- 1Department of Statistics, Computer Science, Applications ≪ G. Parenti ≫, University of Florence, Florence, Italy
- 2NEUROFARBA Department, Neuroscience Section, University of Florence, Florence, Italy
- 3Don Carlo Gnocchi Foundation, Milan, Italy
- 4Stroke Unit, Careggi University Hospital, Florence, Italy
- 5Central Laboratory, Careggi University Hospital, Florence, Italy
- 6Department Health Professions, U.O. Research and Development, Careggi University Hospital, Florence, Italy
- 7Department of Electrical, Electronic, and Information Engineering “Guglielmo Marconi”, University of Bologna, Cesena, Italy
- 8Department of Experimental and Clinical Medicine, University of Florence, Florence, Italy
- 9Atherothrombotic Diseases Center, Careggi University Hospital, Florence, Italy
- 10Institute of Applied Physics “Nello Carrara” (IFAC), National Research Council of Italy (CNR), Florence, Italy
- 11Neuroradiology Unit, Department of Experimental and Clinical Biomedical Sciences, Careggi University Hospital, University of Florence, Florence, Italy
Anticoagulants reduce embolic risk in atrial fibrillation (AF), despite increasing hemorrhagic risk. In this context, validity of congestive heart failure, hypertension, age ≥ 75 years, diabetes, stroke, vascular disease, age 65–74 years and sex category (CHA2DS2-VASc) and hypertension, abnormal renal/liver function, stroke, bleeding history or predisposition, labile international normalized ratio, elderly, drugs/alcohol concomitantly (HAS–BLED) scales, used to respectively evaluate thrombotic and hemorrhagic risks, is incomplete. In patients with AF, brain MRI has led to the increased detection of “asymptomatic” brain changes, particularly those related to small vessel disease, which also represent the pathologic substrate of intracranial hemorrhage, and silent brain infarcts, which are considered risk factors for ischemic stroke. Routine brain MRI in asymptomatic patients with AF is not yet recommended. Our aim was to test predictive ability of risk stratification scales on the presence of cerebral microbleeds, lacunar, and non-lacunar infarcts in 170 elderly patients with AF on oral anticoagulants. Ad hoc developed R algorithms were used to evaluate CHA2DS2-VASc and HAS–BLED sensitivity and specificity on the prediction of cerebrovascular lesions: (1) Maintaining original items' weights; (2) augmenting weights' range; (3) adding cognitive, motor, and depressive scores. Accuracy was poor for each outcome considering both scales either in phase 1 or phase 2. Accuracy was never improved by the addition of cognitive scores. The addition of motor and depressive scores to CHA2DS2-VASc improved accuracy for non-lacunar infarcts (sensitivity = 0.70, specificity = 0.85), and sensitivity for lacunar–infarcts (sensitivity = 0.74, specificity = 0.61). Our results are a very first step toward the attempt to identify those elderly patients with AF who would benefit most from brain MRI in risk stratification.
Introduction
Atrial fibrillation (AF) is the most common arrhythmia encountered in clinical practice, and its prevalence is strictly age-related as discussed in the following: ~1% of patients with AF are younger than 60 years of age, whereas up to 12% of patients with AF are 75–84 years of age, with even higher prevalence over 80 years old (1). Atrial fibrillation is associated with high morbidity and mortality, with an increased risk of stroke and thromboembolism. Thromboprophylaxis with oral anticoagulation is effective in reducing stroke risk in patients with AF and is currently recommended by guidelines for patients with moderate/high risk of stroke (1, 2).
To aid decision-making for thromboprophylaxis, several clinical risk stratification schemes have been developed. To estimate thromboembolic risk, guidelines mainly propose the use of congestive heart failure, hypertension, age ≥75 years, diabetes, stroke, vascular disease, age 65–74 years, and sex category (CHA2DS2-VASc) (3– 5). Benefits of anticoagulation have to be balanced against the risk of bleeding, with intracranial hemorrhage being the most feared one. Hypertension, abnormal renal/liver function, stroke, bleeding history or predisposition, labile international normalized ratio, elderly, and drugs/alcohol concomitantly (HAS-BLED) can be used to assess bleeding risk in patients for whom anti-coagulation is being considered (2, 6). Available stroke and bleeding risk stratification schemes are aimed at identifying patients who may benefit most from oral anticoagulation, and also might orient in the choice of the type of oral anticoagulant (7). Such schemes currently rely on just clinical information; the validity of which remains controversial and needs to be improved.
In patients with AF, neuroimaging, mainly MRI, has led to increased detection of “asymptomatic” brain changes, such as those related to small vessel disease (SVD), which also represent the pathologic substrate of intracerebral hemorrhage, and silent brain infarcts, which double the risk of ischemic stroke (8, 9). Such imaging findings are proposed as additional markers for risk stratification (10). Conclusive evidence about their predictive value in single patients can only come from adequately powered large prospective follow-up studies, and some efforts are already under way. In patients with previous stroke and atrial fibrillation, Clinical Relevance Of Microbleeds In Stroke (CROMIS-2) and Hemorrhage Predicted by Resonance in Patients Receiving Oral Anticoagulants (HERO) studies have demonstrated that the presence of severe SVD, particularly cerebral microbleeds (CMBs), is a strong predictor of intracerebral hemorrhage (11, 12). Despite this, debate is ongoing on how to consider such “brain” information in decision making for anticoagulants (13, 14), and considering the MRI-associated costs, the routine use of brain MRI in asymptomatic patients with AF is still not recommended.
In line with precision medicine, a cost-effective strategy would be the development of a method able to classify patients into subgroups that differ in their susceptibility to thromboembolic and bleeding risks, and then decide to collect “brain” information only on those who will benefit (15).
Our aim was to test the predictive ability of the currently recommended risk stratification scales, CHA2DS2-VASc and HAS–BLED, on the presence of brain MRI lesions related to SVD and non-lacunar infarcts in elderly patients with AF on oral anticoagulants.
Materials and Methods
Stratification of cerebral bleeding risk in AF, http://www.strat-af.it (Strat-AF) is an observational, prospective, single-center hospital-based study enrolling elderly patients with AF, referred from the outpatient clinic Center of Thrombosis of Careggi University Hospital in Florence where they are followed for the management of oral anticoagulation therapy in primary or secondary prevention of thromboembolic events. Strat-AF study design and methodology have been previously described (16). In brief, the main aim of Strat-AF study is to evaluate the added value of circulating and brain MRI biomarkers on the prediction of cerebral bleeding risk. For this purpose, patients affected by AF aged ≥65 years on oral anticoagulants and no contraindication to MRI were enrolled. Ethical approval was obtained by our Ethics Committee, and all participants gave written informed consent.
Among collected clinical information, for the purpose of this paper, demographic characteristics (age, sex, and years of education), vascular risk factors and comorbidities (hypertension, diabetes, dyslipidemia, physical activity, smoking habits, alcohol consumption, stroke, peripheral arterial disease, ischemic heart disease, myocardial infarction, and heart failure) were used for the analyses.
Global cognitive efficiency was assessed by the Montreal Cognitive Assessment (MoCA) (17). Italian normative data were used to adjust MoCA raw scores for demographics, and to convert adjusted scores into an ordinal 5-point scale according to the equivalent score non-parametric norming method. Equivalent scores method is based on percentiles distributions, and scores range from ES = 0 (impaired performance) to ES = 4 (optimal performance) (18, 19).
Short physical performance Battery (SPPB) was used to evaluate motor performance with a total score ranging from 0 (not able to walk) to 12 (best motor performance) (20).
The 15-item version of Geriatric Depression Scale (GDS) was used to evaluate depressive symptoms. The total score ranges from 0 to 15 (with each point indicating more severe depressive symptoms) (21).
The CHA2DS2-VASc and HAS–BLED scores were calculated based on the clinical information. The CHA2DS2-VASc scores range from 0 to 9 and are based on the following clinical information: Congestive heart failure (score 1), hypertension or antihypertensive therapy (score 1), age ≥75 years (score 2), diabetes (score 1), stroke (score 2), vascular disease (score 1), age 65–74 years (score 1), and sex category (female, score 1) (3). The HAS-BLED scale is used to estimate the bleeding risk, with scores ranging from 0 to 9, which was calculated based on the following clinical information: Uncontrolled hypertension (systolic blood pressure >160 mm Hg, score 1), abnormal renal and/or hepatic function (score 1 or 2), stroke (score 1), bleeding history or predisposition (score 1), labile international normalized ratio (score 1), age (score 1), and drugs or excessive alcohol drinking (score 1 or 2) (6).
All participants underwent brain MRI on a 1.5 T (Ingenia, Philips Healthcare, Best, The Netherlands). The MRI protocol included the following sequences: Sagittal T1-weighted spin–echo, coronal T2-weighted Turbo spin–echo; axial fluid-attenuated inversion recovery (FLAIR); axial gradient–echo T2* (FFE); axial diffusion weighted imaging (DWI); sagittal magnetization prepared rapid acquisition gradient–echo 3D T1-weighted (T1 TFE 3D) followed by MultiPlanar reconstruction (MPR) in axial, coronal, and sagittal planes. Further details are reported in the methodology study (16).
For the purposes of the present analyses, the following brain MRI lesions were visually rated by a trained and experienced rater:
- Non-lacunar infarcts were assessed in terms of presence and number on T1 and T2 FLAIR sequences as cortical–subcortical lesions in vascular territories.
- The CMBs were detected and counted on axial gradient–echo T2 sequences and classified according to microbleed anatomical rating scale (MARS) (22).
- Lacunes of presumed vascular origin were detected and counted on T1 and T2 FLAIR sequences according to STRIVE criteria (23).
Statistical Analyses
The descriptive analyses (frequencies and percentages or means and standard deviations) were carried out to describe the total cohort in terms of demographics, vascular risk factors, comorbidities, and motor, cognitive, and neuroimaging characteristics.
For statistical purposes, the following variables were dichotomized as follows:
- MoCA performance “impaired” (ES = 0) vs. “normal” (ES ≥ 1) (18).
- Motor performance “impaired” (SPPB score ≤ 10) vs. “normal” (SPPB score >10) (24).
- Depressive symptoms “present” (GDS score > 5) vs. “absent” (GDS score ≤ 5) (25).
- Non-lacunar infarcts absent vs. ≥ 1.
- Cerebral microbleeds absent vs. ≥ 1.
- Lacunar infarcts absent vs. ≥1.
The imaging variables were the three study outcomes for which we evaluated the predictive ability, in terms of sensitivity and specificity, of CHA2DS2-VASc and HAS–BLED. The two scales were evaluated separately.
Algorithms in the R Environment
Version 4.1.0 of R (foundation for statistical computing) was used for the development of dedicated algorithms to test the possible predictive value of the two clinical scales on the presence of the three neuroimaging markers, according to the following phases:
Phase 1: Predictive Ability of CHA2DS2-VASc and HAS–BLED
In this phase, the effectiveness of CHA2DS2-VASc and HAS–BLED scales was tested in relation to the following three study outcomes: Non-lacunar infarcts, CMBs, and lacunar infarcts. Weights associated to each scale item were maintained as originally proposed in literature (3, 6). For both scales, the algorithm returned the sensitivity and the specificity computed by varying all possible scores that each scale can assume.
Phase 2: Changing Weights of CHA2DS2-VASc and HAS–BLED Items
Analyses of Phase 1 were repeated modifying weights assigned to the individual items of each scale. We assumed that the weight could change from 0 (excluded item) to 4 (maximum weight), and only integer values were used. A matrix containing all possible combinations of weights was created. Subsequently, the algorithm attributed the various combinations of weights to the variables and calculated, for each combination, the predictive capability using the Youden index (J) (26). The algorithm identified the combinations “frontier,” i.e., combinations of weights with the maximum Youden index. Then, among all the possible scores that can be assumed by each “frontier” combination, the algorithm looked for the score with the maximum Youden index associated. Such score will be used in prediction as a “cutoff” point, that is the bisection point between a prediction of absence of the outcome with respect to its presence. In relation to the “frontier” combinations and the relative “cutoffs” the relative sensitivity and specificity was calculated. At the end of the computational process, among the “frontier” combinations, we selected the ones with maximum sensitivity. In conclusion, for each scale, the algorithm returned performance indexes (sensitivity and specificity) of the best combinations of weights obtained by maximizing the sensitivity for each neuroimaging outcome.
Phase 3: Adding New Items to CHA2DS2-VASc and HAS–BLED
In the third phase, we tested the effect of the addition of three clinical variables to the scales. The included variables in this testing were as follows: “MoCA impaired performance,” “impaired motor performance” and “presence of depressive symptoms.” Starting from the weight matrix, after the integration of these new variables, the algorithm was repeated for each scale. The predictive ability of the modified scales was expressed in terms of sensitivity and specificity.
Results
Results refer to 170 patients (mean age 77.7 ± 6.8 years, females n = 60, 35%) enrolled in Strat-AF study with complete clinical and brain MRI information. Table 1 shows the demographic and clinical characteristics at baseline.
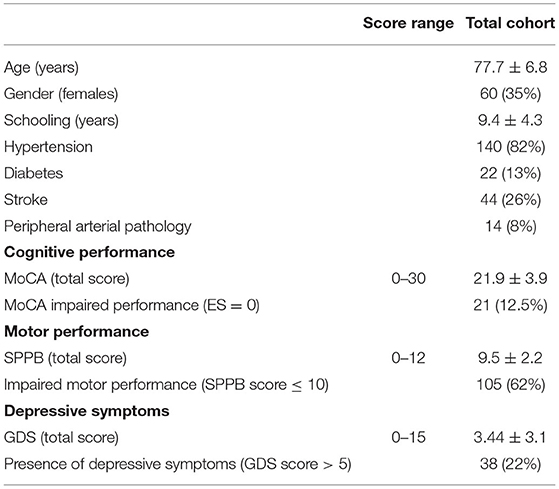
Table 1. Demographic and clinical characteristics, cognitive, and motor performances; and depressive symptoms of the baseline Strat-AF study cohort (n = 170).
Table 2 shows the frequency distributions of CHA2DS2-VASc and HAS–BLED items. The mean total score was 3.7 ± 1.5 for CHA2DS2-VASc and 1.8 ± 0.8 for HAS–BLED. As per the inclusion criteria, all patients were older than 65 years, and 61% older than 75 years. Among the risk factors, 82% of patients had a history of hypertension, which was defined as uncontrolled in 10. Table 1 shows cognitive and motor performances and depressive symptoms at baseline.
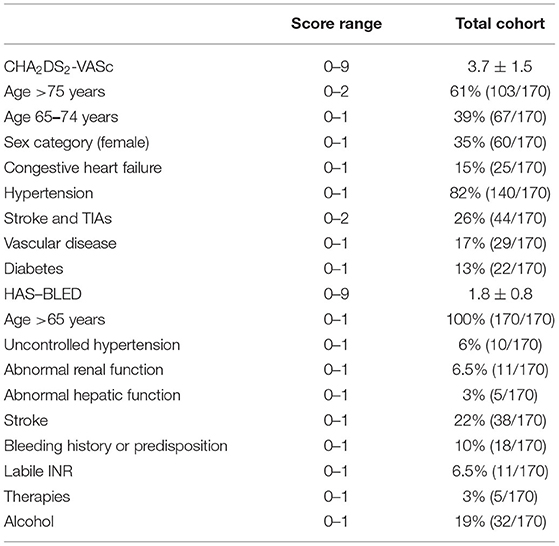
Table 2. Frequency distributions of CHA2DS2-VASc and HAS–BLED items in the baseline Strat-AF study cohort (n = 170).
On brain MRI, 46 patients (27%) had at least one CMB, 54 (32%) at least one lacunar infarct, and 53 (31%) at least one non-lacunar infarct. Figure 1 shows distributions according to the lesion number for each outcome.
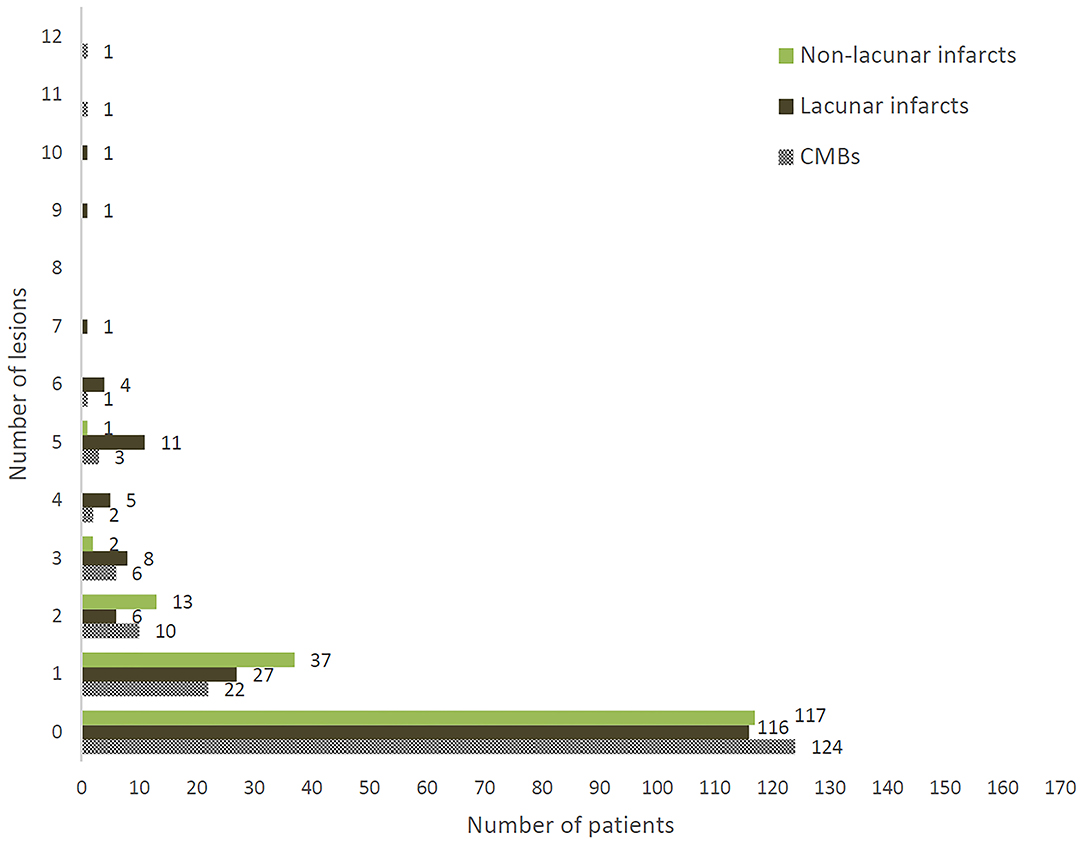
Figure 1. Distribution of number of CMBs, lacunar infarcts, and non-lacunar infarcts in 170 patients enrolled in Strat-AF study.
Phase 1: Predictive Ability of CHA2DS2-VASc and HAS–BLED
The R algorithms returned sensitivity and specificity for all possible combinations of scores assumed by each scale. According to our data, the score ranges went from 0, i.e., absence of all clinical variables, to the maximum value that the scale would assume when all clinical variables were simultaneously present. As reported in Table 3, both scales had a poor predictive ability for each outcome as shown by sensitivity and specificity values.
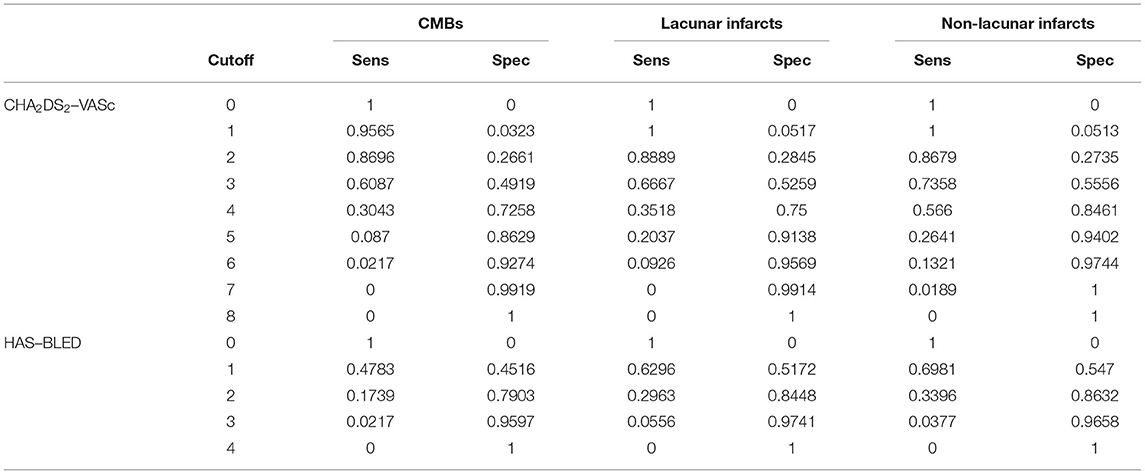
Table 3. Sensitivity and specificity of CHA2DS2-VASc and HAS–BLED scales in relation to the three neuroimaging outcomes.
Phase 2: Changing Weights of CHA2DS2-VASc and HAS–BLED Items
Table 4 reports CHA2DS2-VASc and HAS–BLED accuracy indexes computed by our R algorithms, maximizing sensitivity (see “Methods” section) for each neuroimaging outcome. Overall, the predictive ability was not improved since the sensitivity values ranged from about 0.61–0.63 for CHA2DS2-VASc and from about 0.24–0.60 for HAS–BLED.

Table 4. Sensitivity and specificity of re-weighted CHA2DS2-VASc and HAS–BLED scales in relation to the three neuroimaging outcomes.
Supplementary Tables 1–6 report the most excluded items within the best combinations obtained for each outcome. Briefly, for CMBs, no variable was excluded from CHA2DS2-VASc, while abnormal renal function, INR, drugs and alcohol were the mostly excluded from HAS–BLED. For lacunar infarcts, sex and diabetes were the items more frequently excluded from CHA2DS2-VASc while uncontrolled hypertension, abnormal hepatic function, and drugs were excluded from HAS–BLED. Finally, for non-lacunar infarcts, items more frequently excluded were age, hypertension, vascular disease, and diabetes from CHA2DS2-VASc, and bleeding history or predisposition, drugs, alcohol and age ≥ 75 years from HAS–BLED.
Phase 3: Adding New Items to CHA2DS2-VASc and HAS–BLED
Table 5 reports CHA2DS2-VASc and HAS–BLED accuracy indexes after re-weighting the original items and after the addition of the clinical ones, i.e., MoCA, SPPB, and GDS.

Table 5. Sensitivity and specificity of re-weighted CHA2DS2-VASc and HAS–BLED scales with the addition of MoCA, SPPB, and GDS in relation to the three neuroimaging outcomes.
Overall, HAS–BLED predictive ability was not improved since the sensitivity values still ranged from about 0.24 to 0.64. The CHA2DS2-VASc predictive ability for CMBs was confirmed as insufficient (sensitivity = 0.67, specificity = 0.64). However, CHA2DS2-VASc achieved an adequate sensitivity (0.74) but a low specificity (0.61) for lacunar infarcts and a sufficient predictive ability (sensitivity = 0.70, specificity = 0.85) for non-lacunar infarcts.
Supplementary Tables 8, 9 report the weights of the individual items for all the best combinations of re-weighted CHA2DS2-VASc with the addition of MoCA, SPPB, and GDS in relation to lacunar and non-lacunar infarcts. Focusing on the three new variables, MoCA was mostly excluded by the R algorithms while GDS retained a high weight for lacunar infarcts and SPPB for non-lacunar infarcts. As expected, the weights for stroke/TIA were consistently high across algorithms.
Discussion
This study used ad hoc R algorithms to test the predictive ability of currently recommended risk stratification scales, CHA2DS2-VASc and HAS–BLED, on the presence of brain MRI lesions related to SVD and non-lacunar infarcts in elderly patients with AF on oral anticoagulants. Overall, the results showed a poor predictive ability of CHA2DS2-VASc and HAS–BLED for all outcomes. This was true when scoring the scales according to the original method (Phase 1) and also after changing the weights of each item (Phase 2). We also tested if the addition of the new clinical information, such as cognitive, motor and depressive scores, which are typical clinical hallmarks of cerebrovascular lesions, i.e., SVD markers and non-lacunar infarcts, could improve the performance indexes. Our results seem to point toward a potential role of motor and depressive scores in ameliorating CHA2DS2-VASc predictive ability for lacunar and non-lacunar infarcts. Un-expectedly, the global cognitive efficiency did not increase the predictive ability for any outcomes.
Data from our single center observational study are probably not surprising considering that CHA2DS2-VASc and HAS–BLED were not developed to predict imaging features of SVD. The prediction of such imaging features is a very complex issue, as is that of bleeding and thromboembolic events in elderly patients with AF who take anticoagulants. The topics should be addressed in large, multicentre, prospective studies, possibly with external validation in order to achieve possible answers to such difficult questions.
Concerning bleeding risk score, in Strat-AF we did not achieve any improvement for the hemorrhagic counterpart, i.e., HAS–BLED and CMB. Recently, the limitations of available bleeding risk scores have been the subject of discussion (27). Actually, no bleeding risk score has a clinical impact, i.e., physicians should not change the decision to prescribe anticoagulants in high stroke risk patients. The data of bleeding scores mainly derive from the patients on warfarin. Indeed, intracerebral bleeding risk has been reduced by the therapeutic use of direct oral anticoagulants. The possibility of improving the measurement of thrombotic risk is very important in order to better manage elderly patients avoiding no-treatment or under-treatment (low dosage oral anticoagulants or aspirin). From a clinical point of view, the improvement of the validity of thromboembolic risk stratification schemes on anticoagulant treatment decisions is of upmost relevance.
The research efforts are oriented toward the identification of the real predictive value of SVD-related MRI lesions, focusing on lesion load and possible threshold values, on the prediction of hemorrhagic and thromboembolic risk. The relevance of SVD-related MRI lesions on the prediction of an increased risk of intracerebral hemorrhage in patients with previous stroke and AF has been previously demonstrated in two studies, namely, CROMIS-2 and HERO (11, 12). Still, in asymptomatic elderly patients with AF available evidence does not support the routine use of brain MRI, also from a cost-effectiveness point of view. The development of a method able to identify the subgroup of patients that could maximally benefit from this “brain” additional information in risk stratification would be an optimal strategy for the real-world setting. The clinical variable we tested, cognitive, motor, and depressive scores, are based on widely used and easy to administer instruments.
The limitations of this study needs to be considered. First of all, the use of predictive algorithms required ad hoc computational resources able to estimate thousands of possible combinations, and such technical requirements limited our possibilities to add new clinical information to the risk stratification scales. Furthermore, our limited sample size and possible data overfitting impose caution in the interpretation of results. Neuroimaging outcomes could only be analysed as absent vs. present; thus, without considering the burden of multiple and/or coexistent lesions. Last but not the least, the data on motor performances and depressive symptoms have been registered in patients already on oral anticoagulants after AF diagnosis. Therefore, we do not know if they have a predictive role on MRI alterations. Further studies should confirm these results in AF populations anticoagulant–naive or with CHA2DS2-VASc scores 1 or 2 (males/females) for whom the net clinical benefit of anticoagulants is not clear.
Further investigations on large samples of patients are needed. The availability of big datasets would allow the use of artificial intelligence methods, e.g., machine and deep learning approaches. Moreover, instead of considering just clinical variables, the inclusion of circulating biological markers within these algorithms might augment the predictive ability. At present, we are carrying out a study that represents a continuation of the original Strat-AF. The Strat-AF 2 study (http://www.strat-af.it/2/) will offer the opportunity to analyze both longitudinal data, e.g., progression of cerebrovascular lesions burden, a wide pattern of circulating and omics biomarkers, and finally to test the new set of predictors.
Our results highlight the complexities about generating evidence to support precision medicine for elderly patients with AF (15). Brain MRI in the elderly patients with AF will not be considered in routine clinical practice until there are robust data to support the use of MRI biomarkers to predict the balance of risks of future ischemic and hemorrhagic events. In this sense, future research efforts are needed for the possible identification of elderly patients with AF who might benefit from the knowledge of additional information related to covert cerebrovascular burden.
Data Availability Statement
The datasets presented in this article are not readily available because they are controlled by the Careggi University Hospital. Requests to access the datasets should be directed to AP, anna.poggesi@unifi.it.
Ethics Statement
The studies involving human participants were reviewed and approved by Comitato Etico Area Vasta Centro, Regione Toscana. The patients/participants provided their written informed consent to participate in this study.
Author Contributions
AP, ES, BB, EB, GDF, and RM: conceptualization. AP, ES, BB, EB, and GDF: methodology. EB, GDF, and BB: formal analysis. CB, BF, and SC: investigation. EB, GDF, CB, BF, SC, and ES: data curation. EB, GDF, AP, ES, and BB: original draft preparation. EF, FC, SD, BG, AG, SC, CM, FP, GP, CS, and RM: review and editing of manuscript. AP and BB: supervision. AP: funding acquisition. All authors have read and agreed to the published version of the manuscript.
Funding
This research was funded by Tuscany region and Italian Ministry of Health under Grant Aimed Research Call “Bando Ricerca Finalizzata 2013” GR-2013-02355523; Title of the project, “Role of biological markers for cerebral risk stratification in patients with AF on oral anticoagulants for primary or secondary prevention of ischemic stroke”.
Strat-af Study Group
Coordinating Group
Anna Poggesi (Principal Investigator), Emilia Salvadori, Francesca Cesari, Rossella Marcucci, and Stefano Diciotti.
Collaborators in Alphabetical Order
Carmen Barbato, Giorgio Busto, Eleonora Camilleri, Stefano Chiti, Samira Donnini, Enrico Fainardi, Benedetta Formelli, Francesco Galmozzi, Silvia Galora, Andrea Ginestroni, Betti Giusti, Anna Maria Gori, Elisa Grifoni, Invano Lombardo, Chiara Marzi, Anna Melone, Damiano Mistri, Marco Moretti, Francesca Pescini, Giovanni Pracucci, Valentina Rinnoci, Cristina Sarti.
Conflict of Interest
The authors declare that the research was conducted in the absence of any commercial or financial relationships that could be construed as a potential conflict of interest.
Publisher's Note
All claims expressed in this article are solely those of the authors and do not necessarily represent those of their affiliated organizations, or those of the publisher, the editors and the reviewers. Any product that may be evaluated in this article, or claim that may be made by its manufacturer, is not guaranteed or endorsed by the publisher.
Supplementary Material
The Supplementary Material for this article can be found online at: https://www.frontiersin.org/articles/10.3389/fneur.2022.883786/full#supplementary-material
Supplementary Table 1. Weights of individual items, Youden index, cut-off, sensitivity, and specificity for all best combinations of re-weighted CHA2DS2-VASc in relation to CMBs (Phase 2).
Supplementary Table 2. Weights of individual items, Youden index, cut-off, sensitivity, and specificity for all best combinations of re-weighted CHA2DS2-VASc in relation to lacunar infarcts (Phase 2).
Supplementary Table 3. Weights of individual items, Youden index, cut-off, sensitivity, and specificity for all best combinations of re-weighted CHA2DS2-VASc in relation to non-lacunar infarcts (Phase 2).
Supplementary Table 4. Weights of individual items, Youden index, cut-off, sensitivity, and specificity for all best combinations of re-weighted HAS–BLED in relation to CMBs (Phase 2).
Supplementary Table 5. Weights of individual items, Youden index, cut-off, sensitivity, and specificity for all best combinations of re-weighted HAS–BLED in relation to lacunar infarcts (Phase 2).
Supplementary Table 6. Weights of individual items, Youden index, cut-off, sensitivity, and specificity for all best combinations of re-weighted HAS–BLED in relation to non-lacunar infarcts (Phase 2).
Supplementary Table 7. Weights of individual items, Youden index, cut-off, sensitivity, and specificity for all best combinations of re-weighted CHA2DS2-VASc with the addition of MoCA, SPPB, and GDS in relation to CMBs (Phase 3).
Supplementary Table 8. Weights of individual items, Youden index, cut-off, sensitivity, and specificity for all best combinations of re-weighted CHA2DS2-VASc with the addition of MoCA, SPPB, and GDS in relation to lacunar infarcts (Phase 3).
Supplementary Table 9. Weights of individual items, Youden index, cut-off, sensitivity, and specificity for all best combinations of re-weighted CHA2DS2-VASc with the addition of MoCA, SPPB, and GDS in relation to non-lacunar infarcts (Phase 3).
Supplementary Table 10. Weights of individual items, Youden index, cut-off, sensitivity, and specificity for all best combinations of re-weighted HAS–BLED with the addition of MoCA in relation to CMBs (Phase 3).
Supplementary Table 11. Weights of individual items, Youden index, cut-off, sensitivity, and specificity for all best combinations of re-weighted HAS–BLED with the addition of MoCA in relation to lacunar infarcts (Phase 3).
Supplementary Table 12. Weights of individual items, Youden index, cut-off, sensitivity, and specificity for all best combinations of re-weighted HAS–BLED with the addition of MoCA in relation to non-lacunar infarcts (Phase 3).
References
1. January CT, Wann LS, Alpert JS, Calkins H, Cigarroa JE, Cleveland JC Jr, et al. 2014 AHA/ACC/HRS guideline for the management of patients with atrial fibrillation: a report of the American College of Cardiology/American Heart Association task force on practice guidelines and the heart rhythm society. J Am Coll Cardiol. (2014) 130:2071–104. doi: 10.1161/CIR.0000000000000040
2. January CT, Wann LS, Calkins H, Chen LY, Cigarroa JE, Cleveland JC Jr, et al. 2019 AHA/ACC/HRS Focused Update of the 2014 AHA/ACC/HRS Guideline for the management of patients with atrial fibrillation: a report of the American College of Cardiology/American Heart Association Task Force on Clinical Practice Guidelines and the heart rhythm society in collaboration with the society of thoracic surgeons. Circulation. (2019) 140:e125–51. doi: 10.1161/CIR.0000000000000665
3. Lip GY, Nieuwlaat R, Pisters R, Lane DA, Crijns HJ. Refining clinical risk stratification for predicting stroke and thromboembolism in atrial fibrillation using a novel risk factor-based approach: the euro heart survey on atrial fibrillation. Chest. (2010) 137:263–72. doi: 10.1378/chest.09-1584
4. Camm AJ, Lip GY, De Caterina R, Savelieva I, Atar D, Hohnloser SH, et al. 2012 focused update of the ESC Guidelines for the management of atrial fibrillation: an update of the 2010 ESC Guidelines for the management of atrial fibrillation. Developed with the special contribution of the European Heart Rhythm Association. Eur Heart J. (2012) 33:2719–47. doi: 10.1093/eurheartj/ehs253
5. Kirchhof P, Benussi S, Kotecha D, Ahlsson A, Atar D, Casadei B, et al. 2016 ESC Guidelines for the management of atrial fibrillation developed in collaboration with EACTS. Eur Heart J. (2016) 37:2893–962. doi: 10.1093/eurheartj/ehw210
6. Pisters R, Lane DA, Nieuwlaat R, de Vos CB, Crijns HJ, Lip GY, et al. novel user-friendly score (HAS-BLED) to assess 1-year risk of major bleeding in patients with atrial fibrillation: the Euro Heart Survey. Chest. (2010) 138:1093–100. doi: 10.1378/chest.10-0134
7. Lip GY, Lane DA. Stroke prevention in atrial fibrillation: a systematic review. JAMA. (2015) 313:1950–62. doi: 10.1001/jama.2015.4369
8. Pantoni L. Cerebral small vessel disease: from pathogenesis and clinical characteristics to therapeutic challenges. Lancet Neurol. (2010) 9:689–701. doi: 10.1016/S1474-4422(10)70104-6
9. Gupta A, Giambrone AE, Gialdini G, Finn C, Delgado D, Gutierrez J, et al. Silent brain infarction and risk of future stroke: a systematic review and meta-analysis. Stroke. (2016) 47:719–25. doi: 10.1161/STROKEAHA.115.011889
10. Haeusler KG, Wilson D, Fiebach JB, Kirchhof P, Werring DJ. Brain MRI to personalise atrial fibrillation therapy: current evidence and perspectives. Heart. (2014) 100:1408–13. doi: 10.1136/heartjnl-2013-305151
11. Wilson D, Ambler G, Shakeshaft C, et al. Cerebral microbleeds and intracranial haemorrhage risk in patients anticoagulated for atrial fibrillation after acute ischaemic stroke or transient ischaemic attack (CROMIS-2): a multicentre observational cohort study. Lancet Neurol. (2018) 17:539–47. doi: 10.1016/S1474-4422(18)30145-5
12. Martí-Fàbregas J, Medrano-Martorell S, Merino E, et al. MRI predicts intracranial hemorrhage in patients who receive long-term oral anticoagulation. Neurology. (2019) 92:e2432–43. doi: 10.1212/WNL.0000000000007532
13. Diener HC, Selim MH, Molina CA, Greenberg SM. Embolic stroke, atrial fibrillation, and microbleeds: is there a role for anticoagulation? Stroke. (2016) 47:904–7. doi: 10.1161/STROKEAHA.115.011150
14. Shuaib A, Akhtar N, Kamran S, Camicioli R. Management of cerebral microbleeds in clinical practice. Transl Stroke Res. (2019) 10:449–57. doi: 10.1007/s12975-018-0678-z
15. Currie G, Delles C. Precision medicine and personalized medicine in cardiovascular disease. Adv Exp Med Biol. (2018) 1065:589–605. doi: 10.1007/978-3-319-77932-4_36
16. Poggesi A, Barbato C, Galmozzi F, Camilleri E, Cesari F, Chiti S, et al. Role of biological markers for cerebral bleeding risk STRATification in patients with atrial fibrillation on oral anticoagulants for primary or secondary prevention of ischemic stroke (Strat-AF Study): study design and methodology. Medicina. (2019) 55:626. doi: 10.3390/medicina55100626
17. Nasreddine ZS, Phillips NA, Bédirian V, Charbonneau S, Whitehead V, Collin I, et al. The Montreal Cognitive Assessment, MoCA: a brief screening tool for mild cognitive impairment. J Am Geriatr Soc. (2005) 53:695–9. doi: 10.1111/j.1532-5415.2005.53221.x
18. Conti S, Bonazzi S, Laiacona M, Masina M, Coralli MV. Montreal cognitive assessment (MoCA)-Italian version: regression based norms and equivalent scores. Neurol Sci. (2015) 36:209–14. doi: 10.1007/s10072-014-1921-3
19. Capitani E, Laiacona M. Composite neuropsychological batteries and demographic correction: standardization based on equivalent scores, with a review of published data. The italian group for the neuropsychological study of ageing. J Clin Exp Neuropsychol. (1997) 19:795–809. doi: 10.1080/01688639708403761
20. Guralnik JM, Simonsick EM, Ferrucci L, Glynn RJ, Berkman LF, Blazer DG, et al. A short physical performance battery assessing lower extremity function: association with self-reported disability and prediction of mortality and nursing home admission. J Gerontol. (1994) 49:M85–94. doi: 10.1093/geronj/49.2.M85
22. Gregoire SM, Chaudhary UJ, Brown MM, Yousry TA, Kallis C, Jäger HR, et al. The microbleed anatomical rating scale (MARS): reliability of a tool to map brain microbleeds. Neurology. (2009) 73:1759–66. doi: 10.1212/WNL.0b013e3181c34a7d
23. Wardlaw JM, Smith EE, Biessels GJ, Cordonnier C, Fazekas F, Frayne R, et al. Neuroimaging standards for research into small vessel disease and its contribution to ageing and neurodegeneration. Lancet Neurol. (2013) 12:822–38. doi: 10.1016/S1474-4422(13)70124-8
24. Pavasini R, Guralnik J, Brown JC, di Bari M, Cesari M, Landi F, et al. Short physical performance battery and all-cause mortality: systematic review and meta-analysis. BMC Med. (2016) 14:215. doi: 10.1186/s12916-016-0763-7
25. Dennis M, Kadri A, Coffey J. Depression in older people in the general hospital: a systematic review of screening instruments. Age Ageing. (2012) 41:148–54. doi: 10.1093/ageing/afr169
Keywords: atrial fibrillation, anticoagulation, stroke, intracerebral hemorrhage, cerebral small vessel disease, brain MRI, CHA2DS2-VASc scale, HAS-BLED scale
Citation: Bianconi E, Del Freo G, Salvadori E, Barbato C, Formelli B, Pescini F, Pracucci G, Sarti C, Cesari F, Chiti S, Diciotti S, Gori AM, Marzi C, Fainardi E, Giusti B, Marcucci R, Bertaccini B and Poggesi A (2022) Can CHA2DS2-VASc and HAS–BLED Foresee the Presence of Cerebral Microbleeds, Lacunar and Non-Lacunar Infarcts in Elderly Patients With Atrial Fibrillation? Data From Strat–AF Study. Front. Neurol. 13:883786. doi: 10.3389/fneur.2022.883786
Received: 25 February 2022; Accepted: 13 April 2022;
Published: 13 May 2022.
Edited by:
Andrea Morotti, Neurological Institute Foundation Casimiro Mondino (IRCCS), ItalyReviewed by:
Tom Moullaali, University of Edinburgh, United KingdomMarina Diomedi, University of Rome Tor Vergata, Italy
Copyright © 2022 Bianconi, Del Freo, Salvadori, Barbato, Formelli, Pescini, Pracucci, Sarti, Cesari, Chiti, Diciotti, Gori, Marzi, Fainardi, Giusti, Marcucci, Bertaccini and Poggesi. This is an open-access article distributed under the terms of the Creative Commons Attribution License (CC BY). The use, distribution or reproduction in other forums is permitted, provided the original author(s) and the copyright owner(s) are credited and that the original publication in this journal is cited, in accordance with accepted academic practice. No use, distribution or reproduction is permitted which does not comply with these terms.
*Correspondence: Anna Poggesi, anna.poggesi@unifi.it
†These authors have contributed equally to this work