- 1 Machine Learning Laboratory, Berlin Institute of Technology, Berlin, Germany
- 2 Department of Intelligent Data Analysis, Fraunhofer FIRST, Berlin, Germany
Brain–computer interfaces (BCIs) based on event related potentials (ERPs) strive for offering communication pathways which are independent of muscle activity. While most visual ERP-based BCI paradigms require good control of the user’s gaze direction, auditory BCI paradigms overcome this restriction. The present work proposes a novel approach using auditory evoked potentials for the example of a multiclass text spelling application. To control the ERP speller, BCI users focus their attention to two-dimensional auditory stimuli that vary in both, pitch (high/medium/low) and direction (left/middle/right) and that are presented via headphones. The resulting nine different control signals are exploited to drive a predictive text entry system. It enables the user to spell a letter by a single nine-class decision plus two additional decisions to confirm a spelled word. This paradigm – called PASS2D – was investigated in an online study with 12 healthy participants. Users spelled with more than 0.8 characters per minute on average (3.4 bits/min) which makes PASS2D a competitive method. It could enrich the toolbox of existing ERP paradigms for BCI end users like people with amyotrophic lateral sclerosis disease in a late stage.
1 Introduction
Human communication is based on muscle activity: we speak, write, make use of facial expressions or simply point to transmit information. This work contributes to the area of research which investigates communication interfaces that do not rely on muscle activity but are controlled by brain signals instead.
With a brain–computer interface (BCI) a user can send control signals to an application even if he/she is unable to control any muscle. Recording the electroencephalogram (EEG) of a user, his/her brain signals can be analyzed and classified in real-time by the BCI. Next to non-clinical applications (Tangermann et al., 2009; Blankertz et al., 2010), most research in this field claims to develop tools for patients with locked-in syndrome (LIS) or complete locked-in syndrome (CLIS). While the LIS is defined as a state of almost complete paralysis with remaining voluntary gaze direction control or eye blink control, CLIS patients have lost the volitional control over all muscles. BCI might be the only technology which could establish a communication channel for these patients (Birbaumer et al., 1999).
Patients are not alike. Especially patients suffering from neurodegenerative diseases like amyotrophic lateral sclerosis (ALS) have an individual course of their disease. In order to account for their individual abilities and needs, it is crucial to develop a set of different tools to provide a communication pathway for each patient in every state. The auditory event related potential (ERP) speller presented in this work might be a helpful tool for patients in CLIS or close to CLIS.
Visual matrix speller paradigms, introduced by Farwell and Donchin (1988), are still intensely studied (Krusienski et al., 2008; Guger et al., 2009; Bianchi et al., 2010; Kleih et al., 2010; Jin et al., 2011). The neutral Matrix Speller (Farwell and Donchin, 1988) has shown good results (i.e., fast and reliable) for healthy subjects and people with ALS disease (Sellers and Donchin, 2006; Nijboer et al., 2008b), but recent studies showed that the spelling performance drops dramatically if the subject does not focus the target (Brunner et al., 2010; Treder and Blankertz, 2010). This might render a visual ERP paradigm unusable for people with ALS disease, since they are often unable to reliably maintain gaze. To overcome this problem, ERP speller paradigms that use “center fixation” were recently introduced and tested with healthy subjects (Acqualagna et al., 2010; Treder and Blankertz, 2010; Liu et al., 2011). Another approach of a speller paradigm (Blankertz et al., 2007; Williamson et al., 2009) uses motor imaginary control instead of visual ERP to select letters from a hexagonal group structure and gives visual feedback. With both mentioned approaches, the user does not need to fine-control his/her gaze direction but has to be able to maintain a constant gaze direction, since feedback is given in the visual modality.
Another way to deal with visual impairments is to use auditory paradigms, since even patients in late stage ALS are usually able to hear. Although listening to auditory stimuli is more demanding than attending visual stimuli (Klobassa et al., 2009), it is possible to set up ERP spellers that exclusively use auditory stimuli (Nijboer et al., 2008a; Furdea et al., 2009; Klobassa et al., 2009; Kübler et al., 2009; Schreuder et al., 2009, 2010; Guo et al., 2010; Halder et al., 2010). Those paradigms are typically less accurate and slower than similar visual ERP paradigms, but as Murguialday et al. (2011) recently pointed out, an auditory BCI paradigm is one of the only remaining communication channels for patients in CLIS.
In an approach by Klobassa et al. (2009), 36 characters and symbols were arranged in a 6 × 6 matrix. Each row and column is represented by one of six different sounds. A character is chosen by first attending to the sound representing its row and then to the corresponding column. Thus, each selection of a symbol requires two steps.
A similar approach was studied by Furdea et al. (2009), where 25 letters are presented in a 5 × 5 grid. In this paradigm, each row and column is coded by a number which is presented auditorily. This paradigm was tested with severely paralyzed patients in the end-stage of neurodegenerative diseases (Kübler et al., 2009).
To increase discriminability of auditory stimuli, there are recent approaches for multiclass BCI paradigms (Schreuder et al., 2009, 2010) that include a second spatial dimension. In their paradigm, stimuli are varying in two dimensions (pitch and direction) with both dimensions transmitting the same (redundant) information: a tone with a specific pitch was always presented from the same direction. Letters are organized in six groups and each selection requires two steps.
There are other auditory paradigms for BCI which are not linked to speller applications. In Hill et al. (2005) and Kim et al. (2011), the authors proposed paradigms where subjects focused attention to one of two concurrent auditory stimulus sequences. Both sequences have individual target events and stimulus onset asynchronies (SOA, also called inter stimulus onset interval, or ISOI). A similar approach was described by Kanoh et al. (2008) with two sequences having the same SOA. Those paradigms enable a binary selection. Halder et al. (2010) proposed a three-stimulus paradigm with two target stimuli and one frequent stimulus. Subjects attend to one specified target stimulus, masking the other two non-target events. It was found that a paradigm with targets differing in pitch performs better than a paradigm with targets differing in loudness or direction. Furthermore, there are recent studies that investigate ERP correlates for perceived and imagined rhythmic auditory patterns which can potentially be used for BCI paradigms in the future (Schaefer et al., 2011).
This work presents an approach to combine several characteristics of the above mentioned work by using novel spatial auditory stimuli in a nine-class paradigm. Similarly to the approach of Schreuder et al. (2010) the auditory stimuli applied are varying in both, pitch (high, medium, low) and direction (left, middle, right). However, the information transmitted by the two dimensions is independent and not redundant as in Schreuder’s approach. The resulting 3 × 3 design offers an arrangement of nine stimuli that are easy to discriminate from each other. The effectiveness of this nine-class BCI is proven in a spelling application. It establishes an intuitive spelling procedure that can be used even under the presence of visual impairments. Initial results of this new approach have been presented in Höhne et al. (2010).
The paradigm was named “Predictive Auditory Spatial Speller with two-dimensional stimuli,” or PASS2D. Twelve healthy participants were asked to spell two sentences in an online experiment with the finding that PASS2D is more accurate and faster than most of the auditory ERP spellers previously reported.
2 Materials and Methods
2.1 Participants
Twelve healthy volunteers (9 male, mean age: 25.1 years, range: 21–34, all non-smokers) participated in a single session of a BCI experiment. Table 1 provides details about the age and sex of the participants. A session consisted of a calibration phase first and a subsequent online spelling part. It lasted 3–4 h. Subjects were not paid for participation. Two of them (VPmg and VPja) had previous experience with BCI. Each participant provided informed consent and did not suffer from a neurological disease and had normal hearing. The analysis and presentation of data was anonymized. Two subjects (VPnx and VPmg) were excluded from the online phase due to a poor estimated classification performance based on the calibration data.
2.2 Data Acquisition
Electroencephalogram signals were recorded monopolarly using a Fast’n Easy Cap (EasyCap GmbH) with 63 wet Ag/AgCl electrodes placed at symmetrical positions based on the International 10–20 system. Channels were referenced to the nose. Electrooculogram (EOG) signals were recorded in addition. Signals were amplified using two 32-channel amplifiers (Brain Products), sampled at 1 kHz and filtered by an analog bandpass filter between 0.1 and 250 Hz. Further analysis was performed in Matlab. The online feedback was implemented as pythonic feedback framework (PyFF; Venthur et al., 2010). After applying the analog filter, the data was low-pass filtered to 40 Hz and down sampled to 100 Hz. The data was then epoched between −150 and 800 ms relative to each stimulus onset, using the first 150 ms as a baseline.
2.3 Experimental Design
Figure 1 shows the course of the experiment. Both parts of the experiment consisted of an auditory oddball task where the participants were asked to focus on target stimuli while ignoring all non-target stimuli. Participants were asked to minimize eye movements and the generation of any other muscle artifacts during all parts of the experiment. They sat in a comfortable chair facing a screen that showed the visual representation of a 3 × 3 pad with 9 numbers ordered row-wise (see Figure 2). Light neckband headphones (Sennheiser PMX 200) were positioned comfortably. While preparing the EEG cap, the participants got used to the tonal character and speed of the stimulus presentation, by listening to the stimuli that were used in the spelling paradigm later on.
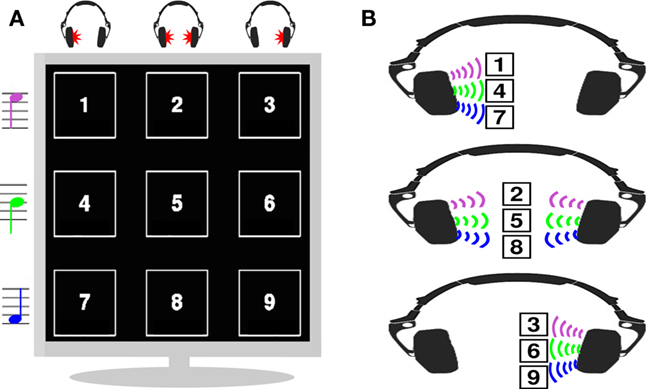
Figure 2. Visualization of the nine auditory stimuli, varying in pitch and direction. The 3 × 3 design (A) was shown on the screen. (B) Distribution of the nine stimulus tones to the stereo channel of the headphone.
2.3.1 Collection of calibration data
Three calibration runs have been recorded per subject. To differentiate target and non-target subtrials in later experimental stages, the collected data was used subsequently for the training of a binary classifier as described in Section 2.5. Each calibration run consisted of nine trials (i.e., nine multiclass selections) with each of the nine sounds being target during one of the trials, see Figure 1. In addition, one practice-run (run 0) without recording was performed initially. Prior to the start of each calibration trial, the current target cue was presented to the subject three times while in addition the corresponding number on the 3 × 3 grid was highlighted on the screen.
During the calibration phase, each trial consisted of 13 or 14 pseudo-random sequences of all nine auditory stimuli. Visual stimuli were not given during these trials. While the last 12 sequences were used to train the classifier, the first one or two sequences were dismissed to ensure a balanced distribution of stimuli in the calibration data. The presentation of one tone stimulus and the corresponding EEG data (an epoch of up to 800 ms after a stimulus) is named subtrial. Thus a single trial provided 9 × 12 subtrial epochs (12 target subtrials and 8 × 12 non-target subtrials) for the classifier training. The combined training data from all runs comprised 108 × 27 = 2916 subtrial epochs and per person (minus a small fraction of artifactual epochs that were discarded).
Participants were asked to count the targets and to report the number of occurrences at the end of each trial (counting task). A simple minimum/maximum-threshold method was applied to exclude artifactual epochs from the calibration data: epochs were rejected if their peak-to-peak voltage difference in any channel exceeded 100 μV.
2.3.2 Online spelling task
After the calibration of the binary classifier (see Section 2.5), two online spelling runs were performed. Subjects were asked to spell a short German sentence (“Klaus geht zur Uni”) composed of 18 characters (including space characters) and a long sentence composed of 36 characters (“Franz jagt im Taxi quer durch Berlin”) in separate runs, see Figure 1. The task was to finish both sentences without mistakes, thus each false selection had to be corrected.
The order of the sentences was randomized. In order to help the participants to remember the text, the whole sentence was printed on a sheet of paper, which was placed next to the screen. Each trial consisted of 135 subtrials – 15 iterations of 9 stimuli (see Figure 1). Artifact correction was not applied in the online runs.
2.4 Auditory Stimuli
The selection of stimuli is a crucial element for any kind of ERP BCI system. Here, three tones varying in pitch (high/medium/low) and tonal character were carefully chosen in a way that they are – on a subjective scale – as different as possible from each other. The tones were generated artificially with 708 Hz (high), 524 Hz (medium), and 380 Hz (low) as base frequencies. Each tone was presented on the headphones with three different directions: only on the left channel, only on the right channel, and on both channels. This two-dimensional 3 × 3 design obeys a close analogy to the number pad of a standard mobile phone (see Figure 2), where, e.g., key 4 is represented by the medium tone pitch (used for keys 4, 5, and 6) and was presented on the left channel only (used for keys 1, 4, and 7).
Each stimulus lasted 100 ms, SOA was 225 ms (see Figure 1), and a low-latency USB sound card (Terratec DMX 6Fire USB) was used to reduce latency and jitter. The pseudo-random sequences of stimuli were generated such that two subsequent stimuli did not have the same pitch. Moreover, the same stimulus was repeated only after at least three other stimuli had appeared. During the calibration and during the spelling of the short sentence, the stimulus classes were sequence-wise balanced1.
The visual domain was only used to report which selections were made, which text had already been spelled, or which words were available to choose from in the so called control mode (see Figure 3B and Section 2.6 for an explanation of the two system modes during the online spelling phase).
2.5 Classification
Binary classification of target and non-target epochs was performed using a (linear) Fisher discriminant analysis (FDA). The features used for classification consisted of two to four amplitude values per channel (2.6 on average). These values represented the mean potential in intervals of epochs that were discriminative for the classification task. The intervals were selected manually based on a discriminance analysis (r-square values of targets vs. non-targets) of the calibration data. Due to the large dimensionality of the features [{2,3,4} intervals × 63 channels = {126, 189, 253} features], a shrinkage method (Blankertz et al., 2011) was applied to regularize the FDA classifier. All 2916 subtrial epochs (minus those discarded with artifact rejection) were used to train the classifier.
The classification error for unseen data was estimated by cross validation. Due to the global selection of feature interval borders, a slight over-optimistic estimate was accepted, as the performance during the online task in a later step would provide a precise error measure.
In the online spelling task, the 1 out of 9 multiclass decisions were based on a fixed number (n = 135) of subtrials and their classifier outputs. To select the attended key based on the 15 classifier outputs for every key, a one sided t-test with unequal variances was applied for each key and the most significant key (i.e., the one with the lowest p-value) was chosen.
2.6 Predictive Text System
For the presented ERP speller, the commonly used T9 predictive text system from mobile phones [discussed in Dunlop and Crossan (2000)] was applied in a modified version. A similar approach was presented in Jin et al. (2010) in order to effectively communicate Chinese characters in a visual BCI paradigm.
The standard T9 system uses more than nine keys: key “1” codes for dot/comma, keys “2” to “9” code for the alphabet, “0” for space, “+,” and “#” for symbols or further functions. The system was modified such that instead of the 12 keys mentioned above, only nine keys were needed for spelling while remaining an intuitive control scheme. The system was constrained to words in a corpus of about 10,000 frequently used words of the German language, which can be arbitrarily extended.
To overcome the problem of having a spelling scheme that is easy and intuitive to use and on the other hand flexible and fast with only nine keys, two modes were implemented: A spelling mode and a control mode, see Figure 3.
In analogy to the predictive text system in mobile phones, a word was spelled by entering a sequence of keys. To spell a character, the user had to select the corresponding key (“2” to “9”) in the spelling mode. Each key codes for three or four characters, see Figure 3A.
After selecting the correct sequence of keys for a specific word, the user chose key “1” to switch the system into the control mode. In this mode, he sees the desired word in a list – together with all other words which can be represented by the entered sequence. By choosing one of the keys “4” to “8” he determines the desired word with one additional selection step. The list of matching words is ordered such that more frequent words (i.e., they have a higher rank according to the underlying corpus) are represented by smaller key numbers. As an example: after entering the keys “6346361” the user can choose from “nehmen,” “meinem,” “meinen,” and “Nehmen” (see Figure 3B) as all these words can be represented by the entered sequence of keys. In the control mode, the system is limited to present a maximum list size of five words, which was sufficient to spell each word of the underlying corpus.
In case the user performed an erroneous multiclass selection, he could correct it by one to three additional selections. If the selection of the last sequence key did not conform to the corpus (there was no word in the corpus that fitted the entered code), it was not accepted and could be corrected with only one selection. If the mode was changed by mistake, it took one selection to return to the correct mode and another to choose the right key. In all other cases of erroneous selections, it took the user two selections to delete an erroneous key (change the mode by entering “1” and then delete last the key by entering “3”) and a third selection to enter the intended key.
3 Results
3.1 Behavioral Data
Table 1 shows the results of the counting task during the calibration phase. Row “|diff| in counts” contains the sum of the absolute differences between the correct and the reported number of target presentations for each trial. A variation ranging from 1 to 35 was observed, which also indicates a varying ability across subjects to discriminate between the stimuli. It can be seen that this behavioral data is not directly linked to the spelling performance: some subjects (VPny, VPoe) have poor behavioral results – i.e., inaccurate counting – but perform well in the online spelling. On the other hand, those subjects with a bad spelling performance did not have particularly bad behavioral results (see VPmg and VPnx). Based on these results, the behavioral data alone could not be used as a predictor for the spelling performance in the online phase.
3.2 Offline Binary Accuracy
The accuracy of a binary decision (based on the epoch of one subtrial) was estimated on the calibration data for each participant. Based on the estimated errors, participants VPnx and VPmg were excluded from the following online experiments due to the poor binary classification performance of less than 70% (classwise balanced). A cross validation analysis (see Table 1) revealed that on average over all 10 remaining subjects, 77.7% of the stimuli were correctly classified. To account for the in balance between non-targets and targets, the classwise balanced accuracy was used, which is the average decision accuracy across classes (target vs. non-target, chance level 50%).
3.3 Location and Latency of N200 and P300
Figure 4 depicts the grand average ERPs at electrodes “Cz” and “FC5” together with the corresponding scalp maps for two time intervals. As expected, the ERPs for the non-target stimuli (gray lines) show a regular pattern that reflects the neural processing of the auditory stimuli. It occurs every 225 ms and is dominated by a N200 component. Moreover, those plots illustrate the different EEG signatures of the non-targets and the targets. At frontal electrodes a lateral and symmetric class discriminative negativity is observed 230–300 ms after stimulus onset. It directly follows up on the N200 component. For simplicity reasons it will be referred to as the N200 component in the following. Starting from approximately 350 ms after the stimulus onset, a second class-discriminant interval is observed for target stimuli. It is a symmetric positive component located at central electrodes and will be referred to as the P300 component in the following. The amount of class discriminability that is contained in the two electrodes during different time intervals is represented by two colored bars (Figure 4B). They depict a modified version of the area-under-the ROC-curve (AUC), which is in the range of [0, 1]. However, the AUC does not provide information about the direction of an effect. Thus, we used a simple modification of the AUC which is signed and linearly scaled to the range of [−1,1]. The resulting loss will be referred to as ssAUC in the following. Positive ssAUC values are colored in red and represent time intervals where target ERP amplitudes are larger than non-target ERP amplitudes. Negative ssAUC values are colored in blue and represent time intervals with target ERP amplitudes smaller than non-target ERP amplitudes.
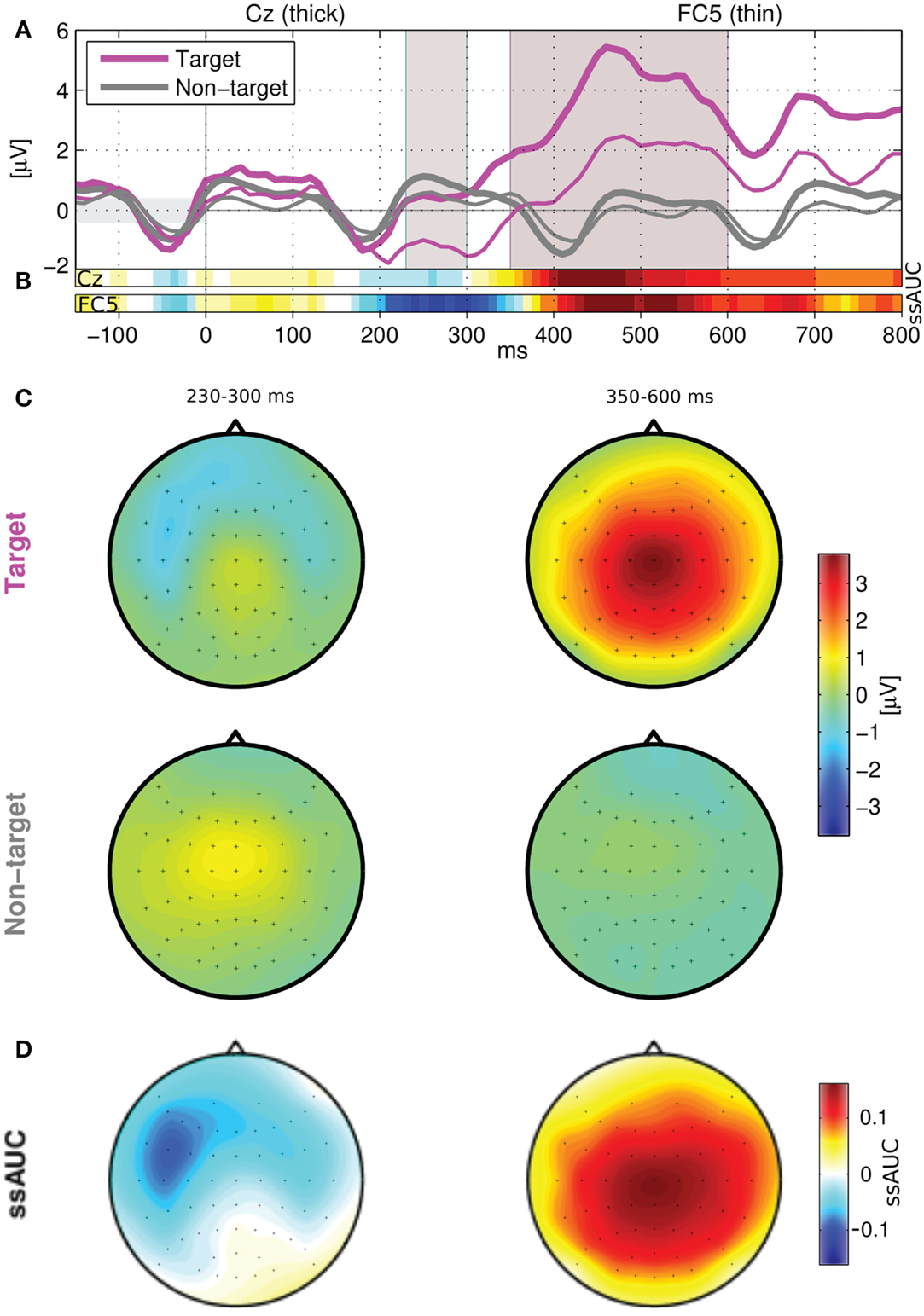
Figure 4. (A) Grand averaged ERPs at electrodes Cz (thick lines) and FC5 (thin lines). The ssAUC bars (B) quantify the discriminative information for the two channels. The averaged ERP scalp maps of target and non-target stimuli for the two marked time frames are shown in (C) and the class discriminability (ssAUC values) is depicted in (D). The scales for (B) and (D) are equal.
Due to the contra-lateral processing of auditory stimuli (Langers et al., 2005), the N200 was expected to vary for each stimulus. Figure 5A depicts the grand average ssAUC scalp maps of N200 for each of the nine stimuli, illustrating that the early negative deflection is spatially varying for different auditory stimuli, but not the P300 (Figure 5B). In most multiclass ERP paradigms the classification is based on a two-class problem (target vs. non-target). Thus, the fact that there might be a variability in the spatial (or temporal) distribution of discriminant information for different stimuli is mostly disregarded. Although the classification procedure in the presented approach is also based on two-class decision, Figure 5A shows that there is some spatial class-discriminant information, which is not exploited yet. Generally, the discriminative information of the N200 component seems to be stronger on the left hemisphere (cp. the grand average in Figure 4D). In addition, this spatial distribution seems to follow a slight contra-lateral tendency (Figure 5A): stimuli presented on the right audio channel (right column in the grid of scalp maps) induce a more discriminative N200 component on the left hemisphere. On the contrary, the class discriminative N200 components induced by stimuli on the left audio channel (left column in the grid of scalp maps) are located rather on the right hemisphere.
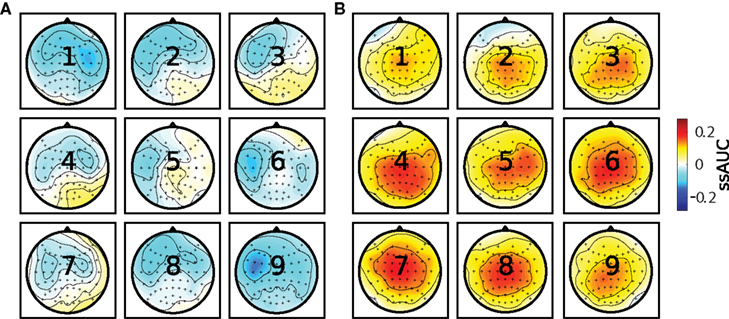
Figure 5. Grand average of the spatial distribution of the stimulus-specific N200 component (A) and P300 component (B). Area-under-the ROC-curve (ssAUC) values are signed such that positive values stand for a positive components and negative values represent negative components. Plots are arranged corresponding to the 3 × 3 design of the PASS2D paradigm.
3.4 Discriminative Information in the Spatial and Temporal Domain
The impact of the spatial and the temporal domain on the classifier was investigated separately (Figure 6) by analysis of isolated short time segments. The most discriminative information was found 400–500 ms after the stimulus onset, which reflects the importance of the P300 component. The most discriminative information was found at central–lateral locations such as C4/C5, when averaging intervals were selected heuristically. Comparing this to the grand average ERP scalp maps in Figure 4, one can find an overlap of N200 and P300 in the mentioned areas. This stresses the importance of the N200, although a stimulus-specific variation (see Figure 5 and results above) was found. It can be concluded that both components N200 and P300 can be used for classification, but the P300 component contains more discriminative information.
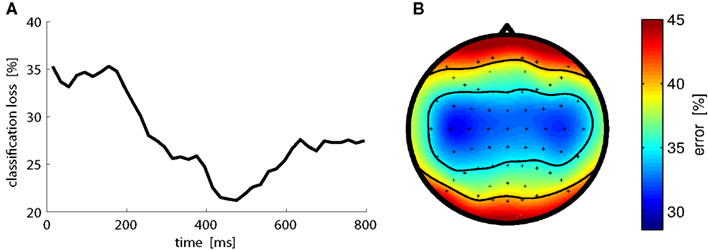
Figure 6. Grand average temporal (A) and spatial (B) distribution of discriminative information. Reported loss values in the temporal domain are obtained for a sliding window of 50 ms. The loss values obtained for each electrode separately are depicted as a scalp topography (B).
3.5 Online Bit Rate and Characters per Minute
It took 15–26 min (μ = 20.9) to spell the short sentence and 31–76 min (μ = 43.5) for the long sentence. Variation in the number of multiclass selections originates from the different number of false selections (see Table 1) which then had to corrected. Since the sentences were not spelled word by word but in one go, all kinds of pauses are taken into account. However, the time for individual relaxation and fixed intertrial periods are among the main influence factors for the spelling speed. Figure 7 shows that neglecting the time for individual relaxation, and thereby only considering the stimulation time (∼31 s) and a fixed inter trial time (4 s), results in an average benefit of more than 1 bit/min or 0.25 char/min.
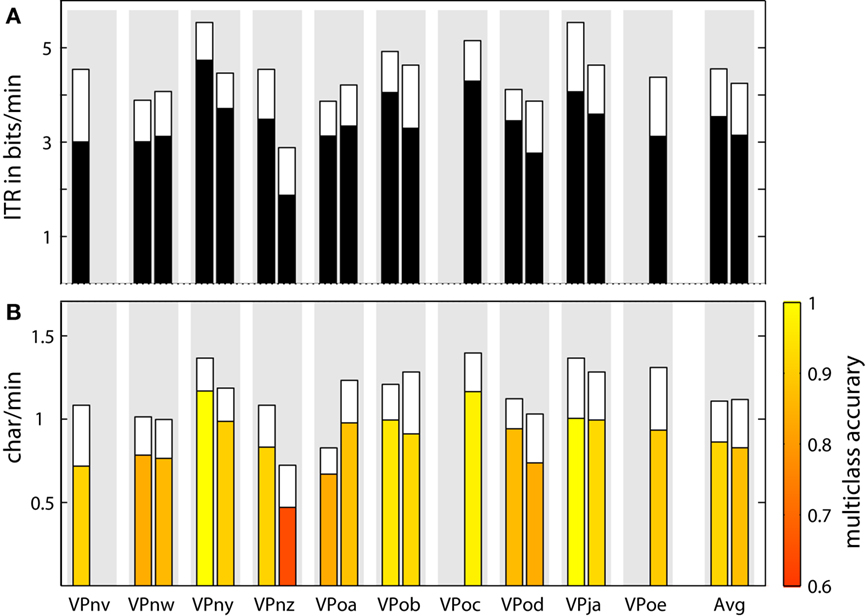
Figure 7. For each subject and both sentences the bar plots show the multiclass accuracy and the resulting Information Transfer Rate (A) and the spelling speed in characters per minute (B). The white extensions of the bars mark the potential increase, that could result if individual pauses are disregarded for the computation of the spelling speed and ITR. For each subject, the left (right) bar represents the performance of the short (long) sentence. For three subjects there is only one bar, because the spelling of the second sentence was canceled or not even started.
In general, a higher multiclass accuracy can be obtained by increasing the number of subtrials. The rate of communication (Wolpaw et al., 2002) counterbalances this effect, enabling to compare different studies more accurately.
On average, subjects achieved an information transfer rate (ITR) of 3.4 bits/min in the online condition (based on the nine-class decision, including all pauses), see Table 2. An average online spelling speed of 0.89 characters/minute was observed, see also Table 1 and Figure 7.
In general, the information of one character is coded by at least 4.75 bits (1 out of 27, 26 letters plus space). Considering that the BCI controlled speller application presented here enables an average spelling speed of 0.89 characters/min, the ITR could also be quantified with 4.23 bits/min (0.89 × 4.75) in a hypothetical BCI paradigm with 27 classes. The discrepancy between 3.4 and 4.23 bits/min can be explained with the predictive text system, which thus increases the ITR by at least 0.83 bits/min or 24%.
3.6 Online Multiclass Accuracy
Averaged over all trials and participants of the online experiments, 89.37% of the multiclass decisions were correct (chance level is 1/9, thus 11.11%). The multiclass accuracy was slightly higher for the short sentence (92.63%) than for the long sentence (87.95%), see Table 1. Table 3 reveals that none of the stimuli has a significantly increased or decreased accuracy. Nevertheless, one can observe that the low-pitch keys “7,” “8,” and “9” – although chosen less frequently than other keys – have the highest selection accuracy.
Furthermore it was found that keys “1,” “3,” and “4” are chosen more frequently than others. This is due to the design of the predictive text system.
In the presented paradigm, the nine auditory stimuli are not completely independent: for each target there are four non-targets being equal in one dimension, i.e., two stimuli with the same pitch (same row) and two stimuli with the same direction (same column). Since these similarities could influence the results, it was tested if this is reflected in the binary classifier outputs or multiclass decisions.
(1) False positives for non-targets with the same pitch as the target: The probability of false positives in single-epoch binary classification for these non-targets was in fact higher than for other non-targets, as the classifier outputs were significantly more negative and thus more similar to target outputs (p < 10−20). An increased probability for erroneous multiclass selections with the correct pitch but wrong direction was observed as well. Table 3 reveals that 47 out of 79 multiclass errors had an equal pitch. Assuming no dependency, one would expect 19.75 (2 out of 8). This is a significant deviation (χ2 Test with p < 10−11).
(2) False positives for non-targets with the same direction as the target: No significant effect was found for non-targets with a correct direction but with different pitch in comparison to other non-targets (p = 0.13), although the average classifier outputs were again more negative. Multiclass selection errors toward a decision with a correct direction but wrong pitch were not accumulated.
According to these results, the classifier could resolve the dimension “pitch” better than the dimension “direction” which also stands in line with the findings by Halder et al. (2010).
Four subjects (VPnv, VPnz, VPoc, VPoe) had a sudden drop of multiclass accuracy within the online phase. The exact reason for that effect remains unclear. Technical problems as well as physiological instabilities or lack of concentration may have caused this effect, but could be neither found, nor excluded. Experiments for VPnv, VPoc, and VPoe were stopped after the drop of accuracy.
4 Discussion
It is clear, that the stimulus characteristics have a strong impact on the BCI performance. Our decision for a 3 × 3 design was partially driven by the possibility to use a T9-like text encoding system, even though other designs could potentially be better in terms of signal-to-noise ratio. Prior work (Schreuder et al., 2010) showed, that both stimulus types (direction and pitch) contain valuable information for a discrimination task, and that a redundant combination can enhance the separability compared to the single stimulus types. For a multiclass approach (nine or more classes) with redundant coding, however, the pitch steps or angle distance might get too small for a reliable perception. Thus, the decision to use the two stimulus dimensions independently instead of using them to code the same information in a redundant way, represents a trade-off between large steps in pitch/angle and a relatively large number of 9 classes.
Our results show that the PASS2D paradigm offers fast spelling speed (average of 0.89 chars/min and 3.4 bits/min) and an intuitive interaction scheme while being driven by simple stimuli from headphones.
Using modern machine learning approaches for ERP classification (Müller et al., 2008), the individual discriminative ERP signatures of subjects could be exploited reasonably well and in real-time and most participants could spell two complete sentences during a single session.
The direct performance comparison of BCI spellers is tedious, as the reported ITRs (in bits/min or char/min) are based on various different assumptions or definitions. For example, the inclusion or exclusion of pause times between trials or runs has a strong impact on the calculation of ITR (see Section 3.5). Moreover, several formulae for the calculation of ITR were proposed by the BCI community (Wolpaw et al., 2002; Schlögl et al., 2007) and are actively used. The majority of reported ITR rates for different visual BCI paradigms with healthy subjects range between approximately 10–20 bits/min (with some positive outliers of up to 80 bits/min).
Although among the fastest currently available audio paradigms for BCI, the present work is not reaching the ITR level of these visual paradigms yet, but it is not far from this performance. As the line of research of auditory BCI is relatively young, the potential future development is promising. Moreover – as pointed out in Section 1 – it represents a qualitatively new solution for end users with visual impairments.
Embedded in the patient-oriented TOBI-project2, the presented paradigm follows principles of user-centered design. Firstly, this is expressed by the decision to use a T9-like text entry method. The spelling process in PASS2D is easy to understand and widely known because of its similarity to T9-spelling in mobile phones. Moreover, it implements a predictive text entry system, that improves the spelling speed and usability.
Secondly – although the spatial dimension as a class discriminative cue could be exploited more fine-grained (cp. to the approach of Schreuder et al. (2010) using up to eight spatial directions) – the PASS2D approach was restricted to three directions only. Taking this decision, the hardware complexity and space requirements for the setup of the system at a patient’s home can be reduced, as three directions can be implemented by off-the-shelf headphones and simple stereo sound cards.
Thirdly, the PASS2D paradigm has the potential to adapt to its user in terms of the underlying language model: the predictive text system can consider individual spelling profiles via updates of the text corpus. This implements an important aspect of flexibility, as patients tend to use a lot of individual abbreviations of frequently used terms in order to speed up their communication.
Fourthly, the presented speller design is flexible with respect to the sensory modality. Although operated as a spelling interface with auditory feedback, the interaction scheme is well suited also for visual ERP stimuli or control via eye tracking assistive technology with full visual feedback. In combination with suitable visual highlighting effects (Hill et al., 2009; Tangermann et al., 2011), the graphical representation of the speller (see Figure 3) can directly be used to elicit ERP effects by a visual oddball. Thus, patients in LIS with remaining gaze control could use both, a visual or a hybrid (del Millán et al., 2010) visual–auditory version of the speller. With a progressing neurodegenerative disease, a further decrease in gaze control or daily changing conditions, the patient has the opportunity to switch from the visual to the hybrid or to the purely auditory setting. As the elicited ERPs are expected to change during this transition, the underlying feature extraction, and classification should of course be adapted. If it is possible to perform this transition in a transparent manner, patients can simply continue to use the same interaction scheme independent of the stimulus modality in action.
It is concluded that this auditory ERP speller enables BCI users to kick-start communication within a single session and thereby offers a promising alternative for patients in LIS or CLIS. The next step will be to further simplify the spelling procedure such that it allows a purely auditory navigation.
Future work will also be conducted to further improve the paradigm with respect to spelling speed, pleasantness, intuitiveness, and applicability for patients in locked-in state and complete locked-in state. Experiments with patients are planned.
Conflict of Interest Statement
The authors declare that the research was conducted in the absence of any commercial or financial relationships that could be construed as a potential conflict of interest.
Acknowledgments
The authors would like to thank Klaus-Robert Müller and Sven Dähne for fruitful discussions and the subjects for their kind participation. The authors are grateful for the financial support by several institutions: This work was partly supported by the European Information and Communication Technologies (ICT) Program Project FP7-224631 and 216886, by grants of the Deutsche Forschungsgemeinschaft (DFG; MU 987/3-2) and Bundesministerium fur Bildung und Forschung (BMBF; FKZ 01IB001A, 01GQ0850) and by the FP7-ICT Program of the European Community, under the PASCAL2 Network of Excellence, ICT-216886. This publication only reflects the authors’ views. Funding agencies are not liable for any use that may be made of the information contained herein.
Footnotes
- ^For the long sentence, a dynamic method of unbalanced subtrial presentation was applied: the number of presentations per class was dynamically determined based on preceding classifier outputs of the ongoing trial. While being discussed in Höhne et al. (2010), this detail is disregarded in all the following analysis.
- ^http://www.tobi-project.org
References
Acqualagna, L., Treder, M. S., Schreuder, M., and Blankertz, B. (2010). A novel brain-computer interface based on the rapid serial visual presentation paradigm. Conf. Proc. IEEE Eng. Med. Biol. Soc. 1, 2686–2689.
Bianchi, L., Sami, S., Hillebrand, A., Fawcett, I. P., Quitadamo, L. R., and Seri, S. (2010). Which physiological components are more suitable for visual ERP based brain-computer interface? A preliminary MEG/EEG study. Brain Topogr. 23, 180–185.
Birbaumer, N., Ghanayim, N., Hinterberger, T., Iversen, I., Kotchoubey, B., Kübler, A., Perelmouter, J., Taub, E., and Flor, H. (1999). A spelling device for the paralysed. Nature 398, 297–298.
Blankertz, B., Krauledat, M., Dornhege, G., Williamson, J., Murray-Smith, R., and Müller, K.-R. (2007). “A note on brain actuated spelling with the Berlin brain-computer interface,” in Universal Access in HCI, Part II, HCII 2007, Volume 4555 of LNCS, ed. C. Stephanidis (Berlin Heidelberg: Springer), 759–768.
Blankertz, B., Lemm, S., Treder, M. S., Haufe, S., and Müller, K.-R. (2011). Single-trial analysis and classification of ERP components – a tutorial. Neuroimage 56, 814–825.
Blankertz, B., Tangermann, M., Vidaurre, C., Fazli, S., Sannelli, C., Haufe, S., Maeder, C., Ramsey, L. E., Sturm, I., Curio, G., and Müller, K.-R. (2010). The Berlin brain-computer interface: non-medical uses of BCI technology. Front. Neurosci. 4:198. doi: 10.3389/fnins.2010.00198
Brunner, P., Joshi, S., Briskin, S., Wolpaw, J. R., Bischof, H., and Schalk, G. (2010). Does the “P300” speller depend on eye gaze? J. Neural Eng. 7, 056013.
del Millán, J. R., Rupp, R., Mueller-Putz, G., Murray-Smith, R., Giugliemma, C., Tangermann, M., Vidaurre, C., Cincotti, F., Kübler, A., Leeb, R., Neuper, C., Müller, K.-R., and Mattia, D. (2010). Combining brain-computer interfaces and assistive technologies: state-of-the-art and challenges. Front. Neurosci. 4:161. doi: 10.3389/fnins.2010.00161
Dunlop, M., and Crossan, A. (2000). Predictive text entry methods for mobile phones. Pers. Ubiquitous Comput. 4, 134–143.
Farwell, L., and Donchin, E. (1988). Talking off the top of your head: toward a mental prosthesis utilizing event-related brain potentials. Electroencephalogr. Clin. Neurophysiol. 70, 510–523.
Furdea, A., Halder, S., Krusienski, D. J., Bross, D., Nijboer, F., Birbaumer, N., and Kübler, A. (2009). An auditory oddball (P300) spelling system for brain-computer interfaces. Psychophysiology 46, 617–625.
Guger, C., Daban, S., Sellers, E., Holzner, C., Krausz, G., Carabalona, R., Gramatica, F., and Edlinger, G. (2009). How many people are able to control a P300-based brain-computer interface (BCI)? Neurosci. Lett. 462, 94–98.
Guo, J., Gao, S., and Hong, B. (2010). An auditory brain-computer interface using active mental response. IEEE Trans. Neural Syst. Rehabil. Eng. 18, 230–235.
Halder, S., Rea, M., Andreoni, R., Nijboer, F., Hammer, E. M., Kleih, S. C., Birbaumer, N., and Kübler, A. (2010). An auditory oddball brain-computer interface for binary choices. Clin. Neurophysiol. 121, 516–523.
Hill, J., Farquhar, J., Martens, S., Bießmann, F., and Schölkopf, B. (2009). Effects of stimulus type and of error-correcting code design on BCI speller performance. Adv. Neural Inf. Process. Syst. 21, 665–672.
Hill, N., Lal, T., Bierig, K., Birbaumer, N., and Schölkopf, B. (2005). “An auditory paradigm for brain–computer interfaces,” in Advances in Neural Information Processing Systems, Vol. 17, eds L. K. Saul, Y. Weiss, and L. Bottou (Cambridge, MA: MIT Press), 569–576.
Höhne, J., Schreuder, M., Blankertz, B., and Tangermann, M. (2010). Two-dimensional auditory P300 speller with predictive text system. Conf. Proc. IEEE Eng. Med. Biol. Soc. 1, 4185–4188.
Jin, J., Allison, B. Z., Brunner, C., Wang, B., Wang, X., Zhang, J., Neuper, C., and Pfurtscheller, G. (2010). P300 chinese input system based on bayesian LDA. Biomed. Tech. (Berl.) 55, 5–18.
Jin, J., Allison, B. Z., Sellers, E. W., Brunner, C., Horki, P., Wang, X., and Neuper, C. (2011). Optimized stimulus presentation patterns for an event-related potential EEG-based brain-computer interface. Med. Biol. Eng. Comput. 49, 181–191.
Kanoh, S., Miyamoto, K., and Yoshinobu, T. (2008). “A brain-computer interface (BCI) system based on auditory stream segregation,” in Engineering in Medicine and Biology Society, 2008. EMBS 2008. 30th Annual International Conference of the IEEE, Vancouver, BC, 642–645.
Kim, D.-W., Hwang, H.-J., Lim, J.-H., Lee, Y.-H., Jung, K.-Y., and Im, C.-H. (2011). Classification of selective attention to auditory stimuli: toward vision-free brain-computer interfacing. J. Neurosci. Methods 197, 180–185.
Kleih, S. C., Nijboer, F., Halder, S., and Kubler, A. (2010). Motivation modulates the P300 amplitude during brain-computer interface use. Clin Neurophysiol 121, 1023–1031.
Klobassa, D. S., Vaughan, T. M., Brunner, P., Schwartz, N. E., Wolpaw, J. R., Neuper, C., and Sellers, E. W. (2009). Toward a high-throughput auditory P300-based brain-computer interface. Clin. Neurophysiol. 120, 1252–1261.
Krusienski, D., Sellers, E. W., McFarland, D. J., Vaughan, T. M., and Wolpaw, J. R. (2008). Toward enhanced P300 speller performance. J. Neurosci. Methods 167, 15–21.
Kübler, A., Furdea, A., Halder, S., Hammer, E. M., Nijboer, F., and Kotchoubey, B. (2009). A brain-computer interface controlled auditory event-related potential (p300) spelling system for locked-in patients. Ann. N. Y. Acad. Sci. 1157, 90–100.
Langers, D. R. M., van Dijk, P., and Backes, W. H. (2005). Lateralization, connectivity and plasticity in the human central auditory system. Neuroimage 28, 490–499.
Liu, Y., Zhou, Z., and Hu, D. (2011). Gaze independent brain-computer speller with covert visual search tasks. Clin. Neurophysiol. 122, 1127–1136.
Müller, K.-R., Tangermann, M., Dornhege, G., Krauledat, M., Curio, G., and Blankertz, B. (2008). Machine learning for real-time single-trial EEG-analysis: from brain-computer interfacing to mental state monitoring. J. Neurosci. Methods 167, 82–90.
Murguialday, A. R., Hill, J., Bensch, M., Martens, S., Halder, S., Nijboer, F., Schölkopf, B., Birbaumer, N., and Gharabaghi, A. (2011). Transition from the locked in to the completely locked-in state: a physiological analysis. Clin. Neurophysiol. 122, 925–933.
Nijboer, F., Furdea, A., Gunst, I., Mellinger, J., McFarland, D., Birbaumer, N., and Kübler, A. (2008a). An auditory brain-computer interface (BCI). J. Neurosci. Methods 167, 43–50.
Nijboer, F., Sellers, E. W., Mellinger, J., Jordan, M. A., Matuz, T., Furdea, A., Halder, S., Mochty, U., Krusienski, D. J., Vaughan, T. M., Wolpaw, J. R., Birbaumer, N., and Kübler, A. (2008b). A P300-based brain-computer interface for people with amyotrophic lateral sclerosis. Clin. Neurophysiol. 119, 1909–1916.
Schaefer, R. S., Vlek, R. J., and Desain, P. (2011). Decomposing rhythm processing: electroencephalography of perceived and self-imposed rhythmic patterns. Psychol. Res. 75, 95–106.
Schlögl, A., Kronegg, J., Huggins, J., and Mason, S. G. (2007). “Evaluation criteria for BCI research,” in Towards Brain-Computer Interfacing, eds G. Dornhege, J. R. del Millán, T. Hinterberger, D. McFarland, and K.-R. Müller (Cambridge, MA: MIT press), 297–312.
Schreuder, M., Blankertz, B., and Tangermann, M. (2010). A new auditory multi-class brain-computer interface paradigm: spatial hearing as an informative cue. PLoS ONE 5, e9813. doi: 10.1371/journal.pone.0009813
Schreuder, M., Tangermann, M., and Blankertz, B. (2009). Initial results of a high-speed spatial auditory BCI. Int. J. Bioelectromagn. 11, 105–109.
Sellers, E. W., and Donchin, E. (2006). A P300-based brain-computer interface: initial tests by ALS patients. Clin. Neurophysiol. 117, 538–548.
Tangermann, M., Krauledat, M., Grzeska, K., Sagebaum, M., Blankertz, B., Vidaurre, C., and Müller, K.-R. (2009). “Playing pinball with non-invasive BCI,” in Advances in Neural Information Processing Systems 21, Eds D. Koller, D. Schuurmans, Y. Bengio, and L. Bottou (Cambridge, MA: MIT Press), 1641–1648.
Tangermann, M., Schreuder, M., Dähne, S., Höhne, J., Regler, S., Ramsey, A., Queck, M., Williamson, J., and Murray-Smith, R. (2011). Optimized stimulation events for a visual ERP BCI. Int. J. Bioelectromagn. 13. [Epub ahead of print].
Treder, M. S., and Blankertz, B. (2010). (C)overt attention and visual speller design in an ERP-based brain-computer interface. Behav. Brain Funct. 6, 28.
Venthur, B., Scholler, S., Williamson, J., Dähne, S., Treder, M. S., Kramarek, M. T., Müller, K.-R., and Blankertz, B. (2010). Pyff – a pythonic framework for feedback applications and stimulus presentation in neuroscience. Front. Neurosci. 4:100. doi: 10.3389/fninf.2010.00100
Williamson, J., Murray-Smith, R., Blankertz, B., Krauledat, M., and Müller, K.-R. (2009). Designing for uncertain, asymmetric control: Interaction design for brain-computer interfaces. Int. J. Hum. Comput. Stud. 67, 827–841.
Keywords: brain–computer interface, BCI, auditory ERP, P300, N200, spatial auditory stimuli, T9, user-centered design
Citation: Höhne J, Schreuder M, Blankertz B and Tangermann M (2011) A novel 9-class auditory ERP paradigm driving a predictive text entry system. Front. Neurosci. 5:99. doi: 10.3389/fnins.2011.00099
Received: 04 March 2011; Accepted: 28 July 2011;
Published online: 22 August 2011.
Edited by:
Cuntai Guan, Institute for Infocomm Research, SingaporeReviewed by:
Bo Hong, Tsinghua University, ChinaJeremy Hill, Wadsworth Center, New York State Department of Health, USA
Copyright: © 2011 Höhne, Schreuder, Blankertz and Tangermann. This is an open-access article subject to a non-exclusive license between the authors and Frontiers Media SA, which permits use, distribution and reproduction in other forums, provided the original authors and source are credited and other Frontiers conditions are complied with.
*Correspondence: Johannes Höhne, Machine Learning Laboratory, Berlin Institute of Technology, Franklinstr. 28/19, 10587 Berlin, Germany. e-mail:ai5ob2VobmVAdHUtYmVybGluLmRl