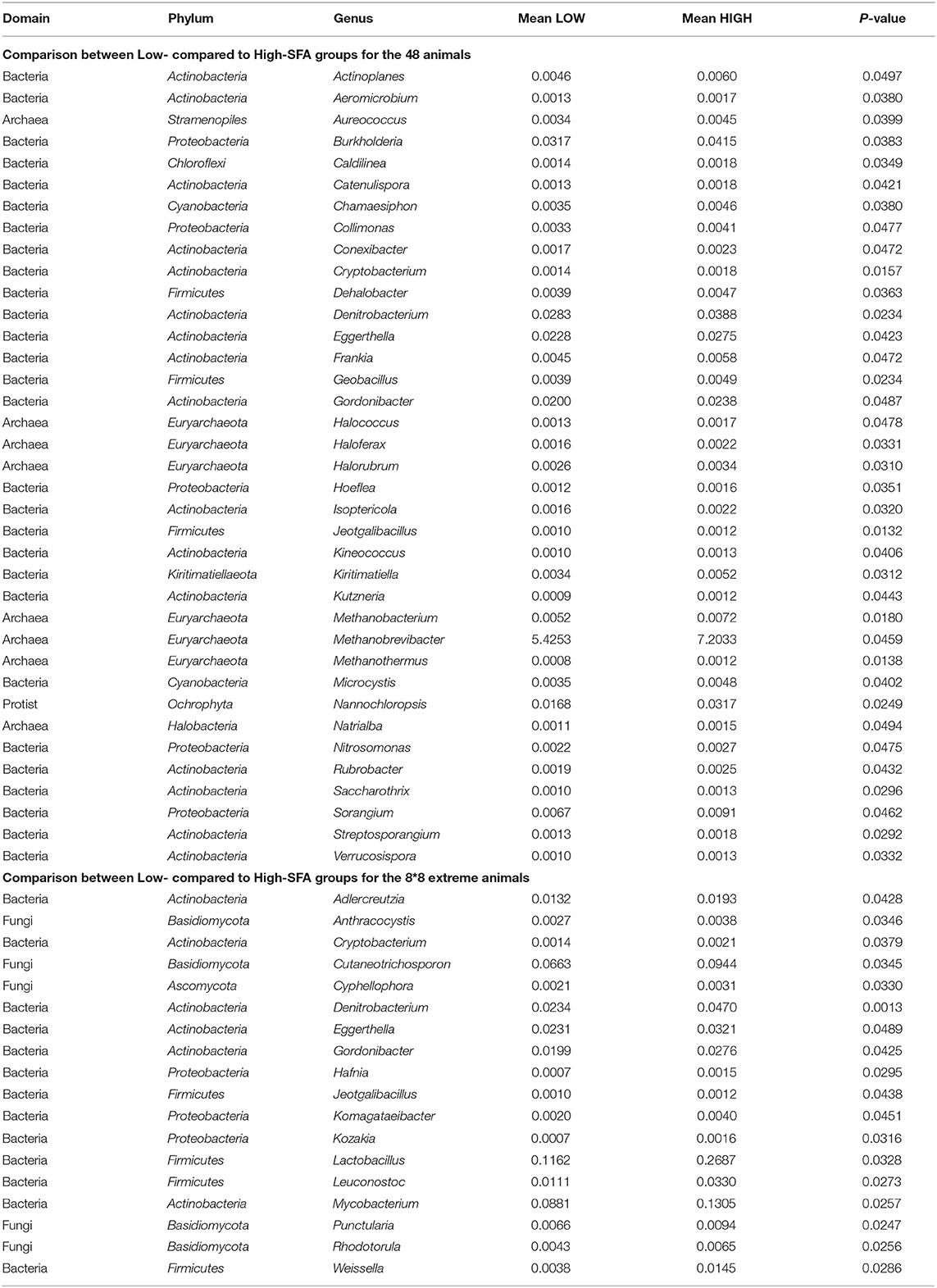
Table 1. ANOVA (Bonferroni corrected) results for genera significantly different between Low- compared to High-SFA groups (based on all 48 animals).
Correlations Between Microbial Communities and Milk Fatty Acid Composition
Microbial genera explaining most of the variability (between 64 and 86%) for a particular group of FA was determined using PLS analysis (VIP > 0.8). Different profiles of genera were identified within SFA (total SFA, C12:0, C14:0 and C16:0) and UFA groups (ALNA, cis-MUFA, cis-PUFA and n-3) based on PLS coefficients with one main branch grouping saturated FA and the second branch unsaturated FA together (Figure 2A, Supplementary Figure 4, Supplementary Table 6). Within 69 genera identified as being important to explain the variability observed for FA groups, 9 genera including Dehalococcoides, Denitrobacterium, Desulfobacula, Lactobacillus, Leuconostoc, Nannochloropsis, Punctularia, Rhodotorula, and Weissella were all significantly positively correlated (positive PLS coefficient) with SFA groups and negatively correlated (negative PLS coefficient) with unsaturated FA groups (Figure 2A, Supplementary Figure 4, Supplementary Table 6). Dehalococcoides, Desulfobacula, Punctularia, and Rhodotorula were strongly positively correlated with C16:0 and in some cases with SFA whilst Denitrobacterium, and the LAB Lactobacillus, Leuconostoc, and Weissella were strongly positively correlated with C12:0, C14:0 and total SFA. Dehalococcoides, Denitrobacterium, and Rhodotorula were all negatively correlated with the unsaturated FA studied. The LAB Leuconostoc and Weissella were all negatively correlated with cis-MUFA and cis-PUFA whilst Lactobacillus only showed a negative correlation with cis-MUFA. The protist Nannochloropsis showed negative correlations with cis-MUFA and n-3 including ALNA, but a strong positive correlation with SFA (Figure 2A, Supplementary Figure 4, Supplementary Table 6).
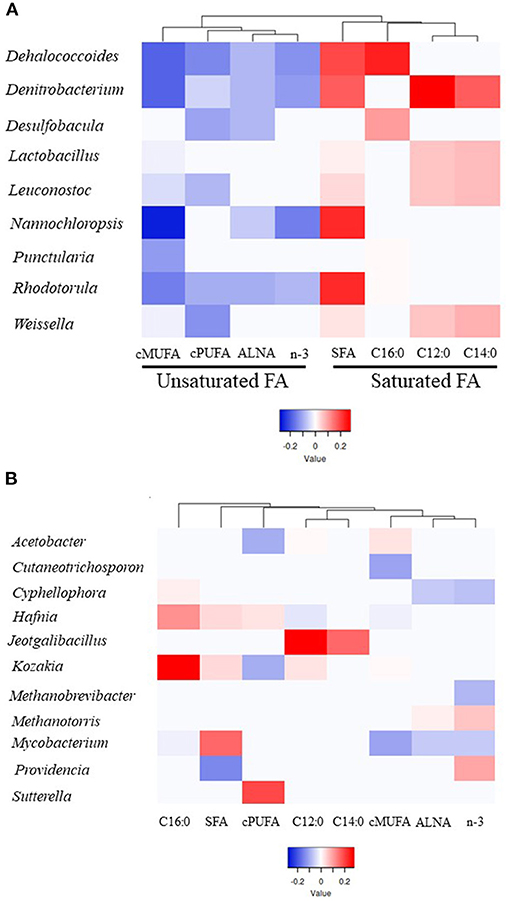
Figure 2. Heatmap of the microbial genera indicating their partial least square coefficient for each fatty acid. (A) Unsaturated or saturated FA groups correlated with a particular genus. (B) Individual FA groups correlated with a particular genus. Blue color indicates a negative PLS coefficient between the genus and the fatty acid, whilst red indicates the opposite.
Individual FA groups were correlated with a particular genus (Figure 2B, Supplementary Figure 4, Supplementary Table 6), such as Providencia (positive with n-3 and negative with SFA), Jeotgalibacillus (positive with C12:0 and C14:0), Kozakia (positive with C16:0 and negative with cis-PUFA), Cutaneotrichosphoron (negative with cis-MUFA), Cyphellophora (negative with ALNA and n-3 and positive with C16:0), and Sutterella (positive with cis-PUFA). Hafnia and Acetobacter genera showed contrasting correlation results within SFA or unsaturated FA. For example, Hafnia was positively correlated with C16:0 and cis-PUFA and negatively correlated with cis-MUFA. The two methanogen genera Methanobrevibacter and Methanotorris were negatively and positively correlated with n-3, respectively. Although Mycobacterium showed a positive correlation with SFA, this genus was negatively correlated with C16:0, n-3, ALNA, and cis-MUFA.
Variation in the Abundance of Rumen Microbial Genes in Cows With Contrasting Milk SFA Content
From the 1,630 microbial genes with a relative abundance above 0.001%, 273 and 40 genes were significantly different (P < 0.05) between Low-SFA and High-SFA animals (48 animals) and also between extreme–low SFA and extreme-high SFA animals (16 animals), respectively (Supplementary Table 7). Within these two sets of genes, only 19 genes were shared between the 48 or the extreme animals with a majority of genes involved in translation, ribosomal structure and biogenesis (n = 6) or energy production and conversion (n = 4) but none directly related to FA biohydrogenation. Genes involved in methanogenesis, and encoding for formylmethanofuran dehydrogenase (K00200-204), methyl-coenzyme M reductase (K00399-402) and tetrahydromethanopterin S-methyltransferase (K00577, K00580, K00581, and K00584) were only significantly different when using the 48 animals.
In term of general functions (COG database), the enriched functions related to higher relative abundance of genes in extremes–low SFA compared to extreme-high SFA (Supplementary Figure 5), were carbohydrate transport and metabolism, intracellular trafficking, secretion, and vesicular transport and nucleotide transport and metabolism; mostly because of the genes involved in starch degradation (K00688), preprotein translocase synthesis (K03070), and genes encoding for adenylosuccinate synthase (K01939) and associated with purine metabolism. Although the general function group translation, ribosomal structure and biogenesis was enriched in extreme-low SFA animals, contrasting results were obtained depending on the genes constituting this group. For example, genes encoding for seryl-tRNA synthetase and GTP-binding protein LepA were significantly more abundant in extreme-low SFA animals and had a relative abundance above 0.1% whilst less abundant genes (<0.05%) were significantly more abundant in extreme-high-SFA animals and encoding for ribosomal subunit (K02956).
A total of 39 genes were highly correlated with FA groups (VIP > 0.8), explaining from 76% (C14:0) to 94% (C16:0) of the variability in milk FA by PLS analysis (Supplementary Table 8), and were associated with different pattern of responses as showed in the heatmap (Figure 3, Supplementary Figure 6). Contrasting with microbial genera, cis-PUFA did not group in the same main branch as other UFA. The genes which were significantly correlated with cis-PUFA by PLS were different for all FA tested and the gene with the highest VIP-value (>1.25) was involved in intracellular sulfate transport (K06020).
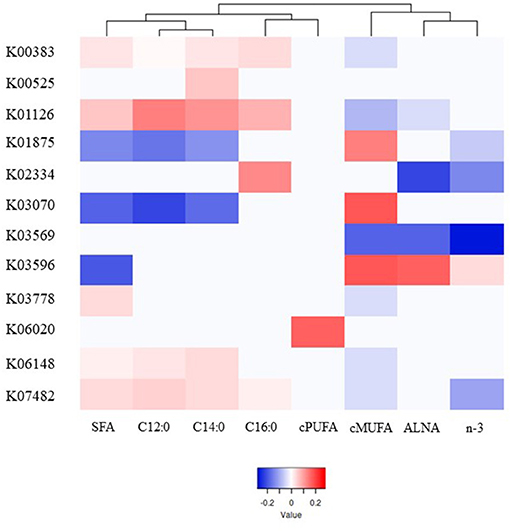
Figure 3. Heatmap of the most significant microbial genes indicating their partial least square coefficient for each fatty acid. Blue color indicates a negative PLS coefficient between the genus and the fatty acid, whilst red color indicates the opposite.
Within the 39 genes, the 8 genes which were positively correlated with SFA, and in general negatively correlated with unsaturated FA, were associated with stress resistance (K00383 and K07482), lactate metabolism (K03778), adaptation to new environments (K01126 and K06148), and cell division (K00525, K02334, and K03569). On the other hand, 3 other genes which are involved in no stress responses (K03070 and K03596) and protein synthesis (K01875) were strongly positively correlated mostly with cis-MUFA.
Links Between the Rumen Microbial Genes and Rumen Metabolome
In total, rumen microbial genes which were significantly correlated to milk SFA concentrations were used to compare the metabolome of cows that they had high vs. low (8 vs. 8) abundance of the gene (Figures 4A–E). The best models were obtained for the genes K01126 (R2Y = 0.74, Q2Y = 0.63) and K03778 (R2Y = 0.74, Q2Yc 0.71) and K06148 (R2Y = 0.69, Q2Y = 0.54). In all cases, higher levels of the gene were associated principally with butyrate, propionate, tyrosine and NADP+ production. Conversely, these individuals had lower levels of hypoxanthine and xanthine in the rumen fluid (see Figures 4A–C). The model produced by the gene K00383 showed the same pattern as the genes described above, but in this case the model was not as strong (R2Y = 0.60, Q2Y = 0.39, Figure 4D). Interestingly, higher levels of the gene K00525 were associated with high propionate, tyrosine and NADP+, but lower levels of butyrate (Figure 4E). However, this model was even weaker (R2Y = 0.55, Q2Y = 0.39).
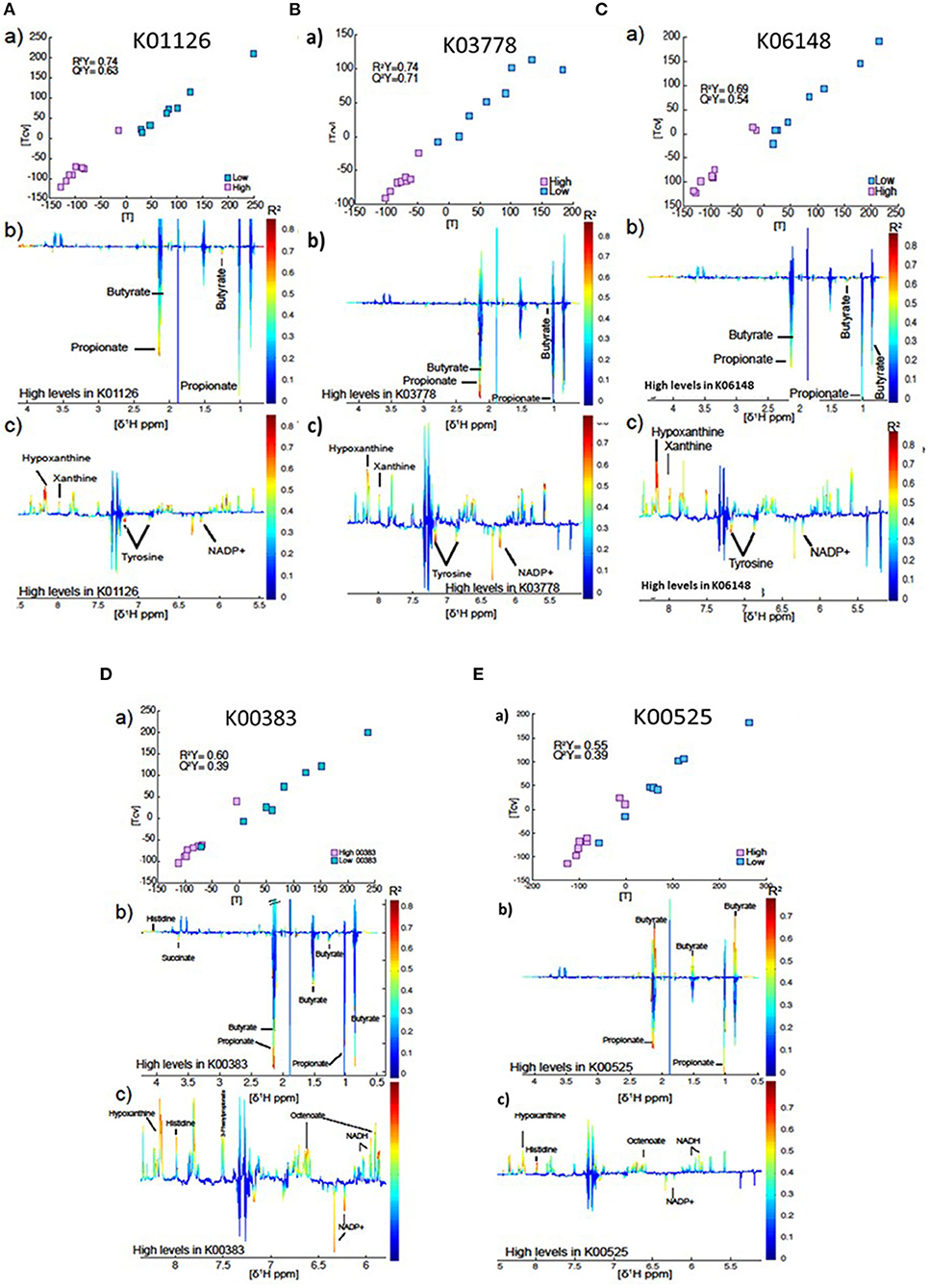
Figure 4. (a) Plot of the scores against the cross validated scores generated from the O-PLS DA model calculated using 16 samples of the spectra as a matrix of independent variables and high vs. low gene index as predictor. (b,c) Aliphatic and aromatic regions of ruminal fluid spectra showing metabolomics differences between groups with high and low levels of the genes K01126 (A), K03778 (B), K06148 (C), K00383 (D), and K00525 (E).
Microorganisms strongly correlated with these 5 genes (VIP > 0.8) were mostly composed of the 3 LAB (Lactobacillus, Leuconostoc, and Weissella), as well as acetogenic bacteria such as Acetobacter and Kozakia, and to a lesser extend Pediococcus (LAB), Komagataeibacter (Acetogen), Hafnia (Phytate degrader) and other genera (Babesia, Bacillus, and Oribacterium). These genera explained between 67 and 88% of the variability of each gene using PLS analysis (Table 2) and the presence of these genes was also confirmed in their genome.
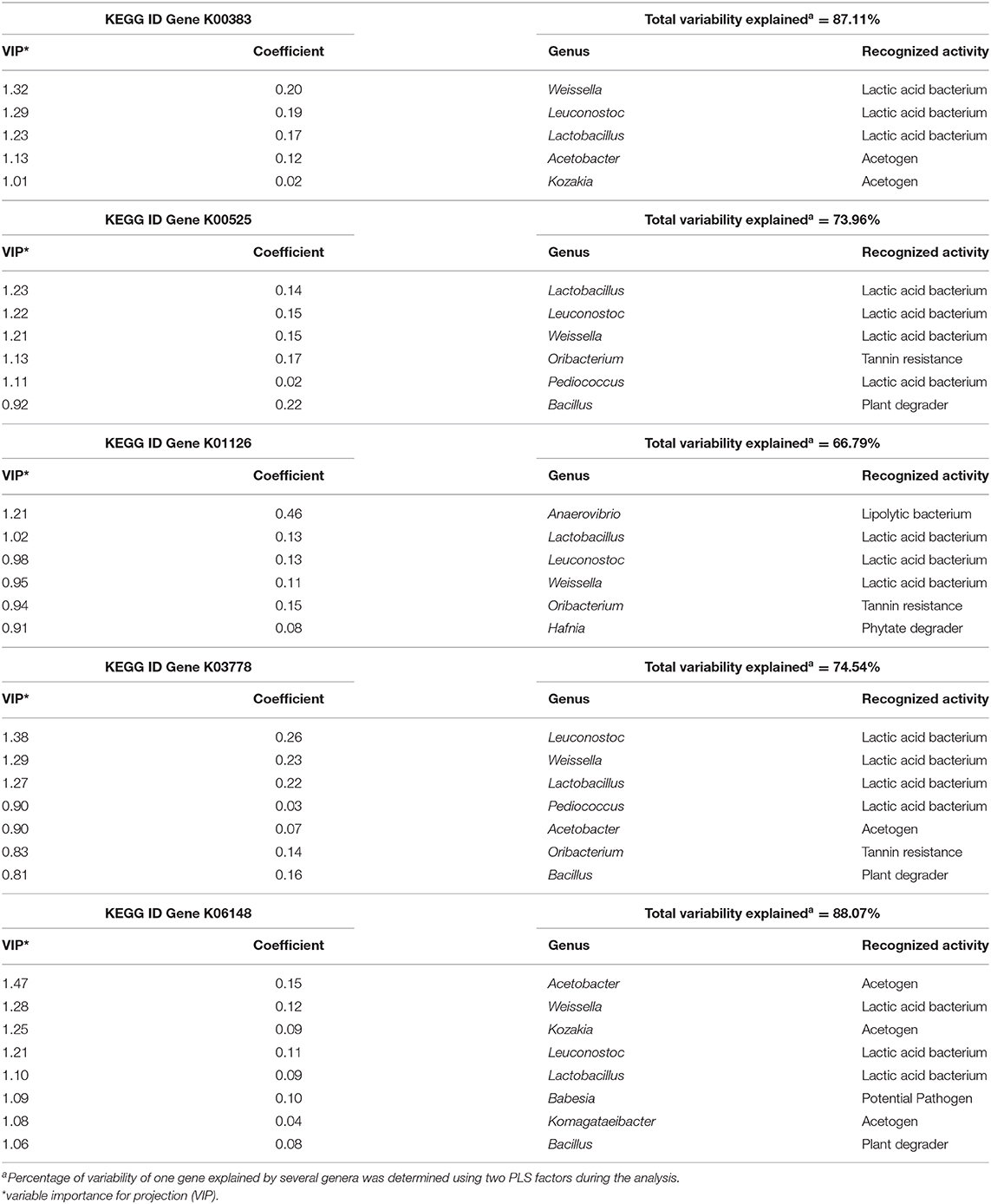
Table 2. Partial Least Square results identifying the most correlated genera with one of the genes identified as of interest explaining metabolomics results (K00383, K00525, K01126, K03778, and K06148).
Discussion
The present study is the first, to our knowledge, to describe rumen microbial communities and genes and their associated mechanisms (using metagenomics), as well as the abundance of rumen metabolites in cows carrying these genes (metabolomics), which explain variation in the main milk SFA and UFA, using data and samples from 48 dairy cows. The present work also increases the number of rumen microbiome and metagenome results for dairy cattle and provides further information on possible mechanisms impacting milk FA profile (Saleem et al., 2012; Pitta et al., 2016; Golder et al., 2018). Milk FA originate by direct transfer from the diet, de novo synthesis in the mammary gland (using VFA produced in the rumen as substrate), rumen microbial biohydrogenation of dietary FA, desaturase enzyme activity in the mammary gland, and release from body fat stores (when the cow is in negative energy balance) (Chilliard et al., 2007). Although this manuscript mainly focused on understanding the importance of the rumen microbiome to explain variation in milk FA profile in dairy cattle, host parameters such as host genetic variation, fat mobilization, volatile fatty acids (VFA) absorption across the rumen wall or mammary gland activities are also important to explain the variability on milk fat profile (e.g., cis-MUFA) (Bielak et al., 2016; Ibeagha-Awemu et al., 2016). However, the impact of the rumen bacterial community on odd chain FA and PUFA with 18 carbon atoms in milk was previously confirmed, and it was notably a better predictor than host genotype information in Holstein cattle (Buitenhuis et al., 2019). As further rumen metagenomic datasets become available, it is likely that the predictive power of such analyses will increase and the role of rumen microbiome and their genes on milk FA profile will further unravel (Stewart et al., 2019).
The Relation Between Rumen Microbiome Communities and Milk Fatty Acid Profile
A number of rumen microorganisms, have been found at higher abundance in the rumen of cows that produced milk with higher concentrations of SFA and were also positively correlated to SFA, C12:0, C14:0, or C16:0 and/or negatively correlated to cis-MUFA, cis-PUFA or n-3 PUFA. These were mainly LAB (Lactobacillus, Leuconoctoc, and Weissella), Proteobacteria (Acetobacter and Kozakia) and Actinobacteria (Mycobacterium), fungi (Cutaneotrichosporon, Cyphellophora), and to a lesser extent (e.g., significant when the 48 cows were used in the analysis but not when the extreme 16 cows were used) protists (Nannochloropsis), and methanogens (Methanobrevibacter).
The abundance of LAB, especially Lactobacillus, is higher in animals fed high-grain diets, and associated with lower rumen pH, because of the intake of rapidly fermentable carbohydrates, such as starch (Oetzel, 2003; Cremonesi et al., 2018). LAB are natural inhabitants of the rumen (Oetzel, 2003; Chen et al., 2012) and in this study were more abundant in extreme High-SFA animals (showing positive and negative correlations on SFA and unsaturated FA, respectively). This may reveal a relationship between slight changes in rumen pH and microbiome adaptation to lower pH, which further impacts milk SFA profile; as microorganisms which explain most of the variation in milk SFA profile are also those which are favored by lower rumen pH. It is however still unclear what is the extend of pH reductions that can trigger such microbiome responses. Lactobacillus species and other LAB are currently proposed as probiotics by Pophaly et al. (2012) to enhance gastrointestinal health in dairy cattle (with contrasting effectiveness between studies) and further studies may be necessary to identify beneficial LAB, that improve gastrointestinal health but also maintain rumen pH homeostasis and milk nutritional quality (Davidson et al., 2008).
Proteobacteria (in particular Acetobacter and Kozakia), which were found at higher concentrations in the rumen of cows producing High-SFA milk, are known to be involved in acetate production (Kersters et al., 2006). Fungi are known to improve fiber fermentation of the feed mainly by complementing mastication effects to break down feed particles and open up the structure (Akin and Borneman, 1990), whilst fermentation of fibrous material (e.g., forage) enhances the synthesis of acetate, at expense of propionate in the rumen (McDonald et al., 2011). It is therefore likely that the higher abundance of acetate-producing bacteria, and also potentially to a lesser extent, fiber-fermenting fungi, increase acetate synthesis, absorption and transfer to mammary gland. Acetate is extensively used by the mammary gland for the de novo synthesis of milk fat via the cytosolic malonyl-CoA pathway, while the main source of acetate in the mammary gland is the acetate which is produced in the rumen and subsequently absorbed and circulated in blood (McDonald et al., 2011). Given that de novo fat synthesis in the mammary gland includes solely SFA (all milk SFA from C4:0-C14:0 and 50% of milk C16:0 concentrations) (Chilliard et al., 2007), it is likely that higher supply of acetate would increase milk SFA synthesis and secretion to milk.
Previous work has shown that methanogens may enhance the ability of fungi to ferment fiber and well shift electron use toward the transformation of lactate and ethanol to methane via hydrogen; which is in turn converts to methane and carbon dioxide (Mountfort et al., 1982); while an overall increase in acetate abundance in the rumen may also be enhanced by such increase in fiber fermentation process. In the present study, methanogens were correlated with high concentrations of milk SFA when the 24 high-SFA vs. 24 low-SFA cows were compared. This may reveal a complementary role that methanogens play in increasing synthesis of acetate, as shown above for Proteobacteria and fungi, and potentially also adapting to slight reductions in rumen pH, as shown above for LAB; which further supports the suggestion that milk SFA content relates to such microbial activities.
In addition, the fungi, Cutaneotrichosporon (more abundant in the rumen of cows producing less cis-MUFA) is a lipase producing yeast (Kuncharoen et al., 2020), which may therefore enhance the hydrolysation of ester linkages in lipids causing the breakdown of triacylglycerols into glycerol and free fatty acids. This process will subsequently increase the availability of free fatty acids for ruminal biohydrogenation, the transformation cis-PUFA to a great range of trans-MUFA and trans-PUFA, and the saturated FA C18:0, and eventually reduce the flow of cis-UFA in the small intestine for absorption (Jenkins et al., 2008). The higher concentrations of Nannochloropsis in the rumens of cows that produced High-SFA milk (when the 24 high-SFA vs. 24 low-SFA cows were compared) was rather surprising given that Nannochloropsis is known to synthesize and store high concentrations of long chain n-3 PUFA in their triacylglycerol (Ma et al., 2016). It is likely that the negative correlation with milk n-3 is a result of the increased production of pyruvate, via the carbohydrate catabolic activity of Nannochloropsis (Li et al., 2014), which is the source of VFA (including acetate) in the rumen.
As previously shown in beef cattle, a stress on the rumen microbiome, caused by low pH, also increases the relative abundance of pathogens (Auffret et al., 2017). In this study, abundances of Mycobacterium were significantly more abundant in High-SFA animals and several species within the Mycobacterium genus are obligatory or opportunistic human and animal pathogens (Rastogi et al., 2001). Therefore, cows producing milk with more SFA in this study were also increasingly exposed to internal pathogens; although cows in this work did not experience any health incidences, this finding highlights that health benefits (e.g., reduced risk of disease) and improvement of milk nutritional quality may be related.
The Relation Between Rumen Microbial Genes and Milk Fatty Acid Profile
The findings in the present study support a strong correlation between rumen microbial genes and FA profile, as the composition of microbial genes explained from 76 to 94% of the variation in certain individual FA and FA groups. In this study, K00688, a gene involved in starch degradation was more abundant in extreme High-SFA animals (positive and negative effects on SFA and unsaturated FA, respectively). In addition, microbial genes explaining most of the variability in milk FA, included those associated with lactic acid metabolism (K03778), and genes related to stress resistance and environmental adaptation by the synthesis of glutathione (K00383), which were mainly carried in LAB. This was coupled with the higher abundance of the cofactor NADPH/NADP+ (which was also identified by metabolomics as significantly higher in cows carrying more of these genes), and cell wall modification (K01126) or ABC transporter (K06148), which are important for LAB survival in lower pH environments (Pophaly et al., 2012; Zehavi et al., 2018). Therefore, the findings further support that microbial genes that may increase the synthesis of lactate in the rumen as well as enhance the adaptation of rumen microbiome in reduced pH conditions are also highly associated with higher concentrations of SFA in milk. This is potentially due to the subsequently increased de novo synthesis of SFA in the mammary gland by using the additional acetate (Chilliard et al., 2007), as described above.
As an outcome of the potential pH adaptation and effective lactate metabolism by rumen microbes, the concentrations of lactic acid were not significantly different between animals that had high concentrations vs. low abundances of these genes. The main explanation may be that lactate is easily metabolized by the rumen microbiome to produce propionate during the digestion of carbohydrates; a process which is also positively correlated with rumen pH (Plaizier et al., 2008; Saleem et al., 2012). Similarly, acetate concentrations in the rumen were not significantly different between animals with extreme abundances in these genes, and this can be partly explained by the fact that the two acetogenic bacteria, Acetobacter, and Kozakia, were positively correlated with the contrasting groups of FA (SFA and cis-MUFA, respectively); although the mechanisms controlling acetate concentration in the rumen, an important source for milk FA synthesis, are still unclear (McDonald et al., 2011).
Although based on the discussion above, it would be expected that acetate concentrations would be higher in the rumen of cows that produced High-SFA milk or carrying more of the correlated microbial genes, this was not observed. However, there is generally a lack of significant relationship between acetate concentrations in rumen fluid and plasma (McDonald et al., 2011), thus revealing that higher concentrations in plasma and mammary gland (that would increase milk SFA synthesis) do not necessarily reflect higher concentrations in rumen fluid. In addition, the rumen microbial genes associated with High-SFA milk were also correlated with increased concentrations of butyrate and propionate, rather than acetate. Their higher concentrations further support the suggestion that cows producing High-SFA milk may undergo higher rates of carbohydrate fermentation, because butyrate and propionate also represent, together with acetate, the major end products of rumen carbohydrate digestion (McDonald et al., 2011). However, the extent of metabolism of acetate during absorption via the rumen wall is lower than that of butyrate and propionate (Bergman and Wolff, 1971; Kristensen et al., 2000) and therefore it is likely that the relative proportions of these VFA in the rumen does not necessarily reflect the relative proportions of these VFA in the blood and mammary gland.
Interestingly, genes identified as important to explain milk SFA variation were associated with lactic acid metabolism, environmental adaptation and stress resistance but not FA biohydrogenation. Ventto et al. (2017) suggested that milk FA profile was under the control of multiple mechanisms in the rumen and the mammary gland and contributing to the regulation of fat synthesis. All milk SFA with carbon chains between C4-14, and 50% of C16:0, originate from de novo synthesis in the mammary gland using VFA as a substrate, while stearic acid, the end-product of rumen hydrogenation of dietary lipids, would partly convert to oleic acid in the mammary gland under the influence of Δ9 desaturase (Chilliard et al., 2007). It therefore seems that rumen hydrogenation, which is expected to play vital role in the concentrations of unsaturated fatty acids which are direct intermediate products of it (Chilliard et al., 2007), is not necessarily the most influential pathway for changes in milk SFA content as their synthesis are largely dictated by the activity in the mammary gland, and the supply of VFA substrates in the mammary gland. It appears that the effort of the microbial population to adapt to lower pH conditions in cows consuming the same diet, relates with the abundance of microbial communities and some of their genes responsible for such adaptation; many of which are also associated with milk SFA content. This demonstrates a potential indirect effect of rumen pH on specific microbial taxa adapted to environments with lower pH and impacting through their metabolism on rumen metabolites, lipolysis and eventually milk SFA content. The same genes which were associated with butyrate, propionate and the purine metabolites xanthine and hypoxanthine were more abundant in cows that produced milk with lower concentrations of SFA and higher concentrations of cis-MUFA.
Although challenging to speculate on any metabolic associations based on the findings of the present work, xanthine and hypoxanthine are known to be of microbial origin and their higher concentrations in the rumen also represent activities related to adaptation mechanisms to rumen pH changes (Saleem et al., 2012). The potential role of these metabolites to support rumen pH homeostasis should be investigated in the future. In addition, the fact that that microbial genes correlated with higher concentrations of milk SFA were also negatively correlated with rumen concentrations of hypoxanthine and xanthine [related to protein metabolism, e.g., protein synthesis and conversion of inosinic acid to uric acid; (McDonald et al., 2011)], may indicate an indirect association between rumen protein metabolism and milk fatty acid profiles; which may be explored further in future.
Tyrosine and propionate (which are contributing to milk and protein synthesis) were identified by metabolomics as highly correlated with microbial genes which were abundant in High-SFA animals, but milk yield between Low-SFA and High-SFA animals was similar. Differences in microbial and liver metabolisms related to tyrosine and propionate could explain this lack of effect on milk yield, in contrast with previous results (Rae and Ingalls, 1984; Xue et al., 2018) but further work is needed to clarify these mechanisms.
To conclude, the present study revealed that some microbial taxa, genes and their activities within the rumen microbiome differ substantially between cows which, under the same diet and housing conditions, produce milk at the high range (66.9–74.4% total FA; 24 cows) than in the low range (60.2–66.6% total FA; 24 cows) of the nutritionally undesirable SFA concentrations; while simultaneously show higher concentrations of the nutritionally beneficial cis-MUFA, cis-PUFA and/or n-3 PUFA. Rumens of cows producing milk with more SFA were characterized by higher abundances of several lactic acid bacteria and acetogenic bacteria, Mycobacterium taxa, fungi, and at a lesser extent methanogens and protists. Rumen microbial genes positively correlated with higher concentrations of SFA in milk were associated with increased activity of adaptation to lower pH, by the presence of xanthine and hypoxanthine, and synthesis of acetate and butyrate (which increases their supply for saturated fatty acid synthesis in the mammary gland). The diversity and abundances of rumen microbial genes explained from 76% (for C14:0) to 94% (for C16:0) of the variation in milk fatty acid profiles, thus highlighting that the rumen microbial environment is highly correlated with milk nutritional quality.
Data Availability Statement
Supplementary Material and Supporting Data for this article are uploaded to figshare and can be found at https://figshare.com/s/b5413ccaf25f9b0d9f87. Metagenomics raw data can be downloaded from the European Nucleotide Archive under accession PRJEB33080. A metadata file giving information on the animal experiment is associated to PRJEB33080 and also summarized in Supplementary Table 4.
Ethics Statement
The animal study was reviewed and approved by the animal experiment was conducted at the Centre for Dairy Research (CEDAR), University of Reading, UK, all experimental procedures used were licensed, regulated, and inspected by the UK Home Office under the Animals (Scientific Procedures) Act, 1996.
Author Contributions
MA and SS: conceptualization and project management. MA, IC-L, MM-O, MW, and RS: formal analysis. MA, MM-O, and SS: original writing. SS, IC-L, MM-O, RD, DH, RR, MW, and MA: review and editing. All authors read and approved the final manuscript.
Funding
The part of the project completed at SRUC was supported by a Research Excellence Grant Contingency fund 2017–2018. The work completed at the University of Reading and Centre for Dairy Research was funded by the University of Reading. The Roslin Institute forms part of the Royal (Dick) School of Veterinary Studies, University of Edinburgh. This project was supported by the Biotechnology and Biological Sciences Research Council (BBSRC, BB/N016742/1, BB/S006680/1, BB/N01720X/1, and BB/R015023/1), including institute strategic programme and national capability awards to The Roslin Institute (BBSRC: BB/P013759/1, BB/P013732/1, BB/J004235/1, and BB/J004243/1), and by the Scottish Government (RESAS) as part of the 2016–2021 Strategic Research Programme. We gratefully acknowledge financial support from the Scotland's Rural College, the University of Reading and The Roslin Institute-University of Edinburgh.
Conflict of Interest
The authors declare that the research was conducted in the absence of any commercial or financial relationships that could be construed as a potential conflict of interest.
Acknowledgments
We would like to thank colleagues at the Centre for Dairy Research (University of Reading) for assistance in the collection of experimental samples and data used in this study, and the care of the experimental animals.
Supplementary Material
The Supplementary Material for this article can be found online at: https://www.frontiersin.org/articles/10.3389/fmicb.2020.590441/full#supplementary-material
References
Agricultural and Food Research Council (1993). Energy and Protein Requirements of Ruminants. Wallingford, UK: CAB International.
Akin, D. E., and Borneman, W. S. (1990). Role of rumen fungi in fiber degradation. J. Dairy Sci. 73, 3023–3032. doi: 10.3168/jds.S0022-0302(90)78989-8
Aue, W. P., Bartholdi, E., and Ernst, R. R. (1975). Two-dimensional spectroscopy. application to nuclear magnetic resonance. J. Chem. Phys. 64:2229. doi: 10.1063/1.432450
Auffret, M. D., Dewhurst, R. J., Duthie, C.-A., Rooke, J. A., Wallace, R. J., Freeman, T. C., et al. (2017). The rumen microbiome as a reservoir of antimicrobial resistance and pathogenicity genes is directly affected by diet in beef cattle. Microbiome 5:159. doi: 10.1186/s40168-017-0378-z
Barcelo-Goblijn, G., and Murphy, E. J. (2009). Alpha-linolenic acid and its conversion to longer chain n-3 fatty acids: benefits for human health and a role in maintaining tissue n-3 fatty acid levels. Prog. Lipid Res. 48, 355–374. doi: 10.1016/j.plipres.2009.07.002
Bates, B., Lennox, A., Prentice, A., Bates, C., Page, P., Nicholson, S., et al. (2014). National Diet and Nutrition Survey: Results From Years 1, 2, 3 and 4 Combined of the Rolling Program (2008/9–2011/12). London: Public Health England.
Bergman, E. N., and Wolff, J. E. (1971). Metabolism of volatile fatty acids by liver and portal-drained viscera in sheep. Am. J. Physiol. 221, 586–592. doi: 10.1152/ajplegacy.1971.221.2.586
Bielak, A., Derno, M., Tuchsherer, A., Hammon, H. M., Susenbeth, A., and Kuhla, B. (2016). Body fat mobilization in early lactation influences methane production of dairy cows. Sci. Rep. 6:28135. doi: 10.1038/srep28135
Buitenhuis, B., Lassen, J., Noel, S. J., Plichta, D. R., Sørensen, P., Difford, G. F., et al. (2019). Impact of the rumen microbiome on milk fatty acid composition of Holstein cattle. Genet. Sel. Evol. 51:23. doi: 10.1186/s12711-019-0464-8
Butler, G., Stergiadis, S., Seal, C., Eyre, M., and Leifert, C. (2011). Fat composition of organic and conventional retail milk in northeast England. J. Dairy Sci. 94, 24–36. doi: 10.3168/jds.2010-3331
Bylesjö, M., Rantalainen, M., Cloarec, O., Nicholson, J. K., Holmes, E., and Trygg, J. (2006). OPLS discriminant analysis: combining the strengths of PLS-DA and SIMCA classification. J. Chemom. 20, 341–351. doi: 10.1002/cem.1006
Chen, Y., Oba, M., and Guan, L. L. (2012). Variation of bacterial communities and expression of Toll-like receptor genes in the rumen of steers differing in susceptibility to subacute ruminal acidosis. Vet Microbiol. 159, 451–459. doi: 10.1016/j.vetmic.2012.04.032
Chilliard, Y., Glasser, F., Ferlay, A., Bernard, L., Rouel, J., and Doreau, M. (2007). Diet, rumen biohydrogenation and nutritional quality of cow and goat milk fat. Eur. J. Lipid Sci. Technol. 109, 828–855. doi: 10.1002/ejlt.200700080
Chilliard, Y., Martin, C., Rouel, J., and Doreau, M. (2009). Milk fatty acids in dairy cows fed whole crude linseed, extruded linseed, or linseed oil, and their relationship with methane output. J. Dairy Sci. 92, 5199–5211. doi: 10.3168/jds.2009-2375
Claus, S. P., Tsang, T. M., Wang, Y., Cloarec, O., Skordi, E., Martin, F.-P., et al. (2008). Systemic multicompartmental effects of the gut microbiome on mouse metabolic phenotypes. Mol. Syst. Biol. 4:219. doi: 10.1038/msb.2008.56
Cloarec, O., Dumas, M.-E., Craig, A., Barton, R. H., Trygg, J., Hudson, J., et al. (2005). Statistical total correlation spectroscopy: an exploratory approach for latent biomarker identification from metabolic 1H NMR data sets. Anal. Chem. 77, 1282–1289. doi: 10.1021/ac048630x
Cremonesi, P., Conte, G., Severgnini, M., Turri, F., Monni, A., Capra, E., et al. (2018). Evaluation of the effects of different diets on microbiome diversity and fatty acid composition of rumen liquor in dairy goat. Animal 12, 1856–1866. doi: 10.1017/S1751731117003433
Davidson, A. L., Dassa, E., Orelle, C., and Chen, J. (2008). Structure, function, and evolution of bacterial ATP-binding cassette systems. Microbiol. Mol. Biol. Rev. 72, 317–364. doi: 10.1128/MMBR.00031-07
Dieterle, F., Ross, A., Schlotterbeck, G., and Senn, H. (2006). Probabilistic quotient normalization as robust method to account for dilution of complex biological mixtures. application in 1H NMR metabonomics. Anal. Chem. 78, 4281–4290. doi: 10.1021/ac051632c
EFSA Panel on Dietetic Products Nutrition, and Allergies (NDA). (2010). Scientific Opinion on Dietary Reference Values for fats, including saturated fatty acids, polyunsaturated fatty acids, monounsaturated fatty acids, trans fatty acids, and cholesterol. EFSA J. 8:1461. doi: 10.2903/j.efsa.2010.1461
FAO (2008). Fats and fatty Acids in Human Nutrition - Report of an Expert Consultation. Geneva: FAO.
Ferlay, A., Bernard, L., Meynadier, A., and Malpuech-Brugère, C. (2017). Production of trans and conjugated fatty acids in dairy ruminants and their putative effects on human health: a review. Biochimie 141, 107–120. doi: 10.1016/j.biochi.2017.08.006
Ferris, C. P. (2007). Sustainable pasture based dairy systems – meeting the challenges. Can. J. Plant Sci. 87, 723–738. doi: 10.4141/CJPS06011
Firkins, J. L., and Yu, Z. (2015). Ruminant nutrition symposium: how to use data on the rumen microbiome to improve our understanding of ruminant nutrition. J. Anim. Sci. 93, 1450–1470. doi: 10.2527/jas.2014-8754
Givens, D. I. (2008). Impact on CVD risk of modifying milk fat to decrease intake of saturated fatty acids and increase intake of cis-monounsaturates. Proc. Nutr. Soc. 67, 419–427. doi: 10.1017/S0029665108008707
Givens, D. I. (2010). Milk and meat in our diet: good or bad for health? Animal 4, 1941–1952. doi: 10.1017/S1751731110001503
Glasser, F., Ferlay, A., and Chilliard, Y. (2008). Oilseed lipid supplements and fatty acid composition of cow milk: a meta-analysis. J. Dairy Sci. 91, 4687–4703. doi: 10.3168/jds.2008-0987
Golder, H. M., Thomson, J. M., Denman, S. E., McSweeney, C. S., and Lean, I. J. (2018). Genetic markers are associated with the ruminal microbiome and metabolome in grain and sugar challenged dairy heifers. Front. Genet. 9:62. doi: 10.3389/fgene.2018.00062
Harfoot, C. G., and Hazlewood, G. P. (1988). “Lipid metabolism in the rumen,” in The Rumen Microbial Ecosystem, ed P. N. Hobson (London: Elsevier Applied Science Publishers), 285–322.
Haug, A., Hostmark, A. T., and Harstad, O. M. (2007). Bovine milk in human nutrition. Lipids Health Dis. 6:25. doi: 10.1186/1476-511X-6-25
Henderson, G., Cox, F., Ganesh, S., Jonker, A., Young, W., Global Rumen Census Collaborators., et al. (2015). Rumen microbial community composition varies with diet and host, but a core microbiome is found across a wide geographical range. Sci. Rep. 5:14567. doi: 10.1038/srep14567
Huws, S. A., Scott, M. B., Tweed, J. K., and Lee, M. R. (2013). Fatty acid oxidation products ('green odour') released from perennial ryegrass following biotic and abiotic stress, potentially have antimicrobial properties against the rumen microbiota resulting in decreased biohydrogenation. J. Appl. Microbiol. 15, 1081–1090. doi: 10.1111/jam.12314
Ibeagha-Awemu, E. M., Peters, S. O., Akwanji, K. A., Imumorin, I. G., and Zhao, X. (2016). High density genome wide genotyping-by-sequencing and association identifies common and low frequency SNPs, and novel candidate genes influencing cow milk traits. Sci. Rep. 6:31109. doi: 10.1038/srep31109
Jami, E., White, B. A., and Mizrahi, I. (2014). Potential role of the bovine rumen microbiome in modulating milk composition and feed efficiency. PLoS ONE 9:e85423. doi: 10.1371/journal.pone.0085423
Jenkins, T. C., Wallace, R. J., Moate, P. J., and Mosley, E. E. (2008). Board-invited review: recent advances in biohydrogenation of unsaturated fatty acids within the rumen microbial ecosystem. J. Anim. Sci. 86, 397–412. doi: 10.2527/jas.2007-0588
Karisa, B. K., Thomson, J., Wang, Z., Stothard, P., Moore, S. S., and Plastow, G. S. (2013). Candidate genes and single nucleotide polymorphisms associated with variation in residual feed intake in beef cattle. Anim. Sci. 91, 3502–3513. doi: 10.2527/jas.2012-6170
Kersters, K., Lisdiyanti, P., Komagata, K., and Swings, J. (2006). “The family Acetobacteraceae: the genera Acetobacter, Acidomonas, Asaia, Gluconacetobacter, Gluconobacter, and Kozakia,” in The Prokaryotes, Vol. 5, eds M. Dworkin, S. Falkow, E. Rosenberg, K. H. Schleifer, and E. Stackebrandt (New York, NY: Springer), 163–200.
Kliem, K. E., and Shingfield, K. J. (2016). Manipulation of milk fatty acid composition in lactating cows: opportunities and challenges. Eur. J. Lipid Sci. Technol. 118, 1661–1683. doi: 10.1002/ejlt.201400543
Kliem, K. E., Shingfield, K. J., Livingstone, K. M., and Givens, D. I. (2013). Seasonal variation in the fatty acid composition of milk available at retail in the United Kingdom and implications for dietary intake. Food Chem. 141, 274–281. doi: 10.1016/j.foodchem.2013.02.116
Kristensen, N. B., Gäbel, G., Pierzynowski, S. G., and Danfær, A. (2000). Portal recovery of short-chain fatty acids infused into the temporarily-isolated and washed reticulo-rumen of sheep. Br. J. Nutr. 84, 477–482. doi: 10.1017/S0007114500001781
Kuncharoen, N., Techo, S., Savarajara, A., and Tanasupawat, S. (2020). Identification and lipolytic activity of yeasts isolated from foods and wastes. Mycology 11, 279–286. doi: 10.1080/21501203.2020.1745922
Li, J., Han, D., Wang, D., Ning, K., Jia, J., Wei, L., et al. (2014). Choreography of transcriptomes and lipidomes of Nannochloropsis reveals the mechanisms of oil synthesis in microalgae. Plant Cell 26, 1645–1665. doi: 10.1105/tpc.113.121418
Lourenco, M., Ramos-Morales, E., and Wallace, R. J. (2010). The role of microbes in rumen lipolysis and biohydrogenation and their manipulation. Animal 4, 1008–1023. doi: 10.1017/S175173111000042X
Ma, X.-N., Chen, T.-P., Yang, B., Liu, J., and Chen, F. (2016). Lipid production from nannochloropsis. Mar. Drugs 14:61. doi: 10.3390/md14040061
McDonald, P., Edwards, R. A., Greenhalgh, J. F. D., Morgan, C. A., Sinclair, L. A., and Wilkinson, R. (2011). Digestion in Animal Nutrition. 7th Edn. Harlow, UK: Pearson Education Limited.
Meiboom, S., and Gill, D. (1958). Modified spin-echo method for measuring nuclear relaxation times. Rev. Sci. Instruments 29:688. doi: 10.1063/1.1716296
Mountfort, D. O., Asher, R. A., and Bauchop, T. (1982). Fermentation of cellulose to methane and carbon dioxide by a rumen anaerobic fungus in a triculture with Methanobrevibacter sp. strain RA1 and Methanosarcina barkeri. Appl. Environ. Microbiol. 44, 128–134. doi: 10.1128/AEM.44.1.128-134.1982
Nettleton, J. A., Brouwer, I. A., Geleijnse, J. M., and Hornstra, G. (2017). Saturated fat consumption and risk of coronary heart disease and ischemic stroke: a science update. Ann. Nutr. Metab. 70, 26–33. doi: 10.1159/000455681
Petri, R. M., Schwaiger, T., Penner, G. B., Beauchemin, K. A., and Forster, R. J. (2013). Characterization of the core rumen microbiome in cattle during transition from forage to concentrate as well as during and after an acidotic challenge. PLoS ONE 8:e83424. doi: 10.1371/journal.pone.0083424
Pitta, D. W., Indugu, N., Kumar, S., Vecchiarelli, B., Sinha, R., Baker, L. D., et al. (2016). Metagenomic assessment of the functional potential of the rumen microbiome in Holstein dairy cows. Anaerobe 38, 50–60. doi: 10.1016/j.anaerobe.2015.12.003
Plaizier, J. C., Krause, D. O., Gozho, G. N., and McBride, B. W. (2008). Subacute ruminal acidosis in dairy cows: the physiological causes, incidence and consequences. Vet. J. 176, 21–31. doi: 10.1016/j.tvjl.2007.12.016
Pophaly, S. D., Singh, R., Pophaly, S. D., Kaushik, J. K., and Tomar, S. K. (2012). Current status and emerging role of glutathione in food grade lactic acid bacteria. Microb. Cell Fact. 11:114. doi: 10.1186/1475-2859-11-114
Rae, R. C., and Ingalls, J. R. (1984). Lactational response of dairy cows to oral administration of L-tyrosine. J. Dairy Sci. 67, 1430–1438. doi: 10.3168/jds.S0022-0302(84)81458-7
Rastogi, N., Legrand, E., and Sola, C. (2001). The mycobacteria: an introduction to nomenclature and pathogenesis. Rev. Sci. Tech. 20, 21–54. doi: 10.20506/rst.20.1.1265
Roehe, R., Dewhurst, R. J., Duthie, C.-A., Rooke, J. A., Mckain, N., Ross, D. W., et al. (2016). Bovine host genetic variation influences rumen microbial methane production with best selection criterion for low methane emitting and efficiently feed converting hosts based on metagenomic gene abundance. PLoS Genet. 12:e1005846. doi: 10.1371/journal.pgen.1005846
Rooke, J. A., Wallace, R. J., Duthie, C.-A., McKain, N., Motta de Souza, S., Hyslop, J. J., et al. (2014). Hydrogen and methane emissions from beef cattle and their rumen microbial community vary with diet, time after feeding and genotype. Br. J. Nutr. 112, 398–407. doi: 10.1017/S0007114514000932
Russell, J. B. (1998). The importance of pH in the regulation of ruminal acetate to propionate ratio and methane production in vitro. J. Dairy Sci. 81, 3222–3230. doi: 10.3168/jds.S0022-0302(98)75886-2
Saleem, F., Ametaj, B. N., Bouatra, S., Mandal, R., Zebeli, Q., Dunn, S. M., et al. (2012). A metabolomics approach to uncover the effects of grain diets on rumen health in dairy cows. J. Dairy Sci. 95, 6606–6623. doi: 10.3168/jds.2012-5403
Saleem, F., Bouatra, S., Guo, A. C., Psychogios, N., Mandal, R., Dunn, S. M., et al. (2013). The bovine ruminal fluid metabolome. Metabolomics 9, 360–378. doi: 10.1007/s11306-012-0458-9
Snelling, T. J., Auffret, M. D., Duthie, C.-A., Stewart, R. D., Watson, M., Dewhurst, R. J., et al. (2019). Temporal stability of the rumen microbiota in beef cattle, and response to diet and supplements. Anim. Microbiome 1:16. doi: 10.1186/s42523-019-0018-y
Stergiadis, S., Berlitz, C. B., Hunt, B., Garg, S., Givens, D. I., and Kliem, K. E. (2019). An update to the fatty acid profiles of bovine retail milk in the United Kingdom: implications for nutrition in different age and gender groups. Food Chem. 276, 218–230. doi: 10.1016/j.foodchem.2018.09.165
Stergiadis, S., Leifert, C., Seal, C. J., Eyre, M. D., Larsen, M. K., Slots, T., et al. (2015). A 2-year study on milk quality from three pasture-based dairy systems of contrasting production intensities in Wales. J. Agric. Sci. 153, 708–731. doi: 10.1017/S0021859614000963
Stergiadis, S., Leifert, C., Seal, C. J., Eyre, M. D., Nielsen, J. H., Larsen, M. K., et al. (2012). Effect of feeding intensity and milking system on nutritionally relevant milk components in dairy farming systems in the north east of England. J. Agric. Food Chem. 60, 7270–7281. doi: 10.1021/jf301053b
Stergiadis, S., Leifert, C., Seal, C. J., Eyre, M. D., Steinshamn, H., and Butler, G. (2014). Improving the fatty acid profile of winter milk from housed cows with contrasting feeding regimes by oilseed supplementation. Food Chem. 164, 293–300. doi: 10.1016/j.foodchem.2014.05.021
Stewart, R. D., Auffret, M. D., Warr, A., Walker, A. W., Roehe, R., and Watson, M. (2019). Compendium of 4,941 rumen metagenome-assembled genomes for rumen microbiome biology and enzyme discovery. Nat. Biotechnol. 37, 953–961. doi: 10.1038/s41587-019-0202-3
Stewart, R. D., Auffret, M. D., Warr, A., Wiser, A. H., Press, M. O., and Langford, K. W.. (2018). Assembly of 913 microbial genomes from metagenomic sequencing of the cow rumen. Nat. Commun. 9:870. doi: 10.1038/s41467-018-03317-6
Swanson, D., Block, R., and Mousa, S. (2012). Omega-3 fatty acids EPA and DHA: health benefits throughout life. Adv. Nutr. 3, 1–7. doi: 10.3945/an.111.000893
Ulberth, F., Gabernig, R. G., and Schrammel, F. (1999). Flame-ionization detector response to methyl, ethyl, propyl, and butyl esters of fatty acids. J. Am. Oil Chem. Soc. 76, 263–266. doi: 10.1007/s11746-999-0228-7
Ventto, L., Leskinen, H., Kairenius, P., Stefański, T., Bayat, A. R., Vilkki, J., et al. (2017). Diet-induced milk fat depression is associated with alterations in ruminal biohydrogenation pathways and formation of novel fatty acid intermediates in lactating cows. Br. J. Nutr. 117, 364–376. doi: 10.1017/S0007114517000010
Viant, M. R. (2007). Revealing the metabolome of animal tissues using 1H nuclear magnetic resonance spectroscopy. Methods Mol. Biol. 358, 229–246. doi: 10.1007/978-1-59745-244-1_13
Wallace, R. J., Rooke, J. A., Duthie, C.-A., Hyslop, J. J., Ross, D. W., McKain, N., et al. (2014). Archaeal abundance in postmortem ruminal digesta may help predict methane emissions from beef cattle. Sci. Rep. 4:5892. doi: 10.1038/srep05892
Wallace, R. J., Rooke, J. A., Mckain, N., Duthie, C.-A., Hyslop, J. J., Ross, D. W., et al. (2015). The rumen microbial metagenome associated with high methane production in cattle. BMC Genomics 16:839. doi: 10.1186/s12864-015-2032-0
Wold, S. (1995). “PLS for multivariate linear modelling,” in Chemometric Methods in Molecular Design, ed H. Van de Waterbeemd (Weinheim: VCH Publishers), 195–218.
Wood, D. E., and Salzberg, S. L. (2014). Kraken: ultrafast metagenomic sequence classification using exact alignments. Genome Biol. Evol. 15:R46. doi: 10.1186/gb-2014-15-3-r46
World Health Organization (WHO) (2003). Food and Agriculture Organization of the United Nations, Diet, Nutrition and the Prevention of Chronic Diseases 2003. Geneva: World Health Organization.
Xue, M., Sun, H., Wu, X., Guan, L. L., and Liu, J. (2018). Assessment of rumen microbiota from a large dairy cattle cohort reveals the pan and core bacteriomes contributing to varied phenotypes. Appl. Environ. Microbiol. 84, e00970–18. doi: 10.1128/AEM.00970-18
Keywords: rumen microbiome, microbial genes, cow, milk fatty acids, metagenomics, metabolomics, rumen stress
Citation: Stergiadis S, Cabeza-Luna I, Mora-Ortiz M, Stewart RD, Dewhurst RJ, Humphries DJ, Watson M, Roehe R and Auffret MD (2021) Unravelling the Role of Rumen Microbial Communities, Genes, and Activities on Milk Fatty Acid Profile Using a Combination of Omics Approaches. Front. Microbiol. 11:590441. doi: 10.3389/fmicb.2020.590441
Received: 01 August 2020; Accepted: 21 December 2020;
Published: 21 January 2021.
Edited by:
Samodha Charaka Fernando, University of Nebraska-Lincoln, United StatesReviewed by:
Shengguo Zhao, Chinese Academy of Agricultural Sciences, ChinaThomas E. Burkey, University of Nebraska-Lincoln, United States
Copyright © 2021 Stergiadis, Cabeza-Luna, Mora-Ortiz, Stewart, Dewhurst, Humphries, Watson, Roehe and Auffret. This is an open-access article distributed under the terms of the Creative Commons Attribution License (CC BY). The use, distribution or reproduction in other forums is permitted, provided the original author(s) and the copyright owner(s) are credited and that the original publication in this journal is cited, in accordance with accepted academic practice. No use, distribution or reproduction is permitted which does not comply with these terms.
*Correspondence: Sokratis Stergiadis, cy5zdGVyZ2lhZGlzQHJlYWRpbmcuYWMudWs=; Marc D. Auffret, bWFyYy5hdWZmcmV0QGRhbm9uZS5jb20=
†These authors have contributed equally to this work
‡Present address: Marina Mora-Ortiz, Department of Twin Research, Kings College London, St Thomas' Hospital, London, United Kingdom
Marc D. Auffret, Gut & Microbiology Laboratories, Danone Nutricia Research, Innovation, Science & Nutrition Department, Palaiseau, France