- 1Center for Environmental Biotechnology, University of Tennessee, Knoxville, TN, United States
- 2Department of Civil and Environmental Engineering, Tickle College of Engineering, University of Tennessee, Knoxville, TN, United States
- 3Environmental Laboratory, U.S. Army Engineer Research and Development Center, Vicksburg, MS, United States
- 4Department of Microbiology, College of Arts and Sciences, The University of Tennessee, Knoxville, TN, United States
- 5Department of Biosystems Engineering and Soil Science, Herbert College of Agriculture, The University of Tennessee, Knoxville, TN, United States
- 6Oak Ridge National Laboratory, Biosciences Division, Oak Ridge, TN, United States
- 7Department of Chemistry, College of Arts and Sciences, The University of Tennessee, Knoxville, TN, United States
- 8Biological and Small Molecule Mass Spectrometry Core, College of Arts and Sciences, The University of Tennessee, Knoxville, TN, United States
- 9University of Tennessee-Oak Ridge Innovation Institute, University of Tennessee, Knoxville, TN, United States
Ultra-high performance liquid chromatography–high-resolution mass spectrometry (UPHLC–HRMS) is used to discover and monitor single or sets of biomarkers informing about metabolic processes of interest. The technique can detect 1000’s of molecules (i.e., metabolites) in a single instrument run and provide a measurement of the global metabolome, which could be a fingerprint of activity. Despite the power of this approach, technical challenges have hindered the effective use of metabolomics to interrogate microbial communities implicated in the removal of priority contaminants. Herein, our efforts to circumvent these challenges and apply this emerging systems biology technique to microbiomes relevant for contaminant biodegradation will be discussed. Chlorinated ethenes impact many contaminated sites, and detoxification can be achieved by organohalide-respiring bacteria, a process currently assessed by quantitative gene-centric tools (e.g., quantitative PCR). This laboratory study monitored the metabolome of the SDC-9™ bioaugmentation consortium during cis-1,2-dichloroethene (cDCE) conversion to vinyl chloride (VC) and nontoxic ethene. Untargeted metabolomics using an UHPLC-Orbitrap mass spectrometer and performed on SDC-9™ cultures at different stages of the reductive dechlorination process detected ~10,000 spectral features per sample arising from water-soluble molecules with both known and unknown structures. Multivariate statistical techniques including partial least squares-discriminate analysis (PLSDA) identified patterns of measurable spectral features (peak patterns) that correlated with dechlorination (in)activity, and ANOVA analyses identified 18 potential biomarkers for this process. Statistical clustering of samples with these 18 features identified dechlorination activity more reliably than clustering of samples based only on chlorinated ethene concentration and Dhc 16S rRNA gene abundance data, highlighting the potential value of metabolomic workflows as an innovative site assessment and bioremediation monitoring tool.
Introduction
Metabolomics (Patti et al., 2012; Viant and Sommer, 2013) has emerged as a powerful technique to monitor microbial processes in biological systems of increasing complexity (Fiehn and Weckwerth, 2003). Specifically, advancements in high-resolution mass spectrometry, such as the Orbitrap mass analyzer, (Fiehn and Weckwerth, 2003; Lu et al., 2010) have allowed to obtain large datasets and generate a more complete picture of the metabolites of a biological system. However, the application of this technique to the monitoring of bioremediation processes is underexplored. Chlorinated solvents, in particular chlorinated ethenes, are common contaminants impacting Superfund sites (Moran et al., 2007) and other groundwater aquifers, and are suspected or proven cancer-causing agents (Huang et al., 2014; Chlorinated Solvents Contamination, 2016). Organohalide-respiring bacteria can detoxify chlorinated ethenes through the stepwise reductive dechlorination to benign ethene (Maymó-Gatell et al., 1997; He et al., 2003; Löffler et al., 2013; Adrian and Löffler, 2016). In this anaerobic respiratory process, chlorinated ethenes are terminal electron acceptors to conserve energy for growth, a process associated with the release of inorganic chloride. Several strains of Dhc have been shown to dechlorinate dichloroethene isomers and vinyl chloride (VC) to ethene (Yang et al., 2017) and the key reductive dehalogenase (RDase) genes involved in this process have been identified (Krajmalnik-Brown et al., 2004; Müller et al., 2004; Lee et al., 2006; Löffler et al., 2013; Zinder, 2016; Yan et al., 2021). The commercial bioaugmentation consortium SDC-9™ is utilized as a bioaugmentation culture to stimulate reductive dechlorination of chlorinated ethenes and ethene formation (Löffler and Edwards, 2006). Documenting this process at field sites is not straightforward because of interferences with the quantitative measurements of chlorinated ethenes and ethene. To establish confidence in detoxification, a multiple lines of evidence approach is desirable, including a direct measure of activity of organohalide-respiring Dhc.
Molecular biological tools (MBTs) targeting biomarker genes, transcripts, and/or proteins of organohalide-respiring bacteria are employed for site assessment and bioremediation monitoring (Amos et al., 2008; Matturro and Rossetti, 2015; Kanitkar et al., 2017). Quantitative PCR (qPCR) targeting of Dehalococcoides mccartyi (Dhc) biomarker genes serves as a robust MBT in bioremediation monitoring regimes, and a Dhc population exceeding cell abundances of 107 l−1 is thought to be sufficiently large to reduce the contaminant concentrations over time periods acceptable for most bioremediation projects (Lu et al., 2006). However, the correlation between Dhc gene copy number and the rate of bioremediation does not provide a direct relationship linking the two, (Lee et al., 2006; Silva and Alvarez, 2008; Michalsen et al., 2022) and the rate can be influenced by environmental factors, such as temperature (Fletcher et al., 2011) or oxygen (Amos et al., 2008). To obtain more direct information about dechlorination activity, MBTs targeting Dhc biomarker transcripts and proteins have been developed; however, the implementation and robustness of these approaches for Dhc monitoring at field sites either have limitations or have yet to be fully validated. These efforts have introduced several biomarkers as possibilities to use monitor the bioremediation strategies associated with the dechlorination process based on the abundances of genes associated with a specific process using qPCR (e.g., Dhc VC RDase genes abundances) (Krajmalnik-Brown et al., 2004; Müller et al., 2004; Löffler et al., 2013) or proteomics (e.g., TceA, VcrA, BvcA protein concentrations) (Solis et al., 2019). However, the mere presence of a metabolic gene or protein does not imply that the host is metabolically active.(Amos et al., 2008).
Metabolomics has the ability to circumvent the limitations of other MBTs by providing direct information about metabolic activity, and an increasing number of metabolomics studies in clinical (Gonzalez-Covarrubias et al., 2022), toxicological (Morgan et al., 2022), and environmental (Wei et al., 2022) settings demonstrated the value of this approach (Pang et al., 2021). Bioremediation of chlorinated ethenes is also often hindered by the process stalling, which leaves behind either cis-1,2-dichloroethene (cDCE) or vinyl chloride (Lihl et al., 2019). Recognizing sites where cDCE/VC stalls are likely to occur would be advantageous, as it would allow timely implementation of adaptive site management decision-making to predict and possibly prevent stalled dechlorination. Supplementing MBTs based on gene copy number with biomarkers of physiological activity would provide a more nuanced understanding of the bioremediation progress and could also help to predict and avoid stalls. Therefore, this work aimed to use metabolomics to identify a set of metabolite biomarkers that could complement current validated MBTs with indicators for the metabolic activity of the dechlorinating consortium. Such targeted analyses for a small set of molecules could be performed using routine analytical instrumentation and methods analogous to those employed in many environmental monitoring laboratories to measure PFAS concentrations. If metabolomics can be used to monitor this process, a snapshot of the in situ metabolic state of the microbiome can be captured and unique profiles/patterns could be identified and associated with Dhc reductive dechlorination activity. For this study, it was hypothesized that organohalide-respiring Dhc produces characteristic metabolic profiles that could be correlated to the activity of the SDC-9™ consortium, and ultra-high performance liquid chromatography—high resolution mass spectrometry (UHPLC-HRMS) metabolomics was used to measure potential biomarkers for the reductive dechlorination processes.
Materials and methods
SDC-9™ consortium growth conditions
The consortium SDC-9™ was provided by APTIM (Lawrenceville, NJ, United States) as a concentrated stock suspension (~109 cells/ml). Glass serum bottles (160 ml) containing 100 ml of bicarbonate-buffered defined mineral salt medium (Löffler et al., 2005) were sealed with rubber stoppers and autoclaved. Once cooled, filter-sterilized vitamin mix (Wolin et al., 1963), lactate (10 mM), and 50 μg/l vitamin B12 were added to the medium. Cultures were then inoculated (5% v/v) directly from the stock culture suspension inside an anoxic chamber filled with hydrogen (3% v/v) and nitrogen (97% v/v). The electron acceptor, cDCE (6 μl, 79.2 μmol) or VC gas (2 ml, 83.3 μmol) was added. Control cultures received no electron acceptor. All cultures were incubated at room temperature (22°C) in the dark under static conditions. Entire cultures were sacrificed before initiation of dechlorination (100% cDCE/VC remaining, t0), at the beginning of dechlorination (>90% cDCE/VC remaining, t1), during active dechlorination (20–80% cDCE/VC remaining, t2-3), and for up to 14 days after dechlorination was completed (0% cDCE/VC remaining, t5-7) (Table 1). Control samples (Con) with no added chlorinated ethenes were also collected as indicated in Table 1.
Analysis of chlorinated ethenes and ethene
Chlorinated ethenes and ethene were analyzed using an Agilent 7,890 gas chromatograph (Yan et al., 2015). Headspace samples (0.2 ml) were directly injected onto a DB-624 capillary column (60 m × 0.32 mm × 1.8 μm) and detected using flame ionization. As dechlorination proceeded, headspace samples for each culture were taken and subjected to GC analysis to determine the concentrations of cDCE, VC, and ethene. The peak areas for these compounds were integrated and converted to normalized concentrations using a combined standard of known concentrations of cDCE, VC, and ethene. The percent of volatile organic compounds (VOCs) remaining in the headspace of each culture were calculated (Figures 1A,B).
Lactate and acetate quantification
At each designated time point, aliquots (1 ml) from replicate cultures were taken and stored at −80°C until analysis. Lactate and acetate were quantified with an Agilent 1,200 series HPLC system equipped with an Aminex HPX-87H column (300 mm × 7.8 mm) and a UV detector set to a wavelength of 210 nm. Peak areas were integrated, and concentrations were determined based on an external calibration curve determined from linear regression using an intercept of 0: Peak Area (Lactate) = 175.27 × Lactate Concentration (mM) (regression R2 = 0.9999) and Peak Area (Acetate) = 77.51 × Acetate Concentration (mM) (regression R2 = 0.9999) (Figure 1E).
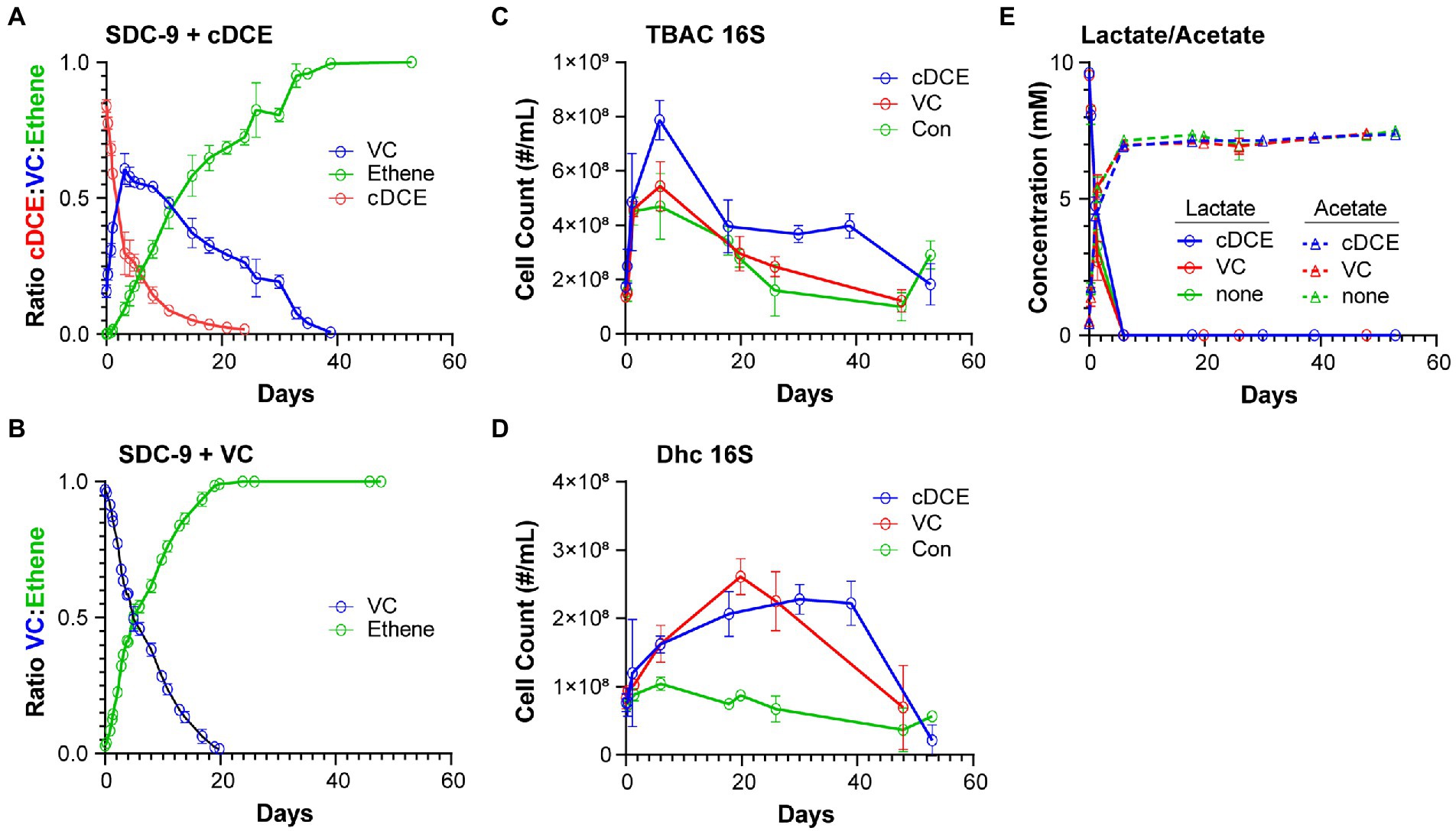
Figure 1. (Chloro)ethene, qPCR, and electron donor data illustrating the performance of SDC-9™ cultures utilizing cDCE or VC as an electron acceptor. (Chloro)ethene concentrations measured during dechlorination of (A) cDCE and (B) VC to ethene; 16S rRNA gene copy numbers for (C) total bacteria (TBAC) and (D) Dhc in cultures under different growth conditions; and (E) lactate consumption and acetate formation in all cultures. Error bars represent standard deviation of replicate samples.
DNA isolation
At each designated, time point aliquots (1 ml) from replicate cultures were collected on 0.2 μm filters (25 mm, Millipore Durapore membrane filter) and stored at −80°C until analysis. DNA was extracted from each filter using the DNeasy PowerLyzer PowerSoil Kit (Qiagen, Valencia, CA) according to the manufacturer’s instructions. To achieve cell lysis, a bead-beating method using the OMNI Bead Ruptor Homogenizer (OMNI International, GA) at 5 m/s for 3 min was used. DNA was eluted with nuclease-free water and quantified using the Qubit dsDNA HS Assay Kit according to the manufacturer’s recommendations (Invitrogen, Carlsbad, CA). DNA was stored at −80°C until qPCR analysis.
qPCR analysis
TaqMan qPCR was conducted in 10-μL reaction volumes containing 5 μl of TaqMan Universal PCR Master Mix No AmpErase UNG (Applied Biosystems, Carlsbad, CA), 0.3 μM of each primer, 0.3 μM of probe and 2 μl of DNA template (undiluted and 1:10 diluted). The PCR cycle parameters applied were as follows: 2 min at 50°C and 10 min at 95°C, followed by 40 cycles of 15 s at 95°C and 1 min at 60°C. All qPCR reactions were performed with the QuantStudio 12 K Flex Real-Time PCR System (Applied Biosystems, Carlsbad, CA) using 384-well qPCR plate. All qPCR assays were performed in triplicate and standard curves were included with every qPCR plate using 10-fold serial dilutions of plasmid DNA for each target gene over 7 orders of magnitude ranging from 1 ng/μL (∼8 log gene copies) to 10−7 ng/μL. The qPCR assay results were analyzed using the QuantStudio 12 K Flex Real-Time PCR System Software (Applied Biosystems, Carlsbad, CA). Previously established primers and probes were used to enumerate total bacteria and Dhc 16S rRNA genes (Figures 1C,D). (Johnson et al., 2005; Ritalahti et al., 2006; Hatt and Löffler, 2012; Hatt et al., 2013).
Water-soluble metabolite extraction from laboratory samples
This method was adapted from Bennett et al. (2008). Individual replicate cultures taken performance liquid chromatography high-resolution mass spectrometry individual replicate cultures taken from the collection of total cultures were sacrificed to collect data for each time point, and a portion (50 ml) of the contents were collected by vacuum filtration through a polycarbonate membrane filter (47 mm diameter, 0.2 μM pore size). Filters were then placed upside down in a plastic Petri dish (47 mm) containing 1.3 ml of chilled (−20°C) extraction solvent (40:40:20 acetonitrile:methanol:water with 0.1 M formic acid). Extraction was continued for 15–20 min at −20°C at which point the filter was flipped right side up and washed. The extraction solvent was transferred to a 2 ml microcentrifuge tube. The filter was then washed with 200 μl chilled (−20°C) extraction solvent and added to the microcentrifuge tube and the combined extracts were centrifuged (5 min, 13.2 rpm, 4°C). The supernatant from each tube was removed and transferred to a clean vial. The pellet was then extracted with 200 μl of extraction solvent (chilled to −20°C) for 15 min at −20°C, after which the samples were once again centrifuged (5 min, 16.6 kg, 4°C). The supernatants were removed and combined with the previously collected supernatant, and the extraction process was repeated once more. The combined supernatants were then dried under a stream of nitrogen and stored at −80°C until analysis.
Ultra-high performance liquid chromatography high-resolution mass spectrometry
This method was adapted from Lu et al. (2010). Prior to analysis, samples were reconstituted in 300 μl of sterile MilliQ water, transferred to an autosampler vial, and placed in an autosampler tray kept at 4°C. Ten microliters from each sample was injected through a Synergi 2.5 micron Hydro-RP 100, 100 × 2.00 mm LC column (Phenomenex, Torrance, CA) kept at 25°C and eluted using the following solvents: (A) 97:3 water:methanol, 10 mM tributylamine, and 15 mM acetic acid, (B) methanol; and gradient: t = 0 min; 0% B; t = 2.5 min, 0% B; t = 5 min, 20% B; t = 7.5 min, 20% B; t = 13 min, 55% B, t = 15.5 min, 95% B; t = 18.5 min, 95% B; t = 19 min, 0% B; t = 25 min, 0% B; using a flow rate of 200 μl/min. The eluent was then introduced into the mass spectrometer (MS) via electrospray ionization (ESI) attached to a Thermo Scientific Exactive Plus Orbitrap MS (Waltham, MA). Data were collected in full scan mode with negative ionization mode using a previously established method (Lu et al., 2010). Samples were run with a spray voltage of 3 kV. The nitrogen sheath gas was set to 10 psi, with a capillary temperature of 320°C; and AGC target was set to 3e6. Samples were analyzed with a resolution of 140,000. A scan window of 85 to 800 m/z (mass-to-charge) was used from 0 to 9 min, and a window of 110 to 1,000 m/z from 9 to 25 min.
Metabolomics data pre-processing, processing, and analysis
Raw spectra files were converted to a universal format, mzML, using msconvert (Chambers et al., 2012). Feature detection was performed using the online MetaboAnalyst 5.0 platform (Pang et al., 2021). Settings were optimized using a pooled sample for quality control (QC) on the UHPLC-Orbitrap platform. Feature detection and removal from instrument blank was also utilized for optimal feature detection. Detected features were then trimmed using a % coefficient of variation (%CV) of less than 40% for pooled samples. The remaining features were normalized using total bacteria 16S qPCR cell count data and the cell normalized ion intensities were used in all following analyses. PLSDA Figures. Normalized features uploaded into the statistical analysis [one-factor] package in the online MetaboAnalyst5.0 platform. Features (40%) were filtered based on interquartile range (IQR) and log transformed prior to PLSDA analysis. Samples were either grouped based on dechlorination activity (active or inactive) or by biological replicates (Figure 2). All samples were included in PLSDA analysis of dechlorination activity (Figure 2A), while only samples within each addition (cDCE or VC) were included in PLSDA analysis of time course (Figure 2B). ANOVA figures. Normalized features uploaded into the statistical analysis [metadata table] package in the online MetaboAnalyst5.0 platform. Features (40%) were filtered based on IQR and log transformed prior to two-way ANOVA analysis. Addition of chlorinated electron acceptor (cDCE, VC, or none) and time point (t0 – t7) were used for ANOVA with interactions. All features that were only significant in time point were removed from post hoc analysis. The remaining features were uploaded into the statistical analysis [one-factor] package in the online MetaboAnalyst5.0 platform, grouped by addition, and one-way ANOVA was performed with Fisher LSD post hoc. This analysis was repeated for the features removed via the IQR data filtration and all significant features from post hoc results (Figure 3) were used for the following analyses. Clustering/heatmap visualization. Clustering was performed using log 10 and mean-centered ion intensities were calculated using Euclidean distances with complete clustering of rows (features) only. Clustering and heatmap visualization of metadata, which are all measurements other than metabolomics for purposes of this manuscript (Figure 4) was performed using the statistical analysis modules of MetaboAnalyst5.0, no data filtering, and log transformed. Clustering and heatmap visualization for significant features (Figure 5A) was performed using the heatmap package available through the R statistical analysis software. Lastly, metabolite biomarkers (Figure 6) were clustered using the statistical analysis modules of MetaboAnalyst5.0 again with no data filtering, and log transformed.
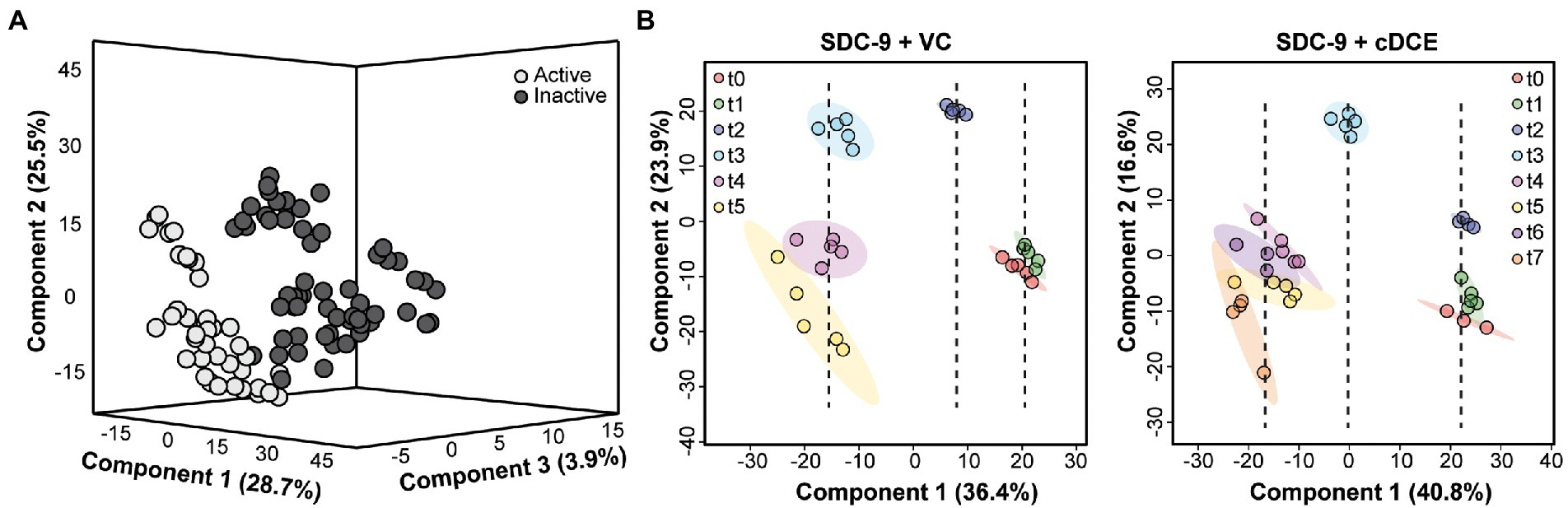
Figure 2. Multivariate analyses of samples classified by dechlorination status or time point. (A) 3D PLSDA showing separation between samples classified by dechlorinating activity (Active or Inactive) but not classified by time; (B) PLSDA showing separation between samples classified by time point, but not dechlorinating activity, for cultures grown with either VC or cDCE.
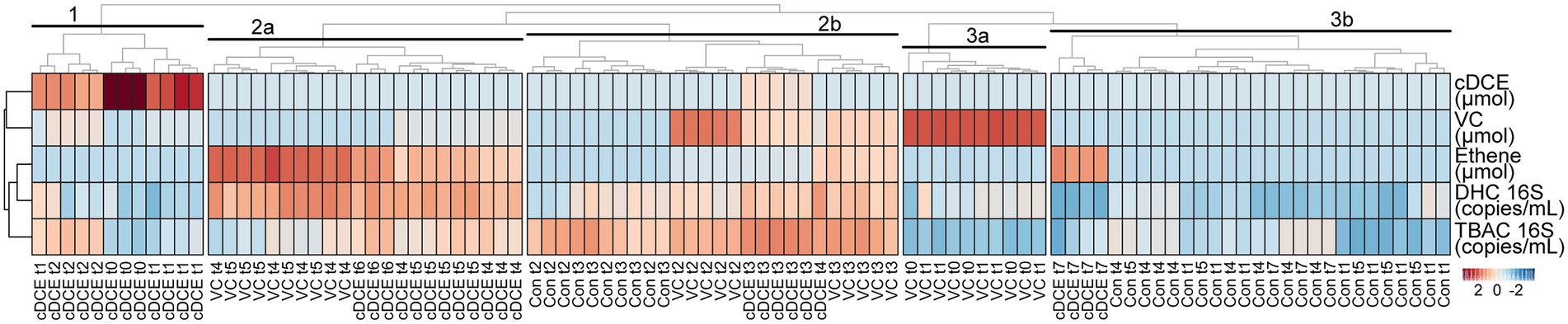
Figure 4. Correlation heatmap of metadata. Abbrev: DHC: Dehalococcoides, TBAC: total bacteria, cDCE: cis-dichloroethene, VC: vinyl chloride.
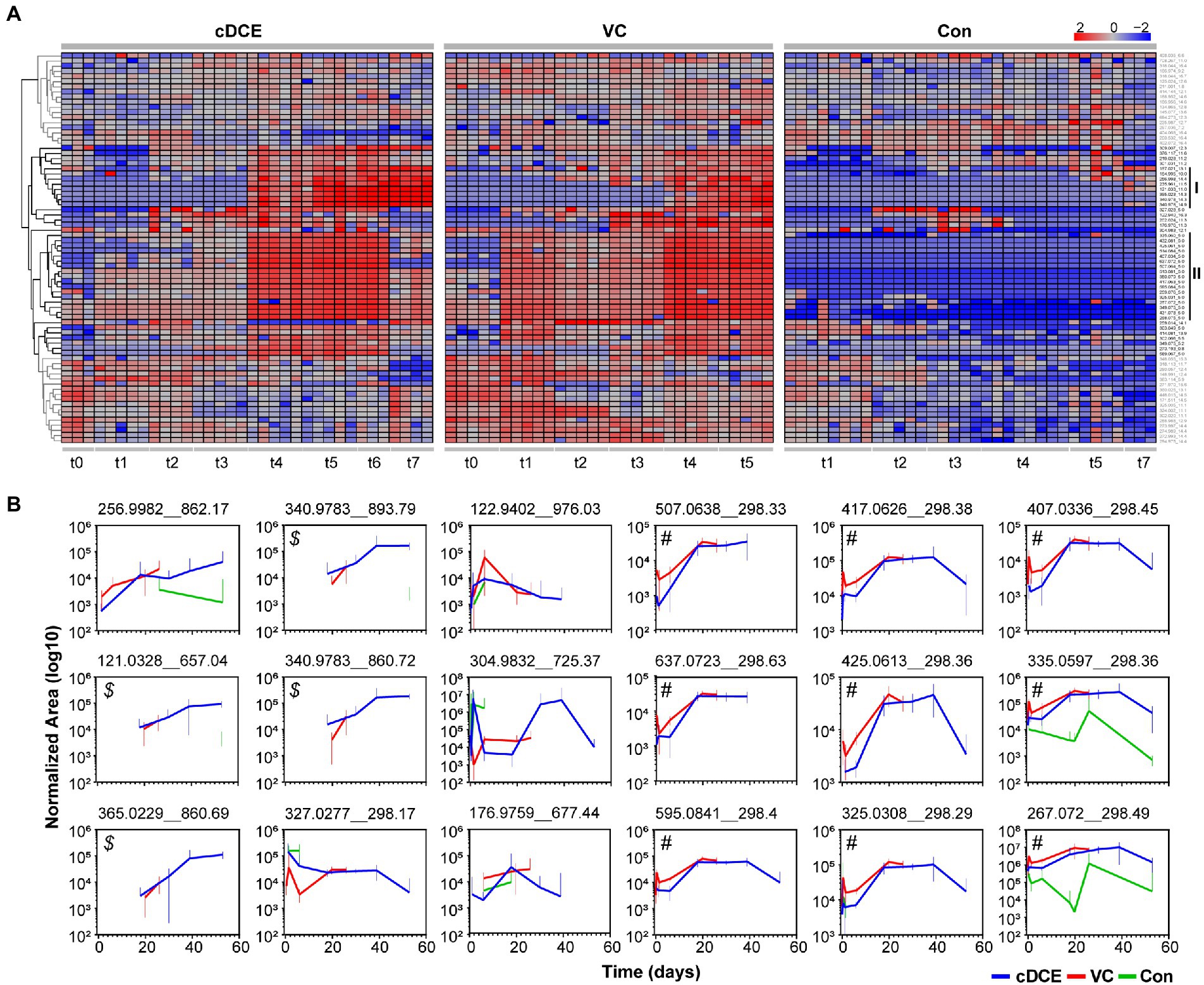
Figure 5. (A) Heatmap of 76 significant features determined to be significant by ANOVA analyses. (B) Temporal abundances of potential biomarkers identified from clustering analyses for cDCE, VC, and no amendment. Error bars represent 95% confidence intervals of replicate data points. Callouts ($ and #) are used for reference in the results and discussion sections.
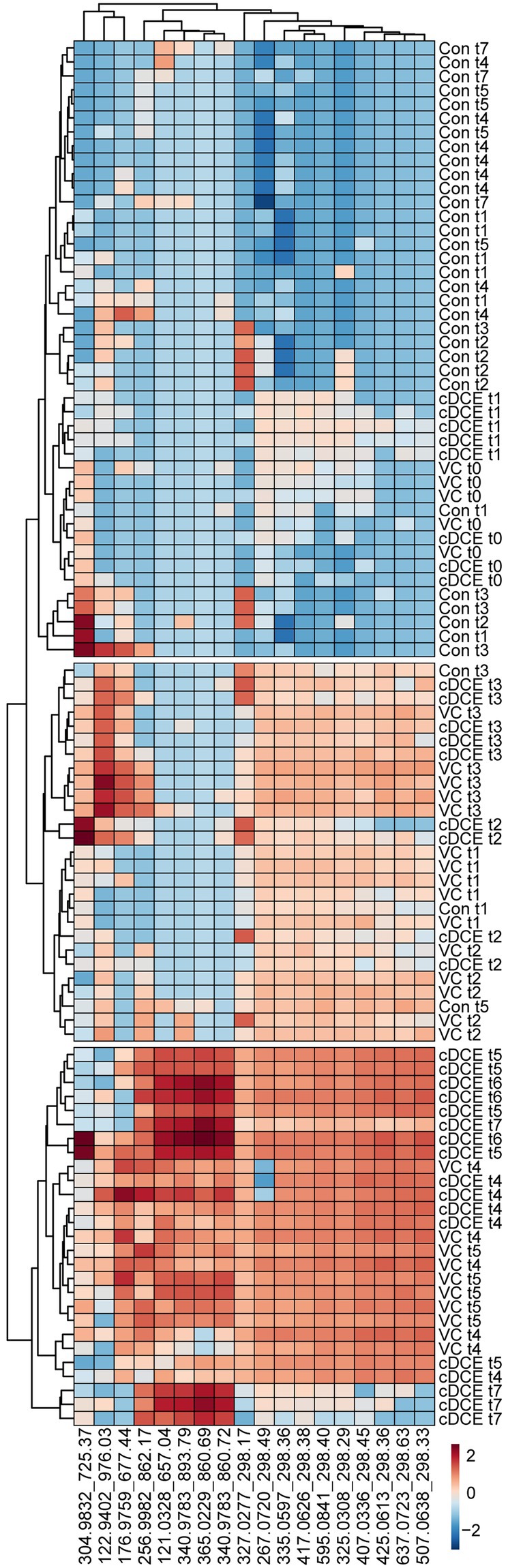
Figure 6. Correlation heatmap of 18 identified biomarkers. Log10 transformed metabolomics data are able to differentiate among dechlorination status and time points.
Results and discussions
Metadata-guided sampling intervals
In order to gain a picture of the metabolic changes that differentiate culture showing active versus inactive dechlorination, the metabolome of the commercial bioaugmentation consortium SDC-9™ was captured at representative points in the dechlorination process. It was determined that the dechlorination process was completed after~20 days for VC amended cultures and after~40 days for cDCE amended cultures (Figures 5A,B). Two of five replicates that received VC as electron acceptor did not complete the dechlorination process and were removed from further analysis. The maximum VC concentration for cDCE amended cultures was reached after~4 days (Figure 5A).
Sampling was performed in intervals with the goal to capture unique stages of the SDC-9™ consortium metabolome as the chlorinated electron acceptor(s) were consumed. The sampling time points were selected to capture changes in metabolism, e.g., when either the ratio of chlorinated ethene species had significantly changed or when either cDCE or VC had been completely dechlorinated as judged by disappearance of the molecules from the headspace (Table 1). The qPCR results (Figures 5C,D) revealed that the total bacterial 16S rRNA gene copies reached 5.4 × 108 ± 9.0 × 107 copies/ml and 7.9 × 108 ± 7.3 × 107 copies/ml in cultures amended with VC and cDCE, respectively. In all cultures, lactate concentrations decreased over the first several days, while the total bacteria 16S rRNA gene copies/ml increased until lactate was completely consumed for all cultures after ~6 days. This corresponded to an increase in acetate concentrations to ~7 mM, which remained constant for the remainder of the experiment in all cultures. When lactate was no longer detected, the total bacterial 16S rRNA gene count decreased for both VC (5.4 × 108 ± 9.0 × 107 to 3.0 × 108 ± 6.4 × 107 copies/ml) and cDCE (7.9 × 108 ± 7.3 × 107 to 4.0 × 108 ± 9.7 × 107 copies/ml) treated cultures. This decrease in the number of total bacteria coincided with an increase in Dhc 16S rRNA genes from 1.6 × 108 ± 2.7 × 107 to 2.6 × 108 ± 2.6 × 107 copies/ml in cultures with VC and 1.6 × 108 ± 1.3 × 107 to 2.1 × 108 ± 3.3 × 107 copies/ml in cultures with cDCE. In control cultures without chlorinated electron acceptor additions, the Dhc 16S rRNA gene copies/ml remained relatively constant over the course of the incubation period (Figure 5E). Due to inconsistent total bacteria numbers from the qPCR results in replicates sampled from VC amended and VC control cultures at t7 (see Table 1), all data from this time point for the VC experiment were removed from further analysis. This variability is likely due to the age of the culture that had been without required substrates for extended periods.
Measuring the dechlorination metabolome
The untargeted UHPLC-HRMS metabolomics approach measured 7,782–11,104 spectral features per sample after pre-processing using the MetaboAnalyst5.0 (Pang et al., 2021) LC–MS spectra processing module (Table 2). Of these features, only ~2.5% could be identified by matching the mass to charge-retention time (m/z-r.t.) pair for the compounds to a library constructed from injected standards. The percent coefficient of variation (%CV) was then used to further trim the number of features for analysis, and only features with less than a 40% CV, as calculated from pooled QC samples were used in further analyses. This left a curated set containing 5,359–6,368 total features (Table 2). As is common in these analyses, the majority of features detected were not accounted for in available metabolite libraries and had unknown structures, and these unknowns are annotated with an m/z-r.t. identifier. Although the molecular structures for these compounds are currently elusive, these compounds can still be useful as biomarkers for the dechlorination process due to the reproducibility of their detection. Further, emerging tools such as those available through the GNPS: Global Natural Products Social Molecular Networking (Wang et al., 2016) platform are accelerating the ability to identify novel metabolites, and work is underway to understand further the unique metabolic processes that lead to dechlorination in this system and to determine whether potential biomarkers for this activity can be measured in field samples.
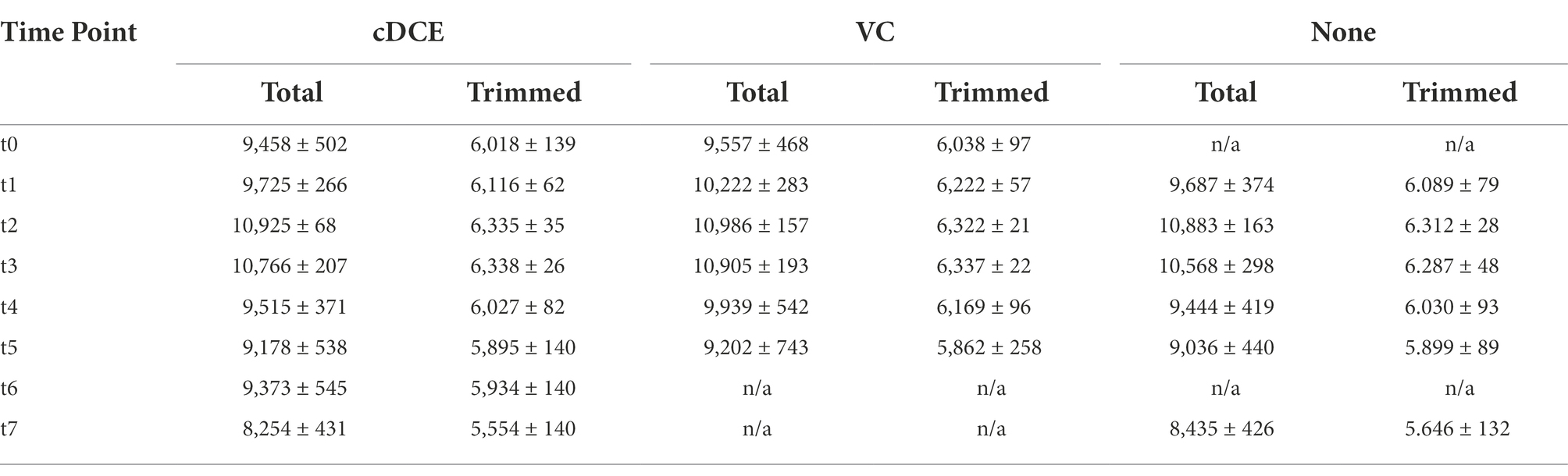
Table 2. Average number of detected features from each sample and time point before and after %CV data processing.
Multivariate analyses differentiate metabolite profiles in dechlorinating versus non-dechlorinating SDC-9™ cultures
The first statistical tool used to determine whether metabolic differences could be observed during the dechlorination process was PLSDA, which allowed testing the hypothesis that samples that were classified by their dechlorination status as judged by metadata also displayed different metabolic profiles. Cultures that did not receive cDCE or VC, that had not started, or that had completed the reductive dechlorination process were grouped as inactive; and all others were grouped as active. Using the multivariate PLSDA approach, minimal separation was seen between these groups when using only two components; however, when the data were plotted using the top three components, good separation between the active and inactive dechlorination metabolomes emerged (Figure 6A).
Next, samples from only the VC- or cDCE amended cultures were analyzed to determine whether differences in the metabolic profiles could be used to determine the extent of dechlorination within these sample sets. The time points of collection were used to classify the samples for these analyses. It was hypothesized that samples from each time point would group together, and that samples from different time points would show divergence based on the extent of dechlorination. Further, components that showed separation among the time points are due to the variability in the metabolite profiles; and the top two components explained ~57% of the variation for cultures that were grown with cDCE and ~ 60% of the variation for cultures that were grown with VC (Figure 6B). Interestingly, the samples seemed to organize based on the extent of dechlorination, which correlated with time. Component 1 for both VC and cDCE amended samples shows separation into three time periods with t2 being the divergence point for VC and t3 for cDCE. These specific samples were collected 1.5–2 days after the start of VC dechlorination indicating there may be metabolites specifically associated with the onset of VC dechlorination. The metabolomes from the beginning stages of dechlorination, t0 and t1, cluster closely, but remain mostly separated, for both VC and cDCE cultures. Next, it was observed that sampling time point t2 cDCE formed a tight cluster distinct from t0 and t1 along the component 2 axis, but not the component 1 axis. This observation indicated that samples collected during the early stages of cDCE dechlorination have a unique metabolic profile. As dechlorination reached completion in the VC amended samples, t3 separated from t2 on the component 1 axis, while t4 and t5 formed a larger cluster that was separated from t3 on the component 2 axis. These data indicated that following consumption of most chlorinated electron acceptor, the metabolic profiles for near complete dechlorination could still be distinguished from those associated with complete dechlorination. As dechlorination progressed in cDCE-amended cultures, the remaining four time points, t4-t7, formed a larger cluster that was distinct from t0-t3 measurements along the component 1 axis indicating a third time period or phase during the dechlorination process, in which the consumption of cDCE was complete but VC dechlorination was still ongoing. Dechlorination was complete at t7 for cDCE and t5 for VC, and the clusters for these samples started to diverge along both component axes indicating that the metabolic profiles are no longer consistent with those observed during active dechlorination.
Assessing the utility of volatile organic compound concentrations and bacterial 16S rRNA gene enumeration metadata for determining dechlorination status
In order to determine whether metabolomics can predict dechlorination status, the metabolome was compared to a set of MBTs commonly used in the field. A correlation heatmap of the metadata, which used concentrations for all measured VOCs and 16S rRNA gene qPCR results, was created and the first five nodes are labeled 1 to 3 (Figure 3). Early stage dechlorination samples clustered separately from all other samples (clusters 1 and 3a), however, cluster 1 was noted to be most divergent from all other samples taken, likely because they were the only ones with high concentration of cDCE (samples cDCE t0-t2). Similarly, time points with high concentrations of only VC (samples VC t0-t1) grouped together in cluster 3a. Samples that were undergoing active dechlorination (VC t2-t3 and cDCE t3 samples plus one cDCE t4 sample) could not be differentiated the from controls taken at similar time points (Con t2-t3) using the metadata collected for this analysis as seen in cluster 2b. This is likely due to the fact that the clustering for these samples is strongly influenced by total bacterial numbers as most other measurements were not sufficiently different to differentiate among sample types. Samples at or near the end of the dechlorination process (VC t4-t5 and cDCE t4-t5) could be differentiated from controls using the metadata, and they grouped in cluster 2a. Finally, it was noted that all of the later stage control samples clustered together and separately from all amended samples except for those from the final time point for the cDCE cultures (cDCE t7), which were taken 14 days after all chlorinated electron acceptors had been consumed. Although several subclusters were observed, e.g., all VC t2 samples grouped separately after two more nodes within cluster 2b, the degree of differentiation is not as large as for the larger clusters, and it is unlikely that the nuances leading to these subclusters would be observable outside of controlled laboratory conditions due to the lack of controls and the expected higher variability of field data. It should be noted that the initial Dhc titer used in this study was high, which could explain the lack of correlation between dechlorination activity and total bacterial 16 S rRNA gene copy numbers. This observation highlights the benefit of having complementary validated techniques to monitor a process as multiple lines of evidence are often needed.
Probing the metabolome for small molecule biomarkers for the reductive dechlorination process
After determining that the metabolome could be used to monitor the dechlorination status of the SDC-9™ cultures, analyses were conducted to explore whether a discrete set of small molecule biomarkers that were indicative of organohalide respiration with cDCE and/or VC as electron acceptor(s) could be identified. To differentiate between features that might be associated with the dechlorination process from those only correlated to bacterial growth not directly linked to organohalide respiration, the metabolome features were subjected to a two-way ANOVA, in which samples from the cultures were grouped by type of addition (cDCE, VC, or none) and the addition by time interactions of the metabolome with time points t0-t7 were assessed (Figure 1). First, the set of 14,130 metabolite features was reduced to 6,574 features by selecting only those that had a CV <40%. Due to limitations within MetaboAnalyst online that limit the total number of features to fewer than the 6,547 that remained in this dataset, interquartile range (IQR) data filtering was used to segregate 40% of the total metabolite features into a set of 2,630 features. Both the IQR (2,630 metabolites) and outer quartile (3,944 metabolites) data sets were analyzed separately using two-way ANOVA to further reduce the number of features before final analyses with one-way ANOVA between subjects to finalize a list of candidate biomarkers. Combined, the two-way ANOVA analyses removed a total of 2,528 features that were either considered non-significant or significant by only time and not type of electron acceptor added. The remaining features (1,599 from the IQR and 3,944 from the outer quartile set) were grouped only by addition (cDCE, VC, or none), and each set was analyzed separately via a one-way ANOVA with Fishers LSD post hoc analysis (Figure 1). Post hoc analyses that placed cultures with no added chlorinated ethenes (VC-none and cDCE-none) in the same direction yielded 76 features in total. This set of features contains the most likely candidates for biomarker potential and was further evaluated. Of the 14,130 features initially detected, only 15 were unique across the time course for VC and cDCE amended cultures and absent in the controls, and 12 (out of 15) of these features were found to be statistically significant among treatments and time points. Further, there were no unique features detected in the control cultures indicating that the metabolism used for the dechlorination process does not downregulate other metabolic functions within the consortium.
This set of 76 features that were statistically correlated to active dechlorination was further analyzed to determine how the concentrations of these molecules related to the phases of the reductive dechlorination process. First, the median area counts for each metabolite feature were subtracted from the absolute area counts of that metabolite for each sample, and the resulting value was log10 normalized before being visualized in a heatmap (Figure 2A). Two distinct clusters of features that are indicative of dechlorination activity were observed (highlighted with black as clusters I and II in Figure 2A), and both have several features not reproducibly detected in the control cultures. Metabolites in cluster I generally increased during the later time points, while the features in cluster II increased over the early time points before decreasing near the end of the experiment for cDCE amended cultures (see cDCE t7). This decrease was not observed in VC amended cultures; however, this could be because the last time point was taken ~20 days earlier at the completion of VC dechlorination, which is much later than the final time point for the cDCE amended cultures. Of the initial 76 metabolite features included in the heatmap, only 41 were found in clusters I and II and utilized for further evaluation. After manual inspection of the peaks for the 41 remaining compounds, it was noted that several of the features arose from isotopes and adducts, which left a total of 18 potential biomarkers for reductive dechlorination once the duplicate measurements for replicate metabolite were removed.
MetaboAnalyst5.0 was only able to annotate two of the 18 features based on the exact mass of these molecules: m/z 121.0328 was annotated as thiodiglycol, and m/z 122.9402 as 1,2,4-trithiolane. Even though only two of the metabolite features have annotated structures, this set of 18 metabolites is still useful as biomarkers for the dechlorination process, and further experiments are underway to determine the structures for these compounds. These metabolites were plotted over time and per condition (Figure 2B), and a set of four features ($ in Figure 2B) began to appear as the consumption of the initial chlorinated ethene was nearly complete for both VC and cDCE amended cultures, and these features increased in abundance until the end of the incubation periods. Another set of nine features (# in Figure 2B) had concentrations that followed the Dhc 16S rRNA gene abundances (monitored by qPCR), and seven of these molecules were only detected in cultures treated with VC or cDCE. Together, these results indicate that there are unique metabolites that are directly associated with both dechlorination activity and the Dhc abundance within the SDC-9™ consortium. Further investigations are warranted to determine whether these compounds can be reproducibly linked with SDC-9™ reductive dechlorination activity in laboratory cultures and at field sites where bioaugmentation with consortium SDC-9™ supports remediation efforts.
Untargeted metabolome analysis of SDC-9™ cultures amended with cDCE or VC as electron acceptors revealed metabolite patterns that distinguish actively dechlorinating cultures from non-dechlorinating cultures (i.e., cultures that had consumed all chlorinated electron acceptors or had not initiated reductive dechlorination activity). A set of 18 small molecule biomarkers that correlated with dechlorination status and/or Dhc abundance were identified, and these molecules revealed a strong correlation with dechlorination status in laboratory SDC-9™ cultures indicating their potential value as process-specific biomarkers (Figure 4). Cluster analyses organized the metabolome into three groups according to the extent of dechlorination, and four metabolite features were detected in increasing temporal abundance in all samples collected during time points t4-t7 from both VC and cDCE amended cultures ($ in Figure 2B). Taken together, these observations suggest that potential metabolome biomarkers for measuring dechlorination activity exist. This study illustrates the potential utility of metabolomics as either a complementary or stand-alone MBT for monitoring the progress of bioremediation at sites impacted by chlorinated ethenes. While demonstration and validation work is needed to ground truth the utility of this technique for field samples, the data presented herein are a crucial first step in the MBT development process and may also lead to a more complete understanding of the metabolic processes that govern reductive dechlorination.
Data availability statement
The datasets generated for this study can be found in the MetaboLights (Haug et al., 2019) database with the identifier MTBLS5219 (www.ebi.ac.uk/metabolights/MTBLS5219).
Author contributions
AM, SC, and FL conceptualized the study. AM, YX, SC, and FL designed the experiments. AM performed the laboratory experiments with assistance from YX and FM. AM performed the analyses. AM, SC, and FL discussed data and interpretation. AM visualized the results. AM, SC, and FL wrote the manuscript. MM contributed to manuscript improvements. All authors contributed to the article and approved the submitted version.
Funding
This work was funded by the US Army Corps of Engineers (W912DW-17-P00) and NIH SBIR R43 (ES030669-01).
Acknowledgments
The authors would like to thank Paul B. Hatzinger and Simon Vainberg with Aptim for graciously donating SDC-9™ cultures. The authors would like to acknowledge Hector F. Castro and Sara Howard of the BSMMSC for help with the metabolomics analysis, and Cynthia Smith for her help with DNA extraction. Samples were processed at the Biological and Small Molecule Mass Spectrometry Core (BSMMSC), University of Tennessee, Knoxville, TN (RRID: SCR_021368).
Conflict of interest
The authors declare that the research was conducted in the absence of any commercial or financial relationships that could be construed as a potential conflict of interest.
Publisher’s note
All claims expressed in this article are solely those of the authors and do not necessarily represent those of their affiliated organizations, or those of the publisher, the editors and the reviewers. Any product that may be evaluated in this article, or claim that may be made by its manufacturer, is not guaranteed or endorsed by the publisher.
References
Amos, B. K., Ritalahti, K. M., Cruz-Garcia, C., Padilla-Crespo, E., and Löffler, F. E. (2008). Oxygen effect on Dehalococcoides viability and biomarker quantification. Environ. Sci. Technol. 42, 5718–5726. doi: 10.1021/es703227g
Bennett, B. D., Yuan, J., Kimball, E. H., and Rabinowitz, J. D. (2008). Absolute quantitation of intracellular metabolite concentrations by an isotope ratio-based approach. Nat. Protoc. 3, 1299–1311. doi: 10.1038/nprot.2008.107
Chambers, M. C., Maclean, B., Burke, R., Amodei, D., Ruderman, D. L., Neumann, S., et al. (2012). A cross-platform toolkit for mass spectrometry and proteomics. Nat. Biotechnol. 30, 918–920. doi: 10.1038/nbt.2377
Chlorinated Solvents Contamination (2016). Available at: toxics.usgs.gov/investigations/chlorinated_solvents.html (Accessed April 14, 2016).
Fiehn, O., and Weckwerth, W. (2003). Deciphering metabolic networks. Eur. J. Biochem. 270, 579–588. doi: 10.1046/j.1432-1033.2003.03427.x
Fletcher, K. E., Costanza, J., Cruz-Garcia, C., Ramaswamy, N. S., Pennell, K. D., and Löffler, F. E. (2011). Effects of elevated temperature on Dehalococcoides Dechlorination performance and DNA and RNA biomarker abundance. Environ. Sci. Technol. 45, 712–718. doi: 10.1021/es1023477
Gonzalez-Covarrubias, V., Martínez-Martínez, E., and del Bosque-Plata, L. (2022). The potential of metabolomics in biomedical applications. Meta 12:194. doi: 10.3390/metabo12020194
Hatt, J. K., and Löffler, F. E. (2012). Quantitative real-time PCR (qPCR) detection chemistries affect enumeration of the Dehalococcoides 16S rRNA gene in groundwater. J. Microbiol. Methods 88, 263–270. doi: 10.1016/j.mimet.2011.12.005
Hatt, J. K., Ritalahti, K. M., Ogles, D. M., Lebrón, C. A., and Löffler, F. E. (2013). Design and application of an internal amplification control to improve Dehalococcoides mccartyi 16S rRNA gene enumeration by qPCR. Environ. Sci. Technol. 47, 11131–11138. doi: 10.1021/es4019817
Haug, K., Cochrane, K., Nainala, V. C., Williams, M., Chang, J., Jayaseelan, K. V., et al. (2019). MetaboLights: a resource evolving in response to the needs of its scientific community. Nucleic Acids Res. 48, D440–D444. doi: 10.1093/nar/gkz1019
He, J., Ritalahti, K. M., Yang, K.-L., Koenigsberg, S. S., and Löffler, F. E. (2003). Detoxification of vinyl chloride to ethene coupled to growth of an anaerobic bacterium. Nature 424, 62–65. doi: 10.1038/nature01717
Huang, B., Lei, C., Wei, C., and Zeng, G. (2014). Chlorinated volatile organic compounds (cl-VOCs) in environment - sources, potential human health impacts, and current remediation technologies. Environ. Int. 71, 118–138. doi: 10.1016/j.envint.2014.06.013
Johnson, D. R., Lee, P. K. H., Holmes, V. F., Fortin, A. C., and Alvarez-Cohen, L. (2005). Transcriptional expression of the tceA gene in a Dehalococcoides-containing microbial enrichment. Appl. Environ. Microbiol. 71, 7145–7151. doi: 10.1128/AEM.71.11.7145-7151.2005
Kanitkar, Y. H., Stedtfeld, R. D., Hatzinger, P. B., Hashsham, S. A., and Cupples, A. M. (2017). Development and application of a rapid, user-friendly, and inexpensive method to detect Dehalococcoides sp. reductive dehalogenase genes from groundwater. Appl. Microbiol. Biotechnol. 101, 4827–4835. doi: 10.1007/s00253-017-8203-y
Krajmalnik-Brown, R., Hölscher, T., Thomson, I. N., Saunders, F. M., Ritalahti, K. M., and Löffler, F. E. (2004). Genetic identification of a putative vinyl chloride reductase in Dehalococcoides sp. strain BAV1. Appl. Environ. Microbiol. 70, 6347–6351. doi: 10.1128/AEM.70.10.6347-6351.2004
Lee, P. K. H., Johnson, D. R., Holmes, V. F., He, J., Alvarez-Cohen, L., et al. (2006). Reductive dehalogenase gene expression as a biomarker for physiological activity of Dehalococcoides spp. Appl. Environ. Microbiol. 72, 6161–6168. doi: 10.1128/AEM.01070-06
Lihl, C., Douglas, L. M., Franke, S., Pérez-de-Mora, A., Meyer, A. H., Daubmeier, M., et al. (2019). Mechanistic dichotomy in bacterial Trichloroethene Dechlorination revealed by carbon and chlorine isotope effects. Environ. Sci. Technol. 53, 4245–4254. doi: 10.1021/acs.est.8b06643
Löffler, F. E., and Edwards, E. A. (2006). Harnessing microbial activities for environmental cleanup. Curr. Opin. Biotechnol. 17, 274–284. doi: 10.1016/j.copbio.2006.05.001
Löffler, F. E., Ritalahti, K. M., and Zinder, S. H. (2013). “Dehalococcoides and reductive dechlorination of chlorinated solvents” in Bioaugmentation for Groundwater Remediation. eds. H. F. Stroo, A. Leeson, and C. H. Ward (New York, NY: Springer), 39–88.
Löffler, F. E., Sanford, R. A., and Ritalahti, K. M. (2005). Enrichment, cultivation, and detection of reductively dechlorinating bacteria. Methods Enzymol. 397, 77–111. doi: 10.1016/S0076-6879(05)97005-5
Löffler, F. E., Yan, J., Ritalahti, K. M., Adrian, L., Edwards, E. A., Konstantinidis, K. T., et al. (2013). Dehalococcoides mccartyi gen. Nov., sp. nov., obligately organohalide-respiring anaerobic bacteria relevant to halogen cycling and bioremediation, belong to a novel bacterial class, Dehalococcoidia classis nov., order Dehalococcoidales Ord. Nov. and family Dehalococcoidaceae fam. Nov., within the phylum Chloroflexi. Int. J. Syst. Evol. 63, 625–635. doi: 10.1099/ijs.0.034926-0
Lu, W., Clasquin, M. F., Melamud, E., Amador-Noguez, D., Caudy, A. A., and Rabinowitz, J. D. (2010). Metabolomic analysis via reversed-phase ion-pairing liquid chromatography coupled to a stand alone Orbitrap mass spectrometer. Anal. Chem. 82, 3212–3221. doi: 10.1021/ac902837x
Lu, X., Wilson, J. T., and Kampbell, D. H. (2006). Relationship between Dehalococcoides DNA in ground water and rates of reductive dechlorination at field scale. Water Res. 40, 3131–3140. doi: 10.1016/j.watres.2006.05.030
Matturro, B., and Rossetti, S. (2015). GeneCARD-FISH: detection of tceA and vcrA reductive dehalogenase genes in Dehalococcoides mccartyi by fluorescence in situ hybridization. J. Microbiol. Methods 110, 27–32. doi: 10.1016/j.mimet.2015.01.005
Maymó-Gatell, X., Chien, Y.-t., Gossett, J. M., and Zinder, S. H. (1997). Isolation of a bacterium that reductively dechlorinates tetrachloroethene to ethene. Science 276, 1568–1571. doi: 10.1126/science.276.5318.1568
Michalsen, M. M., Kara Murdoch, F., Löffler, F. E., Wilson, J., Hatzinger, P. B., Istok, J. D., et al. (2022). Quantitative proteomics and quantitative PCR as predictors of cis-1,2-Dichlorethene and vinyl chloride reductive Dechlorination rates in bioaugmented aquifer microcosms. ACS ES&T Eng. 2, 43–53. doi: 10.1021/acsestengg.1c00207
Moran, M. J., Zogorski, J. S., and Squillace, P. J. (2007). Chlorinated solvents in groundwater of the United States. Environ. Sci. Technol. 41, 74–81. doi: 10.1021/es061553y
Morgan, E. W., Perdew, G. H., and Patterson, A. D. (2022). Multi-omics strategies for investigating the microbiome in toxicology research. Toxicol. Sci. 187, 189–213. doi: 10.1093/toxsci/kfac029
Müller, J. A., Rosner, B. M., von Abendroth, G., Meshulam-Simon, G., McCarty, P. L., and Spormann, A. M. (2004). Molecular identification of the catabolic vinyl chloride reductase from Dehalococcoides sp. strain VS and its environmental distribution. Appl. Environ. Microbiol. 70, 4880–4888. doi: 10.1128/AEM.70.8.4880-4888.2004
Pang, Z., Chong, J., Zhou, G., de Lima Morais, D. A., Chang, L., Barrette, M., et al. (2021). MetaboAnalyst 5.0: narrowing the gap between raw spectra and functional insights. Nucleic Acids Res. 49, W388–W396. doi: 10.1093/nar/gkab382
Patti, G. J., Yanes, O., and Siuzdak, G. (2012). Innovation: metabolomics: the apogee of the omics trilogy. Nat. Rev. Mol. Cell Biol. 13, 263–269. doi: 10.1038/nrm3314
Ritalahti, K. M., Amos, B. K., Sung, Y., Wu, Q., Koenigsberg, S. S., and Löffler, F. E. (2006). Quantitative PCR targeting 16S rRNA and reductive dehalogenase genes simultaneously monitors multiple Dehalococcoides strains. Appl. Environ. Microbiol. 72, 2765–2774. doi: 10.1128/AEM.72.4.2765-2774.2006
Silva, M. L. D., and Alvarez, P. J. (2008). Exploring the correlation between Halorespirer biomarker concentrations and TCE Dechlorination rates. J. Environ. Eng. 134, 895–901. doi: 10.1061/(ASCE)0733-9372(2008)134:11(895)
Solis, M. I. V., Abraham, P. E., Chourey, K., Swift, C. M., Löffler, F. E., and Hettich, R. L. (2019). Targeted detection of Dehalococcoides mccartyi microbial protein biomarkers as indicators of reductive dechlorination activity in contaminated groundwater. Sci. Rep. 9:10604. doi: 10.1038/s41598-019-46901-6
Viant, M. R., and Sommer, U. (2013). Mass spectrometry based environmental metabolomics: a primer and review. Metabolomics 9, 144–158. doi: 10.1007/s11306-012-0412-x
Wang, M., Carver, J. J., Phelan, V. V., Sanchez, L. M., Garg, N., Peng, Y., et al. (2016). Sharing and community curation of mass spectrometry data with global natural products social molecular networking. Nat. Biotechnol. 34, 828–837. doi: 10.1038/nbt.3597
Wei, S., Wei, Y., Gong, Y., Chen, Y., Cui, J., Li, L., et al. (2022). Metabolomics as a valid analytical technique in environmental exposure research: application and progress. Metabolomics 18:35. doi: 10.1007/s11306-022-01895-7
Wolin, E. A., Wolin, M. J., and Wolfe, R. S. (1963). Formation of methane by bacterial extracts. J. Biol. Chem. 238, 2882–2886. doi: 10.1016/S0021-9258(18)67912-8
Yan, J., Şimşir, B., Farmer, A. T., Bi, M., Yang, Y., Campagna, S. R., et al. (2015). The corrinoid cofactor of reductive dehalogenases affects dechlorination rates and extents in organohalide-respiring Dehalococcoides mccartyi. ISME J. 10, 1092–1101. doi: 10.1038/ismej.2015.197
Yan, J., Wang, J., Villalobos Solis, M. I., Jin, H., Chourey, K., Li, X., et al. (2021). Respiratory vinyl chloride reductive dechlorination to ethene in TceA-expressing Dehalococcoides mccartyi. Environ. Sci. Technol. 55, 4831–4841. doi: 10.1021/acs.est.0c07354
Yang, Y., Higgins, S. A., Yan, J., Şimşir, B., Chourey, K., Iyer, R., et al. (2017). Grape pomace compost harbors organohalide-respiring Dehalogenimonas species with novel reductive dehalogenase genes. ISME J. 11, 2767–2780. doi: 10.1038/ismej.2017.127
Keywords: metabolomics, consortium SDC-9™, bioremediation, reductive dechlorination, environmental monitoring, Dehalococcoidia, biomarkers
Citation: May AL, Xie Y, Kara Murdoch F, Michalsen MM, Löffler FE and Campagna SR (2022) Metabolome patterns identify active dechlorination in bioaugmentation consortium SDC-9™. Front. Microbiol. 13:981994. doi: 10.3389/fmicb.2022.981994
Edited by:
Andrea Hanson Rhoades, Colorado State University, United StatesReviewed by:
Chang Ding, Helmholtz Association of German Research Centres (HZ), GermanyBruna Matturro, National Research Council (CNR), Italy
Copyright © 2022 May, Xie, Kara Murdoch, Michalsen, Löffler and Campagna. This is an open-access article distributed under the terms of the Creative Commons Attribution License (CC BY). The use, distribution or reproduction in other forums is permitted, provided the original author(s) and the copyright owner(s) are credited and that the original publication in this journal is cited, in accordance with accepted academic practice. No use, distribution or reproduction is permitted which does not comply with these terms.
*Correspondence: Shawn R. Campagna, Y2FtcGFnbmFAdXRrLmVkdQ==; Frank E. Löffler, ZnJhbmsubG9lZmZsZXJAdXRrLmVkdQ==
†Present addresses: Yongchao Xie, Department of Chemistry and Biochemistry, University of California, Los Angeles, Los Angeles, CA, United States
Fadime Kara Murdoch, Battelle Memorial Institute, Biosciences Center, Columbus, OH, United States