- 1Associação CCG/zgdv—Centro de Computação Gráfica, Universidade Do Minho—Campus de Azurém, Guimarães, Portugal
- 2Centre of Technology and Systems (UNINOVA-CTS), FCT Campus, Caparica, Portugal
- 3Chair of IT Security, Brandenburg University of Technology Cottbus-Senftenberg, Cottbus, Germany
- 4Deutsches Institut für Normung e. V, Berlin, Germany
- 5SIRIUS, Department of Informatics, University of Oslo, Oslo, Norway
- 6CIGIP—Centro de Investigación Gestión e Ingenería de Producción, Ciudad Politécnica de La Innovación, Valencia, Spain
- 7EDEM—Escuela de Empresarios, La Marina de València, Stuttgart, Germany
- 8Fraunhofer Institute for Manufacturing Engineering and Automation IPA, Stuttgart, Germany
Zero-Defect Manufacturing (ZDM) is the next evolutionary step in quality management for manufacturing that makes use of Industry 4.0 technologies to support quality in manufacturing. These technologies help reduce the cost of inspection, allowing for more inspection points throughout the manufacturing process, reducing the size of quality feedback loops, and guaranteeing that no defective product is delivered to the customer. There are several ZDM-related initiatives, but still no harmonized terminology. This article describes the methodological approach to provide a common agreement on the ZDM concept and its associated terminology taking place within an open CEN-CENELEC Workshop. The methodology has the support of ISO standards for terminology work such as ISO 704, ISO 860, and ISO 10241–1/2. This work shows that the terminology for ZDM has a significant overlap with the terminology of quality management, metrology, dependability, statistics, non-destructive inspection, and condition monitoring. The proposed new terms and definitions can be used to further extend ISO’s and IEC’s already available terminologies and support present and future researchers in the field to conduct their research using a common vocabulary.
1 Introduction
With the introduction of the Industry 4.0 concept and the requirement for greater industrial sustainability, the modern manufacturing arena is undergoing drastic changes. As a result, manufacturers are always striving for excellence, which is one of their key strategic objectives. One of the most important sectors in the industrial domain is quality control and assurance, which necessitates training. This field is undergoing significant transformations with the introduction of numerous new technologies and techniques that have surpassed the capabilities of existing methodologies and technologies. Quality control and assurance are critical components of all production systems, whether continuous or discrete. High-quality production is something that manufacturers strive for and invest a large amount of money to attain, yet they do not always succeed. Quality refers not just to product quality but also to process and design quality (Psarommatis et al., 2020a; Psarommatis et al., 2022b; Psarommatis, 2021). Production quality is inextricably linked to manufacturing sustainability, which is a crucial business goal for manufacturers. Operating with low quality has a variety of negative consequences, ranging from direct financial losses to indirect consequences such as a bad influence on the company’s reputation (Jun et al., 2020).
Companies use a variety of quality management methods to improve their operational performance to attain high-quality production (Kumar et al., 2018). The application of Industry 4.0 technological advances along the production process chain has led to a fundamental change in manufacturing quality management systems (Sousa et al., 2021, 2020). These advances make it possible to achieve unprecedented levels of manufacturing quality and to produce high-quality products most efficiently, i.e., to realize the paradigm of zero-defect production (Psarommatis and Bravos, 2022). This is the main goal of an increasing number of research articles, funded research projects, and technical solutions addressing a variety of technologies such as industrial IoT, non-destructive testing, automation, big data, deep learning, or artificial intelligence in manufacturing quality management areas (Psarommatis et al., 2022a). Zero-Defect Manufacturing (ZDM) (Psarommatis et al., 2020a; Psarommatis et al., 2022b; Psarommatis, 2021) is the most recent technique for quality assurance. ZDM, in the era of Industry 4.0, is a domain that is fairly new with the first articles after the introduction of Industry 4.0 explicitly mentioning ZDM in 2013 (Di Foggia and D’Addona, 2013; Ferretti et al., 2013; Myklebust, 2013) and focusing on specific applications.
Traditional quality improvement (QI) methods such as lean manufacturing, Six Sigma, Lean Six Sigma, Theory of Constraints, and total quality management have been utilized in the manufacturing domain for more than 3 decades. Although the traditional QI methods are improving quality, they have reached their full potential and cannot cope with the current needs for quality and sustainability (Psarommatis et al., 2022b). A recent review article on product quality analyzed all the traditional QI methods from 1998 to 2019 and identified the disadvantages of traditional QI compared to ZDM (Psarommatis et al., 2020b). The main disadvantage of those methods is the fact that they do not learn from their defects, they aim to remove them. Additionally, traditional QI methods analyze the past to improve the present ignoring the future, which leads to valuable information loss. Furthermore, traditional QI does not utilize the full potential of Industry 4.0 technologies, which demonstrates that ZDM constitutes a viable replacement for traditional QI methods (Psarommatis et al., 2022b).
In Eger et al., 2018, authors allocated some significant limitations of the current ZDM approaches, namely those that narrowly focused on specific production stages without considering how different stages might affect each other. Additionally, Psarommatis, 2021 presented a methodology for designing a manufacturing system for achieving ZDM and stressed the fact that both the design of the product and the production system are critical for ZDM, and new design methodologies are required. In other words, even if the ZDM effect will be achieved for a certain production stage, it does not automatically mean that the whole production process can be considered ZDM-compliant. Moreover, considering cyber-physical systems as one of the backbones of digital manufacturing, one of the ZDM goals is to minimize or eliminate the waste and scraped output, while in CPS, both the physical waste and the digital waste can affect the system.
Technological advancements are happening very quickly and there is no standard terminology to promote a common understanding and positioning of these initiatives concerning quality management systems to date (Psarommatis et al., 2022b). Standardization is critical for the success of a quality management system (Psarommatis et al., 2022b) and the lack of standardization and implementation framework was identified as one of the 10 most common failure factors of a quality management system (Albliwi et al., 2014; Psarommatis et al., 2020b). Given the constant technological evolution in areas such as real-time data analytics, big data, data mining, digital twins, Internet-of-Things (IoT), CPS, etc., and the ongoing fourth industrial revolution, the definition, and the concept itself, should be reformulated and updated (Psarommatis et al., 2022a). Research on the topics of standardization, integration, and interoperability is also being conducted to achieve ZDM. Considering the importance of the RAMI 4.0 reference model for Industry 4.0, and in particular for the ZDM domain, the authors (Nazarenko et al., 2021) provided the relevant standards alignment to the RAMI 4.0 layers, namely the asset, integration, communication, information, and functions. In Sousa et al., 2022, the authors particularly analyzed the support of the ISO 23952 standards (Quality Information Framework) in the domain of metrology, IEC 62264 in the domain of Quality Operations Management (QOM), and IEC 62541 (OPC UA) to support the vertical integration for metrology devices and metrology information for ZDM. In Psarommatis et al., 2020a, the authors pointed out the lack of well-defined and unambiguous definitions of the concepts related to the ZDM domain and they set the foundation for ZDM by defining some of the fundamental concepts and definitions. There are a few initial attempts for developing an ontology for application in the ZDM domain, but they do not focus on the description of the entire ZDM domain, instead they focus on specific applications that are related to the ZDM domain (Grevenitis et al., 2019; Ameri et al., 2022; Psarommatis and Kiritsis, 2022).
It is evident from the ZDM literature that there is a lack of common terminology which is negatively affecting both the scientific and industrial domains due to miscommunication. The first study that set the foundations of ZDM was done in 2020 by Psarommatis et al, (2020a) where the authors stated the need for common terminology. Furthermore, literature facts also indicate that the transition from classical QI to ZDM has already begun (Psarommatis et al., 2022b). Additionally, because there is no standardized vocabulary and most researchers and manufacturers have used traditional QI methods for decades, they are reluctant to switch to ZDM. Therefore, the current article aims to present the methodology used in an open CEN-CENELEC standardization working group called CEN-CENELEC Workshop focusing on the definition of the ZDM terminology. More specifically, the current article aims to establish a terminological basis related to digital manufacturing in the context of Industry 4.0, encompassing not only the ZDM concept but also its related terms and definitions, and to align them with other quality management initiatives which ultimately will help in the adoption of ZDM and the realization of a zero-defects era.
This article is organized as follows. Section 2 presents some fundamental ZDM background information, and Section 3 is devoted to presenting the methodology that was used to create and define the ZDM terminology. Section 4 presents some proposed ZDM-related terms and provides a discussion around them. Finally, Section 5 summarizes the achievements of the article and provides some future directions for ZDM evolution and adoption.
1.1 ZDM background information
The history of Zero-Defects Manufacturing (ZDM) goes back to the 1960s when the concept was used as a quality and reliability program by the United States Department of Defense (Office of the Assistant Secretary of Defense, 1965). At that time, the ZDM concept was rather focused on a very concrete task of eliminating defects caused by human errors. Zero-defect programs became popular in the 1960s as motivational programs by Philip Crosby, as the ever-increasing and novel demand for integrated defect reduction strategies in industrial production. His program, whose principles were: a) do it right the first time; b) the system of quality is prevention; c) the performance standard is “zero defect”; and d) the measurement of quality is the price of non-conformance, aimed at defect-free production without any scrap or rework (Crosby, 1980). To this end, for the required defect-free products after each step of the process, ZDM should therefore be mandatory throughout the industry. The impact of zero-defect programs was insufficient, and several researchers pointed out that the programs did not achieve their goals (Ghinato, 1998). Later in the late 1980s, the ZDM concept was generalized as a part of the Total Quality Management philosophy and was defined as a process to minimize or get rid of the defects in the output of various production processes, covering all aspects of the process. One commonly used approach that can be considered as the predecessor of zero-defects manufacturing is the Six Sigma technique which uses the standard deviation of the product/service parameters from the mean value. The product or process is in the Six Sigma range if 99.7% of the products/services are defect-free. Thus, the ZDM is practically not achievable under the Six Sigma approach as still a 0.3% defect rate is acceptable. According to Squeglia, 2008, many companies are attempting to reach zero defects through statistical process control, improved processes, closed-loop inspection systems, and other means. A zero-defect manufacturing process is a process with an acceptance criterion of zero defects. The only way of assuring 100% conforming products ( defect-free) is to inspect every quality characteristic using 100% inspection. Since it is impractical to perform 100% inspection, sampling plans are used to achieve near-zero defects. To achieve 100% inspection, automated means for inspection and testing are required such as machine-vision systems. 100% inspection can also be deployed to satisfy legal or political requirements, or it can be the most cost-effective solution. It can also be used in case the process capability is intrinsically low to meet the requirements (Juran and Godfrey, 1999). Regarding quality costs, Juran (Juran and Gryna, 1993) has extensively written this topic stating that the costs of quality comprise the costs associated with finding, repairing, and/or preventing defects (Figure 1).
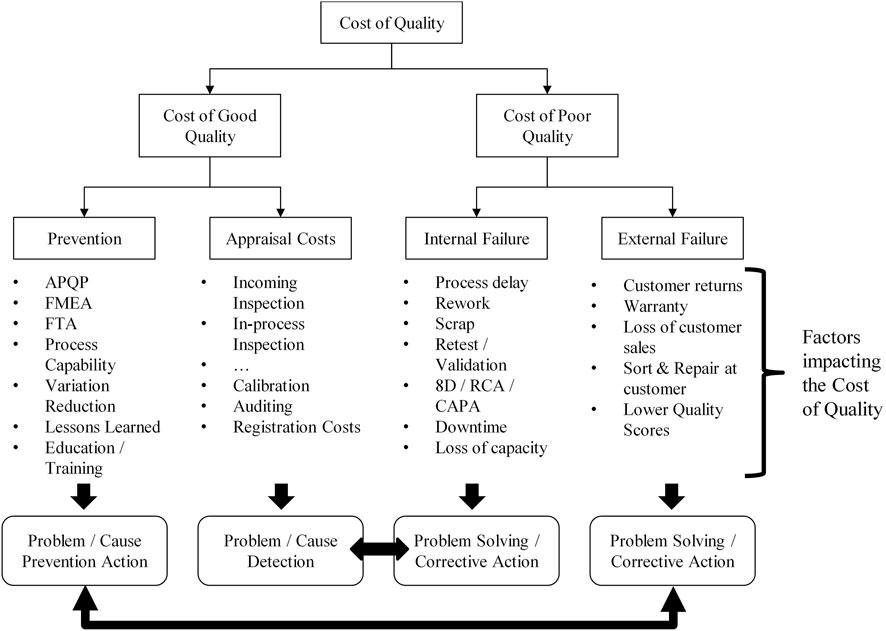
FIGURE 1. The cost of quality according to Juran [adapted from (Kiran, 2017)].
Psarommatis et al., 2020a conducted a review analysis from 1987 to 2019 on the topic of quality management focusing on ZDM and creating a modern version of ZDM. Pillar quality standards such as ISO 9001 and IATF 16949 greatly focus on the need for defect prevention and the application of preventive and corrective actions as the path to quality products and services (ISO, 2015a; IATF, 2016). This focus leads to the application of prevention methods such as mistake-proofing (poka-yoke) (Shingō, 1986; AIAG, 2008; ISO, 2011a). Furthermore, Psarommatis et al., 2022a; Psarommatis et al., 2022b did a direct comparison of ZDM with traditional QI approaches and presented some facts supporting the superiority of ZDM over the traditional QI methods.
The terminology of ZDM is strongly connected to quality management which has a significant number of standards and guidelines, where a broader approach addressing quality improvement in manufacturing and its related processes is defined. The area of ZDM emerged as a natural aim of the manufacturers to reduce or eliminate all defects occurring during the manufacturing process due to the costs that the defective products cause. In general, the concept of ZDM is closely coupled with other related concepts, such as zero waste, zero emissions, zero accidents, and zero faults aiming at achieving the ultimate quality, but also in addressing social and environmental sustainability dimensions, in addition to the economic one. Further advantages of ZDM can be the lower costs of production, lower energy consumption, less scraped output, and material waste.
Considering the importance of the transformations needed during Industry 4.0, a set of European initiatives that contribute to the establishment of ZDM basis can be named, namely: GO0DMAN, ZAero, DAT4. zero, Qu4lity, ForZDM, and ZDMP (Psarommatis et al., 2022b). The GO0DMAN European project (Peres et al., 2018), demonstrates quality-improving solutions contributing to ZDM based on a multi-agent approach and boosted with smart inspection tools, data analysis, and knowledge management. One of the project goals was to demonstrate how important Cyber-Physical Systems (CPS) are for ZDM in the manufacturing context (Chiariotti, 2018). The ZAero project specifically targets the ZDM aspects of composite parts in the aerospace industry (Zörrer et al., 2019). The goal of ForZDM is the development and demonstration of ZDM strategies for production control of multi-stage manufacturing systems including a generic architecture addressing various ZDM aspects, such as centralized data acquisition, data analysis using statistical methods, and artificial intelligence (Magnanini et al., 2020). This project also provided a glossary around ZDM developed in its scope that supported the activity of the CEN-CENELEC Workshop. In the case of the ZDMP–Zero Defect Manufacturing Platform, the goal is to develop a ZDM platform enabling the development of both product and process qualities, and enhancing applications with use-cases in various manufacturing domains (Fraile et al., 2019).
2 Applied method for terminology definition
This work describes the methodological approach to Zero-Defect Manufacturing (ZDM), which took place within the CEN-CENELEC Workshop, to provide a common agreement on the ZDM concept and its associated terminology. The result of the workshop is a CEN-CENELEC Workshop Agreement (CWA), a pre-standard with the proposed terminology for the field of ZDM, which will be open for discussion. Several meetings took place with a body of experts considered to be representatives of different and valid views of the domain of ZDM. The formal phases and procedures for this work followed standard ISO 15188:2001 for project management for terminology standardization (ISO, 2001). Additionally, the following standards were taken into consideration: standard ISO 704 (ISO, 2009) which stipulates the principles of terminology work, standard ISO 860 (ISO, 2007) for the harmonization of concepts, concepts systems, terms, and definitions, and standards ISO 10241–1/2 (ISO, 2011b; ISO, 2012a) for the preparation and layout of international terminology standards. Figure 2 shows the adopted methodology for the CWA creation which is divided into three phases.
2.1 Phase 1—Preliminary gathering of terms
The first phase of the process started with the identification and evaluation of documentation, the analysis, and selection of terms from quality management (assurance, control, planning, and improvement) guidelines such as AIAG’s Effective Problem-Solving Guide, Failure Mode and Effects Analysis, Production Part Approval Process (PPAP), and Advanced Product Quality Planning (APQP)—which provide general guidelines for ensuring advanced product quality planning per customer requirements, Statistical Process Control (SPC), Measurement Systems Analysis (MSA), and IATF 16949: 2016, which define the basic requirements for a quality management system for automotive production and corresponding service-part organizations.
The database was completed with terms from articles related to ZDM collected using Scopus and Google Scholar search engines. The preliminary database of relevant terms was reviewed using online standards and terminology search engines, such as ISO’s Online Browsing Platform (OBP)1, IEC’s Electropedia2, and IEC’s Glossary3 to obtain a formal definition for the previously collected terms. In the next step, equivalent terms were gathered and evaluated. In this step, terms from standards dealing with quality management, quality assurance, quality control, quality improvement, metrology, maintenance, and condition monitoring were included such as:
• ISO 10012:2003 for measurement management–which specifies general requirements and provides guidance for the management of measurement processes and metrological confirmation of measuring equipment used to support and demonstrate compliance with metrological requirements;
• ISO 13053–1/2:2011 for quantitative methods in process improvement—Six Sigma—which recommends the preferred or best practice for each of the phases of the DMAIC methodology used in the execution of a Six Sigma project and describes the tools and techniques applicable to each activity area and company size to achieve a competitive advantage;
• ISO 13372: 2012 for condition monitoring and diagnosis of machinery–which defines the terms used in condition monitoring and diagnostics of machines. It is intended to provide users and manufacturers of condition monitoring and diagnostics systems with a common vocabulary;
• ISO 13381–1: 2015 for condition monitoring and diagnosis of machinery which guides the development and application of prognosis processes;
• ISO 22514–1:2014 for statistical methods in process management which describes the fundamental principles of capability and performance of manufacturing processes;
• ISO 3534–2:2006 for statistics—defines the applied statistics terms and expresses them in a conceptual framework per ISO normative terminology practice;
• ISO 9000:2015 for quality management systems—describes the fundamental concepts and principles of quality management which are universally applicable;
• ISO/IEC Guide 99:2007 for the basic and general concepts and associated terms (VIM)—provides a set of definitions and associated terms, for a system of basic and general concepts used in metrology, together with concept diagrams to demonstrate their relations;
• and ISO/TS 18173:2005 for nondestructive testing—defines the general technical terms that are used in many non-destructive testing disciplines.
The IEC 60050 group of standards was also used as a source of relevant terms and definitions during this phase. Examples include:
• IEC 60050–171:2019, which contains the general terminology for digital technology and general terms for specific applications and associated technologies;
• IEC 60050–192:2015 contains the general terminology for the area of dependability. The terms are generic and apply to all areas of reliability methodology, including electrical engineering applications.
• IEC 60050–351:2013, which provides the general terminology for control engineering, as well as generic terms for specific applications and associated technologies,
• and IEC 60050–741:2020, which provides a definition for the Internet of Things and its associated terms and definitions. It has the status of a horizontal standard according to IEC Guide 108:2006.
At the end of phase 1, the database contained about 271 terms related to ZDM.
2.2 Phase 2—The curation of the preliminary list of terms by domain experts
The 2nd phase of the ZDM standardization procedure was to filter the identified terms and remove those terms that were not relevant to ZDM. Furthermore, the terms that have been defined in other standards and used in the current standardization procedure have been removed from the ZDM terminology list and a reference to the original standard has been made. This procedure was necessary to avoid overlapping and duplication of identical terms. To filter the 271 identified terms, a relevance ranking method was used. A ranking scale between 0–7 was used with 7 being highly relevant with ZDM and 0 as not relevant. The ranking process was executed separately by each subject matter expert, who, based on his/her knowledge, assigned a value for each of the 271 terms. When all the CEN-CENELEC Workshop experts finished the individual ranking, the average value of the assigned values for each term was calculated and the identified terms were sorted from highest to lowest value. The next step was to jointly discuss and evaluate on a case-by-case basis, based on the average ranking score, whether each term was relevant to the selected topic or too generic. Based on the discussion from the experts, the generic and non-relevant terms were then removed from the list, leaving only the ZDM-relevant terms. At this point, it should be mentioned again that the goal of phase two was to remove generic or non ZDM-relevant terms from the identified list and not to compare the different terms with each other. Therefore, expert methods such as Analytic Hierarchy Process (Saaty, 1987; Psarommatis and Vosniakos, 2022) are not suitable for the current case. More specifically, AHP focuses on pairwise comparisons and prioritizing alternative solutions. After the ranking process and the experts’ discussion, the 271-term list ended with approximately 75 terms in the maintenance domain, 21 terms in the metrology domain, 38 in the quality control domain, 24 in the quality management system domain, 20 in the quality tools and techniques domain, 73 in the statistics domain, and 30 were considered “generic".
2.3 Phase 3—Global improvement of the definitions and harmonization
The 3rd phase included the adaptation of already available definitions and their harmonization according to ISO 860:2007 (ISO, 2007). The adaptation and harmonization processes took into consideration the referencing of terms already defined in the standards when consensus was gathered around such definitions. The definitions were also improved by the inclusion of examples and notes that could further enhance the understanding, application, and extent of the definition. The creation of concept diagrams, showing the connections between the terms, was also used in this phase.
This phase dealt with different challenges such as adapting concepts so that they can be more specific to ZDM e.g. detection split into physical detection and virtual detection, the creation of the proper definitions such as the “defect propagation” term, and some of the definitions were adopted and/or merged with other concepts such as capability, resulting in, for instance, ZDM equipment capability. The harmonization of overlapping terms such as defect, non-conformity, non-conformance, abnormality, anomality, fault, failure, and error; dealing with synonyms such as non-destructive testing (NDT), non-destructive examination (NDE), non-destructive inspection (NDI), and non-destructive evaluation (NDE); and finally, the establishment of new definitions if needed, such as the predictive action term.
The improvement and harmonization of definitions and terms were supported by a set of scripts based on Natural Language Processing (NLP) to automate repetitive tasks such as detecting key terms in the definitions, identifying relations between terms, and searching results in search engines (e.g. ISO’ Online Browsing Platform—OBP). Note that the number of terms at this stage is significant, so these scripts provided the means to curate the definitions and identify and validate the references between terms more effectively. Figure 3 represents the data-processing pipelines of these scripts.
The authors used a collaborative spreadsheet (i.e., Google Sheets) to define the methodology. The first step is to read the spreadsheet and extract and filter the relevant data columns (using Python Pandas). The result is a (Pandas) data frame with the columns “terms”—containing the terms, “definitions”—including the definition of each term, and “keep”—containing an indicator with the decision of keeping or removing the term.
The first script checked the definition of each term and validated explicit cross-references between the terms in a two-step process over each definition as follows:
• Collect cross-referenced terms: Look for explicit references to other terms in the terminology list.
• Test that explicit cross-references not removed: This check ensures that terms collected in the previous step have the column “keep” to True (that is, those that are not going to be removed).
The script was built using an ad-hoc Python function that was executed for every definition using the Pandas Apply function.
The second script filtered the definitions and the terms, and for each definition, looked for the presence of terms, so that explicit references within definitions are identified. For this purpose, a powerful NLP library was required. In the case of Python, the two most widely used NLP libraries were considered, namely spaCy and NLTK. Both libraries support a wide variety of functions such as text tokenization, part-of-speech tagging, or entity detection. The most important function for the current task was the ability to identify the noun chunks more quickly and reliably. Based on this, both libraries were analyzed and spaCy was selected, along with the English language package, as the lightweight solution for this purpose. The authors however have not performed detailed tests on the performance of both of those libraries concerning the stated task.
The script powered by spaCy consists of two stages. During the first stage, the text of the definition is tokenized, i.e., split into the smallest discrete units of a spoken language with particular meanings–words, to search for and filter some stop words that do not contribute to the extraction of potential and meaningful keywords. A vivid example of stop words is prepositions, such as: “for”, “by”, “since”, “with”, etc. All the definitions are checked, and the stop words found are removed. During the second stage, potential keyword candidates are extracted under the assumption that a keyword is most likely a noun. This is accomplished thanks to the powerful reasoning capabilities of “spaCy”, allowing it to allocate the nouns and noun chunks. One example of the reasoning mechanism follows the logic that the noun is most likely expected after a definite or indefinite article. Based on the set of experiments, we defined that an algorithm could extract most of the nouns and the noun chunks with several exceptions, for instance, the word “costs” in the sentence “internal failure costs … ” was identified as a verb and thus dropped (see Figure 4), but the word “failure” with the accompanying adjective “internal” was extracted. Some adjectives such as “internal” can improve the keyword suggestion process, as there could be, for instance, two categories of costs, namely the “internal” or “external”, affecting the result. Below is the pseudo-code demonstrating the logic of the second script:
INPUT: Spreadsheet, spaCy
FOR each definition in Spreadsheet
SET keywords array as empty
IF definition has “stop words” THEN
Remove “stop words” from definition
IF definition has “noun chunks” THEN
Extract “noun chunks” from definition and append to keywords
RETURN keywords
ENDFOR
The script also processed the keep column to indicate whether all referenced terms are to be kept in the terminology or if any referenced term is to be removed to avoid inconsistencies.
Finally, another script looked for explicit references to external references in the ISO OBP service and scrapped the results to append the definitions. This script uses a Selenium script to automate the search of ISO definitions mentioned in the definition of the term. The script automates the interactions of a human with the ISO OBP web portal, collecting the references to ISO definitions and collecting the definitions available online. The following pseudo-code describes the steps in the script:
TRY:
Get a web session to ISO OBP
EXCEPT Invalid session
TRY:
Wait until the radio button is visible
Select search terms and conditions from the radio button
Enter search to text in the input field
Click search button
Wait until search results are visible
FOR each result in the three first results
Fetch definition and append to definitions
ENDFOR
RETURN definitions
EXCEPT no such element
The search is executed for every definition using the Pandas apply() function, and the results of the search are appended to the collaborative spreadsheet as new columns so that they can be reviewed and feedback can be given on the terminology definition.
3 Discussion around the terminology
3.1 Scope of the terminology
The terminology related to ZDM strongly overlaps with the terminology of quality management, statistics, metrology, dependability, etc. Nonetheless, there are a few concepts that are unique to ZDM. Table 1 presents some examples of definitions proposed during the Workshop. A zero-defect manufacturing system is a system that implements all the necessary means to achieve zero-defect manufacturing. Such a system is comprised of ZDM tools such as software and hardware (e.g., a digital platform) to avoid defect generation and defect propagation. It uses a combination of different forms of inspection, namely, final inspection, in-process inspection, incoming inspection, off-line inspection, at-line inspection, in-line inspection, and on-machine inspection. A ZDM system is also inclusive of alarms and alerts. An alarm is an operational signal or message designed to notify personnel when a selected anomaly, or a logical combination of anomalies, requiring corrective action is encountered [adapted from (ISO, 2012b)]. An alert is an operational signal or warning message designed to notify personnel when a selected anomaly, or a logical combination of anomalies, requiring heightened awareness of preventive action is encountered [adapted from (ISO, 2012b)]. A zero-defect manufacturing framework is a structure of processes and specifications designed to support the accomplishment of ZDM. A zero-defect manufacturing tool is an asset that assists in achieving results or completing tasks related to zero-defect manufacturing. The tool can be a physical entity or a digital entity as per the definition of asset.
3.2 Terminology in the field of defects, failures, and faults
A simple search with ISO’s OBP search engine resulted in more than 50 unique definitions for defect. Although the objective of the CWA was not to harmonize the terminology associated to defect, this somehow shows the heterogeneity in the field. The CEN-CENELEC Workshop participants chose to use the definitions provided by ISO 9000:2015 when they were available, in cases like this, when multiple definitions were available. Other terms related to defects are already defined, mainly in ISO, such as critical defect, surface defect, internal defect, visible defect, defect repair, and manufacturing defect just to name a few. In Sousa et al., 2022, the authors provide a list of candidate terms for the classification of defect type provided by ISO standards. Nevertheless, in the scope of ZDM, there are two terms, to some extent, that may assist in addressing the origin of the defect and its consequence in the manufacturing system: defect generation and defect propagation. Defect generation is the appearance of a defect on a manufactured part. Defect propagation is the transmission of a defect to subsequent manufacturing steps in the process chain. The relation between defect propagation and defect generation with terminology in the field of requirement in ISO 9000:2015 is shown in Figure 5. Both defects share an associative relationship with the defect concept demonstrating that a thematic connection can be established between the concepts by experience (ISO, 2009).
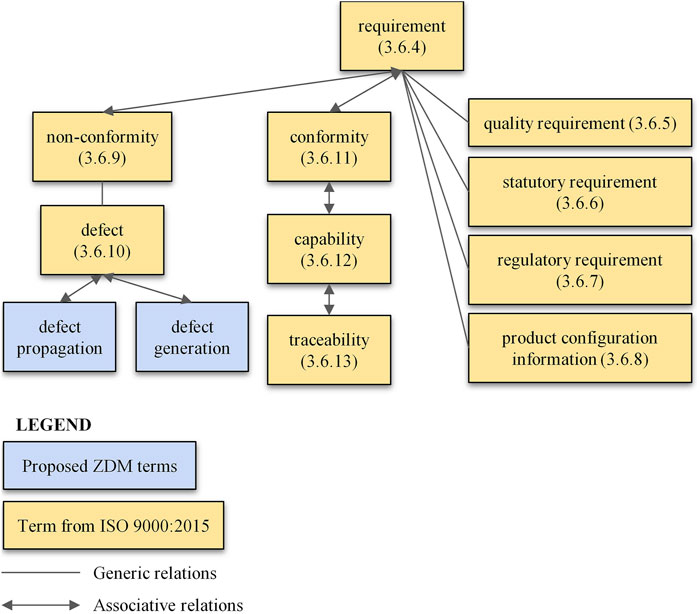
FIGURE 5. Some concepts related to requirement from ISO 9000:2015 and in relation with proposed ZDM term [adapted from (ISO, 2015b)]. Terms collected from ISO 9000:2015 include the entry number in that standard for better reference and traceability.
Switching from the product’s point of view to the process’s (or machine’s) point of view, a failure is the loss of the ability to perform as required and the failure (event) results in a fault of that item. Such a product defect is identified by inspection and testing, and a machine fault is identified through detection—“an event by which the presence of a fault becomes apparent” (IEC, 2015). A fault must also be located (fault localization), diagnosed (fault diagnosis), and corrected (fault correction) (IEC, 2015). The terminology associated with the fault of an item is shown in Figure 6. Associated with the concept of detection, the concepts of physical detection, virtual detection, and hybrid detection can be derived. Physical detection is detection where the data from the physical asset are gathered to identify the presence of a product defect or a process fault/failure. Virtual detection is detection where the process data or any other form of data other than the data coming from a physical asset is gathered to identify the presence of a product defect or process fault/failure.
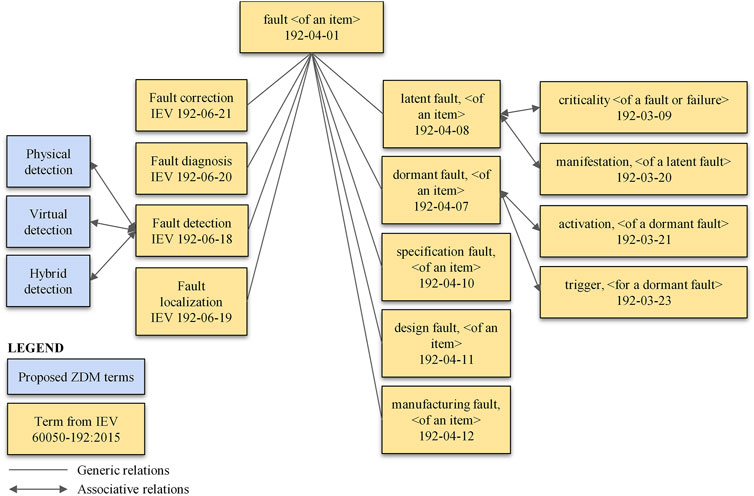
FIGURE 6. Some concepts related to fault in IEV 60500–192:2015 and relation to ZDM terms. Terms collected from the IEV include the entry number in that standard for better reference and traceability.
Associated with the aforementioned is the prediction timeframe concept, which is the timeframe that the prediction method can use to look ahead in time to predict an event (time window) and the predictive time to failure, a measure of the time until a failure event, is predicted through a mathematical method.
3.3 Terminology in the field of inspection
Inspection is defined as the “determination of conformity to specified requirements” by ISO 9000:2015 and can be further categorized as the timing and the location of the inspection. In the scope of smart manufacturing, the inspection can be performed on all received materials before they enter the manufacturing line to be processed–incoming inspection can be performed during the manufacturing cycle and before the completion of all manufacturing processes–in-process inspection, and at the end of the process cycle before shipping to the customer–final inspection. Concerning the location, the inspection can be performed using a measuring device integrated into the manufacturing machine–on-machine inspection, by the operator in a place adjacent to the manufacturing line–at-line inspection, using a measuring device integrated into the production equipment–in-line inspection, or in (typically) a dedicated lab with specialized measuring devices–off-line inspection. Figure 7 shows the proposed terms in the field of inspection and how they relate to some inspection concepts in other standards.
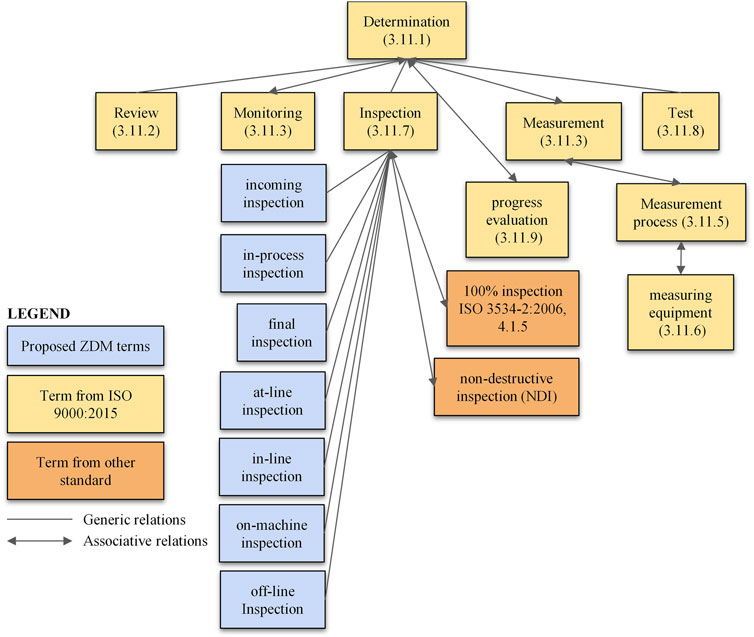
FIGURE 7. Some concepts related to inspection in ISO 9000:2015 and relation to ZDM terms [adapted from (ISO, 2015b)]. Terms collected from ISO 9000:2015 and other standards include the entry number in that standard for better reference and traceability.
3.4 Terminology in the field of actions
Several standards and quality-related guidelines emphasize the appropriate measures to address defects, failures, or problems when encountered during the product’s lifecycle. ISO 9000:2015 and IATF 16494:2016 address preventive action, corrective action, correction, rework, repair and scrap; ISO 9000:2015 also adds regrade and permission terms. ZDM naturally focuses on the same actions with the addition of allowing the introduction of predictive action comprising all the actions applied to forecast the generation of a defect. Figure 8 shows how the concept of predictive action relates to other terms in the field of action. To achieve ZDM, all types of actions are required to ensure coverage for every industrial application and high performance through combinations of different actions.
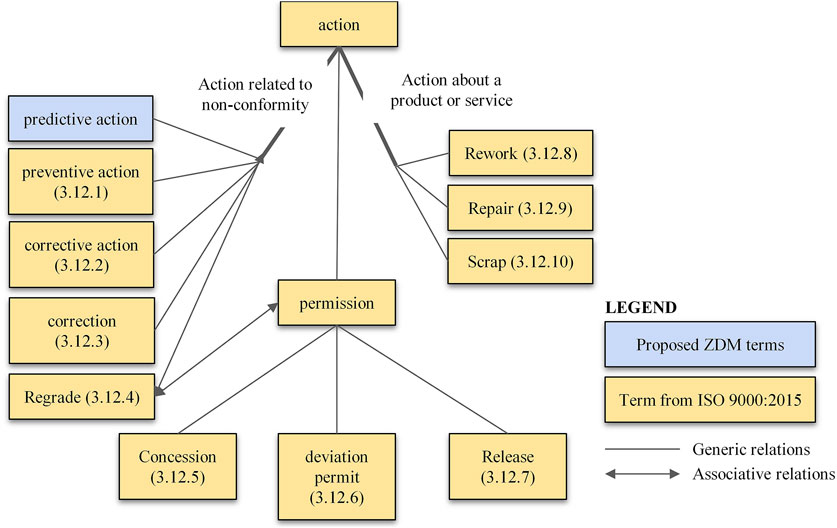
FIGURE 8. Some concepts related to action and the relation to ZDM terms [adapted from (ISO, 2015b)]. Terms collected from ISO 9000:2015 include the entry number in that standard for better reference and traceability.
4 Conclusions and next steps
Zero-defect manufacturing (ZDM) emerges as a new topic for the application of Industry 4.0 technologies to enable the delivery of defect-free products at the highest possible sustainability levels, taking into consideration all four dimensions of sustainability, economy, environment, and society. This article shows the methodological approach to ZDM, to provide a common agreement on the ZDM concept and associated terminology within the CEN-CENELEC Workshop. The construction of terminology is well supported by ISO standards for terminology work such as ISO 704, ISO 860, and ISO 10241–1/2. The applied three-phase methodology showed a significant overlap of the terminology used in ZDM initiatives and articles, with the terminologies of quality management, metrology, dependability, statistics, non-destructive inspection, and condition monitoring. Nonetheless, some terms were proposed as new in the domain, in the sense that a definition was not available in the existing standards, such as in-process inspection and predictive action just to name a few. These proposed concepts can be used to enrich the already available standards in ISO, the IEC’s Electropedia or to be used as a complement document, together with standards such as ISO 9000, IATF 16949, IEC 60050–192, ISO 13372, and ISO 3534 which seem to be the most influential in the domain. The definition of common terminology for the domain of ZDM can help present and future researchers in the field to conduct research with standard terminology.
Data availability statement
The original contributions presented in the study are included in the article/supplementary material; further inquiries can be directed to the corresponding author.
Author contributions
JS has written the article body. AN and FP contributed to the Introduction. FF, AN, and FP contributed to chapter 2. JS, AN, CG, FP, FF, and OM have contributed to the application of the methodology and the discussion around the terminology. CG, AN, FP, and JSa have reviewed the whole article.
Funding
The research leading to these results has received funding from the European Unions Horizon 2020 research and innovation program, mainly project ZDMP under grant agreement 825631, and Eur3ka, TALON, and RE4DY, EU H2020 projects under grant agreements 101016175, 101070181, and 101058384 accordingly.
Acknowledgments
The authors would like to thank the contribution from projects ZDMP, QU4LITY, DigiPrime, the EU, and state Brandenburg EFRE StaF project INSPIRE and the researchers from Universitat Politècnica de València (UPV), Fraunhofer IPA, EPFL, University of Oslo, IKERLAN, UNINOVA, CETECK, Video Systems, Intrasoft, Holonix, Austrian Standards International, the terminology group at DIN, and IEC/TC 65/WG 1 for their valuable inputs and comments during the CWA that contributed to this article.
Conflict of interest
The authors declare that the research was conducted in the absence of any commercial or financial relationships that could be construed as a potential conflict of interest.
Publisher’s note
All claims expressed in this article are solely those of the authors and do not necessarily represent those of their affiliated organizations, or those of the publisher, the editors, and the reviewers. Any product that may be evaluated in this article, or claim that may be made by its manufacturer, is not guaranteed or endorsed by the publisher.
Footnotes
2https://www.electropedia.org/.
3https://std.iec.ch/terms/terms.nsf/.
References
AIAG (2008). Advanced product quality planning (APQP) and control plan: Reference manuabtl. Automotive Industry Action Group (AIAG), 2.
Albliwi, S., Antony, J., Abdul Halim Lim, S., and van der Wiele, T. (2014). Critical failure factors of lean Six Sigma: A systematic literature review. Int. J. Qual. Reliab. Manag. 31, 1012–1030. doi:10.1108/IJQRM-09-2013-0147
Ameri, F., Sormaz, D., Psarommatis, F., and Kiritsis, D. (2022). Industrial ontologies for interoperability in agile and resilient manufacturing. Int. J. Prod. Res. 60, 420–441. doi:10.1080/00207543.2021.1987553
Chiariotti, P. (2018). “Smart measurement systems for zero-defect manufacturing,” in 2018 IEEE 16th International Conference on Industrial Informatics (INDIN), 834–839. doi:10.1109/INDIN.2018.8472016
Di Foggia, M., and D’Addona, D. M. (2013). Identification of critical key parameters and their impact to zero-defect manufacturing in the investment casting process. Procedia CIRP 12, 264–269. doi:10.1016/j.procir.2013.09.046
Eger, F., Coupek, D., Caputo, D., Colledani, M., Penalva, M., Ortiz, J. A., et al. (2018). Zero defect manufacturing strategies for reduction of scrap and inspection effort in multi-stage production systems. Procedia CIRP 67, 368–373. doi:10.1016/j.procir.2017.12.228
Ferretti, S., Caputo, D., Penza, M., and D’Addona, D. M. (2013). Monitoring systems for zero defect manufacturing. Procedia CIRP 12, 258–263. doi:10.1016/j.procir.2013.09.045
Fraile, F., Sanchis, R., Poler, R., and Ortiz, A. (2019). Reference models for digital manufacturing platforms. Appl. Sci. (Basel). 9, 4433. doi:10.3390/app9204433
Ghinato, P. (1998). Quality control methods: Towards modern approaches through well established principles. Total Qual. Manag. 9, 463–477. doi:10.1080/0954412988398
Grevenitis, K., Psarommatis, F., Reina, A., Xu, W., Tourkogiorgis, I., Milenkovic, J., et al. (2019). A hybrid framework for industrial data storage and exploitation. Procedia CIRP 81, 892–897. doi:10.1016/j.procir.2019.03.221
IATF (2016). Iatf 16949:2016 - automotive Quality Management System Standard - quality management system requirements for automotive production and relevant service parts organisation.
IEC (2015). IEC 60050-192:2015 international electrotechnical vocabulary (IEV) - Part 192: Dependability.
ISO (2011a). ISO 13053-2:2011 - quantitative methods in process improvement - Six Sigma - Part 2: Tools and techniques.
ISO (2011b). ISO 10241-1:2011 - terminological entries in standards - Part 1: General requirements and examples of presentation.
ISO (2012a). ISO 10241-2:2012 - terminological entries in standards - Part 2: Adoption of standardized terminological entries.
Jun, J., Chang, T.-W., and Jun, S. (2020). Quality prediction and yield improvement in process manufacturing based on data analytics. Processes 8, 1068. doi:10.3390/pr8091068
Juran, J. M., and Gryna, F. M. (1993). “Quality planning and analysis: From product development through use,” in McGraw-Hill series in industrial engineering and management science (New York, NY: McGraw-Hill).
Kiran, D. R. (2017). Total quality management: Key concepts and case studies. Saint Louis: Elsevier Science.
Kumar, P., Maiti, J., and Gunasekaran, A. (2018). Impact of quality management systems on firm performance. Int. J. Qual. Reliab. Manag. 35, 1034–1059. doi:10.1108/IJQRM-02-2017-0030
Magnanini, M. C., Colledani, M., and Caputo, D. (2020). Reference architecture for the industrial implementation of Zero-Defect Manufacturing strategies. Procedia CIRP 93, 646–651. doi:10.1016/j.procir.2020.05.154
Myklebust, O. (2013). Zero defect manufacturing: A product and plant oriented lifecycle approach. Procedia CIRP 12, 246–251. doi:10.1016/j.procir.2013.09.043
Nazarenko, A. A., Sarraipa, J., Camarinha-Matos, L. M., Grunewald, C., Dorchain, M., and Jardim-Goncalves, R. (2021). Analysis of relevant standards for industrial systems to support zero defects manufacturing process. J. Ind. Inf. Integr. 23, 100214. doi:10.1016/j.jii.2021.100214
Office of the Assistant Secretary of Defense (Installation and Logistics) (1965). A guide to zero defects. Quality and reliability assurance handbook 4155.12-H. Washington DC, Washington, D.C: Assistant Secretary of Defense manpower installations and logistics.
Peres, R., Rocha, A. D., Matos, J. P., and Barata, J. (2018). “GO0DMAN data model,” in Interoperability in Multistage Zero Defect Manufacturing. 2018 IEEE 16th International Conference on Industrial Informatics INDIN. doi:10.1109/indin.2018.8472017
Psarommatis, F., and Bravos, G. (2022). A holistic approach for achieving sustainable manufacturing using zero defect manufacturing: A conceptual framework. Procedia CIRP 107, 107–112. doi:10.1016/j.procir.2022.04.018
Psarommatis, F., and Kiritsis, D. (2022). A hybrid Decision Support System for automating decision making in the event of defects in the era of Zero Defect Manufacturing. J. Ind. Inf. Integr. 26, 100263. doi:10.1016/j.jii.2021.100263
Psarommatis, F., and Vosniakos, G.-C. (2022). Systematic development of a powder deposition system for an open selective laser sintering machine using analytic hierarchy process. J. Manuf. Mat. Process. 6, 22. doi:10.3390/jmmp6010022
Psarommatis, F., May, G., Dreyfus, P.-A., and Kiritsis, D. (2020a). Zero defect manufacturing: State-of-the-art review, shortcomings and future directions in research. Int. J. Prod. Res. 58, 1–17. doi:10.1080/00207543.2019.1605228
Psarommatis, F., Prouvost, S., May, G., and Kiritsis, D. (2020b). Product quality improvement policies in industry 4.0: Characteristics, enabling factors, barriers, and evolution toward zero defect manufacturing. Front. Comput. Sci. 2, 26. doi:10.3389/fcomp.2020.00026
Psarommatis, F., Dreyfus, P. A., and Kiritsis, D. (2022a). “Chapter 9 - the role of big data analytics in the context of modeling design and operation of manufacturing systems,” in Design and operation of production networks for mass personalization in the era of cloud technology. Editor D. Mourtzis (Elsevier), 243–275. doi:10.1016/B978-0-12-823657-4.00012-9
Psarommatis, F., Sousa, J., Mendonça, J. P., and Kiritsis, D. (2022b). Zero-defect manufacturing the approach for higher manufacturing sustainability in the era of industry 4.0: A position paper. Int. J. Prod. Res. 60, 73–91. doi:10.1080/00207543.2021.1987551
Psarommatis, F. (2021). A generic methodology and a digital twin for zero defect manufacturing (ZDM) performance mapping towards design for ZDM. J. Manuf. Syst. 59, 507–521. doi:10.1016/j.jmsy.2021.03.021
Saaty, R. W. (1987). The analytic hierarchy process—What it is and how it is used. Math. Model. 9, 161–176. doi:10.1016/0270-0255(87)90473-8
Shingō, S. (1986). Zero quality control: Source inspection and the poka-yoke system. Stamford, Conn: Productivity Press.
Sousa, J., Ferreira, J., Lopes, C., Sarraipa, J., and Silva, J. (2020). “Enhancing the steel tube manufacturing process with a zero defects approach,” in Volume 2B: Advanced Manufacturing. Presented at the ASME 2020 International Mechanical Engineering Congress and Exposition (American Society of Mechanical Engineers ASME), V02BT02A022. doi:10.1115/IMECE2020-24678
Sousa, J., Nazarenko, A. A., Ferreira, J., Antunes, H., Jesus, E., and Sarraipa, J. (2021). “Zero-Defect Manufacturing using data-driven technologies to support the natural stone industry,” in 2021 IEEE International Conference on Engineering, Technology and Innovation (ICE/ITMC). Presented at the 2021 IEEE International Conference on Engineering, Technology and Innovation (ICE/ITMC) (Cardiff, United Kingdom: IEEE), 1–7. doi:10.1109/ICE/ITMC52061.2021.9570260
Sousa, J., Mendonça, J. P., and Machado, J. (2022). A generic interface and a framework designed for industrial metrology integration for the Internet of Things. Comput. Ind. 138, 103632. doi:10.1016/j.compind.2022.103632
Squeglia, N. L. (2008). Zero acceptance number sampling plans. 5th edn. Milwaukee, Wisconsin: ASQ Quality Press.
Keywords: zero-defect manufacturing (ZDM), terminology, industry 4.0, quality management system, inspection
Citation: Sousa J, Nazarenko A, Grunewald C, Psarommatis F, Fraile F, Meyer O and Sarraipa J (2022) Zero-defect manufacturing terminology standardization: Definition, improvement, and harmonization. Front. Manuf. Technol. 2:947474. doi: 10.3389/fmtec.2022.947474
Received: 18 May 2022; Accepted: 17 November 2022;
Published: 12 December 2022.
Edited by:
Duck Young Kim, Pohang University of Science and Technology, South KoreaReviewed by:
AmirHossein MajidiRad, University of North Florida, United StatesKatarzyna Grobler-Dębska, AGH University of Science and Technology, Poland
Copyright © 2022 Sousa, Nazarenko, Grunewald, Psarommatis, Fraile, Meyer and Sarraipa. This is an open-access article distributed under the terms of the Creative Commons Attribution License (CC BY). The use, distribution or reproduction in other forums is permitted, provided the original author(s) and the copyright owner(s) are credited and that the original publication in this journal is cited, in accordance with accepted academic practice. No use, distribution or reproduction is permitted which does not comply with these terms.
*Correspondence: João Sousa, am9hby5zb3VzYUBjY2cucHQ=