- 1Department of Radiology, Memorial Sloan Kettering Cancer Center, New York, NY, United States
- 2Department of Medical Physics, Memorial Sloan Kettering Cancer Center, New York, NY, United States
- 3Department of Radiation Oncology, Hospital of the University of Pennsylvania, Philadelphia, PA, United States
- 4Department of Radiation Oncology, Memorial Sloan Kettering Cancer Center, New York, NY, United States
Background: Early imaging-based treatment response assessment of brain metastases following stereotactic radiosurgery (SRS) remains challenging. The aim of this study is to determine whether early (within 12 weeks) intratumoral changes in interstitial fluid pressure (IFP) and velocity (IFV) estimated from computational fluid modeling (CFM) using dynamic contrast-enhanced (DCE) MRI can predict long-term outcomes of lung cancer brain metastases (LCBMs) treated with SRS.
Methods: Pre- and post-treatment T1-weighted DCE-MRI data were obtained in 41 patients treated with SRS for intact LCBMs. The imaging response was assessed using RANO-BM criteria. For each lesion, extravasation of contrast agent measured from Extended Tofts pharmacokinetic Model (volume transfer constant, Ktrans) was incorporated into a computational fluid model to estimate tumor IFP and IFV. Estimates of mean IFP and IFV and heterogeneity (skewness and kurtosis) were calculated for each lesion from pre- and post-SRS imaging. The Wilcoxon rank-sum test was utilized to assess for significant differences in IFP, IFV, and IFP/IFV change (Δ) between response groups.
Results: Fifty-three lesions from 41 patients were included. Median follow-up time after SRS was 11 months. The objective response (OR) rate (partial or complete response) was 79%, with 21% demonstrating stable disease (SD) or progressive disease (PD). There were significant response group differences for multiple posttreatment and Δ CFM parameters: post-SRS IFP skewness (mean −0.405 vs. −0.691, p = 0.022), IFP kurtosis (mean 2.88 vs. 3.51, p = 0.024), and IFV mean (5.75e-09 vs. 4.19e-09 m/s, p = 0.027); and Δ IFP kurtosis (mean −2.26 vs. −0.0156, p = 0.017) and IFV mean (1.91e-09 vs. 2.38e-10 m/s, p = 0.013). Posttreatment and Δ thresholds predicted non-OR with high sensitivity (sens): post-SRS IFP skewness (−0.432, sens 84%), kurtosis (2.89, sens 84%), and IFV mean (4.93e-09 m/s, sens 79%); and Δ IFP kurtosis (−0.469, sens 74%) and IFV mean (9.90e-10 m/s, sens 74%).
Conclusions: Objective response was associated with lower post-treatment tumor heterogeneity, as represented by reductions in IFP skewness and kurtosis. These results suggest that early post-treatment assessment of IFP and IFV can be used to predict long-term response of lung cancer brain metastases to SRS, allowing a timelier treatment modification.
Introduction
Brain metastases (BMs) represent the largest category of intracranial malignant tumors with an annual incidence 3–10 times greater than primary brain malignancies (1, 2). Occurring in up to 40% of patients with systemic cancer (3), BMs represent a major source of morbidity and mortality in this population. In particular, the most frequent source are primary lung malignancies, which comprise up to 36–64% of brain metastases (4).
Radiation therapy (RT) is the standard of care for patients in whom complete surgical resection is not possible due to surgically inaccessible lesion locations, disqualifying comorbidities, or uncontrolled systemic disease. Stereotactic radiosurgery (SRS), which employs single high-dose targeted treatment using stereotactic image guidance, has shown comparable efficacy to whole brain radiation therapy (WBRT) in controlling oligometastatic intracranial disease, achieving >80–90% local control while decreasing the risks of toxicities, such as neurocognitive decline (5–8).
Identification of eventual non-response in the early post-SRS time period is difficult. Surveillance MRI, which represents the standard for assessing brain metastasis treatment response, may be confounded in the early post-treatment setting by the tendency of up to one-third of BMs to transiently increase in size following SRS (9). The ability to predict SRS failure has major clinical importance, as it would potentially allow non-responsive tumors to undergo treatment intensification or prompt modifications to systemic therapy regimens.
The limitations of traditional size-based treatment response assessment following locoregional therapies, such as SRS, have driven the development of advanced MR imaging techniques, such as dynamic contrast-enhanced (DCE) perfusion MRI, that go beyond anatomic visualization to characterize tumor physiology. The extended Tofts pharmacokinetic model (ETM) is one such paradigm that quantifies surrogate measures of vascular permeability (i.e., volume transfer constant, Ktrans [min−1]). Interstitial pressures within the tumor affect the extravasation of medications into the interstitium and influence the response and outcome to radiotherapy (10–12).
The disorganized and tortuous architecture of blood vessels results in altered fluid dynamics across the vasculature and in the interstitium. The resulting elevated interstitial fluid pressure (IFP) effectively reduces the hydrostatic pressure differential that normally exists between vasculature and extracellular extravascular spaces, which can adversely impact the successful delivery of anti-tumor therapy (13–15). IFP returns to normal levels in the healthy tissues surrounding the tumor. The precipitous drop in IFP at the tumor periphery results in a zone where the interstitial fluid velocity (IFV) is increased and directed outward, causing rapid exudate flux of interstitial fluid from regions of high to low pressure (16), further diminishing the effectiveness of drug delivery and therapy.
To support this model, the direct invasive measurement of intralesional IFP in cervical cancer using modified wick-in-needle (WIN) probes has shown mid–radiation therapy IFP to be significantly different between patients with complete and partial responses at 1 month post-treatment (17). Fyles et al. reported high IFP measurement to be associated with a negative prognosis in cervical cancer (18). However, the invasive measurement of IFP and interstitial fluid velocity (IFV), which can be derived from the IFP gradient (19), is not feasible in many settings, especially where a tumor cannot be easily or safely accessed. Additionally, single-point WIN probing of tumor does not provide insight into the internal spatial variation of IFP. Therefore, non-invasive computational fluid modeling (CFM) to provide estimates of tumor IFP using the volume transfer constant (Ktrans) obtained from ETM (20, 21) is a desirable alternative. Ktrans is incorporated into an observable CFM equation to modulate the net pressure developed in tissue, including trans-capillary hydrostatic pressure, for the delivery of fluid, which is taken into consideration by conventional DCE-derived pharmacokinetic models.
Previously, we investigated the ability of ETM parameters to predict long-term local tumor control in the early post-SRS setting for patients with lung cancer brain metastases. We showed that Ktrans standard deviation (SD) was highly sensitive (89%) for predicting disease progression vs. no progressive disease (22). This result was not surprising, as Ktrans SD is considered a marker of tumor vascular heterogeneity (22, 23), and tumors are known to recruit disorganized and heterogeneous microvasculature.
In the present study, we have aimed to investigate whether non-invasive IFP and IFV estimates of global tissue physiology can predict the long-term response of lung cancer brain metastases treated within 12 weeks of SRS. The patient cohort is from our previous work that utilized more conventional DCE-derived parameters. These novel imaging biomarkers may further our ability to optimize patient management by demonstrating changes in tumor physiology.
Materials and Methods
Patients and Treatment
This retrospective investigation was performed at a tertiary cancer center following institutional review board approval and in accordance with the Health Insurance Portability and Accountability Act. Patients treated between 2012 and 2015 that met the following inclusion criteria were included in this analysis:
• Histopathologic diagnosis of non-small cell lung cancer (NSCLC).
• Treatment of one or more intact (non-resected) brain metastases with SRS.
• No history of WBRT prior to SRS.
• DCE perfusion MRI scans, including coverage of the treated lesion(s) obtained both pre-treatment and within 12 weeks following SRS treatment.
Patient demographic and treatment data collected included patient age, histologic tumor subtype, three-dimensional lesion size, lesion location, and SRS treatment dose(s). All treatments employed single-fraction SRS. Our study population consisted of 41 patients who were previously included in a study analyzing DCE-MRI parameters (22).
MR Perfusion Imaging Acquisition
Patients were scanned on 1.5T or 3T scanners (Signa Excite, HDx and Discovery 750, GE Healthcare) using an 8-channel head coil. Standard T1-weighted, T2-weighted, diffusion-weighted, fluid-attenuated inversion recovery, susceptibility-weighted, and contrast T1-weighted images were acquired in multiple planes. T1-weighted DCE data were acquired using an axial 3D spoiled gradient-echo sequence (repetition time [TR], 4–5 ms; echo time [TE], 1–2 ms; section thickness, 5 mm; flip angle [FA], 25 degrees; field of view, 24 cm; matrix, 256 × 128). Ten phases were acquired pre-injection followed by another 30 phases during the dynamic injection of intravenous contrast. This was followed by a 40-mL saline flush. The time between phases (temporal resolution) was 5–6 s. Matching contrast T1-weighted (TR/TE, 600/8 ms; thickness, 5 mm; matrix, 256 × 224) spin-echo images were obtained. Ten to twelve slices were obtained to cover the entire volume of each lesion. Gadopentetate dimeglumine (Magnevist; Bayer HealthCare Pharmaceuticals, Berlin, Germany) was power-injected via an intravenous catheter (18–21 gauge) at doses standardized by patient body weight (0.2 mL/kg body weight, maximum 20 mL) at 2–3 mL/s. High resolution 3D T1-weighted contrast-enhanced images in the axial plane with a slice thickness of 1 mm and no gaps between slices were routinely acquired for SRS planning and follow-up after therapy.
DCE MRI Pharmacokinetic Modeling
The two-compartment extended Tofts model (ETM) accounts for vascular space (vp) and extravascular extracellular space [EES], (ve). The ETM expression for modeling Ct(t) is given (24):
where, Ktrans (min−1) is the volume transfer constant of CA, Cp(t) is the delivery time-course of plasma CA concentration (or arterial input function, AIF), and ve and vp are the volume fractions of the EES and blood plasma, respectively. CA transfer from EES back into the vascular space is defined as kep = Ktrans/ve. A detailed calculation of tissue contrast concentration Ct from DCE signal is given elsewhere (25).
AIF for each patient was selected from a sagittal sinus voxel in the imaging time course (25, 26). Brain metastasis volumes-of-interest (VOIs) were manually segmented by an attending neuroradiologist on late phases of the T1w DCE images using ITK-SNAP (27).
In the absence of multi-flip angle pre-contrast T1 images, T10 values were set to either 0.8 or 1s (dependent on magnetic field strength) for each voxel in determination of ΔR1. Tissue concentration time course, Ct(t) [Equation. (1)], was calculated using non-linear fitting to minimize the sum of squared errors (SSE) between model fit and data. The fitting procedure estimates the values of Ktrans, ve and vp, for each voxel. Parameter estimation bound limits were set: Ktrans ε [0, 5] (min−1), ve, and vp ε [0, 1]. All DCE data analysis was performed using in-house MRI-QAMPER software (Quantitative Analysis Multi-Parametric Evaluation Routines) written in MATLAB (The MathWorks, Inc., Natick, MA).
Interstitial Fluid Pressure Simulation
CFM Mathematical Model
The fluid mechanics of a system are given by the Navier-Stokes hydrodynamic mass-balance equation (28). The extracellular matrix is modeled as a porous medium. We assumed the case of an incompressible fluid, ignoring friction within fluid, and exchange of momentum between fluid and solid phases. Fluid movement through EES is approximated with low-Reynolds Number flow (29) and modeled under assumption of steady-state velocity. We applied the well-known Darcy's Law to describe bulk fluid movement, expressing the product of gradient in IFP (∇pi) and the hydraulic conductivity of the porous medium, KH as the interstitial fluid velocity, u:
A dynamic system can then be modeled as follows: fluid enters EES via the vascular compartment. In the human brain, there is no established lymphatic system of clearance, and we take the lymphatic drainage function to be zero in both normal and tumor tissue.
The full derivation for the continuity equation [Equation. 3] is presented in the Appendix. The final form is given in terms of the dependent variable interstitial pressure, pi:
where 〈Ktrans〉 represents mean Ktrans values within the tumor; this term is used to account for heterogeneous fluid leakiness in the tumor (30), LP is the hydraulic conductivity of the capillary wall (or vessel permeability), S/V is microvascular surface area per unit volume, pV is the blood pressure in the microvessel, and pi is interstitial fluid pressure; πV is osmotic pressure in microvasculature, πi is osmotic pressure in interstitial space, and σT is the osmotic reflection coefficient. The lymphatic clearance term, LpLSL/V, is assumed to be zero in the brain. Estimates for physical parameters were selected in agreement with previous literature on modeling IFP in brain tumors (21, 31–34).
Computational Fluid Modeling
The continuity partial differential equation (PDE) was implemented using the COMSOL CFM simulation PDE module. Solving [Equation. 7] provides the basis for estimation of p–i and 3D parametric maps of IFP and IFV.
The 3D physiological mesh model was generated from each patient's T1w DCE tumor images. ROIs for tumor in the simulation domain were resliced to be 1 mm3-isotropic in MATLAB using the NIfTI Toolbox (35) and converted to stereolithography (STL) file format. STL files were imported into the simulation software and interpreted as boundary meshes for the model.
ETM-estimated Ktrans maps were co-registered to match the simulation mesh space. Ktrans maps were incorporated in COMSOL as a scalar field over the simulation domain and numerical values for physical constants in normal and tumor tissue were defined in the appropriate regions of the 3D STL domain mesh, as listed in Table 1. A stationary solution of Equation 13 was computed on the 3D extended domain ROI, and pressure at the simulation boundary was set to zero to agree with pressure conditions in normal brain tissue.
Simulation was conducted using the general coefficient form PDE module in a commercial multiphysics software package (COMSOL Inc., Stockholm, Sweden) using the finite element method to solve PDE computations.
Data Analysis
We analyzed IFP and IFV parameters as follows. For each metric, we computed the mean and descriptive statistics such as standard deviation (SD), kurtosis, and skewness, leading to a set of eight features. We generated three sets of these eight features: pre-RT, post-RT, and the change of values (denoted as Δ) between pre-RT and post-RT. For each imaging feature, an average value was computed across multiple slices on each lesion. Univariate analysis was performed using the Wilcoxon rank-sum test to find the degree of differences in these features between patients with an objective response (either partial or complete response, OR) vs. non-OR (either stable disease or progressive disease). A receiver operating characteristic (ROC) curve analysis was performed to find the best cutoffs on these features using Youden's index.
For clinical data, local control was assessed by the modified Response Assessment in Neuro-Oncology Brain Metastases (RANO-BM) criteria using conventional MRI (40), with additional information from surgical resection if performed after SRS. The modification we made to standard RANO-BM criteria was to lower the minimum size limit of measurable disease to 5 mm. We chose to lower the limit because we routinely treat BMs measuring between 5 and 10 mm with SRS at our institution. Local relapse-free survival was calculated from day of treatment to most recent imaging. Failure was determined by progressive disease defined by RANO-BM or surgical resection indicating viable tumor. All statistical analysis was performed using R language version 3.5.2 and MATLAB version R2018b.
Results
Forty-one patients with 53 BMs were included in the analysis (Table 2). Thirty-two patients had a single brain metastasis, seven patients had two metastases, and two patients had three or more metastases. Median SRS treatment dose was 21 Gy (range, 18–22 Gy).
No patients received concurrent systemic therapy with SRS. Per our institution's general practice, most patients (n = 39) had a washout period before and following SRS: at least 2 weeks for systemic therapies and at least 1 week for targeted systemic therapies.
Post-treatment imaging was obtained 7–8 weeks (n = 19), 9–10 weeks (n = 16), or 11–12 weeks (n = 18) following SRS. Median duration of post-treatment follow-up was 11 months (range, 3.7–8.3 months), including 73% of patients who were followed until death. Following SRS, one patient subsequently underwent resection for a growing mass and was found to have viable tumor. Eight patients were treated with WBRT after SRS for control of non-index metastases. Local control at 1-year post-treatment was 85% as determined by subsequent histopathologic sampling, where available, or RANO-BM imaging criteria. Rates of complete response, partial response, stable disease, and progressive disease were 9, 49, 21, and 21%, respectively (Table 3).
Univariate analysis using Wilcoxon rank-sum test (Table 4) showed a significant difference between lesions showing OR vs. non-OR: post-SRS IFP skewness (mean −0.405 vs. −0.691, p = 0.022), IFP kurtosis (mean 2.88 vs. 3.51, p = 0.024), and IFV mean (5.75e-09 vs. 4.19e-09 m/s, p = 0.027); and Δ IFP kurtosis (mean −2.26 vs. −0.0156, p = 0.017) and IFV mean (1.91e-09 vs. 2.38e-10 m/s, p = 0.013). Using the Youden index, balanced thresholds for differentiating non-OR vs. OR were determined: post-SRS IFP skewness −0.432 (sensitivity 84%, specificity 59%), IFP kurtosis 2.89 (sensitivity 84%, specificity 63%), and IFV mean 4.93e-09 m/s (sensitivity 79%, specificity 67%); and Δ IFP kurtosis −0.469 (sensitivity 74%, specificity 59%) and IFV mean 9.90e-10 m/s (sensitivity 74%, specificity 74%). SRS dose was not significantly correlated with RANO-BM treatment response.
Figure 1A shows representative pre-SRS and post-SRS anatomic MR images and corresponding lesion Ktrans, IFP, and IFV color maps. Figure 1B exhibits representative histograms showing the distribution of intratumoral voxel values for IFP in two patients who experienced OR vs. non-OR. The left-shift in the IFP values (kPa) of the histogram for the patient who experienced OR shows a decrease in pressure after treatment. In contrast, the histogram of a patient who experienced non-OR shows a subtle upward shift in mean IFP values and has many voxels with the same IFP value, resulting in a skew distribution. Receiver operating characteristic areas-under-curve for post-SRS IFP skewness (0.70), IFP kurtosis (0.70), and IFV mean (0.69); and Δ IFP kurtosis (0.71) and IFV mean (0.72) are shown in Figures 2, 3.
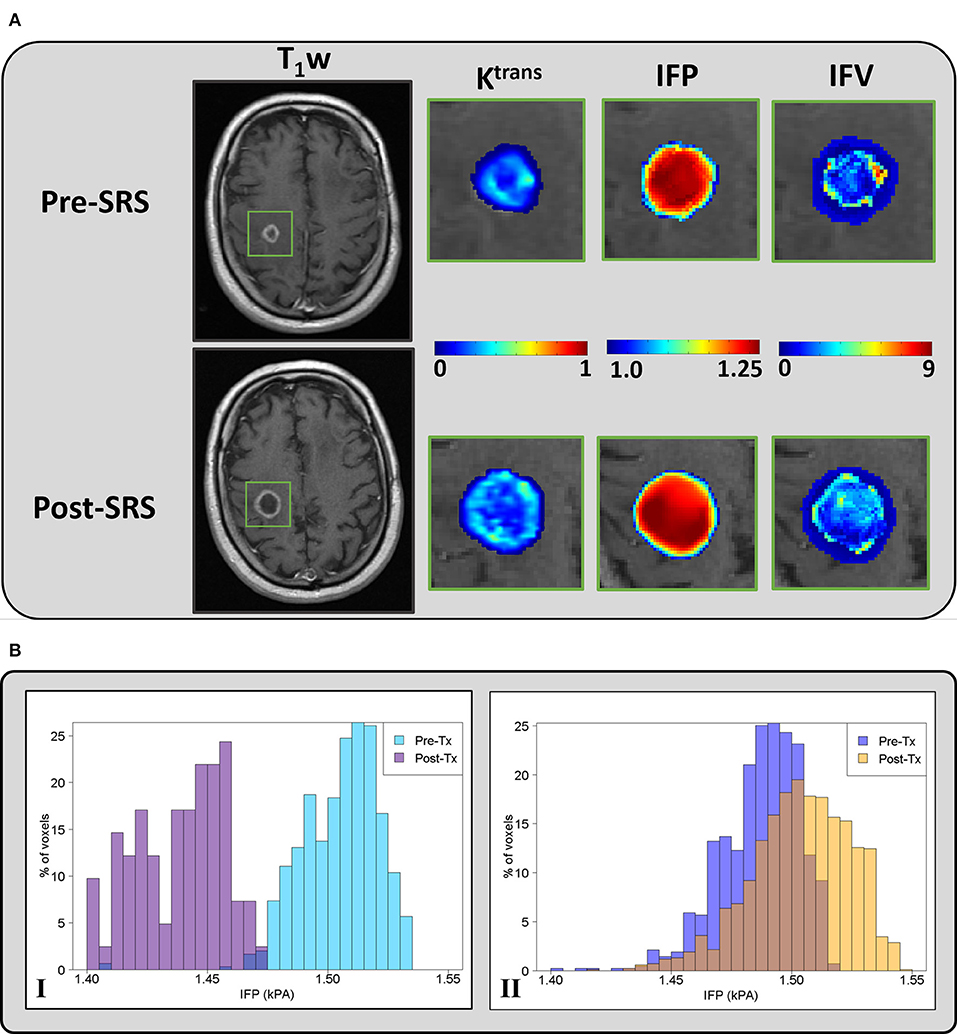
Figure 1. (A) - Representative pre-SRS and post-SRS T1-weighted post-contrast MR images of a patient (59 years, male) with brain metastases who experienced progressive disease (PD). The green rectangle delineates the ROI at the metastatic lesion. Ktrans (min−1), IFP (kPa), and IFV (10−9 m/s) maps are zoomed at the location of the ROI. (B) - Histograms of percentage (%) voxel-wise IFP values at pre- and post-SRS treatment from representative patients who experienced (I) OR (male, 73 years old) and (II) non-OR (male, 47 years old).
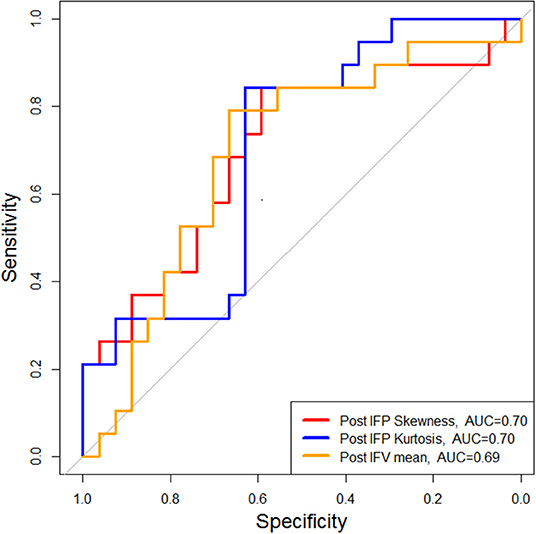
Figure 2. Patients with OR showed significantly lower mean IFP skewness and kurtosis and higher mean IFV within 12 weeks post-SRS compared with patients with non-OR (either SD or PD). OR, objective response; SD, stable disease; PD, progressive disease.
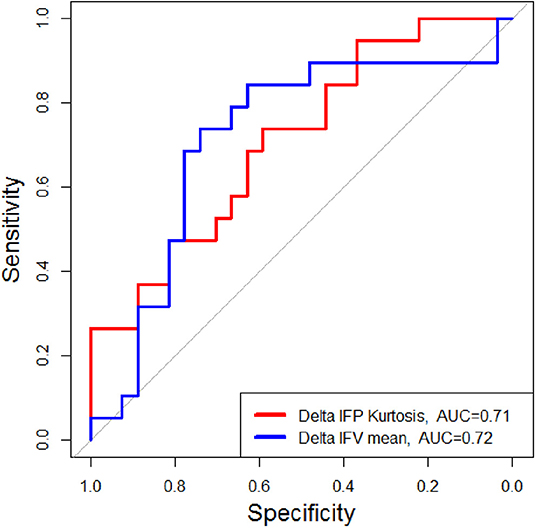
Figure 3. Patients with OR showed significantly greater pre-SRS to post-SRS (Δ) declines in mean IFP kurtosis and greater increases in mean IFV within 12 weeks post-SRS compared with patients with non-OR (either SD or PD). OR, objective response; SD, stable disease; PD, progressive disease.
Discussion
We investigated whether non-invasive estimates of intratumoral IFP and IFV in the early post-treatment setting using ETM-derived Ktrans can predict the long-term response of lung cancer brain metastases to SRS. IFP and IFV parameters estimated from CFM were able to accurately predict long-term response using both isolated post-treatment values (IFP kurtosis, IFP skewness, and IFV mean) and changes between post- and pre-treatment values (Δ IFP kurtosis and Δ IFV mean). Our results support the use of these biomarkers as early post-SRS predictors of long-term treatment response in LCBMs. These parameters may enable the earlier identification of LCBM non-responders, allowing more timely treatment intensification or modifications to systemic therapies.
A major cause of elevated IFP within tumors is aberrant microvasculature resulting in altered fluid dynamics (41). Radiation therapy causes early and sustained damage to vasculature (42–44), which tends to lower intratumoral microvascular heterogeneity (45). In our cohort, IFP kurtosis and skewness, measures of tumoral IFP heterogeneity, showed early decreases in patients who ultimately showed objective response to SRS. This is intuitive, since post-treatment necrosis would be expected to smooth tumoral IFP distribution, resulting in decreased heterogeneity. Furthermore, Smith et al. showed that a necrotic tumor core, which lacks functioning vasculature necessary for fluid resorption, results in decreased pressure decay within the core and thus promotes increased IFV (31). This may provide a framework to explain why IFV mean was both significantly higher following SRS and showed greater relative increases from baseline in patients with objective response. Conversely, the correlation between poor outcomes and persistent intratumoral hypertension following treatment may be related to previous observations that high IFP in extracranial tumors decreases the uptake of chemotherapy drugs (41, 46) and promotes the outward flow of tumor-promoting growth factors and chemoreceptor ligands (47, 48).
To the best of our knowledge, ours is the first study to assess the predictive capabilities of non-invasively estimated IFP and IFV parameters for brain metastases that have been treated with SRS. A prior investigation successfully used these parameters to predict outcomes in cervical cancer (49). Utilizing histogram analysis, we showed a difference in the distribution of IFP in patients who experienced OR vs. non-OR. Similar to prior studies in cervical cancer (17, 50), IFP may be a useful prognostic indicator for brain metastases. Further investigation is needed to determine whether the evaluation of metastasis microenvironments from CFM estimated IFP and IFV can be utilized to personalize therapy regimens and improve outcomes. This may be especially relevant in the context of targeted chemotherapeutic agents and immunotherapy since elevated intratumoral IFP can prevent adequate penetration of intravenous drugs.
Boucher et al. (51) reported direct WIN measurements of IFP in rodent models, and from 11 human primary brain tumors during intracranial brain surgery. The rodent brain tumor mean IFP in n = 4 small F98 gliomas (Vmean = 10 ± 2.5 mm3) was 1.2 + 0.33 kPa. In 10 of the 11 human cases, IFP ranged from 0.066 to 0.4 kPa; in one astrocytoma, IFP was found to be 1.2 kPa. The estimates of IFP in our CFM are consistent with the measured results in the small-scale rodent tumors. Similar results were found in measurements of IFP in preclinical study by Navalitloha et al. (52) on rat gliomas.
A strength of our study is the inclusion of only NSCLC brain metastases undergoing single modality locoregional therapy with SRS. This allows for the relative control of potential confounders, including heterogeneous tumor histologies and variable baseline treatment effects from non-ablative modalities like WBRT. Additionally, the majority of our patients were followed until death, providing a clearer understanding of individual lesion outcomes.
As a retrospective investigation limited to BMs of a single, albeit common, histopathology, our results cannot be generalized to the treatment of BMs with SRS more broadly. With respect to our fluid model, the parameter values for hydraulic conductivity, vessel permeability, effective pressure, and microvascular surface area need to be verified experimentally to increase the simulation accuracy. Furthermore, the lack of confirmatory direct lung cancer brain metastasis pressure measurements within our cohort, for example, via the WIN approach, precludes the comparison of our derived IFP and IFV estimations against a gold standard.
In conclusion, this study shows that IFP and IFV parameters in lung cancer brain metastases derived from DCE-MRI within 12 weeks of SRS can predict long-term local tumor control. These results suggest that IFP and IFV represent promising imaging biomarkers that can non-invasively characterize global tissue physiology in lung cancer brain metastases. Further investigation is needed to validate these results for other brain metastasis histologies and to assess the use of these non-invasive biomarkers to guide personalized treatment regimens that target the tumor microenvironment.
Data Availability Statement
The datasets generated for this study will not be made publicly available The source data contain inherent protected health information. Requests to access the data are directable to the corresponding author.
Ethics Statement
The studies involving human participants were reviewed and approved by Memorial Sloan Kettering Cancer Center Institutional Review Board. Written informed consent for participation was not required for this study in accordance with the national legislation and the institutional requirements.
Author Contributions
VH, AS-D, and EL conceived the idea. NS, VH, EL, RP, and AS-D collected and analyzed data and wrote the manuscript. All authors discussed results and made substantive changes to the final manuscript.
Funding
This study was supported by National Institutes of Health/National Cancer Institute (Cancer Center Support Grant P30 CA008748).
Conflict of Interest
The authors declare that the research was conducted in the absence of any commercial or financial relationships that could be construed as a potential conflict of interest. The handling editor is currently organizing a Research Topic with one of the authors BV.
Supplementary Material
The Supplementary Material for this article can be found online at: https://www.frontiersin.org/articles/10.3389/fneur.2020.00402/full#supplementary-material
References
1. Davis FG, Dolecek TA, McCarthy BJ, Villano JL. Toward determining the lifetime occurrence of metastatic brain tumors estimated from 2007 United States cancer incidence data. Neuro-Oncol. (2012) 14:1171–77. doi: 10.1093/neuonc/nos152
2. Ostrom QT, Gittleman H, Farah P, Ondracek A, Chen Y, Wolinsky Y, et al. CBTRUS statistical report: primary brain and central nervous system tumors diagnosed in the United States in 2006-2010. Neuro-Oncol. (2013) 15:ii1–56. doi: 10.1093/neuonc/not151
3. Gavrilovic IT, Posner JB. Brain metastases: epidemiology and pathophysiology. J Neurooncol. (2005) 75:5–14. doi: 10.1007/s11060-004-8093-6
4. Schiff D, Arrillaga I, Wen PY (editors). Cancer Neurology in Clinical Practice. Cham: Springer International Publishing (2018). p. 3–10. doi: 10.1007/978-3-319-57901-6
5. Aoyama H, Shirato H, Tago M, Nakagawa K, Toyoda T, Hatano K, et al. Stereotactic radiosurgery plus whole-brain radiation therapy vs stereotactic radiosurgery alone for treatment of brain metastases: a randomized controlled trial. JAMA. (2006) 295:2483–91. doi: 10.1001/jama.295.21.2483
6. Sahgal A, Aoyama H, Kocher M, Neupane B, Collette S, Tago M, et al. Phase 3 trials of stereotactic radiosurgery with or without whole-brain radiation therapy for 1 to 4 brain metastases: individual patient data meta-analysis. Int J Radiat Oncol Biol Phys. (2015) 91:710–7. doi: 10.1016/j.ijrobp.2014.10.024
7. Brown PD, Jaeckle K, Ballman KV, Farace E, Cerhan JH, Anderson SK, et al. Effect of radiosurgery alone vs radiosurgery with whole brain radiation therapy on cognitive function in patients with 1 to 3 brain metastases. JAMA. (2016) 316:401–9. doi: 10.1001/jama.2016.9839
8. Lippitz B, Lindquist C, Paddick I, Peterson D, O'Neill K, Beaney R. Stereotactic radiosurgery in the treatment of brain metastases: the current evidence. Cancer Treat Rev. (2014) 40:48–59. doi: 10.1016/j.ctrv.2013.05.002
9. Patel TR, McHugh BJ, Bi WL, Minja FJ, Knisely JPS, Chiang VL. A comprehensive review of MR imaging changes following radiosurgery to 500 brain metastases. Am J Neuroradiol. (2011) 32:1885–92. doi: 10.3174/ajnr.A2668
10. Tofts PS, Kermode AG. Measurement of the blood-brain barrier permeability and leakage space using dynamic MR imaging. 1. fundamental concepts. Magn Reson Med. (1991) 17:357–67. doi: 10.1002/mrm.1910170208
11. Zahra MA, Hollingsworth KG, Sala E, Lomas DJ, Tan LT. Dynamic contrast-enhanced MRI as a predictor of tumour response to radiotherapy. Lancet Oncol. (2007) 8:63–74. doi: 10.1016/S1470-2045(06)71012-9
12. Baronzio G, Parmar G, Baronzio M. Overview of methods for overcoming hindrance to drug delivery to tumors, with special attention to tumor interstitial fluid. Front Oncol. (2015) 5:165. doi: 10.3389/fonc.2015.00165
13. Jain RK. Transport of molecules in the tumor interstitium: a Review. Cancer Res. (1987) 47:3039–51.
14. Boucher Y, Leunig M, Jain RK. Tumor angiogenesis and interstitial hypertension. Cancer Res. (1996) 56:4264–6.
15. Jain RK, Martin JD, Stylianopoulos T. The role of mechanical forces in tumor growth and therapy. Annu Rev Biomed Eng. (2014) 16:321–46. doi: 10.1146/annurev-bioeng-071813-105259
16. Ewing JR, Nagaraja TN, Aryal MP, Keenan KA, Elmghirbi R, Bagher-Ebadian H, et al. Peritumoral tissue compression is predictive of exudate flux in a rat model of cerebral tumor: an MRI study in an embedded tumor. NMR Biomed. (2015) 28:1557–69. doi: 10.1002/nbm.3418
17. Yeo S-G, Kim J-S, Cho M-J, Kim K-H, Kim J-S. Interstitial fluid pressure as a prognostic factor in cervical cancer following radiation therapy. Clin Cancer Res. (2009) 15:6201–7. doi: 10.1158/1078-0432.CCR-09-0560
18. Fyles A, Milosevic M, Pintilie M, Syed A, Levin W, Manchul L, et al. Long-term performance of interstial fluid pressure and hypoxia as prognostic factors in cervix cancer. Radiother Oncol. (2006) 80:132–7. doi: 10.1016/j.radonc.2006.07.014
19. Soltani M, Chen P. Numerical modeling of fluid flow in solid tumors. PLoS ONE. (2011) 6:e20344. doi: 10.1371/journal.pone.0020344
20. Magdoom KN, Pishko GL, Rice L, Pampo C, Siemann DW, Sarntinoranont M. MRI-based computational model of heterogeneous tracer transport following local infusion into a mouse hind limb tumor. PLoS ONE. (2014) 9:e89594. doi: 10.1371/journal.pone.0089594
21. Bhandari A, Bansal A, Singh A, Sinha N. Perfusion kinetics in human brain tumor with DCE-MRI derived model and CFD analysis. J Biomech. (2017) 59:80–9. doi: 10.1016/j.jbiomech.2017.05.017
22. Taunk NK, Oh JH, Shukla-Dave A, Beal K, Vachha B, Holodny A, et al. Early posttreatment assessment of MRI perfusion biomarkers can predict long-term response of lung cancer brain metastases to stereotactic radiosurgery. Neuro-Oncol. (2018) 20:567–75. doi: 10.1093/neuonc/nox159
23. Jackson A, O'Connor JPB, Parker GJM, Jayson GC. Imaging tumor vascular heterogeneity and angiogenesis using dynamic contrast-enhanced magnetic resonance imaging. Clin Cancer Res. (2007) 13:3449–59. doi: 10.1158/1078-0432.CCR-07-0238
24. Tofts PS, Brix G, Buckley DL, Evelhoch JL, Henderson E, Knopp MV, et al. Estimating kinetic parameters from dynamic contrast-enhanced T(1)-weighted MRI of a diffusable tracer: standardized quantities and symbols. J Magn Reson Imaging. (1999) 10:223–32. doi: 10.1002/(SICI)1522-2586(199909)10:3<223::AID-JMRI2>3.0.CO;2-S
25. Bagher-Ebadian H, Jain R, Nejad-Davarani SP, Mikkelsen T, Lu M, Jiang Q, et al. Model selection for DCE-T1 studies in glioblastoma. Magn Reson Med. (2012) 68:241–51. doi: 10.1002/mrm.23211
26. Cramer SP, Larsson HB. Accurate determination of blood-brain barrier permeability using dynamic contrast-enhanced T1-weighted MRI: a simulation and in vivo study on healthy subjects and multiple sclerosis patients. J Cereb Blood Flow Metab. (2014) 34:1655–65. doi: 10.1038/jcbfm.2014.126
27. Yushkevich PA, Piven J, Hazlett HC, Smith RG, Ho S, Gee JC, et al. User-guided 3D active contour segmentation of anatomical structures: significantly improved efficiency and reliability. NeuroImage. (2006) 31:1116–28. doi: 10.1016/j.neuroimage.2006.01.015
28. Dullien FAL. Porous Media: Fluid Transport and Pore Structure /F. A. L. Dullien. New York, NY: Academic Press (1979). doi: 10.1016/B978-0-12-223650-1.50008-5
29. Yao W, Li Y, Ding G. Interstitial fluid flow: the mechanical environment of cells and foundation of meridians. Evid Based Complement Altern Med. (2012) 2012:853516. doi: 10.1155/2012/853516
30. Pishko GL, Astary GW, Mareci TH, Sarntinoranont M. Sensitivity analysis of an image-based solid tumor computational model with heterogeneous vasculature and porosity. Ann Biomed Eng. (2011) 39:2360–73. doi: 10.1007/s10439-011-0349-7
31. Smith JH, Humphrey JAC. Interstitial transport and transvascular fluid exchange during infusion into brain and tumor tissue. Microvasc Res. (2007) 73:58–73. doi: 10.1016/j.mvr.2006.07.001
32. Bhandari A, Bansal A, Singh A, Sinha N. Transport of liposome encapsulated drugs in voxelized computational model of human brain tumors. IEEE Trans NanoBiosci. (2017) 16:634–44. doi: 10.1109/TNB.2017.2737038
33. Bhandari A, Bansal A, Singh A, Gupta RK, Sinha N. Comparison of transport of chemotherapeutic drugs in voxelized heterogeneous model of human brain tumor. Microvasc Res. (2019) 124:76–90. doi: 10.1016/j.mvr.2019.03.003
34. Bhandari A, Bansal A, Jain R, Singh A, Sinha N. Effect of tumor volume on drug delivery in heterogeneous vasculature of human brain tumors. J Eng Sci Med Diagn Ther. (2019) 2:021004. doi: 10.1115/1.4042195
35. Tools for NIfTI and ANALYZE image - File Exchange - MATLAB Central. Available online at: https://www.mathworks.com/matlabcentral/fileexchange/8797 (accessed November 22, 2019).
36. Swabb EA, Wei J, Gullino PM. Diffusion and convection in normal and neoplastic tissues. Cancer Res. (1974) 34:2814–22.
37. Netti PA, Berk DA, Swartz MA, Grodzinsky AJ, Jain RK. Role of extracellular matrix assembly in interstitial transport in solid tumors. Cancer Res. (2000) 60:2497–2503.
38. Paulson OB, Hertz MM, Bolwig TG, Lassen NA. Filtration and diffusion of water across the blood-brain barrier in man. Microvasc Res. (1977) 13:113–23. doi: 10.1016/0026-2862(77)90120-0
39. Arifin DY, Lee KYT, Wang C-H. Chemotherapeutic drug transport to brain tumor. J Controlled Release. (2009) 137:203–10. doi: 10.1016/j.jconrel.2009.04.013
40. Lin NU, Lee EQ, Aoyama H, Barani IJ, Barboriak DP, Baumert BG, et al. Response assessment criteria for brain metastases: proposal from the RANO group. Lancet Oncol. (2015) 16:e270–8. doi: 10.1016/S1470-2045(15)70057-4
41. Heldin C-H, Rubin K, Pietras K, Östman A. High interstitial fluid pressure — an obstacle in cancer therapy. Nat Rev Cancer. (2004) 4:806–13. doi: 10.1038/nrc1456
42. O'Connor MM, Mayberg MR. Effects of radiation on cerebral vasculature: a review. Neurosurgery. (2000) 46:138–51. doi: 10.1093/neurosurgery/46.1.138
43. Song CW, Kim M-S, Cho LC, Dusenbery K, Sperduto PW. Radiobiological basis of SBRT and SRS. Int J Clin Oncol. (2014) 19:570–8. doi: 10.1007/s10147-014-0717-z
44. Lucas J, Mack WJ. Effects of ionizing radiation on cerebral vasculature. World Neurosurg. (2014) 81:490–1. doi: 10.1016/j.wneu.2014.01.006
45. de Lussanet QG, Backes WH, Griffioen AW, Padhani AR, Baeten CI, van Baardwijk A, et al. Dynamic contrast-enhanced magnetic resonance imaging of radiation therapy-induced microcirculation changes in rectal cancer. Int J Radiat Oncol. (2005) 63:1309–15. doi: 10.1016/j.ijrobp.2005.04.052
46. Salnikov AV, Iversen VV, Koisti M, Sundberg C, Johansson L, Stuhr LB, et al. Lowering of tumor interstitial fluid pressure specifically augments efficacy of chemotherapy. FASEB J. (2003) 17:1756–8. doi: 10.1096/fj.02-1201fje
47. Shields JD, Fleury ME, Yong C, Tomei AA, Randolph GJ, Swartz MA. Autologous chemotaxis as a mechanism of tumor cell homing to lymphatics via interstitial flow and autocrine CCR7 signaling. Cancer Cell. (2007) 11:526–38. doi: 10.1016/j.ccr.2007.04.020
48. Mumprecht V, Detmar M. Lymphangiogenesis and cancer metastasis. J Cell Mol Med. (2009) 13:1405–16. doi: 10.1111/j.1582-4934.2009.00834.x
49. Simonsen TG, Lund KV, Hompland T, Kristensen GB, Rofstad EK. DCE-MRI-derived measures of tumor hypoxia and interstitial fluid pressure predict outcomes in cervical carcinoma. Int J Radiat Oncol Biol Phys. (2018) 102:1193–201. doi: 10.1016/j.ijrobp.2018.04.035
50. Haider MA, Sitartchouk I, Roberts TPL, Fyles A, Hashmi AT, Milosevic M. Correlations between dynamic contrast-enhanced magnetic resonance imaging-derived measures of tumor microvasculature and interstitial fluid pressure in patients with cervical cancer. J Magn Reson Imaging JMRI. (2007) 25:153–9. doi: 10.1002/jmri.20795
51. Boucher Y, Salehi H, Witwer B, Harsh GR, Jain RK. Interstitial fluid pressure in intracranial tumours in patients and in rodents. Br J Cancer. (1997) 75:829–36. doi: 10.1038/bjc.1997.148
Keywords: Stereotactic radiosurgery (SRS), brain metastases from lung cancer, perfusion MRI, computational fluid modeling, interstitial fluid pressure, treatment response
Citation: Swinburne N, LoCastro E, Paudyal R, Oh JH, Taunk NK, Shah A, Beal K, Vachha B, Young RJ, Holodny AI, Shukla-Dave A and Hatzoglou V (2020) Computational Modeling of Interstitial Fluid Pressure and Velocity in Non-small Cell Lung Cancer Brain Metastases Treated With Stereotactic Radiosurgery. Front. Neurol. 11:402. doi: 10.3389/fneur.2020.00402
Received: 29 January 2020; Accepted: 17 April 2020;
Published: 28 May 2020.
Edited by:
Tarik F. Massoud, Stanford University, United StatesReviewed by:
Bo Gao, Affiliated Hospital of Guizhou Medical University, ChinaAli Alaraj, University of Illinois at Chicago, United States
Copyright © 2020 Swinburne, LoCastro, Paudyal, Oh, Taunk, Shah, Beal, Vachha, Young, Holodny, Shukla-Dave and Hatzoglou. This is an open-access article distributed under the terms of the Creative Commons Attribution License (CC BY). The use, distribution or reproduction in other forums is permitted, provided the original author(s) and the copyright owner(s) are credited and that the original publication in this journal is cited, in accordance with accepted academic practice. No use, distribution or reproduction is permitted which does not comply with these terms.
*Correspondence: Vaios Hatzoglou, aGF0em9nbHZAbXNrY2Mub3Jn
†These authors have contributed equally to this work and share senior authorship