- 1College of Pharmacy, Shenzhen Technology University, Shenzhen, China
- 2Guangdong Provincial Key Laboratory of Regional Immunity and Diseases, Department of Pathogen Biology, School of Medicine, Shenzhen University, Shenzhen, China
Tuberculosis (TB) continues to threaten many peoples’ health worldwide, regardless of their country of residence or age. The current diagnosis of TB still uses mainly traditional, time-consuming, and/or culture-based techniques. Efforts have focused on discovering new biomarkers with higher efficiency and accuracy for TB diagnosis. Proteomics—the systematic study of protein diversity—is being applied to the discovery of novel protein biomarkers for different types of diseases. Mass spectrometry (MS) technology plays a revolutionary role in proteomics, and its applicability benefits from the development of other technologies, such as matrix-based and immune-based methods. MS and derivative strategies continuously contribute to disease-related discoveries, and some promising proteomic biomarkers for efficient TB diagnosis have been identified, but challenges still exist. For example, there are discrepancies in the biomarkers identified among different reports and the diagnostic accuracy of clinically applied proteomic biomarkers. The present review summarizes the current status and future perspectives of proteomics in the field of TB biomarker discovery and aims to elicit more promising findings for rapid and accurate TB diagnosis.
Introduction
Tuberculosis (TB), caused by the pathogenic bacteria Mycobacterium tuberculosis (Mtb), continues to be a leading public health threat that affects all countries and age groups (Zumla et al., 2013). In 2020, approximately 10 million people developed TB, and 1.3 million people died from the infection worldwide. Of increasing concern, a total of 157,903 drug-resistant cases were reported, with 132,222 cases of multidrug- or rifampicin-resistant TB and 25,681 cases of pre-extensively drug-resistant TB or extensively drug-resistant TB detected, although this was a large fall (of 22%) from the total of 201,997 people detected with drug-resistant TB in 2019 (WHO, 2021). Tuberculosis control strategies aim to reduce the spread of the infection and cure infectious TB patients, who need rapid and accurate diagnosis to facilitate the administration of prompt anti-TB treatment, thereby helping to reduce the transmission of TB and development of drug resistance.
Mtb is an intracellular pathogen that preferentially infects host macrophages and primarily resides within lung granulomas (Brites and Gagneux, 2015; Elkington et al., 2021), making directly detecting Mtb in human body fluids, such as blood, saliva, or urine, impossible. A current challenge in TB diagnosis is the development of rapid point-of-care tests. Sputum smear microscopy is the most common way of diagnosing TB worldwide (Steingart et al., 2006; Molicotti et al., 2014); however, the sensitivity of sputum smear microscopy ranges from 30 to 60% and is largely dependent on the operator and the abundance of Mtb in the samples (Molicotti et al., 2014). Microbiological culture is considered a diagnostic gold standard, but it requires several weeks and laboratory containment facilities to culture and identify Mtb in samples due to the slow growth rate and biohazard level of the microbe (Lagier et al., 2015). The rapid molecular-based diagnostic test Xpert MTB/RIF has high sensitivity and specificity for the diagnosis of smear-positive sputum TB patients (Steingart et al., 2013); however, Xpert MTB/RIF has a lower sensitivity for smear-negative sputum samples, leaving the diagnosis of a significant proportion of TB-infected cases reliant on diagnostic tests with sub-optimal accuracy.
Recently, many researchers have focused on the discovery of host biomarkers for TB diagnostics. Host immune responses to Mtb infection (Dey and Bishai, 2014) leave traceable signals within the host that may prove valuable for the accurate diagnosis and/or prognosis of TB (Parida and Kaufmann, 2010). Increasingly, investigators are validating the feasibility and accuracy of using proteomic signatures for TB diagnosis and prognosis (De Groote et al., 2017b; Penn-Nicholson et al., 2019). For example, our team previously screened for and validated key proteomic TB biomarkers using an antibody-based array for whole-blood samples that were stimulated with pooled Mtb peptides (ESAT-6 and CFP-10 derived peptides) (Chen et al., 2009) or mitogen, and we successfully identified an eight-protein bio-signature of I-TAC, I-309, MIG, granulysin, FAP, MEP1B, furin, and LYVE-1. The combination of the eight biomarkers allowed us to distinguish TB from healthy control individuals in a test cohort with a specificity and sensitivity of 83% (95% CI, 71–91%) and 76% (95% CI, 56–90%), respectively (Yang et al., 2020). In this review, we summarize the proteomics research approaches followed over the past several years, focusing on the progress of TB biomarker discovery via proteomics, with the hope of eliciting more promising biomarker discoveries for rapid and accurate TB diagnosis.
Proteomics Research Approaches
Proteomics is the systematic study of the proteome with the aim of uncovering the nature of sophisticated protein-interaction networks with respect to protein expression, structure, function, and control of biological processes within an organism (Patterson and Aebersold, 2003). By comparing different patterns within the proteome, proteomics has continued to provide a powerful method for studying the changes in protein diversity that accompany health and disease processes, making the clinical diagnosis, prognosis, and even treatment of different diseases possible (Kavallaris and Marshall, 2005).
It is the development of technology and informatics that make a new concept achievable, as is the case for proteomics. Figure 1 illustrates the timeline of proteomic technology and protein database development that has promoted proteomics research. However, the proteome of a particular cell type or tissue is a mixture of all the proteins expressed (Wilkins, 2009); thus, protein separation is a prerequisite for single-protein analysis. As early as 1975, two-dimensional polyacrylamide gel electrophoresis (2D-PAGE), which separates proteins based on their size and surface charge, was first employed to separate ribosomal proteins of Escherichia coli (Knopf et al., 1975). Mass spectrometry (MS), which is usually applied to the identification of individual protein spots separated by 2D-PAGE, was first described in 1899. It was not, however, until the development of non-destructive large-biomolecule ionization methods, including matrix-assisted laser desorption/ionization (MALDI) (Karas and Hillenkamp, 1988) and electrospray ionization (Fenn et al., 1989), that the widespread application of MS became feasible. Peptide mass fingerprinting (Henzel et al., 2003) and isotope-coded affinity tag (Gygi et al., 1999) approaches were then combined with different MS strategies for the accurate quantification of concurrent peptides from proteins that are found abundantly or even in trace amounts in complex mixtures.
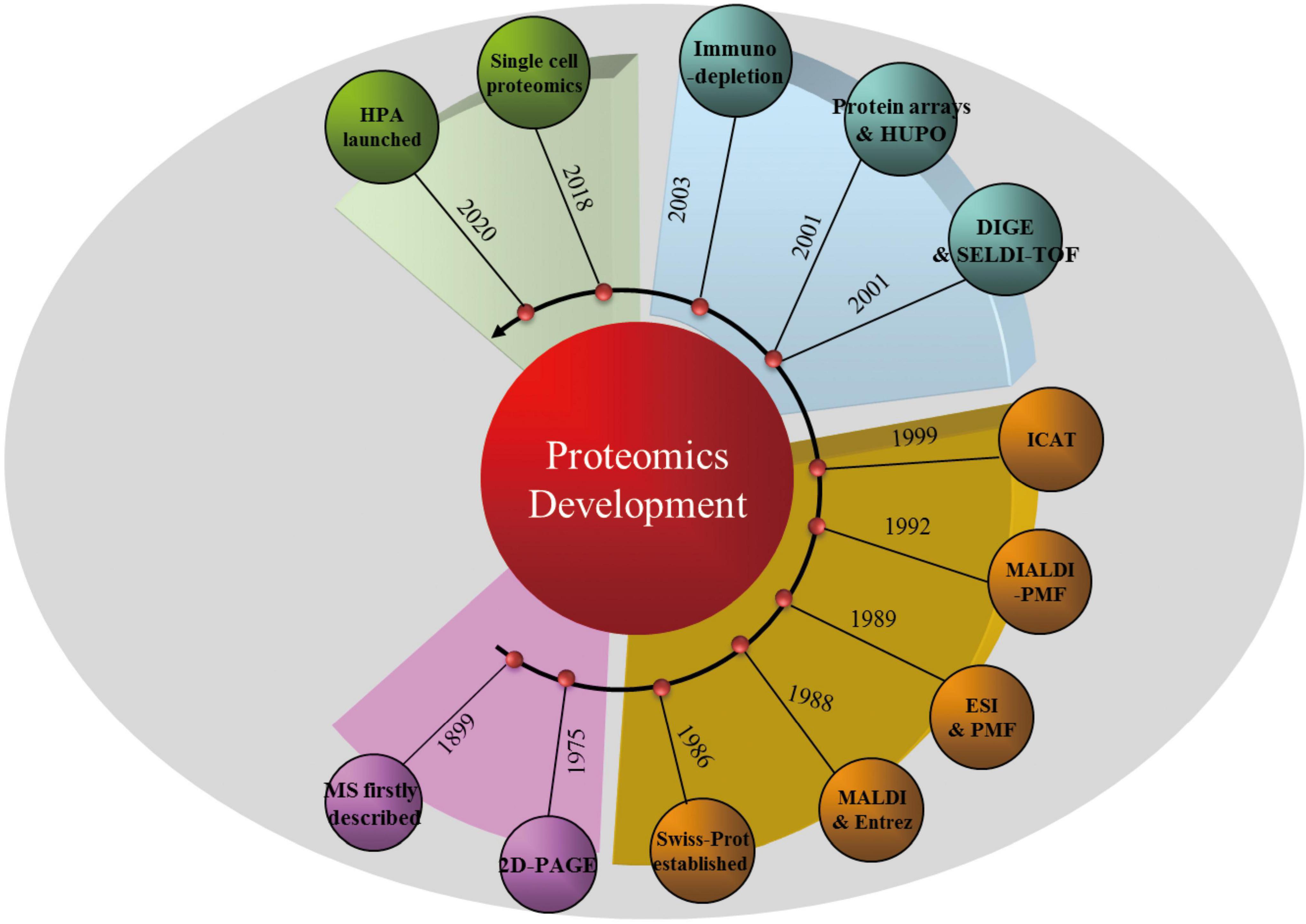
Figure 1. Timeline of proteomic technologies and protein database development. MS, mass spectrometry; 2D-PAGE, two-dimensional polyacrylamide gel electrophoresis; MALDI, matrix-assisted laser desorption/ionization; ESI, electrospray ionization; PMF, peptide mass fingerprinting; ICAT, isotope-coded affinity tag; DIGE, difference-gel 2D-electrophoresis; SELDI-TOF, surface-enhanced laser desorption/ionization time of flight; HUPO, Human Proteome Organization; HPA, Human Protein Atlas.
Benefiting from the rapid development of technology during the 21st century, some classical technologies have helped to further revolutionize proteomics. For example, 2D-PAGE and MS have been developed into techniques such as difference-gel 2D-electrophoresis (Larbi and Jefferies, 2009), surface-enhanced laser desorption/ionization time of flight (SELDI-TOF) MS (Marcos et al., 2013), quantitative chemical cross-linking with MS (Wippel et al., 2021), and immunodepletion techniques for “low-abundance” protein determination (Liu et al., 2021b). Moreover, “protein chips” have been designed and combined with MS approaches for the specific analysis of selected proteins (Zhou et al., 2001). It is important to consider that, coupled with proteomic technologies, proteomics databases, including Swiss-Prot, Entrez, and the Human Proteome Organization, also continue to contribute to the development of proteomics (Kavallaris and Marshall, 2005). Moreover, in addition to other single-cell approaches (Mauger et al., 2021), single-cell proteomics is emerging as a way to identify and quantify the proteins in individual cells (Li et al., 2021). Although there are no high-throughput proteomic “sequencers” available as yet, it is believed that this technology will contribute to the discovery of new aspects of protein and cell biology in the future.
Diagnostic Application of Proteomic Biomarkers for Tuberculosis
Upon Mtb infection, the host cells’ response is to produce and secrete certain effectors to deal with the invading bacteria (Dey and Bishai, 2014; Brites and Gagneux, 2015; Llibre et al., 2021). Blood is the primary vehicle for the transport of either host effectors or Mtb factors and is usually routinely sampled for clinical testing, making blood samples readily accessible for research purposes and diagnostic tests. The recent advances in proteomics make the simultaneous detection of thousands of proteins possible, facilitating the capture of effectors and thus paving the way for alternative and efficient TB biomarker discovery. In this section, we summarize the recent developments in the identification of proteomic biomarkers that can be used for differentiating TB from healthy or other disease status individuals. This has been achieved by categorizing the origin of the biomarkers from either blood samples, urine samples, or other types of human body fluids.
Identification of Tuberculosis Biomarkers in Human Blood Samples
Human blood and derivative samples are ideal for TB diagnosis with respect to convenience, feasibility, and the amount of sample that can be collected. In addition, upon Mtb infection, molecules secreted during host immune responses, such as cytokines, are mainly delivered via the blood. The advantages of blood samples make them a priority choice, and most studies of proteomic biomarkers for TB diagnosis are based on blood samples. Some biomarkers, for example, ESAT6 (Chiappini et al., 2012; Liu et al., 2017), IP-10 (Estevez et al., 2020), and CD161 (Yang et al., 2015), are secreted by either Mtb or the host and have been verified and validated for the detection of Mtb and TB diagnosis. But due to the low chance of detecting Mtb traces in blood samples and the reported inconsistencies among different research findings, more intensive investigations are necessary in the search for further potential biomarkers for TB diagnosis.
Agranoff et al. (2006) undertook research to discriminate between TB-infected and control individuals by utilizing SELDI-TOF MS (von Eggeling et al., 2001; Issaq et al., 2002) to search for proteomic biomarkers in serum samples. In the first phase of the study, they recruited 349 individuals and studied 179 confirmed culture-positive TB samples and 170 controls collected at St George’s Hospital, United Kingdom; Angola; the Gambia; and Uganda. After profiling all the serum samples with weak cation exchange (CM10) protein chip arrays and supervised machine learning classification methods, the authors chose a Gaussian kernel support vector machine classifier to discriminate the proteomic profile of patients with active TB from that of controls, and the diagnosis sensitivity was 93.5%, specificity was 94.9%, and overall diagnostic accuracy of 94% as the best discriminator for the TB and control groups. A second independent and prospectively collected testing set that included 41 validation samples (18 TB and 23 controls) achieved a sensitivity of 88.9% and specificity of 77.2%. This study was robust but did not clarify the identity of the potential protein biomarkers, which hampered their subsequent application in other sets of samples. In addition, the size of the second testing set was limited, and a larger set of testing samples would have provided a more accurate validation.
Another study (De Groote et al., 2017b) reported the execution of a large multi-center study designed to search for a diagnostic serum protein signature for pulmonary TB. Using the 4,000-plex SOMAscan assay (De Groote et al., 2017a), they performed in-depth proteomic analysis of 1,470 serum samples from seven TB-endemic countries: South Africa, Peru, Zimbabwe, Uganda, Vietnam, Colombia, and Bangladesh. A total of 504 samples (252 non-TB and 252 TB) were tested on SOMAscan for biomarker discovery. The identified HR6 model of host response markers, which included SYWC, kallistatin, complement C9, gelsolin, testican-2, and aldolase C, was subjected to testing with a blinded verification set of 204 samples and reached an area under the curve (AUC) of 0.87 (95% CI, 0.81–0.91). Besides the identified HR6 protein signature, several previously described TB markers, such as IP-10, LBP, FCG3B, and TSP4, were also detected in this study. The reason that IP-10 and other known markers were not included in the HR6 signature may be the statistical methods applied or other reasons. Although there were claims that the 4,000-plex SOMAscan assay is able to simultaneously measure > 4,000 proteins in serum samples, many other proteins, and thus other more promising biomarkers, could have been missed.
Tuberculosis (TB) co-infection is a leading cause of death among people living with HIV, and diagnostic biomarker discovery among this group of people is necessary to reduce mortality (Corbett et al., 2003). Singer et al. (2021) analyzed and compared plasma host proteins from subjects from South Africa (n = 30, representing a region of high TB incidence) and the United States (n = 24, representing a region of low TB incidence), and CD14, A2GL, NID1, SCTM1, and A1AG1 were identified as overlapping between both cohorts. The authors further assessed the diagnostic performance of these host proteins using cross-validation and found that panels of 5–12 proteins had excellent accuracy 0–6 (AUC 0.93) at 6–12 months (AUC 0.86) prior to TB diagnosis for the South African cohort and good accuracy 0–6 (AUC 0.74) at 6–12 months (AUC 0.76) prior to TB diagnosis for the United States cohort. In addition, Shen et al. (2020) analyzed 200 HIV-positive plasma samples using data-independent acquisition MS-based proteomics, and they reported that, in combination, the proteins markers AMACR, LDHB, and RAP1B may serve as TB markers for HIV-infected patients (Shen et al., 2020). Overall, the TB diagnosis of patients co-infected with HIV is difficult due to interference from the HIV infection. Intensive studies of large cohorts with diverse genetic backgrounds are needed to achieve accurate and consistent data.
Recently, an increasing number of investigations have reported new proteomic biomarkers for TB diagnosis using either blood, urine, or other body fluid samples (Table 1). It should be noted that discrepancies exist among the different investigation outcomes, even among similar studies (Table 1). In addition, most of the proteomic biomarkers identified in various body fluid samples originated from preliminary investigations, and there is still a long journey ahead before these potential biomarkers can be applied in clinical diagnosis. Moreover, due to the intracellular survival and other adaptation features of the bacterium (Brites and Gagneux, 2015), few studies have discovered mycobacterium-derived proteomic biomarkers that have the potential to be used in the diagnosis of active TB (Hendrickson et al., 2000; Chen et al., 2018). Some additional identified blood-based proteomic biomarkers are summarized in Table 1.
Identification of Tuberculosis Biomarkers in Human Urine Samples
Besides blood samples, urine samples are another common choice for identifying proteomic biomarkers for human TB diagnosis, and some relevant investigations have been reported. In 2021, Liu et al. (2021a) analyzed and compared the urinary proteomic profiles of TB patients and healthy controls. They first screened for potential biomarkers using the liquid chromatography coupled with tandem mass spectrometry (LC–MS/MS) technique on 20 TB and 20 healthy control samples, then further validated the identified proteomic biomarkers in another 52 TB, 52 latent tuberculosis infection (LTBI), and 52 healthy control samples. Based on the data, they concluded that a combination of glutathione peroxidase 3 (P22352), neurotrimin (Q9P121), poliovirus receptor (P15151), signaling lymphocytic activation molecule family 1 (Q13291), and hemicentin-2 (Q8NDA2) could potentially be applied to the diagnosis of TB, with an 82.7% sensitivity for TB diagnosis and a 92.3% specificity for the diagnosis of TB in the LTBI category. By comparing urine samples from 21 active TB, 24 LTBI, and 18 healthy controls via LC–MS/MS, Young et al. identified IGKC, RBP4, PTGDS, AMBP, ORM1, IGCL2, and SECTM1 as potential protein biomarkers for distinguishing TB from LTBI or healthy control samples. However, they did not validate the group of biomarkers in a second cohort and did not clarify the diagnostic sensitivity or specificity (Young et al., 2014). In addition, a unique 21-mer Mtb peptide sequence (VVLGLTVPGGVELLPGVALPR) was identified (Pollock et al., 2018) from urine samples of Zimbabwean patients that showed 95% sequence homology with Mtb oxidoreductase (MRGA423_21210) from the clinical isolate MTB423 (identified in Kerala, India), but the relevance of this occasionally identified Mtb-originating protein biomarker needs to be verified in further investigations.
Identification of Tuberculosis Biomarkers in Other Human Body Fluids
Saliva and sputum, which contain thousands of proteins, mRNA, and bacterial species, have been used widely for biomarker studies and as samples for disease diagnosis and assessment (Ruhl, 2012; Carpenter, 2013; Kaczor-Urbanowicz et al., 2017; Sun et al., 2017). Collecting a saliva/sputum sample is easy, non-invasive, and more acceptable for repeat testing. Several biomarkers for the diagnosis of TB have been identified in saliva by proteomics approaches. Recently, P01011, Q8NCW5, P28072, A0A2Q2TTZ9, and Q99574 were identified using a QExactive Orbitrap mass spectrometer, with which the combined five-protein bio-signature was shown, after leave-one-out cross validation, to yield an AUC of 1.00 (95% CI, 1.00–1.00), sensitivity of 100% (95% CI, 76.2–100%), and specificity of 90.9% (95% CI, 58.7–99.8%) in TB diagnosis (Mutavhatsindi et al., 2021). Using MS, a signature comprising β-integrin, vitamin D-binding protein, uteroglobin, profilin, and cathelicidin antimicrobial peptide in saliva was confirmed to differentiate active TB patients from non-TB patients with an AUC of 0.75 (Bishwal et al., 2019). Mariam and colleagues investigated the sputum proteome of patients with active and latent TB infections as well as community controls using an ultrafast sample-preparation approach (HaileMariam et al., 2018). A 49-protein signature was used to successfully distinguish TB from control subjects; however, this panel of proteins was unable to differentiate LTBI from healthy subjects. In another study, salivary proteomic analysis demonstrated that TB patients exhibit a specific accumulation of proteins related to complement activation, inflammation, and the modulation of the immune response and a decrease in proteins related to glucose and lipid metabolism. A group of proteins, including haptoglobin, alpha-1-acid glycoprotein 1 and 2, immunoglobulin gamma 4 chain, fibrinogens, dermcidin, protein disulfide isomerase, triosephosphate isomerase, and ras GTPase-activating-like protein, are other potential biomarkers for the diagnosis of TB (Mateos et al., 2019).
Challenges and Future Perspectives
Intensive investigations into Mtb–host interactions have broadened and deepened our knowledge on the strategies applied by Mtb to infect hosts and the host responses upon infection. The development of technologies, especially MS, has facilitated proteomic biomarker identification with high efficiency, but there are still some technical challenges slowing down the application of proteomics to the diagnosis of active TB. Sampling complexities (e.g., the timing of sample collection, small-molecule interference, and the coexistence of TB with other types of disease), differences in protein abundances, the presence of isoforms, and post-translational modifications are barriers preventing the identification of accurate and universal biomarkers with current technologies and strategies. More powerful techniques with improved sensitivity will be required, especially for low-abundance proteins and for differentiating protein isoforms and modifications. In addition, there is a risk of diagnostic bias when using a single protein biomarker, and a group of biomarkers within a panel could be detected simultaneously using current technologies, but discrepancies among different reports are increasing, providing confusing or even misleading evidence for the optimum TB diagnostic markers. One reason for the unsatisfactory reproducibility of the discovered biomarkers is that the studied cohorts have differed with regard to their genomic backgrounds, immune responses, or other factors. Large cohorts of samples from various populations that include a diversity of TB patient statuses may be needed to overcome this problem. Moreover, a combination of different “omics” techniques may be useful for improving TB diagnostic accuracy and consistency, and single-cell proteomics holds particular promise as a method that will advance active TB diagnosis in the future (Petelski et al., 2021).
Author Contributions
JG and YC contributed to the conceptualization and design, reviewed the literature, prepared the figures and tables, and wrote the original draft of the manuscript. XZ contributed to literature review and data preparation. XC and YC supervised the data and wrote, reviewed, and edited the manuscript. JG, XC, and YC contributed to the project administration, funding acquisition, and resources. All authors contributed to the article and approved the submitted version.
Funding
This study was supported by the National Natural Science Foundation of China (Grant Nos. 31800064 and 82072252), the Science and Technology Project of Shenzhen (Grant No. JCYJ20180507182049853), and Guangdong Provincial Key Laboratory of Regional Immunity and Diseases (2019B030301009).
Conflict of Interest
The authors declare that the research was conducted in the absence of any commercial or financial relationships that could be construed as a potential conflict of interest.
Publisher’s Note
All claims expressed in this article are solely those of the authors and do not necessarily represent those of their affiliated organizations, or those of the publisher, the editors and the reviewers. Any product that may be evaluated in this article, or claim that may be made by its manufacturer, is not guaranteed or endorsed by the publisher.
References
Agranoff, D., Fernandez-Reyes, D., Papadopoulos, M. C., Rojas, S. A., Herbster, M., Loosemore, A., et al. (2006). Identification of diagnostic markers for tuberculosis by proteomic fingerprinting of serum. Lancet 368, 1012–1021. doi: 10.1016/S0140-6736(06)69342-2
Bark, C. M., Manceur, A. M., Malone, L. L., Nsereko, M., Okware, B., Mayanja, H. K., et al. (2017). Identification of host proteins predictive of early stage Mycobacterium tuberculosis infection. EBioMedicine 21, 150–157. doi: 10.1016/j.ebiom.2017.06.019
Bishwal, S. C., Das, M. K., Badireddy, V. K., Dabral, D., Das, A., Mahapatra, A. R., et al. (2019). Sputum proteomics reveals a shift in vitamin d-binding protein and antimicrobial protein axis in tuberculosis patients. Sci. Rep. 9:1036. doi: 10.1038/s41598-018-37662-37669
Brites, D., and Gagneux, S. (2015). Co-evolution of Mycobacterium tuberculosis and Homo sapiens. Immunol. Rev. 264, 6–24. doi: 10.1111/imr.12264
Carpenter, G. H. (2013). The secretion, components, and properties of saliva. Annu Rev Food Sci Technol. 4, 267–276. doi: 10.1146/annurev-food-030212-182700
Chen, J., Han, Y. S., Yi, W. J., Huang, H., Li, Z. B., Shi, L. Y., et al. (2020). Serum sCD14, PGLYRP2 and FGA as potential biomarkers for multidrug-resistant tuberculosis based on data-independent acquisition and targeted proteomics. J. Cell Mol. Med. 24, 12537–12549. doi: 10.1111/jcmm.15796
Chen, X., Yang, Q., Zhang, M., Graner, M., Zhu, X., Larmonier, N., et al. (2009). Diagnosis of active tuberculosis in China using an in-house gamma interferon enzyme-linked immunospot assay. Clin. Vaccine Immunol. 16, 879–884. doi: 10.1128/CVI.00044-49
Chen, Y., Cao, S., Liu, Y., Zhang, X., Wang, W., and Li, C. (2018). Potential role for Rv2026c- and Rv2421c- specific antibody responses in diagnosing active tuberculosis. Clin. Chim. Acta 487, 369–376. doi: 10.1016/j.cca.2018.09.008
Chiappini, E., Della Bella, C., Bonsignori, F., Sollai, S., Amedei, A., Galli, L., et al. (2012). Potential role of M. tuberculosis specific IFN-gamma and IL-2 ELISPOT assays in discriminating children with active or latent tuberculosis. PLoS One 7:e46041. doi: 10.1371/journal.pone.0046041
Corbett, E. L., Watt, C. J., Walker, N., Maher, D., Williams, B. G., Raviglione, M. C., et al. (2003). The growing burden of tuberculosis: global trends and interactions with the HIV epidemic. Arch. Intern. Med. 163, 1009–1021. doi: 10.1001/archinte.163.9.1009
De Groote, M. A., Higgins, M., Hraha, T., Wall, K., Wilson, M. L., Sterling, D. G., et al. (2017a). Highly multiplexed proteomic analysis of quantiferon supernatants to identify biomarkers of latent tuberculosis infection. J. Clin. Microbiol. 55, 391–402. doi: 10.1128/JCM.01646-1616
De Groote, M. A., Sterling, D. G., Hraha, T., Russell, T. M., Green, L. S., Wall, K., et al. (2017b). Discovery and validation of a six-marker serum protein signature for the diagnosis of active pulmonary tuberculosis. J. Clin. Microbiol. 55, 3057–3071. doi: 10.1128/JCM.00467-417
Dey, B., and Bishai, W. R. (2014). Crosstalk between Mycobacterium tuberculosis and the host cell. Semin. Immunol. 26, 486–496. doi: 10.1016/j.smim.2014.09.002
Elkington, P., Polak, M. E., Reichmann, M. T., and Leslie, A. (2021). Understanding the tuberculosis granuloma: the matrix revolutions. Trends Mol. Med. 28, 143–154. doi: 10.1016/j.molmed.2021.11.004
Estevez, O., Anibarro, L., Garet, E., Pallares, A., Pena, A., Villaverde, C., et al. (2020). Identification of candidate host serum and saliva biomarkers for a better diagnosis of active and latent tuberculosis infection. PLoS One 15:e0235859. doi: 10.1371/journal.pone.0235859
Fenn, J. B., Mann, M., Meng, C. K., Wong, S. F., and Whitehouse, C. M. (1989). Electrospray ionization for mass spectrometry of large biomolecules. Science 246, 64–71. doi: 10.1126/science.2675315
Fu, Y. R., Yi, Z. J., Guan, S. Z., Zhang, S. Y., and Li, M. (2012). Proteomic analysis of sputum in patients with active pulmonary tuberculosis. Clin. Microbiol. Infect. 18, 1241–1247. doi: 10.1111/j.1469-0691.2012.03824.x
Garay-Baquero, D. J., White, C. H., Walker, N. F., Tebruegge, M., Schiff, H. F., Ugarte-Gil, C., et al. (2020). Comprehensive plasma proteomic profiling reveals biomarkers for active tuberculosis. JCI Insight 5:e137427. doi: 10.1172/jci.insight.137427
Gygi, S. P., Rist, B., Gerber, S. A., Turecek, F., Gelb, M. H., and Aebersold, R. (1999). Quantitative analysis of complex protein mixtures using isotope-coded affinity tags. Nat. Biotechnol. 17, 994–999. doi: 10.1038/13690
Hadizadeh Tasbiti, A., Yari, S., Siadat, S. D., Karimipoor, M., Badmasti, F., Masoumi, M., et al. (2021). Comparing mRNA expression and protein abundance in MDR Mycobacterium tuberculosis: novel protein candidates, Rv0443, Rv0379 and Rv0147 as TB potential diagnostic or therapeutic targets. Biotechnol. Rep. (Amst) 30:e00641. doi: 10.1016/j.btre.2021.e00641
HaileMariam, M., Eguez, R. V., Singh, H., Bekele, S., Ameni, G., Pieper, R., et al. (2018). S-Trap, an ultrafast sample-preparation approach for shotgun proteomics. J. Proteome Res. 17, 2917–2924. doi: 10.1021/acs.jproteome.8b00505
Hendrickson, R. C., Douglass, J. F., Reynolds, L. D., McNeill, P. D., Carter, D., Reed, S. G., et al. (2000). Mass spectrometric identification of mtb81, a novel serological marker for tuberculosis. J. Clin. Microbiol. 38, 2354–2361. doi: 10.1128/JCM.38.6.2354-2361.2000
Henzel, W. J., Watanabe, C., and Stults, J. T. (2003). Protein identification: the origins of peptide mass fingerprinting. J. Am. Soc. Mass Spectrom. 14, 931–942. doi: 10.1016/S1044-0305(03)00214-219
Issaq, H. J., Veenstra, T. D., Conrads, T. P., and Felschow, D. (2002). The SELDI-TOF MS approach to proteomics: protein profiling and biomarker identification. Biochem. Biophys. Res. Commun. 292, 587–592. doi: 10.1006/bbrc.2002.6678
Jacobs, R., Tshehla, E., Malherbe, S., Kriel, M., Loxton, A. G., Stanley, K., et al. (2016). Host biomarkers detected in saliva show promise as markers for the diagnosis of pulmonary tuberculosis disease and monitoring of the response to tuberculosis treatment. Cytokine 81, 50–56. doi: 10.1016/j.cyto.2016.02.004
Kaczor-Urbanowicz, K. E., Martin Carreras-Presas, C., Aro, K., Tu, M., Garcia-Godoy, F., and Wong, D. T. (2017). Saliva diagnostics - current views and directions. Exp. Biol. Med. (Maywood) 242, 459–472. doi: 10.1177/1535370216681550
Karas, M., and Hillenkamp, F. (1988). Laser desorption ionization of proteins with molecular masses exceeding 10,000 daltons. Anal. Chem. 60, 2299–2301. doi: 10.1021/ac00171a028
Kavallaris, M., and Marshall, G. M. (2005). Proteomics and disease: opportunities and challenges. Med. J. Aust. 182, 575–579. doi: 10.5694/j.1326-5377.2005.tb06817.x
Knopf, U. C., Sommer, A., Kenny, J., and Traut, R. R. (1975). A new two-dimensional gel electrophoresis system for the analysis of complex protein mixtures: application to the ribosome of E. coli. Mol. Biol. Rep. 2, 35–40. doi: 10.1007/BF00357295
Lagier, J. C., Edouard, S., Pagnier, I., Mediannikov, O., Drancourt, M., and Raoult, D. (2015). Current and past strategies for bacterial culture in clinical microbiology. Clin. Microbiol. Rev. 28, 208–236. doi: 10.1128/CMR.00110-114
Larbi, N. B., and Jefferies, C. (2009). 2D-DIGE: comparative proteomics of cellular signalling pathways. Methods Mol. Biol. 517, 105–132. doi: 10.1007/978-1-59745-541-1_8
Li, Y., Li, H., Xie, Y., Chen, S., Qin, R., Dong, H., et al. (2021). An integrated strategy for mass spectrometry-based multiomics analysis of single cells. Anal. Chem. 93, 14059–14067. doi: 10.1021/acs.analchem.0c05209
Liu, C., Zhao, Z., Fan, J., Lyon, C. J., Wu, H. J., Nedelkov, D., et al. (2017). Quantification of circulating Mycobacterium tuberculosis antigen peptides allows rapid diagnosis of active disease and treatment monitoring. Proc. Natl. Acad. Sci. U S A. 114, 3969–3974. doi: 10.1073/pnas.1621360114
Liu, J. Y., Jin, L., Zhao, M. Y., Zhang, X., Liu, C. B., Zhang, Y. X., et al. (2011). New serum biomarkers for detection of tuberculosis using surface-enhanced laser desorption/ionization time-of-flight mass spectrometry. Clin. Chem. Lab. Med. 49, 1727–1733. doi: 10.1515/CCLM.2011.634
Liu, J., Jiang, T., Wei, L., Yang, X., Wang, C., Zhang, X., et al. (2013). The discovery and identification of a candidate proteomic biomarker of active tuberculosis. BMC Infect. Dis. 13:506. doi: 10.1186/1471-2334-13-506
Liu, L., Deng, J., Yang, Q., Wei, C., Liu, B., Zhang, H., et al. (2021a). Urinary proteomic analysis to identify a potential protein biomarker panel for the diagnosis of tuberculosis. IUBMB Life 73, 1073–1083. doi: 10.1002/iub.2509
Liu, L., Kwak, H., Lawton, T. L., Jin, S. X., Meunier, A. L., Dang, Y., et al. (2021b). An ultra-sensitive immunoassay detects and quantifies soluble Abeta oligomers in human plasma. Alzheimers Dement. doi: 10.1002/alz.12457 [Epub ahead of print].
Llibre, A., Dedicoat, M., Burel, J. G., Demangel, C., O’Shea, M. K., and Mauro, C. (2021). Host immune-metabolic adaptations upon mycobacterial infections and associated co-morbidities. Front. Immunol. 12:747387. doi: 10.3389/fimmu.2021.747387
Marcos, B., Gou, P., Guardia, M. D., Hortos, M., Colleo, M., Mach, N., et al. (2013). Surface-enhanced laser desorption/ionisation time-of-flight mass spectrometry: a tool to predict pork quality. Meat Sci. 95, 688–693. doi: 10.1016/j.meatsci.2012.10.014
Mateos, J., Estevez, O., Gonzalez-Fernandez, A., Anibarro, L., Pallares, A., Reljic, R., et al. (2019). High-resolution quantitative proteomics applied to the study of the specific protein signature in the sputum and saliva of active tuberculosis patients and their infected and uninfected contacts. J. Proteomics 195, 41–52. doi: 10.1016/j.jprot.2019.01.010
Mauger, S., Monard, C., Thion, C., and Vandenkoornhuyse, P. (2021). Contribution of single-cell omics to microbial ecology. Trends Ecol. Evol. 37, 67–78. doi: 10.1016/j.tree.2021.09.002
Molicotti, P., Bua, A., and Zanetti, S. (2014). Cost-effectiveness in the diagnosis of tuberculosis: choices in developing countries. J. Infect. Dev. Ctries 8, 24–38. doi: 10.3855/jidc.3295
Mutavhatsindi, H., Calder, B., McAnda, S., Malherbe, S. T., Stanley, K., Kidd, M., et al. (2021). Identification of novel salivary candidate protein biomarkers for tuberculosis diagnosis: a preliminary biomarker discovery study. Tuberculosis (Edinb) 130:102118. doi: 10.1016/j.tube.2021.102118
Parida, S. K., and Kaufmann, S. H. E. (2010). The quest for biomarkers in tuberculosis. Drug Discov. Today 15, 148–157. doi: 10.1016/j.drudis.2009.10.005
Patterson, S. D., and Aebersold, R. H. (2003). Proteomics: the first decade and beyond. Nat. Genet. 33(Suppl.), 311–323. doi: 10.1038/ng1106
Penn-Nicholson, A., Hraha, T., Thompson, E. G., Sterling, D., Mbandi, S. K., Wall, K. M., et al. (2019). Discovery and validation of a prognostic proteomic signature for tuberculosis progression: a prospective cohort study. PLoS Med. 16:e1002781. doi: 10.1371/journal.pmed.1002781
Petelski, A. A., Emmott, E., Leduc, A., Huffman, R. G., Specht, H., Perlman, D. H., et al. (2021). Multiplexed single-cell proteomics using SCoPE2. Nat. Protoc. 16, 5398–5425. doi: 10.1038/s41596-021-00616-z
Pollock, N., Dhiman, R., Daifalla, N., Farhat, M., and Campos-Neto, A. (2018). Discovery of a unique Mycobacterium tuberculosis protein through proteomic analysis of urine from patients with active tuberculosis. Microbes Infect. 20, 228–235. doi: 10.1016/j.micinf.2017.12.011
Ruhl, S. (2012). The scientific exploration of saliva in the post-proteomic era: from database back to basic function. Expert Rev. Proteomics 9, 85–96. doi: 10.1586/epr.11.80
Shen, Y., Xun, J., Song, W., Wang, Z., Wang, J., Liu, L., et al. (2020). Discovery of potential plasma biomarkers for tuberculosis in HIV-Infected patients by data-independent acquisition-based quantitative proteomics. Infect. Drug Resist. 13, 1185–1196. doi: 10.2147/IDR.S245460
Singer, S. N., Ndumnego, O. C., Kim, R. S., Ndung’u, T., Anastos, K., French, A., et al. (2021). Plasma host protein biomarkers correlating with increasing Mycobacterium tuberculosis infection activity prior to tuberculosis diagnosis in people living with HIV. EBioMedicine 75:103787. doi: 10.1016/j.ebiom.2021.103787
Steingart, K. R., Henry, M., Ng, V., Hopewell, P. C., Ramsay, A., Cunningham, J., et al. (2006). Fluorescence versus conventional sputum smear microscopy for tuberculosis: a systematic review. Lancet Infect. Dis. 6, 570–581. doi: 10.1016/S1473-3099(06)70578-70573
Steingart, K. R., Sohn, H., Schiller, I., Kloda, L. A., Boehme, C. C., Pai, M., et al. (2013). Xpert(R) MTB/RIF assay for pulmonary tuberculosis and rifampicin resistance in adults. Cochrane Database Syst. Rev. 2014:CD009593. doi: 10.1002/14651858.CD009593.pub2
Sun, Y., Liu, S., Qiao, Z., Shang, Z., Xia, Z., Niu, X., et al. (2017). Systematic comparison of exosomal proteomes from human saliva and serum for the detection of lung cancer. Anal. Chim. Acta 982, 84–95. doi: 10.1016/j.aca.2017.06.005
von Eggeling, F., Junker, K., Fiedle, W., Wollscheid, V., Durst, M., Claussen, U., et al. (2001). Mass spectrometry meets chip technology: a new proteomic tool in cancer research? Electrophoresis 22, 2898–2902. doi: 10.1002/1522-2683(200108)22:14<2898::AID-ELPS2898>3.0.CO;2-A
Wang, J., Zhu, X., Xiong, X., Ge, P., Liu, H., Ren, N., et al. (2018). Identification of potential urine proteins and microRNA biomarkers for the diagnosis of pulmonary tuberculosis patients. Emerg. Microbes Infect. 7:63. doi: 10.1038/s41426-018-0066-65
Wippel, H. H., Chavez, J. D., Tang, X., and Bruce, J. E. (2021). Quantitative interactome analysis with chemical cross-linking and mass spectrometry. Curr. Opin. Chem. Biol. 66:102076. doi: 10.1016/j.cbpa.2021.06.011
Xu, D. D., Deng, D. F., Li, X., Wei, L. L., Li, Y. Y., Yang, X. Y., et al. (2014). Discovery and identification of serum potential biomarkers for pulmonary tuberculosis using iTRAQ-coupled two-dimensional LC-MS/MS. Proteomics 14, 322–331. doi: 10.1002/pmic.201300383
Xu, D., Li, Y., Li, X., Wei, L. L., Pan, Z., Jiang, T. T., et al. (2015). Serum protein S100A9, SOD3, and MMP9 as new diagnostic biomarkers for pulmonary tuberculosis by iTRAQ-coupled two-dimensional LC-MS/MS. Proteomics 15, 58–67. doi: 10.1002/pmic.201400366
Yang, Q., Chen, Q., Zhang, M., Cai, Y., Yang, F., Zhang, J., et al. (2020). Identification of eight-protein biosignature for diagnosis of tuberculosis. Thorax 75, 576–583. doi: 10.1136/thoraxjnl-2018-213021
Yang, Q., Xu, Q., Chen, Q., Li, J., Zhang, M., Cai, Y., et al. (2015). Discriminating active tuberculosis from latent tuberculosis infection by flow cytometric measurement of CD161-expressing T cells. Sci. Rep. 5:17918. doi: 10.1038/srep17918
Young, B. L., Mlamla, Z., Gqamana, P. P., Smit, S., Roberts, T., Peter, J., et al. (2014). The identification of tuberculosis biomarkers in human urine samples. Eur. Respir. J. 43, 1719–1729. doi: 10.1183/09031936.00175113
Zhang, J., Wu, X., Shi, L., Liang, Y., Xie, Z., Yang, Y., et al. (2012). Diagnostic serum proteomic analysis in patients with active tuberculosis. Clin. Chim. Acta 413, 883–887. doi: 10.1016/j.cca.2012.01.036
Zhou, H., Roy, S., Schulman, H., and Natan, M. J. (2001). Solution and chip arrays in protein profiling. Trends Biotechnol 19, S34–S39. doi: 10.1016/S0167-7799(01)01798-X
Keywords: tuberculosis, proteomics, biomarker, diagnosis, mass spectrometry
Citation: Guo J, Zhang X, Chen X and Cai Y (2022) Proteomics in Biomarker Discovery for Tuberculosis: Current Status and Future Perspectives. Front. Microbiol. 13:845229. doi: 10.3389/fmicb.2022.845229
Received: 29 December 2021; Accepted: 24 February 2022;
Published: 26 April 2022.
Edited by:
Xiao-Yong Fan, Fudan University, ChinaReviewed by:
Suereta Fortuin, Stellenbosch University, South AfricaJianping Xie, Southwest University, China
Copyright © 2022 Guo, Zhang, Chen and Cai. This is an open-access article distributed under the terms of the Creative Commons Attribution License (CC BY). The use, distribution or reproduction in other forums is permitted, provided the original author(s) and the copyright owner(s) are credited and that the original publication in this journal is cited, in accordance with accepted academic practice. No use, distribution or reproduction is permitted which does not comply with these terms.
*Correspondence: Yi Cai, caiyi0113@szu.edu.cn; Xinchun Chen, chenxinchun@szu.edu.cn