- 1CSIRO Australian National Herbarium, Centre for Australian National Biodiversity Research, National Research Collections Australia, NCMI, Canberra, ACT, Australia
- 2CSIRO Land and Water, Canberra, ACT, Australia
- 3CSIRO Synthetic Biology Future Science Platform, Canberra, ACT, Australia
- 4Department of Biology and Center for Biodiversity and Conservation Research, The University of Mississippi, University City, MS, United States
- 5Department of Biological Sciences, Louisiana State University, Baton Rouge, LA, United States
Lichen associations, a classic model for successful and sustainable interactions between micro-organisms, have been studied for many years. However, there are significant gaps in our understanding about how the lichen symbiosis operates at the molecular level. This review addresses opportunities for expanding current knowledge on signalling and metabolic interplays in the lichen symbiosis using the tools and approaches of systems biology, particularly network modelling. The largely unexplored nature of symbiont recognition and metabolic interdependency in lichens could benefit from applying a holistic approach to understand underlying molecular mechanisms and processes. Together with ‘omics’ approaches, the application of signalling and metabolic network modelling could provide predictive means to gain insights into lichen signalling and metabolic pathways. First, we review the major signalling and recognition modalities in the lichen symbioses studied to date, and then describe how modelling signalling networks could enhance our understanding of symbiont recognition, particularly leveraging omics techniques. Next, we highlight the current state of knowledge on lichen metabolism. We also discuss metabolic network modelling as a tool to simulate flux distribution in lichen metabolic pathways and to analyse the co-dependence between symbionts. This is especially important given the growing number of lichen genomes now available and improved computational tools for reconstructing such models. We highlight the benefits and possible bottlenecks for implementing different types of network models as applied to the study of lichens.
Introduction
Lichens are often seen as a typical example of successful and sustainable symbiotic interactions between micro-organisms (Ahmadjian, 1993; Honegger, 1998). With the long evolutionary history of these fungal-algal associations (Gueidan et al., 2011; Prieto and Wedin, 2013; Lutzoni et al., 2018; Nelsen et al., 2019) and their multiple origins within the evolution of fungi (Gueidan et al., 2008; Schoch et al., 2009; Nelsen et al., 2020), lichens have colonised and diversified greatly in most terrestrial and some aquatic environments, including the most inhospitable niches (Kappen, 2000; Sadowsky and Ott, 2016). They are a discrete but inherent part of most of our landscapes, including both natural and man-made. This success stems from their ability to act as self-sustainable ecosystems, for which an evolutionary modularity (i.e., selection of the most fitted partners for a particular environment) has allowed adaptation to a broad range of habitats. Because of their slow growth, they particularly excel in colonising harsh habitats in which competition with faster growing micro-organisms is low. As such, they have adapted to surviving on nutrient-poor substrates and under drastically fluctuating environmental conditions, and play key roles in their ecosystems. In the future, lichen adaptations and their natural ecological flexibility may prove to be key to the successful responses of lichens to climate change.
The lichen symbiosis is no longer perceived to be the simple union of a fungal partner (i.e., mycobiont) and a microalgal partner (i.e., photobiont), either an alga (i.e., chlorolichen) or a cyanobacterium (i.e., cyanolichen). Instead, previous studies have shown that lichens harbour a diverse microbiome (e.g., Petrini et al., 1990; Hofstetter et al., 2007; Grube et al., 2009; Hodkinson and Lutzoni, 2009), and more recent studies corroborate lichens as multi-symbioses, i.e., complex multi-species associations including bacteria and other fungi or algae (Spribille et al., 2016; Onut-Brannstrom et al., 2018; Tuovinen et al., 2019; Smith et al., 2020; Leiva et al., 2021). In such symbioses, each partner contributes to the association: the primary mycobiont provides shelter and minerals to the photobiont, while the photobiont provides organic carbon fixed from atmospheric CO2 via photosynthesis (Nash, 2008a) as well as nitrogen if it is a cyanobacteria. Additional bacteria, algae, and/or fungi have also been shown to serve certain functions in the lichen symbiosis (Cernava et al., 2017; Smith et al., 2020; Tagirdzhanova et al., 2021), although much more remains to be explored. Additionally, the levels of dependence and specificity of some of these microbes to the symbiosis are still debated (Grube et al., 2015; Kono et al., 2017; Jenkins and Richards, 2019; Lendemer et al., 2019; Smith et al., 2020). Lichens demonstrate unique physiological properties and ecosystem functions (Porada et al., 2014). All lichens contribute to atmospheric carbon fixation, with global net carbon uptake by both lichens and bryophytes predicted to be 0.34–3.3 Gt carbon per year (Palmqvist, 1995; Green et al., 2008; Palmqvist et al., 2008; Porada et al., 2013). Cyanolichens are capable of both carbon and nitrogen fixation (Dahlman et al., 2004; Nash, 2008b; Porada et al., 2017). Lichens grow on various substrates (including rocks, trees, and soil), can survive extreme temperatures, tolerate desiccation (poikilohydric) and high levels of UV radiation, and form morphologically diverse structures (Beckett et al., 2008; Kranner et al., 2008). Many lichens produce unique specialised/secondary metabolites, including depsides, xanthones and dibenzofurans, some of which have been shown to have medicinal properties (Fahselt, 1994; Elix and Stocker-Worgotter, 2008; Calcott et al., 2018).
The establishment of the lichen symbiosis, or “lichenisation,” has been described as a four-stage process (Ahmadjian et al., 1978): (A) a pre-contact phase (chemical interactions between symbionts but no physical contact), (B) a post-contact phase (with chemical and physical interactions), (C) a phase of growth characterised by an un-differentiated mass, and (D) a phase of differentiation that leads to a stratified thallus (Figure 1). Because mycobionts grow relatively slowly, the application of classical experimental microbiology techniques and co-culture/resynthesis experiments to the understanding of the development and functioning of the lichen symbiosis has lagged. Despite some recent studies focusing on early stages of lichenisation (Joneson et al., 2011; Armaleo et al., 2019; Kono et al., 2020), the molecular basis of fungal-algal interactions during lichenisation remains mostly uncharacterised, and processes involved in signalling and metabolic interplays between the symbionts are poorly understood. Contemporary systems biology approaches may facilitate tackling long-standing questions about the lichen symbiosis.
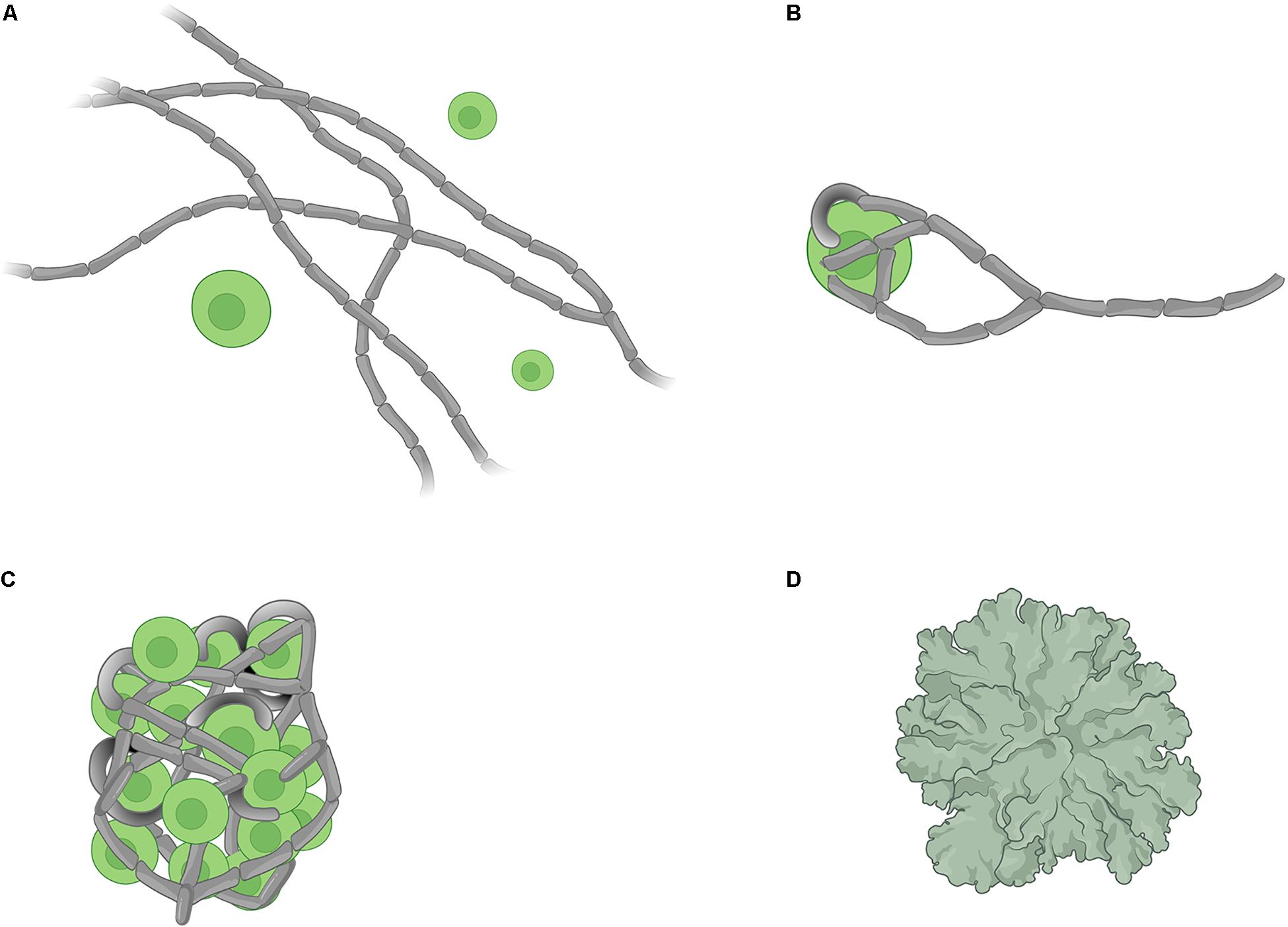
Figure 1. Schematic representation of the four stages of lichen formation. (A) pre-contact where the symbionts are located at the proximity of each other but not in physical contact, (B) post-contact which marks the initiation of physical contacts between symbionts, (C) growth of an undifferentiated mass consisting of fungal hyphae and algal cells, and (D) formation of a differentiated thallus. (Image created with BioRender.com).
Systems biology is the study of living systems through the joint application of advanced high-data-volume generating technologies (e.g., ‘omics’) and computational tools (e.g., multi-scale or constraint-based modelling) to gain a more holistic understanding of the inter-dependencies of system components and underlying system complexity. Hypotheses are generally tested using iterative cycles of ‘wet’ (lab-based) and ‘dry’ (simulation-based) experiments, by which systems-level data are generated, analysed, and then used to inspire new insights and hypotheses about the biological system at hand (Kitano, 2002a, b). For instance, applying systems- and genome-level approaches to the legume-rhizobium symbiosis has greatly enhanced the knowledge on the underlying mechanisms of symbiotic interactions at molecular level, moving us one step closer to improving agricultural crop yields through the development of more efficient symbiotic N2 fixation processes (diCenzo et al., 2019). A similar systems biology approach has not yet been applied to the study of the lichen symbiosis.
In this review, we summarise the current knowledgebase of signalling and recognition mechanisms in the lichen symbiosis. We then discuss the modelling of signalling networks as a tool to extend our understanding of such mechanisms in lichens. We review the literature on lichen metabolism and propose that modelling fluxes in metabolic networks could be a powerful tool for providing insights into lichen metabolism in particular, and the metabolic interplays between symbiotic partners in general. We provide a broad overview of metabolic network models and their applications in addition to a review of some of the symbiotic systems that have been studied through the lens of metabolic models. Finally, the opportunities and challenges of modelling both signalling networks and metabolic fluxes are discussed.
Signalling and Recognition Pathways in the Lichen Symbiosis
Distinct small molecules are produced by lichen symbionts during symbiosis that are absent when mycobiont and photobiont are grown separately (Green and Smith, 1974; Elshobary et al., 2015). Whether symbiont signalling and recognition processes in lichens are driven initially by those small molecules, or whether recognition processes are initiated by other regulatory mechanisms is not known. The available data for molecules with potential roles in signalling and/or recognition mechanisms during lichen symbiosis are summarised in Table 1. So far, there is no direct evidence confirming the production of compounds with a potential role in signalling and/or recognition during lichenisation by inhabiting fungi or bacteria. Several studies have shown that signalling between lichen symbionts can be initiated as early as the pre-contact stage of lichenisation (Joneson et al., 2011; Meessen and Ott, 2013; Piercey-Normore and Athukorala, 2017; Armaleo et al., 2019). At present and for a few reasons, it is difficult to propose universal signalling models that initiate lichen symbiosis. Firstly, there is no single signalling molecule with a known or proposed role that has been studied across different lichens. Secondly, signalling pathways of those molecules with putative recognition roles have not been elucidated. Thirdly, lichens have likely evolved independently in several fungal lineages (Gueidan et al., 2008; Schoch et al., 2009), suggesting that the nature of these signalling pathways might differ depending on the species of interest. Nonetheless, owing to advances in genetic and analytical tools, several studies have begun to uncover mechanistic details underlying partner signalling and recognition at various stages of lichenisation (Meessen et al., 2013; Meessen and Ott, 2013; Athukorala et al., 2014; Athukorala and Piercey-Normore, 2015).
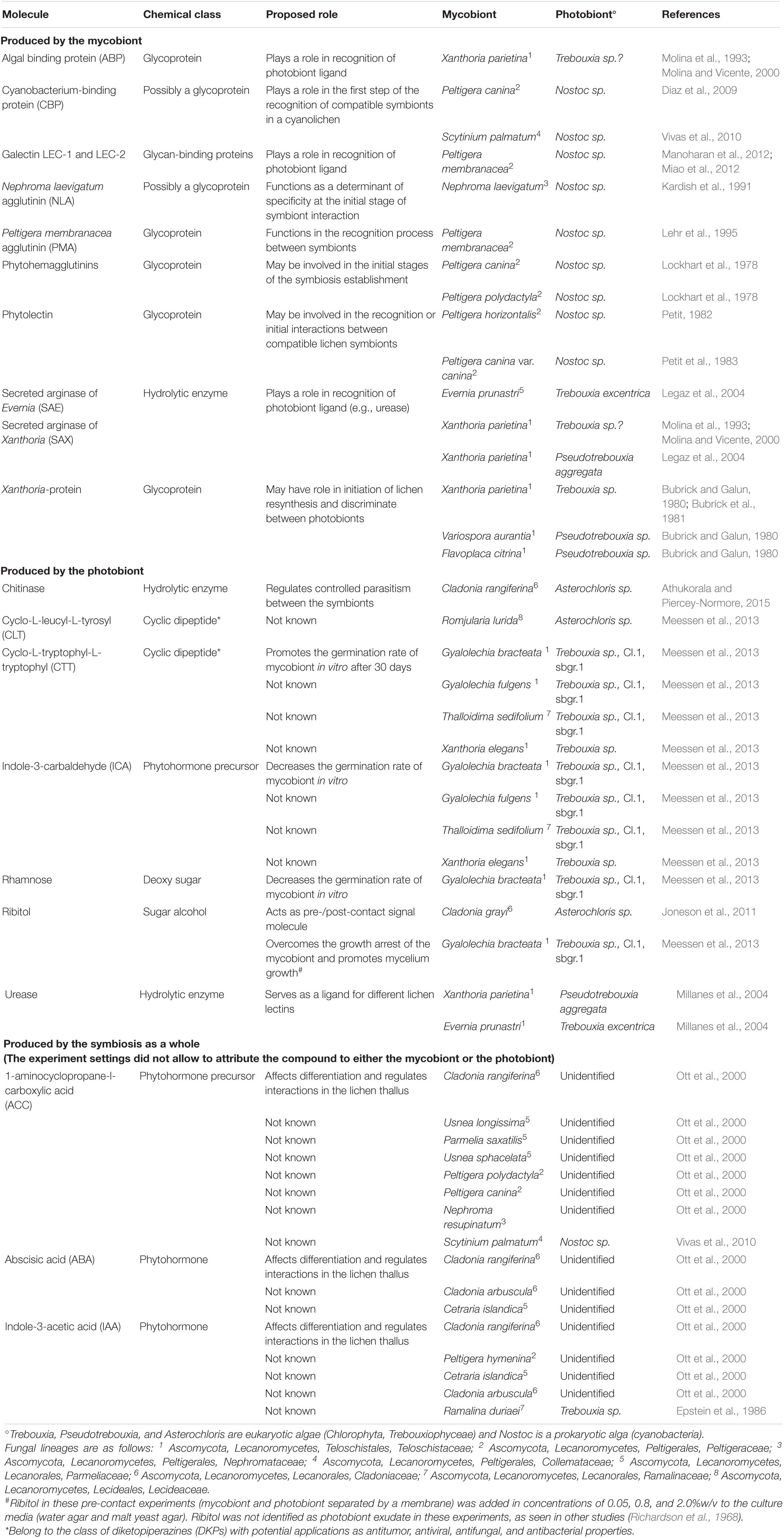
Table 1. Molecules produced by different lichen symbionts with proposed roles in symbiotic signalling and recognition.
Lectin-Ligand Signalling in Lichens
Lectins are glycoproteins that occur ubiquitously across all domains of life (Kennedy et al., 1995). Lectins have also been isolated and characterised from both chlorolichens and cyanolichens (Table 1). Possessing versatile carbohydrate-binding site(s), lectins can act as receptors and/or bind/agglutinate cells that may facilitate further interfacial communication between cells. The glycosidic moieties of lectins synthesised by the mycobiont may contain various combinations of carbohydrate groups that bind to specific ligands from the photobiont. In this atypical receptor-ligand system, lectins from mycobionts act as receptors for photobiont-attached ligands. A proposed mechanism of photobiont recognition and recruitment by the mycobiont is illustrated in Figure 2, based on the extensive studies of the lichens Xanthoria parietina and Evernia prunastri (Bubrick and Galun, 1980; Bubrick et al., 1981; Perezurria and Vicente, 1989; Vicente and Perezurria, 1989; Rodriguez and Vicente, 1991; Molina et al., 1993, 1998; Molina and Vicente, 1995, 2000; Legaz et al., 2004; Millanes et al., 2004). Lectins characterised from other lichens also have been proposed to have roles in the establishment and/or maintenance of compatible symbiotic relationships (Table 1).
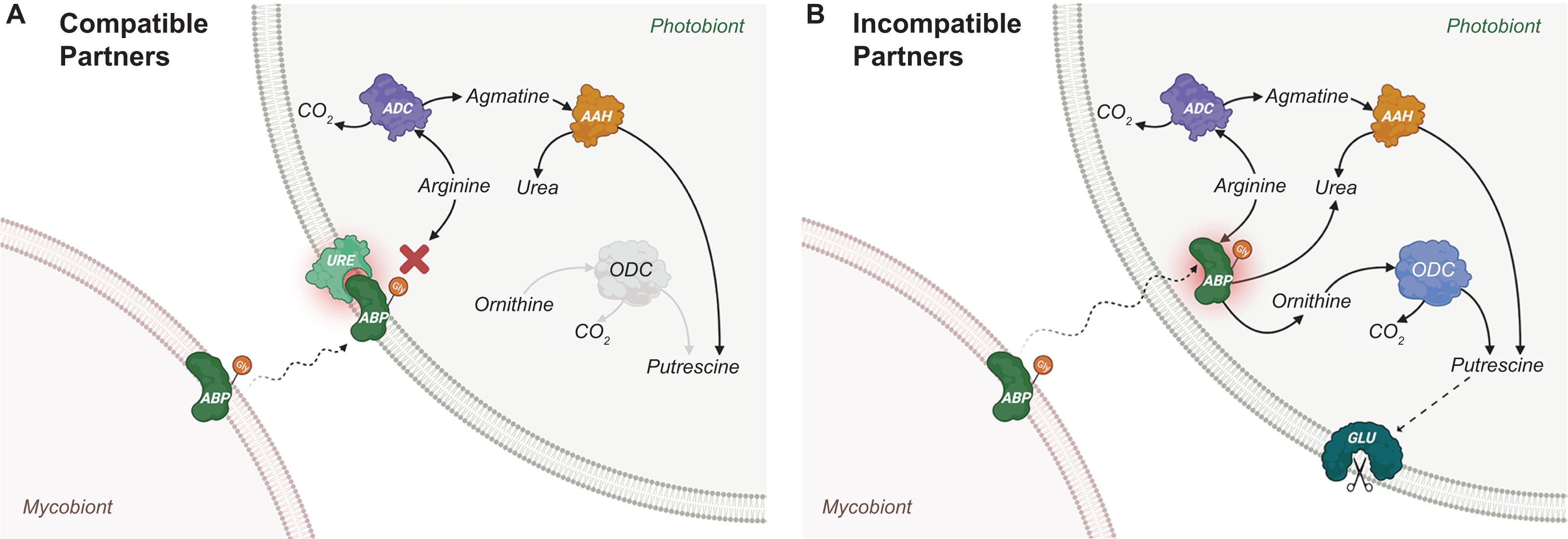
Figure 2. Proposed lectin-ligand recognition and signalling for Xanthoria parietina. (A) For compatible partners. The process starts by the production and positioning of algal binding protein (ABP) on the cell wall of the mycobiont. ABP is a lectin (“receptor”) which is glycosylated and secreted into the intercellular space (Molina et al., 1993; Molina and Vicente, 1995) to bind specific cognate ligands of a photobiont, with which a potential lichenisation could be established. It is speculated that ABP is part of a group of “recognition lectins” that is responsible for engaging the mycobiont with a specific photobiont, and another group of “recruitment lectins” that play a role in recruiting the photobiont cells to mycobiont hyphae (Sacristán et al., 2007). In the lichen X. parietina, a recruitment lectin was identified as a secreted arginase of the Xanthoria mycobiont (SAX). Although both ABP (recognition) and SAX (recruitment) mycobiont lectins were shown to bind to the ligand URE, a glycosylated urease located on the compatible photobiont cell wall (Millanes et al., 2004), it is not clear whether both must attach to urease for lichenisation to proceed. Both ABP and SAX lectins possess identical peptide sequences and Mn2+-dependent arginase activity, hydrolysing arginine to produce urea and ornithine (Legaz et al., 2004). However, the glycosidic moiety of SAX contains galactose and glucose, whereas that of ABP contains glucose and N-acetyl-glucosamine (Molina et al., 1993). Upon binding, enzymatic activities of ABP (recognition lectin and receptor) and URE (ligand) are inhibited. As a result, putrescine concentrations in a compatible photobiont are kept at relatively low endogenous levels because it is only produced through arginine decarboxylase (ADC) and agmatine amidinohydrolase (AAH). (B) For incompatible partners. The binding selectivity of ABP (receptor on mycobiont) for URE (ligand on photobiont) is the key in compatible partner recognition. For example, the ABP lectin receptor of E. prunastri can only bind to a single galactosylated ligand (Diaz et al., 2016c). Thus, an incompatible photobiont lacking galactosylated URE would not be recognised, resulting in an internalisation of ABP by the photobiont. Without URE ligand-induced inhibition, ABP goes on to hydrolyse cytoplasmic arginine stores in the photobiont (ABP has a higher affinity for arginine than that of ADC), producing urea and ornithine and a reaction cascade (involving both ornithine decarboxylase (ODC) and AAH) that results in increased cytoplasmic putrescine levels. High intracellular putrescine concentrations lead to the activation of glucanase (GLU), which results in cell wall disruptions and, ultimately, death of the incompatible photobiont cells (Molina et al., 1998). (Image created with BioRender.com).
In several lichen associations (including X. parietina and E. prunastri shown in Figure 2), the ligand for lectin receptors has been identified as urease, which is bound to the cell wall of the photobiont (Molina et al., 1993; Millanes et al., 2004; Diaz et al., 2009). In the lichen Cladonia rangiferina, a urease-like recognition-related protein (RR1) was characterised and speculated to act as a ligand on the cell wall of the compatible photobiont of this lichen association (Athukorala et al., 2014; Athukorala and Piercey-Normore, 2015). Urease is produced by several lichens (presumably by the photobiont) and is secreted into the culture medium under laboratory conditions (Perezurria et al., 1989, 1993). The secretion of urease into the medium is hypothesised to be the consequence of its transfer from the photobiont to the mycobiont, depending on the nitrogen content of the mycobiont as well as the water content of the lichen thallus (Perezurria et al., 1989). However, it is not clear, whether the secreted ureases play a role similar to that of membrane-bound urease.
The lectin recognition and signalling mechanism summarised for chlorolichens in Figure 2 can be true of all or some cyanolichens (Sacristán et al., 2007; Vivas et al., 2010; Díaz et al., 2016a). Diaz et al. (2015), Diaz et al. (2016b) showed that actin- and myosin-like proteins produced by the cyanobacterial photobiont Nostoc of the lichen Peltigera canina is involved in the chemotactic movement of photobiont cells towards the lectin of the mycobiont. The process also involves a contractile protein and ATPase of photobiont, which creates a series of contraction-relaxation steps that result in photobiont movement towards mycobiont lectin (Diaz et al., 2011). Upon cell contact of photobiont and mycobiont, desensitisation occurs and photobiont contractile motility stops. It is yet unknown if a similar type of chemotaxis applies to chlorolichens.
It is speculated that mycobionts of some lichens not only rely on lectin-ligand recognition mechanisms for establishing the initial photobiont contact, but that these mechanisms might also be involved in further replication and growth of young photobiont cells within the lichen thallus (Díaz et al., 2016a). The factors triggering the initiation of symbiont recognition through lectin-ligand binding and the ways in which symbionts increase the probability of association have been poorly understood, although several hypotheses have been proposed (Díaz et al., 2016a). For example, the photobiont could secrete a yet unknown diffusible compound that is sensed by a compatible mycobiont to trigger mycobiont lectin biosynthesis. Mycobionts may also produce multiple lectins with competing specificities for different photobionts, which may also be a strategy for rejecting incompatible photobionts. We could test some of these hypotheses using a systems biology approach, for example, through time-course analysis of coupled gene expression and metabolome profiles of lichen co- and mono-cultures to identify candidate genes and molecules with potential signalling roles. Armaleo et al. (2019) recently pursued a transcriptome study exploring the differential expression of genes involved in symbiosis and signalling between Cladonia grayi and its algal partner Asterochloris glomerata. While only a snapshot in time, this work provided unprecedented insights into the complexity of responses underlying lichen symbioses.
Exudates Signalling in Lichens
Carbohydrate release and translocation from photobiont to mycobiont of a lichen was first proposed in the mid-1960s by Drew and Smith, who used radioactive isotope tracing to estimate the proportion of labelled carbon in sodium [14C]-bicarbonate fixed to [14C]-glucose by the cyanobacterial symbiont (Nostoc) of Peltigera polydactyla compared with its free-living and cultured forms (Drew and Smith, 1967a, b). Carbohydrate movement from photobiont to mycobiont has been investigated for more than 30 additional lichens and is reviewed elsewhere (Smith et al., 1969). The results of these studies showed that glucose and sugar alcohols are the main forms of carbohydrates released by cyanobacterial and microalgal photobionts, respectively, and that they are translocated to the respective mycobionts. In the absence of a symbiotic relationship, the levels of carbohydrate released by the photobionts decline significantly or drop to zero. Following these initial studies, the importance of carbohydrate release by lichen photobionts gained a renewed interest in efforts to uncover the molecular mechanisms behind the early stage of lichenisation (Joneson et al., 2011; Meessen et al., 2013; Meessen and Ott, 2013; Athukorala et al., 2014; Athukorala and Piercey-Normore, 2014; Armaleo et al., 2019). A possible exudate signalling model based on the release and movement of ribitol is shown in Figure 3, and is largely based on independent studies observing ribitol release in the cultures of Gyalolechia bracteata (Meessen et al., 2013; Meessen and Ott, 2013) and Cladonia grayi (Joneson et al., 2011). Although the exact nature of the secreted molecules in this exudate-signalling model has not been fully elucidated, it is speculated that an exchange of carbon and nitrogen could be the driver for uniting symbionts in the first place. Hom and Murray (Hom and Murray, 2014) showed that co-culturing of model fungi Saccharomyces cerevisiae, Aspergillus nidulans, or Neurospora crassa with the alga Chlamydomonas reinhardtii could facilitate mutualistic interactions through exchanging carbon and nitrogen under specific growth conditions; their results also suggest that carbon released by mycobiont respiration (as CO2) could be recaptured by the photobiont for efficient carbon recycling within the lichen symbiosis (Schwartzman, 2010). Thus, the need for nutrient exchange between species could trigger the initiation of symbiotic interaction in lichens. Signalling network modelling, discussed in the following section, is one approach to generate insights on how specific exudate compounds could play a role in the overall flow of signals through the proposed ‘exudates signalling’ mechanism.
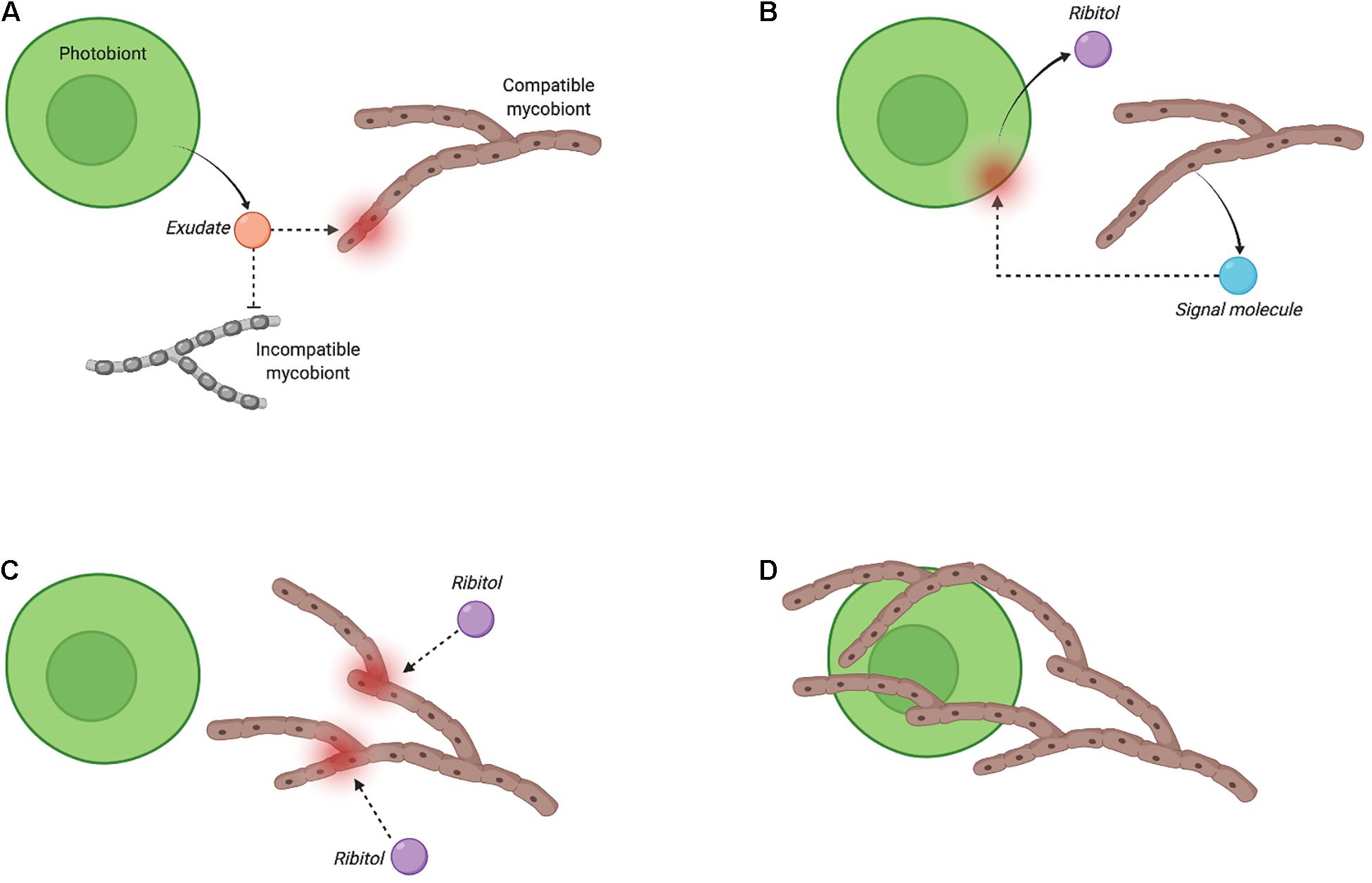
Figure 3. Proposed exudate-based recognition and signalling for Fulgensia bracteata (adapted from Meessen et al., 2013). The bi-directional and multi-step recognition process (i.e., pre-contact stage) is initiated by a compatible photobiont through secretion of an exudate, which is speculated to be species-dependent but has yet to be identified for most lichen species (A). The exudate induces the production and secretion by the mycobiont of a yet unknown signalling molecule that stimulates ribitol release by the photobiont (B). Ribitol then triggers fungal growth by relieving hyphal growth arrest (C), and allowing hyphal branching, and the engulfment of the photobiont cell by the mycobiont (D). The two last steps may be accompanied by the secretion of a mucilage in some lichens (Meessen et al., 2013; Meessen and Ott, 2013). (Image created with BioRender.com).
Signalling Network Modelling: Challenges and Opportunities for the Lichen Symbiosis
A signalling network consists of a series of ‘signals’ and ‘receptors’ whose relationships are determined by the signal transduction mechanisms governing the network. These signals and receptors could be any or combination of enzymes (e.g., kinases), organic substances (e.g., ATP), inorganic molecules (e.g., phosphates), or other proteins or biomolecules. Reactions connecting these molecules frame the underlying signalling mechanisms and the goal of signalling network modelling would be to predict such interactions and the emergent cascade of signalling events that can explain or predict the behaviour of the signalling network. Signalling network models are often divided into descriptive and predictive subtypes. Descriptive models are usually simpler and provide a qualitative overview of the signalling pathway structure (i.e., topology of signal molecules and reactions), whereas predictive models may capture kinetics of the signalling pathway (i.e., reaction rates) and be capable of estimating system behaviours under new perturbations. The application of diverse descriptive and predictive modelling to signalling networks has been reviewed elsewhere (Hyduke and Palsson, 2010; Morris et al., 2010; Terfve and Saez-Rodriguez, 2012; Rother et al., 2013; Lavrik and Samsonova, 2016; Antebi et al., 2017). The scope and choice of signalling network modelling approach vary with the complexity of the network being explored. For example, some of the most detailed and comprehensive predictive signalling models have been developed for complex but known signalling networks of human B-cells (Papin and Palsson, 2004), prostate cancer cells (Dasika et al., 2006; Vardi et al., 2012), and Toll-like receptors (TLRs) functioning in immune system (Li et al., 2009).
In symbiotic systems, signalling pathways have been a topic of particular focus for legumes-rhizobia and plants-root fungi (mycorrhiza) symbioses (Bonfante and Genre, 2010; Bonfante and Requena, 2011; Oldroyd, 2013; Venkateshwaran et al., 2013; Mohanta and Bae, 2015; Martin et al., 2017; Poole et al., 2018; Clear and Hom, 2019). However, modelling the signalling networks in these systems has not received much attention, perhaps due largely to the knowledge gap in certain key signalling steps. For example, in the common symbiotic signalling “SYM” pathway, which shares similar signalling steps between arbuscular mycorrhizal and rhizobial symbioses, it remains unclear how symbiosis receptor kinases (SYMRK) transmit signals to downstream cation channelling proteins (i.e., CASTOR/POLLUX) located in the nucleus (Huisman and Geurts, 2020). Moreover, the precise mechanisms for how plants discriminate between arbuscular mycorrhiza and rhizobia symbionts are still unknown, although signalling pathways functioning in parallel to the SYM seem likely to be involved. Modelling signalling networks could represent a complementary approach to fill such gaps by simulating system behaviours with proposed/candidate mechanisms implemented by which symbionts transduce signals and communicate.
Faced with the paucity of detailed mechanistic knowledge on signalling networks in lichens (despite several potential signal molecules identified; see Table 1), the modelling of signalling networks in lichens suffers from similar challenges as those of other symbiotic systems and no models have yet been reported. Nevertheless, given the recent availability of ‘omics’ data for a variety of lichens (Mittermeier et al., 2015; Wang et al., 2015; Armaleo et al., 2019), there are now new opportunities to develop signalling models of lichens. For instance, a proteomics approach could enable measuring lectin and urease levels of lichen cultures at pre- and post-contact stages informing the relative abundances of these proteins. The proteome profile of such cultures could also indicate the presence/absence of other specific proteins at the corresponding stages of lichenisation that may correlate with lectin/urease activity levels and provide deeper insights into how the recognition process initiates. A signalling pathway model could be developed to explore the link between putrescine biosynthesis and lectin production in repression of cell wall disruption of compatible photobiont as described in Figure 3.
Metabolic Interplay in the Lichen Symbiosis
The literature on lichen metabolism has been largely focused on understanding the exchange of key nutrients between symbionts (Lines et al., 1989; Kono et al., 2020; ten Veldhuis et al., 2020) and identifying lichen secondary metabolites and their biosynthetic pathways (i.e., metabolite profiling) (Fahselt, 1994; Aubert et al., 2007; Elix and Stocker-Worgotter, 2008; Mittermeier et al., 2015; Bertrand et al., 2018b; Brakni et al., 2018; Calcott et al., 2018; Kuhn et al., 2019; Goga et al., 2020; Figure 4). In the 1960s, observations of carbohydrate storage and translocation between the symbionts of Peltigera polydactyla (Smith and Drew, 1965; Drew and Smith, 1967a, b) together with a series of similar studies on other lichens (Smith et al., 1969) established the foundations for studying the metabolic interplay in lichens. The primary aim of those studies was to identify the form of carbon translocated between lichen symbionts, as explained in the previous sections. Next to nothing is known about the metabolic program and gene expression in lichen symbionts following carbohydrate exchange and assimilation. Most metabolic studies in lichens have concentrated on understanding the overall carbon and nitrogen economy in lichens, mainly with respect to overall carbon fixation, carbon sinks, lichen growth, and nitrogen fixation by cyanolichens (Honegger et al., 1993; Dahlman et al., 2004; Nash, 2008b; Palmqvist et al., 2008). Eisenreich and colleagues (Eisenreich et al., 2011) suggested that using ‘omics’ methods together with isotope labelling experiments (increasingly referred to as ‘fluxomics’) could enhance our understanding of lichen metabolic pathways, although this has yet to be fully realised to study lichen metabolism at a systems-level.
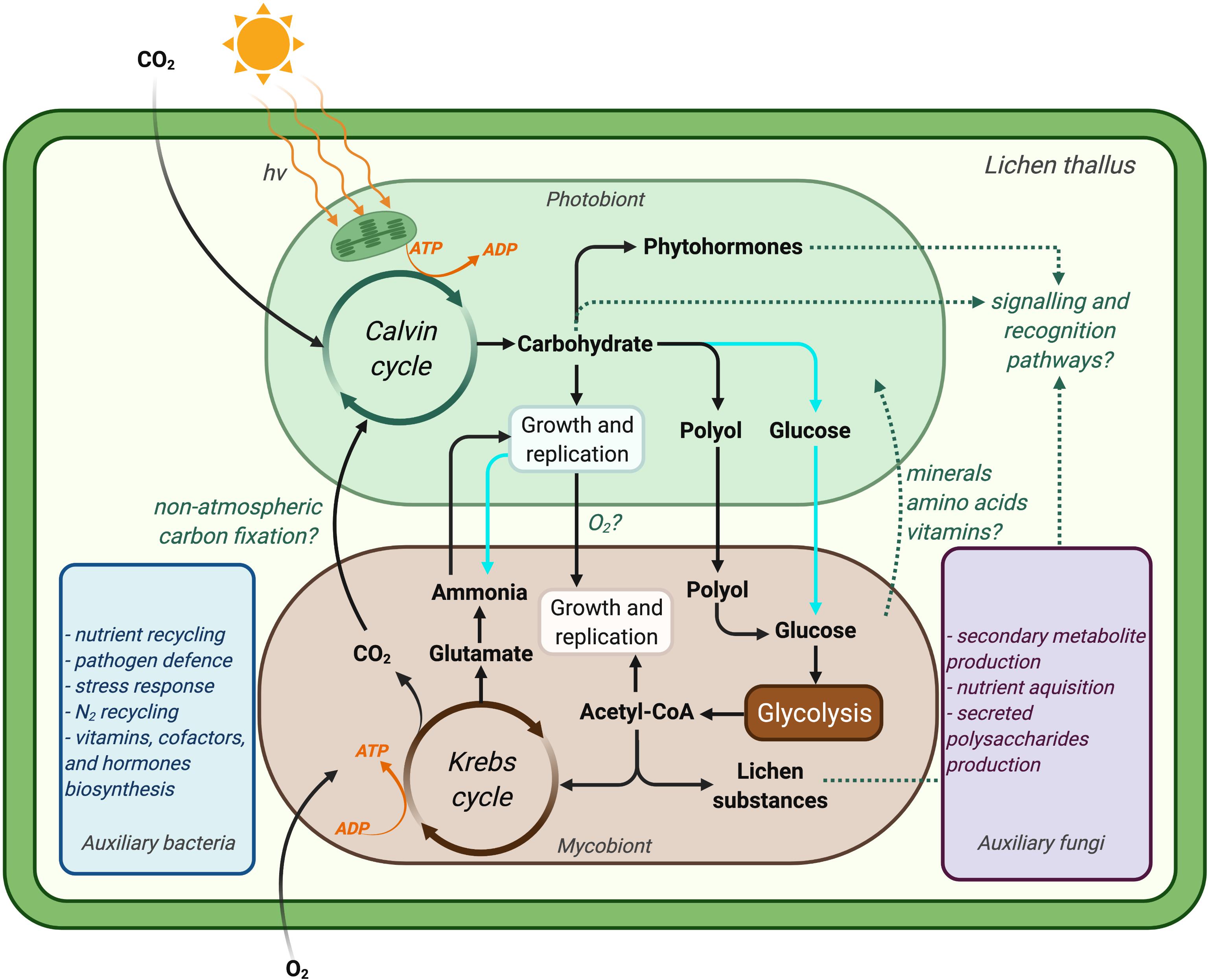
Figure 4. Simplified illustration of a lichen symbiosis showing our current understanding of the metabolic interactions between lichen symbionts. Dotted line indicates knowledge gaps that may not be generalisable to all lichens. Atmospheric carbon is fixed to carbohydrates by the photobiont at the expense of ATP, which is generated by photosynthesis. Chlorolichens convert carbohydrate to polyols and transport it to their respective mycobionts, whereas cyanolichens (cyan pathways) translocate carbohydrate in the form of glucose to their mycobionts. Mycobiont metabolism is fuelled by such carbohydrate translocation, which results in the provisioning of nitrogen for the photobiont (in case for chlorolichens). Recent studies also suggest coupled metabolisms for lichen symbionts with respect to the supply of oxygen (in addition to carbohydrates) by photobionts in return for carbon dioxide from their mycobionts (Schwartzman, 2010; Hom and Murray, 2014; ten Veldhuis et al., 2020). The production of lichen substances (mainly by mycobionts) and the production of phytohormones and/or carbohydrates by photobionts are believed to play key roles in the recognition of symbionts. The proposed functions of auxiliary lichen bacteria and fungi during the symbiosis are also indicated based on comprehensive multi-omics studies (blue and purple rectangles). It is speculated that the lichen microbiome forms a biofilm on the surface of the mycobiont, through which they communicate (Image created with BioRender.com).
Thus, despite of being broadly recognised that carbohydrates and inorganic molecules are exchanged between lichen symbionts, a systems-level molecular understanding of metabolism is still lacking for lichens, including their primary symbionts and auxiliary partners. This lack has left key features of metabolism unexplored, including, for example, central aspects of carbon metabolism with respect to lichen compartmentalisation or the role of cell wall components and biosynthesis on the growth and metabolite exchange between symbionts. A systems-level understanding of lichen metabolism will become more likely in near future in light of the recent insights on lichen microbiota composition and functions within the lichen symbiosis (Spribille et al., 2016; Cernava et al., 2017; Smith et al., 2020).
Rhizobiales have been found to be a dominant bacterial order in the microbiome of various terrestrial or marine lichens examined to date (Grube et al., 2009; Hodkinson and Lutzoni, 2009; Hodkinson et al., 2012; Erlacher et al., 2015). Specifically, Rhodospirillales were found to be common in chlorolichens, and Sphingomonadales and Bacteroidetes in cyanolichens (Hodkinson et al., 2012; Graham et al., 2018; West et al., 2018). Several factors are believed to influence lichen-associated bacterial community composition and diversity. These include the nature of lichen secondary metabolites (driven mainly by the type of primary mycobiont), large-scale geography, growth type, and the type of primary lichen photobiont (Grube et al., 2009; Hodkinson et al., 2012; Aschenbrenner et al., 2016). Some of these auxiliary bacteria were thought to be able to fix atmospheric nitrogen and, as cyanobacterial photobionts, might play an important role as a nitrogen source for the lichen symbiosis (Hodkinson and Lutzoni, 2009). Additionally, cyanobacterial lichens, which often grow in nitrogen-limited environments, were shown to harbour a diversity of bacteria that would otherwise not grow in such nitrogen-limited environments (Hodkinson et al., 2012). Apart from nitrogen fixation, meta-omics (e.g., meta-genomics, meta-transcriptomics, and meta-proteomics) studies have revealed functional roles for the microbiome of the lichen Lobaria pulmonaria, including: nutrient recycling in the decaying parts of the lichen thallus, pathogen defence, detoxification processes, protection against oxidative stress, biosynthesis of vitamins, cofactors, and hormones, activation of ketone metabolism during dehydration, and upregulated transcription of transport systems, tRNA modification and various porins during hydration (Cernava et al., 2015; Grube et al., 2015; Aschenbrenner et al., 2016; Sigurbjornsdottir et al., 2016; Cernava et al., 2017; Cernava et al., 2019). The role of these auxiliary bacteria is thus critical to the maintenance and functioning of the lichen symbiosis.
The large diversity of lichen-associated fungi has been revealed through culture-dependent methods first (Petrini et al., 1990; Arnold et al., 2009), then meta-omics data analyses (Spribille et al., 2016; Smith et al., 2020). The low biomass of these auxiliary fungi relative to the primary mycobiont and the inability to culture them have prevented a detailed analysis of their functional roles in the lichen symbiosis. However, based on the analysis of meta-genome of the lichen Alectoria sarmentosa, a recent study showed that auxiliary fungi (two basidiomycete yeasts) may play roles in producing secreted extracellular polysaccharides, lichen nutrient acquisition, and secondary metabolite production (Tagirdzhanova et al., 2021). They are therefore also likely to play an important role in the maintenance and functioning of the lichen symbiosis.
Although meta-omics analyses of lichen microbiomes have provided invaluable insights on the diversity and function of multi-species lichen symbioses, constraint-based metabolic modelling could potentially enable a deeper understanding of the multi-species metabolic interplay. For example, by applying a systems biology approach using genome-scale metabolic reconstructions for 773 human gut bacteria (AGORA), a more sophisticated understanding of the interactions between the host and gut microbiome was achieved, revealing how system responses depended upon the metabolic potential of each component species and the nutrients available (Magnusdottir et al., 2017). The AGORA framework confirmed that a high fibre diet (usually linked to a healthy microbiome) would result in higher proportion of commensal and mutualistic pair-wise interactions between gut microbes. This framework was able to show how the host-microbiome operates mechanistically and indicate how many positive interactions are sufficient to maintain a healthy gut community. A similar systems-level understanding of lichens could help in understanding the metabolic interdependency for symbiotic establishment and maintenance, and in predicting the role of associated lichen microbes and lichen responses to environmental changes or likely environmental niches. This would also aid in re-creating/re-synthesizing lichens in vitro and using them for biotechnological applications.
Genome-Scale Metabolic Flux Modelling: Challenges and Opportunities for the Lichen Symbiosis
Genome-scale metabolic network models simulate the metabolism of a living cell as a collection of hundreds to thousands of biochemical reactions (forming metabolic pathways of an organism) and enable quantitative and gene-grounded predictions of phenotypes under different growth conditions (Varma and Palsson, 1994; Covert et al., 2001). This set of reactions is framed as a set of ordinary differential equations, in which the number of variables and equations are defined by the number of metabolites and reactions, respectively. Solving this system of equations under a given set of assumptions (e.g., net zero system flux or “flux balance”) allows for determining optimal fluxes for each reaction in the metabolic network. Specific constraints describing the physico-chemical, environmental, regulatory, and/or topological conditions of the metabolic network can be imposed to identify optimal flux distributions consistent with these assumptions (Price et al., 2004). Such constraint-based metabolic modelling enables a wide range of applications including, but not limited to, predicting cellular functions (e.g., energy production) (Edwards et al., 2001; Orth and Palsson, 2012; Bordbar et al., 2014), identifying optimal strains and culture media conditions for specific applications (Pharkya et al., 2004; Nazem-Bokaee and Senger, 2015), formulating metabolic/strain engineering strategies (Burgard et al., 2003; Chung et al., 2010; Kim and Reed, 2010; Ranganathan et al., 2010; Rocha et al., 2010; McAnulty et al., 2012; Yen et al., 2013; Kim et al., 2019), identifying drug targets (Kim et al., 2011, 2012; Angione, 2019; Gu et al., 2019), producing natural/non-natural chemicals and precursors (Yim et al., 2011; Ye et al., 2014; Nazem-Bokaee et al., 2016; Wei et al., 2017; Nazem-Bokaee and Maranas, 2018; Biz et al., 2019; Gu et al., 2019), creating knowledgebases of metabolic, genomic, and biodiversity information (Kumar et al., 2012; Pabinger et al., 2014; King et al., 2016; Nazem-Bokaee et al., 2017; Norsigian et al., 2020), and studying syntrophic/symbiotic communities (see below). Table 2 lists select examples of two-species metabolic models that have been studied.
Techniques developed for the characterisation of metabolic interactions among members of microbial communities based on genome-scale metabolic modelling can be classified into two main groups: lumped (also called enzyme soup, mixed bag, or metagenome-scale modelling (Chan et al., 2017a)) and compartmentalised (Biggs et al., 2015; Henry et al., 2016; Zomorrodi and Segre, 2016). The analysis of interactions in a microbial community can be performed under steady-state or dynamic conditions. While an extensive description of these techniques and their implementation can be found elsewhere (Biggs et al., 2015; Zomorrodi and Segre, 2016; Chan et al., 2017a; Ang et al., 2018; Garcia-Jimenez et al., 2021) and is beyond the scope of this review, it is worth broadly covering the general aim of each technique. The lumped modelling approach seeks to find optimal conditions that benefits the whole community (e.g., mutualistic symbiosis) by neglecting boundaries between members of the community (Taffs et al., 2009; Henry et al., 2016). The compartmentalised modelling approach, on the other hand, retains boundaries between members while also allowing individual members to share a compartment and transfer metabolites. For example, the compartmentalised modelling approach enables considering a member-level objective towards achieving a community-level objective by imposing a constant growth rate across all members for a community to ensure co-existence and stability (Chan et al., 2017b). Although computationally more expensive, the compartmentalised modelling approach also allows for the study of different types of species-species interactions (e.g., parasitism) (Zomorrodi and Maranas, 2012). A dynamic modelling approach enables predictions of changes in metabolites and biomass over time within the community and relies on kinetic data of uptake reactions. The dynamic approach has been extended to enable spatial analysis of communities, as in the COMETS (Computation Of Microbial Ecosystems in Time and Space) framework, which coupled metabolic with diffusion modelling and was applied to understand metabolite exchange within a three-member microbial community (Harcombe et al., 2014).
To our knowledge, no genome-scale metabolic network model has yet been constructed for any lichen association or its symbionts. With the first genomes of mycobionts (Park et al., 2013a, b, 2014a, b; Armstrong et al., 2018; Bertrand et al., 2018a; Wang et al., 2018) and photobionts (Armaleo et al., 2019) of several lichens assembled and more foreseen to come, it is a timely opportunity to understand the lichen symbiosis through the lens of genome-scale metabolic models. Since little is known about the metabolic response of lichens to different environmental conditions (e.g., light intensity, water content, nutrient availability, etc.), developing a metabolic network model could shed invaluable insights on symbiosis at the molecular level. Furthermore, the available computational tools for modelling community interactions could allow for predicting the role of a specific symbiont on the performance of a lichen under a known environmental perturbation (e.g., nutrient limitation). A lichen metabolic model could be used as the framework for the integration of ‘omics’ data obtained for lichens to test multiple hypotheses including, for example, the regulatory effect of different carbohydrates on the growth and exchange of metabolites between lichen symbiont. Since in vitro lichen re-synthesis is still hampered by the complexity of the lichenisation process, metabolic modelling could highlight potential metabolites that may need to be exchanged between symbionts as well as the metabolic pathways that may lead to successful differentiation and growth. Moreover, metabolic modelling could be used to examine the potential for symbiosis between various combinations of mycobionts and photobionts, and provide insights into the evolution of the lichen symbiosis. Validating predictions of flux distribution by community metabolic models could be a challenge, due to multi-compartmental nature of lichen symbiosis and difficulties in measuring fluxes through each compartment in vivo. However, recent advances in the field of metabolic flux analysis now make it possible to resolve fluxes by carefully designing the isotope labels and tracing them across different compartments (Schwechheimer et al., 2018). Another practical challenge for the development of lichen metabolic models may pertain to the characterisation of the cellular composition of individual lichen symbionts. For example, many lichen mycobionts grow slowly, making it experimentally difficult to obtain sufficient cell mass needed to formulate a ‘biomass’ reaction in a metabolic model representing cellular growth. Moreover, due to the lack of data specific to the metabolic pathways of lichens, the model curation process may be patchy, with irreconcilable gaps and network disconnects. However, metabolic models for lichens could be reconstructed by leveraging the ever-increasing number of high-quality metabolic models becoming available for not-too-distantly related filamentous fungi, microalgae, or cyanobacteria (Brandl and Andersen, 2015; Gomez et al., 2016; Santos-Merino et al., 2019).
Conclusion and Future Perspectives
Lichens, although historically well-known and iconic symbioses, still bear a sense of mystery as our understanding of the signalling networks and pathways responsible for their symbiotic establishment and maintenance is still in its infancy. Two signalling mechanisms were reviewed in this article but many more could be explored with the aid of techniques such as untargeted metabolomics. Signalling/metabolic network modelling approaches could support the field of experimental lichenology by providing insights into: (1) the signalling molecules and the roles they play at different stages of lichenisation, (2) how lichen symbionts benefit from the symbiosis with regards to carbon, nitrogen, and other limiting nutrients or environmental conditions, (3) which conditions allow lichens to produce secondary metabolites and the genes that are involved, and (4) how lichens manage to accumulate and tolerate high levels of toxic metals. Advances in DNA sequencing technologies in recent years have significantly reduced the cost of generating genome sequences. At the same time, improvements in high performance computing and development of more biologist-friendly tools for modelling and analysing ‘genome-scale’ metabolic networks have enabled the exploration of metabolically-coupled microbial communities. Combining these genome resources and systems biology tools could open up a whole new era for the study of the lichen symbiosis.
Author Contributions
HN-B and CG conceptualised and wrote the manuscript. HN-B and CG designed and created figures. EFYH, ACW, and SM revised the manuscript. All authors read and approved the final manuscript.
Funding
This work was supported by funding from the CSIRO Synthetic Biology Future Science Platform (FSP), Grant # OD-206013. EFYH was funded in part by NSF grants #1541538 and #1846376.
Conflict of Interest
The authors declare that the research was conducted in the absence of any commercial or financial relationships that could be construed as a potential conflict of interest.
Acknowledgments
We would like to thank Colin Scott (CSIRO) as well as the reviewers for critical reading and suggesting constructive improvements to the manuscript.
References
Ahmadjian, V., Jacobs, J. B., and Russell, L. A. (1978). Scanning electron-microscope study of early lichen synthesis. Science 200, 1062–1064. doi: 10.1126/science.200.4345.1062
Ang, K. S., Lakshmanan, M., Lee, N. R., and Lee, D. Y. (2018). Metabolic modeling of microbial community interactions for health, environmental and biotechnological applications. Curr. Genom. 19, 712–722. doi: 10.2174/1389202919666180911144055
Angione, C. (2019). Human systems biology and metabolic modelling: a reviewfrom disease metabolism to precision medicine. Biomed. Res. Intern. 2019:8304260.
Antebi, Y. E., Nandagopal, N., and Elowitz, M. B. (2017). An operational view of intercellular signaling pathways. Curr. Opin. Syst. Biol. 1, 16–24. doi: 10.1016/j.coisb.2016.12.003
Armaleo, D., Muller, O., Lutzoni, F., Andresson, O. S., Blanc, G., Bode, H. B., et al. (2019). The lichen symbiosis re-viewed through the genomes of Cladonia grayi and its algal partner Asterochloris glomerata. BMC Genom. 20:605. doi: 10.1186/s12864-019-5629-x
Armstrong, E. E., Prost, S., Ertz, D., Westberg, M., Frisch, A., and Bendiksby, M. (2018). Draft genome sequence and annotation of the lichen-forming fungus Arthonia radiata. Genome Announc. 6:e0281-18.
Arnold, A. E., Miadlikowska, J., Higgins, K. L., Sarvate, S. D., Gugger, P., Way, A., et al. (2009). A phylogenetic estimation of trophic transition networks for Ascomycetous fungi: are lichens cradles of symbiotrophic fungal diversification? Syst. Biol. 58, 283–297. doi: 10.1093/sysbio/syp001
Aschenbrenner, I. A., Cernava, T., Berg, G., and Grube, M. (2016). Understanding microbial multi-species symbioses. Front. Microbiol. 7:180. doi: 10.3389/fmicb.2016.00180
Athukorala, S. N. P., Huebner, E., and Piercey-Normore, M. D. (2014). Identification and comparison of the 3 early stages of resynthesis for the lichen Cladonia rangiferina. Can. J. Microbiol. 60, 41–52. doi: 10.1139/cjm-2013-0313
Athukorala, S. N. P., and Piercey-Normore, M. D. (2014). Recognition between Cladonia rangiferina and the algal partner during resynthesis. Bot. Botaniq. 92, 640–640.
Athukorala, S. N. P., and Piercey-Normore, M. D. (2015). Recognition- and defense-related gene expression at 3 resynthesis stages in lichen symbionts. Can. J. Microbiol. 61, 1–12. doi: 10.1139/cjm-2014-0470
Aubert, S., Juge, C., Boisson, A. M., Gout, E., and Bligny, R. (2007). Metabolic processes sustaining the reviviscence of lichen Xanthoria elegans (Link) in high mountain environments. Planta 226, 1287–1297. doi: 10.1007/s00425-007-0563-6
Beckett, R. P., Kranner, I., and Minibayeva, F. V. (2008). “Stress physiology and the symbiosis,” in Lichen Biology, 2nd Edn, ed. T. H. Nash (Cambridge: Cambridge University Press), 134–151. doi: 10.1017/cbo9780511790478.009
Bertrand, R. L., Abdel-Hameed, M., and Sorensen, J. L. (2018a). Lichen biosynthetic gene clusters. Part I. Genome sequencing reveals a rich biosynthetic potential. J. Nat. Prod. 81, 723–731. doi: 10.1021/acs.jnatprod.7b00769
Bertrand, R. L., Abdel-Hameed, M., and Sorensen, J. L. (2018b). Lichen biosynthetic gene clusters Part II: Homology mapping suggests a functional diversity. J. Nat. Prod. 81, 732–748. doi: 10.1021/acs.jnatprod.7b00770
Biggs, M. B., Medlock, G. L., Kolling, G. L., and Papin, J. A. (2015). Metabolic network modeling of microbial communities. Wiley Interdiscip. Rev. Syst. Biol. Med. 7, 317–334. doi: 10.1002/wsbm.1308
Biz, A., Proulx, S., Xu, Z., Siddartha, K., Mulet Indrayanti, A., and Mahadevan, R. (2019). Systems biology based metabolic engineering for non-natural chemicals. Biotechnol. Adv. 37:107379. doi: 10.1016/j.biotechadv.2019.04.001
Bonfante, P., and Genre, A. (2010). Mechanisms underlying beneficial plant-fungus interactions in mycorrhizal symbiosis. Nat. Commun. 1:48.
Bonfante, P., and Requena, N. (2011). Dating in the dark: how roots respond to fungal signals to establish arbuscular mycorrhizal symbiosis. Curr. Opin. Plant Biol. 14, 451–457. doi: 10.1016/j.pbi.2011.03.014
Bordbar, A., Monk, J. M., King, Z. A., and Palsson, B. O. (2014). Constraint-based models predict metabolic and associated cellular functions. Nat. Rev. Genet. 15, 107–120. doi: 10.1038/nrg3643
Brakni, R., Ahmed, M. A., Burger, P., Schwing, A., Michel, G., Pomares, C., et al. (2018). UHPLC-HRMS/MS based profiling of algerian lichens and their antimicrobial activities. Chem. Biodiver. 15:e1800031. doi: 10.1002/cbdv.201800031
Brandl, J., and Andersen, M. R. (2015). Current state of genome-scale modeling in filamentous fungi. Biotechnol. Lett. 37, 1131–1139. doi: 10.1007/s10529-015-1782-8
Bubrick, P., and Galun, M. (1980). Proteins from the lichen Xanthoria parietina which bind to phycobiont cell walls correlation between binding patterns and cell wall Cyto Chemistry. Protoplasma 104, 167–173. doi: 10.1007/bf01279379
Bubrick, P., Galun, M., and Frensdorff, A. (1981). Proteins from the lichen Xanthoria parietina which bind to phycobiont cell walls localization in the intact lichen and cultured mycobiont. Protoplasma 105, 207–211. doi: 10.1007/bf01279219
Burgard, A. P., Pharkya, P., and Maranas, C. D. (2003). OptKnock: a bilevel programming framework for identifying gene knockout strategies for microbial strain optimization. Biotechnol. Bioeng. 84, 647–657. doi: 10.1002/bit.10803
Calcott, M. J., Ackerley, D. F., Knight, A., Keyzers, R. A., and Owen, J. G. (2018). Secondary metabolism in the lichen symbiosis. Chem. Soc. Rev. 47, 1730–1760. doi: 10.1039/c7cs00431a
Cernava, T., Aschenbrenner, I. A., Soh, J., Sensen, C. W., Grube, M., and Berg, G. (2019). Plasticity of a holobiont: desiccation induces fasting-like metabolism within the lichen microbiota. ISME J. 13, 547–556. doi: 10.1038/s41396-018-0286-7
Cernava, T., Erlacher, A., Aschenbrenner, I. A., Krug, L., Lassek, C., Riedel, K., et al. (2017). Deciphering functional diversification within the lichen microbiota by meta-omics. Microbiome 5:82.
Cernava, T., Muller, H., Aschenbrenner, I. A., Grube, M., and Berg, G. (2015). Analyzing the antagonistic potential of the lichen microbiome against pathogens by bridging metagenomic with culture studies. Front. Microbiol. 6:620. doi: 10.3389/fmicb.2015.00620
Chan, S. H. J., Simons, M., and Maranas, C. D. (2017a). Computational modeling of microbial communities. Syst. Biol. 163–189. doi: 10.1002/9783527696130.ch6
Chan, S. H. J., Simons, M. N., and Maranas, C. D. (2017b). SteadyCom: predicting microbial abundances while ensuring community stability. PLoS Comput. Biol. 13:e1005539. doi: 10.1371/journal.pcbi.1005539
Chung, B. K., Selvarasu, S., Andrea, C., Ryu, J., Lee, H., Ahn, J., et al. (2010). Genome-scale metabolic reconstruction and in silico analysis of methylotrophic yeast Pichia pastoris for strain improvement. Microb. Cell Fact. 9:50. doi: 10.1186/1475-2859-9-50
Clear, M. R., and Hom, E. F. (2019). The evolution of symbiotic plant–microbe signalling. Annu. Plant Rev. 2:684.
Covert, M. W., Schilling, C. H., Famili, I., Edwards, J. S., Goryanin, I. I., Selkov, E., et al. (2001). Metabolic modeling of microbial strains in silico. Trends Biochem. Sci. 26, 179–186. doi: 10.1016/s0968-0004(00)01754-0
Dahlman, L., Persson, J., Palmqvist, K., and Nasholm, T. (2004). Organic and inorganic nitrogen uptake in lichens. Planta 219, 459–467.
Dasika, M. S., Burgard, A., and Maranas, C. D. (2006). A computational framework for the topological analysis and targeted disruption of signal transduction networks. Biophys. J. 91, 382–398. doi: 10.1529/biophysj.105.069724
Díaz, E., Sánchez Elordi, E., Santiago, R., Vicente Córdoba, C., and Legaz González, M. E. (2016a). Algal-fungal mutualism: cell recognition and maintenance of the symbiotic status of lichens. J. Vet. Med. Res. 3:1052.
Diaz, E. M., Ampe, C., Van Troys, M., Vicente-Manzanares, M., Legaz, M. E., and Vicente, C. (2016b). An actomyosin-like cytoskeleton in the cyanobiont (Nosctoc sp.) of Peltigera canina. Phytochem. Lett. 16, 249–256. doi: 10.1016/j.phytol.2016.05.005
Diaz, E. M., Cutrona, C., Sanchez-Elordi, E., Legaz, M. E., and Vicente, C. (2016c). Direct and cross-recognition of lichenized Trebouxia puymaly (Chlorophyta, Trebouxiophyceae) and Nostoc Vaucher ex Bornet (Cyanobacteria, Cyanophyceae) by their homologous and heterologous fungal lectins. Braz. J. Bot. 39, 507–518. doi: 10.1007/s40415-016-0268-9
Diaz, E. M., Sacristan, M., Legaz, M. E., and Vicente, C. (2009). Isolation and characterization of a cyanobacterium-binding protein and its cell wall receptor in the lichen Peltigera canina. Plant Signal. Behav. 4, 598–603. doi: 10.4161/psb.4.7.9164
Diaz, E. M., Vicente-Manzanares, M., Legaz, M. E., and Vicente, C. (2015). A cyanobacterial beta-actin-like protein, responsible for lichenized Nostoc sp motility towards a fungal lectin. Acta Physiol. Plant. 37:249.
Diaz, E. M., Vicente-Manzanares, M., Sacristan, M., Vicente, C., and Legaz, M. E. (2011). Fungal lectin of Peltigera canina induces chemotropism of compatible Nostoc cells by constriction-relaxation pulses of cyanobiont cytoskeleton. Plant Signal. Behav. 6, 1525–1536. doi: 10.4161/psb.6.10.16687
diCenzo, G. C., Zamani, M., Checcucci, A., Fondi, M., Griffitts, J. S., Finan, T. M., et al. (2019). Multidisciplinary approaches for studying rhizobium-legume symbioses. Can. J. Microbiol. 65, 1–33. doi: 10.1139/cjm-2018-0377
Drew, E. A., and Smith, D. C. (1967a). Studies in physiology of Lichens 7. Physiology of Nostoc Symbiont of Peltigera polydactyla compared with cultured and free-living forms. New Phytol. 66, 379–388. doi: 10.1111/j.1469-8137.1967.tb06017.x
Drew, E. A., and Smith, D. C. (1967b). Studies in physiology of Lichens.8. Movement of glucose from alga to fungus during photosynthesis in thallus of Peltigera polydactyla. New Phytol. 66, 389–389. doi: 10.1111/j.1469-8137.1967.tb06018.x
Edwards, J. S., Ibarra, R. U., and Palsson, B. O. (2001). In silico predictions of Escherichia coli metabolic capabilities are consistent with experimental data. Nat. Biotechnol. 19, 125–130.
Eisenreich, W., Knispel, N., and Beck, A. (2011). Advanced methods for the study of the chemistry and the metabolism of lichens. Phytochem. Rev. 10, 445–456. doi: 10.1007/s11101-011-9215-3
Elix, J. A., and Stocker-Worgotter, E. (2008). “Biochemistry and secondary metabolites,” in Lichen Biology, 2nd Edn, ed. T. H. Nash III (Cambridge: Cambridge University Press), 104–133. doi: 10.1017/cbo9780511790478.008
El-Semman, I. E., Karlsson, F. H., Shoaie, S., Nookaew, I., Soliman, T. H., and Nielsen, J. (2014). Genome-scale metabolic reconstructions of Bifidobacterium adolescentis L2-32 and Faecalibacterium prausnitzii A2-165 and their interaction. BMC Syst. Biol. 8:41. doi: 10.1186/1752-0509-8-41
Elshobary, M. E., Osman, M. E. H., Abushady, A. M., and Piercey-Normore, M. D. (2015). Comparison of lichen-forming cyanobacterial and green algal photobionts with free-living algae. Cryptogam. Algol. 36, 81–100. doi: 10.7872/crya.v36.iss1.2015.81
Epstein, E., Sagee, O., Cohen, J. D., and Garty, J. (1986). Endogenous auxin and ethylene in the lichen Ramalina duriaei. Plant Physiol. 82, 1122–1125. doi: 10.1104/pp.82.4.1122
Erlacher, A., Cernava, T., Cardinale, M., Soh, J., Sensen, C. W., Grube, M., et al. (2015). Rhizobiales as functional and endosymbiontic members in the lichen symbiosis of Lobaria pulmonaria L. Front. Microbiol. 6:53. doi: 10.3389/fmicb.2015.00053
Fondi, M., and Di Patti, F. (2019). A synthetic ecosystem for the multi-level modelling of heterotroph-phototroph metabolic interactions. Ecol. Model. 399, 13–22. doi: 10.1016/j.ecolmodel.2019.02.012
Garcia-Jimenez, B., Torres-Bacete, J., and Nogales, J. (2021). Metabolic modelling approaches for describing and engineering microbial communities. Comput. Struct. Biotechnol. J. 19, 226–246. doi: 10.1016/j.csbj.2020.12.003
Goga, M., Eleèko, J., Marcinèinová, M., Ruèová, D., Baèkorová, M., and Baèkor, M. (2020). “Lichen metabolites: an overview of some secondary metabolites and their biological potential,” in Co-Evolution of Secondary Metabolites, eds J. M. Merillon and K. Ramawat (Cham: Springer), 175–209. doi: 10.1007/978-3-030-16814-8_6
Gomez, J. A., Hoffner, K., and Barton, P. I. (2016). From sugars to biodiesel using microalgae and yeast. Green Chem. 18, 461–475. doi: 10.1039/c5gc01843a
Graham, L. E., Trest, M. T., Will-Wolf, S., Miicke, N. S., Atonio, L. M., Piotrowski, M. J., et al. (2018). Microscopic and metagenomic analyses of Peltigera ponojensis (Peltigerales, Ascomycota). Intern. J. Plant Sci. 179, 241–255. doi: 10.1086/696534
Green, T. G. A., Nash, T. H., and Lange, O. L. (2008). “Physiological ecology of carbon dioxide exchange,” in Lichen Biology, 2nd Edn, eds T. G. A. Green, T. H. Nash, and O. L. Lange (Cambridge: Cambridge University Press), 152–181. doi: 10.1017/cbo9780511790478.010
Green, T. G. A., and Smith, D. C. (1974). Lichen Physiology XIV. Differences between lichen algae in symbiosis and in isolation. New Phytol. 73, 753–766. doi: 10.1111/j.1469-8137.1974.tb01303.x
Grube, M., Cardinale, M., De Castro, J. V., Muller, H., and Berg, G. (2009). Species-specific structural and functional diversity of bacterial communities in lichen symbioses. ISME J. 3, 1105–1115. doi: 10.1038/ismej.2009.63
Grube, M., Cernava, T., Soh, J., Fuchs, S., Aschenbrenner, I., Lassek, C., et al. (2015). Exploring functional contexts of symbiotic sustain within lichen-associated bacteria by comparative omics. ISME J. 9, 412–424. doi: 10.1038/ismej.2014.138
Gu, C., Kim, G. B., Kim, W. J., Kim, H. U., and Lee, S. Y. (2019). Current status and applications of genome-scale metabolic models. Genome Biol. 20:121.
Gueidan, C., Ruibal, C., De Hoog, G. S., and Schneider, H. (2011). Rock-inhabiting fungi originated during periods of dry climate in the late devonian and middle triassic. Fungal Biol. 115, 987–996. doi: 10.1016/j.funbio.2011.04.002
Gueidan, C., Villasenor, C. R., De Hoog, G. S., Gorbushina, A. A., Untereiner, W. A., and Lutzoni, F. (2008). A rock-inhabiting ancestor for mutualistic and pathogen-rich fungal lineages. Stud. Mycol. 61, 111–119. doi: 10.3114/sim.2008.61.11
Hanly, T. J., and Henson, M. A. (2013). Dynamic metabolic modeling of a microaerobic yeast co-culture: predicting and optimizing ethanol production from glucose/xylose mixtures. Biotechnol. Biofuels 6:44. doi: 10.1186/1754-6834-6-44
Harcombe, W. R., Riehl, W. J., Dukovski, I., Granger, B. R., Betts, A., Lang, A. H., et al. (2014). Metabolic resource allocation in individual microbes determines ecosystem interactions and spatial dynamics. Cell Rep. 7, 1104–1115. doi: 10.1016/j.celrep.2014.03.070
Henry, C. S., Bernstein, H. C., Weisenhorn, P., Taylor, R. C., Lee, J. Y., Zucker, J., et al. (2016). Microbial community metabolic modeling: a community data-driven network reconstruction. J. Cell. Physiol. 231, 2339–2345. doi: 10.1002/jcp.25428
Hodkinson, B. P., Gottel, N. R., Schadt, C. W., and Lutzoni, F. (2012). Photoautotrophic symbiont and geography are major factors affecting highly structured and diverse bacterial communities in the lichen microbiome. Environ. Microbiol. 14, 147–161. doi: 10.1111/j.1462-2920.2011.02560.x
Hodkinson, B. P., and Lutzoni, F. (2009). A microbiotic survey of lichen-associated bacteria reveals a new lineage from the Rhizobiales. Symbiosis 49, 163–180. doi: 10.1007/s13199-009-0049-3
Hofstetter, V., Miadlikowska, J., Kauff, F., and Lutzoni, F. (2007) Phylogenetic comparison of protein-coding versus ribosomal RNA-coding sequence data: A case study of the Lecanoromycetes (Ascomycota). Mol. Phylogenet Evol. 44, 412–426. doi: 10.1016/j.ympev.2006.10.016
Hom, E. F. Y., and Murray, A. W. (2014). Niche engineering demonstrates a latent capacity for fungal-algal mutualism. Science 345, 94–98. doi: 10.1126/science.1253320
Honegger, R. (1998). The Lichen symbiosis - What is so spectacular about it? Lichenologist 30, 193–212. doi: 10.1017/s002428299200015x
Honegger, R., Kutasi, V., and Ruffner, H. P. (1993). Polyol patterns in 11 species of aposymbiotically cultured lichen mycobionts. Mycol. Res. 97, 35–39. doi: 10.1016/s0953-7562(09)81109-x
Huisman, R., and Geurts, R. (2020). A roadmap toward engineered nitrogen-fixing nodule symbiosis. Plant Commun. 1:100019. doi: 10.1016/j.xplc.2019.100019
Hyduke, D. R., and Palsson, B. O. (2010). Towards genome-scale signalling network reconstructions. Nat. Rev. Genet. 11, 297–307. doi: 10.1038/nrg2750
Jenkins, B., and Richards, T. A. (2019). Symbiosis: wolf lichens harbour a choir of fungi. Curr. Biol. 29, R88–R90.
Joneson, S., Armaleo, D., and Lutzoni, F. (2011). Fungal and algal gene expression in early developmental stages of lichen-symbiosis. Mycologia 103, 291–306. doi: 10.3852/10-064
Kappen, L. (2000). Some aspects of the great success of lichens in Antarctica. Antarct. Sci. 12, 314–324. doi: 10.1017/s0954102000000377
Kardish, N., Silberstein, L., Fleminger, G., and Galun, M. (1991). Lectin from the lichen Nephroma laevigatum Ach localization and function. Symbiosis 11, 47–62.
Kennedy, J. F., Palva, P. M. G., Corella, M. T. S., Cavalcanti, M. S. M., and Coelho, L. C. B. B. (1995). Lectins, versatile proteins of recognition - a review. Carbohydr. Polym. 26, 219–230. doi: 10.1016/0144-8617(94)00091-7
Kim, H. U., Kim, S. Y., Jeong, H., Kim, T. Y., Kim, J. J., Choy, H. E., et al. (2011). Integrative genome-scale metabolic analysis of Vibrio vulnificus for drug targeting and discovery. Mol. Syst. Biol. 7:460. doi: 10.1038/msb.2010.115
Kim, H. U., Sohn, S. B., and Lee, S. Y. (2012). Metabolic network modeling and simulation for drug targeting and discovery. Biotechnol. J. 7, 330–342. doi: 10.1002/biot.201100159
Kim, J., and Reed, J. L. (2010). OptORF: optimal metabolic and regulatory perturbations for metabolic engineering of microbial strains. BMC Syst. Biol. 4:53. doi: 10.1186/1752-0509-4-53
Kim, M., Park, B. G., Kim, E. J., Kim, J., and Kim, B. G. (2019). In silico identification of metabolic engineering strategies for improved lipid production in Vibrio vulnificus by genome-scale metabolic modeling. Biotechnol. Biofuels 12:187.
King, Z. A., Lu, J., Drager, A., Miller, P., Federowicz, S., Lerman, J. A., et al. (2016). BiGG Models: a platform for integrating, standardizing and sharing genome-scale models. Nucleic Acids Res. 44, D515–D522.
Kitano, H. (2002b). Systems biology: a brief overview. Science 295, 1662–1664. doi: 10.1126/science.1069492
Kono, M., Kon, Y., Ohmura, Y., Satta, Y., and Terai, Y. (2020). In vitro resynthesis of lichenization reveals the genetic background of symbiosis-specific fungal-algal interaction in Usnea hakonensis. BMC Genom. 21:671. doi: 10.1186/s12864-020-07086-9
Kono, M., Tanabe, H., Ohmura, Y., Satta, Y., and Terai, Y. (2017). Physical contact and carbon transfer between a lichen-forming Trebouxia alga and a novel Alphaproteobacterium. Microbiol. SGM 163, 678–691. doi: 10.1099/mic.0.000461
Kranner, I., Beckett, R., Hochman, A., and Nash, T. H. (2008). Desiccation-tolerance in lichens: a review. Bryologist 111, 576–593. doi: 10.1639/0007-2745-111.4.576
Kuhn, V., Geisberger, T., Huber, C., Beck, A., and Eisenreich, W. (2019). A facile in vivo procedure to analyze metabolic pathways in intact lichens. New Phytol. 224, 1657–1667. doi: 10.1111/nph.15968
Kumar, A., Suthers, P. F., and Maranas, C. D. (2012). MetRxn: a knowledgebase of metabolites and reactions spanning metabolic models and databases. BMC Bioinform. 13:6. doi: 10.1186/1471-2105-13-
Lavrik, I. N. S., and Samsonova, M. G. (2016). The systems biology of signaling pathways. Biophysics 61, 78–84.
Legaz, M. E., Fontaniella, B., Millanes, A. M., and Vicente, C. (2004). Secreted arginases from phylogenetically far-related lichen species act as cross-recognition factors for two different algal cells. Eur. J. Cell Biol. 83, 435–446. doi: 10.1078/0171-9335-00384
Lehr, H., Fleminger, G., and Galun, M. (1995). Lectin from the lichen Peltigera membranacea (Ach) Nyl - characterization and function. Symbiosis 18, 1–13.
Leiva, D., Fernandez-Mendoza, F., Acevedo, J., Caru, M., Grube, M., and Orlando, J. (2021). The bacterial community of the foliose macro-lichen Peltigera frigida is more than a mere extension of the microbiota of the subjacent substrate. Microb. Ecol. doi: 10.1007/s00248-020-01662-y. Epub ahead of print.
Lendemer, J. C., Keepers, K. G., Tripp, E. A., Pogoda, C. S., Mccain, C. M., and Kane, N. C. (2019). A taxonomically broad metagenomic survey of 339 species spanning 57 families suggests cystobasidiomycete yeasts are not ubiquitous across all lichens. Am. J. Bot. 106, 1090–1095.
Li, F., Thiele, I., Jamshidi, N., and Palsson, B. O. (2009). Identification of potential pathway mediation targets in toll-like receptor signaling. PLoS Computat. Biol. 5:e1000292. doi: 10.1371/journal.pcbi.1000292
Lines, C. E. M., Ratcliffe, R. G., Rees, T. A. V., and Southon, T. E. (1989). A C-13 Nmr-study of photosynthate transport and metabolism in the lichen Xanthoria calcicola Oxner. New Phytol. 111, 447–456. doi: 10.1111/j.1469-8137.1989.tb00707.x
Lockhart, C. M., Rowell, P., and Stewart, W. D. P. (1978). Phytohemagglutinins from nitrogen-fixing lichens Peltigera canina and Peltigera polydactyla. FEMS Microbiol. Lett. 3, 127–130.
Lutzoni, F., Nowak, M. D., Alfaro, M. E., Reeb, V., Miadlikowska, J., Krug, M., et al. (2018). Contemporaneous radiations of fungi and plants linked to symbiosis. Nat. Commun. 9:5489.
Magnusdottir, S., Heinken, A., Kutt, L., Ravcheev, D. A., Bauer, E., Noronha, A., et al. (2017). Generation of genome-scale metabolic reconstructions for 773 members of the human gut microbiota. Nat. Biotechnol. 35, 81–89. doi: 10.1038/nbt.3703
Manoharan, S. S., Miao, V. P., and Andresson, O. S. (2012). LEC-2, a highly variable lectin in the lichen Peltigera membranacea. Symbiosis 58, 91–98. doi: 10.1007/s13199-012-0206-y
Martin, F. M., Uroz, S., and Barker, D. G. (2017). Ancestral alliances: plant mutualistic symbioses with fungi and bacteria. Science 356:eaad4501. doi: 10.1126/science.aad4501
McAnulty, M. J., Yen, J. Y., Freedman, B. G., and Senger, R. S. (2012). Genome-scale modeling using flux ratio constraints to enable metabolic engineering of clostridial metabolism in silico. BMC Syst. Biol. 6:42. doi: 10.1186/1752-0509-6-42
Meessen, J., Eppenstein, S., and Ott, S. (2013). Recognition mechanisms during the pre-contact state of lichens: II. Influence of algal exudates and ribitol on the response of the mycobiont of Fulgensia bracteata. Symbiosis 59, 131–143. doi: 10.1007/s13199-012-0219-6
Meessen, J., and Ott, S. (2013). Recognition mechanisms during the pre-contact state of lichens: I. Mycobiont-photobiont interactions of the mycobiont of Fulgensia bracteata. Symbiosis 59, 121–130. doi: 10.1007/s13199-013-0232-4
Mellbye, B. L., Giguere, A. T., Murthy, G. S., Bottomley, P. J., Sayavedra-Soto, L. A., and Chaplen, F. W. R. (2018). Genome-scale, constraint-based modeling of nitrogen oxide fluxes during coculture of Nitrosomonas europaea and Nitrobacter winogradskyi. mSystems 3:e0170-17.
Merino, M. P., Andrews, B. A., and Asenjo, J. A. (2015). Stoichiometric model and flux balance analysis for a mixed culture of Leptospirillum ferriphilum and Ferroplasma acidiphilum. Biotechnol. Prog. 31, 307–315. doi: 10.1002/btpr.2028
Miao, V. P. W., Manoharan, S. S., Snaebjarnarson, V., and Andresson, O. S. (2012). Expression of lec-1, a mycobiont gene encoding a galectin-like protein in the lichen Peltigera membranacea. Symbiosis 57, 23–31. doi: 10.1007/s13199-012-0175-1
Millanes, A. M., Fontaniella, B., Garcia, M. L., Solas, M. T., Vicente, C., and Legaz, M. E. (2004). Cytochemical location of urease in the cell wall of two different lichen phycobionts. Tissue Cell 36, 373–377. doi: 10.1016/j.tice.2004.06.007
Mittermeier, V. K., Schmitt, N., Volk, L. P. M., Suarez, J. P., Beck, A., and Eisenreich, W. (2015). Metabolic profiling of alpine and ecuadorian lichens. Molecules 20, 18047–18065. doi: 10.3390/molecules201018047
Mohanta, T. K., and Bae, H. (2015). Functional genomics and signaling events in mycorrhizal symbiosis. J. Plant Interact. 10, 21–40. doi: 10.1080/17429145.2015.1005180
Molina, M. C., Stocker-Worgotter, E., Turk, R., Bajon, C., and Vicente, C. (1998). Secreted, glycosylated arginase from Xanthoria parietina thallus induces loss of cytoplasmic material from Xanthoria photobionts. Cell Adhesion Commun. 6, 481–490. doi: 10.3109/15419069809010796
Molina, M. C., and Vicente, C. (1995). Correlationships between enzymatic-activity of lectins, putrescine content and chloroplast damage in Xanthoria parietina phycobionts. Cell Adhesion Commun. 3, 1–12. doi: 10.3109/15419069509081274
Molina, M. C., and Vicente, C. (2000). Purification and characterization of two isolectins with arginase activity from the lichen Xanthoria parietina. J. Biochem. Mol. Biol. 33, 300–307.
Molina, M. D., Muniz, E., and Vicente, C. (1993). Enzymatic-activities of algal-binding protein and its algal cell-wall receptor in the lichen Xanthoria parietina - an approach to the parasitic basis of mutualism. Plant Physiol. Biochem. 31, 131–142.
Morris, M. K., Saez-Rodriguez, J., Sorger, P. K., and Lauffenburger, D. A. (2010). Logic-based models for the analysis of cell signaling networks. Biochemistry 49, 3216–3224. doi: 10.1021/bi902202q
Nagarajan, H., Embree, M., Rotaru, A. E., Shrestha, P. M., Feist, A. M., Palsson, B. O., et al. (2013). Characterization and modelling of interspecies electron transfer mechanisms and microbial community dynamics of a syntrophic association. Nat. Commun. 4:2809.
Nash, T. H. (2008b). “Nitrogen, its metabolism and potential contribution to ecosystems,” in Lichen Biology, 2nd Edn, ed. T. H. Nash (Cambridge: Cambridge University Press), 216–233. doi: 10.1017/cbo9780511790478.012
Nazem-Bokaee, H., Gopalakrishnan, S., Ferry, J. G., Wood, T. K., and Maranas, C. D. (2016). Assessing methanotrophy and carbon fixation for biofuel production by Methanosarcina acetivorans. Microb. Cell Fact. 15:10.
Nazem-Bokaee, H., and Maranas, C. D. (2018). A prospective study on the fermentation landscape of gaseous substrates to biorenewables using Methanosarcina acetivorans metabolic model. Front. Microbiol. 9:1855. doi: 10.3389/fmicb.2018.01855
Nazem-Bokaee, H., and Senger, R. S. (2015). ToMI-FBA: a genome-scale metabolic flux based algorithm to select optimum hosts and media formulations for expressing pathways of interest. Aims Bioeng. 2, 335–374. doi: 10.3934/bioeng.2015.4.335
Nazem-Bokaee, H., Yen, J. Y., Athamneh, A. I. M., Apte, A. A., Mcanulty, M. J., and Senger, R. S. (2017). SyM-GEM: a pathway builder for genome-scale models. Adv. Biochem. Biotechnol. 2:141. doi: 10.29011/2574-7258.000041
Nelsen, M. P., Lucking, R., Boyce, C. K., Lumbsch, H. T., and Ree, R. H. (2019). No support for the emergence of lichens prior to the evolution of vascular plants. Geobiology 18, 3–13. doi: 10.1111/gbi.12369
Nelsen, M. P., Lucking, R., Boyce, C. K., Lumbsch, H. T., and Ree, R. H. (2020). The macroevolutionary dynamics of symbiotic and phenotypic diversification in lichens. Proc. Natl. Acad. Sci. U.S.A. 117, 21495–21503. doi: 10.1073/pnas.2001913117
Norsigian, C. J., Pusarla, N., Mcconn, J. L., Yurkovich, J. T., Drager, A., Palsson, B. O., et al. (2020). BiGG models 2020: multi-strain genome-scale models and expansion across the phylogenetic tree. Nucleic Acids Res. 48, D402–D406.
Oldroyd, G. E. D. (2013). Speak, friend, and enter: signalling systems that promote beneficial symbiotic associations in plants. Nat. Rev. Microbiol. 11, 252–263. doi: 10.1038/nrmicro2990
Onut-Brannstrom, I., Benjamin, M., Scofield, D. G., Heidmarsson, S., Andersson, M. G. I., Lindstrom, E. S., et al. (2018). Sharing of photobionts in sympatric populations of Thamnolia and Cetraria lichens: evidence from high-throughput sequencing. Sci. Rep. 8:4406.
Orth, J. D., and Palsson, B. (2012). Gap-filling analysis of the iJO1366 Escherichia coli metabolic network reconstruction for discovery of metabolic functions. BMC Syst. Biol. 6:30. doi: 10.1186/1752-0509-6-30
Ott, S., Krieg, T., Spanier, U., and Schieleit, P. (2000). Phytohormones in lichens with emphasis on ethylene biosynthesis and functional aspects on lichen symbiosis. Phyton Annale. Rei Bot. 40, 83–94.
Pabinger, S., Snajder, R., Hardiman, T., Willi, M., Dander, A., and Trajanoski, Z. (2014). MEMOSys 2.0: an update of the bioinformatics database for genome-scale models and genomic data. Datab. J. Biol. Datab. Curat. 2014:bau004.
Palmqvist, K., Dahlman, L., Jonsson, A., and Nash, T. H. (2008). “The carbon economy of lichens,” in Lichen Biology, 2nd Edn, ed. T. H. Nash (Cambridge: Cambridge University Press), 182–215. doi: 10.1017/cbo9780511790478.011
Papin, J. A., and Palsson, B. O. (2004). The JAK-STAT signaling network in the human B-cell: an extreme signaling pathway analysis. Biophys. J. 87, 37–46. doi: 10.1529/biophysj.103.029884
Park, S. Y., Choi, J., Kim, J. A., Jeong, M. H., Kim, S., Lee, Y. H., et al. (2013a). Draft genome sequence of Cladonia macilenta KoLRI003786, a lichen-forming fungus producing biruloquinone. Genome Announc. 1:e0695-13.
Park, S. Y., Choi, J., Kim, J. A., Yu, N. H., Kim, S., Kondratyuk, S. Y., et al. (2013b). Draft genome sequence of lichen-forming fungus Caloplaca flavorubescens Strain KoLRI002931. Genome Announc. 1:e0678-13.
Park, S. Y., Choi, J., Lee, G. W., Kim, J. A., Oh, S. O., Jeong, M. H., et al. (2014a). Draft genome sequence of lichen-forming fungus Cladonia metacorallifera Strain KoLRI002260. Genome Announc. 2:e01065-13.
Park, S. Y., Choi, J., Lee, G. W., Park, C. H., Kim, J. A., Oh, S. O., et al. (2014b). Draft genome sequence of Endocarpon pusillum strain KoLRILF000583. Genome Announc. 2:e0452-14.
Perezurria, E., Avalos, A., Guzman, G., and Vicente, C. (1993). Urease production and secretion by 3 antarctic lichen species. Endocytobios. Cell Res. 9, 239–243.
Perezurria, E., and Vicente, C. (1989). Purification and some properties of a secreted urease from Evernia prunastri thallus. J. Plant Physiol. 133, 692–695. doi: 10.1016/s0176-1617(89)80074-4
Perezurria, E., Vicente, C., and Filho, L. X. (1989). Screening of urease production and secretion by 7 species of finnish lichens. Biochem. Syst. Ecol. 17, 359–363. doi: 10.1016/0305-1978(89)90048-3
Petit, P. (1982). Phytolectins from the nitrogen-fixing lichen Peltigera horizontalis - the binding pattern of primary-protein extract. New Phytol. 91, 705–710. doi: 10.1111/j.1469-8137.1982.tb03349.x
Petit, P., Lallemant, R., and Savoye, D. (1983). Purified phytolectin from the lichen Peltigera canina var canina which binds to the phycobiont cell-walls and its use as cytochemical marker insitu. New Phytol. 94, 103–110. doi: 10.1111/j.1469-8137.1983.tb02726.x
Petrini, O., Hake, U., and Dreyfuss, M. M. (1990). An analysis of fungal communities isolated from fruticose lichens. Mycologia 82, 444–451. doi: 10.2307/3760015
Pfau, T., Christian, N., Masakapalli, S. K., Sweetlove, L. J., Poolman, M. G., and Ebenhoh, O. (2018). The intertwined metabolism during symbiotic nitrogen fixation elucidated by metabolic modelling. Sci. Rep. 8:12504.
Pharkya, P., Burgard, A. P., and Maranas, C. D. (2004). OptStrain: a computational framework for redesign of microbial production systems. Genome Res. 14, 2367–2376. doi: 10.1101/gr.2872004
Piercey-Normore, M. D., and Athukorala, S. N. P. (2017). Interface between fungi and green algae in lichen associations. Botany 95, 1005–1014. doi: 10.1139/cjb-2017-0037
Poole, P., Ramachandran, V., and Terpolilli, J. (2018). Rhizobia: from saprophytes to endosymbionts. Nat. Rev. Microbiol. 16, 291–303. doi: 10.1038/nrmicro.2017.171
Porada, P., Poschl, U., Kleidon, A., Beer, C., and Weber, B. (2017). Estimating global nitrous oxide emissions by lichens and bryophytes with a process-based productivity model. Biogeosciences 14, 1593–1602. doi: 10.5194/bg-14-1593-2017
Porada, P., Weber, B., Elbert, W., Poschl, U., and Kleidon, A. (2013). Estimating global carbon uptake by lichens and bryophytes with a process-based model. Biogeosciences 10, 6989–7033. doi: 10.5194/bg-10-6989-2013
Porada, P., Weber, B., Elbert, W., Poschl, U., and Kleidon, A. (2014). Estimating impacts of lichens and bryophytes on global biogeochemical cycles. Glob. Biogeochem. Cycles 28, 71–85. doi: 10.1002/2013gb004705
Price, N. D., Reed, J. L., and Palsson, B. O. (2004). Genome-scale models of microbial cells: evaluating the consequences of constraints. Nat. Rev. Microbiol. 2, 886–897. doi: 10.1038/nrmicro1023
Prieto, M., and Wedin, M. (2013). Dating the diversification of the major lineages of ascomycota (Fungi). PLoS One 8:e65576. doi: 10.1371/journal.pone.0065576
Ranganathan, S., Suthers, P. F., and Maranas, C. D. (2010). OptForce: an optimization procedure for identifying all genetic manipulations leading to targeted overproductions. PLoS Computat. Biol. 6:e1000744. doi: 10.1371/journal.pcbi.1000744
Richardson, D. H., Hill, D. J., and Smith, D. C. (1968). Lichen physiology XI. Role of alga in determining pattern of carbohydrate movement between lichen symbionts. New Phytol. 67, 469–486. doi: 10.1111/j.1469-8137.1968.tb05476.x
Rocha, I., Maia, P., Evangelista, P., Vilaca, P., Soares, S., Pinto, J. P., et al. (2010). OptFlux: an open-source software platform for in silico metabolic engineering. BMC Syst. Biol. 4:45. doi: 10.1186/1752-0509-4-45
Rodriguez, M., and Vicente, C. (1991). Water status and urease secretion from 2 ecotypes of Xanthoria parietina. Symbiosis 11, 255–262.
Rother, M., Munzner, U., Thieme, S., and Krantz, M. (2013). Information content and scalability in signal transduction network reconstruction formats. Mol. Biosyst. 9, 1993–2004. doi: 10.1039/c3mb00005b
Sacristán, M., Vivas, M., Millanes, A. M., Fontaniella, B., Vicente, C., and Legaz, M. E. (2007). “The recognition pattern of green algae by lichenized fungi can be extended to lichens containing a cyanobacterium as photobiont,” in Communicating Current Research and Educational Topics and Trends in Applied Microbiology, Vol. 1, ed. A. M. éndez-Vilas (Cham: Springer), 213–219.
Sadowsky, A., and Ott, S. (2016). Symbiosis as a successful strategy in continental Antarctica: performance and protection of Trebouxia photosystem II in relation to lichen pigmentation. Polar Biol. 39, 139–151. doi: 10.1007/s00300-015-1677-0
Santos-Merino, M., Singh, A. K., and Ducat, D. C. (2019). New applications of synthetic biology tools for cyanobacterial metabolic engineering. Front. Bioeng. Biotechnol. 7:33. doi: 10.3389/fbioe.2019.00033
Schoch, C. L., Sung, G. H., Lopez-Giraldez, F., Townsend, J. P., Miadlikowska, J., Hofstetter, V., et al. (2009). The ascomycota tree of life: a phylum-wide phylogeny clarifies the origin and evolution of fundamental reproductive and ecological traits. Syst. Biol. 58, 224–239.
Schwartzman, D. W. (2010). Was the origin of the lichen symbiosis triggered by declining atmospheric carbon dioxide levels? Biol. Lichens Symbios. 105, 191–196.
Schwechheimer, S. K., Becker, J., and Wittmann, C. (2018). Towards better understanding of industrial cell factories: novel approaches for (13)C metabolic flux analysis in complex nutrient environments. Curr. Opin. Biotechnol. 54, 128–137. doi: 10.1016/j.copbio.2018.07.001
Sigurbjornsdottir, M. A., Andresson, O. S., and Vilhelmsson, O. (2016). Nutrient scavenging activity and antagonistic factors of non-photobiont lichen-associated bacteria: a review. World J. Microbiol. Biotechnol. 32:68.
Smith, D., Muscatine, L., and Lewis, D. (1969). Carbohydrate movement from autotrophs to heterotrophs in parasitic and mutualistic symbiosis. Biol. Rev. 44, 17–85. doi: 10.1111/j.1469-185x.1969.tb00821.x
Smith, D. C., and Drew, E. A. (1965). Studies in physiology of lichens v. translocation from algal layer to medulla in Peltigera polydactyla. New Phytol. 64, 195–200. doi: 10.1111/j.1469-8137.1965.tb05390.x
Smith, H. B., Dal Grande, F., Muggia, L., Keuler, R., Divakar, P. K., Grewe, F., et al. (2020). Metagenomic data reveal diverse fungal and algal communities associated with the lichen symbiosis. Symbiosis 82, 133–147. doi: 10.1007/s13199-020-00699-4
Spribille, T., Tuovinen, V., Resl, P., Vanderpool, D., Wolinski, H., Aime, M. C., et al. (2016). Basidiomycete yeasts in the cortex of ascomycete macrolichens. Science 353, 488–492. doi: 10.1126/science.aaf8287
Stolyar, S., Van Dien, S., Hillesland, K. L., Pinel, N., Lie, T. J., Leigh, J. A., et al. (2007). Metabolic modeling of a mutualistic microbial community. Mol. Syst. Biol. 3:92. doi: 10.1038/msb4100131
Taffs, R., Aston, J. E., Brileya, K., Jay, Z., Klatt, C. G., Mcglynn, S., et al. (2009). In silico approaches to study mass and energy flows in microbial consortia: a syntrophic case study. BMC Syst. Biol. 3:114. doi: 10.1186/1752-0509-3-114
Tagirdzhanova, G., Saary, P., Tingley, J. P., Diaz-Escandon, D., Abbott, D. W., Finn, R. D., et al. (2021). Predicted input of uncultured fungal symbionts to a lichen symbiosis from metagenome-assembled genomes. Genome Biol. Evol. 2021:evab047.
ten Veldhuis, M. C., Ananyev, G., and Dismukes, G. C. (2020). Symbiosis extended: exchange of photosynthetic O-2 and fungal-respired CO2 mutually power metabolism of lichen symbionts. Photosynthes. Res. 143, 287–299. doi: 10.1007/s11120-019-00702-0
Terfve, C., and Saez-Rodriguez, J. (2012). Modeling signaling networks using high-throughput phospho-proteomics. Adv. Exp. Med. Biol. 736, 19–57. doi: 10.1007/978-1-4419-7210-1_2
Tuovinen, V., Ekman, S., Thor, G., Vanderpool, D., Spribille, T., and Johannesson, H. (2019). Two basidiomycete fungi in the cortex of wolf lichens. Curr. Biol. 29, 476–483. doi: 10.1016/j.cub.2018.12.022
Vardi, L., Ruppin, E., and Sharan, R. (2012). A linearized constraint-based approach for modeling signaling networks. J. Comput. Biol. 19, 232–240. doi: 10.1089/cmb.2011.0277
Varma, A., and Palsson, B. O. (1994). Stoichiometric flux balance models quantitatively predict growth and metabolic by-product secretion in wild-type Escherichia coli W3110. Appl. Environ. Microbiol. 60, 3724–3731. doi: 10.1128/aem.60.10.3724-3731.1994
Venkateshwaran, M., Volkening, J. D., Sussman, M. R., and Ane, J. M. (2013). Symbiosis and the social network of higher plants. Curr. Opin. Plant Biol. 16, 118–127. doi: 10.1016/j.pbi.2012.11.007
Vicente, C., and Perezurria, E. (1989). Production and secretion of urease by Evernia prunastri thallus and its symbionts. Endocytobios. Cell Res. 6, 87–97.
Vivas, M., Sacristan, M., Legaz, M. E., and Vicente, C. (2010). The cell recognition model in chlorolichens involving a fungal Lectin binding to an algal ligand can be extended to cyanolichens. Plant Biol. 12, 615–621.
Wang, Y., Yuan, X., Chen, L., Wang, X., and Li, C. (2018). Draft genome sequence of the lichen-forming fungus Ramalina intermedia strain YAF0013. Genome Announc. 6:e0478-18.
Wang, Y., Zhang, X., Zhou, Q., Zhang, X., and Wei, J. (2015). Comparative transcriptome analysis of the lichen-forming fungus Endocarponpusillum elucidates its drought adaptation mechanisms. Sci. China Life Sci. 58, 89–100. doi: 10.1007/s11427-014-4760-9
Wei, S. S., Jian, X. X., Chen, J., Zhang, C., and Hua, Q. (2017). Reconstruction of genome-scale metabolic model of Yarrowia lipolytica and its application in overproduction of triacylglycerol. Bioresourc. Bioprocess. 4:51.
West, N. J., Parrot, D., Fayet, C., Grube, M., Tomasi, S., and Suzuki, M. T. (2018). Marine cyanolichens from different littoral zones are associated with distinct bacterial communities. PeerJ 6:e5208. doi: 10.7717/peerj.5208
Ye, C., Zou, W., Xu, N., and Liu, L. (2014). Metabolic model reconstruction and analysis of an artificial microbial ecosystem for vitamin C production. J. Biotechnol. 182–183, 61–67. doi: 10.1016/j.jbiotec.2014.04.027
Yen, J. Y., Nazem-Bokaee, H., Freedman, B. G., Athamneh, A. I. M., and Senger, R. S. (2013). Deriving metabolic engineering strategies from genome-scale modeling with flux ratio constraints. Biotechnol. J. 8, 581–594. doi: 10.1002/biot.201200234
Yim, H., Haselbeck, R., Niu, W., Pujol-Baxley, C., Burgard, A., Boldt, J., et al. (2011). Metabolic engineering of Escherichia coli for direct production of 1,4-butanediol. Nat. Chem. Biol. 7, 445–452.
Zhang, X., and Reed, J. L. (2014). Adaptive evolution of synthetic cooperating communities improves growth performance. PLoS One 9:e108297. doi: 10.1371/journal.pone.0108297
Zhuang, K., Izallalen, M., Mouser, P., Richter, H., Risso, C., Mahadevan, R., et al. (2011). Genome-scale dynamic modeling of the competition between Rhodoferax and Geobacter in anoxic subsurface environments. ISME J. 5, 305–316. doi: 10.1038/ismej.2010.117
Zomorrodi, A. R., and Maranas, C. D. (2012). OptCom: a multi-level optimization framework for the metabolic modeling and analysis of microbial communities. PLoS Computat. Biol. 8:e1002363. doi: 10.1371/journal.pcbi.1002363
Keywords: systems biology, network modelling, signalling, metabolic model, lichen symbiosis
Citation: Nazem-Bokaee H, Hom EFY, Warden AC, Mathews S and Gueidan C (2021) Towards a Systems Biology Approach to Understanding the Lichen Symbiosis: Opportunities and Challenges of Implementing Network Modelling. Front. Microbiol. 12:667864. doi: 10.3389/fmicb.2021.667864
Received: 14 February 2021; Accepted: 09 April 2021;
Published: 03 May 2021.
Edited by:
Enrica Pessione, University of Turin, ItalyReviewed by:
Tomislav Cernava, Graz University of Technology, AustriaChristoph Scheidegger, Swiss Federal Institute for Forest, Snow and Landscape Research (WSL), Switzerland
Copyright © 2021 Nazem-Bokaee, Hom, Warden, Mathews and Gueidan. This is an open-access article distributed under the terms of the Creative Commons Attribution License (CC BY). The use, distribution or reproduction in other forums is permitted, provided the original author(s) and the copyright owner(s) are credited and that the original publication in this journal is cited, in accordance with accepted academic practice. No use, distribution or reproduction is permitted which does not comply with these terms.
*Correspondence: Hadi Nazem-Bokaee, SGFkaS5OYXplbS1Cb2thZWVAY3Npcm8uYXU=; Cécile Gueidan, Q2VjaWxlLkd1ZWlkYW5AY3Npcm8uYXU=