- 1Laboratório de Genetica e Cardiologia Molecular, Instituto do Coração (InCor), Hospital das Clinicas HCFMUSP, Faculdade de Medicina, Universidade de Sao Paulo, Sao Paulo, Brazil
- 2School of Biological Sciences, Faculty of Biology, Medicine and Health, University of Manchester, Manchester, United Kingdom
- 3Manchester Cell-Matrix Centre, Division of Cell-Matrix Biology and Regenerative Medicine, School of Biological Sciences, Faculty of Biology, Medicine and Health, University of Manchester, Manchester, United Kingdom
The dynamic behavior of proteins within cellular structures can be studied using fluorescence recovery after photobleaching (FRAP) and fluorescence loss after photobleaching (FLAP) experiments. These techniques provide insights into molecular mobility by estimating parameters such as turnover rates
1 Introduction
Fluorescence recovery after photobleaching (FRAP) and fluorescence loss after photobleaching (FLAP) are two related imaging techniques used in cell biology to study the dynamics of fluorescently labeled proteins within living cells. These techniques provide valuable insights into the movement, interactions, and turnover rates of cellular components (Day et al., 2012; Dunn et al., 2002). In FRAP, a specific region of interest within a cell is selected to study a labeled molecule with a fluorescent marker, typically a fluorescently tagged protein or lipid. This region is subjected to intense light, such as from a laser, which bleaches the fluorophores in that area, rendering them non-fluorescent. The fluorescence recovery in the bleached area over time is then monitored using a fluorescence microscope. As fluorescent molecules from the surrounding unbleached areas diffuse into the bleached region, the fluorescence signal gradually returns, allowing researchers to measure the rate and extent of recovery. This recovery can provide valuable information about the labeled molecule’s mobility, turnover, and interactions within the cell (Sprague and McNally, 2005; Lippincott-Schwartz et al., 2001; Axelrod et al., 1976; Hickey et al., 2021). FLAP, on the other hand, is focused on studying the mobility and dynamics of specific proteins within living cells. The molecule to be located carries two fluorophores: one to be photobleached and the other to act as a reference label. The use of a reference fluorophore permits the distribution of the photo-labeled molecules themselves to be tracked by simple image differencing. FLAP is comparable with methods to track fluorescent proteins by direct photoactivation, however, instead of monitoring the overall recovery of fluorescence within the bleached area alike in FRAP, it involves tracking the movement of fluorescently labeled molecules into and out of subcellular structures. This allows researchers to assess not only the overall mobility of the labeled molecules but also their specific localization within different cellular compartments (Lippincott-Schwartz et al., 2001; Hickey et al., 2021).
Mathematical modeling of FRAP and FLAP data allows the determination of dissociation and association rates (
Existing models analyzing FRAP and FLAP experiments are often deterministic and rely on simplification and assumptions about particular parameter values to calculate the analytical solution (Phair et al., 2003; Phair and Misteli, 2001; Lippincott-Schwartz et al., 2018). In this work, we are proposing the first stochastic mathematical model that relies on only 2 parameters to explain the dynamics of protein behavior inside the FA (region of interest - R.O.I.). The model is very straightforward and does not require any prior assumptions. It uses only the information of the turnover rate
Our study examines FRAP and FLAP outcomes for 12 proteins within the focal adhesion (FA). FA are multiprotein assemblies that manifest as localized adhesive structures, readily observable through fluorescence microscopy, as illustrated in Figure 1. More than 2,000 proteins have been associated with a wider protein network of FAs, and about 60 of them are core adhesion proteins that play a direct role in regulating cell-matrix adhesion (Atherton et al., 2015; 2016; Jansen et al., 2017; Kanchanawong et al., 2010). Based on their dynamic turnover rates, these proteins can be distinctly categorized into mechanosignaling, intermediate and mechanosensing modules, as proposed by (Stutchbury et al., 2017) and illustrated in Figure 1. Mechanosensing proteins are those that link integrins to the contractile force machinery and mechanosignalling proteins modulate this link through signals that control Rho GTPases which in turn influence the actin polymerization or/and actomyosin contractility. Despite the advances in our understanding, the mechanisms by which the different proteins cooperate and coordinate the communication of the cells with the surrounding extracellular matrix (ECM) are still unclear.
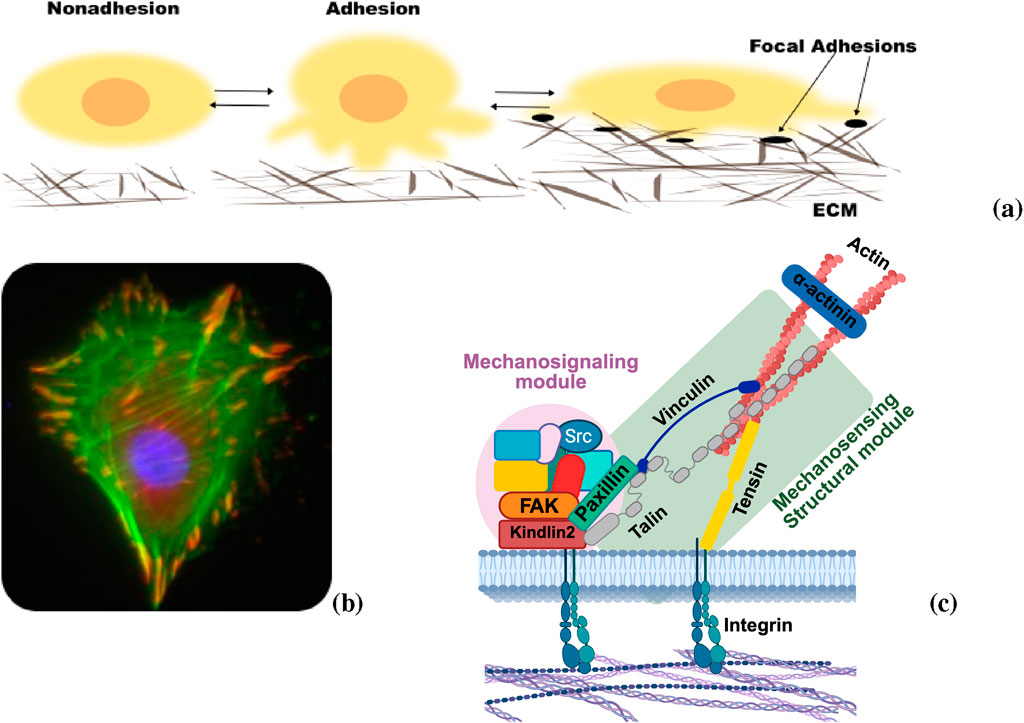
Figure 1. Integrin-mediated cell-matrix adhesions. (a) During cell spreading, cells form cell-matrix adhesions, which in cultured cells are called Focal Adhesions (FAs). (b) Fluorescence microscopy of. Smooth muscle cell stained for the FA marker paxillin (red) and filamentous actin (F-actin; green); nucleus in blue (image captured by the authors). (c) Model of molecular constituents of FA proposed by (Stutchbury et al., 2017). The model displays two modules involved in mechanotransduction: the mechanosensing module, comprising proteins that form a direct link to the contractile actomyosin (e.g., talin and vinculin), and other regulatory proteins that are involved in signaling processes (kindlin, FAK, paxillin and other proteins) (Created in BioRender. DE OLIVEIRA, L. (2025) https://BioRender.com/2q1onya).
Here, we introduce a stochastic model grounded in the analytical solution of the chemical master equation (CME), which we apply to analyze FRAP and FLAP data. Our model estimates the rates of protein entrance
2 Methods
2.1 Experimental data
The experimental data were obtained from previous FRAP and FLAP data sets. For FRAP experiments, NIH3T3 fibroblasts were transfected with GPF-tagged FA proteins of interest (tensin 1, talin, vinculin, a-actinin, ILK, a-parvin, kindlin-2, paxillin, p130Cas, VASP, FAK or zyxin, Figure 2a). Photobleaching was achieved with a 488 nm laser and fluorescence recovery monitoring at 10-s intervals for up to 5 min using a DeltaVision system RT microscope (Stutchbury et al., 2017).
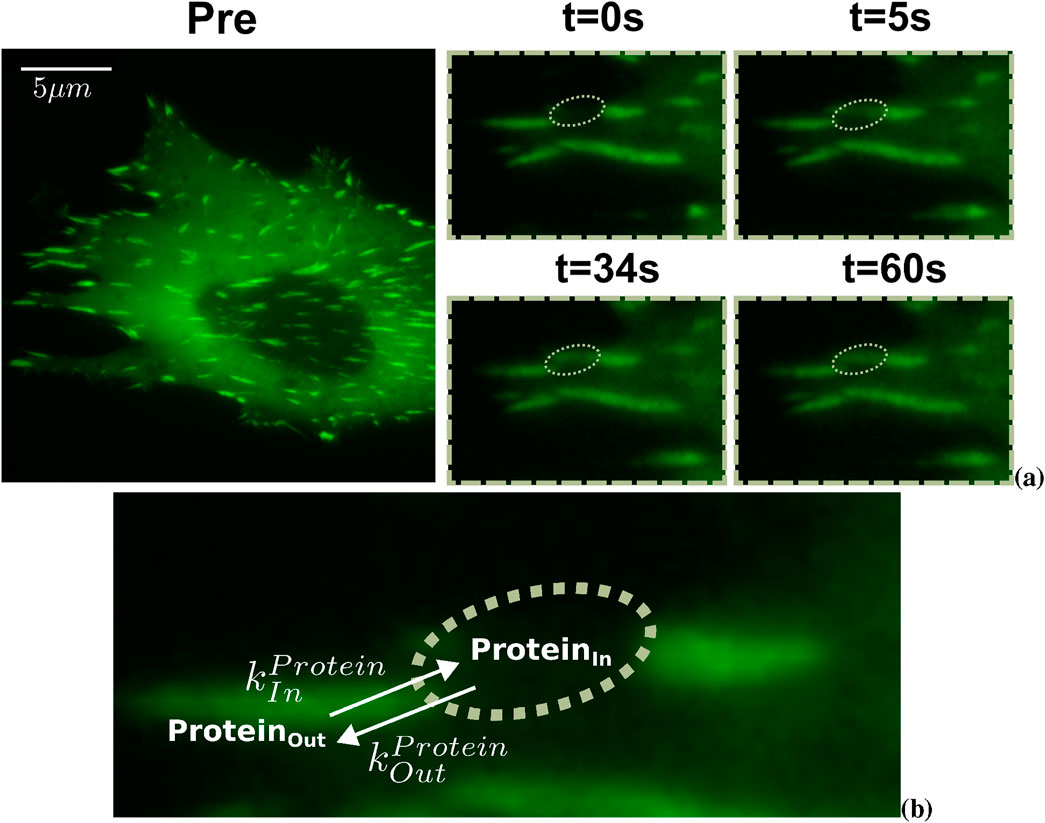
Figure 2. Experimental and theoretical framework for studying focal adhesion protein dynamics. (a) Example of time-lapse images from a Fluorescence Recovery After Photobleaching (FRAP) experiment in NIH3T3 fibroblasts transfected with a GFP-tagged FA protein. The dashed box indicates the region of interest (R.O.I.) selected for photobleaching, corresponding to a FA. Insets show magnified views of the R.O.I. at different time points post-bleaching, highlighting fluorescence recovery within the bleached region (dotted ellipse). (b) Schematic representation of the abstract model used for interpreting FRAP and Fluorescence Loss After Photobleaching (FLAP) experiments. Proteins outside the FA region (ProteinOut) can enter (rate
For FLAP experiments, NIH 3T3 cells were transfected with PAGFP-tagged protein of interest (talin-full length, talinΔR1R10, talinΔR2R3 or talinΔR4R10) and mCherry-tagged marker. Photoactivation was performed with a 405 nm laser and imaging was conducted using a spinning disk confocal microscope (Atherton et al., 2015).
2.2 Extracting dynamic parameters from FRAP and FLAP experiments
FRAP and FLAP experimental data consist of fluorescence intensity curves representing the recovery or the loss of intensity, respectively (Ishikawa-Ankerhold et al., 2012). These curves have an exponential shape and can be fitted using the equation
The analytical solution of the chemical master equation used in our model relies on two experimental parameters: the turnover rate
2.3 Stochastic model of master equation
The stochastic mathematical model is built using a combination of experimental data from FRAP and FLAP (Atherton et al., 2015; Stutchbury et al., 2017) and the formalism of the chemical master equation (De Oliveira, 2014; Qian and Bishop, 2010; Van Kampen, 1992). The chemical master equation is a class of discrete-state, continuous-time Markov jump processes, known as multi-dimensional birth-death processes in probability theory (Pinsky and Karlin, 2010; Van Kampen, 1992). In this formalism, the concentration of proteins is modeled as temporal variables assuming non-negative real values. These processes are continuous in time, their range consists of integers, and only jumps between adjacent states are permitted (Van Kampen, 1992).
2.3.1 Protein in-out model
Based on the experimental data, we propose a model to describe protein dynamics in FRAP/FLAP experiments. In this model, the protein population is represented by its concentration inside
This means that the system is fully described in terms of one of the two populations,
2.3.2 Inference of dynamic parameter using the analytical solution of the chemical master equation
Following the Protein-In-Out model 2.3.1, the one protein FRAP/FLAP experiment is fully described by a one-dimensional chemical master equation (Van Kampen, 1992), that is written as:
Where the protein concentration over time inside the FA is described by the probability
1. The gain term,
2. The recombination term,
The system represented by Equation 2 respects the detailed balance condition, which means the system has an exact analytical solution (Toral and Colet, 2014),
Where
From Equation 3, the concentration of proteins in the stationary state
The turnover rate of FRAP/FLAP experiments is represented as the combination of
With Equations 4, 5 the experimental values of
Where the stationary concentration of mobile proteins
2.3.3 Inference of protein interaction using the chemical master equation
Data from individual protein experiments (Section 2.3.1, Section 2.3.2) was used to build a network representing the interaction of proteins inside the FA. At this step, the dynamic interaction between proteins was described by the chemical master equation divided into two steps: 1) the entrance and exit of proteins in the FA, and 2) the interaction of those proteins (Figure 3). FA is a complex of more than 60 proteins (Jansen et al., 2017; Kanchanawong et al., 2010; Zaidel-Bar et al., 2003), and the experimental data measure a combinatory behavior of the interaction of the protein with some other proteins in the FA. Figure 3 is a simplification of this system considering 3 proteins, where all other proteins of the FA complex are taken into account by the term “other proteins”.
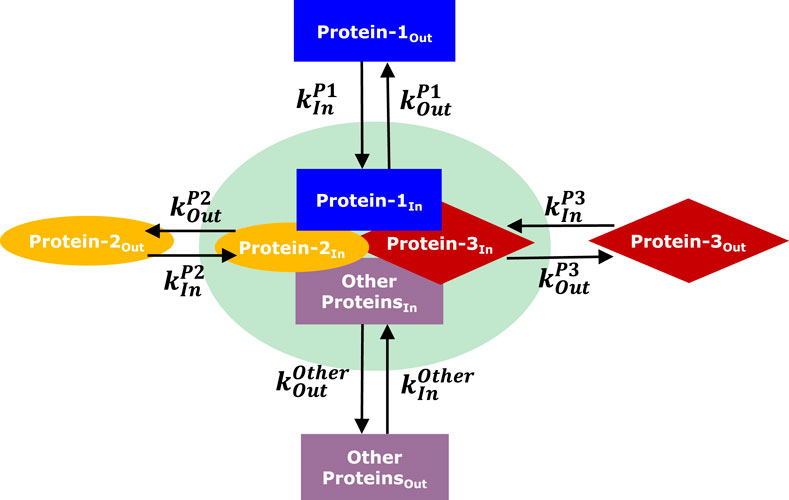
Figure 3. Representation of FA protein interactions considering 3 proteins to be studied, where only the interactions of protein 2 and 3 with protein 1 are considered. The model considers the entrance and exit of proteins in and out of the FA (green region). Each protein has one pair of dynamic rates
In order to understand the influence of
Where
2.4 Statistical methods
2.4.1 Outliers removal
The presence of outliers in the experimental data was examined, presenting a challenge due to the experiments having a bi-dimensional nature (temporal curves) rather than one-dimensional points. To address this, each curve of each protein was individually fitted, resulting in a unique
Outliers were identified from the
Also the definition of the upper
After determining
2.4.2 Cross-validation
As the experimental data used to calculate the dynamic parameters
2.4.2.1 K-Fold cross-validation
K-Fold cross-validation (Borra and Di Ciaccio, 2010) is a technique that partitions the dataset into K subsets. Each iteration of this method involves training the model K times, with each subset serving as an independent validation set to calculate the prediction error. This process yields K estimates of the model’s predictive performance. To qualitatively interpret the predictive capacity of the model, the Mean Absolute Percentage Error (MAPE) was used Table 1 (Lewis, 1982). A MAPE value below 20% indicates that the model is suitable for the prediction of the protein behavior.
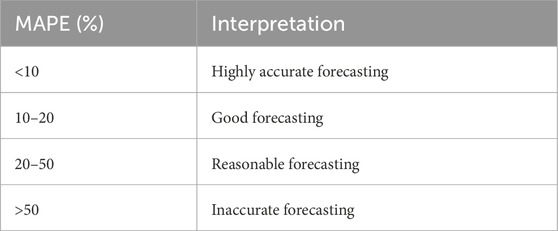
Table 1. MAPE interpretability (Lewis, 1982).
3 Results
In this section, we present the results of our analysis using the analytical solution of the chemical master equation applied to FRAP and FLAP data. The experimental data used in this study were extracted from previously published datasets of FRAP and FLAP experiments (Stutchbury et al., 2017; Atherton et al., 2015). The dynamic parameters, turnover rate
The analysis of the dynamic parameters was performed in several steps: first, we evaluated the performance prediction of the analytical solution of the chemical master equation, followed by the determination of the dynamic parameters. Next, we explored the influence of
Our findings demonstrate that the model effectively captures the dynamics of proteins within the FA, offering insights into protein turnover and interactions that go beyond the traditional analysis of
3.1 Performance evaluation of the analytical solution to the chemical master equation using K-Fold cross-validation
The K-Fold cross-validation was performed on the outlier-free dataset. The choice of K represents a trade-off between bias and variance (Borra and Di Ciaccio, 2010), therefore the data was cross-validated using K = 3, K = 5 and K = 10. For each value of K the cross-validation procedure was reproduced 100 times. The MAPE obtained across all K values fall within the “Highly accurate” to “Good” ranges, as defined by Table 2. Therefore, the model possesses strong predictive capabilities of protein dynamic behavior by the analytical solution. The model’s validity is maintained even when outlier data is retained in the dataset (Supplementary Table S3).
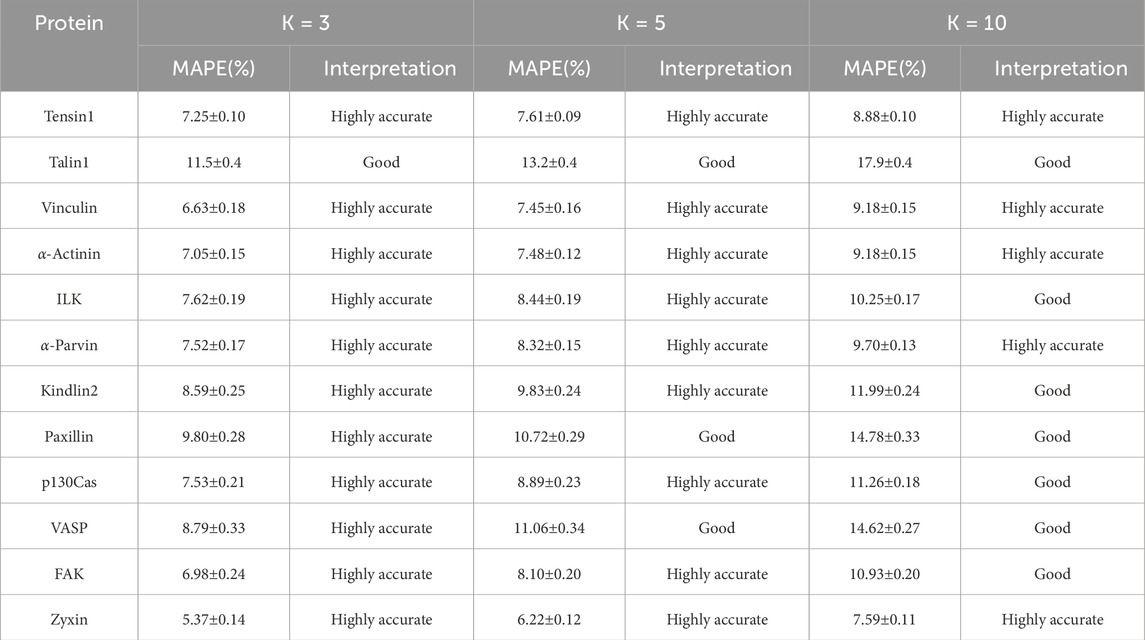
Table 2. K-Fold cross-validation results: The results are interpreted following Table 1. For the three studied values of K (3, 5 and 10), 100 reproductions were performed, the averages of MAPE values are presented with their respective uncertainties.
3.2 Determination of dynamic parameters through the analytical solution using the chemical master equation
The analytical solution of the chemical master equation (see Section 2.3.2) demonstrated strong predictive performance in modeling protein dynamics, as indicated in Table 2. The dynamic parameters
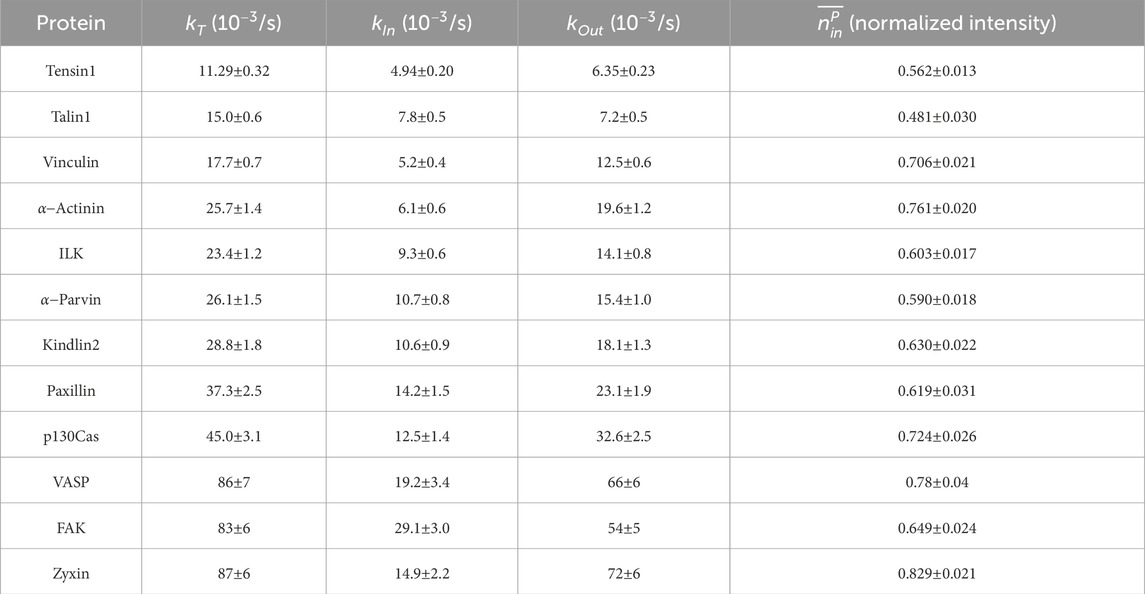
Table 3. Dynamic rates of FA proteins: The turnover rate
In Figure 4, a representative protein from each mechanotransduction module is presented: structural (talin1, Figure 4a), intermediate (
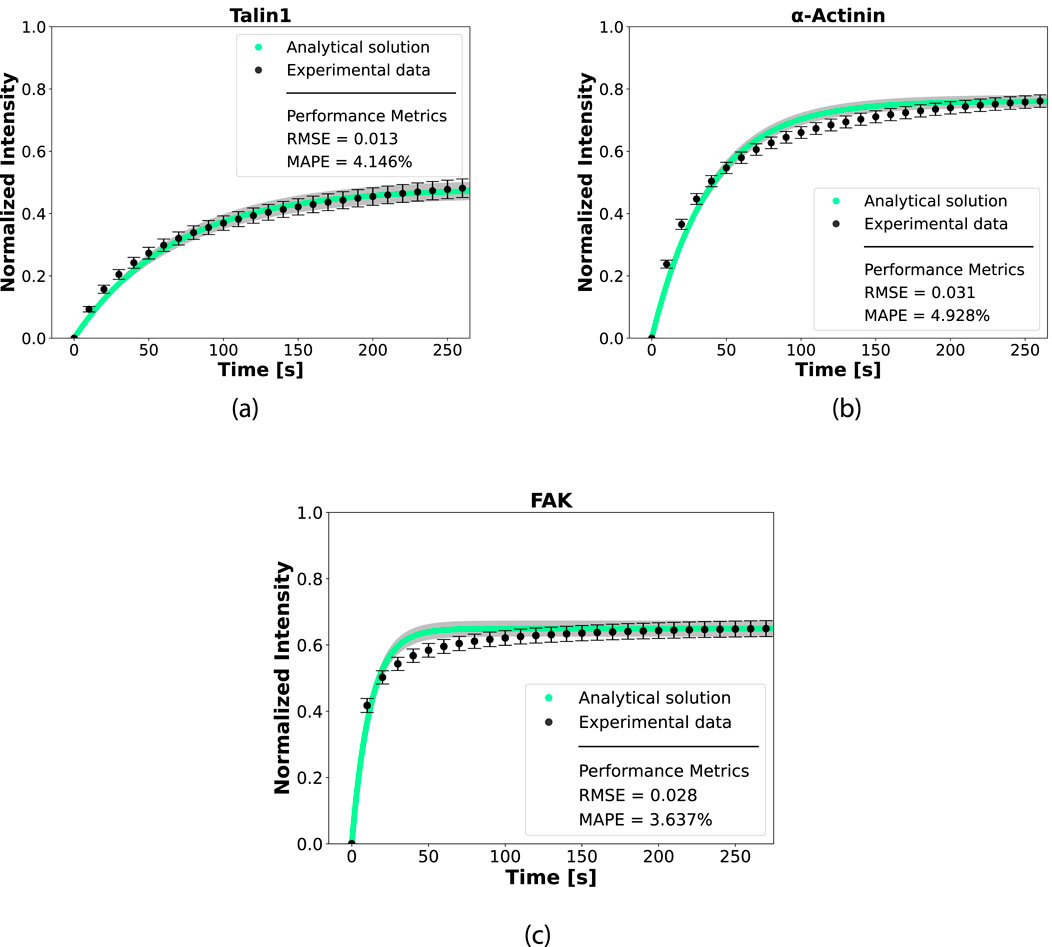
Figure 4. Performance of the model Protein In-Out model using the analytical solution of the chemical master equation for three proteins of each mechanotransduction modules. (a) mechanosensing module: Talin1; (b) intermediate module:
Although the model does not explicitly calculate protein interactions, the dynamic parameters
The dynamic curves derived from these parameters align with the FRAP experimental data reported by (Stutchbury et al., 2017) (see Supplementary Figures S2–S4). In conclusion, the dynamic parameters
3.2.1 Exploring the influence of and on the dynamics FA proteins
To elucidate the impact of dynamic parameters,
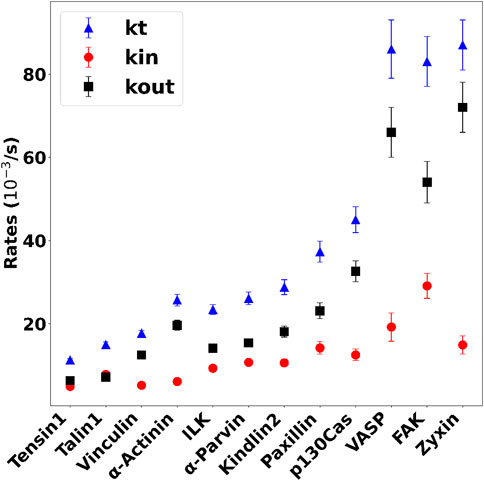
Figure 5. Plot of the normalized rates of all FA proteins belonging to different modules. Blue triangles are the turnover rate
3.3 Explore dynamic interaction between proteins using and
To assess the ability of the analytical solution to describe protein-protein interactions, talin was selected as a model. Talin plays a central role in cell adhesion by linking integrin receptors to the actin cytoskeleton (Figure 1). It is a large protein with a modular domain organization that contributes to its structural flexibility and diverse functions (Critchley, 2009; Gingras et al., 2009; Anthis et al., 2009; Calderwood et al., 1999; Atherton et al., 2015). In addition, talin binds to vinculin via multiple vinculin-binding sites in the talin rod region (Zhang et al., 2008; Wang, 2012; Ruoslahti, 1991). This interaction stabilizes FA and is a process that is thought to be regulated by mechanical force through talin’s interaction with integrins at the N-terminal FERM region and with F-actin at its C-terminal ABS2 and ABS3 regions (Yao et al., 2016; Chorev et al., 2018). To facilitate the understanding of the contribution of each domain of talin, the protein was divided into functional sites as shown in Supplementary Figure S5.
The network representing the interactions of talin with vinculin and
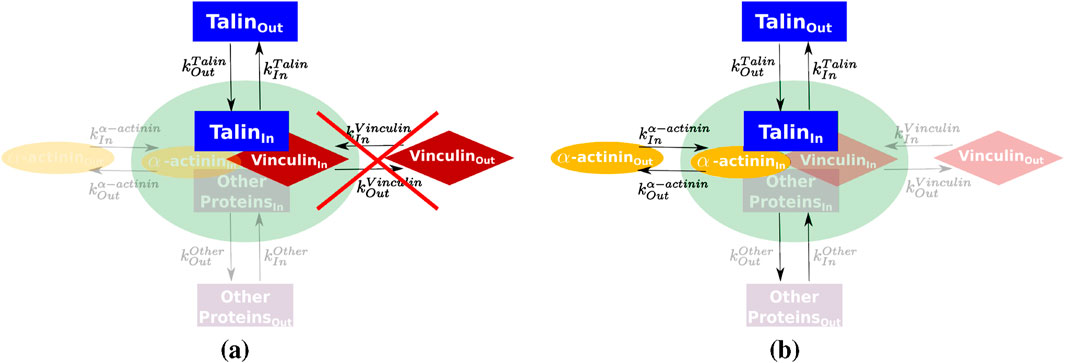
Figure 6. Abstract model for the network of interactions of vinculin and
To evaluate whether our stochastic framework can capture the regulatory influence of vinculin on talin dynamics (Figure 6a), we define a modified differential equation that incorporates the effect of vinculin into the dynamics of talin, as introduced in Section 2.3.3. The master equation describing the time evolution of the probability distribution of talin molecules in the bound state is given by:
The term
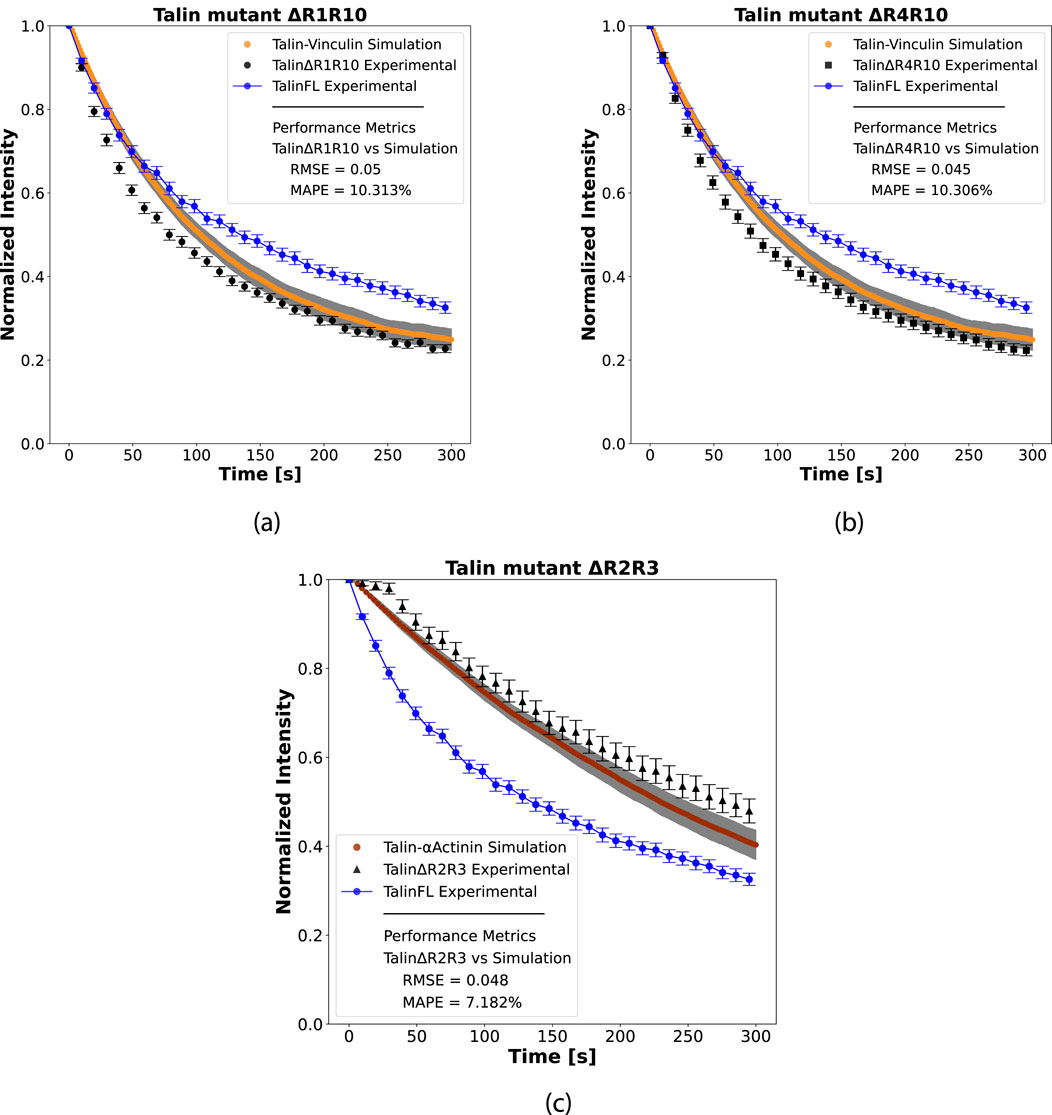
Figure 7. Numerical solution of the stochastic model taking into account the influence of vinculin and actin in talin dynamics. The dynamic of talin-FL at FA is represented in blue (−), which was obtained by FLAP experimental data and chemical master equation solution. The exclusion of vinculin (a,b) contribution resulted in an increased turnover rate of the talin dynamics (orange line represents the mean curve of the 1000 simulations, and the grey area represents the standard error). It is similar to FLAP experimental data of (a) talin
To incorporate the regulatory influence of actomyosin on talin dynamics, we extend the differential equation to:
The term
Altogether, our data demonstrate that our model can be extended to infer protein interactions. By using the value of the dynamic rates of full-length talinFL (wild-type) and modifying its interactions with vinculin and actomyosin, we effectively simulated scenarios representing a loss of vinculin interaction (resulting in increased talin turnover rate), and constitutive binding to actomyosin (resulting in reduced talin turnover).
4 Discussion
We developed a stochastic mathematical model based on the analytical solution of the chemical master equation to infer dynamic rates,
The model describes different protein dynamics with the same accuracy as determined experimentally, distinguishing between proteins in the mechanotransduction modules as previously proposed (Stutchbury et al., 2017): structural (tensin1, talin1, and vinculin, Supplementary Figure S2), intermediate (
Several models have been described in the literature to extract dynamic parameters from FRAP and FLAP experiments. The reaction-diffusion model integrates molecular diffusion processes and chemical reactions (such as binding and unbinding) to represent molecular movement and interactions within cells (Carrero et al., 2003; Phair and Misteli, 2001; Sprague et al., 2004; Mueller et al., 2008). Key parameters inferred from FRAP experiments using this model include the diffusion coefficient, binding rates, and unbinding rates. However, a significant challenge is to accurately estimate these parameters when the underlying model assumptions are oversimplified, potentially resulting in misleading interpretations and compromising the predictive reliability of the model (Mai et al., 2011). On the other hand, kinetic models focus on biochemical reaction rates, such as binding and unbinding, and use ordinary differential equations (ODEs) to describe the temporal evolution of molecular concentrations (Mai et al., 2011; Sprague et al., 2004). It is a deterministic model that may not fully capture the stochastic nature of biological systems. For more realistic representations, stochastic models such as Monte Carlo simulations can apply random sampling to probabilistically model molecular movements and interactions in FRAP experiments (Mueller et al., 2008).
However, these simulations are computationally demanding, and require substantial computational resources and time, particularly for complicated or large-scale models (Lorén et al., 2015). Another alternative for parameter estimation is the Hidden Markov Model (HMM), which uses probabilistic frameworks to describe systems where the state (hidden) is inferred from observable data (emissions). In FRAP experiments, HMMs can model the temporal dynamics of molecular interactions through transitions between hidden states governed by transition and emission probabilities (Braeckmans et al., 2003; Braga et al., 2004; Mazza et al., 2008). However, the challenges of this type of modeling include the complex estimation of these probabilities, especially in the presence of noisy data or numerous hidden states. In the present work, we present a straightforward model using the chemical master equation and two parameters traditionally obtained from FRAP and FLAP experiments: the turnover rate
The chemical master equation also efficiently describes protein-protein interactions and how they affect protein dynamics. This information cannot be obtained from experimental data alone (Sprague and McNally, 2005; Lippincott-Schwartz et al., 2018; Geverts et al., 2015; Williamson et al., 2021). Knowing the values of
Here, we used
In summary, our model navigates in various environments and can describe very different protein dynamics. Furthermore, it predicts information for the influence of protein interaction using only
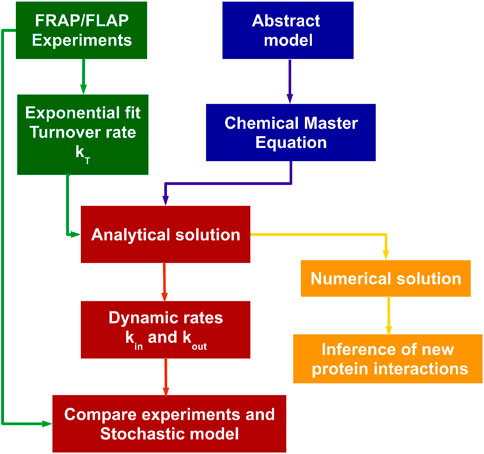
Figure 8. Strategy workflow of the analytical solution of the chemical master equation to infer dynamic rates from FRAP and FLAP experiments. The experimental data (green boxes) allow the extraction of turnover rate
Data availability statement
The original contributions presented in the study are included in the article/Supplementary Material, further inquiries can be directed to the corresponding authors.
Author contributions
LO: Conceptualization, Data curation, Formal Analysis, Methodology, Writing – original draft. MF: Conceptualization, Data curation, Formal Analysis, Methodology, Writing – original draft. JP: Data curation, Methodology, Writing – review and editing. J-MS: Supervision, Writing – review and editing. JK: Funding acquisition, Resources, Writing – review and editing. CB: Conceptualization, Funding acquisition, Resources, Supervision, Writing – review and editing. AM: Conceptualization, Funding acquisition, Resources, Supervision, Writing – original draft, Writing – review and editing.
Funding
The author(s) declare that financial support was received for the research and/or publication of this article. LO was a recipient of a fellowship from the Coordination of Superior Level Staff Improvement (CAPES 88887.136406/2017-00). JK is supported by National Council for Scientific and Technological Development (CNPq 442643/2020-9 and CNPq 408426/2021-7) and the National Institute of Science and Technology Medicine Assisted by Scientific Computing (INCT-MACC) funded by Sao Paulo Research Foundation (FAPESP 14/50889-7). The CB laboratory is part of the Wellcome Trust Centre for Cell-Matrix Research, University of Manchester, supported by core funding from the Wellcome Trust (grant number 203128/Z/16/Z). CB is supported by grants from Biotechnology and Biological Sciences Research Council (BBSRC; BB/P000681/1, BB/V016326/1). AM is supported by grants from Sao Paulo Research Foundation (FAPESP 23/03079-9) and National Council for Scientific and Technological Development (CNPq 407911/2021-9).
Acknowledgments
The authors want to thank Devina Misha Jethwa for the initial mathematical analysis and Professor Alexandre Ramos for the discussions regarding the development and application of the stochastic model.
Conflict of interest
The authors declare that the research was conducted in the absence of any commercial or financial relationships that could be construed as a potential conflict of interest.
Generative AI statement
The author(s) declare that Gen AI was used in the creation of this manuscript. Generative AI was used to review the English grammar.
Publisher’s note
All claims expressed in this article are solely those of the authors and do not necessarily represent those of their affiliated organizations, or those of the publisher, the editors and the reviewers. Any product that may be evaluated in this article, or claim that may be made by its manufacturer, is not guaranteed or endorsed by the publisher.
Supplementary material
The Supplementary Material for this article can be found online at: https://www.frontiersin.org/articles/10.3389/fmolb.2025.1587608/full#supplementary-material
References
Alexander, A. M., and Lawley, S. D. (2022). Inferences from frap data are model dependent: a subdiffusive analysis. Biophysical J. 121, 3795–3810. doi:10.1016/j.bpj.2022.09.015
Anthis, N. J., Wegener, K. L., Ye, F., Kim, C., Goult, B. T., Lowe, E. D., et al. (2009). The structure of an integrin/talin complex reveals the basis of inside-out signal transduction. EMBO J. 28, 3623–3632. doi:10.1038/emboj.2009.287
Atherton, P., Stutchbury, B., Jethwa, D., and Ballestrem, C. (2016). Mechanosensitive components of integrin adhesions: role of vinculin. Exp. Cell. Res. 343, 21–27. doi:10.1016/j.yexcr.2015.11.017
Atherton, P., Stutchbury, B., Wang, D.-Y., Jethwa, D., Tsang, R., Meiler-Rodriguez, E., et al. (2015). Vinculin controls talin engagement with the actomyosin machinery. Nat. Commun. 6, 10038. doi:10.1038/ncomms10038
Axelrod, D., Koppel, D., Schlessinger, J., Elson, E., and Webb, W. W. (1976). Mobility measurement by analysis of fluorescence photobleaching recovery kinetics. Biophysical J. 16, 1055–1069. doi:10.1016/S0006-3495(76)85755-4
Bläßle, A., Soh, G., Braun, T., Mörsdorf, D., Preiß, H., Jordan, B. M., et al. (2018). Quantitative diffusion measurements using the open-source software pyfrap. Nat. Commun. 9, 1582. doi:10.1038/s41467-018-03975-6
Blumenthal, D., Goldstien, L., Edidin, M., and Gheber, L. A. (2015). Universal approach to frap analysis of arbitrary bleaching patterns. Biophysical J. 108, 77a. doi:10.1016/j.bpj.2014.11.455
Borra, S., and Di Ciaccio, A. (2010). Measuring the prediction error. a comparison of cross-validation, bootstrap and covariance penalty methods. Comput. statistics and data analysis 54, 2976–2989. doi:10.1016/j.csda.2010.03.004
Braeckmans, K., Peeters, L., Sanders, N. N., De Smedt, S. C., and Demeester, J. (2003). Three-dimensional fluorescence recovery after photobleaching with the confocal scanning laser microscope. Biophysical J. 85, 2240–2252. doi:10.1016/S0006-3495(03)74649-9
Braga, J., Desterro, J. M., and Carmo-Fonseca, M. (2004). Intracellular macromolecular mobility measured by fluorescence recovery after photobleaching with confocal laser scanning microscopes. Mol. Biol. Cell. 15, 4749–4760. doi:10.1091/mbc.e04-06-0496
Calderwood, D. A., Zent, R., Grant, R., Rees, D. J. G., Hynes, R. O., and Ginsberg, M. H. (1999). The talin head domain binds to integrin β subunit cytoplasmic tails and regulates integrin activation. J. Biol. Chem. 274, 28071–28074. doi:10.1074/jbc.274.40.28071
Carisey, A., Tsang, R., Greiner, A. M., Nijenhuis, N., Heath, N., Nazgiewicz, A., et al. (2013). Vinculin regulates the recruitment and release of core focal adhesion proteins in a force-dependent manner. Curr. Biol. 23, 271–281. doi:10.1016/j.cub.2013.01.009
Carnell, M., Macmillan, A., and Whan, R. (2015). Fluorescence recovery after photobleaching (frap): acquisition, analysis, and applications. Methods Membr. lipids 1232, 255–271. doi:10.1007/978-1-4939-1752-5_18
Carrero, G., McDonald, D., Crawford, E., de Vries, G., and Hendzel, M. J. (2003). Using frap and mathematical modeling to determine the in vivo kinetics of nuclear proteins. Methods 29, 14–28. doi:10.1016/s1046-2023(02)00288-8
Chorev, D. S., Volberg, T., Livne, A., Eisenstein, M., Martins, B., Kam, Z., et al. (2018). Conformational states during vinculin unlocking differentially regulate focal adhesion properties. Sci. Rep. 8, 2693. doi:10.1038/s41598-018-21006-8
Critchley, D. R. (2009). Biochemical and structural properties of the integrin-associated cytoskeletal protein talin. Annu. Rev. biophysics 38, 235–254. doi:10.1146/annurev.biophys.050708.133744
Dallon, J., Leduc, C., Grant, C. P., Evans, E. J., Etienne-Manneville, S., and Portet, S. (2022). Using fluorescence recovery after photobleaching data to uncover filament dynamics. PLoS Comput. Biol. 18, e1010573. doi:10.1371/journal.pcbi.1010573
Day, C. A., Kraft, L. J., Kang, M., and Kenworthy, A. K. (2012). Analysis of protein and lipid dynamics using confocal fluorescence recovery after photobleaching (frap). Curr. Protoc. Cytom. 62, Unit2.19–19. doi:10.1002/0471142956.cy0219s62
Dunn, G., Dobbie, I., Monypenny, J., Holt, M., and Zicha, D. (2002). Fluorescence localization after photobleaching (flap): a new method for studying protein dynamics in living cells. J. Microsc. 205, 109–112. doi:10.1046/j.0022-2720.2001.001007.x
Ellenberg, J., Siggia, E. D., Moreira, J. E., Smith, C. L., Presley, J. F., Worman, H. J., et al. (1997). Nuclear membrane dynamics and reassembly in living cells: targeting of an inner nuclear membrane protein in interphase and mitosis. J. Cell. Biol. 138, 1193–1206. doi:10.1083/jcb.138.6.1193
Elowitz, M. B., Levine, A. J., Siggia, E. D., and Swain, P. S. (2002). Stochastic gene expression in a single cell. Science 297, 1183–1186. doi:10.1126/science.1070919
Geverts, B., van Royen, M. E., and Houtsmuller, A. B. (2015). Analysis of biomolecular dynamics by frap and computer simulation. Adv. Fluoresc. Microsc. Methods Protoc. 1251, 109–133. doi:10.1007/978-1-4939-2080-8_7
Giakoumakis, N. N., Rapsomaniki, M. A., and Lygerou, Z. (2017). Analysis of protein kinetics using fluorescence recovery after photobleaching (frap). Light Microsc. Methods Protoc. 1563, 243–267. doi:10.1007/978-1-4939-6810-7_16
Gillespie, D. T. (1977). Exact stochastic simulation of coupled chemical reactions. J. Phys. Chem. 81, 2340–2361. doi:10.1021/j100540a008
Gingras, A. R., Ziegler, W. H., Bobkov, A. A., Joyce, M. G., Fasci, D., Himmel, M., et al. (2009). Structural determinants of integrin binding to the talin rod. J. Biol. Chem. 284, 8866–8876. doi:10.1074/jbc.M805937200
Groeneweg, F. L., van Royen, M. E., Fenz, S., Keizer, V. I., Geverts, B., Prins, J., et al. (2014). Quantitation of glucocorticoid receptor dna-binding dynamics by single-molecule microscopy and frap. PloS one 9, e90532. doi:10.1371/journal.pone.0090532
Hickey, S. M., Ung, B., Bader, C., Brooks, R., Lazniewska, J., Johnson, I. R., et al. (2021). Fluorescence microscopy—an outline of hardware, biological handling, and fluorophore considerations. Cells 11, 35. doi:10.3390/cells11010035
Houtsmuller, A. B., Rademakers, S., Nigg, A. L., Hoogstraten, D., and Vermeulen, W. (1999). Action of dna repair endonuclease ercc1/xpf in living cells. Science 284, 958–961. doi:10.1126/science.284.5416.958
Ishikawa-Ankerhold, H. C., Ankerhold, R., and Drummen, G. P. (2012). Advanced fluorescence microscopy techniques—frap, flip, flap, fret and flim. Molecules 17, 4047–4132. doi:10.3390/molecules17044047
Jansen, K., Atherton, P., and Ballestrem, C. (2017). Mechanotransduction at the cell-matrix interface. Seminars Cell. and Dev. Biol. 71, 75–83. doi:10.1016/j.semcdb.2017.07.027
Kanchanawong, P., Shtengel, G., Pasapera, A. M., Ramko, E. B., Davidson, M. W., Hess, H. F., et al. (2010). Nanoscale architecture of integrin-based cell adhesions. Nature 468, 580–584. doi:10.1038/nature09621
Kang, M. (2020). Diffusion theory for cell membrane fluorescence microscopy. Fluoresc. Methods Investigation Living Cells Microorg., 397. doi:10.5772/intechopen.91845
Kang, M., Day, C. A., Drake, K., Kenworthy, A. K., and DiBenedetto, E. (2009). A generalization of theory for two-dimensional fluorescence recovery after photobleaching applicable to confocal laser scanning microscopes. Biophysical J. 97, 1501–1511. doi:10.1016/j.bpj.2009.06.017
Lewis, C. (1982). Industrial and business forecasting methods: a practical guide to exponential smoothing and curve fitting. Butterworth scientific (Butterworth Scientific.
Liang, J., and Qian, H. (2010). Computational cellular dynamics based on the chemical master equation: a challenge for understanding complexity. J. Comput. Sci. Technol. 25, 154–168. doi:10.1007/s11390-010-9312-6
Lippincott-Schwartz, J., and Patterson, G. H. (2008). Fluorescent proteins for photoactivation experiments. Methods Cell. Biol. 85, 45–61. doi:10.1016/S0091-679X(08)85003-0
Lippincott-Schwartz, J., Snapp, E., and Kenworthy, A. (2001). Studying protein dynamics in living cells. Nat. Rev. Mol. Cell. Biol. 2, 444–456. doi:10.1038/35073068
Lippincott-Schwartz, J., Snapp, E. L., and Phair, R. D. (2018). The development and enhancement of frap as a key tool for investigating protein dynamics. Biophysical J. 115, 1146–1155. doi:10.1016/j.bpj.2018.08.007
Liu, J., Wang, Y., Goh, W. I., Goh, H., Baird, M. A., Ruehland, S., et al. (2015). Talin determines the nanoscale architecture of focal adhesions. Proc. Natl. Acad. Sci. 112, E4864–E4873. doi:10.1073/pnas.1512025112
Lorén, N., Hagman, J., Jonasson, J. K., Deschout, H., Bernin, D., Cella-Zanacchi, F., et al. (2015). Fluorescence recovery after photobleaching in material and life sciences: putting theory into practice. Q. Rev. biophysics 48, 323–387. doi:10.1017/s0033583515000013
Mai, J., Trump, S., Ali, R., Schiltz, R. L., Hager, G., Hanke, T., et al. (2011). Are assumptions about the model type necessary in reaction-diffusion modeling? a frap application. Biophysical J. 100, 1178–1188. doi:10.1016/j.bpj.2011.01.041
Mazza, D., Braeckmans, K., Cella, F., Testa, I., Vercauteren, D., Demeester, J., et al. (2008). A new frap/frapa method for three-dimensional diffusion measurements based on multiphoton excitation microscopy. Biophysical J. 95, 3457–3469. doi:10.1529/biophysj.108.133637
Moraru, I. I., Schaff, J. C., Slepchenko, B. M., Blinov, M., Morgan, F., Lakshminarayana, A., et al. (2008). Virtual cell modelling and simulation software environment. IET Syst. Biol. 2, 352–362. doi:10.1049/iet-syb:20080102
Mueller, F., Wach, P., and McNally, J. G. (2008). Evidence for a common mode of transcription factor interaction with chromatin as revealed by improved quantitative fluorescence recovery after photobleaching. Biophysical J. 94, 3323–3339. doi:10.1529/biophysj.107.123182
Muñoz-Cobo, J.-L., and Berna, C. (2019). Chemical kinetics roots and methods to obtain the probability distribution function evolution of reactants and products in chemical networks governed by a master equation. Entropy 21, 181. doi:10.3390/e21020181
Nicolau, D. V., Hancock, J. F., and Burrage, K. (2007). Sources of anomalous diffusion on cell membranes: a Monte Carlo study. Biophysical J. 92, 1975–1987. doi:10.1529/biophysj.105.076869
Phair, R. D., Gorski, S. A., and Misteli, T. (2003). Measurement of dynamic protein binding to chromatin in vivo, using photobleaching microscopy. Methods Enzym. (Elsevier) 375, 393–414. doi:10.1016/s0076-6879(03)75025-3
Phair, R. D., and Misteli, T. (2001). Kinetic modelling approaches to in vivo imaging. Nat. Rev. Mol. Cell. Biol. 2, 898–907. doi:10.1038/35103000
Qian, H., and Bishop, L. M. (2010). The chemical master equation approach to nonequilibrium steady-state of open biochemical systems: linear single-molecule enzyme kinetics and nonlinear biochemical reaction networks. Int. J. Mol. Sci. 11, 3472–3500. doi:10.3390/ijms11093472
Riznichenko, G. Y., and Rubin, A. (2021). Mathematical modeling in biology: Part 2. models of protein interaction processes in a photosynthetic membrane. Biol. Bull. Rev. 11, 110–121. doi:10.1134/s2079086421020080
Röding, M., Lacroix, L., Krona, A., Gebäck, T., and Lorén, N. (2019). A highly accurate pixel-based frap model based on spectral-domain numerical methods. Biophysical J. 116, 1348–1361. doi:10.1016/j.bpj.2019.02.023
Ruoslahti, E. (1991). “Integrins as receptors for extracellular matrix,” in Cell biology of extracellular matrix. Second Edition (Springer), 343–363.
Sprague, B. L., and McNally, J. G. (2005). Frap analysis of binding: proper and fitting. Trends Cell. Biol. 15, 84–91. doi:10.1016/j.tcb.2004.12.001
Sprague, B. L., Pego, R. L., Stavreva, D. A., and McNally, J. G. (2004). Analysis of binding reactions by fluorescence recovery after photobleaching. Biophysical J. 86, 3473–3495. doi:10.1529/biophysj.103.026765
Stutchbury, B., Atherton, P., Tsang, R., Wang, D.-Y., and Ballestrem, C. (2017). Distinct focal adhesion protein modules control different aspects of mechanotransduction. J. Cell. Sci. 130, 1612–1624. doi:10.1242/jcs.195362
Toral, R., and Colet, P. (2014). Stochastic numerical methods: an introduction for students and scientists. John Wiley and Sons, 235–238.
Vilaseca, E., Pastor, I., Isvoran, A., Madurga, S., Garcés, J.-L., and Mas, F. (2011). Diffusion in macromolecular crowded media: Monte Carlo simulation of obstructed diffusion vs. frap experiments. Theor. Chem. Accounts 128, 795–805. doi:10.1007/s00214-010-0840-5
Wang, J.-h. (2012). Pull and push: talin activation for integrin signaling. Cell. Res. 22, 1512–1514. doi:10.1038/cr.2012.103
Watanabe, N., and Mitchison, T. J. (2002). Single-molecule speckle analysis of actin filament turnover in lamellipodia. Science 295, 1083–1086. doi:10.1126/science.1067470
Williamson, D. E., Sahai, E., Jenkins, R. P., O’Dea, R. D., and King, J. R. (2021). Parameter estimation in fluorescence recovery after photobleaching: quantitative analysis of protein binding reactions and diffusion. J. Math. Biol. 83 (1), 1. doi:10.1007/s00285-021-01616-z
Yamashiro, S., Rutkowski, D. M., Lynch, K. A., Liu, Y., Vavylonis, D., and Watanabe, N. (2023). Force transmission by retrograde actin flow-induced dynamic molecular stretching of talin. Nat. Commun. 14, 8468. doi:10.1038/s41467-023-44018-z
Yao, M., Goult, B. T., Sheetz, M. P., and Yan, J. (2016). The mechanical properties of talin rod domain. Biophysical J. 110, 620a–621a. doi:10.1016/j.bpj.2015.11.3329
Zaidel-Bar, R., Ballestrem, C., Kam, Z., and Geiger, B. (2003). Early molecular events in the assembly of matrix adhesions at the leading edge of migrating cells. J. Cell. Sci. 116, 4605–4613. doi:10.1242/jcs.00792
Zhang, X., Jiang, G., Cai, Y., Monkley, S. J., Critchley, D. R., and Sheetz, M. P. (2008). Talin depletion reveals independence of initial cell spreading from integrin activation and traction. Nat. Cell. Biol. 10, 1062–1068. doi:10.1038/ncb1765
Keywords: chemical master equation, focal adhesion, FRAP, FLAP, protein dynamics, protein interaction
Citation: de Oliveira LR, Fernandes MG, Patane JSL, Schwartz J-M, Krieger JE, Ballestrem C and Miyakawa AA (2025) Exploring focal adhesion data: dynamic parameter extraction from FRAP and FLAP experiments using chemical master equation. Front. Mol. Biosci. 12:1587608. doi: 10.3389/fmolb.2025.1587608
Received: 04 March 2025; Accepted: 18 April 2025;
Published: 06 May 2025.
Edited by:
Li-Tang Yan, Tsinghua University, ChinaReviewed by:
Ze Gong, University of Science and Technology of China, ChinaSawako Yamashiro, Kyoto University, Japan
Copyright © 2025 de Oliveira, Fernandes, Patane, Schwartz, Krieger, Ballestrem and Miyakawa. This is an open-access article distributed under the terms of the Creative Commons Attribution License (CC BY). The use, distribution or reproduction in other forums is permitted, provided the original author(s) and the copyright owner(s) are credited and that the original publication in this journal is cited, in accordance with accepted academic practice. No use, distribution or reproduction is permitted which does not comply with these terms.
*Correspondence: Luciana Renata de Oliveira, bHVjaWFuYXJlbmF0YWRlb2xpdmVpcmFAZ21haWwuY29t; Ayumi Aurea Miyakawa, YXl1bWkubWl5YWthd2FAaW5jb3IudXNwLmJy
†These authors have contributed equally to this work and share first authorship
‡These authors have contributed equally to this work and share senior authorship