- 1 Indications Discovery Research Unit, Pfizer Inc, St. Louis, MO, USA
- 2 Behavioral Research and Development, PsychoGenics Inc, Tarrytown, NY, USA
The lack of predictive in vitro models for behavioral phenotypes impedes rapid advancement in neuropharmacology and psychopharmacology. In vivo behavioral assays are more predictive of activity in human disorders, but such assays are often highly resource-intensive. Here we describe the successful application of a computer vision-enabled system to identify potential neuropharmacological activity of two new mechanisms. The analytical system was trained using multiple drugs that are used clinically to treat depression, schizophrenia, anxiety, and other psychiatric or behavioral disorders. During blinded testing the PDE10 inhibitor TP-10 produced a signature of activity suggesting potential antipsychotic activity. This finding is consistent with TP-10’s activity in multiple rodent models that is similar to that of clinically used antipsychotic drugs. The CK1ε inhibitor PF-670462 produced a signature consistent with anxiolytic activity and, at the highest dose tested, behavioral effects similar to that of opiate analgesics. Neither TP-10 nor PF-670462 was included in the training set. Thus, computer vision-based behavioral analysis can facilitate drug discovery by identifying neuropharmacological effects of compounds acting through new mechanisms.
Introduction
Pharmaceutical companies and many academic centers have thousands of high-quality compounds that represent potential new drugs. These high-quality compound collections contain thoroughly studied development candidates and, in many cases, “old” drugs that were generated for non-CNS disorders but that might be useful therapies for one or more psychiatric diseases. However, testing these compounds for activity relevant to treating psychiatric diseases is challenging. Whereas some disease areas, such as cancer or infectious disease, are relatively amenable to high-throughput in vitro testing of hundreds of thousands of compounds, psychiatric diseases are not. Because psychiatric diseases generally result from disorders of cell–cell communication or circuitry, intact systems are required to detect improvement in disease-relevant endpoints. These endpoints are typically behavioral in nature, often requiring human observation and interpretation.
Resource-intensive behavioral experiments are expensive, slow, and frequently subjective. The need for observers to be blinded to the treatment group of each animal increases the resources required to appropriately dose and randomize animals in a way that is invisible to the observer. Furthermore, multiple complementary behavioral assays are required to determine whether observed effects are robust and are not due to undesired effects, such as sedation (Crawley and Paylor, 1997).
Thus, the discovery of new neuropharmacological drugs with novel mechanisms of action is impeded by the absence of high-throughput, robust, and objective behavioral assays. Very recently a 96-well behavioral assay measuring the photomotor response of zebrafish embryos demonstrated that different classes of neuropharmacological compounds cause distinct patterns of responses (Kokel et al., 2010). Such an assay is relatively inexpensive and is well suited to screening high numbers of compounds about which little is known.
For high-quality compounds, particularly compounds that have entered human testing, a rodent system offers advantages. Rodent behaviors in response to existing neuropharmacological agents are very well characterized. For advanced compounds, rodent pharmacokinetics and safety are often known, and sufficient quantities of these compounds generally exist to support rodent studies.
To enable testing of many compounds for behavioral effects relevant to psychiatric disease, PsychoGenics developed SmartCube™, an automated system in which behaviors of compound-treated mice are captured by digital video and analyzed with computer algorithms (Brunner et al., 2002). PGI Analytical Systems uses data from SmartCube™ to compare the behavioral signature of a test compound to a database of behavioral signatures obtained using a large set of diverse reference compounds. In this way, the neuropharmacological effects of a test compound can be predicted by similarity to major classes of compounds, such as antipsychotics, anxiolytics, and antidepressants. This approach is ideally suited to screening collections of existing drugs or drug candidates for previously unknown neuropharmacology, which could expedite the development of new and unexpected treatments for psychiatric disorders. In this paper, we demonstrate that this system can detect relevant CNS activity of compounds representing mechanisms beyond those used clinically to treat psychiatric disorders.
Materials and Methods
An overview of PGI Analytical Systems, a rapid, computer vision-enabled murine screening system for neuropharmacological activity, is shown in Figure 1. The PGI Analytical Systems training set consisted of hundreds of doses of clinically approved reference drugs, grouped per indication (e.g., anxiolytics, antidepressants, etc.). Drugs were injected 15 min before the test, and multiple challenges were presented over the course of the test session. At least 12 mice were used in each treatment group. Digital videos of the subjects were processed with computer vision algorithms to extract over 2,000 dependent measures including frequency and duration of behavioral states such as grooming, rearing, etc., and many other features obtained during the test session.
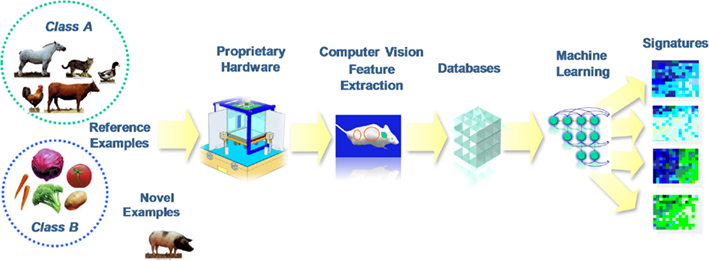
Figure 1. PGI Analytical Systems combine proprietary hardware, robotics, computer vision, and data mining techniques to collect thousands of behavioral features in a single test session and extract unique signatures for each compound. These signatures are compared with the signatures obtained from reference compounds (shown to have clinical efficacy) in different therapeutic classes and subclasses to predict clinical utility.
A proprietary machine learning algorithm was developed to train a probabilistic classifier that mapped the extracted computer vision feature values from the training set to their corresponding CNS indications. This tool was used to establish a reference database of therapeutic class signatures and provided a mechanism to determine the CNS probabilistic profile of an arbitrary test sample.
Our reference database comprises 14 classes of drugs with some of the major classes, such as the antidepressant class, comprising several subclasses with representatives of most of the drugs in the market. The best performing classifiers during “test set” assessment were chosen from our evaluation tests and two separate types of classifiers were built that make independent predictions: one at the therapeutic class level and one at the level of highly performing subclasses. The behavioral signatures of the test drugs were scored by these classifiers to predict potential therapeutic utility.
To evaluate the ability of the PGI Analytical System to detect relevant behavioral responses to novel compounds, we tested two compounds that represent mechanisms not yet used clinically to treat psychiatric disorders but that impact relevant behavioral endpoints in rodents. The two test compounds, TP-10 and PF-670462, had not been included in the computer algorithm training set. TP-10 is a sub-nanomolar inhibitor of PDE10A, a dual-substrate phosphodiesterase expressed in medium spiny neurons of the striatum that regulates striatal output by regulating both cAMP and cGMP hydrolysis (Strick et al., 2007). TP-10 demonstrates multiple behavioral effects in rodents that are consistent with clinically effective antipsychotics, including decreased locomotor activity, inhibition of conditioned avoidance response, and improvement of amphetamine-induced deficits in auditory gating (Schmidt et al., 2008). PF-670462 is a casein kinase Iε (CK1ε) inhibitor (Badura et al., 2007).
All compounds were dissolved in a pharmasolve, PEG, PG mixture, and were injected i.p. 15 min before the behavioral test. In a follow-up experiment PF-670462 was administered s.c. 13 h before the behavioral test because of its known effects on circadian rhythm at 50 mg/kg s.c. (Badura et al., 2007); this procedure was done both in the morning and evening.
As a follow-up test to confirm the anxiolytic signature of PF-670462 we use the marble burying test. PF-670462 was dissolved in 40% cyclodextrin and injected at 10 and 30 mg/kg, s.c. 15 min before the 30-min test. We measured number of marbles buried and distance traveled.
Experimenters were blind to the mechanisms of action of both compounds and to the dose being used. The Institutional Animal Care and Use Committee of PsychoGenics reviewed and approved the animal use in these studies. The animal care and use program is fully accredited by the Association for Assessment and Accreditation of Laboratory Animal Care, International.
Results
In the PGI Analytical System, TP-10 produced a dose-dependent signature of activity most similar to that of known antipsychotics, particularly at the 1-mg/kg dose (Figure 2). TP-10 exhibited a steep dose–response curve in this system, consistent with its steep dose–response curve in other rodent models (Schmidt et al., 2008). At the lower 0.5 mg/kg dose, TP-10’s activity is more similar to that of the vehicle than to any class of drugs in the reference database. At the higher dose of 2 mg/kg, TP-10 produced a behavioral pattern that is quite different from that of the vehicle yet not specific to any one class. Notably, TP-10 activity at the 2-mg/kg dose has a large behavioral component similar to that of high-dose antidepressants but not medium-dose antidepressants. Interestingly, three of the four reference antipsychotic signatures shown in Figure 3 also have a larger high-dose antidepressant-like component at their highest doses. Overall, the profile of TP-10 in this computer-aided screening system is similar to that of clinically used antipsychotic drugs (Gleason and Shannon, 1997; Powell et al., 2008; Ishii et al., 2010). This observation is consistent with TP-10’s activity in multiple rodent models (Schmidt et al., 2008), suggesting that the PGI Analytical Systems can identify antipsychotic-like activity of compounds acting through novel mechanisms.
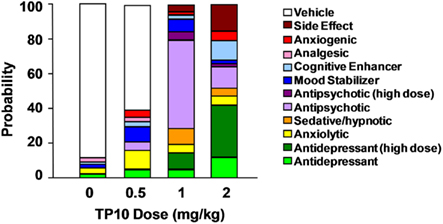
Figure 2. The PDE10A inhibitor TP-10 produced a signature of activity suggesting potential antipsychotic activity. The size of a given colored region on each bar indicates the probability that the activity of TP-10 at that dose matches a given class of neuropharmacological agents. The size of the white area represents that probability that the activity is more similar to that of the vehicle rather than to that of any compounds in the reference database. The drug was injected i.p., 15 min before testing.
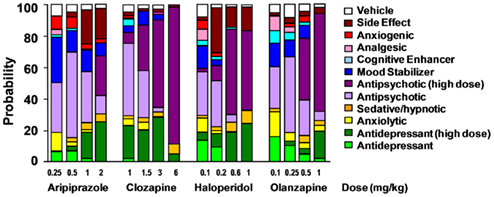
Figure 3. Drug signatures of four of the antipsychotic drugs included in the reference dataset: aripiprazole, clozapine, haloperidol, and olanzapine. Numbers below each bar represent doses in mg/kg administered i.p. 15 min before testing.
In contrast to PDE10A, for which considerable literature data support its role as an antipsychotic target, the potential usefulness of altering CK1ε’s effects on circadian rhythm for treating psychiatric disorders is unknown. CK1ε is an important regulator of period proteins (PER) that regulate the clock mechanism in cells (Lee et al., 2001). Clearly, improved regulation of circadian rhythm could improve functioning in patients with sleep disorders. Furthermore, disruptions of the sleep–wake cycle can affect cognition, memory, and mood (Benca et al., 2009). CK1ε is also involved in DARP32 phosphorylation and thus participates in dopamine-dependent behavioral activation (Bryant et al., 2009). Thus, the CK1ε mechanism is a good candidate for screening in a system that may identify behavioral signatures relevant to a wide variety of psychiatric disorders.
In the PGI Analytical System at intermediate doses of 30 and 50 mg/kg, the CK1ε inhibitor PF-670462 (Badura et al., 2007) demonstrated a signature consistent with anxiolytic, but not sedative/hypnotic, activity (Figure 4). Because PF-670462 produces changes in circadian rhythm (Badura et al., 2007), we tested whether administering PF-670462 to mice 13 h prior to testing might produce a more relevant behavioral signature. Interestingly, the compound had almost no detectable behavioral effects in this system when dosed 13 h before testing, either in the morning (5:30 am) or the afternoon (7 pm). The anxiolytic signature of PF-670462 seen at the 30, but not 10, mg/kg dose was confirmed in the marble burying test, in which the higher dose decreased marbles buried without a decrease in locomotion (Figure 5).
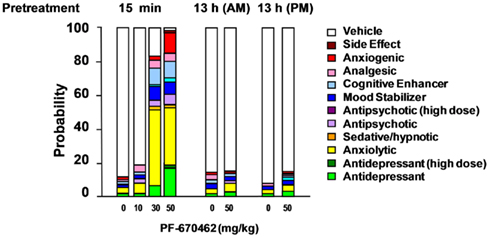
Figure 4. The CK1ε inhibitor PF-670462 demonstrated a signature consistent with anxiolytic activity (yellow) and, at the highest dose tested, behavioral effects similar to that of opiate analgesics (pink), although this dose is higher that doses tested previously in rodents and may reflect non-specific effects. The drug was injected i.p. 15 min before the test in studies represented by the five left-most bars of the chart. To assess potentially delayed behavioral effects due to its effects on circadian rhythm, PF-670462 was injected s.c. 13 h before testing in the morning (AM) or in the afternoon (PM). This procedure revealed no delayed effects.
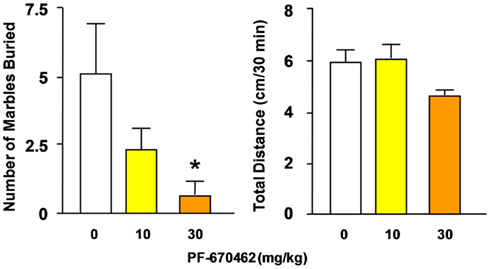
Figure 5. The CK1ε inhibitor PF-670462 shows anxiolytic activity in the marble burying test with a strong yet non-significant trend at 10 mg/kg and a significant effect at 30 mg/kg, consistent with the dose–response anxiolytic signature uncovered by the high-throughput screen [ANOVA F(4,43) > 8.8, p < 0.0001; Fisher PLSD p < 0.02]. The drug was injected s.c. 15 min before the 30-min test. There were no significant effects on locomotion.
Discussion
Our work demonstrates the usefulness of a computer vision-based system for identifying behavioral effects of novel compounds and mechanisms in a relatively high-throughput in vivo assay. As one example, the PDE10A inhibitor TP-10 demonstrated a behavioral signature in the PGI Analytical System that was more similar to that of known antipsychotics than to that of other classes of neuropharmacological agents. Although the efficacy of PDE10A inhibition in humans has not been tested, the activity of TP-10 here is consistent with effects of clinically used antipsychotics in multiple rat in vivo assays (Schmidt et al., 2008). Importantly, the steep dose–response curve of TP-10 was also consistent between the computer vision-based system and traditional rodent assays. Interestingly, TP-10 was ineffective in two mouse pre-pulse inhibition models (Schmidt et al., 2008), although it showed an antipsychotic-like signature in mice in the present study. The computer vision-based system, which measures hundreds of small behavioral events, may reveal more relationships because of its ability to assess compound effects more broadly than a model designed to test one specific functional hypothesis.
The TP-10 behavioral signature at the highest dose tested suggested a small degree of activity similar to that of high-dose antidepressants. This feature was observed with the higher doses of most of the four reference antipsychotics in Figure 3, as well, suggesting that the similarity is due to side effects caused by high doses of all such compounds.
The computer vision-based system also suggested new hypotheses regarding the behavioral effects of CK1ε inhibition. Other than modulation of circadian rhythm (Badura et al., 2007), behavioral effects of PF-670462 are unknown. We found that moderate doses of PF-670462 produced activity similar to that of anxiolytic agents, but not sedative/hypnotics. We followed up this result in the marble burying test, an assay predictive of anxiolytic therapeutic potential, and confirmed that a dose of 30 mg/kg has anxiolytic activity (Figure 5).
In summary, our data demonstrate that the PGI Analytics computer vision-based system rapidly detects relevant behavioral effects of compounds acting through new mechanisms. Because the assay and data analysis are highly automated, use of the system eliminates the need for subjective human observation and evaluation of behavior, thus eliminating significant resource requirements along with much of the potential for bias inherent in subjective measurements. This approach is ideally suited to screening libraries of existing drugs or drug candidates for previously unknown neuropharmacology, which could expedite the development of new and unexpected treatments for psychiatric disorders.
Conflict of Interest Statement
Dr. Roberds was an employee of Pfizer and Drs. Filippov, Alexandrov, Hanania and Brunner are employees of Psycho-Genics.
Acknowledgments
We thank Dr. Emer Leahy for her help with this manuscript, Jessica Malberg, Mary Cummins, and Emily Sabath for their work on the high-throughput in vivo system, and Sam Malekiani for the validation assay. We also thank Frank Menniti and Travis Wager for providing compounds and advice on their formulation.
References
Badura, L., Swanson, T., Adamowicz, W., Adams, J., Cianfrogna, J., Fisher, K., Holland, J., Kleiman, R., Nelson, F., Reynolds, L., St Germain, K., Schaeffer, E., Tate, B., and Sprouse, J. (2007). An inhibitor of casein kinase iïμ induces phase delays in circadian rhythms under free-running and entrained conditions. J. Pharmacol. Exp. Ther. 322, 730–738.
Benca, R., Duncan, M. J., Frank, E., McClung, C., Nelson, R. J., and Vicentic, A. (2009). Biological rhythms, higher brain function, and behavior: gaps, opportunities, and challenges. Brain Res. Rev. 62, 57–70.
Brunner, D., Nestler, E., and Leahy, E. (2002). In need of high-throughput behavioral systems. Drug Discov. Today 7, S107–S112.
Bryant, C. D., Graham, M. E., Distler, M. G., Munoz, M. B., Li, D., Vezina, P., Sokoloff, G., and Palmer, A. A. (2009). A role for casein kinase 1 epsilon in the locomotor stimulant response to methamphetamine. Psychopharmacology (Berl.) 203, 703–711.
Crawley, J. N., and Paylor, R. (1997). A proposed test battery and constellations of specific behavioral paradigms to investigate the behavioral phenotypes of transgenic and knockout mice. Horm. Behav. 31, 197–211.
Gleason, S. D., and Shannon, H. E. (1997). Blockade of phencyclidine-induced hyperlocomotion by olanzapine, clozapine and serotonin receptor subtype selective antagonists in mice. Psychopharmacology (Berl.) 129, 79–84.
Ishii, D., Matsuzawa, D., Kanahara, N., Matsuda, S., Sutoh, C., Ohtsuka, H., Nakazawa, K., Kohno, M., Hashimoto, K., Iyo, M., and Shimizu, E. (2010). Effects of aripiprazole on MK-801-induced prepulse inhibition deficits and mitogen-activated protein kinase signal transduction pathway. Neurosci. Lett. 471, 53–57.
Kokel, D., Bryan, J., Laggner, C., White, R., Cheung, C. Y. J., Mateus, R., Healey, D., Kim, S., Werdich, A. A., Haggarty, S. J., Macrae, C. A., Shoichet, B., and Peterson, R. T. (2010). Rapid behavior-based identification of neuroactive small molecules in the zebrafish. Nat. Chem. Biol. 6, 231–237.
Lee, C., Etchegaray, J.-P., Cagampang, F. R. A., Loudon, A. S. I., and Reppert, S. M. (2001). Posttranslational mechanisms regulate the mammalian circadian clock. Cell 107, 855–867.
Powell, S. B., Young, J. W., Ong, J. C., Caron, M. G., and Geyer, M. A. (2008). Atypical antipsychotics clozapine and quetiapine attenuate prepulse inhibition deficits in dopamine transporter knockout mice. Behav. Pharmacol. 19, 562–565.
Schmidt, C. J., Chapin, D. S., Cianfrogna, J., Corman, M. L., Hajos, M., Harms, J. F., Hoffman, W. E., Lebel, L. A., McCarthy, S. A., Nelson, F. R., Proulx-LaFrance, C., Majchrzak, M. J., Ramirez, A. D., Schmidt, K., Seymour, P. A., Siuciak, J. A., Tingley, F. D. III., Williams, R. D., Verhoest, P. R., and Menniti, F. S. (2008). Preclinical characterization of selective phosphodiesterase 10a inhibitors: a new therapeutic approach to the treatment of schizophrenia. J. Pharmacol. Exp. Ther. 325, 681–690.
Keywords: mouse, behavioral models, antipsychotic, anxiolytic, high-throughput screening, machine learning, computer vision, drug development
Citation: Roberds SL, Filippov I, Alexandrov V, Hanania T and Brunner D (2011) Rapid, computer vision-enabled murine screening system identifies neuropharmacological potential of two new mechanisms. Front. Neurosci. 5:103. doi: 10.3389/fnins.2011.00103
Received: 30 March 2011; Paper pending published: 20 April 2011;
Accepted: 18 August 2011; Published online: 09 September 2011.
Edited by:
Nick Andrews, Pfizer, UKCopyright: © 2011 Roberds, Filippov, Alexandrov, Hanania and Brunner. This is an open-access article subject to a non-exclusive license between the authors and Frontiers Media SA, which permits use, distribution and reproduction in other forums, provided the original authors and source are credited and other Frontiers conditions are complied with.
*Correspondence: Steven L. Roberds, Tuberous Sclerosis Alliance, 801 Roeder Road, Suite 750, Silver Spring, MD 20910, USA. e-mail:c3JvYmVyZHNAdHNhbGxpYW5jZS5vcmc=