- School of Biological and Health Systems Engineering, Arizona State University, Tempe, AZ, USA
Implantable microelectrodes that are currently used to monitor neuronal activity in the brain in vivo have serious limitations both in acute and chronic experiments. Movable microelectrodes that adapt their position in the brain to maximize the quality of neuronal recording have been suggested and tried as a potential solution to overcome the challenges with the current fixed implantable microelectrodes. While the results so far suggest that movable microelectrodes improve the quality and stability of neuronal recordings from the brain in vivo, the bulky nature of the technologies involved in making these movable microelectrodes limits the throughput (number of neurons that can be recorded from at any given time) of these implantable devices. Emerging technologies involving the use of microscale motors and electrodes promise to overcome this limitation. This review summarizes some of the most recent efforts in developing movable neural interfaces using microscale technologies that adapt their position in response to changes in the quality of the neuronal recordings. Key gaps in our understanding of the brain–electrode interface are highlighted. Emerging discoveries in these areas will lead to success in the development of a reliable and stable interface with single neurons that will impact basic neurophysiological studies and emerging cortical prosthetic technologies.
Implantable microelectrodes are still the preferred and commonly used method for monitoring electrical activity of single neurons in the brain, particularly from deep brain structures. Multiple microelectrodes are typically used to capture emerging functional activity from ensemble of neurons. One of the advantages of the implantable microelectrode technology is that it allows real-time functional monitoring of single neurons in the brain while the animal is behaving. The limitations of the microelectrode technology are quite well known now. In general, microelectrodes are biased toward the sample of neurons that are active or have higher firing rates during the time of implantation or recording. When using multiple microelectrodes in a bundle or multi-channel microelectrodes, the yield (in terms of number of microelectrodes that actually capture neuronal activity) is not consistent across experiments and depends on the level of anesthesia, location of the microelectrodes, user skills, experimental protocol used, and the type of microelectrode used. Several neurophysiological studies also require monitoring single neurons and ensembles of neurons over a period of several weeks and months. Neuronal recordings from microelectrodes have been found to be inconsistent and/or unreliable in long-term experiments with current implantable microelectrode technologies (Vetter et al., 2004; Engel et al., 2005; Polikov et al., 2005; Liu et al., 2006; Eliades and Wang, 2008; Grill et al., 2009). The above limitation is probably the single most significant impediment toward the success of other emerging, exciting applications such as the cortical prostheses that rely on being able to monitor single neuronal or multi-neuronal activity over the life-time of patients who will use such prosthetic devices. Movable microelectrodes, as opposed to fixed microelectrodes in the brain, have been suggested as a potential approach to mitigate some of the above limitations.
Advantages of Movable Microelectrodes
Technologies that enable us to move the microelectrodes after implantation promise to dramatically enhance our ability to (a) isolate activity from single neurons and maintain stable neuronal recordings over longer durations and also to carefully and unambiguously monitor changes in small population of single neurons undergoing neuronal plasticity, for instance (b) enhance and maintain the signal-to-noise ratio in the neuronal recordings (c) seek neurons of interest after implantation and probe neuronal tracts and connectivity (d) overcome the inherent bias in the neuronal recordings toward the more active neurons. Movable microelectrodes now give us the opportunity to seek other neurons that might have been silent during the time of implantation. (e) Potentially enhance the reliability of prosthetic devices in applications that require neuronal recordings over the life-time of the patient. Movable microelectrode technology appears to be particularly suited to recording from neurons in banks of sulci where the neurons are located at multiple different depths along the banks of sulci. The reason for expecting movable microelectrodes to offer advantages (a)–(d) is quite intuitive and some of the above capabilities have already been demonstrated. Microelectrodes have to be typically positioned within tens or hundreds of microns (depending on the type and orientation of the neuron) from a neuronal cell body to record its action potentials. Therefore, any ability to fine-tune the geographical position of the microelectrode after implantation will allow us to potentially maintain the microelectrodes within the recording radius of a neuron over a reasonable length of time.
Trying to record the same unit or neuron over a long period of time with conventional microelectrodes that are fixed in position is a very challenging task particularly in large animals such as non-human primates in unrestrained behavioral contexts. In these applications, some of the commonly used methods to confirm the preservation of the identities of the recorded neurons are (a) clean ISI (b) consistent shapes and peak-to-peak amplitudes of action potentials and (c) consistent behavioral correlates. However, the above methods by themselves still do not guarantee a confirmed neuronal identity because there is evidence that distinct neurons can often register identical action potentials at the recording electrode. In addition, the same neuron might also undergo changes in its action potential as a result of underlying neuronal plasticity which might actually be the reason for monitoring the neuron in the first place. Further confirmatory evidence can be obtained by antidromic stimulation of output pathways. However, the stability of ISIs, behavioral tuning curves and/or stimulation thresholds and latencies in response to antidromic stimulation will have to either be assumed or proved a priori if the above methods are to be truly effective. Self-contained continuous recording systems that can monitor and track gradual changes in action potential amplitudes, shapes, and firing rates will allow for the development of rigorous predictive models that will confirm if the recorded changes occur due to neuronal plasticity or due to a change in the neuronal identity (Tolias et al., 2007; Dickey et al., 2009). Movable microelectrodes have been demonstrated to achieve stability of single neuronal recordings over several weeks in (Yamamoto and Wilson, 2008) and non-human primates (Jackson and Fetz, 2007). Isolation and stability of recordings from specifically identified neurons do not appear to be as critical for motor cortical prostheses as demonstrated by the relative success using local field potentials (Scherberger et al., 2005; Hwang and Andersen, 2009) and also by the increase in “efficiency” of the decoding algorithms by increasing the quality of single unit recording using movable microelectrodes without necessarily verifying if the original neuron or cell type was maintained before and after microelectrode movement to enhance the quality of single unit recording (Mulliken et al., 2008).
Recent studies using moveable microelectrodes have shown that the ability to reposition the microelectrodes before or during each recording session dramatically enhances the yield and signal-to-noise ratios of the neuronal recordings (Fee and Leonardo, 2001; Cham et al., 2005; Yamamoto and Wilson, 2008; Wolf et al., 2009) and consequently the “decoding-efficiency” in a neural prosthetic application (Mulliken et al., 2008; Wolf et al., 2009). The reliability of neuronal recordings in long-term experiments and clinical applications such as the cortical prostheses can also be potentially enhanced using movable microelectrode by now giving us the ability to seek new neurons in the event of loss of signal due to biological reasons such as tissue reaction around the microelectrode resulting in neuronal migration or due to relative micromotion between the microelectrode and surrounding tissue.
Current Technologies for Adaptive Movable Microelectrodes
Movement of microelectrodes after implantation has so far been achieved using piezoelectric motors (Cham et al., 2005; Park et al., 2008; Wolf et al., 2009), piezomotor (Yang et al., 2011), stepper motors (Gray et al., 2007), dc servomotors (Yamamoto and Wilson, 2008), synchronous motors (Fee and Leonardo, 2001; Kern et al., 2008), hydraulic positioning (Decharms et al., 1999; Sato et al., 2007), and screw based microdrives (Swadlow et al., 2005; Korshunov, 2006; Dobbins et al., 2007; Lansink et al., 2007; Battaglia et al., 2009; Haiss et al., 2010). These technologies with varying degrees of success have been tested in song birds, mice, rats, non-human primates, etc. Motorized microelectrodes are generally preferred over the microelectrodes that have to be moved manually. Manual movement of microelectrodes involves constraining the animal behaviorally while the microelectrode is being moved and may impact its spontaneous behaviors such as motor activity or singing (in song birds), etc. Besides, there is the possibility of the animal resisting such manual handling and perturbing the positioning of the microelectrode. Motorized microelectrodes with as many as 21 tetrodes (Yamamoto and Wilson, 2008) have been successfully demonstrated in rat models. While screw based manually movable systems can handle more number of movable tetrodes or microelectrodes, the significant disadvantages of manually movable microelectrodes mentioned earlier and the potentially higher reliability and consistency offered by motorized microelectrodes make the latter generally preferable over the former. So there is a need for a fundamentally new technology that is scalable, small in form factor and weight, which will enable the realization of large numbers of independently motorized, movable microelectrodes.
In summary, there is strong experimental evidence to support the fact that the strategy of moving the microelectrode leads to:
(a) Significantly enhanced signal qualities (Fee and Leonardo, 2001; Jackson and Fetz, 2007; Yamamoto and Wilson, 2008; Wolf et al., 2009; Jackson et al., 2010).
(b) Isolation of single units and stability of recordings for durations running into weeks (Fee and Leonardo, 2001; Jackson and Fetz, 2007; Yamamoto and Wilson, 2008).
(c) Dramatically improved yield (Fee and Leonardo, 2001; Jackson and Fetz, 2007; Yamamoto and Wilson, 2008; Jackson et al., 2010).
(d) Simultaneous monitoring of pairs and triplets.
(e) Increased decoding-efficiency in BCI applications.
Technical Challenges
The significant challenges that remain now are to (1) find motor technologies that will allow us to increase the number of movable microelectrodes in the recording system while simultaneously maintaining a form factor that is suitable for chronic implantation in animal models (2) demonstrate stable interface with single neurons for durations that last longer than few weeks (3) demonstrate reliable interfaces with single neurons that last the life-time of a patient for applications such as cortical prostheses. Reliable interfaces is a less challenging requirement than stable interfaces with single neurons in that it could include establishing new interfaces with other neurons in the vicinity in the event of a loss of functionality in one neuron–electrode interface. The above challenges can only be overcome by a better understanding of the neuron–electrode interface in combination with fundamentally new microscale motor technology development.
MEMS Based Technologies for Movable Microelectrodes
Microscale electro-mechanical systems (MEMS) offers an attractive array of technologies (including micromotors or microactuators) to realize a movable microelectrode array system that is high-density, light-weight, and small in size. Besides, MEMS based microfabrication technologies provide other significant advantages such as (a) a batch fabrication approach (b) reliable interconnects and (c) possibilities for seamless integration of other functional modules such as on-board signal conditioning (amplification, filtering, etc.), control and telemetry modules.
We have developed and tested (a) novel electrostatic comb-drive microactuators (Muthuswamy et al., 2005b) and (b) novel electrothermal microactuators for their ability to enable neuronal recordings in vivo experiments (Muthuswamy et al., 2005a). Pictures of both movable microelectrode systems are shown in Figure 1. The electrostatic microactuators required voltages in the order of 90–110 V (with currents in micro-amperes) and were found to have several limitations for both acute and long-term in vivo experiments. They occupied almost twice as large footprint on the microchip as the electrothermal microactuators. Therefore, on a typical microchip of 7 mm × 3 mm, we were able to fabricate a maximum of two electrostatic microactuator driven microelectrodes compared to three electrothermal microactuator driven microelectrodes. While this difference may not seem like much on a single chip, it starts to become significant when the system is scaled to accommodate 60–100 movable microelectrodes. More importantly, the electrostatic microactuators and the gears often suffered from stiction (where the microstructures such as microscale beams, cantilevers, and gears often stuck to each other or stuck to the substrate) resulting in failure of the movable microelectrodes. In addition, the electrostatic microelectrodes were not robust enough to sustain the mechanical stresses typically encountered during animal behavior. In contrast, the electrothermal microactuators only required 9–11 V of activation voltages with currents in the order of few tens of milliamps. We have found that these electrothermal microactuator driven microelectrodes are robust against mechanical stresses encountered during animal behavior and also against moisture. We have also very few instances of stiction related failures. Both of the above technologies are capable of moving the microelectrodes over a distance of 5–8 mm with a displacement resolution of 6–10 μm. The microelectrodes can be spaced 500–800 μm apart on a single substrate.
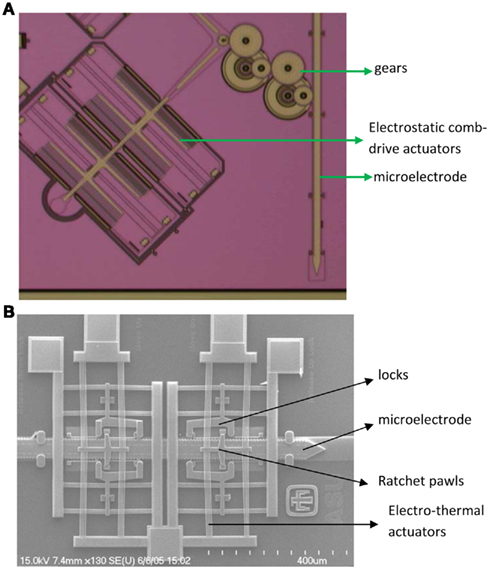
Figure 1. (A) Micrograph of one of the electrostatic comb-drive microactuators coupled to a microelectrode through gears. Two such comb-drive actuators drive a single microelectrode. (B) SEM of electrothermal microactuator, microelectrode and associated ratchet pawls and locks. The microelectrodes in both cases are 50 μm wide.
Microelectrodes that have to be moved manually range in size from 5.85 mm × 14.5 mm (6-channel system; Venkatachalam et al., 1999) to 26 mm × 22 mm × 16 mm (49 channel system; Decharms et al., 1999); weigh from 0.39 g for a one-channel system (Bilkey et al., 2003) to 20 g for a 49 channel system (Decharms et al., 1999). The motorized versions vary from 6 mm × 17 mm (3-channel system; Fee and Leonardo, 2001) to over 143 mm (largest dimension in an 8-channel system; Gray et al., 2007) and weigh from 1.5 g (3-channel system; Fee and Leonardo, 2001) to a 129.5-g (8-channel system; Gray et al., 2007). The first generation of MEMS based movable microelectrode system resulted in a 3-channel system with a size of 14 mm × 17 mm × 3 mm and a weight of 1.9 g (Jackson et al., 2010) with bulk of the size and weight contributions from interconnects and packaging. However, with optimal packaging and interconnects, the 3-channel system can be reduced to 3 mm × 6 mm × 0.6 mm weighing as little as 0.25 g or less. We are currently developing novel stacking technologies to create higher density microelectrode arrays. For instance, we are currently developing a movable microelectrode system that will have 60–84 independently positionable microelectrodes with overall dimensions of 7–13 mm in the anterior–posterior direction, 6–9 mm in the medio-lateral direction, and a height 6–10 mm as illustrated in Figure 2. Using the above MEMS based technologies will potentially help us simultaneously achieve high yield and high neuronal count (electrical recordings from a large sample of neurons in the brain).
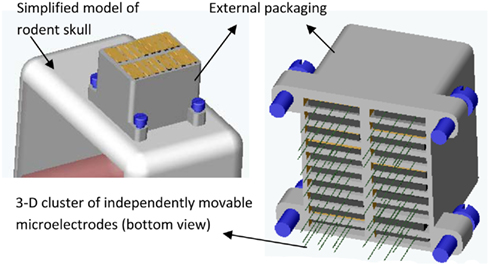
Figure 2. A CAD illustration of the proposed 3-D cluster of independently movable microelectrodes anchored on a simplified model of a rodent skull (in left). A 10 × 2 array of movable microelectrode chips (total of 20 chips each with 3 movable microelectrodes) is shown here but other layouts such as 3 × 5 and 3 × 7 microchips will also be developed and tested. (right) Bottom view of the 10 × 2 array of chips showing the 60 independently positionable microelectrodes. The anterior–posterior dimension of the packaged cluster is expected to be 7–13 mm, medio-lateral dimension 6–9 mm, and height 6–10 mm.
In our most recent long-term study on the performance of movable microelectrode arrays in rodent experiments, we demonstrated successful multi-unit recordings in rodents for over 80 days (Jackson et al., 2010). In the first 3 weeks of implantation, moving the microelectrodes lead to significant improvements in the SNR in 44 out of 46 instances. Beyond 3 weeks, however, moving the microelectrodes resulted in significant improvement in the SNR in only 6 out of 11 instances. The above results suggested that beyond 3 weeks, the forces generated by the microactuators might be inadequate to overcome and move the microelectrode past the glial sheath that is likely to be encapsulating the microelectrode. The other possibility is that there are no viable neurons in the vicinity of the microelectrodes along the z-axis and therefore any repositioning of the microelectrode in search of viable neurons is likely to be in vain. However, past histological and immunochemical studies that have reported neuronal migration have indicated a loss or decrease in neuronal densities only in the near vicinity (<100 μm from the center of the microelectrode tip) of the microelectrode (McCreery et al., 2010). In our study, the microelectrodes were moved in increments of 100 μm in the event of loss of neuronal recording. Therefore, it is more likely that after 3 weeks of implantation, inadequate forces generated by the microactuators is the dominant factor contributing to the lack of significant improvement in the SNR of neuronal recordings in 5 out of 11 cases where the microelectrode was moved.
The signal-to-noise ratio in the above study was estimated by calculating the ratio of signal power to background noise power. The signal power was estimated by mean of square of unit signal amplitudes and the noise power was estimated by mean of square of amplitudes of background noise (segments of data that did not have any signal or units). The increase in SNR obtained after microelectrode movement in the above study was most likely due to isolating and recording new cells because (a) in response to decrease in SNR below 10 dB the microelectrodes were moved in increments of 100 μm that would have likely moved the microelectrodes well past the original set of neurons and (b) the cluster of points in the principal component space (and the corresponding shapes of action potentials) after microelectrode movement was distinct from the cluster of points before microelectrode movement. However, a more careful functional analysis will need to be done to confirm if the improvement in SNR after microelectrode movement was due to recordings from new cells or due to better isolation of the older neurons.
Some of the key gaps in our understanding of the neuron–electrode interface that continue to impact the quality of neuronal recordings from movable microelectrodes in chronic experiments include (a) response of the brain tissue surrounding the microelectrode and (b) relative micromotion between the implanted microelectrode and the surrounding brain tissue. A better understanding of the above two issues promises to dramatically improve the performance of the movable microelectrodes by identifying design parameters and establishing constraints for movement protocols (timing, direction, displacement, and speed of movement), microelectrode geometry, surface and bulk properties and rational design of closed-loop control algorithms.
Tissue Response to Movable Microelectrodes
The inflammatory and foreign body responses elicited by microelectrodes implanted in the brain have been carefully documented by several groups (Szarowski et al., 2003; Polikov et al., 2005; McConnell et al., 2009; Stice and Muthuswamy, 2009; Winslow and Tresco, 2010). The presence of activated microglia, glial hypertrophy, and a glial sheath formation around the microelectrode that sets in beyond 4–6 weeks of implantation in the brain have been consistently documented by these groups in a variety of animal models. Data also indicates some neuronal migration away from the microelectrode over the same duration. However, the tissue response elicited by a movable microelectrode in the brain is not well understood. More importantly, how does the tissue response impact the use and performance (in terms of quality of neuronal recording over time) of a movable microelectrode? The level of tissue response elicited due to the movement of microelectrodes in the brain can be carefully assessed by carefully controlling mechanical parameters such as timing of microelectrode movement (in terms of days after implantation), distance over which the microelectrode is moved, direction of movement (up or down), and speed of movement (Stice and Muthuswamy, 2009). A prior study on the tissue response to microelectrode movement revealed some interesting and somewhat unexpected results (Stice and Muthuswamy, 2009) as descriptively summarized in Figure 3. Three cohorts were investigated in the above study – one where the microelectrode was moved by 500 μm on day 2 after implantation, one where the microelectrode was moved by 500 μm on day 14 after implantation and in the last cohort, the microelectrode was moved by 500 μm on day 28 after implantation. In all three cases, the brain tissue around the microelectrode was assessed for glial fibrillary acidic protein (a marker of reactive astrocytes) in the astrocytes 28 days after the day of movement (to allow for chronic glial reaction to set in). The GFAP expression was then compared with those in control animals in which the microelectrode was implanted in a similar location but was not moved over the duration of the implantation. Rigorous quantitative assessments of GFAP expression and statistical analysis of GFAP levels in the different cohorts were performed. The GFAP response in the brain tissue around the microelectrode that was moved on day 2 was similar to the GFAP response of the brain tissue in the control experiment. However, the level of reactive astrocytes was significantly lower near the tip of the microelectrodes that were moved either on day 14 or day 28 compared to those around microelectrodes in control experiments that were not moved at all. The data from this study suggested that the reactive astrocytic response to implanted microelectrode could be minimized by using a two-step implant procedure where the microelectrode will be moved to the final recording location in two stages. The first stage will be the implantation procedure itself to locate the microelectrode approximately 500 μm or more away from the final destination. The second stage will be a movement of the microelectrode to the final destination 2 weeks after the day of implantation. Longer terms studies lasting more than 56 days will have to be performed to confirm if the above hypothesis is true.
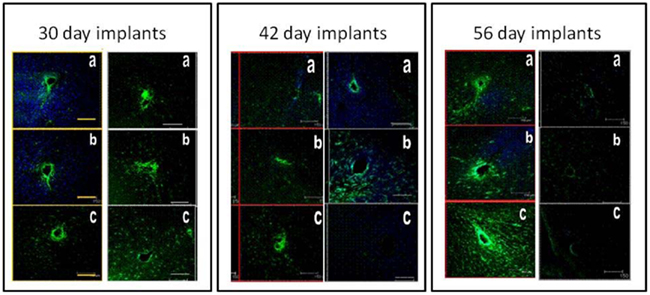
Figure 3. Typical immunohistochemical responses of brain tissue around microelectrodes that moved in the brain by 500 μm at days 2 (30-day implants), 14 (42-day implants), and 28 (56-day implants) after implantation. Each of the three panels show tissue sections from control animals (left column in each panel) and the experimental animals (right column in each panel). The brain sections from experimental animals where the microelectrode was moved at days 14 and 28 after implantation show significantly reduced GFAP expression (indicating reduced reactive astrocytic response) compared to the corresponding control brain sections. The tissue sections are perpendicular to the direction of the microelectrode shank. GRAP expression is shown in green and MAP2 expression is shown in blue.
Tissue Micromotion
The second key gap in our understanding of the neuron–electrode interface is the level of relative micromotion between the microelectrode (that is often fixed to the skull) and the surrounding brain tissue. Brain tissue moves relative to the microelectrode due to pulsations in the vasculature, propagating mechanical pressure waves due to breathing (that can be eliminated in acute experiments by performing a pneumothoraces), and also due to animal behavior. It is hypothesized that tissue micromotion is less of a challenge while recording from neurons in the sub-cortical and other deep brain structures, but this is not proven yet. The level of tissue micromotion is also species dependent with large relative displacements observed in cats and humans, and smaller, less catastrophic displacements observed in rodent models. Displacements of 100–250 μm due to vascular pulsations, 300–900 μm due to breathing were observed in the brainstem of cats using an optical interferometry approach (Britt and Rossi, 1982). In humans, brains can displace and have torsional displacements over several millimeters under certain behavioral conditions. On the other hand, anesthetized rat models have periodic cortical tissue displacements of 1–4 μm due to pulsations in the brain vasculature and 10–30 μm due to propagating pressure waves during breathing. Anecdotal evidence seems to suggest approximately 30–40 μm of brain tissue displacement in the cortex while an adult rat was walking on a treadmill.
So what is the impact of these relative displacements between the recording microelectrode and the surrounding brain tissue on recordings from single neurons in the brain? Is the technology of moveable microelectrode capable of providing an optimal solution to mitigate the deleterious consequences of tissue micromotion. Unfortunately, data on relative micromotion between implanted microelectrode and the surrounding brain tissue remains sparse leaving us with more questions than answers at this point. At the same time, there is enough evidence from anecdotal experiments, and short-term studies suggesting that relative tissue micromotion most commonly associated with routine behavioral motions and tasks could have a significant impact on the electrical recordings from single neurons (Fee, 2000; Ryu and Shenoy, 2009). The impact of tissue micromotion on recordings from single neurons may be at least twofold with a direct (short-term) and indirect (long-term) impact. Examples of short-term impact of micromotion are as follows. There have been several anecdotal and published results indicating significant change in electrical recordings from single neurons during behavior related movements (Fee and Leonardo, 2001; Chestek et al., 2009) that might have been mediated by a relative micromotion between the microelectrode and surrounding brain tissue. Conversely, several recent studies have also proven that occasional purposeful movement of microelectrodes either manually or using motors results in restoration of fading action potentials and therefore increased stability of neuronal recordings (Jackson and Fetz, 2007; Yamamoto and Wilson, 2008). Experience from the above studies seems to suggest that the deterioration or loss of recordings from specific single neurons was caused by an increase in distance between the microelectrode and the single neuron being recorded. The reasons for such presumed increase in distance between the microelectrode and the single neuron are speculative in the above studies.
The long-term impact, if any of tissue micromotion on the recordings from single neurons remains unknown. It is possible that chronic shear stresses, however low that might be at the brain–electrode interface caused by pulsations in the vasculature and the propagating mechanical waves during breathing and behavior related tasks, can potentially lead to long-term changes in the cellular homeostasis. Data from in vitro studies in traumatic brain injury involving primary neuronal cultures suggest that the spectrum of induced cellular pathologies that are possible due to such induced mechanical stresses include intracellular calcium ion elevation, immediate early gene (IEG) expression, and even neuronal necrosis depending on the level of induced stress at the interface (Laplaca and Prado, 2010). All of the above cellular changes can potentially lead to moderate to significant changes in the neuronal recording.
So how does a behavior related movement of the animal get transduced to a change in the electrical recording from implanted microelectrodes? It is intuitive to expect that relative displacements between the recording site of the microelectrode and the neuron(s) in the surrounding brain tissue will cause changes in the amplitudes and shapes of the recorded neuronal signal. Depending on the brain region being monitored (and the associated spatial distribution and orientation of individual neurons), and also the relative position of the implanted microelectrode with respect to cortical fissures (sulci), there could be several tens of microns of relative displacement between the microelectrode and the surrounding brain tissue before we see any significant change in the action potential(s) recorded. Prediction of any change in the electrical recording from single neurons due to the relative micromotion between the brain tissue and the recording microelectrode also becomes complicated due to the viscoelastic (and sometimes hyperelastic) nature of the surrounding brain tissue. That means, the real distance between the recording site of the microelectrode and the neuron that is being recorded will have a very different trend as a function of time compared to the propagating pressure waves (due to animal behavior primarily) that cause the tissue micromotion.
So is it possible to build a model using the constitutive mechanical properties of the brain tissue and deconvolve the actual distance between the recording site and recorded neuron from known pressure waves that propagate through the brain? The answer is yes, but the accuracy of such deconvolution will depend on the accuracy of the constitutive properties of brain tissue used. Adding to this complexity is the fact that the brain–electrode interface undergoes significant changes (in its composition, texture, and function) in the weeks following the implantation of the microelectrode (Polikov et al., 2005; Marin and Fernandez, 2010). A range of different constitutive properties of the brain have been used in mechanical models of brain tissue in prior studies.
Control Algorithms
The second part of a system that can move microelectrodes and record from specific neurons is the control algorithm that drives the hardware. Typically, the measured variable is some metric of signal quality (signal-to-noise ratio or peak-to-peak amplitude of the action potential) of the single neuron or average SNR and peak-to-peak amplitude in the case of multi-unit activity. So the typical sequence of operation of an autonomous microelectrode system would be as follows: (a) spike detection (b) spike sorting, if there are multiple units (c) compare with optimized action potential amplitudes that were recorded earlier and compute metrics of signal quality and signal deviation or deterioration (if any) (d) compute a control signal for the micromotor that will either move the microelectrode or leave the microelectrode unmoved (e) go back to step (a). Broadly, there have been three kinds of efforts so far to implement control algorithms:
(a) Manually operated or screw based microdrives where the experimenter adjusts the position of the microelectrode (Bragin et al., 2000; Wilson et al., 2003; Jackson and Fetz, 2007).
(b) Semi-automated systems where the microelectrodes are driven by motors and the experimenter moves them to seek good quality recordings through external inputs (Fee and Leonardo, 2001; Yamamoto and Wilson, 2008).
(c) Fully automated systems where the microelectrodes automatically position themselves and are programmed to provide high quality recordings or recordings from specific population of neurons without any external monitoring (Wolf et al., 2009).
The last category of controls is desirable for cortical prosthetic applications that work in a closed-loop system along with neural signal decoders to operate a prosthetic device. Such prosthetic applications require on-demand, reliable single neuronal signals typically from the motor and/or pre-motor cortical locations. An additional requirement of prosthetic application is that such systems operate reliably over the life-time of a patient. However, prosthetic systems do not necessarily require a stable interface with single neurons. The decoders can be made to adapt to the best single neuronal recording that is available at any given instant without significant loss of efficiency.
Control algorithms designed for movable microelectrodes would be broadly required to meet the following end-goals:
(a) Automated/remote positioning of microelectrodes with decreased manual intervention and handling of awake animals, particularly in the case of small animal models such as song birds and rodents.
(b) Stability of recordings from single neurons of interest (in basic neurobiological studies on learning, memory, and plasticity; as mentioned above, not a requirement for prosthetic applications).
(c) Increase in yield of active electrodes with well-isolated units.
There is no clear consensus on a single control strategy to meet all of the above requirements. Rudimentary automation can be achieved by recording segments of neural signal, sorting the spike waveforms, identifying well-isolated clusters, and maximizing the SNR of sorted units. More rigorous controls based on stochastic models have been used to position the microelectrodes at the start of each recording session to isolate specific units (Wolf et al., 2009). However, such models ignore the mechanical properties of the medium (the brain tissue, in this case) through which the microelectrode is moving. The actual displacement of the microelectrode in brain cannot be determined as the viscoelastic properties of the brain tissue are not well understood (McConnell et al., 2007; Clayton et al., 2011; Elkin et al., 2011). Tissue compression and relaxation during microelectrode movement can also contribute to variability in the relative position between the electrode and neuron that would naturally lead to variabilities in neuronal recordings. A thorough understanding of tissue biomechanics is therefore required to more accurately predict the location of the microelectrode with respect to the neuron. The dynamics of micromotion between the brain tissue and the implanted microelectrode will also dictate the requirements of response time of such controlled microelectrode movement machinery. An example of forces experienced by a microelectrode during penetration of the adult rodent brain is shown in Figure 4. The periodicity of these measured forces appears to coincide with respiratory patterns. The peak-to-peak amplitude of these forces is over 200 μN after the microelectrode reaches a depth of 1 mm. If the microelectrode is left in place without any further movement into the brain, the forces relax toward zero typically in a non-uniform manner indicating the presence of non-linearities in the relaxation response of the brain tissue. A control algorithm that compensates for such relative tissue micromotion will have to model such non-linearities if a robust, stable interface with a neuron of interest is desired.
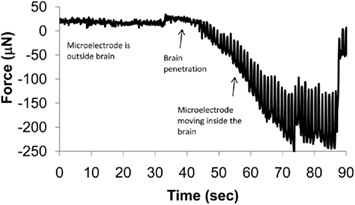
Figure 4. Dynamic changes in force experienced by a 200-μm diameter stainless steel microelectrode with a sharp taper as it penetrates the brain. The microneedle was mounted on a sensitive tension/compression load cell attached to a micromanipulator and inserted into the brain of a stereotaxically immobilized rat. The needle was moved at 10 μm/s. The periodic oscillations in the force are speculated to be due to micromotion of the brain corresponding to the periodic breathing of the animal.
Stability of neural recordings is also influenced by putative factors like neuronal migration, movement, and knocking of implants around the animal cage during animal behavior, influence of circadian rhythms, inherent neuronal circuit variations, and several other unexplored factors (Suner et al., 2005; Liu et al., 2006; Biran et al., 2007; Tolias et al., 2007). The general consensus among users of movable microelectrodes lean toward slow movements of the microelectrode in fine steps with intermediate intervals to allow for tissue relaxation that will yield stable units for longer durations of time (Yamamoto and Wilson, 2008). Also brain micromotion can be countered by using floating electrodes or developing controls that sense the brain micromotion and move the electrodes to offset it (Fee, 2000; Musallam et al., 2007; Vahasoyrinki et al., 2009).
Neuronal yield can be maximized by repositioning all the electrodes in an array with due consideration to tissue displacements from movement of adjacent electrodes. There is no study comparing the yield between stationary and movable electrodes in a chronic experiment and therefore it is not possible to predict increased longevity of movable microelectrode implants as yet. Chronically implanted arrays with movable microelectrodes have previously lasted upto 447 days and yielded 3211 units (Eliades and Wang, 2008). Improvement in the number of units recorded in a particular recording session after repositioning the electrodes have been consistently reported before (Deadwyler et al., 1979; Venkatachalam et al., 1999; Bragin et al., 2000; Fee and Leonardo, 2001; Bilkey et al., 2003; Wilson et al., 2003; Cham et al., 2005; Venkateswaran et al., 2005; Dobbins et al., 2007; Jackson and Fetz, 2007; Sato et al., 2007). Control algorithms that search and optimize for maximum cluster separation and units in all recording channels can probabilistically increase the neuronal yield manifold in an implanted array.
Thus along with miniature movable microelectrode array technology, intelligent adaptive controls would enhance the reliability of the neural recording system and is a promising approach to ensure the stability, consistency, yield, and longevity of neural recordings from implanted microelectrodes. With simultaneous developments in novel packaging and interconnect microtechnologies that will allow these microtechnologies to perform in challenging in vivo behavioral contexts in long-term experiments, the movable microelectrode approach will dramatically enhance the information throughput from single neurons in the brain. Achieving the elusive goal of stable long-term interfaces with single neurons will also facilitate discoveries in neuronal plasticity, learning, and memory studies. In the longer term, the tunable nature of these microelectrodes will allow the possibility of tracking specific neuronal circuits of interest and changes in their connectivity and synaptic strengths with time.
Conclusion
Microelectrodes that can adapt their position in the brain can therefore potentially fulfill a long-standing need in Neurophysiology for stable, consistent, long-term recordings from single neurons in awake behaving animals and also enable reliable single neuronal recordings that are key to the success of emerging cortical prostheses applications. Current movable microelectrode technologies are typically large which hinders the development of large arrays of movable microelectrodes to sample ensembles of neurons. MEMS based technologies outlined here potentially fill the need for movable microelectrode systems that can record from large ensembles of neurons with high yield, stability, and reliability with overall form factors that do not hinder behavior in awake animals and patients. Within the MEMS approaches available, the electrothermal actuation approach offers significant advantages over an electrostatic actuation approach and is therefore recommended for future work. The key knowledge gaps that hinder us from realizing movable microelectrode systems that can record from large numbers of neurons in long-term experiments or over the life-time of a patient are (a) response of the brain tissue surrounding the microelectrode and (b) relative micromotion between the implanted microelectrode and brain tissue surrounding and how these two factors impact neuronal recording in acute and chronic experiments. Further development of moveable microelectrode systems and the optimal control algorithms to move the microelectrodes to achieve the goal of neuronal recordings in chronic experiments or over the life-time of the patient will be guided by new knowledge in the above two areas.
Conflict of Interest Statement
The authors declare that the research was conducted in the absence of any commercial or financial relationships that could be construed as a potential conflict of interest.
Key Concepts
Cortical prostheses
Artificial systems to extract neuronal signals from cortical regions of the brain and translate them into control signals that move a robotic limb or a computer cursor. Efforts are on to develop more advanced cortical prosthetic systems that are also able to incorporate sensory feedback to the brain to provide a more realistic experience for the user of such systems.
Microactuators
Microscale systems that typically convert electrical signals to physical movement. Examples of such systems include motors, speakers, etc. Typical parameters of interest in microactuators include operating voltages or currents, displacement resolution, total displacement, force generated during displacement, etc.
Comb-drive microactuators
Comb-drive electrostatic microactuators reported here use fringe fields generated in response to voltage applied between the two plates (one fixed and one floating) of a parallel plate capacitor to generate force causing the movable plate to move incrementally. A stack of parallel plate capacitors with interdigitated plates (having the appearance of a comb) is used to generate the required forces.
Electrothermal microactuators
Electrothermal microactuators reported here operate on the principle of generating displacement using heat strips that thermally expand in response to application of voltage pulses across them. Displacement in a specific direction is typically achieved by biasing the orientation of the heat strips.
Glial sheath
Gliosis refers to activation of astrocytes in the vicinity of implants in the brain. Over a period of 4 weeks or more after implantation, these activated astrocytes along with other reactive microglia in the brain form a tightly adhered sheath around the implant which is generally hypothesized as a failure mode for implanted recording microelectrodes.
Hyperelastic
Material property that is characterized by non-linear relationship between stress and strain. Typical materials that demonstrate hyperelastic behavior include foam, gel, etc.
Viscoelastic property
Material property that is characterized by a time-varying stress generated in response to a step change in one of its dimensions or conversely a time-varying change in one of its dimensions generated in response to a step change in stress.
References
Battaglia, F. P., Kalenscher, T., Cabral, H., Winkel, J., Bos, J., Manuputy, R., Van Lieshout, T., Pinkse, F., Beukers, H., and Pennartz, C. (2009). The lantern: an ultra-light micro-drive for multi-tetrode recordings in mice and other small animals. J. Neurosci. Methods 178, 291–300.
Bilkey, D. K., Russell, N., and Colombo, M. (2003). A lightweight microdrive for single-unit recording in freely moving rats and pigeons. Methods 30, 152–158.
Biran, R., Martin, D. C., and Tresco, P. A. (2007). The brain tissue response to implanted silicon microelectrode arrays is increased when the device is tethered to the skull. J. Biomed. Mater. Res. A 82, 169–178.
Bragin, A., Hetke, J., Wilson, C. L., Anderson, D. J., Engel, J., and Buzsaki, G. (2000). Multiple site silicon-based probes for chronic recordings in freely moving rats: implantation, recording and histological verification. J. Neurosci. Methods 98, 77–82.
Britt, R. H., and Rossi, G. T. (1982). Quantitative analysis of methods for reducing physiological brain pulsations. J. Neurosci. Methods 6, 219–229.
Cham, J. G., Branchaud, E. A., Nenadic, Z., Greger, B., Andersen, R. A., and Burdick, J. W. (2005). Semi-chronic motorized microdrive and control algorithm for autonomously isolating and maintaining optimal extracellular action potentials. J. Neurophysiol. 93, 570–579.
Chestek, C. A., Cunningham, J. P., Gilja, V., Nuyujukian, P., Ryu, S. I., and Shenoy, K. V. (2009). “Neural prosthetic systems: current problems and future directions,” in Annual International Conference of the IEEE Engineering in Medicine and Biology Society, Minneapolis, MN, 3369–3375.
Clayton, E. H., Garbow, J. R., and Bayly, P. V. (2011). Frequency-dependent viscoelastic parameters of mouse brain tissue estimated by MR elastography. Phys. Med. Biol. 56, 2391–2406.
Deadwyler, S. A., Biela, J., Rose, G., West, M., and Lynch, G. (1979). Microdrive for use with glass or metal microelectrodes in recording from freely moving rats. Electroencephalogr. Clin. Neurophysiol. 47, 752–754.
Decharms, R. C., Blake, D. T., and Merzenich, M. M. (1999). A multielectrode implant device for the cerebral cortex. J. Neurosci. Methods 93, 27–35.
Dickey, A. S., Suminski, A., Amit, Y., and Hatsopoulos, N. G. (2009). Single-unit stability using chronically implanted multielectrode arrays. J. Neurophysiol. 102, 1331–1339.
Dobbins, H. D., Marvit, P., Ji, Y. D., and Depireux, D. A. (2007). Chronically recording with a multi-electrode array device in the auditory cortex of an awake ferret. J. Neurosci. Methods 161, 101–111.
Eliades, S. J., and Wang, X. Q. (2008). Chronic multi-electrode neural recording in free-roaming monkeys. J. Neurosci. Methods 172, 201–214.
Elkin, B. S., Ilankovan, A. I., and Morrison, B. (2011). A detailed viscoelastic characterization of the P17 and adult rat brain. J. Neurotrauma. [Epub ahead of print].
Engel, A. K., Moll, C. K., Fried, I., and Ojemann, G. A. (2005). Invasive recordings from the human brain: clinical insights and beyond. Nat. Rev. Neurosci. 6, 35–47.
Fee, M. S. (2000). Active stabilization of electrodes for intracellular recording in awake behaving animals. Neuron 27, 461–468.
Fee, M. S., and Leonardo, A. (2001). Miniature motorized microdrive and commutator system for chronic neural recording in small animals. J. Neurosci. Methods 112, 83–94.
Gray, C. M., Goodell, B., and Lear, A. (2007). Multichannel micromanipulator and chamber system for recording multineuronal activity in alert, non-human primates. J. Neurophysiol. 98, 527–536.
Grill, W. M., Norman, S. E., and Bellamkonda, R. V. (2009). Implanted neural interfaces: bio challenges and engineered solutions. Annu. Rev. Biomed. Eng. 11, 1–24.
Haiss, F., Butovas, S., and Schwarz, C. (2010). A miniaturized chronic microelectrode drive for awake behaving head restrained mice and rats. J. Neurosci. Methods 187, 67–72.
Hwang, E. J., and Andersen, R. A. (2009). Brain control of movement execution onset using local field potentials in posterior parietal cortex. J. Neurosci. 29, 14363–14370.
Jackson, A., and Fetz, E. E. (2007). Compact movable microwire array for long-term chronic unit recording in cerebral cortex of primates. J. Neurophysiol. 98, 3109–3118.
Jackson, N., Sridharan, A., Anand, S., Baker, M., Okandan, M., and Muthuswamy, J. (2010). Long-term neural recordings using MEMS based movable microelectrodes in the brain. Front. Neuroeng. 3:10.
Kern, T. A., Rorup, H., Werthschutzky, R., and Tammer, R. (2008). A remotely controlled lightweight MRI compatible ultrasonic actuator for micrometer positioning of electrodes during neuroethological primate research. Biomed. Tech. (Berl.) 53, 292–299.
Korshunov, V. A. (2006). Miniature microdrive-headstage assembly for extracellular recording of neuronal activity with high-impedance electrodes in freely moving mice. J. Neurosci. Methods 158, 179–185.
Lansink, C. S., Bakker, M., Buster, W., Lankelma, J., Van Der Blom, R., Westdorp, R., Joosten, R., Mcnaughton, B. L., and Pennartz, C. M. A. (2007). A split microdrive for simultaneous multi-electrode recordings from two brain areas in awake small animals. J. Neurosci. Methods 162, 129–138.
Laplaca, M. C., and Prado, G. R. (2010). Neural mechanobiology and neuronal vulnerability to traumatic loading. J. Biomech. 43, 71–78.
Liu, X., Mccreery, D. B., Bullara, L. A., and Agnew, W. F. (2006). Evaluation of the stability of intracortical microelectrode arrays. IEEE Trans. Neural Syst. Rehabil. Eng. 14, 91–100.
Marin, C., and Fernandez, E. (2010). Biocompatibility of intracortical microelectrodes: current status and future prospects. Front. Neuroeng. 3:8.
McConnell, G. C., Rees, H. D., Levey, A. I., Gutekunst, C. A., Gross, R. E., and Bellamkonda, R. V. (2009). Implanted neural electrodes cause chronic, local inflammation that is correlated with local neurodegeneration. J. Neural Eng. 6, 056003.
McConnell, G. C., Schneider, T. M., Owens, D. J., and Bellamkonda, R. V. (2007). Extraction force and cortical tissue reaction of silicon microelectrode arrays implanted in the rat brain. IEEE Trans. Biomed. Eng. 54, 1097–1107.
McCreery, D., Pikov, V., and Troyk, P. R. (2010). Neuronal loss due to prolonged controlled-current stimulation with chronically implanted microelectrodes in the cat cerebral cortex. J. Neural Eng. 7, 036005.
Mulliken, G. H., Musallam, S., and Andersen, R. A. (2008). Decoding trajectories from posterior parietal cortex ensembles. J. Neurosci. 28, 12913–12926.
Musallam, S., Bak, M. J., Troyk, P. R., and Andersen, R. A. (2007). A floating metal microelectrode array for chronic implantation. J. Neurosci. Methods 160, 122–127.
Muthuswamy, J., Okandan, M., Gilletti, A., Baker, M. S., and Jain, T. (2005a). An array of microactuated microelectrodes for monitoring single-neuronal activity in rodents. IEEE Trans. Biomed. Eng. 52, 1470–1477.
Muthuswamy, J., Okandan, M., Jain, T., and Gilletti, A. (2005b). Electrostatic microactuators for precise positioning of neural microelectrodes. IEEE Trans. Biomed. Eng. 52, 1748–1755.
Park, S., Yoon, E., Lee, S., Shin, H. S., Park, H., Kim, B., Kim, D., and Park, J. (2008). The development of a PZT-based microdrive for neural signal recording. Smart Mater. Struct. 17, 1–7.
Polikov, V. S., Tresco, P. A., and Reichert, W. M. (2005). Response of brain tissue to chronically implanted neural electrodes. J. Neurosci. Methods 148, 1–18.
Ryu, S. I., and Shenoy, K. V. (2009). Human cortical prostheses: lost in translation? Neurosurg. focus 27, E5.
Sato, T., Suzuki, T., and Mabuchi, K. (2007). A new multi-electrode array design for chronic neural recording, with independent and automatic hydraulic positioning. J. Neurosci. Methods 160, 45–51.
Scherberger, H., Jarvis, M. R., and Andersen, R. A. (2005). Cortical local field potential encodes movement intentions in the posterior parietal cortex. Neuron 46, 347–354.
Stice, P., and Muthuswamy, J. (2009). Assessment of gliosis around moveable implants in the brain. J. Neural Eng. 6, 046004.
Suner, S., Fellows, M. R., Vargas-Irwin, C., Nakata, G. K., and Donoghue, J. P. (2005). Reliability of signals from a chronically implanted, silicon-based electrode array in non-human primate primary motor cortex. IEEE Trans. Neural Syst. Rehabil. Eng. 13, 524–541.
Swadlow, H. A., Bereshpolova, Y., Bezdudnaya, T., Cano, M., and Stoelzel, C. R. (2005). A multi-channel, implantable microdrive system for use with sharp, ultra-fine “Reitboeck” microelectrodes. J. Neurophysiol. 93, 2959–2965.
Szarowski, D. H., Andersen, M. D., Retterer, S., Spence, A. J., Isaacson, M., Craighead, H. G., Turner, J. N., and Shain, W. (2003). Brain responses to micro-machined silicon devices. Brain Res. 983, 23–35.
Tolias, A. S., Ecker, A. S., Siapas, A. G., Hoenselaar, A., Keliris, G. A., and Logothetis, N. K. (2007). Recording chronically from the same neurons in awake, behaving primates. J. Neurophysiol. 98, 3780–3790.
Vahasoyrinki, M., Tuukkanen, T., Sorvoja, H., and Pudas, M. (2009). A minimally invasive displacement sensor for measuring brain micromotion in 3D with nanometer scale resolution. J. Neurosci. Methods 180, 290–295.
Venkatachalam, S., Fee, M. S., and Kleinfeld, D. (1999). Ultra-miniature headstage with 6-channel drive and vacuum-assisted micro-wire implantation for chronic recording from the neocortex. J. Neurosci. Methods 90, 37–46.
Venkateswaran, R., Boldt, C., Ziaie, B., Erdman, A. G., and Redish, A. D. (2005). A motorized microdrive for recording of neural ensembles in awake behaving rats. J. Biomech. Eng. 127, 1035–1040.
Vetter, R. J., Williams, J. C., Hetke, J. F., Nunamaker, E. A., and Kipke, D. R. (2004). Chronic neural recording using silicon-substrate microelectrode arrays implanted in cerebral cortex. IEEE Trans. Biomed. Eng. 51, 896–904.
Wilson, F. A. W., Ma, Y. Y., Greenberg, P. A., Ryou, J. W., and Kim, B. H. (2003). A microelectrode drive for long term recording of neurons in freely moving and chaired monkeys. J. Neurosci. Methods 127, 49–61.
Winslow, B. D., and Tresco, P. A. (2010). Quantitative analysis of the tissue response to chronically implanted microwire electrodes in rat cortex. Biomaterials 31, 1558–1567.
Wolf, M. T., Cham, J. G., Branchaud, E. A., Mulliken, G. H., Burdick, J. W., and Andersen, R. A. (2009). A robotic neural interface for autonomous positioning of extracellular recording electrodes. Int. J. Robot Res. 28, 1240–1256.
Yamamoto, J., and Wilson, M. A. (2008). Large-scale chronically implantable precision motorized microdrive array for freely behaving animals. J. Neurophysiol. 100, 2430–2440.
Keywords: neural prostheses, microelectrode, MEMS, microsystems, implantable microtechnologies
Citation: Muthuswamy J, Anand S and Sridharan A (2011) Adaptive movable neural interfaces for monitoring single neurons in the brain. Front. Neurosci. 5:94. doi: 10.3389/fnins.2011.00094
Received: 08 April 2011; Paper pending published: 17 May 2011;
Accepted: 14 July 2011; Published online: 08 September 2011.
Edited by:
Martin Stelzle, University of Tübingen, GermanyReviewed by:
Leandro Lorenzelli, Fondazione Bruno Kessler, ItalyPaolo Medini, The Italian Institute of Technology, Italy
Copyright: © 2011 Muthuswamy, Anand and Sridharan. This is an open-access article subject to a non-exclusive license between the authors and Frontiers Media SA, which permits use, distribution and reproduction in other forums, provided the original authors and source are credited and other Frontiers conditions are complied with.
*Correspondence: Prof. Jit Muthuswamy, Arizona State University, School of Biological and Health Systems Engineering, Tempe, 85287-9709, AZ, USA,aml0QGFzdS5lZHU=Miss. Sindhu Anand, Arizona State University, School of Biological and Health Systems Engineering, Tempe, 85287-9709, AZ, USA,c2FuYW5kOEBhc3UuZWR1Dr. Arati Sridharan, Arizona State University, School of Biological and Health Systems Engineering, Tempe, 85287-9709, AZ, USA,YXNyaWRoYXJAYXN1LmVkdQ==