- 1Department of Neurosurgery, The First Hospital of Jilin University, Changchun, China
- 2Department of Hepatobiliary Pancreatic Surgery, The First Hospital of Jilin University, Changchun, China
- 3Department of Spine Surgery, The First Hospital of Jilin University, Changchun, China
Background: The histone deacetylase (HDAC) family limited accessibility to chromatin containing tumor suppressor genes by removing acetyl groups, which was deemed a path for tumorigenesis. Considering glioma remained one of the most common brain cancers with a dichotomy prognosis and limited therapy responses, HDAC inhibitors were an area of intensive research. However, the expression profiles and prognostic value of the HDACs required more elucidation.
Methods: Multiple biomedical databases were incorporated, including ONCOMINE, GEPIA, TCGA, CGGA, GEO, TIMER, cBioPortal, and Metascape, to study expression profiles, prognostic value, immune infiltration, mutation status, and enrichment of HDACs in glioma. STRING and GeneMANIA databases were used to identify HDAC1-related molecules. LASSO regression, Cox regression, Kaplan-Meier plot, and receiver operating characteristic (ROC) analyses were performed for HDAC1-related signature construction and validation.
Results:HDAC1 was significantly overexpressed in glioma, while HDAC11 was downregulated in glioblastoma. Except for HDAC 6/9/10, the HDAC family expression was significantly associated with glioma grade. Most of the HDAC family also correlated with glioma genetic mutations. Higher HDAC1 expression level predicted more dismal overall survival (OS) (p < 0.0001) and disease-free survival (DFS) (p < 0.0001), but a higher level of HDAC11 held more favorable OS (p = 2.1e−14) and DFS (p = 4.8e−08). HDAC4 displayed the highest mutation ratio, at 2.6% of the family. The prognostic value of HDAC1 was validated with ROC achieving 0.70, 0.77, 0.75, and 0.80 as separability for 1-, 3-, 5-, and 10-years OS predictions in glioma, respectively. Moreover, HDAC1 expression positively correlated with neutrophil (r = 0.60, p = 2.88e-47) and CD4+ T cell infiltration (r = 0.52, p = 3.96e-35) in lower-grade glioma. The final HDAC1-related signature comprised of FKBP3, HDAC1 (Hazard Ratio:1.49, 95%Confidence Interval:1.20–1.86), PHF21A, RUNX1T1, and RBL1, and was verified by survival analysis (p < 0.0001) and ROC with 0.80, 0.84, 0.83, and 0.88 as separability for 1-, 3-, 5-, and 10-years OS predictions, respectively. The signature was enriched in chromatin binding.
Conclusion: HDAC family was of clinical significance for glioma. Most of the HDAC family significantly correlated with the glioma grade, IDH1 mutation, and 1p/19q codeletion. HDAC1 was both a prognostic and immune infiltration indicator and a central component of the HDAC1-related signature for precise prognosis prediction in glioma.
Introduction
Glioma, characterized by its dichotomy prognosis, is one of the most common primary brain tumors in adults (Schiff et al., 2019; Wen et al., 2020). Patients diagnosed with glioblastoma multiforme (GBM, WHO IV grade) typically hold a median survival time of merely 14 months, whereas most cases of low-grade glioma, like pilocytic astrocytoma (WHO I grade), attained clinical cure after surgical resection (Louis et al., 2016; Wen et al., 2020). The malignancy might come from a highly unstable genome and extensive epigenetic deregulation. Since histone modifications were one of the key mechanisms in epigenetics, investigations related to the imbalance between histone acetyltransferases (HATs) and histone deacetylases (HDACs) in glioma are emerging (Kunadis et al., 2021).
Histone acetylation by HATs relaxed chromatin structure and facilitated the transcriptional complex accessing the core histone (Ruijter et al., 2003). By manipulating acetyl groups, HATs and HDACs reached a balance in the regulation of histone structure under physical conditions (Eyüpoglu and Savaskan, 2016). However, a carcinogenic transformation would initiate once the equilibrium was disrupted (Minucci and Pelicci, 2006). One possible mechanism behind the transformation was mediated by the activation of oncogenic genes like c-Myc and the repression of the tumor suppressor gene when the HDACs took dominance (Bolden et al., 2006; Nguyen et al., 2020). Therefore, it was of necessity to explore the HDACs in the context of glioma based on their oncogenic properties. The HDAC family so far encompassed four classes, which were further categorized by their dependency on zinc, namely zinc-dependent class I (HDAC1, 2, 3, and 8), class IIa (HDAC4, 5, 7, and 9), class IIb (HDAC 6 and 10), and class IV (HDAC11), and zinc-independent class III (SIRT proteins) (Chen et al., 2020). This study would mainly discuss the HDAC family.
In this research, the HDAC family was systemically analyzed across varieties of databases to evaluate its clinical and prognostic value for glioma. It helped discern the differentially expressed HDAC family genes with significance in glioma compared to normal, as well as the general expression level of each HDAC family member in glioma. The potential ties of the HDAC family to glioma were assessed by detecting the associations between the HDAC family’s expression and glioma grade, and genetic mutations, and by conducting survival analysis on the HDAC family. Genetic alterations, interactive network, and functional enrichment annotations were additionally acquired for the HDAC family. The prognostic gene HDAC1 and the HDAC1-related genes were prepared for the final HDAC1-related signature construction. In conclusion, the HDAC family was of prognostic significance and clinical interest for glioma. It exposed the pivotal role of HDAC1 in glioma, as both an independent prognostic and immune infiltration biomarker and a central component of the HDAC1-related signature for precise prognosis prediction.
Materials and Methods
Oncomine
The HDAC family member expression profiles in cancers were analyzed in the ONCOMINE database (www.ONCOMINE.org), which enabled public access to resourceful genome-wide cancer microarray data that originated from various studies (Rhodes et al., 2004). Significance thresholds were set as a p-value less than 0.01, a fold-change over 2, and gene rank within the top 10%. The detailed information for each study included was listed in Table 1.
Gene Expression Profiling Interactive Analysis
The mRNA sequencing data of the HDAC family together with its corresponding clinical information of glioma patients were retrieved from GEPIA (http://gepia2.cancer-pku.cn/), a web-based interactive tool providing comprehensive and customizable analyses with The Cancer Genome Atlas (TCGA) and Genotype-Tissue Expression Project (GTEx) RNA sequencing data as resources (Tang et al., 2017). In this study, differential expression analysis comparing 681 gliomas (518 cases of lower-grade glioma, 163 cases of glioblastoma) with 207 normal samples was performed, and survival analysis with Kaplan-Meier (KM) plot and survival heatmap was also included.
TCGA, Chinese Glioma Genome Atlas, and Gene Expression Omnibus
The glioma cohort in the TCGA research program (https://www.cancer.gov/tcga), including 505 cases of lower-grade glioma (LGG) and 155 cases of GBM RNA sequencing counts data with clinical information, was acquired with the R package “TCGAbiolinks” (Colaprico et al., 2016). The dataset was mainly used as a developing cohort for the construction of the HDAC1-related signature. The TCGA developing cohort was then randomly split at a ratio of 3:7 using the R package “caret” for internal validation (Kuhn, 2008).
The glioma RNA-seq dataset “mRNAseq_693” recruited in the CGGA (http://www.cgga.org.cn/) contains 693 glioma samples. It was selected to conduct clinical correlation analysis by linking RNA-seq data with pathological grade and typical mutation statuses, such as IDH1 mutation and 1p/19q codeletion. Moreover, the “mRNAseq_693” dataset was employed as verification for previous results from the differential expression analysis and the survival analysis in GEPIA.
The microarray dataset GSE16011 from the Gene Expression Omnibus (GEO) database was gathered as an external validation cohort for the HDAC1-related signature (Gravendeel et al., 2009). It consisted of 284 samples, including 117 LGG cases, 156 GBM cases, and normal controls. Patients with clear mutation records were kept.
cBioPortal
The mutation profiles of each HDAC family member were further analyzed in glioma with help from the cBioPortal for Cancer Genomics (http://cbioportal.org), which was an intuitive web tool for exploring analysis and visualization on cancer genomic data collected from several platforms (Gao et al., 2013). The detailed mutation statuses of the HDAC family in glioma pathological subtypes were also shown.
Search Tool for the Retrieval of Interacting Genes
STRING (https://string-db.org) pictured both physical and functional protein-protein interaction (PPI) networks based on current knowledge and prediction via systemic co-expression analysis and text-mining of literature (Szklarczyk et al., 2017). The PPI network analysis was used to identify genes associated with the HDAC family members and HDAC1. The HDAC family PPI network was visualized by the Cytoscape app (version 3.7.2).
Metascape
Metascape (http://metascape.org) served as a web-based portal mainly for gene annotation, interactome analysis, and functional enrichment analysis (Zhou et al., 2019). The HDAC family and the HDAC1-related gene signature were uploaded to query for the functional interpretation of those genes in fixed combination, thereby enlightening future investigation.
Tumor Immune Estimation Resource
TIMER database (http://timer.cistrome.org) was introduced to measure immune infiltration in the tumor microenvironment and attain a better comprehension of tumor-immune interactions (Li et al., 2020). Considering feasibility, “immune privilege” in the central nervous system (CNS), and loading capacity for each immune infiltration estimation analysis, only a fraction of typical infiltrated immune cells in glioma, including neutrophils, macrophages, myeloid-derived suppressive cells (MDSCs), CD4+ and CD8+ T cells, and regulatory T cells (Tregs), was filtered out to check for potential links with HDAC1 expression.
GeneMANIA
Except for STRING, GeneMANIA (http://www.genemania.org) was also used for identifying possible HDAC1-related genes as candidate genes for further HDAC1-related gene signature construction (Franz et al., 2018). The network depicting genes functionally close to HDAC1 was illustrated by recognizing patterns of gene co-annotation in the Gene Ontology or by using enrichment analysis.
Prognostic HDAC1-Related Gene Signature Development and Validation
The time-dependent receiver operating characteristic (ROC) analysis was conducted to test the predictive value for HDAC1, HDAC2, and HDAC11. Further, the HDAC1-related genes derived from GeneMANIA and STRING were regarded as predictive candidates for the HDAC1-related signature development. LASSO regression, univariate, and multivariate Cox regression analyses were consecutively applied to calculate the prognostic risk. Only genes with prognostic value and significance when fitting into the signature would be selected. The coefficients in the calculated regression model for each included gene were utilized to calculate the risk score,
Statistical Analysis
The statistical analyses and graphs associated with HDAC1-related gene signature development and validation were achieved in R 3.6.2 (R Core Team, 2019) and RStudio (version 1.1.463). The Least Absolute Shrinkage and Selection Operator (LASSO) regression analysis, Cox regression analysis, and Kaplan-Meier survival analysis along with proportional hazards (PH) test were performed with the R package “survminer” and the R package “survival.” Wilcoxon test and Kruskal Wallis test were used for statistical comparisons. In this study, a p-value less than 0.05 was regarded as statistically significant, and the p-values were adjusted with the “BH” method if involved with multiple hypothesis testing.
Results
Differential Expression Analysis of HDAC Family Genes in Glioma
The transcriptional profiles of the HDAC family in brain and CNS cancers were investigated in the ONCOMINE database (Figure 1). It revealed that HDAC1 and HDAC6 were significantly over-expressed and within the top 5% gene rank in glioma compared to the normal group, which was evidenced by three and two studies, respectively. In contrast, under-expressed HDAC4, HDAC5, and HDAC11 ranking within the top 1% gene rank were observed in glioma. It showed that two studies supported significantly overexpressed HDAC2 in CNS cancer, but an equal number of studies concluded otherwise. The evidence with differential significance and top gene ranking for the rest of the HDAC family members remained scarce. The detailed study information regarding the HDAC family in ONCOMINE was summarized in Table 1.
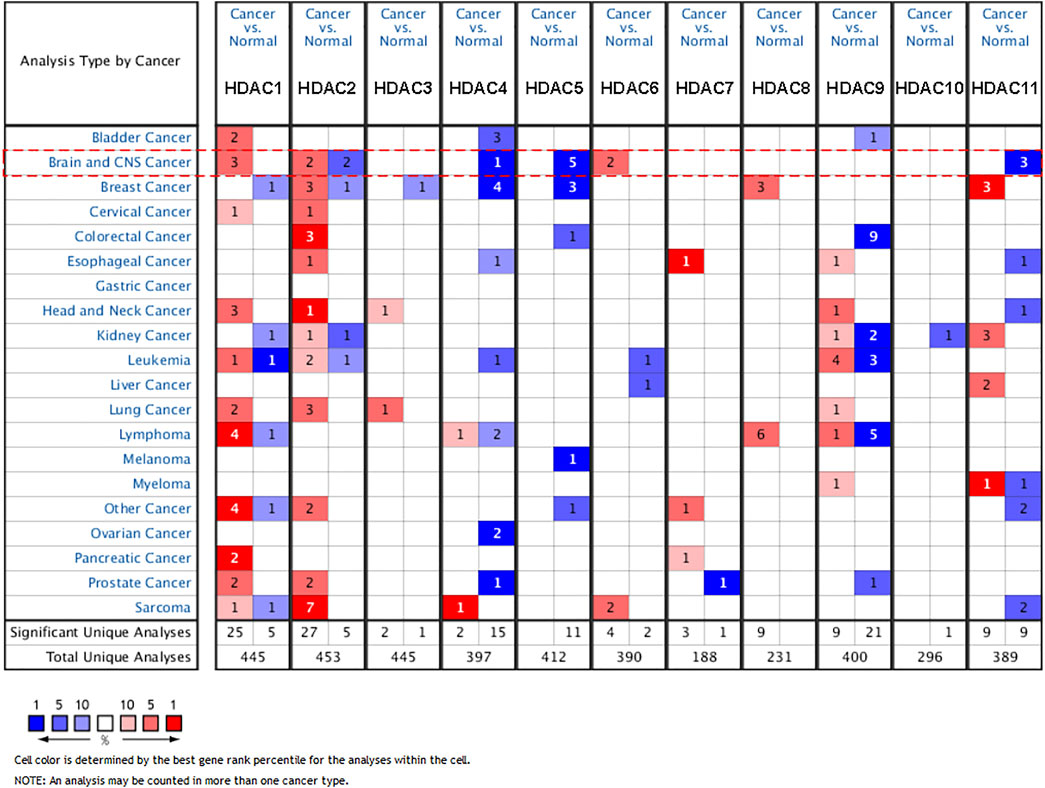
FIGURE 1. Expression microarray of HDAC family across varieties of cancers and corresponding control groups in ONCOMINE. The number in cells indicated the significant datasets showing the differentially expressed genes of the HDAC family. The red color in the cell was for upregulation, and the blue color was for downregulation. The student’s t-test was used to compare the different transcriptional values. The threshold was set as p-value < 0.01, fold-change = 2, gene rank < 10%.
HDAC1 mRNA over-expression in anaplastic oligoastrocytoma (n = 4) reached a fold change (FC) of 2.56 compared with the normal tissues (n = 6) (p = 4.41E-04) (French et al., 2005). In GBM samples (n = 542) from the TCGA cohort, HDAC1 showed 3.13-fold increase of expression (p = 3.08E-08). Over-expressed HDAC6 showed FCs of 3.22 and 2.61 for GBM (n = 81) (p = 8.88E-13) and anaplastic astrocytoma (n = 19) (p = 2.34E-06) groups, respectively (Sun et al., 2006).
Moreover, it was detected that the HDAC4 expression in GBM (n = 80) was 2.15-fold lower than the control group (n = 4) (p = 1.70E-13) (Murat et al., 2008). The comparisons of HDAC5 expression between anaplastic oligoastrocytoma (n = 6), anaplastic oligodendroglioma (n = 3), GBM (n = 27), and normal (n = 4) exposed a 2.10-fold (p = 4.64E-05), 2.44-fold (p = 0.003), 2.38-fold (p = 1.46E-07) decrease, respectively (Bredel et al., 2005). HDAC5 mRNA level in GBM (n = 81) was down-regulated with a FC of 2.22 in Sun’s 2006 study (p = 6.80E-20), and a fold change of 2.74 in the GBM cohort (n = 542) from TCGA (p = 3.08E-08) (Sun et al., 2006). HDAC11 displayed 4.20-fold downregulation in anaplastic oligoastrocytoma (n = 4) (p = 1.09E-05), and 3.93-fold downregulation in anaplastic oligodendroglioma (n = 23) in the French 2005 study (p = 7.32E-06) (French et al., 2005). Similarly, HDAC11 was found to transcriptionally decrease with a FC of 3.54 in GBM (n = 81) (p = 3.14E-14) (Sun et al., 2006).
The studies focusing on HDAC2 were of interest in ONCOMINE. One medulloblastoma study was filtered out. However, the rest of the three studies led to conflicting conclusions. As oligodendroglioma (n = 3) was compared with normal (n = 7) in Shai’s 2003 study, HDAC2 was upregulated and showed a fold change of 3.24 (p = 6.99E-04). The other two studies found that HDAC2 significantly under-expressed in anaplastic oligoastrocytoma (n = 6) with a fold change of 4.40 (p = 5.95E-04), and in GBM (n = 22) with a fold change of 3.68 (p = 2.70E-10).
To fully investigate the HDAC family expression in glioma, the GEPIA database, which incorporated the GTEx and the TCGA data, was explored for verification. In the differentially expressed gene analysis, each HDAC family member was studied in both LGG (n = 518) and GBM (n = 163) subsets with thresholds of |Log2FC| over 1 and q-value less than 0.01. Of the entire HDAC family, only HDAC1, HDAC2, and HDAC11 were tested to have more than a 2-fold alteration in expression with significance compared to normal tissue (Figure 2). HDAC1 and HDAC2 mRNA level was upregulated in both LGG and GBM, which was over 2-fold higher than the normal, whereas HDAC11 expression level was more than 2-fold lower in the GBM group only.
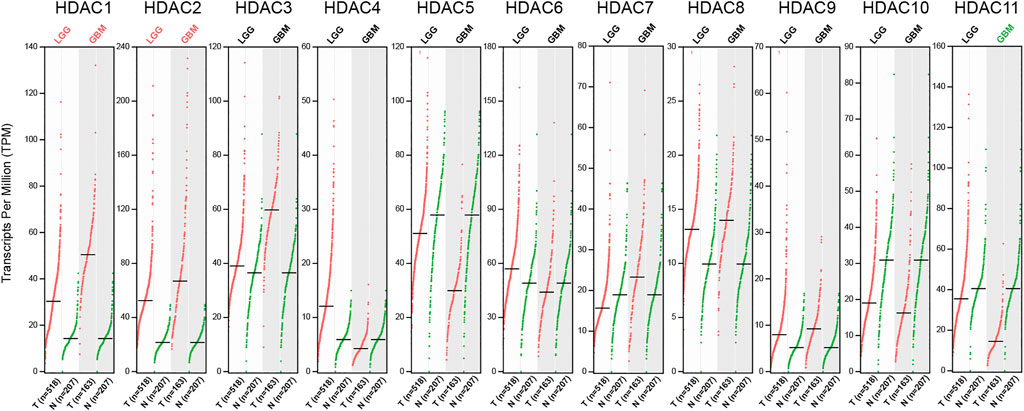
FIGURE 2. RNA-seq data of HDAC family in LGG and GBM accessed from GEPIA. The Scatter plots showed expression comparisons of the HDAC family between the tumor and the normal groups. The statistically significant comparison with |Log2FC| over 1 was marked either in red (upregulation) or green (downregulation) (q < 0.01).
Links Between HDAC Family and Glioma Grade, and Genetic Mutations
The relationship between the HDAC family and clinical features was studied. It was of priority to check whether the HDAC family’s expression altered with glioma pathological grade using the glioma cohort in CGGA. Except for HDAC6 (p = 0.367), HDAC9 (p = 0.870), and HDAC10 (p = 0.715), the majority of HDAC family expression levels correlated significantly with glioma grade (Figure 3). Specifically, the mRNA level of HDAC1 (p = 1.25e−13), HDAC3 (p = 2.51e−09), HDAC7 (p = 1.43e−19), and HDAC8 (p = 3.48e−10) showed an increasing trend with glioma grade progressing (Figures 3A,C). On the contrary, HDAC11 (p = 3.74e−12) expression was negatively associated with glioma grade (Figure 3D). HDAC2 (p = 0.0025) exhibited the highest expression level in the WHO III group but the lowest one in the WHO II group (Figure 3A). It also revealed that HDAC4 (p = 4.79e−18) and HDAC5 (p = 2.48e−12) expressed the most in the WHO III group but the least in the WHO IV group (Figure 3B).
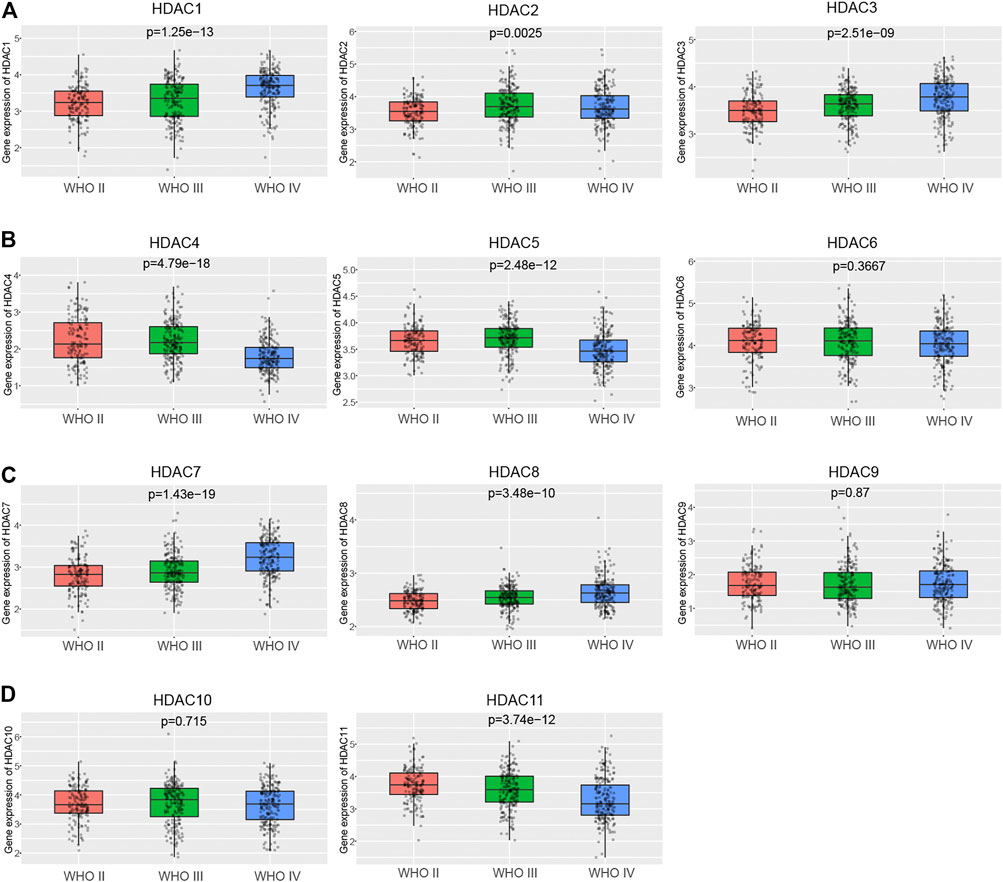
FIGURE 3. Links between HDAC family and glioma grade in CGGA. (A–D) The box plots depicted that the expression of the HDAC family changed with glioma grade.
Since the prognosis of glioma mainly depends on the pathological grade and genetic mutations, among which isocitrate dehydrogenase 1 (IDH1) and 1p/19q status represented primary concern, further investigation was conducted in an attempt to research relations between HDAC family and IDH1 and 1p/19q based on the CGGA glioma cohort. The HDAC family, other than HDAC6 (p = 0.187), HDAC9 (p = 0.0526), and HDAC10 (p = 0.91), are differentially expressed in the IDH1 mutant and IDH1 wildtype group with significance (Figure 4). As shown in the figure, HDAC1 (p = 5.5e−16), HDAC3 (p = 3.3e−05), HDAC7 (p = 1.21e−28), and HDAC8 (p = 0.0098) expression levels were significantly higher in the IDH1 wildtype than the mutant (Figures 4A–C). However, HDAC2 (p = 0.0098), HDAC4 (p = 8.80e−22), HDAC5 (p = 1.95e−15), and HDAC11 (p = 9.53e−05) significantly expressed more transcripts in the IDH1 mutant group compared to the wildtype (Figures 4A–C).
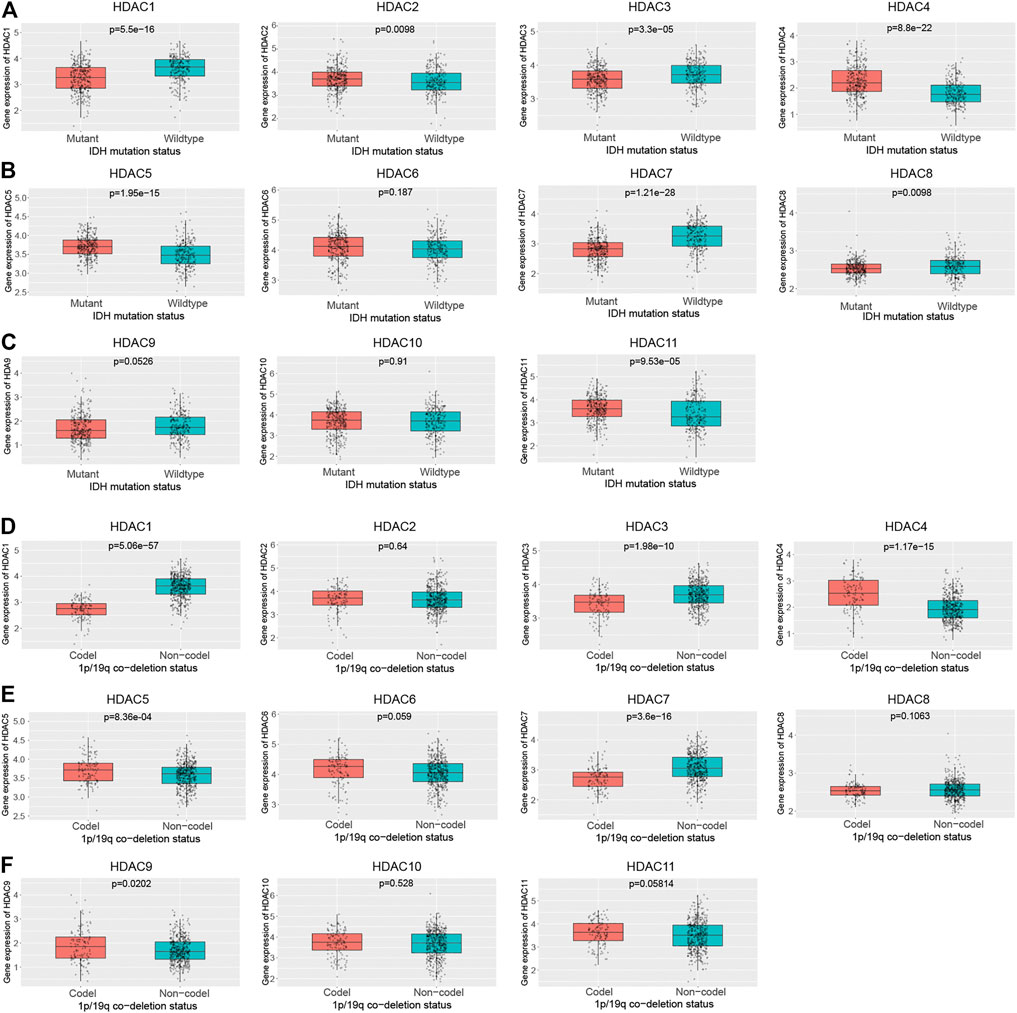
FIGURE 4. Correlation between HDAC family and glioma genetic mutation in CCGA. (A–F) The box plots showed that the expression of the HDAC family varied according to the glioma mutations.
The HDAC family members were also characterized by 1p/19q mutation (Figure 4). It turned out to have the same expression levels regarding the 1p/19q co-deletion statuses in HDAC2 (p = 0.64), HDAC6 (p = 0.059), HDAC8 (p = 0.106), and HDAC10 (p = 0.528), and HDAC11 (p = 0.0581) (Figures 4D–F). HDAC1 (p = 5.06e−57), HDAC3 (p = 1.98e−10), and HDAC7 (p = 3.6e−16) showed higher expression levels in the 1p/19q non-codeletion group in contrast to the codeletion group (Figures 4D,E). While HDAC4 (p = 1.17e−15), HDAC5 (p = 8.36e-04), and HDAC9 (p = 0.0202) showed higher expression levels in the 1p/19q codeletion group (Figures 4D–F).
Prognostic Characteristics of HDAC Family Genes in Glioma
Apart from the links with glioma grade and genetic mutations, survival analysis based on GEPIA (n = 676) and CGGA (n = 404) data enabled the prognostic evaluation for the clinical value of the HDAC family. The survival analysis mainly focused on overall survival (OS) and disease-free survival (DFS).
The OS analysis based on GEPIA revealed that the glioma patients with high expression levels of HDAC1 (HR:3.9, p < 0.0001), HDAC2 (HR:1.3, p = 0.024), HDAC3 (HR:4.4, p < 0.0001), and HDAC7 (HR:3.3, p < 0.0001) would face with more risks compared to the ones with low expression of these genes (Figures 5A,B). However, the patients would reap OS benefits if they expressed high levels of HDAC4 (HR:0.23, p < 0.0001), HDAC5 (HR:0.31, p < 0.0001), HDAC6 (HR:0.7, p = 0.0063), and HDAC11 (HR:0.37, p = 2.1e−14) (Figures 5A–C). The transcriptional levels of HDAC8 (p = 0.55), HDAC9 (p = 0.084), and HDAC10 (p = 0.061) imposed less influence on OS than the other HDAC family members (Figures 5B,C). Further research was performed to detect the impact that the HDAC family exerted on the OS of patients with LGG and GBM, in which HDAC1, HDAC3, and HDAC7 remained the top three risk genes, whereas HDAC4 was a remarkably protective gene (Figure 5D).
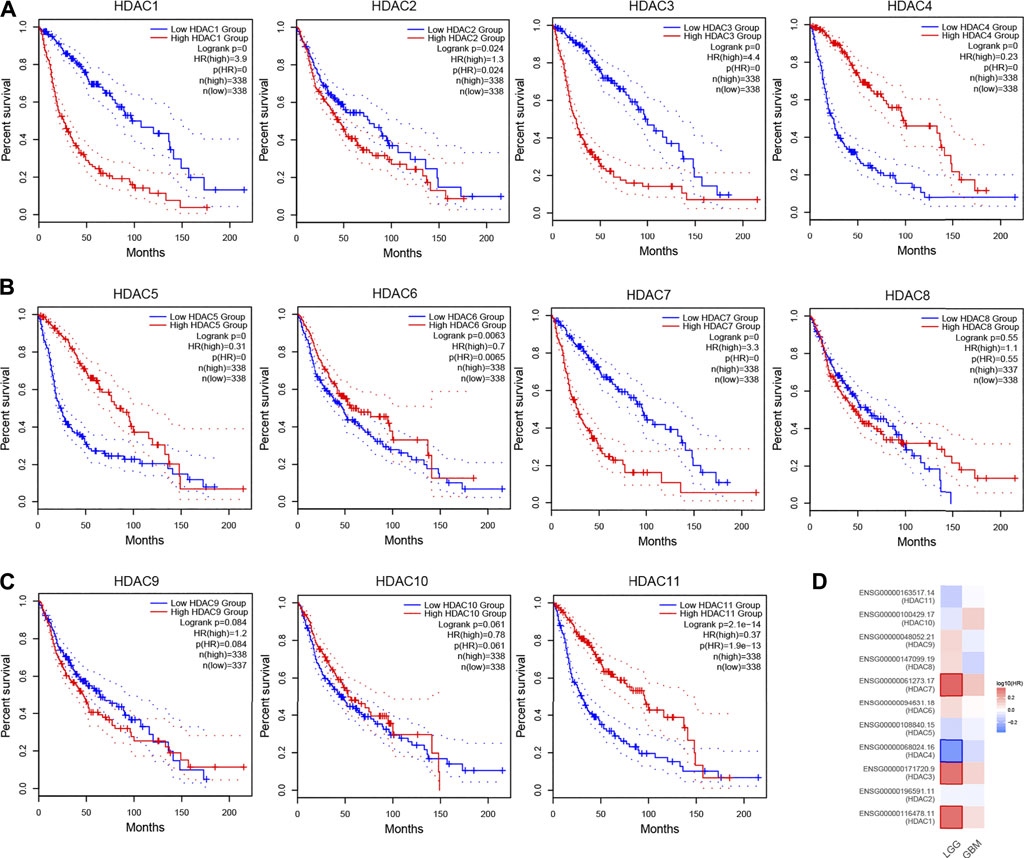
FIGURE 5. Prognostic evaluation (OS) of HDAC family in glioma based on GEPIA. (A–C) The KM survival curves showed distinct survival possibility predicted by varying expression of HDAC family. (D) The heatmap was used for the link between the HDAC family members’ expression and the OS of LGG and GBM patients.
The survival analysis was also applied in the CGGA cohort to validate the prognostic value of the HDAC family. Only HDAC1 (p < 0.0001), HDAC3 (p = 0.012), HDAC7 (p < 0.0001), and HDAC8 (p = 0.0056) were tested with significance for a favorable prognosis if modestly expressed (Figures 6A,B). In addition, overexpressed HDAC4 (p < 0.0001), HDAC5 (p < 0.0001), HDAC6 (p = 0.0012), and HDAC11 (p < 0.0001) enabled the patients to survive longer (Figures 6A–C). The other HDACs showed neither survival benefits nor risks.
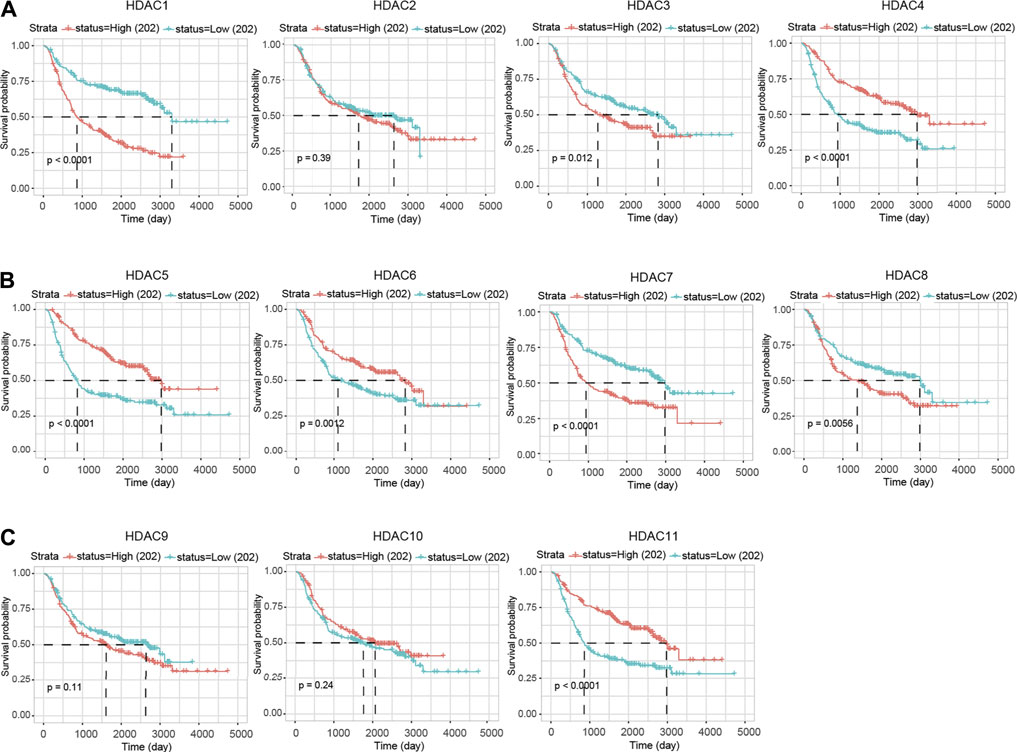
FIGURE 6. Prognostic feature (OS) of HDAC family in glioma based on CGGA. (A–C) The KM survival analysis was performed for verification regarding the prediction efficiency of the HDAC family.
The DFS-oriented study using GEPIA data showed that more transcripts of HDAC1 (HR:3.0, p < 0.0001), HDAC3 (HR:2.7, p = 2.1e−15), HDAC7 (HR:2.1, p = 3e−09), and HDAC9 (HR:1.5, p = 0.0026) accompanied with less DFS probabilities in glioma patients (Figures 7A–C). However, extended DFS would be observed in patients with high expression of HDAC4 (HR:0.35, p = 1.1e−16), HDAC5 (HR:0.43, p = 9.4e−12), HDAC6 (HR:0.73, p = 0.014), HDAC10 (HR:0.72, p = 0.0092), and HDAC11 (HR:0.5, p = 4.8e−08). The rest of the HDAC family displayed no risks or benefits for DFS in glioma patients (Figures 7A–C). Similarly, HDAC1, HDAC3, and HDAC7 accounted for the major risk factors for LGG, but HDAC4 and HDAC5 enhanced DFS probabilities (Figure 7D).
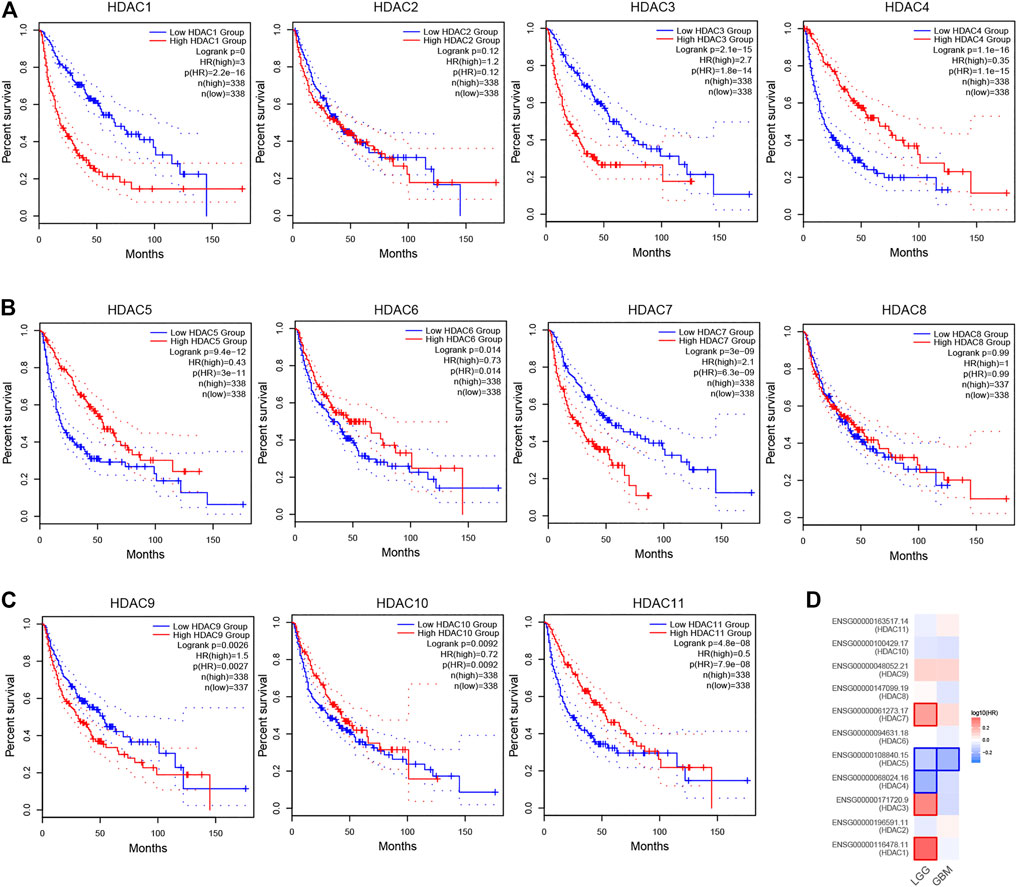
FIGURE 7. Prognostic value (DFS) of HDAC family in glioma based on GEPIA. (A–C) The KM survival curves revealed contrasting survival possibility predicted by varying expression of HDAC family. (D) The heatmap was used for detecting the relations between the HDAC family members’ expression and the DFS of LGG and GBM patients.
Genetic Mutations, Interactive Network, and Functional Enrichment Analysis of HDAC Family
The genetic mutations of the HDAC family were analyzed with the TCGA data available at the cBioPortal database. Each of the HDAC family members harbored genetic mutations, in which HDAC4 was the most prominent with a mutation ratio of 2.6% (Figure 8A). It was followed by the mutation ratio of HDAC9, HDAC10, and HDAC6 being 1.5, 1.5, and 1.3%, respectively (Figure 8A). Interestingly, the mutation ratio of HDAC1 and HDAC11 were the same at 0.5% (Figure 8A). In terms of mutation statuses in the detailed glioma subtypes, astrocytoma and GBM shared a similar distribution of mutation patterns. However, oligoastrocytoma only showed three kinds of genetic alterations, “mutation,” “deep deletion,” and “multiple alterations,” and oligodendroglioma harbored one more type compared to oligoastrocytoma, “amplification” (Figure 8B).
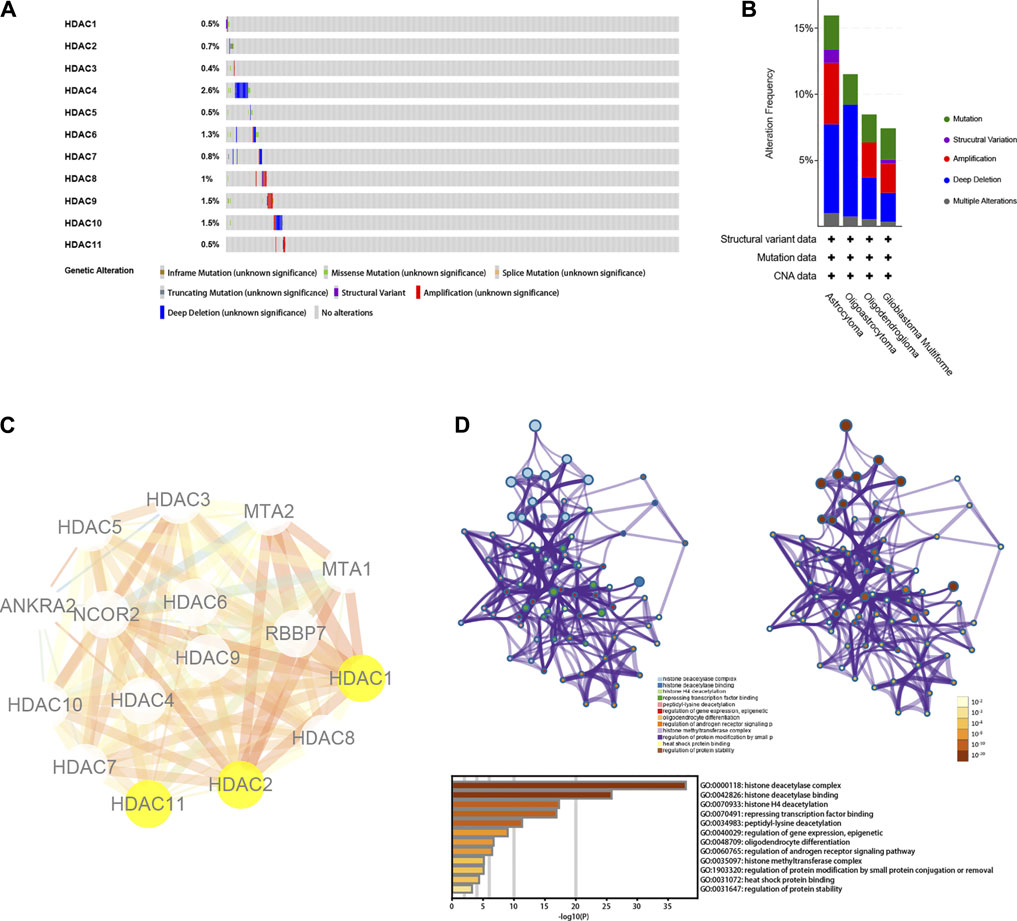
FIGURE 8. Genetic mutation, interactive network, and functional enrichment of HDAC family. (A,B) Summary for genetic alterations of HDAC family in glioma. (C) Protein-protein interactive network of HDAC family by STRING. The size of the edges in the network was set to change according to the co-expression value among the nodes. The color of the edges in the network altered with the combined scores from the low to the high, which were all the evidence scores (including transferred scores). (D) Functional enrichment plot and annotation by Metascape.
Further, interaction analysis was performed in the HDAC family using the STRING web tool. Beyond the HDAC family were MTA1, MTA2, RBBP7, NCOR2, and ANKRA2 that were incorporated in the PPI network (Figure 8C). The functional enrichment annotated the interactive network mainly as “histone deacetylation” (Figure 8D). Nevertheless, enrichment results, such as “oligodendrocyte differentiation,” “histone methyltransferase,” and “heat shock protein binding,” were also noticeable.
Associate Between Prognostic HDAC1 and Immune Infiltration in Glioma
Based on the results summarized in Table 2, HDAC1, HDAC2, and HDAC11 were selected as the predictive genes with significant clinical value considering their significant and consistent performance in the previous analyses. ROC analysis was applied to assess the discrimination efficiency of the three genes in the TCGA glioma cohort. The separability of the HDAC1 turned out to be valid, reaching 0.70, 0.77, 0.75, and 0.80 for 1-, 3,- 5-, and 10-years OS predictions in glioma, respectively (Figure 9A). However, the area under the ROC curve (AUC) of the HDAC2 and the HDAC11 was calculated with no value exceeding 0.5 throughout the time points in the study (Figures 9B,C).
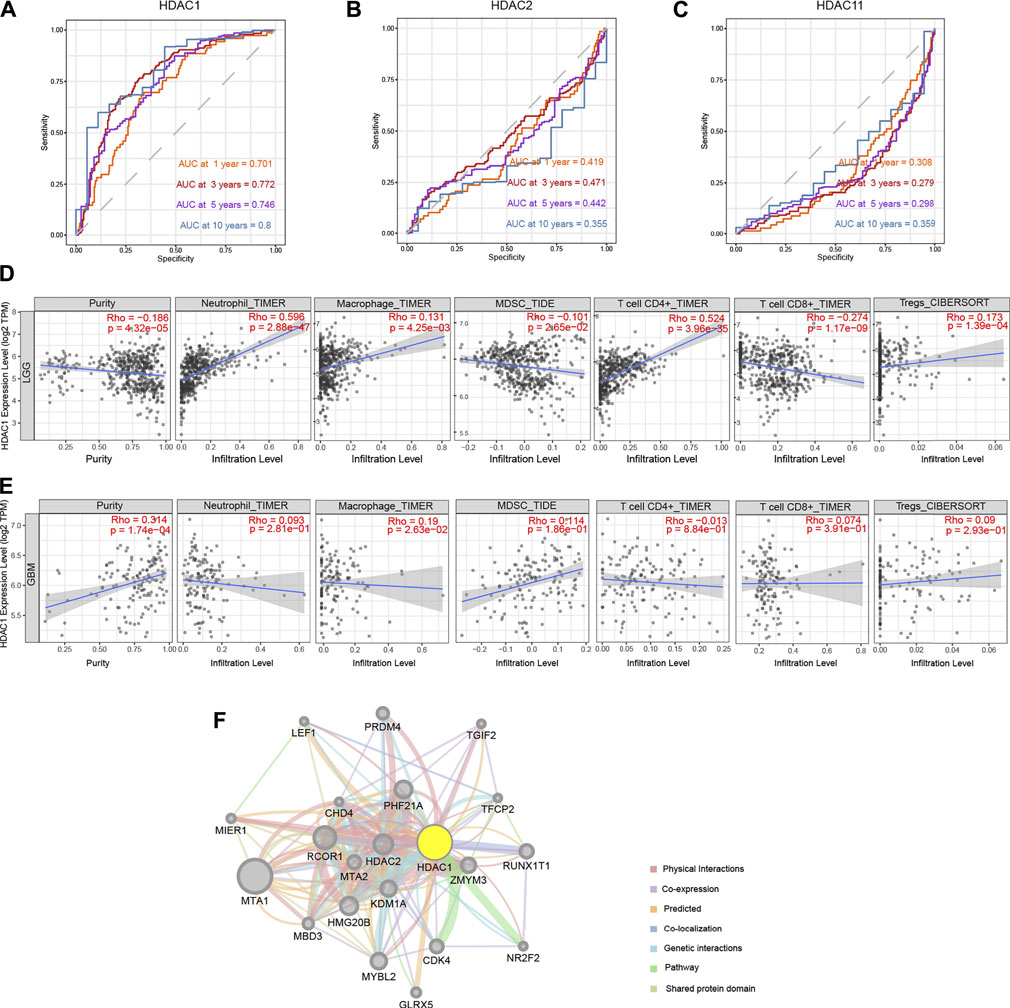
FIGURE 9. Correlation between prognostic gene HDAC1 and immune infiltration, and related proteins. (A–C) Time-dependent ROC curve on HDAC1, HDAC2, and HDAC11 checking for OS prediction accuracy at 1-, 3-, 5-, and 10-years time points. (D,E) Correlation between HDAC1 and neutrophil, macrophage, MDSC, CD4+ T cell, CD8+ T cell, and Treg infiltration in LGG and GBM. (F) Identification of HDAC1-related gene network by GeneMANIA.
Immune infiltration presumably involved with glioma progression and prognosis. Since HDAC1 was filtered out to be the prognostic gene for glioma, the association between the immune infiltration and the HDAC1 expression was analyzed by the TIMER database. Considering the limited immune cells in the CNS system due to the BBB, neutrophils, macrophages, MDSCs, CD4+ T cells, CD8+ T cells, and Tregs were regarded as the major infiltrated immune cell types in glioma for the evaluation. The correlation test was categorized by LGG and GBM.
It revealed that each result of the immune correlation analysis was of significance (p < 0.0 1) but a relatively strong relation (r > 0.5) was limited either in LGG or GBM (Figures 9D,E). Intriguingly, HDAC1 mRNA level was relatively closely and positively tied to neutrophil infiltration in the LGG group (r = 0.60, p = 2.88e-47) (Figure 9D). The HDAC1 expression also positively associated with CD4+ T cell infiltration (r = 0.52, p = 3.96e-35) (Figure 9D) in the LGG group. The negative relation between CD8+ T cell infiltration and HDAC1 expression level was noticeable (r = -0.27, p = 1.17e-09) (Figure 9D). In the GBM group, the strongest relationship was between macrophage and HDAC1 expression (r = 0.19, p = 2.81e-01) (Figure 9E). Additionally, HDAC1 was used to explore the potential HDAC1-related genes in the GeneMANIA (Figure 9F). The entire HDAC1-centered interactive network (Figure 9F) together with supplementary genes acquired from the STRING in the same way as the GeneMANIA, the candidate risk genes for HDAC1-related signature construction, were thus recruited. The HDAC1-related genes were listed in Supplementary Table S1.
Development and Validation of HDAC1-Related Gene Signature
Fifteen genes were screened out of the initial HDAC1-related genes through LASSO regression analysis (Supplementary Figure S1). Univariate and multivariate Cox regression analyses were then performed for the signature development (Supplementary Table S2). The HDAC1-related gene signature was ultimately constructed in the TCGA cohort and comprised of five promising prognostic genes: HDAC1 (HR:1.4938, 95%CI:1.1997–1.8600), RUNX1T1 (HR:0.7349, 95%CI:0.6483–0.8330), FKBP3 (HR:0.6382, 95%CI:0.4782–0.8517), RBL1 (HR:1.7148, 95%CI:1.3826–2.1268), and PHF21A (HR:0.4956, 95%CI:0.3874–0.6340) (Figure 10A). The signature model satisfied the proportional hazards (PH) assumption (Supplementary Figure S2). And it was also adjusted and tested with other prognostic factors, including gender, grade, IDH1 mutation, and 1p/19q codeletion, to be an independent prognostic indicator (Riskscore, HR:1.0935, 95%CI:1.0045–1.1904, p = 0.039) (Supplementary Figure S3). The signature risk score was calculated as follows: Riskscore = 0.4013×HDAC1-0.3081×RUNX1T1-0.4491×FKBP3+0.5393×RBL1-0.7020×PHF21A.
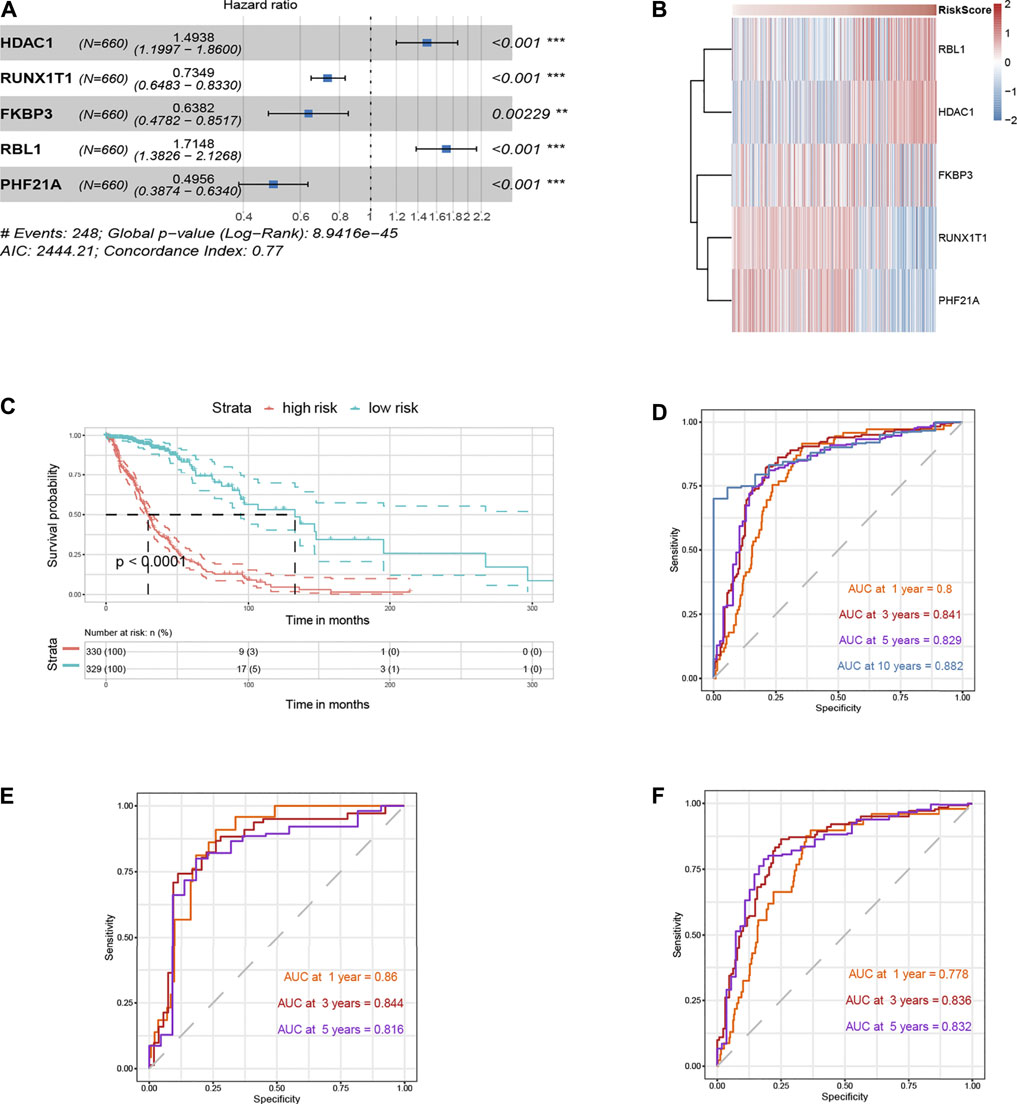
FIGURE 10. HDAC1-related gene signature construction and internal validation. (A) Forest plot for the HDAC1-related gene signature. (B) Heatmap for expression profiles of the signature components in the TCGA glioma cohort. (C) Survival curve for survival possibility test between high- and low-risk group according to the signature risk score in the TCGA cohort. (D) Time-dependent ROC curve for the separability test of the HDAC1-related signature at 1-, 3-, 5-, and 10-years time points (OS) using the TCGA developing cohort. (E) Time-dependent ROC curve for the HDAC1-related signature using the TCGA training set. (F) Time-dependent ROC curve for the HDAC1-related signature using the TCGA testing set.
The expression profiles of the HDAC1-related signature components were investigated in the TCGA glioma cohort (Figure 10B). HDAC1 and RBL1 were upregulated with the increasing risk score, while the expression levels of FKBP3, RUNX1T1, and PHF21A reversed (Figure 10B). To validate the prognostic efficiency, the signature was tested by survival analysis and ROC curve in the TCGA developing cohort. It showed that the high-risk group which scored high according to the equation held poorer survival probabilities than the low-risk (p < 0.0001) (Figure 10C). Additionally, the discrimination of the signature was measured by the AUC being 0.800, 0.841, 0.829, and 0.882 for 1-, 3-, 5- and 10-years OS, respectively (Figure 10D).
The signature was also validated internally and externally. As for internal validation, the TCGA developing cohort was randomly divided into two sets, one for training containing 30% of the cohort cases, and the other as the testing set. It classified the data with the 1-year OS AUC being 0.860, the 3-years OS AUC being 0.844, and the 5-years OS AUC being 0.816 in the training set (Figure 10E). Moreover, the 1-year OS AUC being 0.778, the 3-years OS AUC being 0.836, and the 5-years OS AUC being 0.832 were measured in the testing set (Figure 10F). In the GEO validation cohort used for external validation, the high-risk group consistently had a higher survival risk compared to the low one (p < 0.0001) (Figure 11A). And the discrimination of the signature was confirmed in the GEO cohort regarding being 0.695, 0.834, 0.831, and 0.826 for 1-, 3-, 5-, and 10-years OS, respectively (Figure 11B). The signature was annotated as “chromatin binding” by the functional enrichment analysis in Metascape (Figure 11C). The clinical baseline information of the TCGA developing cohort and the GEO validation cohort were listed in Supplementary Table S3.
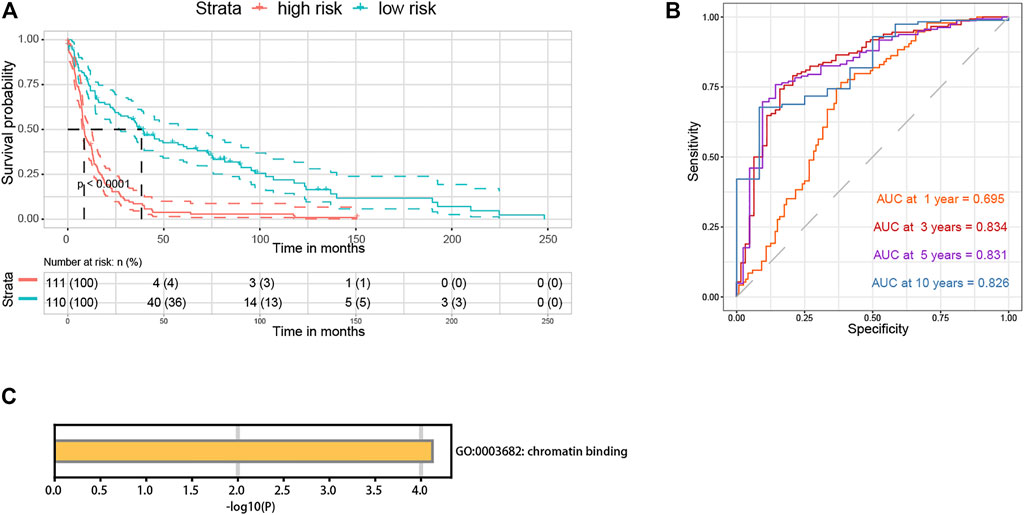
FIGURE 11. HDAC1-related gene signature external validation and enrichment analysis. (A) Survival curve for survival possibility test between high- and low-risk group according to the signature risk score in the GEO cohort. (B) Time-dependent ROC curve for the separability test of the HDAC1-related signature at 1-, 3-, 5-, and 10-years time points (OS) using the GEO validation cohort. (C) Functional enrichment analysis of the HDAC1-related signature.
Discussion
Differentially expressed HDAC family members were identified with significance in ONCOMINE, namely the upregulated HDAC1 and HDAC6, as well as the downregulated HDAC4, HDAC5, and HDAC11 in glioma (Figure 1). After ruling out the unrelated medulloblastoma study, the contradictory expression profiles in the three studies on HDAC2 resulted in a perplexing conclusion, of which one indicated overexpression while the other two opposed. However, the study size of the three studies was too limited to conclude firmly regarding HDAC2 expression level in glioma. The Lee 2006 study recruiting 22 cases of GBM which was larger than the size of the other two HDAC2 studies might suggest that HDAC2 was under-expressed in glioma (Table 1).
Further validation of the HDAC family differential expression was conducted in GEPIA. Only the comparison with a change over 2-fold and q-value less than 0.01 was marked in the exhibition (Figure 2). The HDAC1 and HDAC2 comparisons in glioma, and the HDAC11 comparison in GBM, were remarkable for their significantly contrasting expression. The remaining groups were neither of significance nor holding differential changes exceeding 2-fold. A previous study composed of 20 low-grade and 23 high-grade glioma patients concluded that class II and IV HDACs expressed less in GBM compared to low-grade glioma and normal tissue (Lucio-Eterovic et al., 2008; Williams et al., 2017). However, it seemed too bold and assertive to firmly conclude with such a limited sample size. Besides, the single-centered data might be insufficient and unpersuasive since the differential expression results from multiple sources in this study only agree with a few genes to be further analyzed. Considering the findings from ONCOMINE, GEPIA, and CGGA, HDAC1, HDAC2, and HDAC11 were thus verified for their differential expression in glioma (Figures 1, 2, 3; Table 2).
It was assumed that the differentially expressed HDAC family might contribute to the clinical and genetic features of glioma. The investigation on the potential links between the HDAC family and the pathological grade, and the genetic alterations of glioma, revealed that the expression levels of HDAC1, HDAC2, and HDAC11 significantly altered with the glioma grade (HDAC1, p = 1.25e−13; HDAC2, p = 0.0025; HDAC11, p = 3.74e−12) and the IDH1 mutation status (HDAC1, p = 5.5e−16; HDAC2, p = 0.0098; HDAC11, p = 9.53e−05) (Figures 3A,D; Figures 4A,C; Table 2). The transcriptional level of HDAC1 (p = 5.06e−57), but not HDAC2 (p = 0.64) and HDAC11 (p = 0.058), significantly varied with the 1p/19q mutation (Figures 4D,E).
Given that low pathological grade, IDH1 mutant, and 1p/19q codeletion were favorable prognostic factors, the fact that HDAC1 overexpressed in the WHO III and WHO IV group (Figure 3A), in the IDH1 wildtype group (Figure 4A), and the 1p/19q non-codeletion (Figure 4D) implied that HDAC1 tended to be a detrimental prognostic biomarker in glioma (Lapointe et al., 2018). It also suggested the role of HDAC11 as a protective factor concerning its opposite expression pattern relative to HDAC1 (Figures 3D, 4C,F). However, HDAC2 expressed the most in the WHO III group but the least in the WHO IV group, indicating a baffling part HDAC2 played in the glioma progression (Figure 3A). It was still reasonable that HDAC2 was involved with the IDH1 mutation (Figure 4A).
Consistent with the earlier results and assumption, the OS-oriented survival analysis in both the GEPIA (n = 676) and the CGGA (n = 404) showed that HDAC1 overexpression (GEPIA, HR:3.9, p < 0.0001; CGGA, p < 0.0001) brought risks to glioma patients (Figures 5A, 6A), while HDAC11 served as a favorable prognostic indicator (GEPIA, HR:0.37, p = 2.1e−14; CGGA, p < 0.0001) (Figures 5C, 6C). Although HDAC2 (HR:1.3, p = 0.024) significantly distinguished the group with poorer prognosis in GEPIA (Figure 5A), it failed to impose any disadvantages to the HDAC2-overexpressed group (p = 0.39) when validated (Figure 6A). Moreover, HDAC1 (HR:3.0, p < 0.0001) acted as a hazard predictive biomarker, and HDAC11 (HR:0.5, p = 4.8e−08) was still a protective factor in the DFS analysis (Figures 7A,C). The relatively low mutation ratio of HDAC1 and HDAC11 probably suggested the stability of the genes (Figure 8A).
In contrast to less efficient discrimination of HDAC2 and HDAC11, the AUC value of HDAC1 over 0.7 throughout the time points manifested that HDAC1 was identified to be a promising prognostic biomarker for glioma (Figures 9A–C; Table 2). It was found recently that knockdown of HDAC1 with siRNA reduced LN18 GBM cell proliferation, leaving cell viability unaffected (Was et al., 2019). The result that simultaneous inhibition of HDAC1 and HDAC2 led to a significant drop in GBM cell proliferation synergistically suggested an efficient combination anticancer strategy (Was et al., 2019). More in-depth research found the involvement of HDAC1 together with HDAC2 in the regulation of a transcription factor c-Myc (Nguyen et al., 2020). The selective and broad inhibition of HDAC1 and HDAC2 disrupted c-Myc regulation on aerobic glycolysis enhancing oxidative metabolism, followed by peroxisome proliferator-activated receptor γ coactivator1 α (PGC1α) and peroxisome proliferator-activated receptor δ (PPARδ), thereby extending overall survival of patient-derived xenograft (Nguyen et al., 2020). The additional mechanism of HDAC1-involved invasive and proliferative phenotype in GBM cells could be attributed to the interaction between HDAC1 and phosphorylated special AT-rich sequence-binding protein 1 (SATB1) (Han et al., 2013).
It also exposed the moderate relationship between HDAC1 expression and neutrophil infiltration (r = 0.60, p = 2.88e-47) in LGG, as well as CD4+ T cell infiltration (r = 0.52, p = 3.96e-35) (Figure 9D). Although the studies on how the HDAC1 expression predicted immune infiltration in LGG were urgently needed, it was demonstrated that IFN-β silenced interleukin-8 (IL-8) transcription by increasing HDAC1 expression level in GBM cells (Nozell et al., 2006). Given that IL-8 was one of the chemokines that recruited migrating neutrophils, impaired IL-8 release likely resulted in reduced neutrophil infiltration, which might explain the absence of relation between HDAC1 and neutrophil infiltration in the GBM.
The components of the final HDAC1-related signature could be the reason why the separability of the signature exhibited robust efficiency (1-year OS AUC = 0.80, 3-years OS AUC = 0.84, 5-years OS AUC = 0.829, 10-years OS AUC = 0.882) (Figures 10D,E). In the signature, HDAC1 (HR:1.4938) and RBL1 (HR:1.7148) were deemed a hazard, while RUNX1T1 (HR:0.7349), FKBP3 (HR:0.6382), and PHF21A (HR:0.4956) promoted survival possibilities for glioma patients (Figure 10A). The HDAC1-related signature was adjusted as an independent risk indicator and eventually validated both internally and externally showing reliable discrimination for glioma prognosis prediction (Supplementary Figure S3; Figures 10D–F; Figures 11A,B).
Of note, HDAC1 was transcriptionally regulated by the nuclear factor of activated T cell (NFAT), which played a role in glioma stem cell growth and mesenchymal transition (Song et al., 2020). Retinoblastoma transcriptional corepressor like 1 (RBL1), known for its modulation in the G1/S cell cycle, behaved oppositely and functioned as a tumor suppressor in a GBM model, conflicts of which could come from either the species’ differences or some other regulations unidentified (Naert et al., 2020). RUNX1 partner transcriptional co-repressor 1 (RUNX1T1) earned prestige for its fusion with Runt-related transcription factor 1 (RUNX1) in acute myeloid leukemia (Beghini, 2019). Related to HDAC class I signaling, FK506 Binding Protein 3 (FKBP3) regulated HDAC2 expression contributing to the drug resistance in tumor cells (Tong et al., 2019). PHD finger protein 21A (PHF21A) was mainly studied in neurodevelopmental disorders (Vallianatos and Iwase, 2015). Though the research on RUNX1T1, FKBP3, and PH21A in glioma was definite, the signature was annotated as “chromatin binding” by the functional enrichment analysis (Figure 10E).
However, the prognostic HDAC1 per se and the HDAC1-related gene signature were yet to be employed and verified universally. Considering some potential barriers when promoting sequencing techniques in clinical oncology testing, it would require more time for the real arrival of the molecular prognostic biomarker era. In addition, it remains a question to be solved regarding how HDAC1 was involved with the glioma progression and the modulation of immune infiltration in glioma. Acetylation and deacetylation were assumed to stand downstream in the HDAC-involved glioma modulation and thereby possibly influence glioma prognosis, which also warranted large-scale data to testify in the future. How epigenetics like methylation affected glioma through regulation of the HDACs remains another interesting topic.
Conclusion
Conclusively, the comprehensive analysis of the HDAC family exposed that the HDAC family components were of prognostic significance for glioma. Overexpressed HDAC1 in glioma positively altered its expression with glioma grade, IDH1 wildtype, and 1p/19q non-codeletion, while HDAC11 was downregulated and acted oppositely compared to HDAC1. The majority of the HDAC family significantly correlated with the grade, IDH1 mutation, and 1p/19q codeletion in glioma. HDAC1 was manifested to serve as a prognostic biomarker for glioma, and an indicator for neutrophil and CD4+ T cell infiltration in LGG. Ultimately, the HDAC1-centered signature composed of the HDAC1-related genes was developed and validated for precise prognosis prediction in glioma. It was tempting to speculate an optimistic future for glioma not only by implementing HDAC1 and other HDACs as prognostic biomarkers but also by targeting HDAC1 and its closely related genes.
Data Availability Statement
The original contributions presented in the study are included in the article/Supplementary Material, further inquiries can be directed to the corresponding author.
Author Contributions
The study and conception were designed by YF, YW, and GZ; the coding and data analysis was done by YF, XP, and YW; figures and tables were prepared by YF, XP, and BL; the manuscript was drifted by YF; reviewing and editing was completed by YW and GZ. All authors contributed to the manuscript revision. All authors read and approved the final version of the manuscript.
Funding
We acknowledge the funding support from the National Natural Science Foundation of China (Grant Number: 81772684, Beijing, China).
Conflict of Interest
The authors declare that the research was conducted in the absence of any commercial or financial relationships that could be construed as a potential conflict of interest.
Publisher’s Note
All claims expressed in this article are solely those of the authors and do not necessarily represent those of their affiliated organizations, or those of the publisher, the editors and the reviewers. Any product that may be evaluated in this article, or claim that may be made by its manufacturer, is not guaranteed or endorsed by the publisher.
Supplementary Material
The Supplementary Material for this article can be found online at: https://www.frontiersin.org/articles/10.3389/fmolb.2021.720020/full#supplementary-material
Supplementary Figure S1 | LASSO regression of the HDAC1-related genes. The trajectory plot on the left panel was for independent variables sourced from the HDAC1-related genes. The plot on the right panel showed that each lambda and confidence interval. LASSO, least absolute shrinkage, and selection operator.
Supplementary Figure S2 | Candidate HDAC1-related genes for the signature under proportional hazard assumption.
Supplementary Figure S3 | The HDAC1-related signature adjusted to be an independent risk indicator.
Supplementary Table S1 | HDAC1-related genes collected from STRING and GeneMANIA.
Supplementary Table S2 | HDAC1-related genes with significance in univariate and multivariate Cox regression analyses.
Abbreviations
AUC, area under the curve ; CNS, central nervous system; CI, confidence interval; DFS, disease-free survival; FC, fold change; FKBP3, FK506 binding protein 3; GEPIA, gene expression profiling interactive analysis; GTEx, genotype-tissue expression project; GBM, glioblastoma multiforme; HR, hazard ratio; HAT, histone acetyltransferase; HDAC, histone deacetylase; IDH1, isocitrate dehydrogenase 1; KM, Kaplan-Meier; LGG, lower-grade glioma; MDSC, myeloid-derived suppressive cell; NFAT, nuclear factor of activated T cell; OS, overall survival; PPARδ, peroxisome proliferator-activated receptor δ; PGC1α, peroxisome proliferator-activated receptor γ coactivator1 α; PHF21A, PHD finger protein 21A; PH, proportional hazards; ROC, receiver operating characteristic; Tregs, regulatory T cells; RBL1, retinoblastoma transcriptional corepressor like 1; RUNX1, runt-related transcription factor 1; RUNX1T1, RUNX1 partner transcriptional co-repressor 1; STRING, search tool for the retrieval of interacting genes; SATB1, special AT-rich sequence-binding protein 1; TCGA, the cancer genome atlas; LASSO, the least absolute shrinkage and selection operator; TIMER, tumor immune estimation resource.
References
Beghini, A. (2019). Core Binding Factor Leukemia: Chromatin Remodeling Moves towards Oncogenic Transcription. Cancers 11 (12), 1973. doi:10.3390/cancers11121973
Bolden, J. E., Peart, M. J., and Johnstone, R. W. (2006). Anticancer Activities of Histone Deacetylase Inhibitors. Nat. Rev. Drug Discov. 5 (9), 769–784. doi:10.1038/nrd2133
Bredel, M., Bredel, C., Juric, D., Harsh, G. R., Vogel, H., Recht, L. D., et al. (2005). Functional Network Analysis Reveals Extended Gliomagenesis Pathway Maps and Three Novel MYC-Interacting Genes in Human Gliomas. Cancer Res. 65 (19), 8679–8689. doi:10.1158/0008-5472.CAN-05-1204
Chen, R., Zhang, M., Zhou, Y., Guo, W., Yi, M., Zhang, Z., et al. (2020). The Application of Histone Deacetylases Inhibitors in Glioblastoma. J. Exp. Clin. Cancer Res. 39 (1), 138. doi:10.1186/s13046-020-01643-6
Colaprico, A., Silva, T. C., Olsen, C., Garofano, L., Cava, C., Garolini, D., et al. (2016). TCGAbiolinks: an R/Bioconductor Package for Integrative Analysis of TCGA Data. Nucleic Acids Res. 44 (8), e71. doi:10.1093/nar/gkv1507
Eyüpoglu, I. Y., and Savaskan, N. E. (2016). Epigenetics in Brain Tumors: HDACs Take Center Stage. Cn 14 (1), 48–54. doi:10.2174/1570159x13666151030162457
Franz, M., Rodriguez, H., Lopes, C., Zuberi, K., Montojo, J., Bader, G. D., et al. (2018). GeneMANIA Update 2018. Nucleic Acids Res. 46 (W1), W60–W64. doi:10.1093/nar/gky311
French, P. J., Swagemakers, S. M. A., Nagel, J. H. A., Kouwenhoven, M. C. M., Brouwer, E., van der Spek, P., et al. (2005). Gene Expression Profiles Associated with Treatment Response in Oligodendrogliomas. Cancer Res. 65 (24), 11335–11344. doi:10.1158/0008-5472.CAN-05-1886
Gao, J., Aksoy, B. A., Dogrusoz, U., Dresdner, G., Gross, B., Sumer, S. O., et al. (2013). Integrative Analysis of Complex Cancer Genomics and Clinical Profiles Using the cBioPortal. Sci. Signaling 6 (269), pl1. doi:10.1126/scisignal.2004088
Gravendeel, L. A. M., Kouwenhoven, M. C. M., Gevaert, O., de Rooi, J. J., Stubbs, A. P., Duijm, J. E., et al. (2009). Intrinsic Gene Expression Profiles of Gliomas Are a Better Predictor of Survival Than Histology. Cancer Res. 69 (23), 9065–9072. doi:10.1158/0008-5472.CAN-09-2307
Han, S., Xia, J., Qin, X., Han, S., and Wu, A. (2013). Phosphorylated SATB1 Is Associated with the Progression and Prognosis of Glioma. Cell Death Dis 4, e901. doi:10.1038/cddis.2013.433
Kuhn, M. (2008). Building Predictive Models inRUsing thecaretPackage. J. Stat. Soft. 28 (5), 1–26. doi:10.18637/jss.v028.i05
Kunadis, E., Lakiotaki, E., Korkolopoulou, P., and Piperi, C. (2021). Targeting post-translational Histone Modifying Enzymes in Glioblastoma. Pharmacol. Ther. 220, 107721. doi:10.1016/j.pharmthera.2020.107721
Lapointe, S., Perry, A., and Butowski, N. A. (2018). Primary Brain Tumours in Adults. The Lancet 392 (10145), 432–446. doi:10.1016/s0140-6736(18)30990-5
Li, T., Fu, J., Zeng, Z., Cohen, D., Li, J., Chen, Q., et al. (2020). TIMER2.0 for Analysis of Tumor-Infiltrating Immune Cells. Nucleic Acids Res. 48 (W1), W509–W514. doi:10.1093/nar/gkaa407
Louis, D. N., Perry, A., Reifenberger, G., von Deimling, A., Figarella-Branger, D., Cavenee, W. K., et al. (2016). The 2016 World Health Organization Classification of Tumors of the Central Nervous System: a Summary. Acta Neuropathol. 131 (6), 803–820. doi:10.1007/s00401-016-1545-1
Lucio-Eterovic, A. K., Cortez, M. A., Valera, E. T., Motta, F. J., Queiroz, R. G., Machado, H. R., et al. (2008). Differential Expression of 12 Histone Deacetylase (HDAC) Genes in Astrocytomas and normal Brain Tissue: Class II and IV Are Hypoexpressed in Glioblastomas. BMC Cancer 8, 243. doi:10.1186/1471-2407-8-243
Minucci, S., and Pelicci, P. G. (2006). Histone Deacetylase Inhibitors and the Promise of Epigenetic (And More) Treatments for Cancer. Nat. Rev. Cancer 6 (1), 38–51. doi:10.1038/nrc1779
Murat, A., Migliavacca, E., Gorlia, T., Lambiv, W. L., Shay, T., Hamou, M.-F., et al. (2008). Stem Cell-Related "Self-Renewal" Signature and High Epidermal Growth Factor Receptor Expression Associated with Resistance to Concomitant Chemoradiotherapy in Glioblastoma. Jco 26 (18), 3015–3024. doi:10.1200/JCO.2007.15.7164
Naert, T., Dimitrakopoulou, D., Tulkens, D., Demuynck, S., Carron, M., Noelanders, R., et al. (2020). RBL1 (P107) Functions as Tumor Suppressor in Glioblastoma and Small-Cell Pancreatic Neuroendocrine Carcinoma in Xenopus Tropicalis. Oncogene 39 (13), 2692–2706. doi:10.1038/s41388-020-1173-z
Nguyen, T. T. T., Zhang, Y., Shang, E., Shu, C., Torrini, C., Zhao, J., et al. (2020). HDAC Inhibitors Elicit Metabolic Reprogramming by Targeting Super-enhancers in Glioblastoma Models. J. Clin. Invest. 130 (7), 3699–3716. doi:10.1172/JCI129049
Nozell, S., Laver, T., Patel, K., and Benveniste, E. N. (2006). Mechanism of IFN-β-Mediated Inhibition ofIL-8Gene Expression in Astroglioma Cells. J. Immunol. 177 (2), 822–830. doi:10.4049/jimmunol.177.2.822
Rhodes, D. R., Yu, J., Shanker, K., Deshpande, N., Varambally, R., Ghosh, D., et al. (2004). ONCOMINE: A Cancer Microarray Database and Integrated Data-Mining Platform. Neoplasia 6 (1), 1–6. doi:10.1016/s1476-5586(04)80047-2
Ruijter, A. J. M. d., Gennip, A. H. v., Caron, H. N., Kemp, S., and Kuilenburg, A. B. P. v. (2003). Histone Deacetylases (HDACs): Characterization of the Classical HDAC Family. Biochem. J. 370 (Pt 3), 737–749. doi:10.1042/BJ20021321
Schiff, D., Van den Bent, M., Vogelbaum, M. A., Wick, W., Miller, C. R., Taphoorn, M., et al. (2019). Recent Developments and Future Directions in Adult Lower-Grade Gliomas: Society for Neuro-Oncology (SNO) and European Association of Neuro-Oncology (EANO) Consensus. Neuro Oncol. 21 (7), 837–853. doi:10.1093/neuonc/noz033
Song, Y., Jiang, Y., Tao, D., Wang, Z., Wang, R., Wang, M., et al. (2020). NFAT2-HDAC1 Signaling Contributes to the Malignant Phenotype of Glioblastoma. Neuro Oncol. 22 (1), 46–57. doi:10.1093/neuonc/noz136
Sun, L., Hui, A.-M., Su, Q., Vortmeyer, A., Kotliarov, Y., Pastorino, S., et al. (2006). Neuronal and Glioma-Derived Stem Cell Factor Induces Angiogenesis within the Brain. Cancer Cell 9 (4), 287–300. doi:10.1016/j.ccr.2006.03.003
Szklarczyk, D., Morris, J. H., Cook, H., Kuhn, M., Wyder, S., Simonovic, M., et al. (2017). The STRING Database in 2017: Quality-Controlled Protein-Protein Association Networks, Made Broadly Accessible. Nucleic Acids Res. 45 (D1), D362–D368. doi:10.1093/nar/gkw937
Tang, Z., Li, C., Kang, B., Gao, G., Li, C., and Zhang, Z. (2017). GEPIA: a Web Server for Cancer and normal Gene Expression Profiling and Interactive Analyses. Nucleic Acids Res. 45 (W1), W98–W102. doi:10.1093/nar/gkx247
Tong, J., Shen, Y., Chen, X., Wang, R., Hu, Y., Zhang, X., et al. (2019). FKBP3 Mediates Oxaliplatin Resistance in Colorectal Cancer Cells by Regulating HDAC2 Expression. Oncol. Rep. 42 (4), 1404–1412. doi:10.3892/or.2019.7259
Vallianatos, C. N., and Iwase, S. (2015). Disrupted Intricacy of Histone H3K4 Methylation in Neurodevelopmental Disorders. Epigenomics 7 (3), 503–519. doi:10.2217/epi.15.1
Was, H., Krol, S. K., Rotili, D., Mai, A., Wojtas, B., Kaminska, B., et al. (2019). Histone Deacetylase Inhibitors Exert Anti-tumor Effects on Human Adherent and Stem-like Glioma Cells. Clin. Epigenet 11 (1), 11. doi:10.1186/s13148-018-0598-5
Wen, P. Y., Weller, M., Lee, E. Q., Alexander, B. M., Barnholtz-Sloan, J. S., Barthel, F. P., et al. (2020). Glioblastoma in Adults: a Society for Neuro-Oncology (SNO) and European Society of Neuro-Oncology (EANO) Consensus Review on Current Management and Future Directions. Neuro Oncol. 22 (8), 1073–1113. doi:10.1093/neuonc/noaa106
Williams, M. J., Singleton, W. G. B., Lowis, S. P., Malik, K., and Kurian, K. M. (2017). Therapeutic Targeting of Histone Modifications in Adult and Pediatric High-Grade Glioma. Front. Oncol. 7, 45. doi:10.3389/fonc.2017.00045
Keywords: glioma, HDAC1, HDAC family, immune infiltration, prognosis, signature
Citation: Fan Y, Peng X, Wang Y, Li B and Zhao G (2021) Comprehensive Analysis of HDAC Family Identifies HDAC1 as a Prognostic and Immune Infiltration Indicator and HDAC1-Related Signature for Prognosis in Glioma. Front. Mol. Biosci. 8:720020. doi: 10.3389/fmolb.2021.720020
Received: 03 June 2021; Accepted: 19 July 2021;
Published: 01 September 2021.
Edited by:
William C. Cho, QEH, Hong Kong, SAR ChinaReviewed by:
Ajay Chatrath, University of Virginia, United StatesMonal Sharma, Duke University, United States
Copyright © 2021 Fan, Peng, Wang, Li and Zhao. This is an open-access article distributed under the terms of the Creative Commons Attribution License (CC BY). The use, distribution or reproduction in other forums is permitted, provided the original author(s) and the copyright owner(s) are credited and that the original publication in this journal is cited, in accordance with accepted academic practice. No use, distribution or reproduction is permitted which does not comply with these terms.
*Correspondence: Gang Zhao, emhhb2dqZHl5QDEyNi5jb20=