- 1 Istituto di Linguistica Computazionale “Antonio Zampolli,” Consiglio Nazionale delle Ricerche, Pisa, Italy
- 2 Istituto di Scienze e Tecnologie della Cognizione, Consiglio Nazionale delle Ricerche, Roma, Italy
- 3 Università di Siena, Siena, Italy
Traditional theories of decision-making assume that utilities are based on the intrinsic value of outcomes; in turn, these values depend on associations between expected outcomes and the current motivational state of the decision-maker. This view disregards the fact that humans (and possibly other animals) have prospection abilities, which permit anticipating future mental processes and motivational and emotional states. For instance, we can evaluate future outcomes in light of the motivational state we expect to have when the outcome is collected, not (only) when we make a decision. Consequently, we can plan for the future and choose to store food to be consumed when we expect to be hungry, not immediately. Furthermore, similarly to any expected outcome, we can assign a value to our anticipated mental processes and emotions. It has been reported that (in some circumstances) human subjects prefer to receive an unavoidable punishment immediately, probably because they are anticipating the dread associated with the time spent waiting for the punishment. This article offers a formal framework to guide neuroeconomic research on how prospection affects decision-making. The model has two characteristics. First, it uses model-based Bayesian inference to describe anticipation of cognitive and motivational processes. Second, the utility-maximization process considers these anticipations in two ways: to evaluate outcomes (e.g., the pleasure of eating a pie is evaluated differently at the beginning of a dinner, when one is hungry, and at the end of the dinner, when one is satiated), and as outcomes having a value themselves (e.g., the case of dread as a cost of waiting for punishment). By explicitly accounting for the relationship between prospection and value, our model provides a framework to reconcile the utility-maximization approach with psychological phenomena such as planning for the future and dread.
1 Introduction
In line with the expected utility theory (EUT), most economic and neuroeconomic models view decision-making as aimed at the maximization of expected utility (von Neumann and Morgenstern, 1944). With regard to the computational processes involved in utility assignment and choice, it has been proposed that the brain can use at least two instrumental controllers: a habitual mechanism, which retrieves the cached values of actions that have successfully led to reward in similar contexts, and a goal-directed mechanism, which explicitly calculates and compares the costs of actions and the values of their outcomes. Both mechanisms have been studied within the reinforcement learning (RL) framework (Sutton and Barto, 1998). Habitual and goal-directed controllers have been described with model-free and model-based RL methods, respectively (Daw et al., 2005). Both controllers (aim to) maximize reward, but the former (learns and) uses action–value associations, whereas the latter (learns and) uses action–outcome and outcome–value associations. Although these two systems co-exist and compete, the former tends to be selected only in simple environments and after sufficient experience is acquired, whereas the latter is mostly selected in novel or more dynamic environments (Daw et al., 2005). Because they represent action–outcome transitions explicitly, goal-directed controllers have been traditionally linked to planning, which requires the mental generation and exploration of possible alternative courses of actions (or more generally future events).
It has been reported that the brain (e.g., the orbitofrontal cortex) represents subjective reward values during goal-directed decision-making (Padoa-Schioppa and Assad, 2006, 2008; Kable and Glimcher, 2007). However, why values are assigned to certain outcomes remains unclear. Recent computational models suggest that animals’ motivations are responsible for assigning specific utilities to outcomes. It follows that different motivational states may correspond to different utility functions. In this regard, Niv et al. (2006) define motivation as the mapping between outcomes and their utilities, and refer to “motivational states” (e.g., hunger or thirst) as indices of such different mappings, as one in which foods are mapped to high utilities, and another in which liquids are mapped to high utilities. This means that valuation is influenced by both external factors, such as outcomes and their probability of occurrence, and the internal context (i.e., the motivational, emotional, and cognitive state) of the decision-maker. However, in this framework, only the external factors are explicitly represented by the decision-maker during planning; internal context influences utility assignment only indirectly, as it determines the utility function.
This approach is successful in the case of outcomes collected immediately after choice, since the internal (e.g., motivational) context usually remains the same during the time between choice and delivery of reward. However, in the case of choices that involve delayed outcomes, the decision-maker’s motivation may change during the interval between choice and delivery, hence the value of outcomes may in turn change drastically when they are collected compared to when the choice is made. If an agent does not consider how contextual factors change, it risks obtaining less reward than expected (Loewenstein et al., 2003). For example, consider the following case: when you order a piece of pie at the beginning of a dinner, you are evaluating the pleasure you will receive on the basis of your current hunger, disregarding the fact that at the end of the meal you will be satiated. Eating the pie risks being far less rewarding than expected before, because there is an asymmetry between the value of the pie when you make the choice and when you eat it. To correctly evaluate future events, an agent must simulate future internal (motivational and cognitive) context as well as the future external environment (future outcomes).
Numerous researchers have investigated how humans (and possibly even some non-human animals) anticipate future internal contexts, specifically those related to future mental processes, such as motivational and emotional states. These abilities have been related to various concepts, including “mental time travel,” “episodic time travel,” “self-projection,” “prospection,” and “foresight.” For instance, prospection has been described as the ability to project the self into the future, connected to the episodic memory ability (Buckner and Carroll, 2007; Schacter et al., 2007); see also (Gilbert and Wilson, 2009) for a taxonomy of potential flaws in decision-making associated with prospection abilities. In a similar vein, Suddendorf and Corballis (1997) describe mental time travel as combining prediction and episodic memory; see also Suddendorf (2006). This ability underlies prospective planning, or planning for future needs and circumstances that are independent of the current motivational and perceptual context. For example, we go to the supermarket even when we are not hungry, because we anticipate that we will be hungry at a later stage.
A second way prospection abilities affect decision-making is through anticipation of emotions. First, humans seem able to anticipate pleasure or displeasure associated with a future outcome just by imagining it. This ability has been called pre-feeling (Gilbert and Wilson, 2007). Second, not only pre-feeling is triggered by imagining future outcomes; emotions are also generated by imagining future cognitive processes associated to prospects that are unrelated to outcomes. For instance, we can choose not to achieve a desired goal because we anticipate that it will make us feel guilty, or that we will regret it. Recent neuroscientific research has focused on how anticipated emotions unrelated to outcomes change the utility of prospects. Coricelli et al. (2005) have studied how anticipating regret influences choice. Along similar lines, Berns et al. (2006) reported that subjects preferred to receive an electric shock immediately rather than after a given amount of time; in some cases, subjects preferred a stronger electric shock immediately rather than waiting for a weaker one. According to the experimenters, the subjects assigned negative utility to waiting, because they anticipated their negative emotional state during the waiting time.
The aforementioned phenomena are surprising from the perspective of economic theories that consider the utility of prospects as depending only on the intrinsic value of outcomes. In this article, we propose a computational model that extends utility-maximization theories of decision-making to the case of agents provided with prospection abilities. Our key proposal is that the anticipation of future motivations, emotions, and, more generally, cognitive processes influences the “utility assignment” process, in two ways. First, anticipated future cognitive processes can affect the values of future outcomes (e.g., food will be rewarding only if we are hungry). Second, anticipated cognitive processes can have a value in themselves (e.g., dread has a negative value). In other words, on the one hand the ability of anticipating motivations permits evaluating future outcomes in relation to future internal contexts. On the other hand, anticipated emotions associated with prospects, such as fear, dread, and regret, can be treated by the decision-maker as “outcomes” themselves.
We explore these two aspects of the theory from a computational viewpoint, starting from the computational (Bayesian) model of decision-making proposed by Botvinick and collaborators(Botvinick and An, 2008; Solway and Botvinick, submitted; see Section 2) and extending it with two critical features. In Section 3, we extend the model with a component for anticipating motivational dynamics (called motivational forward model), and test it in three scenarios in which utility related to future motivations has to be considered in the maximization of reward. This model highlights how the same utility-maximization framework can explain present-directed and future-directed choices as dependent on considerations about current and expected motivations, respectively. In Section 4 we extend the model by including the ability to assign a value to anticipated emotional states, and test it in a scenario in which choice has future negative emotional effects (dread) that have to be avoided in order to maximize reward. This model shows that, for an agent provided with prospection abilities, the influence of anticipated emotional factors on decision-making can be incorporated in an utility-maximization framework, rather than considered as an irrational phenomenon. In Section 5, we discuss the implications of our theory for neuroeconomics, and how our computational models can guide the study of the brain mechanisms implied in prospection abilities and associated decision-making processes.
2 The “Baseline Model”: A Bayesian Model of Goal-Directed Decision-Making
The computational models we present extend the Bayesian model of goal-directed decision-making proposed by Botvinick and collaborators(Botvinick and An, 2008; Solway and Botvinick, submitted; hereafter, the baseline model; see Figure 1), which we will introduce here. The authors use the formalism of Dynamic Bayesian Networks (Murphy, 2002) to represent the goal-directed computational processes involved in solving Markov Decision Problems. In particular, they adopt a model-based approach, in which (stochastic) action–outcome and outcome–utility transitions are represented explicitly.
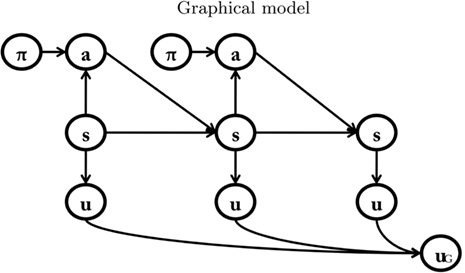
Figure 1. The Bayesian model of goal-directed decision-making proposed by Botvinick and An (2008); Solway and Botvinick (submitted), which we use as our “baseline model. ” See main text for explanation.
Each node represents a discrete random variable and each arrow represents the conditional dependence between two random variables. The model shown in Table 1 and Figure 1 represents the unfolding of three time slices (time indexes are omitted), but the Dynamic Bayesian Networks formalism can be used to design models of arbitrary length. The variables adopted by the baseline model are presented in Table 1: state (s) variables represent the set of world states; action (a) variables represent the set of available actions; policy (Π) variables represent the set of actions associated with a specific state; finally, utility (u) variables represent the utility function corresponding to a given state. Rather than viewing utility as a continuous variable, the baseline model adopts an approach introduced by Cooper (1988) in which utility is represented through the probability of a binary variable. The following linear transformation maps from scalar reward values to p(u/si)
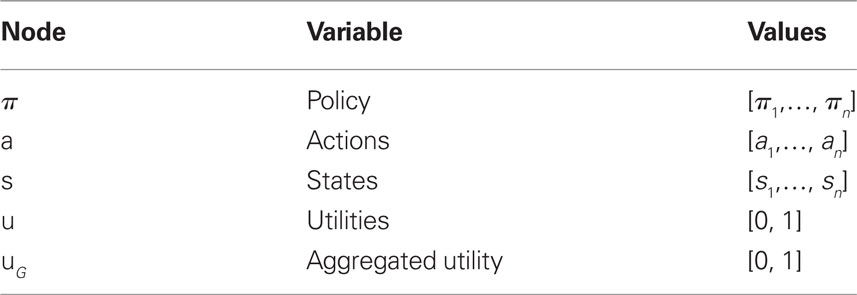
Table 1. List of variables used in Figure 1.

In situations involving sequential actions, this model uses a technique proposed by Shachter and Peot (1992) which allows integrating all rewards in a single representation. This is achieved by introducing a global utility (uG) variable:

where N is the number of u nodes.
Within this model, the utility of alternative courses of action (e.g., a navigation episode in a labyrinth with different rewards in its branches) can be calculated and maximized by a form of probabilistic inference called reward query. In short, the aggregated utility node uG is set to one (its maximum value). Then, a standard probabilistic inference algorithm (belief propagation, Pearl, 2000) is used to compute the posterior probabilities of the policy nodes p. This process is iterated by replacing the prior probability of p with the posterior probability and repeating the inference procedure. The result of reward query is that the optimal policy is computed (see Botvinick and An, 2008; Solway and Botvinick, submitted for more details). For instance, in a double T-maze, which has the highest reward in its upper right corner, the selected policy will encode “go right twice.”
The baseline model successfully replicates data from many animal experiments, including devaluation (Balleine and Dickinson, 1998), labyrinth navigation, latent learning, and detour behavior (Tolman, 1948), all of which are hallmarks of goal-directed behavior. The authors of the model discuss how each of its components can be related to a brain subsystem. They propose that the policy system is implemented by the dorsolateral prefrontal cortex, the action system is implemented by the premotor cortex and the supplementary motor area, the state system by the medial temporal cortex, the medial frontal/parietal cortex and the caudate nucleus, and, finally, the reward system is associated with the orbitofrontal cortex and the basolateral amygdala.
Following an approach that is typical of RL architectures, the baseline model assigns values to outcomes based on the current motivational state of the agent. When the motivational state changes, the utility function changes accordingly and new utility values are assigned; see also Niv et al. (2006). However, the agent is unable to anticipate its future motivational states. In the next section, we describe an extension of the baseline model that can take both present and future motivational states into account during the utility-maximization process.
3 Anticipating Motivations
In order to describe how anticipating motivation influences decision-making, our proposal extends the baseline model (see Table 2; Figure 2) by considering both future and current motivational states. To do this, our model includes a novel component, a motivational forward model that represents explicitly motivational dynamics, which permits an agent to anticipate its motivational states.
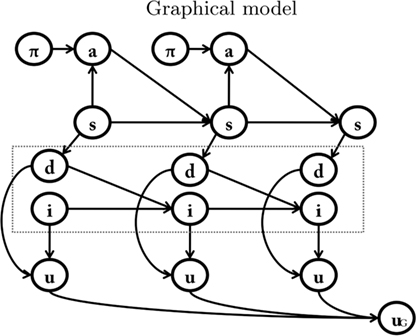
Figure 2. Bayesian model of anticipated motivation. The motivational forward model is inside the box. See main text for explanation.
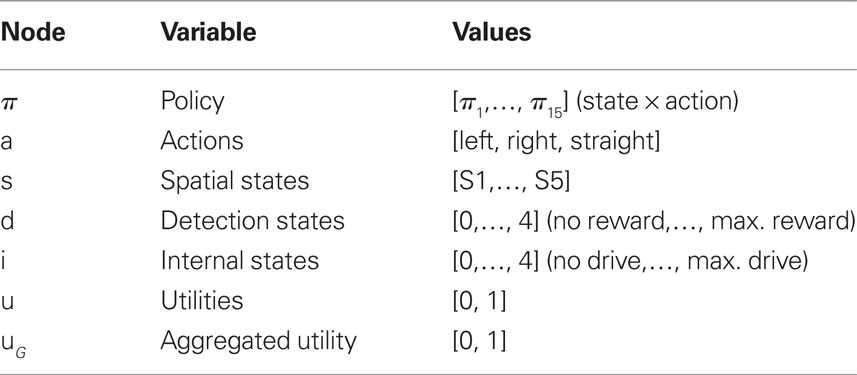
Table 2. List of variables used in Figure 2.
In short, the agent is provided with a simplified homeostatic system (or a system that monitors internal variables that are significant for the survival of the agent), which includes one or more drives, such as hunger, thirst, or sex (Hull, 1943). The motivational forward model explicitly represents the dynamics of the agent’s homeostatic system. Specifically, future motivational states depend jointly on the previous motivational state and on whether (and to what extent) the agent has been satiated or not at the previous time steps.
In the model of anticipated motivation, state nodes are broken down into sub-nodes: spatial states (s), which represent the spatial position, internal states (i) which represent the motivational state, and detection states (d), which record the presence of potential rewards. Different motivations, such as hunger and thirst, have separate motivational state nodes and detection state nodes. For each motivation, the spatial state influences the detection state. In other words, if the food is in a given place, the agent must be in that place (spatial state) to detect it (detection state). The detection state, together with the internal state, influences the internal state at the following time step. For example, at t1 the agent is hungry (internal state) and is in the food place (spatial state). Once the agent detects (detection state) and eats the food, at t2 it is less hungry (internal state at time t2 is lowered). The motivational forward models explicitly represent these transitions, permitting us to infer that, for instance, if at tx I am hungry (internal state) and I see and eat a certain amount of food (detection state), than at tx+1 I am going to be less hungry (proportionally to the amount of food eaten).
Compared to standard RL models, in the model of anticipated motivation the ability to anticipate motivations changes the way utility is assigned. At each time step, utility u depends jointly on the motivational state i and on the potential reward detected d. Each motivation has its own associated utility node u. As in the baseline model, utility is represented as the conditional probability of the binary variable p(u/i,d).
It is worth noting that although the baseline model could in principle account for motivational dynamics by adding motivational variables to the state s, the substantial difference in factoring the graph in the way we propose is that it results in different implied conditional dependence relationships between the parts of the (factorized) state: spatial state versus detection and internal states. Not only does this factorization influence how inference is performed in the graphical model, it also makes explicit claims about the mutual dependencies among components, which is essential for mapping formal models into psychological and neural hypotheses.
3.1 Experiments: Methods and Results
We tested the model of anticipated motivation in three simulated scenarios. Because we considered the case of an agent with two motivations (hunger and thirst), the model includes two separate sets of nodes for internal states [hunger (h) and thirst (t)] and detection states [food (f) and water (w)]; see Table 3 and Figure 3.
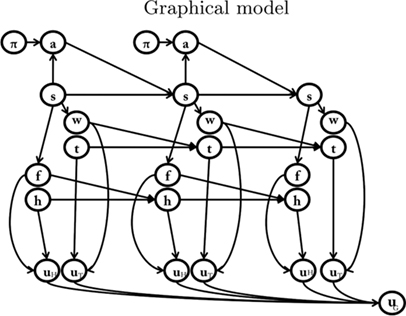
Figure 3. The model of anticipated motivations adopted in the simulations, which includes two drives, that is, hunger and thirst, and two motivational forward models.
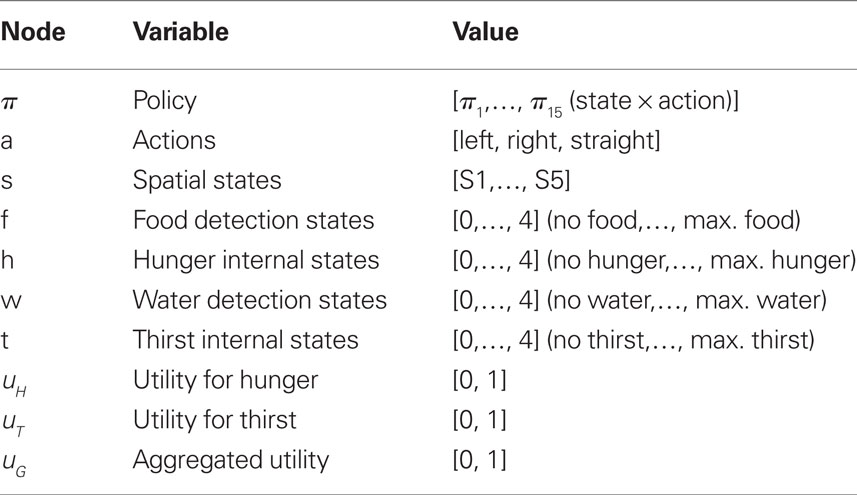
Table 3. List of variables used in Figure 3.
At every time step, the internal node and the detection node of each motivation jointly influence the corresponding utility, as described in the general model. Thus, the model has two utility nodes for each time step: uH and uT, for hunger and thirst, respectively. All utility nodes at all time steps are summed in the global utility node (uG), as in the baseline model.
Considering hunger as a paradigmatic example, “internal state nodes” can assume five values: 0, 1, 2, 3, 4 (0 indicates no hunger and 4 maximum hunger). Similarly “detection nodes” can assume five values: 0, 1, 2, 3, 4 (0 indicates no food detected and 4 maximum food detected). Spatial state values represent positions in a maze and can assume five values in the experiments (S1 to S5). Action values are: “left,” “right,” and “straight.” Policy values correspond to the combination between action and state values. The conditional probabilities of all nodes are deterministic, except p(u/i,d). This implies that if the agent is in a certain position in the maze and makes a certain action, it will go deterministically to another given position. Similarly, if the agent is in a certain position and follows a given policy, it will always make a certain action. The relationship between spatial states and actions depends on the maze configuration (see below).
The value of the nodes in the motivational forward model (of each motivation) are calculated as follows: the value of a detection state depends deterministically on the associated spatial state at that time step (i.e., specifically on the amount of potential reward present in the corresponding position of the maze, see below). The value of the internal state is the difference between the value of the internal state at the previous time-step minus the value of the detection state at the previous time step (if the former is greater than the latter; otherwise it is zero). This accounts for the fact that hunger is decreased by eating (to the same degree as the value of the food eaten). When the value of the internal state at the previous time step is zero, the successive value is raised by 2; this represents the increased hunger associated with the passage of time. Finally, the value of internal state and detection state jointly determine the conditional probability of the utility corresponding to that motivation. Because we model potential rewards that have only positive values in our experiments, utilities range from neutral [p(u = 1/i,d) = 0] and maximally positive [p(u = 1/i,d) = 1]. Nevertheless, it is possible to model a continuum of negative and positive utilities, as in the baseline model, in which negative utilities range between 0 and 0.5, and positive utilities between 0.5 and 1. In our experiments the probability p(u = 1/i,d) is the lowest one between the detection state and the internal state, over 4. For example, if potential reward detected is 2 and motivation is 0, then p(u = 1/i,d) = 0/4; if motivation is 1, then p(u = 1/i,d) = 1/4; if motivation is 2 and potential reward is 4, then p(u = 1/i,d) = 2/4.
Anticipating motivations provides several advantages to an agent. Below we describe three simulated experiments that are intended to test three abilities: (1) strategic planning, or disregarding currently available rewards in favor of higher future ones; (2) considering future motivational switches in the planning process; (3) planning for the future, such as storing food in view of future needs.
3.1.1 Experiment 1: strategic planning
Humans and other animals can act impulsively or strategically. In the former case, they assign outcome values only according to their current preponderant motivational state. In the latter case, they consider a complex prospect of future motivational states and corresponding future rewards. The ability to choose “reflexive” strategies might be more advantageous in complex environments. We argue that a motivational forward model might underlie the ability to assign values according to future motivations, which in turn might lead to selecting courses of actions that maximize reward in the long run.
To test this idea, we designed a simulated experiment in which an agent has to choose between two alternatives: a smaller reward, which satisfies its immediate preponderant motivation (e.g., hunger), and a larger reward, which also satisfies the weaker motivation (e.g., thirst) by postponing the satisfaction of the preponderant one. We hypothesized that in this condition an agent provided with the motivational forward model would be able to maximize its reward, whereas an agent without such a mechanism would select less rewarding, impulsive behavior aimed at satisfying only the preponderant motivation.
The experimental design is illustrated by the T-maze shown in Figure 4A. We considered three time steps: at t0 the agent is in S1; at t1 it can go left to S2 or right to S3; at t2 it goes from S2 to S4 and from S3 to S5. In each of the five positions of the T-maze, a certain amount of food, water, or both can be found. The configuration chosen in our simulation is the following: food 3 in S2, water 2 in S3, food 3 in S4, and food 3 in S5. Then we set the initial internal states as follows: H1 = 4, T1 = 2.
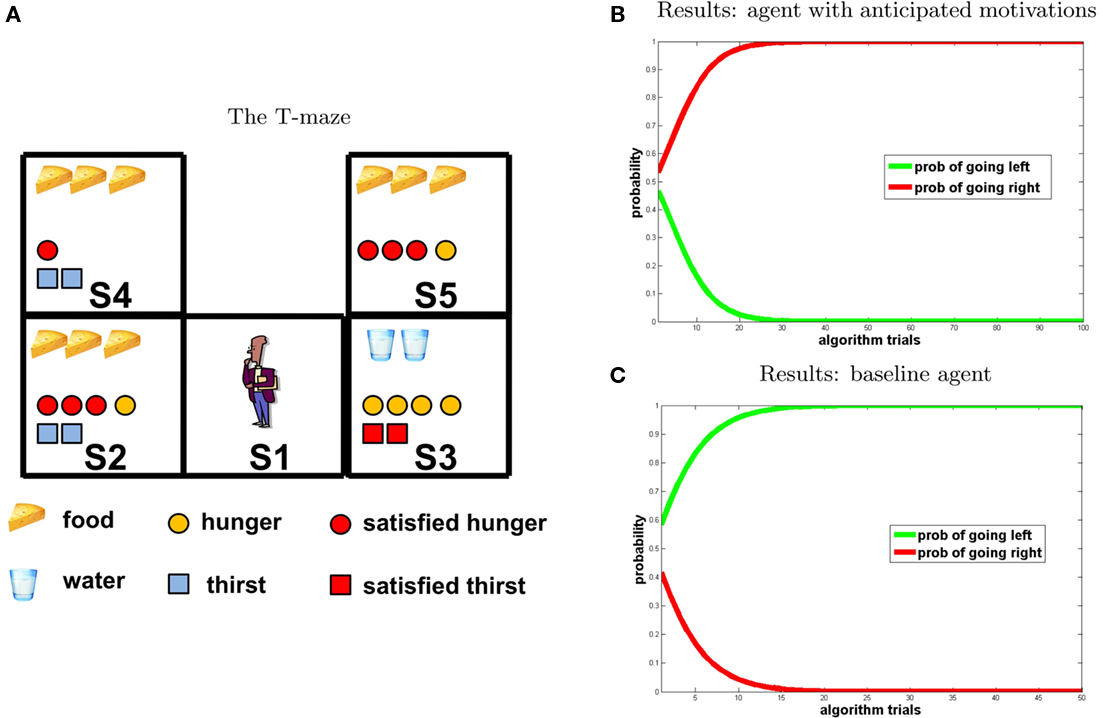
Figure 4. Experiment 1. (A) T-maze. Symbols represent values of detection states and internal states that are computed during the inference process by the agent that anticipates motivation. Potential reward pattern (corresponding to potential reward in each position of the maze) and initial motivational states (corresponding to motivational states in S2 and S3) are set by the experimenter, all further information is computed by reward query. Red forms indicate motivational values that are satiated by consumption of potential rewards in the corresponding position of the maze. Graphically, optimal behavior corresponds with choosing the path with the largest number of red forms. (B,C) Results of the first experiment (B) agent with anticipated motivations; (C) baseline model. The graph represents the probability assigned to the policy associated to “going right” (red) and “going left” (green), respectively, at each iteration of the reward query.
The agent provided with anticipatory motivations is implemented using the graphical model shown in Table 3 and Figure 3. In the experiment, it is compared with the baseline model (shown in Table 1 and Figure 1) in which utility is assigned only to rewards that are congruent with the highest of the actual motivational states of the agent (hunger in this case).
Figures 4B,C, shows the results of the experiment (Figure 4B agent with anticipated motivations; Figure 4C baseline model). The two graphs show that, for the agent provided with anticipated motivations, the probability of selecting the “going right” policy increases monotonically toward one at every iteration of the reward query. By going right, the agent satisfies both thirst (at the second step) and hunger (at the third step). On the contrary, the baseline model, which takes into account only its present motivational state (in this case hunger is higher than thirst), selects an impulsive behavior and goes left toward the immediate maximum amount of reward corresponding to its actual motivation.
Our first simulation describes the motivational forward model as an essential element for the goal-directed ability of shifting from impulsive strategies to more “reflexive” ones. Note however that strategic planning plausibly requires additional mechanisms to exert cognitive control and inhibit prepotent responses (dictated by habitual or Pavlovian mechanisms) before the goal-directed utility-maximization process is completed (Barkley, 2001; Botvinick et al., 2001). These mechanisms are not implemented in our model, because we modeled only the goal-directed aspects of choice. However, they would be necessary in more sophisticated models that include multiple cognitive controllers that interact and compete (Daw et al., 2005; Rigoli et al., 2011).
3.1.2 Experiment 2: considering future motivational switches
The ability to predict future motivations permits taking future changes of motivations into account during the planning process. In turn, this permits predicting that a future outcome will be more or less rewarding, depending on the future motivational context. In keeping with our previous assumptions, we argue that the motivational forward model could be a key mechanism for maximizing reward in situations in which the internal motivational context can change before the outcome is delivered.
To test this idea, we designed a simulated experiment in which an agent has to choose between two alternatives: a path in which the cumulative reward is higher given the current motivation, and a path in which the cumulative reward is higher if one considers how its motivations will change. We hypothesized that an agent provided with the motivational forward model would be able to maximize its reward, whereas an agent without such mechanism would tend to choose the path associated with higher rewards for its current motivation.
The T-maze in Figure 5, left, illustrates the set-up. Here potential reward has the following pattern: food 3 in S2 and S3; food 4 in S4; water 2 in S5. The initial internal states were: H1 = 4; T1 = 0. According to our hypothesis, if a hungry agent (H1 = 4) predicts that in the near future it will be satiated (i.e., it will collect food = 3), it can choose future potential rewards that at the moment seem lower (water = 2 rather than food = 4) but that will be higher when the agent is satiated (remember that in our model if a motivational state value is 0 at ti it will be 2 at ti+1). Our results show that the agent provided with anticipatory motivations maximizes utility.
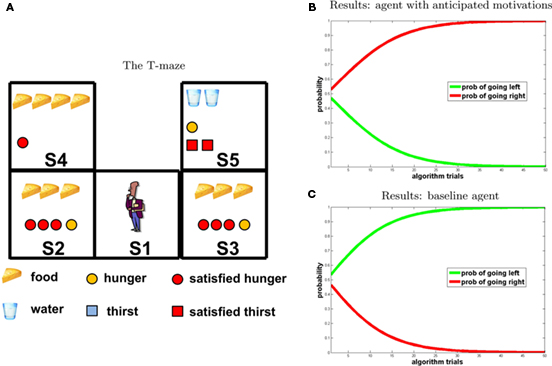
Figure 5. Experiment 2. (A) T-maze. (B,C) Results [(A) agent with anticipated motivations; (B) baseline model], “going right” = red; “going left” = green.
Note that our set-up is conceptually similar to the experiment conducted by Naqshbandi and Roberts (2006), in which squirrel monkeys could eat either four dates or one date. Given that eating dates makes monkeys thirsty, experimenters manipulated the delay between the meal and the availability of water. In the one date case, water was available sooner with respect to the four dates case. Although the monkeys chose four dates at the beginning, they gradually shifted their preference toward one date. It should be noted, however, that the interpretation of this experiment is controversial, as it is still unclear whether the choice was goal-directed or induced by simpler mechanisms (Suddendorf and Corballis, 2008).
3.1.3 Experiment 3: planning for the future
According to the Bischof-Kohler’s hypothesis (Suddendorf and Corballis, 1997), only humans act in a complex and flexible way to achieve rewards in view of future motivations, even if not motivated at the present moment (e.g., going to the supermarket even when not hungry). Contrary to this idea, Raby et al. (2007) argued that even some other animals such as western scrub-jays (Aphelocoma californica) have this ability. In this work, experimenters taught scrub-jays to foresee conditions in which they would receive no food and thus be hungry; after this learning phase, experimenters unexpectedly gave the scrub-jays the chance to cache food. As a result, scrub-jays cached a larger amount of food when they foresaw a future condition of deprivation compared to other conditions, suggesting that they are able to flexibly account for their future motivational states (although it is unclear if they use the same mechanisms as humans, see below).
Our third simulation is conceptually similar to the study of Raby et al. (2007), which aimed to assess the ability of scrub-jays to store potential rewards in view of future motivational states. The authors report that scrub-jays cached food only when they expected future deprivation, suggesting that they consider their future motivations and plan for the future.
The scenario is illustrated in the T-maze of Figure 6: at t0 the agent is in S1; at t1 it can go left (to S2) or right (to S3); at t2 it goes from S2 to S4 and from S3 to S5. Once a potential reward is detected, the agent has two options: to consume it immediately or to consume it later, that is, at the following time steps. Crucially, in our model of anticipated motivation, once the agent detects potential rewards but is not motivated, and at the same time it anticipates that it will be motivated in the future, it stores them (as represented by the padlock symbol in Figure 6). We positioned the following potential rewards: food 3 in S2 and water 1 in S3, and set the initial internal state values to H1 = 0 and T1 = 1 (as shown in Figure 6, left). By going right, an agent can collect a small reward immediately (water). Instead, by going left and storing food (which is automatic in our model if the agent is not currently motivated and anticipates its future hunger) it can collect a higher reward at the next time step, when it will be hungry (note that in our model if a motivational state value is 0 at ti it becomes 2 at ti+1).
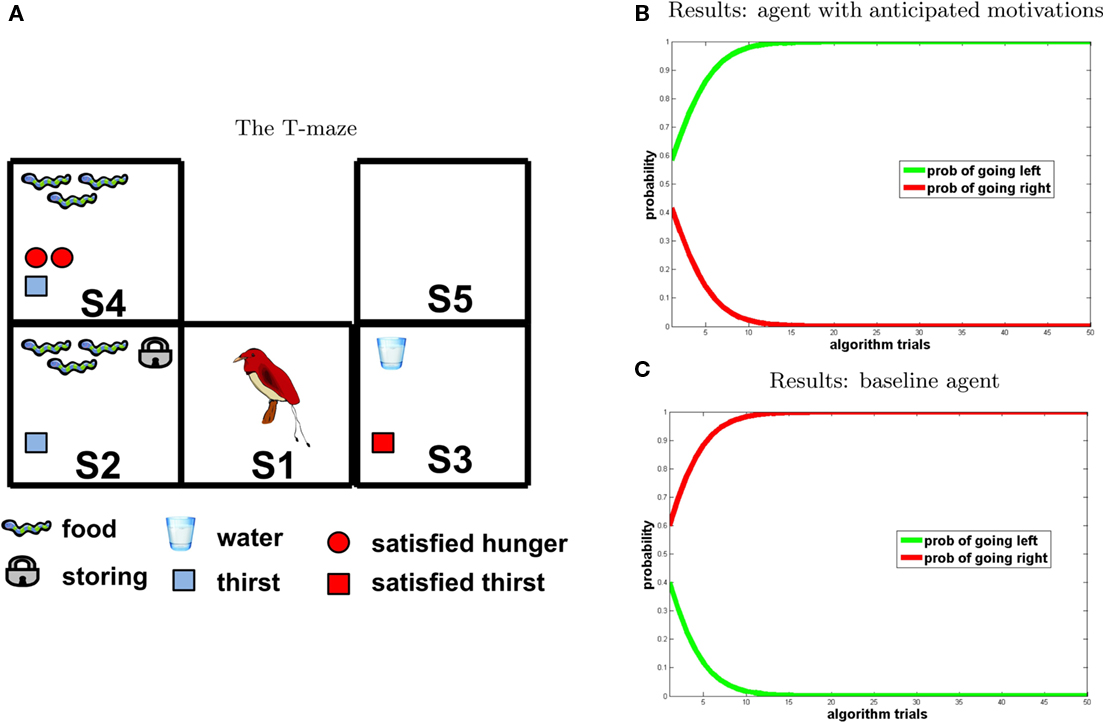
Figure 6. Experiment 3. (A) T-maze. (B,C) Results [(A) agent with anticipated motivations; (B) baseline model], “going right” = red; “going left” = green.
Performance of our model of anticipated motivation is shown in Figure 6B. According to our prediction, the agent chooses to go left, storing a large amount of food and eating it later, instead of immediately drinking a bit of water. In other words, rather than selecting the prepotent response of consuming the immediate reward (water, because it is a little thirsty), it is able to choose the action sequence that leads to higher reward in the future. On the contrary, the baseline agent (Figure 6C) behaves impulsively. The fact that the probability of going right increases toward one indicates that the baseline agent is attracted only by the immediate reward, and is unable to plan instrumental actions leading to the future consumption of a larger amount of reward.
3.2 Discussion
In this section, we have presented a Bayesian model of goal-directed behavior that accounts for future motivations during planning. Our model includes a motivational forward model that permits evaluating outcomes as related to future rather than only current motivations, as is common in RL models (Sutton and Barto, 1998). Indeed, within the RL framework, it has been proposed that motivations change the utility function (Niv et al., 2006). By contrast, in our model motivations are explicitly represented and influence the value of future potential rewards. Specifically, utility values of outcomes depend jointly on potential reward amount and on motivation at the corresponding time, rather than only on the former. Another aspect that distinguishes our model from most RL models is the consideration of multiple motivational dynamics integrated in a unitary utility-maximization process.
In three simulated scenarios, in which choices had distal implications, we show that an agent that anticipates its motivational dynamics is able to gain more reward than an agent that only considers its current motivational state. We propose that the computational mechanism responsible for the prediction of motivational dynamics, the motivational forward model, could be an essential (though not sufficient) element for the implementation of complex prospection abilities such as planning for the future.
The debate on how human and non-human brains represent future motivations during planning is still controversial. Both Raby et al. (2007) and Osvath and Osvath (2008) report evidence suggesting that animals have foresight abilities (but see Suddendorf and Corballis, 2008 for concerns relative to these results). The former study shows that scrub-jays cached food only when they expected a future condition of deprivation. The latter study shows that chimpanzees and orangutans flexibly chose a tool for future use taking future needs into account.
Despite these demonstrations that, at least in some circumstances, some animals plan in view of future needs, whether or not they adopt the same mechanisms as humans is still controversial. Suddendorf and Corballis(1997, 2007) proposed the “mental time travel hypothesis” to interpret the human ability to anticipate motivations. According to that hypothesis, only humans can mentally simulate past and future circumstances from a subjective perspective in a vivid and flexible manner; other animals might use simpler methods, which include some anticipation of motivations but lack the vividness and richness of human experience. While mental time travel might be linked to episodic memory, animals rudimental ability to anticipate future motivations might be linked to semantic memory (Raby and Clayton, 2009).
In relation to this debate, our proposed model of anticipated motivation describes both human and animal foresight abilities in terms of a motivational forward model. This mechanism, which projects only some internal states (motivation variables) in the future, could be a rudimental ability of “mental time travel” shared by some animals. Nevertheless, unlike the animal brain the human brain might project other internal variables and possibly episodic information into the future and, thus, obtain a more accurate estimate of the self in the future. Enhanced prospection abilities could then determine qualitative (and perhaps phenomenological) differences between humans and animals, and at the same time maintain continuity from the simpler control architectures of our remote ancestors to our more sophisticated cognitive abilities (Pezzulo and Castelfranchi, 2007, 2009; Cisek and Kalaska, 2010).
Regarding the neural mechanisms involved in foresight, we hypothesize that variables in the model of anticipated motivation might be related to two distinct brain processes. The former process may be related to more abstract mechanisms of generating future prospects (linked to sensorimotor and motivational forward model nodes) and inhibiting preponderant responses triggered by reactive systems (not implemented in our model), and might be connected to areas such as dorsolateral prefrontal cortex and cingulate cortex. The latter process (associated with utility nodes) might be linked to the activation of “as-if” motivations (Damasio, 1994) and hence may involve cortico-limbic structures directly related to motivations themselves, such as the amygdala, orbitofrontal cortex, parahippocampal gyrus, and anterior fusiform gyrus (LaBar et al., 2001). These two processes may be connected as follows: cortical anterior structures may modulate the activation of cortico-limbic structures related to simulated motivations. In other words, anticipation of future needs might partially activate brain structures associated to those needs and motivations. For instance, even if my homeostatic system does not currently require food intake, thinking about the next Christmas dinner triggers my hunger. As the ability to imagine future hunger may be similar to hunger itself, it might activate the same brain areas activated when desiring food in a hungry state.
4 Anticipating Cognitive and Emotional Processes
In addition to motivational processes, cognitive, and emotional processes in general can be anticipated during decision-making. Indeed, a central point of theories of prospection and mental time travel is that an agent can project itself into the future, possibly with the same level of detail as episodic memory. Therefore, not only it can simulate future events, but also what it will think, pay attention to and feel in these future events. In turn, the value of these simulated cognitive and emotional states can be considered in the reward-maximization process of decision-making.
Although it is still unclear how the evaluation of simulated cognitive and emotional states is implemented in the brain, recent research suggests that the simulation of future events elicits at least two kinds of affective processes. First, just imagining a reward or punishment is sufficient to elicit a feeling congruent to the one elicited by the occurrence of that reward or punishment, a so called pre-feeling (Breiter et al., 2001; Gilbert and Wilson, 2007). For instance, when one imagines the joy associated with a future event (e.g., winning a match) it can pre-feel joy. Rick and Loewenstein (2008) argued that the reason why pre-feelings are elicited automatically is that they can be used as proxies when making decisions in which it is impossible to calculate action outcomes or associated rewards exactly. When action effects are difficult to predict or “intangible,” people can, instead, use more tangible anticipated emotions to decide among alternative options (see also Damasio, 1994 for a similar view on how pre-feelings are used as proxies to evaluate an imagined situation).
Second, anticipating prospects can trigger different emotions from those elicited by outcomes, but strictly related to them. For instance, the anticipation of a future loss can elicit frustration, disappointment or regret, and the anticipation of pain can elicit fear or rage1. The adaptive value of such anticipatory emotions could be related to preparatory processes aimed at approaching or avoiding salient outcomes; for instance, fear could help in preparing to deal with future dangers (e.g., predators).
As prospection elicits pre-feelings and anticipatory emotions, the value of the latter becomes part of the decision-making process. The fact that anticipation of emotions influences decision-making is incompatible with economic theories that disregard psychological variables in modeling value assignment. This fact has recently been acknowledged by different areas of research that aim to develop novel theories of decision-making that incorporate the role of anticipated emotions within EUT (see e.g., Caplin and Leahy, 2001; Mellers and McGraw, 2001; Coricelli et al., 2007).
One condition in which anticipated emotions influence decision-making is intertemporal choices. Traditional intertemporal choice models (such as discounted utility theory, an extension of EUT) assume that human and non-human animals exponentially discount the utility assigned to outcomes as a function of their delayed presentation. As a consequence, agents should prefer immediate rewards to delayed ones and vice versa in the case of punishment. Contrary to this hypothesis, Loewenstein (1987) found that, at least in some circumstances, participants preferred to receive shock immediately rather than wait a few more seconds for a postponed shock of the same voltage. Furthermore, the more participants were asked to wait, the more they were affected by the (negative) pre-feelings, suggesting that they were assigning a (negative) value to the passage of time.
The same scenario was studied in an fMRI experiment (Berns et al., 2006). This study reveals the existence of neural bases of dread, or the anticipated neural representation of punishment, which might be located in the posterior elements of the cortical pain matrix (SI, SII, the posterior insula, and the caudal cingulate cortex). The activity of these brain areas is proportional to time delay of the shock. Furthermore, “extreme dreaders,” or participants whose subjective feeling of dread was particularly significant, preferred receiving a higher voltage rather than waiting, which shows that the cost of waiting was higher than the cost associated with the difference in voltage. As the posterior pain matrix, that is, the brain area associated with dread, is usually involved in attentional processes connected to pain modulation, the authors hypothesized that dread involves attentional phenomena as well as emotional ones. Nevertheless, how attentional and emotional processes are integrated in planning processes related to utility-maximization is still unknown.
In keeping with (Loewenstein, 1987), we argue that subjects use prospection abilities to anticipate their cognitive and emotional processes while they wait for the punishment (see Caplin and Leahy, 2001 for a related view). The effects of dread on choice can be explained by two processes: the anticipation of directing future attention toward punishment and the emotional reaction to this anticipation (dread), which in turn may influence the utility values of prospects. The influence of these two processes may be proportional to delay, namely to how long the agent believes it will pay attention to the outcome and pre-feel dread2. Following this logic, in Berns et al.’s (2006) experiment, subjects might not only pre-feel dread, but also anticipate that they will pre-feel the same way until they receive the shock, because they will be aware and pay attention to the feared outcome (the incoming pain) for the entire time preceding punishment. Considering a prospect characterized by these future cognitive and emotional states, all of which are negative, the cost of waiting sums up to the shock pre-feeling, proportionally to delay of its occurrence. This is where a cost for waiting comes from. This anticipation of future attention processes might activate areas of posterior pain matrix linked to attention modulation, such as caudal cingulate cortex and posterior insula, which in turn might increase pre-feeling (dread), possibly causing the activation of areas associated with the perception of pain, namely SI and SII.
As we have discussed, dread is just one of the many examples of how anticipated cognitive and emotional processes affect decision-making. Indeed, the anticipation of cognitive and emotional states is a multifaceted process, which plausibly involves several brain areas. However, we argue that it is possible to identify common (computational-level) principles for studying how anticipated cognitive and emotional states are elicited and how in turn they affect choice. In particular, the projection of the self in the future, the anticipation of cognitive and emotional factors and the focus on salient events might also play a role besides dread when behavior is influenced by anticipated emotion. For example, we tend to overestimate the happiness or sadness caused by a future event, say winning a lottery or becoming paraplegic (Ubel et al., 2003; Gilbert and Wilson, 2009). The fact that we overestimate the time we will spend in a positive or negative emotional state might be one cause of this phenomenon. A third example is that of anticipated regret (Coricelli et al., 2007). It has been reported that subjects can decide not to pursue a given course of actions because they anticipate they will regret it if it results in a loss. In this case, they might anticipate ruminating on the decision-making process itself, being attentive to the alternative choices they discarded, which might also elicit an uncomfortable emotional state (regret).
In the rest of this section, we will propose a computational model that extends the baseline model by incorporating the anticipation of cognitive and emotional processes along the lines we have sketched here; then, we will test it in the paradigmatic case of dread.
4.1 Computational Model
In order to account for the ability to project oneself into the future, so as to anticipate cognitive and emotional processes, we have added an additional set of nodes to the baseline model: imagined states (is). The resulting model is shown in Table 4 and Figure 7. Imagined states represent salient information the agent expects to focus on. In other words, the agent anticipates that at time t it will focus its cognitive and attentive resources on the state of the world represented by the imagined state ist.
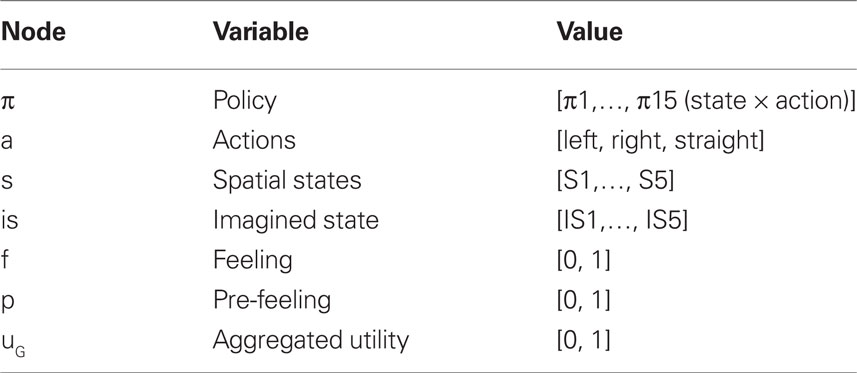
Table 4. List of variables used in Figure 7.
Imagined states depend on the value of one or more real states (s), specifically the ones associated with the higher reward or punishment value. In this way, we implicitly assume that people anticipate paying attention to states having strong emotional value. These can be future states, as in the case of dread or anticipation of future punishment, meaning that ist corresponds to a future real state st+n. Or they can be past states, as in the case of regret, meaning that ist corresponds to a past real state st−n.
Once imagined states are introduced, they can be associated with utilities (as real states are). At every time step, both real state nodes and imagined state nodes have a corresponding utility node, respectively called (utility of) feelings (uF) and (utility of) pre-feelings (uP). Both can range between 0 and 1, as in baseline model (values between 0 and 0.5 correspond to punishments). In other words, anticipating both real and fictitious experience influences the estimated values of prospects. All utility nodes are summed up by uG.
Similarly to baseline model, this model maximizes expected utility by computing the optimal policy through reward query.
4.2 Experiments: Methods and Results
4.2.1 Experiment 4: dread
To test our model, we designed an experimental scenario that is conceptually similar to that in Berns et al.’s (2006) study. These authors found that people prefer immediate electric shock rather than a postponed shock at the same (or even minor) level of intensity, and linked this preference to the anticipation of pain. The scenario is schematized in Figure 8.
Similar to the previous experiments, we represented the decision-making scenario as a T-maze in which punishment is positioned in one branch at the beginning and in the other branch at the end (see Figure 8A). Like in previous simulations, all transitions are deterministic except for utility assignment. We consider three time steps: at t0 the agent is in S1; after 5 s (t1) it can go left to S2 or right to S3; after 20 s (t2) it goes to S4 from S2 and to S5 from S3. We positioned two punishments with the same felt value F = −5, one in S2 (at t1 = 5) the other in S5 (at t2 = 20). As stated above, at every time step, the imagined state value is equal to the following real state value associated to the maximum absolute value of punishment compared to all other future states. Indeed, at t0, if the agent imagines going left, is0 is influenced by s1 node because punishment is found at t1 (the value of is0 is influenced by the position in the maze S2). If the agent imagines going right, is0 is influenced by s2 node because punishment is found at t2 (the value of is0 is influenced by the position in the maze S5). Finally, at t1, is1 node is influenced by s2: going left, the value of is1 is influenced by the position in the maze S4; going right, the value of is1 is influenced by the position in the maze S5. Pre-felt values associated to every imagined state are a function of F and time to punishment [P = f(F,t)]. We adopted Loewenstein’s (1987) model to calculate the pre-felt values. Given the instantaneous intensity of dread (a = 0.05) as constant, the pre-felt value during the interval tj–tj−1 is:

Going left, during t1 − t0, ; during t2 − t1,
. Going right, during t1 − t0, P1 is the same as going left; but during t2 − t1,
Total dread (D = ΣP) is respectively Dleft = 1,25, Dright = 4.
Results of the simulation are shown in Figure 8B. In accordance with Berns et al.’s (2006) findings, the agent chooses to go left and to receive the shock as early as possible, in order to avoid the “costs of waiting” (i.e., the pre-feelings associated to the states in which it self-projects).
4.3 Discussion
In this section, we have presented a theoretical and computational model of how the ability to anticipate emotions and cognitive processes influences choice. Specifically, we have focused on a particular case that has been widely studied, namely, dread. Different from previous mathematical characterizations aimed at behavioral description (Loewenstein, 1987), we have focused on the possible computational mechanisms behind this phenomenon, and have related them to neural processes. In particular, we have argued that dread depends on anticipation of future cognitive and emotional processes, such as continuous attention to the future shock (associated with the posterior cingulate cortex and posterior insula), which – once anticipated – produces a prospect of negative future pre-feelings (connected to SI and SII). Both processes are proportional to time delay of the shock.
Our model permits advancing some specific hypotheses about dread. First, because we described the anticipation of cognitive processes, such as attention, as an important feature of the model, we hypothesize that the effect of dread should not be present when an agent cannot anticipate those cognitive processes, or when it thinks that attention will be focused on other information. Second, we hypothesize that both lesions of the posterior cingulate cortex and the posterior insula, on one hand, and SI and SII, on the other hand, may impair dread effects. However, as we believe that the activation of the former causes the activation of the latter, we expect that during anticipation of punishment lesioning of the posterior cingulate cortex and the posterior insula may prevent the activation of SI and SII, but not vice versa. Third, we argue that the difference between extreme and mild dreaders might be linked to the ability to modulate perception through attention, connected with the functioning of the posterior cingulate cortex. Indeed, subjects that are more able to enhance or attenuate perceptive stimuli via attention might be more prone to dread, because they anticipate paying more attention to outcomes, increasing activation of the posterior cingulate cortex (Villemure and Bushnell, 2002). In this case, having weaker prospection abilities might mitigate the effects of dread. Finally, because dread requires the ability to project complex information about the self into the future (e.g., anticipation of the focus of cognitive and attention resources) in our model, we expect that non-human animals will not be prone to dread.
We have suggested that our model captures common computational mechanisms across several anticipatory emotional phenomena, such as dread, the anticipation of regret, and the (mis)judgment of how happy or sad we will be in the future. The core mechanism is the anticipation of internal, cognitive, and emotional states, in particular those associated with the more salient real states that one expects to face in the future. In turn, these anticipations assume a value themselves, and elicit associated pre-feelings. However, anticipatory emotions can be extremely variable, ranging from fear associated with a future punishment to complex emotions involving personality and social and cultural aspects, such as frustration over being unable to pursue a goal, the shame of being exposed in public, and the sense of impotence in the face of death; in this case, anticipation involves a constellation of cognitive and hedonic states, (see, e.g., Castelfranchi and Miceli, 2011). For this reason, applying our model to all these circumstances requires making specific assumptions about which environmental, cognitive, and emotional states are represented and anticipated during planning and their associated valence.
Furthermore, it is still unclear in which circumstances and to what extent the ability to anticipate emotional, motivational, and cognitive processes affects decision-making. In this regard, it is worth noting that our model accounts for anticipation of both negative and positive emotions. However, although clear results have emerged for dread, it is currently unclear whether symmetrical effects exist in the case of anticipated rewards. In this regard, some studies have detected anticipatory activity in the ventral striatum and the orbitofrontal cortex during expectancy of rewards; nevertheless, it is difficult to ascertain whether this activity depends on time delays (Breiter et al., 2001). Furthermore, it is still unclear whether dread is present during the anticipation of punishments that are more complex than electric shocks, such as monetary losses.
5 Conclusion
In this article, we have presented a theoretical and computational proposal on how prospection abilities in human and (at least partially) non-human animals affect decision-making, focusing on the role of anticipation of cognitive processes, motivations, and emotions. It is still not clear which computational mechanisms the brain exploits in these processes. We have proposed that, in general, the anticipation of future cognitive processes influences decision-making via two processes: first, the value of future outcomes is weighted in relation to the internal context at the time of the occurrence of those outcomes; second, future internal states are treated as outcomes, hence a value is directly assigned to them.
We have investigated the general issue of prospection abilities in two specific problems, namely, the anticipation of motivation and dread. In our model of anticipated motivation, we propose a mechanism that represents future motivational states and future potential rewards and permits determining the latter based on prediction of the former. In our model of dread, we propose that anticipating future attention toward an unavoidable shock and associated pre-feelings may lead people to choose to receive punishment as soon as possible. However, the framework we have proposed is more general in that it describes how the anticipation of contextual factors and of internal variables can influence decision-making. For this reason, we believe that the mechanisms we have described so far apply to a wide range of phenomena linked to prospection abilities, such as the anticipation (and evaluation) of ones own emotional states following a decision. In this respect, our model can be considered as an extension of EUT that takes psychological considerations into account and uses them in the utility-maximization process.
Assigning utility in view of future cognitive processes is a complex ability, which has been linked to concepts such as prospection and mental time travel. Further investigations are necessary to identify the circumstances in which the complex decision-making strategies we have discussed (as opposed to simpler, myopic alternatives suggested in earlier RL studies) are really used. Furthermore, it is still unclear whether or not non-human animals have prospection abilities, and if they do use similar brain mechanisms (Raby et al., 2007; Clayton et al., 2009).
Regarding which brain mechanisms underlie prospection abilities, we propose a common neural implementation of anticipated motivational, cognitive, and emotional processes. This mechanism has two components: the former one related to prospect exploration, and the other related to value assignment. First, during planning, frontal areas, such as the dorsolateral prefrontal cortex, the cingulate cortex, and the hippocampus may be responsible for the anticipation of future cognitive processes related to prospects. In turn, these areas may activate cortico-limbic and sensory structures, such as the amygdala, the orbitofrontal cortex, and the somatosensory cortex (SI and SII in the case of dread), related to imagined feelings and emotions associated with the anticipation of future cognitive processes, thus assigning utility to those processes. Although this view is speculative, it has generated some specific testable hypotheses and, indeed, some findings are in accordance with it (Breiter et al., 2001; Berns et al., 2006; van der Meer and Redish, 2010).
5.1 Implications for neuroeconomy
The possibility to use computational models to connect formal methods in economics and machine learning with neural descriptions, and to use the former to derive predictions for the latter, is one of the strengths of the new field of neuroeconomy (Glimcher and Rustichini, 2004; Glimcher et al., 2009), which is also connected to a large body of studies in computational motor control (Kording and Wolpert, 2006; TrommershŠuser, 2009; Diedrichsen et al., 2010). So far, however, most studies in neuroeconomy have focused on tasks that involve model-free controllers associated with habitual components of behavior, leveraging on the striking similarities between learning signals in the brain and formal methods used in machine learning. For instance, it has been noted that temporal difference learning signals used in model-free methods of RL (Sutton and Barto, 1998) have characteristics that are similar to the burst pattern of striatal dopamine neurons (Schultz et al., 1997).
It has been proposed, however, that although model-free methods adequately describe habitual behavior, the more flexible mechanisms underlying goal-directed choice are better formalized using model-based methods. This analysis suggests the importance of pursuing a new perspective in neuroeconomic experiments that focuses on goal-directed decision-making and aims at formally describing its neural mechanisms. Indeed, the neural underpinnings of model-based methods (associated with goal-directed controllers) are not completely known (but see Glascher et al., 2010; Simon and Daw, 2011). A key element distinguishing model-based from model-free methods is that the former learn and use explicit state predictions; however, it is still unknown which tasks require explicit state predictions and which can be accomplished also with model-free methods. We believe that studying the more flexible, goal-directed forms of decision-making is an important goal for future research and that this research initiative could benefit from a cross-fertilization of neuroscience and model-based machine learning methods, as in studies using model-free methods.
In keeping with this view, the models we have proposed extend the model-based computational framework of Botvinick and collaborators(Botvinick and An, 2008; Solway and Botvinick, submitted; the baseline model) with future-directed actions, such as the ability to anticipate future cognitive processes during planning. In particular, we have added two components, a motivational forward model and a mechanism for generating and evaluating imaginary states, both of which explicitly represent future states, that is, internal and imagined states and associated utilities. In other words, prospection abilities are represented as model-based processes. We hypothesize that a model-based implementation of prospection abilities is advantageous for agents that act in complex environments in which rewards are volatile. Although model-free models are also capable of learning future-oriented actions, they produce rigid outputs and exploit a slow trial-and-error learning procedure, which requires a stable environment. Furthermore, during the learning of future-oriented actions, model-free models collect the learning signal (reward or punishment) with a delay with respect to when the action is executed, and it is unclear how the brain solves the credit assignment problem necessary to reinforce remote actions. For this reason, we argue that future-oriented actions that are so flexible, rapidly learned, and ready for use in volatile environments are likely to depend on model-based computations (see Pezzulo, 2007, 2011 for a discussion of implicit and explicit predictions).
Overall, we have proposed a formal framework for studying prospection abilities and their influence on decision-making within a model-based approach. Specifically, in this study decision-making is framed as a (computational and neural) process aimed at maximizing the probability of expected utility using model-based methods. The first implication of this view is that phenomena such as the choice to receive punishments as early as possible (Berns et al., 2006) should not be considered as violations of the utility-maximization process, but should be considered within a formal framework that extends EUT with the effects of prospection.
Besides a computational-level description of how optimization of reward can incorporate prospection abilities, the use of probabilistic models permits making explicit claims about their mechanistic implementation in the brain. In this sense, our models have implications at the psychological and neural levels, which mainly concern the factorization of the state space, the causal relations among variables, the use of explicit representations of (internal, imagined) states and associated values to implement prospection abilities, as well as the nature of dynamics of the computations performed (e.g., the reward query). Although our knowledge is still incomplete regarding the neural underpinnings of the processes we have described, our model could help in formulating hypotheses, as in the work of Botvinick and An, (2008), Solway and Botvinick (submitted).
Another assumption, which is common of Bayesian systems, is that the brain encodes relevant variables, such as state and action variables, probabilistically (Doya et al., 2007). All these assumptions deserve rigorous empirical validation through novel experimental paradigms that explore anticipatory dynamics during choice.
In keeping with the baseline model, we have adopted a “reward query” and exact Bayesian inference to describe how computations are performed. As discussed in Botvinick and An’s (2008) study, this method guarantees maximization of expected utility (see Section 6 for a discussion of how our extensions of the model maintain the same characteristics). Although this property is appealing and has the advantage of linking our model to mathematical descriptions of EUT, which are more common in neuroeconomy, prudence is necessary to apply this normative model to real-world economic scenarios. Indeed, many factors could limit optimality in these situations. First, the quality of choice depends on the knowledge available to the decision-maker. Uncertain or limited knowledge potentially leads to sub-maximal decisions or choosing exploration rather than exploitation (Cohen et al., 2007). Furthermore, it is likely that prospection involves the simulation of few salient events or the elicitation of incomplete and erroneous simulations, and this limits the amount of (future) knowledge incorporated in decision-making. Second, the need to use bounded computational and cognitive resources can lead to sub-optimal use of available knowledge. Note that this does not necessarily mean that the Bayesian scheme is inapplicable; a possible alternative, which is currently pursued in many studies, is to explain these phenomena using approximate rather than exact Bayesian inferences (Chater et al., 2006; Daw and Courville, 2008; Sanborn et al., 2010; Dindo et al., 2011). Finally, a recent view is that decision-making and behavior result from the interaction between different controllers. In some circumstances goal-directed and habitual controllers, which tend to optimize performance (using different methods), are influenced or replaced by Pavlovian processes, which drive innate responses that can produce undesired effects (Daw et al., 2005; Niv et al., 2006; Rangel et al., 2008; Dayan, 2009). Furthermore, the conditions in which the competition of multiple controllers is adaptive (Livnat and Pippenger, 2006) or maladaptive (Dayan et al., 2006) are still unknown. Elucidating the interactions between multiple controllers, and the resulting effects for (optimal) behavior, remains as an open objective for future research.
6 The Model of Anticipated Motivation and EUT
Botvinick and An (2008) demonstrated that the inferential method adopted in the baseline model guarantees maximization of expected utility. In this section, we show how EUT concepts and procedures adopted to maximize expected utility are translated in our model of anticipated motivation, which introduces additional elements with respect to the baseline model (This is unnecessary for our second model, in which the way utilities are assigned and maximized is not substantially different from the baseline model). Specifically, we are going to explain how scalar rewards are assigned and mapped into conditional probability distributions in our model and how finding the policy that maximizes expected utility corresponds to reward query, the method adopted in the baseline model and our model to infer the optimal policy.
Consider it node as a stochastic variable representing the motivational state at time 0 < t ≤ T. Each value of it is xij ∈ , such that 0 ≤ xij ≥ Xmax, where Xmax > 0 is the maximum motivational need. The corresponding detection node represents the variable dt. Each value of dt, ytk ∈
such that 0 ≤ ytk ≥ Xmax, represents the value of the potential reward detected. Given all combinations of values (it, dt), it is possible to compute the scalar reward vector Rt, as defined within the EUT framework. It is also possible to compute p(ut = 1/it,dt), the conditional probability distribution of binary node ut, as represented in our model. Thus, ∀ j ∈ J and ∀ k ∈ K:


From the previous equation it is easy to see that:

Suppose that a certain number of motivational systems are implemented, hence there are M nodes im and dm at each time step. Also in this case, scalar rewards and conditional probabilities of um are calculated as in Eqs 4 and 5 for each motivational system. We know that, in sequential decisions, being the present state (i0, d0) at t = 0, EUT defines expected utility at time T, given policy π, as depending on future Rewards:

where E is the expectancy, or the probability of obtaining rewards. Note that in the previous equation the discounting factor is γ = 1. From EUT, we know that maximizing expected utility corresponds with choosing the optimal policy, which we call πoptEU:

At the same time, with regard to the probabilistic framework adopted by the baseline model and our model, the probability distribution of node uG can be computed as follows:

where N is the total number of u nodes. Within this probabilistic framework, reward query is a method for computing the policy ΠoptRQ according to this equation:

Given Eq. 6, we can also demonstrate that

It follows that once scalar rewards have been assigned, as described in Eqs 4 and 5, our model of anticipated motivation is able to represent the EUT scenario and compute the policy associated with maximum expected utility.
Conflict of Interest Statement
The authors declare that the research was conducted in the absence of any commercial or financial relationships that could be construed as a potential conflict of interest.
Acknowledgments
Research was funded by the EU’s FP7 under grant agreement no FP7-ICT-270108 (Goal-Leaders). We thank Matthew Botvinick for his insightful comments on an earlier version of this article.
Footnotes
- ^A third potential mechanism could be a “cold” anticipation that an emotion will result from a choice; for instance, one can anticipate that it will regret a decision without actually feeling regret. We do not discuss this issue here; (see Castelfranchi and Miceli, 2011) for a more detailed analysis of the relations between anticipation and emotional processes.
- ^Although here we assume that self-projection is relative to all future states preceding the electric shock, in general simulations of future events need not be complete, but more likely focus on selected, salient events. This aspect is captured in our model in two ways: first, the granularity of states can be arbitrary; second, not all states are considered in the computation of utility, only those having higher valence. Although simulating only salient events is more parsimonious, at the same time it could determine biases about how the imagined situation is evaluated, causing misbehavior (Gilbert and Wilson, 2009). In addition, it can produce different evaluations depending on when the future event is simulated. For instance, the effects of dread can be mitigated in the case of outcomes that are far away in time, because the imagined event is not judged as salient, and increase when it approaches; for example, this could be true for exam fear, which increases as the exam date approaches.
References
Balleine, B. W., and Dickinson, A. (1998). Goal-directed instrumental action: contingency and incentive learning and their cortical substrates. Neuropharmacology 37, 407–419.
Barkley, R. A. (2001). The executive functions and self-regulation: an evolutionary neuropsychological perspective. Neuropsychol. Rev. 11, 1–29.
Berns, G. S., Chappelow, J., Cekic, M., Zink, C. F., Pagnoni, G., and Martin-Skurski, M. E. (2006). Neurobiological substrates of dread. Science 312, 754–758.
Botvinick, M. M., and An, J. (2008). “Goal-directed decision making in prefrontal cortex: a computational framework,” in Advances in Neural Information Processing Systems (NIPS), Vol. 21, eds D. Koller, Y. Y. Bengio, D. Schuurmans, L. Bouttou, and A. Culotta (Cambridge, MA: MIT Press), 169–176.
Botvinick, M. M., Braver, T. S., Barch, D. M., Carter, C. S., and Cohen, J. D. (2001). Conflict monitoring and cognitive control. Psychol. Rev. 108, 624–652.
Breiter, H. C., Aharon, I., Kahneman, D., Dale, A., and Shizgal, P. (2001). Functional imaging of neural responses to expectancy and experience of monetary gains and losses. Neuron 30, 619–639.
Buckner, R. L., and Carroll, D. C. (2007). Self-projection and the brain. Trends Cogn. Sci. (Regul. Ed.) 11, 49–57.
Caplin, A., and Leahy, J. (2001). Psychological expected utility theory and anticipatory feelings*. Q. J. Econ. 116, 55–79.
Castelfranchi, C., and Miceli, M. (2011). Anticipation and emotion, in Emotion-Oriented Systems, Cognitive Technologies, eds R. Cowie, C. Pelachaud, and P. Petta (Berlin: Springer), 483–500.
Chater, N., Tenenbaum, J. B., and Yuille, A. (2006). Probabilistic models of cognition: conceptual foundations. Trends Cogn. Sci. (Regul. Ed.) 10, 287–291.
Cisek, P., and Kalaska, J. F. (2010). Neural mechanisms for interacting with a world full of action choices. Annu. Rev. Neurosci. 33, 269–298.
Clayton, N., Russell, J., and Dickinson, A. (2009). Are animals stuck in time or are they chronesthetic creatures? Top. Cogn. Sci. 1, 59–71.
Cohen, J. D., McClure, S. M., and Yu, A. J. (2007). Should i stay or should i go? how the human brain manages the trade-off between exploitation and exploration. Philos. Trans. R. Soc. Lond. B Biol. Sci. 362, 933–942.
Cooper, G. F. (1988). “A method for using belief networks as influence diagrams,” in Proceedings of the Twelfth Conference on Uncertainty in Artificial Intelligence, Minneapolis, MN, 55–63.
Coricelli, G., Critchley, H., Joffily, M., O’Doherty, J., Sirigu, A., and Dolan, R. (2005). Regret and its avoidance: a neuroimaging study of choice behavior. Nat. Neurosci. 8, 1255–1262.
Coricelli, G., Dolan, R. J., and Sirigu, A. (2007). Brain, emotion and decision making: the paradigmatic example of regret. Trends Cogn. Sci. (Regul. Ed.) 11, 258–265.
Damasio, A. R. (1994). Descartes’ Error: Emotion, Reason and the Human Brain. New York: Grosset/Putnam.
Daw, N., and Courville, A. C. (2008). “The pigeon as particle filter,” in Advances in Neural Information Processing Systems, Vol. 20, eds J. C. Platt, D. Koller, Y. Singer, and S. Roweis (Cambridge, MA: MIT Press), 369–376.
Daw, N. D., Niv, Y., and Dayan, P. (2005). Uncertainty-based competition between prefrontal and dorsolateral striatal systems for behavioral control. Nat. Neurosci. 8, 1704–1711.
Dayan, P., Niv, Y., Seymour, B., and Daw, N. D. (2006). The misbehavior of value and the discipline of the will. Neural Netw. 19, 1153–1160.
Diedrichsen, J., Shadmehr, R., and Ivry, R. B. (2010). The coordination of movement: optimal feedback control and beyond. Trends Cogn. Sci. (Regul. Ed.) 14, 31–39.
Dindo, H., Zambuto, D., and Pezzulo, G. (2011). “Motor simulation via coupled internal models using sequential monte carlo,” in Proceedings of IJCAI 2011, Barcelona.
Doya, K., Ishii, S., Pouget, A., and Rao, R. P. N. (eds). (2007). Bayesian Brain: Probabilistic Approaches to Neural Coding, 1st Edn. Boston: The MIT Press.
Gilbert, D. T., and Wilson, T. D. (2007). Prospection: experiencing the future. Science 317, 1351–1354.
Gilbert, D. T., and Wilson, T. D. (2009). Why the brain talks to itself: sources of error in emotional prediction. Philos. Trans. R. Soc. Lond. B Biol. Sci. 364, 1335–1341.
Glascher, J., Daw, N., Dayan, P., and O’Doherty, J. (2010). States vs rewards: dissociable neural prediction error signals underlying model-based and model-free reinforcement learning. Neuron 66, 585–595.
Glimcher, P., Fehr, E., Camerer, C., and Poldrack, R. (eds). (2009). Neuroeconomics: Decision Making and the Brain. London: Academic Press.
Glimcher, P. W., and Rustichini, A. (2004). Neuroeconomics: the consilience of brain and decision. Science 306, 447–452.
Kable, J. W., and Glimcher, P. W. (2007). The neural correlates of subjective value during intertemporal choice. Nat. Neurosci. 10, 1625–1633.
Kording, K., and Wolpert, D. (2006). Bayesian decision theory in sensorimotor control. Trends Cogn. Sci. (Regul. Ed.) 10, 319–326.
LaBar, K., Gitelman, D., Parrish, T., Kim, Y., Nobre, A., and Mesulam, M. (2001). Hunger selectively modulates corticolimbic activation to food stimuli in humans. Behav. Neurosci. 115, 493–500.
Livnat, A., and Pippenger, N. (2006). An optimal brain can be composed of conflicting agents. Proc. Natl. Acad. Sci. U.S.A. 103, 3198–3202.
Loewenstein, G. (1987). Anticipation and the valuation of delayed consumption. Econ. J. 97, 666–684.
Loewenstein, G., O’Donoghue, T., and Rabin, M. (2003). Projection bias in predicting future utility. Q. J. Econ. 118, 1209–1248.
Mellers, B. A., and McGraw, A. P. (2001). Anticipated emotions as guides to choice. Curr. Dir. Psychol. Sci. 10, 210–214.
Murphy, K. P. (2002). Dynamic Bayesian Networks: Representation, Inference and Learning. Ph.D. thesis, Computer Science Division, University of California, Berkeley.
Naqshbandi, M., and Roberts, W. (2006). Anticipation of future events in squirrel monkeys (Saimiri sciureus) and Rats (Rattus norvegicus): tests of the Bischof–Kohler hypothesis. J. Comp. Psychol. 120, 345–357.
Niv, Y., Joel, D., and Dayan, P. (2006). A normative perspective on motivation. Trends Cogn. Sci. (Regul. Ed.) 8, 375–381.
Osvath, M., and Osvath, H. (2008). Chimpanzee (Pan troglodytes) and orangutan (Pongo abelii) forethought: self-control and pre-experience in the face of future tool use. Anim. Cogn. 11, 661–674.
Padoa-Schioppa, C., and Assad, J. A. (2006). Neurons in the orbitofrontal cortex encode economic value. Nature 441, 223–226.
Padoa-Schioppa, C., and Assad, J. A. (2008). The representation of economic value in the orbitofrontal cortex is invariant for changes of menu. Nat. Neurosci. 11, 95–102.
Pezzulo, G. (2007). “Anticipation and future-oriented capabilities in natural and artificial cognition,” in 50 Years of AI, Festschrift, eds M. Lungarella, F. Iida, J. C. Bongard, and R. Pfeifer (Berlin: Springer), 258–271.
Pezzulo, G. (2011). Grounding procedural and declarative knowledge in sensorimotor anticipation. Mind Lang. 26, 78–114.
Pezzulo, G., and Castelfranchi, C. (2007). The symbol detachment problem. Cogn. Process. 8, 115–131.
Pezzulo, G., and Castelfranchi, C. (2009). Thinking as the control of imagination: a conceptual framework for goal-directed systems. Psychol. Res. 73, 559–577.
Raby, C. R., Alexis, D. M., Dickinson, A., and Clayton, N. S. (2007). Planning for the future by western scrub-jays. Nature 445, 919–921.
Raby, C. R., and Clayton, N. S. (2009). Prospective cognition in animals. Behav. Processes 80, 314–324.
Rangel, A., Camerer, C., and Montague, P. R. (2008). A framework for studying the neurobiology of value-based decision making. Nat. Rev. Neurosci. 9, 545–556.
Rick, S., and Loewenstein, G. (2008). Intangibility in intertemporal choice. Philos. Trans. R. Soc. Lond. B Biol. Sci. 363, 3813–3824.
Rigoli, F., Pavone, E. F., and Pezzulo, G. (2011). “Interaction of goal-directed and pavlovian systems in aversive domains,” in Proceedings of CogSci 2011, Boston.
Sanborn, A. N., Griffiths, T. L., and Shiffrin, R. M. (2010). Uncovering mental representations with markov chain monte carlo. Cogn. Psychol. 60, 63–106.
Schacter, D. L., Addis, D. R., and Buckner, R. L. (2007). Remembering the past to imagine the future: the prospective brain. Nat. Rev. Neurosci. 8, 657–661.
Schultz, W., Dayan, P., and Montague, P. (1997). A neural substrate of prediction and reward. Science 275, 1593–1599.
Shachter, R. D., and Peot, M. A. (1992). “Decision making using probabilistic inference methods,” in UAI ’92: Proceedings of the Eighth Conference on Uncertainty in Artificial Intelligence (San Francisco, CA: Morgan Kaufmann Publishers Inc.), 276–283.
Simon, D. A., and Daw, N. D. (2011). Neural correlates of forward planning in a spatial decision task in humans. J. Neurosci. 31, 5526–5539.
Suddendorf, T., and Corballis, M. C. (1997). Mental time travel and the evolution of the human mind. Genet. Soc. Gen. Psychol. Monogr. 123, 133–167.
Suddendorf, T., and Corballis, M. C. (2007). The evolution of foresight: what is mental time travel and is it unique to humans? Behav. Brain Sci. 30, 299–313.
TrommershŠuser, J. (2009). Biases and optimality of sensory-motor and cognitive decisions. Prog. Brain Res. 174, 267–278.
Ubel, P. A., Loewenstein, G., and Jepson, C. (2003). Whose quality of life? a commentary exploring discrepancies between health state evaluations of patients and the general public. Qual. Life Res. 12, 599–607.
van der Meer, M., and Redish, A. (2010). Expectancies in decision making, reinforcement learning, and ventral striatum. Front. Neurosci. 4:6. doi: 10.3389/neuro.01.006.2010
Keywords: prospection, model-based, Bayesian, goal-directed, anticipatory planning, dread, anticipation, forward model
Citation: Pezzulo G and Rigoli F (2011) The value of foresight: how prospection affects decision-making. Front. Neurosci. 5:79. doi: 10.3389/fnins.2011.00079
Received: 29 December 2010; Accepted: 06 June 2011;
Published online: 30 June 2011.
Edited by:
Paul Glimcher, New York University, USAReviewed by:
Nathaniel D. Daw, New York University, USAAnne Churchland, Cold Spring Harbor Laboratories, USA
Copyright: © 2011 Pezzulo and Rigoli. This is an open-access article subject to a non-exclusive license between the authors and Frontiers Media SA, which permits use, distribution and reproduction in other forums, provided the original authors and source are credited and other Frontiers conditions are complied with.
*Correspondence: Giovanni Pezzulo, Istituto di Scienze e Tecnologie della Cognizione, Consiglio Nazionale delle Ricerche, Via S. Martino della Battaglia 44, 00185 Roma, Italy. e-mail:Z2lvdmFubmkucGV6enVsb0BjbnIuaXQ=