- 1Department of Agricultural Engineering, University of Bonn, Bonn, Germany
- 2ADDCON GmbH, Bitterfeld-Wolfen, Germany
- 3Institute of Nutrition and Food Science, University of Bonn, Bonn, Germany
- 4Department of Botany and Plant Sciences, Kearney Agricultural Center, University of California, Riverside, Riverside, CA, United States
The microbiome in silage may vary substantially from the onset to the completion of fermentation. Improved additives and inoculants are being developed to accelerate the ensiling process, to enhance fermentation quality, and to delay spoilage during feed-out. However, current methods for preselecting and characterizing these amendments are time-consuming and costly. Here, we have developed a multi-sensor mini-bioreactor (MSMB) to track microbial fermentation in situ and additionally presented a mathematical model for the optimal assessment among candidate inoculants based on the Bolza equation, a fundamental formula in optimal control theory. Three sensors [pH, CO2, and ethanol (EtOH)] provided data for assessment, with four additional sensors (O2, gas pressure, temperature, and atmospheric pressure) to monitor/control the fermentation environment. This advanced MSMB is demonstrated with an experimental method for evaluating three typical species of lactic acid bacteria (LAB), Lentilactobacillus buchneri (LB) alone, and LB mixed with Lactiplantibacillus plantarum (LBLP) or with Enterococcus faecium (LBEF), all cultured in De Man, Rogosa, and Sharpe (MRS) broth. The fermentation process was monitored in situ over 48 h with these candidate microbial strains using the MSMB. The experimental results combine acidification characteristics with production of CO2 and EtOH, optimal assessment of the microbes, analysis of the metabolic sensitivity to pH, and partitioning of the contribution of each species to fermentation. These new data demonstrate that the MSMB associated with the novel rapid data-processing method may expedite development of microbial amendments for silage additives.
Introduction
Silage is a major feedstuff for ruminant animal production worldwide. The biochemical production of silage relies on anaerobic lactic acid fermentation. Homofermentative lactic acid bacteria (LAB) ferment glucose to lactic acid as the primary by-product, whereas heterofermentative LAB ferment glucose to lactic acid, acetic acid, ethanol (EtOH), and carbon dioxide (CO2) (Muck, 2004; Kung et al., 2018a; Muck et al., 2018). Testing for heterofermentative fermentation generally involves gas phase sampling of CO2 and EtOH (McEniry et al., 2011; Li et al., 2017; Kung et al., 2018b).
Though silage is a nutritious and palatable animal feed, its aerobic deterioration is inevitable when the silo is opened for feeding out, but spoilage can be reduced significantly using either biological or chemical additives (Ranjit and Kung, 2000; Borreani and Tabacco, 2010; Tabacco et al., 2011; Wilkinson and Davies, 2012). Commercial silage inoculants contain highly selected bacteria that speed up silage acidification during anaerobic fermentation (Queiroz et al., 2013; Muck et al., 2018). This typically involves strains of facultative heterofermentative bacteria such as Lactiplantibacillus plantarum (LP), and obligate heterofermentative bacteria such as Lentilactobacillus buchneri (LB) are used to enhance the aerobic stability of silage (Bolsen et al., 1996; Kung et al., 2018a; Muck et al., 2018). Rapid acidification significantly inhibits the growth of undesirable microorganisms and reduces fermentative loss, while acetic acid, the by-product of heterofermentation, suppresses the aerobic proliferation of spoilage-causing fungi and minimizes oxidative losses during feed-out (Pahlow et al., 2003; Muck, 2004; Kung et al., 2018b).
To continuously improve these biological silage additives requires selection of improved microbial strains from among the abundant species and strains of LAB that are present in natural populations (Muck et al., 2018). Selection is commonly performed during the natural ensiling process, which is time-consuming, laborious, and costly, requiring large numbers of ex situ samples and intensive laboratory analyses (Weinberg and Ashbell, 2003). To resolve these bottlenecks in research for silage inoculants, this study presents a multi-sensor-based screening method with three major objectives: (i) to present an alternative model for selecting microbes, (ii) to devise a multi-sensor mini-bioreactor (MSMB) for screening microbial strains during fermentation in situ, and (iii) to evaluate the capabilities of the advanced MSMB in selection among candidate inoculants.
Materials and Methods
Microbial Selection and Optimal Fermentation
With the use of control system theory (Sargent, 2000), a LAB fermentation process [X(t)] can be described as a set of state variables [x1(t) – x3(t)] under microbial control um such that
where x1, x2, and x3 refer to the accumulated productions of organic acids (lactic and acetic), CO2, and EtOH, respectively, with respect to the initial time (t0) and the final time (tf) of fermentation. As the function of time (t), these state variables can be expressed as the outputs of triple integrators such that
where [acid] is the instantaneous production of organic acids (primary lactic and acetic), resulting from LAB fermentation. Similar representations apply to carbon dioxide [CO2] and [EtOH]. According to optimal control theory (Sargent, 2000), an optimal/minimum cost function of Ĵ subject to Eq. 2 exists, and an optimal control (ȗm–best) fulfills
where min.(tf –t0)pH↓ is the shortest time of acidification dynamics of Eq. 1, i.e., the optimal time to be determined by fermentation. The two integrations of x2(t) and x3(t) are related to minimum fermentative loss. Eq. 3 is a special case of the generalized problem of Bolza (Clarke, 1976; Sargent, 2000), which contains a global solution of the time-energy optimization subject to an optimal control function (ȗm–best). In this study, the optimal time-energy trajectories of fermentation were determined experimentally by selecting candidate microbes.
Multi-Sensor-Based Experimental System
The instrumental structure of the MSMB (Figure 1) contains seven different functional sensors (Table 1), with six enclosed in an air-tight chamber. According to Eq. 2, pH, CO2, and EtOH are the three indicators of the LAB fermentation, each corresponding to an analog integrator. Additionally, micro-environmental parameters, i.e., O2 concentration, ambient temperature (Ta), and gas pressure (Pgas) in the sealed chamber, were measured during the fermentation process. Pgas was measured relative to ambient air pressure (Pair), which was measured using a digital barometer placed on the outside of the sealed chamber (Figure 1). Throughout the test, Pgas remains positive (Pgas > Pair) due to accumulation of CO2 and volatile EtOH. The Pgas measurement has two functions: (i) to determine the seal characteristics of the chamber before and during the experiment and (ii) to compensate the O2 measurement since the optical O2 sensor was calibrated under Pair.
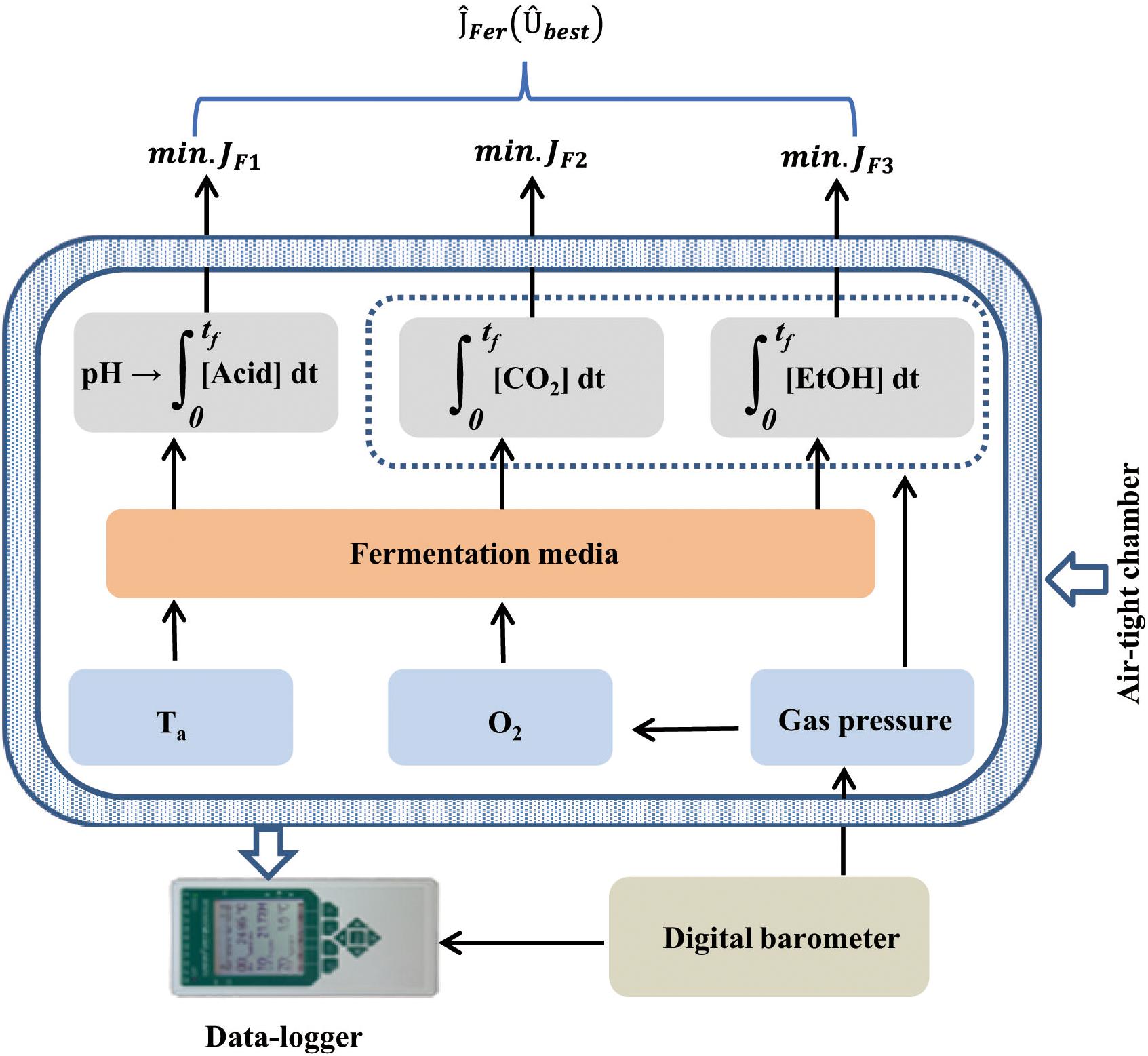
Figure 1. The multi-parameter measurement system devised for selecting microbes used as silage inoculant, based on the characteristics of lactic acid bacterial (LAB) fermentation and the theory of optimal control.
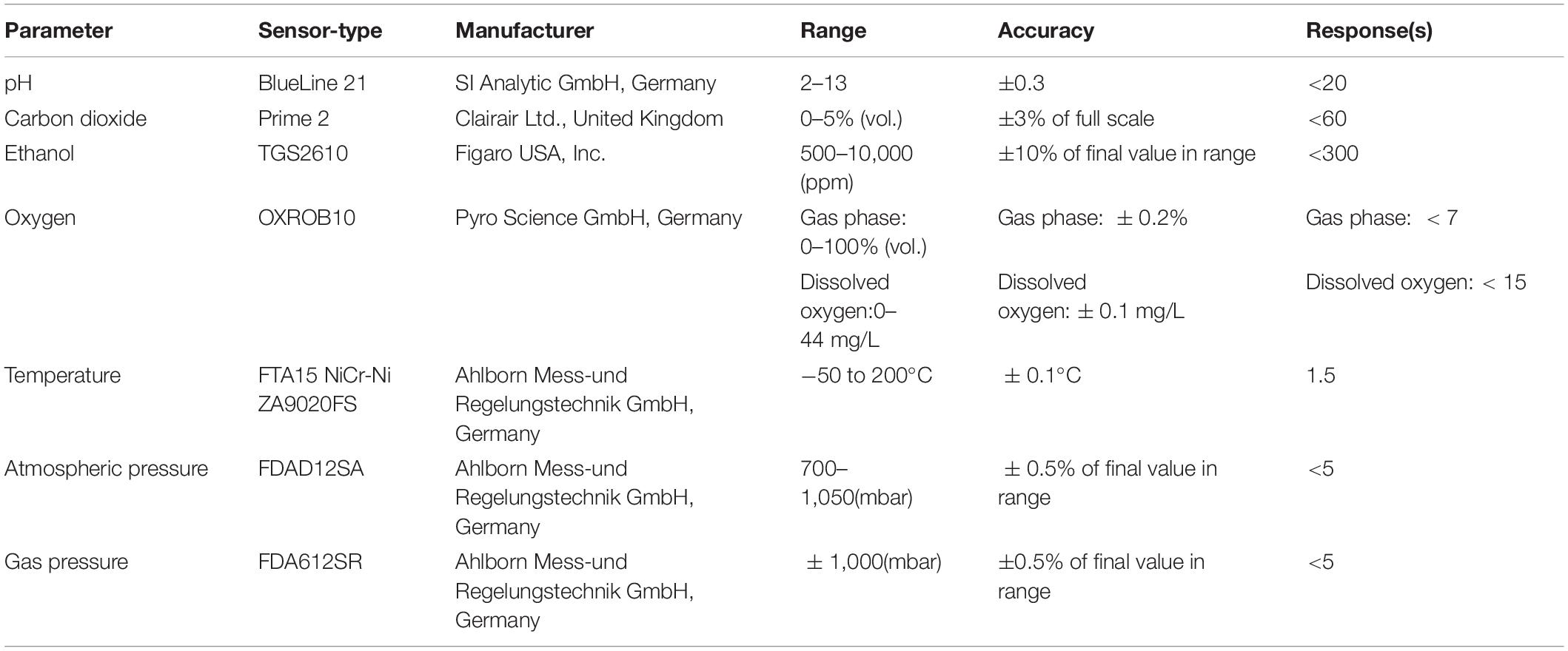
Table 1. Technical information of the pH, CO2, ethanol (EtOH), O2, temperature, and pressure sensors used.
The MSMB including the air-tight chamber (glass jar, 1.5 L) is schematically shown in Figure 2. A centrifuge tube (inner diameter 2.5 cm, height 12 cm) was filled with fermentation medium (orange color) for culturing the candidate LAB. The pH electrode was immersed in the medium, as the integrator to determine the acidification characteristics of the fermentation relative to each strain or species. The resulting gas from the fermentation diffuses out of the centrifuge tube through six holes (diameter 2 mm) in the wall of the upper tube. Because CO2 is unreactive with EtOH, this allows the glass jar to act as a dual integrator for simultaneous collection of the CO2 and the volatile EtOH from the fermentation process in the centrifuge tube. The O2 sensor can be moved vertically to measure the O2 concentration in the gas space or O2 dissolved in the medium. To remove O2 for anaerobic requirements, paired holes (diameter 3 mm) were perforated in the lid (Figure 2B) for purging with N2. Three sets of the MSMB provided replication for simultaneous testing. Three data loggers were linked to (i) the thermocouples and pH electrodes (ALMEMO-2890-9, nine-channel, Ahlborn Mess-und Regelungstechnik GmbH, Ilmenau, Germany), (ii) CO2 and EtOH sensors (own manufacture, eight-channel), and (iii) the O2 sensor (four-channel, Pyro Science GmbH, Aachen, Germany), all sampled at 10-min intervals.
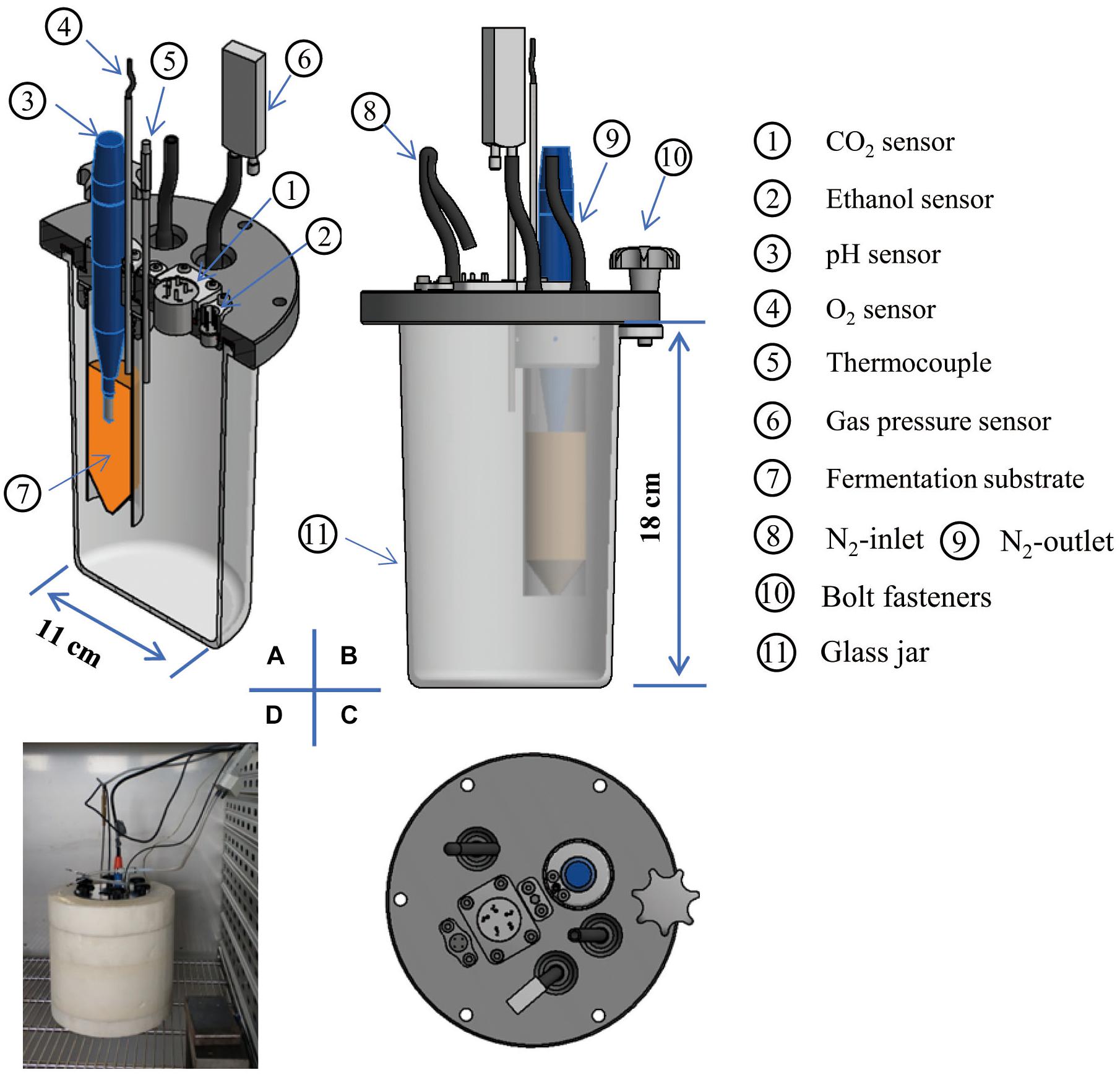
Figure 2. A novel multi-sensor jar, manufactured according to Figure 1, is shown in cross section (A), front view (B), and top view (C) and measured in situ in the incubator (D).
Sample Preparation
Strains of typical species of obligate heterofermentative bacteria, Lt. buchneri DSM 13573 (LB) and two typical species of facultative heterofermentative bacteria, Lp. plantarum DSM 3676, Lp. plantarum DSM 3677, and Enterococcus faecium NCIMB 11181 (EF), were chosen for the experiment. The two strains of Lb. plantarum were used as a mixture (1:1) in all experiments and designated as LP. Lt. buchneri DSM 13573 (LB) was used individually and mixed with Lp. plantarum DSM 3676/DSM 3677 (LBLP) or with E. faecium NCIMB 11181 (LBEF).
All candidate bacteria were prepared as lyophilizates by cultivating the strains on synthetic medium and harvesting the cells by centrifugation. The harvested biomass was lyophilized at −40°C for 2 days; and the lyophilizates were stored at −18°C. The cell density of the lyophilized LB was 1.6 × 1012 CFU/g. As 0.1 g of the lyophilized LB with 40 ml of De Man, Rogosa, and Sharpe (MRS) (Table 2) was cultured during the experiment, the resulting inoculum density of LB was 4 × 109 CFU/ml. For LBLP and LBEF, either Lp. plantarum DSM 3676/DSM 3677 or E. faecium NCIMB 11181 was added with 10% of the cell density of the LB (i.e., 4 × 108 CFU/ml). The cell density of the lyophilized LP was 1 × 1011 CFU/g, and that of the lyophilized EF was 3 × 1012 CFU/g. Thus, 0.160 g of the lyophilized LP and 0.005 g of the lyophilized EF were mixed with 0.1 g of the lyophilized LB. These microbial samples, in 40 ml of sterile MRS broth in each centrifuge tube (vol. 50 ml), were incubated inside the multi-sensor instrumented jar at 30°C for 48 h.
Chemical Analyses
All the fermented samples were frozen in the centrifuge tubes at −20°C immediately after incubation, prior to chemical analyses. The fermentation acids (lactic and acetic), EtOH, and propanediol were determined using high-performance liquid chromatography (HPLC; KNAUER Azura, Wissenschaftliche Geräte GmbH, Berlin, Germany), coupled with integrated UV and refractive index (RI) detectors as described by Shan et al. (2019).
Signal Processing
Normalized Productions of and
To compare the relative rate of increase of the CO2 or EtOH in the fermentation process, the normalized productions of carbon dioxide () and were calculated, respectively, as
and
where both and vary from 0 to 1 as functions of t.
Temporal Rate of ΔCO2 and ΔEtOH
This differential variable may reflect the dynamics of metabolic activity of microorganisms. As sequences of discrete-time data, the differentials of CO2 and EtOH were calculated over time as
Signal Decomposition
Signal decomposition, a function of smart instruments, is often used to partition a mixed source signal into its constitutive pure components for various engineering problems (Li et al., 2013; Shan et al., 2019). In this study, the fermentation characteristics relative to different strains or combinations are regarded as mathematical curves in functional space. Three time courses of pH (i.e., pHLB, pHLBLP, and pHLBEF) are directly tracked in situ from the experiment. Through data decomposition, pHLP and pHEF can also be obtained, such that
where pH0 is the initial value of each substrate.
Statistical Analysis
The experimental data were analyzed using IBM SPSS v25.0 (IBM Co., Armonk, NY, United States). Linear regression, curve fitting, and fitting errors were evaluated using coefficient of determination (R2) and root mean square error (RMSE). Two-way analysis of variance (ANOVA) was conducted for effects of the experimental scheme (two air environments, i.e., anaerobic and aerobic), treatment (three types, i.e., LB, LBEF, and LBLP), and the interactions of the chemical compositions for the final-data processing. One-way ANOVA was used to evaluate the statistical significance among anaerobic and aerobic environments.
Results
Acidification Characteristics
Two sets of time courses of pH (Figure 3), each with respect to the mean of three replicates, were recorded in situ from the fermentation process in the MSMB, the first set (Figure 3A) from anaerobic fermentation and the second set (Figure 3B) from aerobic fermentation. The patterns observed were similar. Figure 4 shows the time courses of O2 concentration over the experiment with two parts, i.e., as liquid phase dissolved in the MRS and as gaseous phase distributed in the glass jars. When comparing the anaerobic fermentation (Figure 4A) and aerobic one (Figure 4B), the amounts of gaseous oxygen in these jars remained two constant levels throughout the experiment, i.e., around 0.2 vol. % of O2 concentration in the anaerobic jars and 20 vol. % of O2 concentration in the aerobic jars. The O2 dissolved in the MRS in both anaerobic and aerobic jars varied with similar trends in the initial 5 h, i.e., declined from 0.157 to 0.138 mg/L, and then reached a plateau. This and the chemical analyses from aerobic and anaerobic fermentations at the end of the experiment (Table 3) demonstrate that the oxygen in the MSMB had minimal impact on the respiratory metabolism of the microbes tested.
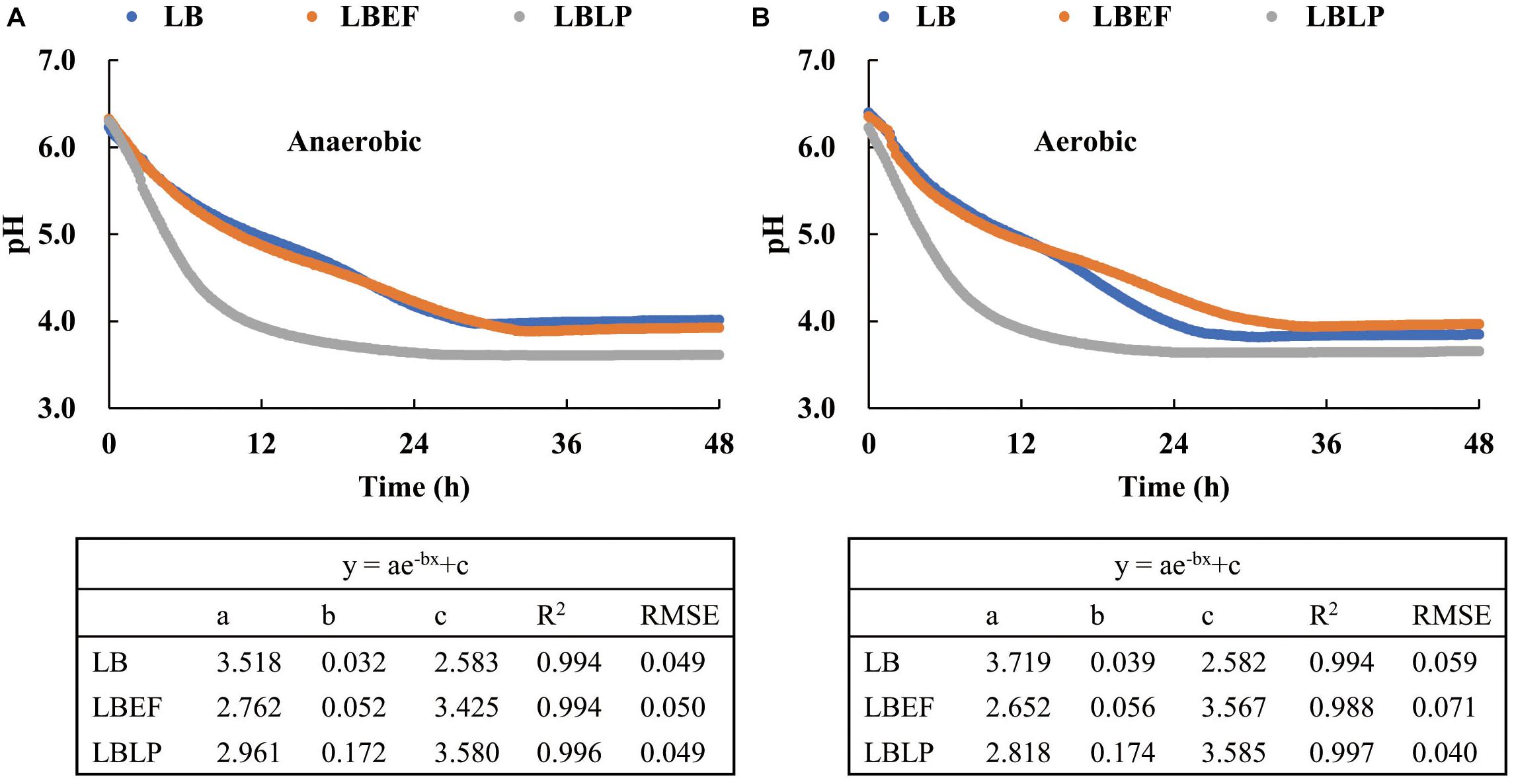
Figure 3. Three time courses of the acidification process with respect to Lentilactobacillus buchneri (LB), LB mixed with Enterococcus faecium (LBEF), and LB mixed with Lactiplantibacillus plantarum (LBLP), under anaerobic (A) and aerobic conditions (B). LB, Lentilactobacillus buchneri; LBEF, LB mixed with Enterococcus faecium; LBLP, LB mixed with Lactiplantibacillus plantarum.
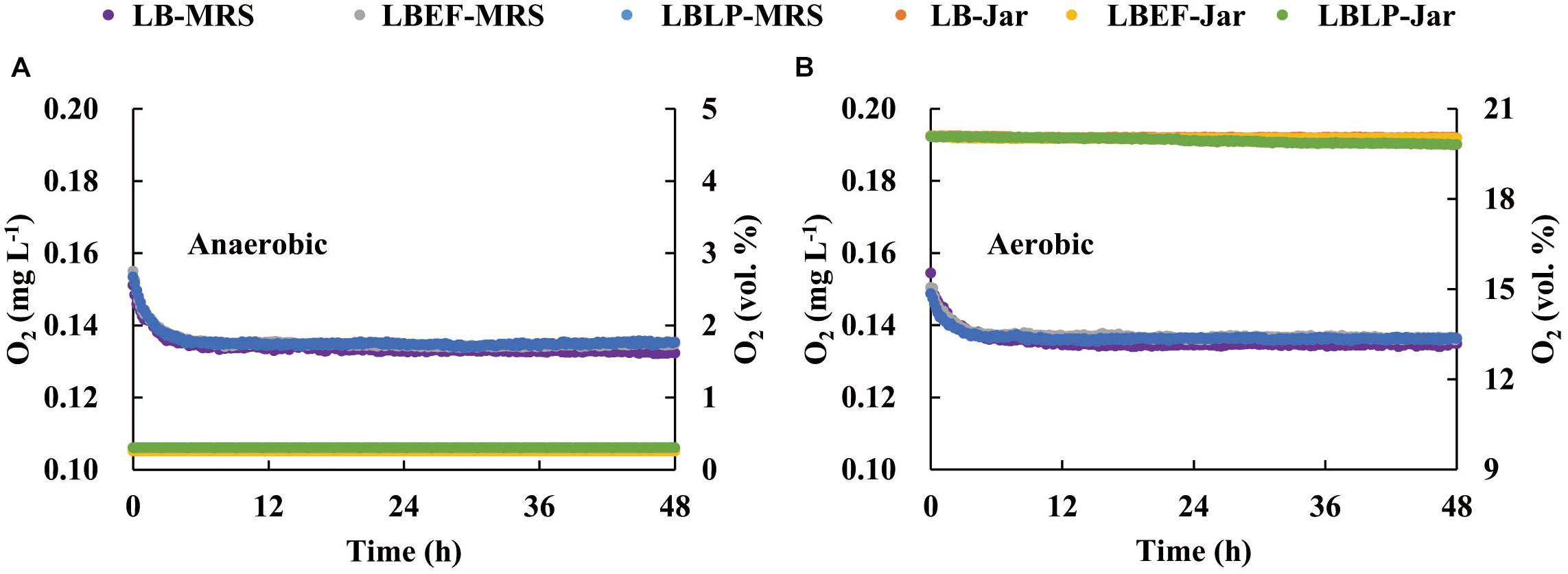
Figure 4. Time courses of O2 concentration dissolved in the De Man, Rogosa, and Sharpe (MRS) and distributed in the sealed jars during the anaerobic (A) and aerobic (B) fermentations. MRS, De Man, Rogosa, and Sharpe.
In general, all time courses of pH (Figures 3A,B), i.e., the acidification characteristics, were well described by exponential regressions, in both anaerobic and aerobic fermentations. The steepest decline was observed from the LBLP (pHLBLP = 5, t = 4.5 h, anaerobic), evidently faster than that of LBEF (pHLBEF = 5, t = 10 h, anaerobic) or that of the LB (pHLB = 5, t = 11.5 h, anaerobic). Additionally, the pHLBLP had the lowest values (pHLBLP = 3.61 or 3.65) in both anaerobic and aerobic fermentations, which agreed with the finding that the contents of lactic acid in the fermented MRS of LBLP are slightly higher than those of LB and LBEF (Table 3).
The final time (tf) was determined as pH ≤ 4 over six consecutive measurements (i.e., 1 h). The tf of the pHLBLP was only 12 h, whereas the tf for both pHLBEF and pHLB was more than double at 28 h. Therefore, for these microbial samples in the same experimental conditions, the acidification characteristics of the LBLP had the lowest tf (i.e., the fastest process). Therefore, the resulting time course of pHLBLP is time optimal, but this only fulfilled by one of the two necessary criteria for a global optimal solution of the Bolza equation (i.e., the term of min.(tf – t0)pH↓ in Eq. 3).
Formations of CO2 and EtOH
Three time courses of CO2 and EtOH formation (Figure 5) were recorded from the LB (A), the LBEF (B), and the LBLP (C). The fermentation governed by the LBLP yielded the lowest CO2 (48.12 mg) and EtOH (14.55 mg) in the period of 48 h. In contrast, the fermentation with the LB or the LBEF yielded more than twice as much CO2 (86.21–90.72 mg) and EtOH (28.14–32.10 mg). Figure 6 shows the normalized CO2 production (, Figure 6A) and (Figure 6B) from the three samples. For the LBLP, the reached 0.8 of the final production in 24.5 h, while the = 0.8 for the LB was 28 h or for the LBEF was 31 h. Similarly, the transition times of increased to 0.8 for the LBLP, LB, and LBEF at 12.3, 19.5, and 21.8 h, respectively. In contrast, the rate of EtOH increase was greater than that of CO2 in all the samples.
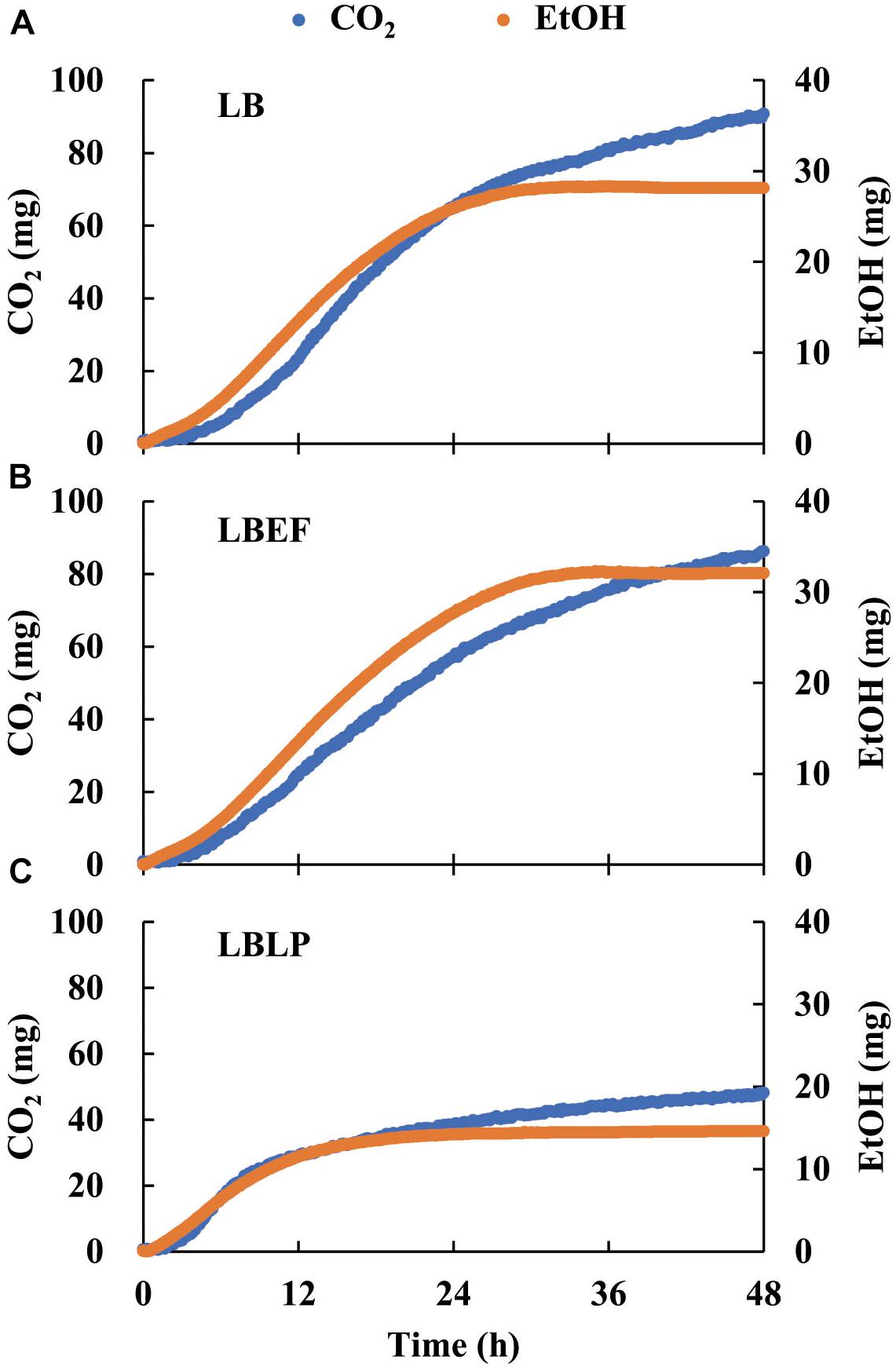
Figure 5. Time courses of CO2 and ethanol production with respect to LB (A), LBEF (B) and LBLP (C) under anaerobic conditions. LB, Lentilactobacillus buchneri; LBEF, LB mixed with Enterococcus faecium; LBLP, LB mixed with Lactiplantibacillus plantarum.
Metabolic Sensitivity to pH
Figure 7 shows stepwise tracing (ti − ti–1 = 2 h, Eq. 6) for the temporal production of both CO2 and EtOH. In the initial period of fermentation (pH > 5), the increasing ΔCO2 and ΔEtOH reflected the increasing microbial activity for all microbes. A turning point of ΔCO2 and ΔEtOH occurred around pH 5. Below pH 5, the metabolic activity decreased as the pH declined. After the pH declined to 4, both ΔCO2 and ΔEtOH reached minima and then achieved steady state. The patterns in Figure 7 not only characterize the metabolic sensitivity of these microbes to pH but also contain the dynamic information of the acidification process from each sample. For the LBLP sample, pHLBLP decreased to 5 in less time (4.5 h), resulting in only three data points of ΔCO2 (ti - ti–1 = 2 h) while pH ≥ 5. In contrast, seven data points of ΔCO2 for LB and LBEF were recorded due to the longer periods of pH ≥ 5 (pHLB, 11.5 h; pHLBEF, 10 h). The patterns of EtOH (Figures 7B,D) had similar temporal implications. Because the turning points of ΔCO2 and ΔEtOH at pH 5 correlated for all the microbial samples (Figure 7), the general effect of pH on the metabolic activity can be estimated. Figure 8 presents four piecewise linear regressions corresponding to ΔCO2 (Figure 8A pH < 5, Figure 8B pH > 5) and ΔEtOH (Figure 8C pH < 5, Figure 8D pH > 5), related to the three microbial samples.
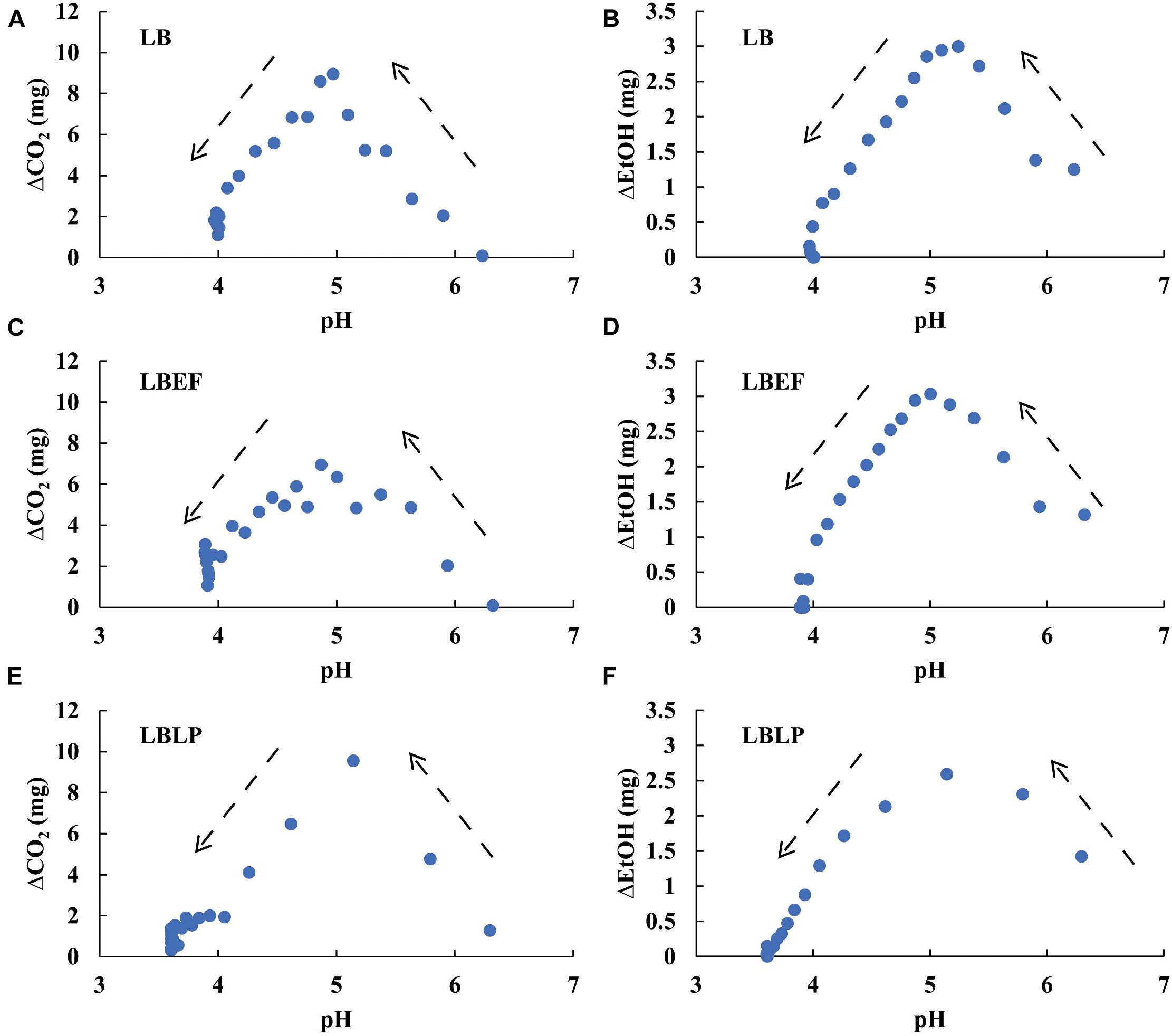
Figure 7. The relationships between the pH of the three microbial samples and the differential variables of both CO2 production (A,C,E) and EtOH production (B,D,F), where ΔCO2 = CO2 (ti) − CO2 (ti–1) and ΔEtOH = EtOH(ti) − EtOH(ti–1), both calculated with ti − ti–1 = 2 h, i = 0, 1, …,n. The arrows denote the time course of decreasing pH.
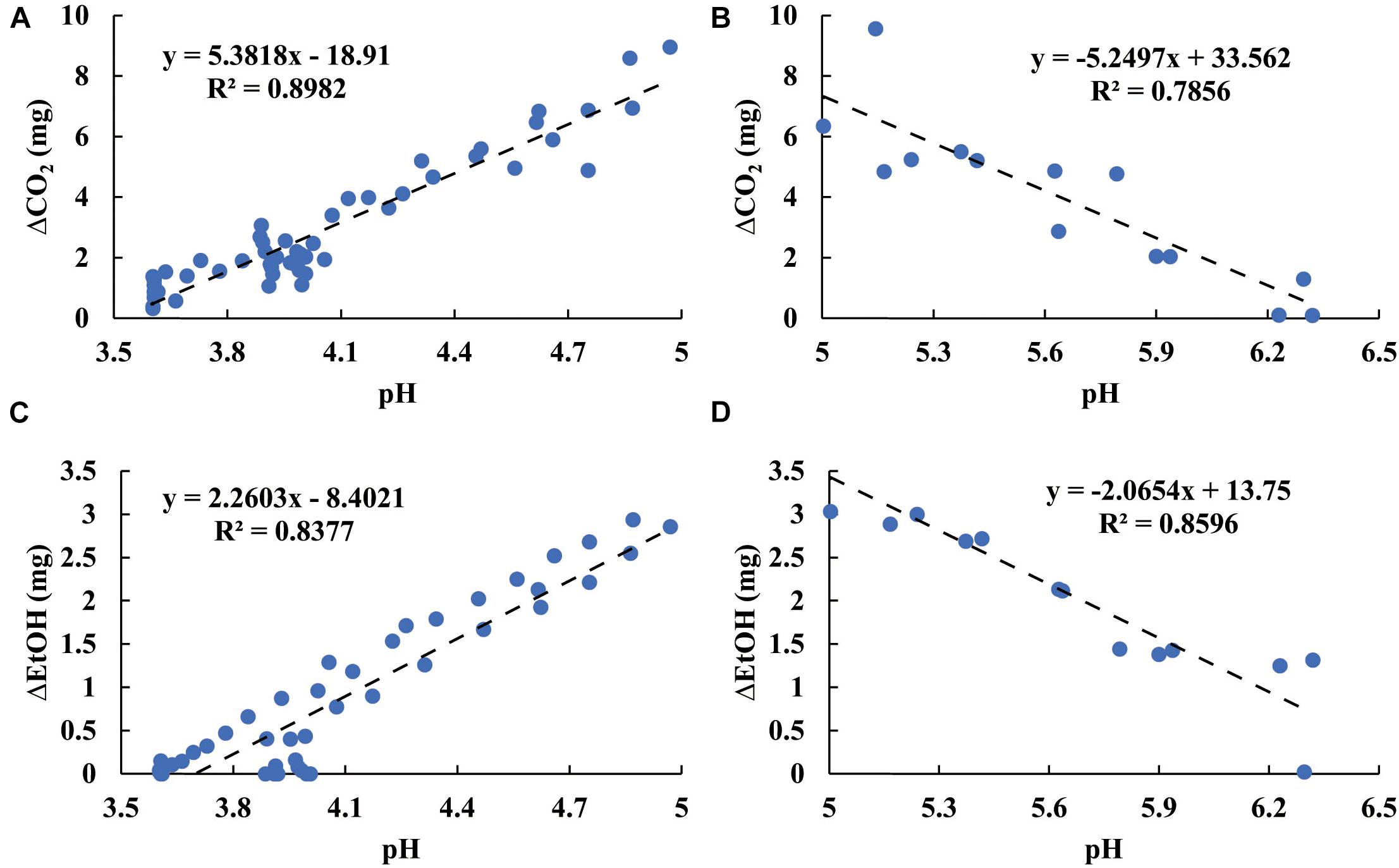
Figure 8. Effect of pH on the rate of microbial respiration in relation to ΔCO2 with pH < 5 (A), ΔCO2 with pH ≥ 5 (B), ΔEtOH with pH < 5 (C), and ΔEtOH with pH ≥ 5 (D).
Role Partition of Each Strain
In functional space, the time courses of both pHLBLP and pHLBEF (Figure 3) are mathematically decomposable in relation to the time course of pHLB. With the use of Eq. 7 together with the time course of pHLB (Figure 3) as reference, Figure 9 shows these separate time courses for pHLP and pHEF over the initial 12 h of the fermentation. We presented the decomposed data in the early stage (0–12 h) because pHLBLP reached a plateau within 12 h ≤ t ≤ 48 h (Figure 3). The regression demonstrated that the LP species played an exponentially accelerating role (R2 = 0.982, RMSE = 0.053) in the acidification process over pH ranging from 6.23 to 5.0. The major contribution of LP to the fermentation process was observed in the initial period of 0–6 h, i.e., pH ≥ 5.5. Subsequently, the accelerating role of the LP attenuated quickly as the pH decreased, and this could be attributed to the stronger suppression from the increasing organic acid in the fermentation medium. Alternatively, the separate role of the EF is a straight line, perpendicular to the pH axis at the initial point (pH0 = 6.23). This demonstrates that the role of the EF in accelerating the acidification process is negligible, not an optimal solution for Eq. 3.
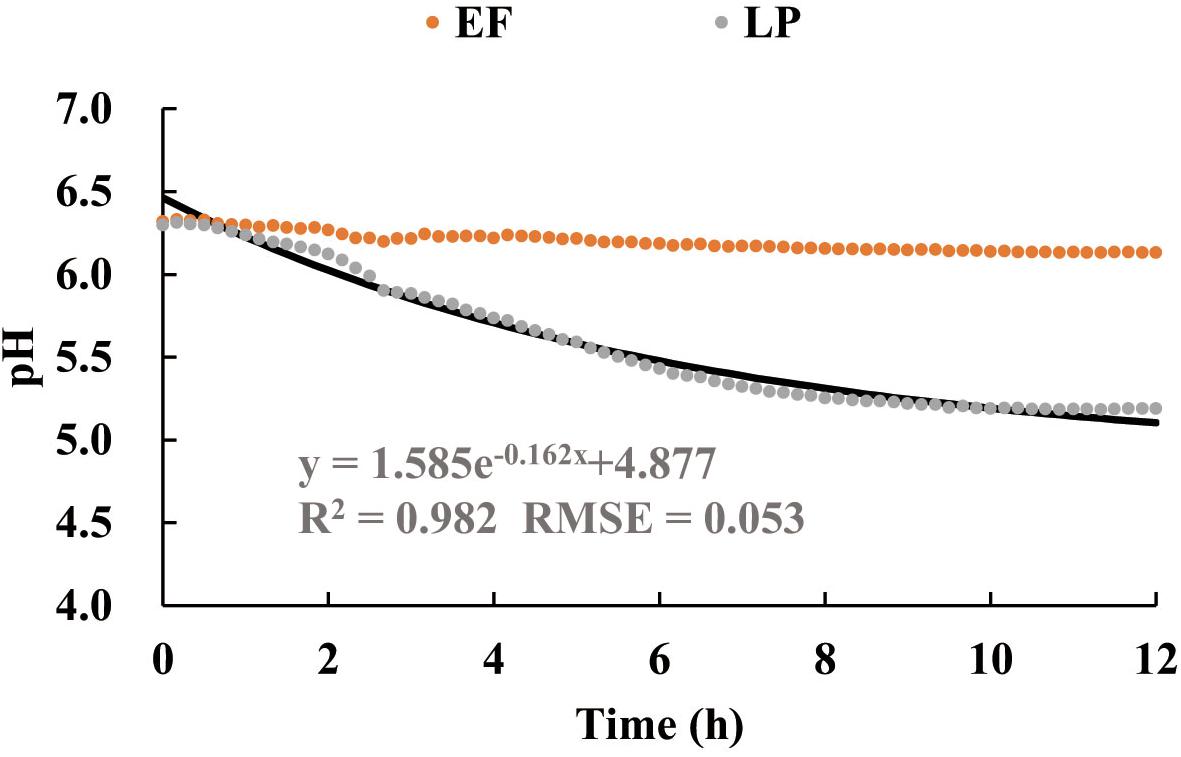
Figure 9. The data decomposition of the time courses of pH in Figure 3A to separate the additional roles of Enterococcus faecium (EF) or Lactiplantibacillus plantarum (LP) to support LB in accelerating the acidification process of the substrate (MRS). The solid line is the regression approximation to the separate data of LP. EF, Enterococcus faecium; LP, Lactiplantibacillus plantarum; LB, Lentilactobacillus buchneri; MRS, De Man, Rogosa, and Sharpe.
Discussion
Both the shortest acidification process (min. tf in section “Acidification Characteristics”) and the minimum gas production (see section “Formations of CO2 and EtOH”) resulted from the LBLP, and therefore, this is a time-energy optimal fermentation reaction (Sargent, 2000). Moreover, the time course of pHLBLP (Figure 3) is the optimal trajectory of the fermentation process, and the exponential equation of the LP (Figure 9) is the optimal control function (ȗm–best) determined. The paired optimal solutions were found experimentally and subject to the Bolza equation (Eq. 3) (Clarke, 1976).
As expected, lactic acid was the primary metabolite of LAB fermentation (Table 3). Our experimental data supported the hypothesis that pH was primarily dominated by variations in lactic acid during the LAB fermentation process (Kung et al., 2018a) from two notes: (i) the Lp. plantarum strains DSM 3676 and DSM 3677 (LP), the facultative heterofermentative strains, played a major role in producing lactic acid during the initial 0–6 h of the fermentation (Figures 3, 9). At t = 6 h, the pHLBLP = 4.62 indicated that most lactic acid had already been formed. (ii) The pKa of acetic acid (4.75) is higher than that of lactic acid (3.86), reflecting it being a 10 times weaker acid than lactic acid (Danner et al., 2003; Graves et al., 2006; Kung et al., 2018a).
Our data of the relationship between CO2 and pH (Figures 7, 8) support a value of pH 5 as a critical value governing microbial growth, with rapid (pH > 5) or slower to no growth (pH < 5) during the ensiling process (Kung et al., 2003; Pahlow et al., 2003). We show this for the first time using the dynamics of microbial respiration over the course of fermentation. This had previously been suggested from an ex situ determination of microbial counts (Oliveira et al., 2017). Our in situ method obviates the process of plate-culture counts, which create assessment delays of several days (Wilkinson and Muck, 2019) and may require multiple samplings over time. In contrast to the ex situ method, our MSMB provides both real-time anaerobic measurements of CO2 and EtOH productions and instantaneous microbial activity.
A few studies tested silage inoculants using MRS broth or the aqueous extract of silage crop (Oude Elferink et al., 2001; Danner et al., 2003; Holzer et al., 2003; Graves et al., 2006; Arasu et al., 2015; Blajman et al., 2020). Fermentation characteristics, such as production of organic acids, decreasing dynamics of pH, and CO2 production, depend on medium composition (Danner et al., 2003; Blajman et al., 2020). The next step of our study is to replace MRS broth with the aqueous extract of silage, creating a testing condition that may be closer to the natural culture of silage ensiling for LAB. However, the effect of the buffering capacity of silage crop on pH is inherent (Kung et al., 2003; Pahlow et al., 2003; Shan et al., 2021) and should be taken into account. Unfortunately, this effect cannot be evaluated when either MRS broth or the liquid extraction of silage crop is used as fermentation medium.
High concentrations of EtOH are usually attributed to large numbers of yeasts (Kung and Ranjit, 2001; Kung et al., 2018a). However, the silage containing LB (Oude Elferink et al., 2001) produced EtOH from anaerobic degradation of lactic acid in corn silage. Kung and Ranjit (2001) noted the high EtOH from treated barley silage, but not from the control. The EtOH data of our study from the MRS broth support the previous observations. Moreover, in this study, EtOH was measured in situ as the gaseous concentration in the sealed jar, which by Henry’s law is directly proportional to the concentration of EtOH dissolved in the fermentation medium (Sun et al., 2015). While the partition coefficient of Henry’s law is temperature-dependent, the fermentation here was carried out at constant temperature (30°C).
During the ensiling process, the CO2 recorded in the fermentation phase consists of two parts, one from the initial aerobic phase and one from anaerobic fermentation (Li et al., 2017). It is challenging to separate them into two CO2 pools for the different phases (Shan et al., 2019). In this study, the measured CO2 (Figures 5–8) derived completely from anaerobic heterofermentative LAB fermentation because the MSMB provided a manageable environment between anaerobic and aerobic seals. This is also an advantage of the MSMB over the ensiling experiment with the natural culture of silage.
It is not surprising that Figures 3A,B had similar patterns. Members of the family Lactobacillaceae are anaerobic, but the majority of species are oxygen tolerant to some degree and often completely. Only very few species of LAB were observed to react to O2 (Condon, 1987). On the technical side, Figure 3 demonstrates that the multi-sensor instrument presented here is suitable for both anaerobic and aerobic experiments. Since the aerobic stability of silage refers to a de-acidification process governed by fungus in silage (Wilkinson and Davies, 2012; Kung et al., 2018a), and the aerobic deterioration of silage is commonly associated with changes in temperature, pH, CO2 production, and O2 consumption (Honig, 1990; Muck and Pitt, 1994; Weinberg and Ashbell, 2003; Sun et al., 2015; Robinson and Swanepoel, 2016), this novel MSMB could also be useful to qualitatively observe the role of acetic acid in inhibiting fungal growth during aerobic phases of silage production.
Conclusion
We have devised an MSMB to facilitate preselection of microbes as silage inoculants. We demonstrate successful screening of the dynamics of the acidification process, gas production, and metabolic activity from the MRS-based LAB fermentation, using in situ simultaneous measurements of pH, CO2, and EtOH. We have further used this novel information to introduce an optimal control model, using signal decomposition, for selecting candidate microbes. Future studies are planned to evaluate this novel prototype with aqueous extracts of common silage materials during anaerobic LAB fermentation and to extend its applicability to silage aerobic stability.
Data Availability Statement
The original contributions presented in the study are included in the article/supplementary material, further inquiries can be directed to the corresponding author.
Author Contributions
AM and YS designed the study. WBu, GS, WBe, CM, and YS devised the instrument. GS, VR, YW, and YS performed the experiment. YS designed the mathematical frame. GS and YS designed the data process. GS made the statistical analysis. VR conducted the chemical analysis. GS, AM, AL, DG, and YS wrote the manuscript. All authors contributed to the article and approved the submitted version.
Funding
This study was funded by the German Research Foundation [Deutsche Forschungsgemeinschaft (DFG)] under the projects BU 1235/11-1, 414320577 and BU 1235/13-1, 449744781. GS was funded with the Sino–German postdoctoral scholarship joined by Deutscher Akademischer Austauschdienst (DAAD) and China Scholarship Council (CSC).
Conflict of Interest
VR and AM were employed by company ADDCON GmbH.
The remaining authors declare that the research was conducted in the absence of any commercial or financial relationships that could be construed as a potential conflict of interest.
Publisher’s Note
All claims expressed in this article are solely those of the authors and do not necessarily represent those of their affiliated organizations, or those of the publisher, the editors and the reviewers. Any product that may be evaluated in this article, or claim that may be made by its manufacturer, is not guaranteed or endorsed by the publisher.
Acknowledgments
We thank R. Lutz (working at the Department of Agricultural Engineering, University of Bonn) for his help in our experiment, and we gratefully acknowledge the assistance of Fabian Weber (University of Bonn) and Michael Short in improving an earlier draft of the English text.
References
Arasu, M. V., Jung, M. W., Kim, D. H., Park, H. S., Ilavenil, S., Al-Dhabi, N. A., et al. (2015). Identification and phylogenetic characterization of novel Lactobacillus plantarum species and their metabolite profiles in grass silage. Ann. Microbiol. 65, 15–25. doi: 10.1007/s13213-014-0830-2
Blajman, J. E., Vinderola, G., Cuatrin, A., Lingua, M. S., and Páez, R. B. (2020). Technological variables influencing the growth and stability of a silage inoculant based on spray-dried lactic acid bacteria. J. Appl. Microbiol. 129, 1486–1496. doi: 10.1111/jam.14750
Bolsen, K. K., Aschbell, G., and Weinberg, Z. G. (1996). Silage fermentation and silage additives – Review. Asian-Australas. J. Anim. Sci. 9, 483–493. doi: 10.5713/ajas.1996.483
Borreani, G., and Tabacco, E. (2010). The relationship of silage temperature with the microbiological status of the face of corn silage bunkers. J. Dairy Sci. 93, 2620–2629. doi: 10.3168/jds.2009-2919
Clarke, F. H. (1976). The generalized problem of Bolza. SIAM J. Control Optim. 14, 682–699. doi: 10.1137/0314044
Condon, S. (1987). Responses of lactic acid bacteria to oxygen. FEMS Microbiol. Rev. 46, 269–280. doi: 10.1111/j.1574-6968.1987.tb02465.x
Danner, H., Holzer, M., Mayrhuber, E., and Braun, R. (2003). Acetic acid increases stability of silage under aerobic conditions. Appl. Environ. Microbiol. 69, 562–567. doi: 10.1128/AEM.69.1.562-567.2003
Graves, T., Narendranath, N. V., Dawson, K., and Power, R. (2006). Effect of pH and lactic or acetic acid on ethanol productivity by Saccharomyces cerevisiae in corn mash. J. Ind. Microbiol. Biotechnol. 33, 469–474. doi: 10.1007/s10295-006-0091-6
Holzer, M., Mayrhuber, E., Danner, H., and Braun, R. (2003). The role of Lactobacillus buchneri in forage preservation. Trends Biotechnol. 21, 282–287. doi: 10.1016/S0167-7799(03)00106-9
Honig, H. (1990). “Evaluation of aerobic stability in Grass and forage reports,” in Proceedings of the Eurobac Conference, Uppsala, 76–82.
Kung, L., and Ranjit, N. K. (2001). The effect of Lactobacillus buchneri and other additives on the fermentation and aerobic stability of barley silage. J. Dairy Sci. 84, 1149–1155. doi: 10.3168/jds.S0022-0302(01)74575-4
Kung, L., Shaver, R. D., Grant, R. J., and Schmidt, R. J. (2018a). Silage review: interpretation of chemical, microbial, and organoleptic components of silages. J. Dairy Sci. 101, 4020–4033. doi: 10.3168/jds.2017-13909
Kung, L., Smith, M. L., da Silva, E. B., Windle, M. C., da Silva, T. C., and Polukis, S. A. (2018b). An evaluation of the effectiveness of a chemical additive based on sodium benzoate, potassium sorbate, and sodium nitrite on the fermentation and aerobic stability of corn silage. J. Dairy Sci. 101, 5949–5960.
Kung, L., Stocks, M., and Lin, C. J. (2003). Silage Additives, eds D. R. Buxton, R. E. Muck, and J. H. Harrison (Madison, WI: American Society of Agronomy Inc), 31–93. doi: 10.2134/agronmonogr42.c7
Li, M., Shan, G., Zhou, H., Buescher, W., Maack, C., Jungbluth, K. H., et al. (2017). CO2 production, dissolution and pressure dynamics during silage production: multi-sensor-based insight into parameter interactions. Sci. Rep. 7:14721. doi: 10.1038/s41598-017-14187-1
Li, T., Chang, H., and Wu, J. (2013). Nonnegative signal decomposition with supervision. Math Proble Eng. 2013, 1–8. doi: 10.1155/2013/620537
McEniry, J., Forristal, P. D., and O’Kiely, P. (2011). Gas composition of baled grass silage as influenced by the amount, stretch, colour and type of plastic stretch-film used to wrap the bales, and by the frequency of bale handling. Grass Forage Sci. 66, 277–289. doi: 10.1111/j.1365-2494.2011.00788.x
Muck, R. E. (2004). Effects of corn silage inoculants on aerobic stability. Trans. ASAE 47, 1011–1016. doi: 10.13031/2013.16571
Muck, R. E., Nadeau, E. M. G., McAllister, T. A., Contreras-Govea, F. E., Santos, M. C., and Kung, L. (2018). Silage review: recent advances and future uses of silage additives. J. Dairy Sci. 101, 3980–4000. doi: 10.3168/jds.2017-13839
Muck, R. E., and Pitt, R. E. (1994). Aerobic deterioration in corn silage relative to the silo face. Trans. ASAE 37, 735–743. doi: 10.13031/2013.28134
Oliveira, A. S., Weinberg, Z. G., Ogunade, I. M., Cervantes, A. A. P., Arriola, K. G., Jiang, Y., et al. (2017). Meta-analysis of effects of inoculation with homofermentative and facultative heterofermentative lactic acid bacteria on silage fermentation, aerobic stability, and the performance of dairy cows. J. Dairy Sci. 100, 4587–4603. doi: 10.3168/jds.2016-11815
Oude Elferink, S. J. W. H., Krooneman, J., Gottschal, J. C., Spoelstra, S. F., Faber, F., and Driehuis, F. (2001). Anaerobic conversion of lactic acid to acetic acid and 1,2-propanediol by Lactobacillusbuchneri. Appl. Environ. Microbiol. 67, 125–132. doi: 10.1128/AEM.67.1.125-132.2001
Pahlow, G., Muck, R. E., Driehuis, F., Elferink, S. J. O., and Spoelstra, S. F. (2003). “Microbiology of ensiling,” in Silage Science and Technology, eds D. R. Buxton, R. E. Muck, and J. H. Harrison (Madison, WI: American Society of Agronomy Inc), 31–93. doi: 10.2134/agronmonogr42.c2
Queiroz, O. C. M., Arriola, K. G., Daniel, J. L. P., and Adesogan, A. T. (2013). Effects of 8 chemical and bacterial additives on the quality of corn silage. J. Dairy Sci. 96, 5836–5843. doi: 10.3168/jds.2013-6691
Ranjit, N. K., and Kung, L. (2000). The effect of Lactobacillus buchneri, Lactobacillus plantarum, or a chemical preservative on the fermentation and aerobic stability of corn silage. J. Dairy Sci. 83, 526–535. doi: 10.3168/jds.S0022-0302(00)74912-5
Robinson, P. H., and Swanepoel, N. (2016). Impacts of a polyethylene silage pile underlay plastic with or without enhanced oxygen barrier (EOB) characteristics on preservation of whole crop maize silage, as well as a short investigation of peripheral deterioration on exposed silage faces. Anim. Feed Sci. Technol. 215, 13–24. doi: 10.1016/j.anifeedsci.2016.02.001
Sargent, R. W. H. (2000). Optimal control. J. Comput. Appl. Math. 124, 361–371. doi: 10.1016/S0377-0427(00)00418-0
Shan, G., Buescher, W., Maack, C., Lipski, A., Acir, I., Trimborn, M., et al. (2021). Dual sensor measurement shows that temperature outperforms pH as an early sign of aerobic deterioration in maize silage. Sci. Rep. 11:8686. doi: 10.1038/s41598-021-88082-1
Shan, G., Buescher, W., Maack, C., Zhou, H., and Sun, Y. (2019). An automatic smart measurement system with signal decomposition to partition dual-source CO2 flux from maize silage. Sens. Actuators B 300:127053. doi: 10.1016/j.snb.2019.127053
Sun, Y., Li, M., Cheng, Q., Jungbluth, K. H., Maack, C., Buescher, W., et al. (2015). Tracking oxygen and temperature dynamics in maize silage-novel application of a Clark oxygen electrode. Biosyst. Eng. 139, 60–65. doi: 10.1016/j.biosystemseng.2015.08.004
Tabacco, E., Piano, S., Revello-Chion, A., and Borreani, G. (2011). Effect of Lactobacillus buchneri LN4637 and Lactobacillus buchneri LN40177 on the aerobic stability, fermentation products, and microbial populations of corn silage under farm conditions. J. Dairy Sci. 94, 5589–5598. doi: 10.3168/jds.2011-4286
Weinberg, Z. G., and Ashbell, G. (2003). Engineering aspects of ensiling. Biochem. Eng. J. 13, 181–188. doi: 10.1016/S1369-703X(02)00130-4
Wilkinson, J. M., and Davies, D. R. (2012). The aerobic stability of silage: key findings and recent developments. Grass Forage Sci. 68, 1–19. doi: 10.1111/j.1365-2494.2012.00891.x
Keywords: lactic acid bacteria (LAB), multi-sensor mini-bioreactor (MSMB), fermentation, silage additive, metabolic sensitivity, pH, carbon dioxide (CO2), ethanol (EtOH)
Citation: Shan G, Rosner V, Milimonka A, Buescher W, Lipski A, Maack C, Berchtold W, Wang Y, Grantz DA and Sun Y (2021) A Multi-Sensor Mini-Bioreactor to Preselect Silage Inoculants by Tracking Metabolic Activity in situ During Fermentation. Front. Microbiol. 12:673795. doi: 10.3389/fmicb.2021.673795
Received: 28 February 2021; Accepted: 29 June 2021;
Published: 12 August 2021.
Edited by:
Fuyu Yang, China Agricultural University, ChinaReviewed by:
Roberta Comunian, Sardegna Agricoltura, ItalyYanhong Yan, Sichuan Agricultural University, China
Copyright © 2021 Shan, Rosner, Milimonka, Buescher, Lipski, Maack, Berchtold, Wang, Grantz and Sun. This is an open-access article distributed under the terms of the Creative Commons Attribution License (CC BY). The use, distribution or reproduction in other forums is permitted, provided the original author(s) and the copyright owner(s) are credited and that the original publication in this journal is cited, in accordance with accepted academic practice. No use, distribution or reproduction is permitted which does not comply with these terms.
*Correspondence: Yurui Sun, eXN1bkB1bmktYm9ubi5kZQ==
†These authors have contributed equally to this work