- Department of Psychological and Brain Sciences, Indiana University, Bloomington, IN, USA
A key feature of human intelligence is the ability to predict the outcomes of one’s own actions prior to executing them. Action values are thought to be represented in part in the dorsal and ventral medial prefrontal cortex (mPFC), yet current studies have focused on the value of executed actions rather than the anticipated value of a planned action. Thus, little is known about the neural basis of how individuals think (or fail to think) about their actions and the potential consequences before they act. We scanned individuals with fMRI while they thought about performing actions that they knew would likely be rewarded or unrewarded. Here we show that merely imagining an unrewarded action, as opposed to imagining a rewarded action, increases activity in the dorsal anterior cingulate cortex, independently of subsequent actions. This activity overlaps with regions that respond to actual unrewarded actions. The findings show a distinct network that signals the prospective outcomes of one’s possible actions. A number of clinical disorders such as schizophrenia and drug abuse involve a failure to take the potential consequences of an action into account prior to acting. Our results thus suggest how dysfunctions of the mPFC may contribute to such failures.
Introduction
A key feature of human intelligence is the ability to predict the outcomes of one’s own actions prior to executing them. Much of the literature on decision-making and reinforcement learning focuses on learning the value of various available options. The optimal decision is one that has the highest value in the decision-maker’s subjective evaluation (Thorndike, 1911), with perhaps some value on exploring new options (Kaelbling et al., 1996). Environmental cues indicate what options are available, and the cues in turn guide instrumental responding via learned stimulus–response (S–R) associations (Sutton and Barto, 1998). This is the essence of model-free reinforcement learning (Dayan and Niv, 2008). Such constitutes an inverse model (Shadmehr and Wise, 2004), in that stimulus cues (S) activate a representation of the desired goal such as a piece of food, and this goal is mapped backward to the response (R) necessary to achieve the goal. The values of stimuli and the goals they represent are likely represented in the orbitofrontal cortex (OFC; Tremblay and Schultz, 1999; Schoenbaum et al., 2003). All of this works fine for habit learning.
The situation is more difficult when an animal faces a novel environment in which the S–R association has not been learned, or there is a more complex set of constraints, so that there is no one automatic best response. This is where forward models as in model-based reinforcement learning (Shadmehr and Wise, 2004; Daw et al., 2005; Glascher et al., 2010) are useful. A forward model predicts the outcome of a planned action. This is a learned response–outcome (R–O) association (Colwill and Rescorla, 1990) which affords a “dynamic evaluation lookahead” (Van Der Meer and Redish, 2010). Favorable outcome predictions might further activate the corresponding response plan, while unfavorable or risky outcome predictions might suppress it.
The process of employing a forward model to predict the likely outcomes of planned actions is akin to the popular notion of thinking before acting. Humans can think about or imagine (with varying accuracies) what might be the outcome of a planned action. Nonetheless, relatively little research has been done on the neural basis of thinking ahead, with just a few cognitive (Johnson, 2000; Hassabis et al., 2007), neuroimaging (Newman et al., 2009; Glascher et al., 2010), and rat (Van Der Meer and Redish, 2010) studies. Some results suggest that anterior cingulate cortex (ACC) may be involved in anticipating adjustments in control (Sohn et al., 2007; Aarts et al., 2008; Aarts and Roelofs, 2011). We previously showed that the medial prefrontal cortex (mPFC), and especially ACC may learn to predict the likelihood of an impending error resulting from current actions (Brown and Braver, 2005). Here we use fMRI to ask whether and how the ACC may signal the non-rewarded action likelihood of imagined responses, as distinct from the alternative hypothesis that ACC is activated only by impending actions. We use a simple task that isolates the outcome prediction by asking subjects to imagine performing an action and experiencing its consequences, while controlling for the subsequent action execution.
Materials and Methods
Participants
Data from 22 right-handed participants were collected (mean age = 23.42, SD = 2.80). Data from two participants were discarded due to insufficient reward outcomes and data from one participant was excluded due to a scanning artifact, leaving 19 usable participants (11 female). Participants reported no history of psychiatric or neurological disorder, and reported no current use of psychoactive medications. Participants were compensated $25/h for their time. Participants were trained on the task on a computer outside of the scanner until they gave verbal confirmation that they understood the task. The experimenter observed the participant’s performance and judged whether they demonstrated sufficient understanding of the task.
Participants were informed that they would receive compensation based on their performance, although they were unaware of how much they would receive for rewarding feedback. They received $0.05 for each reward outcome (described in further detail below in section Experimental Paradigm).
Participants gave informed consent prior to participating in the experiment. The experiment was approved by the Indiana University Institutional Review Board.
Experimental Paradigm
The task consisted of two phases: an imagine phase and a response phase. During the imagine phase, participants were instructed to imagine making particular responses and experiencing the corresponding consequences. During the response phase, participants were instructed to choose one of two possible responses. The appropriate response was determined by feedback history. When a particular response was rewarded, participants were instructed to make that response again. If a response was not rewarded, participants were instructed to make the alternate response. Hence, prior to each trial, participants had a belief about the response that would most likely result in a reward outcome, and could therefore imagine the consequences of a response that matched that belief (i.e., imagine rewarded action) or violated it (i.e., imagine non-rewarded action).
On each trial, the imagine phase began with the sequential presentation of two white arrow cues on a black background, with one pointing left and the other pointing right (Figure 1). The order of presentation of the arrow cues was counterbalanced. Participants were instructed to simply imagine themselves pressing the corresponding left or right buttons with the left or right index finger, along with the corresponding outcome they would expect if they were to actually press the button. Both left and right responses were imagined separately on each trial, so the probabilities of imagining each event were equal. After a variable delay, the response phase was signaled by an exclamation mark (“!”) which cued them to respond with either a left or right actual button press. Crucially, the responses that they imagined were independent of the actual response that they made. Participants would then be presented with a “$” or a “0” as feedback. A “$” would mean that they had gained a point, while a “0” would mean that they had gained nothing. Participants were informed that if they were rewarded on a trial (i.e., if they received a “$” as feedback) that they should make the same button response on the next trial, but a “0” indicated that they should switch. The appropriate button response (left vs. right) switched across trials with a relatively low probability resulting in a high likelihood of reward outcome. With this design, subjects could predict the outcome of each possible button press with good confidence. This allowed us to examine neural activity related to imagining distinct non-rewarded actions and rewarded actions without confounding the results with a particular effector. The probability of an underlying switch was 0 for the first two trials following a switch, then 0.33 per trial for trials three through seven, and 1.0 after eight trials. This distribution ensured that switches occurred but were unpredictable and less likely than chance. After receiving feedback, participants were presented with a blank screen that lasted either 1, 3, 5, or 7 s, based on an exponential distribution function (Dale, 1999).
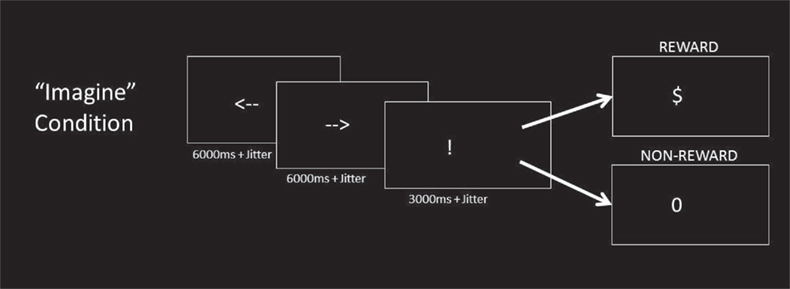
Figure 1. Imagine condition. In the Imagine condition, participants saw a sequence of two arrows, one facing left and the other facing right (order randomized across trials). As each arrow appeared, participants were instructed to imagine performing the corresponding button press response (left or right) and the outcome associated with it. An exclamation mark (“!”) cued the subjects to make a response of their choice. One of the two options was rewarded action, and the other would be unrewarded action. The rewarded action response in the preceding trial was more likely to be rewarded action in the current trial. Participants received either rewarded (“$”) or non-rewarded (“0”) feedback as a result of their choice. The response cue and outcome cues were identical to the Imagine condition.
On 20% of the trials, a question mark (“?”) was presented instead of arrow cues. During this condition, participants were instructed to recall the last response they had made and the corresponding outcome they had received, whether it was an outcome signaling a reward or not gaining a reward. When the exclamation mark cue was presented, participants were to make the same response they had made on the previous trial, whether it was rewarded or not. These trials were included for purposes not relevant here and were modeled separately.
fMRI Acquisition and Data Preprocessing
The experiment was conducted with a 3 T Siemens TIM Trio scanner using a 32-channel head coil. Foam padding was inserted around the sides of the head to increase participant comfort and reduce head motion. Imaging data was acquired at a 30° angle from the anterior commissure–posterior commissure line in order to maximize signal-to-noise ratio in the orbital and ventral regions of the brain (Deichmann et al., 2003). Functional T2* weighted images were acquired using a gradient echo planar imaging sequence (30 mm × 3.8 mm interleaved slices; TE = 25 ms; TR = 2000 ms; 64 × 64 voxel matrix; 220 mm × 220 mm field of view). Three runs of data were collected with 240 functional scans each. High resolution T1-weighted images for anatomical data (256 × 256 voxel matrix) were collected at the end of each session.
SPM5 (Wellcome Department of Imaging Neuroscience, London, UK; www.fil.ion.ucl.ac.uk/spm) was used for preprocessing and data analysis. The functional data for each run for each participant was slice-time corrected and realigned to each run’s mean functional image using a 6 degree-of-freedom rigid body spatial transformation. The resulting images were then coregistered to the participant’s structural image. The structural image was normalized to standard Montreal Neurological Institute (MNI) space and the warps were applied to the functional images. The functional images were then spatially smoothed using an 8-mm Gaussian kernel.
fMRI Analysis
Functional neuroimaging data were analyzed using a general linear model (GLM) with random effects. Feedback for rewarded actions and non-rewarded actions responses were modeled with a canonical hemodynamic response function (HRF) at the time of feedback. Two regressors modeled each imagine event. A delta regressor locked to the onset of stimulus presentation was included to capture initial perceptual activation. An epoch regressor onsetting 1 s after stimulus presentation and spanning the duration of the imagine event was included to capture the act of imagining itself. These epoch regressors are the regressors of interest for present purposes. Separate regressors were included for imagining non-rewarded action and imagining rewarded action events. These regressors were subdivided into imagining rewards associated with left button responses (ImagineLeftReward) and imagining rewards associated with right button responses (ImagineRightReward), as well as non-rewards associated with both button responses (ImagineLeftNon-Reward, ImagineRightNon-Reward).
Additional regressors modeled left vs. right button presses. Contrasts were conducted on imagining a potential non-rewarded action outcome (ImagineLeftNon-Reward, ImagineRightNon-Reward) compared to imagining a potential rewarded action outcome (ImagineLeftReward, ImagineRightReward). This contrast would reveal whether there was significantly more activity for merely imagining a non-rewarded action outcome as opposed to a rewarded action outcome. Separate contrasts were computed for each subject, and results are based on a group-level random effects analysis on these contrasts.
Unless otherwise stated, all whole-brain results were thresholded at p < 0.01 uncorrected at the voxel-level with a 238 voxel cluster extent providing a corrected p < 0.05 threshold according to AlphaSim.
Results
Behavioral Results
Behavioral data were analyzed in order to confirm that subjects performed the task appropriately. If participants successfully followed instructions on either switching or repeating their response on the next trial, participants would on average receive 17 reward outcomes per run, or 51 reward outcomes over all three runs. However, participants could also commit errors if the instructions were not successfully followed, which resulted in an incorrect switch or an incorrect stay (e.g., switching the button response when the previous trial had yielded a reward outcome). Failure to follow the instructions resulted in fewer reward outcomes and increased the probability of receiving a non-reward outcome. On average, participants performed the task at a high level (mean number of reward outcomes per run = 15.95, SD = 1.02; mean number of errors committed per run = 1.05, SD = 1.11). Participants who received 12 or fewer reward outcomes for two or more runs were excluded from further analysis.
A subset of participants (N = 10) were given a debriefing survey after scanning asking whether they were able to visualize the motor response associated with each arrow, whether they were able to imagine the outcome associated with each button press, and whether they felt motivated to respond to gain the bonus money. Ratings were made on a Likert scale from 1 to 5, with 1 being the lowest confidence in the given response and 5 being the highest. In general, participants rated that they were able to visualize the motor response (mean rating = 4.3) and able to imagine the outcome associated with each button press (mean rating = 4.7). Participants also appeared to be motivated to perform the task well (mean rating = 4.6). A Wilcoxon Signed-Rank test showed that all ratings were significantly different from an average score of 3, which would represent indifference toward each of the questions (all P’s < 0.01). Hence, the behavioral data indicated that subjects understood and performed the task as instructed.
Imaging Results
We began by confirming that non-reward feedback produced heightened activation in the ACC compared to reward feedback as would be expected by prior literature (Hohnsbein et al., 1989; Gehring et al., 1990). Confirming these activations, the contrast of FeedbackNon-Rewarded–FeedbackReward produced robust activations in the dorsal ACC and pre-SMA, as well as lateral frontal and parietal regions. These results indicate that the paradigm appropriately elicited non-reward signals in the ACC.
Next, we examined the neural correlates of imagining non-rewarded actions. A whole-brain contrast of ImagineNon-Reward–ImagineReward revealed significant activations in bilateral dorsal ACC (MNI −10, 8, 46; k = 360 voxels; peak voxel z-value = 4.21), left precuneus (MNI −12, −62, 42; k = 264 voxels; peak voxel z-value = 3.66), right precuneus (MNI 14, −64, 24; k = 434 voxels; peak voxel z-value = 3.27), and left superior frontal sulcus gyrus (MNI −26, −2, 42; k = 263 voxels; peak voxel z-value = 3.41; see Figure 2). We have proposed that ACC activity signals in part the likelihood of an error in a particular condition (Brown and Braver, 2005, 2007), as part of a more general function of predicting the outcome of an action (Alexander and Brown, 2010). Our finding here of greater activity for imagining non-rewarded actions relative to imagining rewarded actions is consistent with this possibility (Refer to Table 1 for a summary of these activations.).
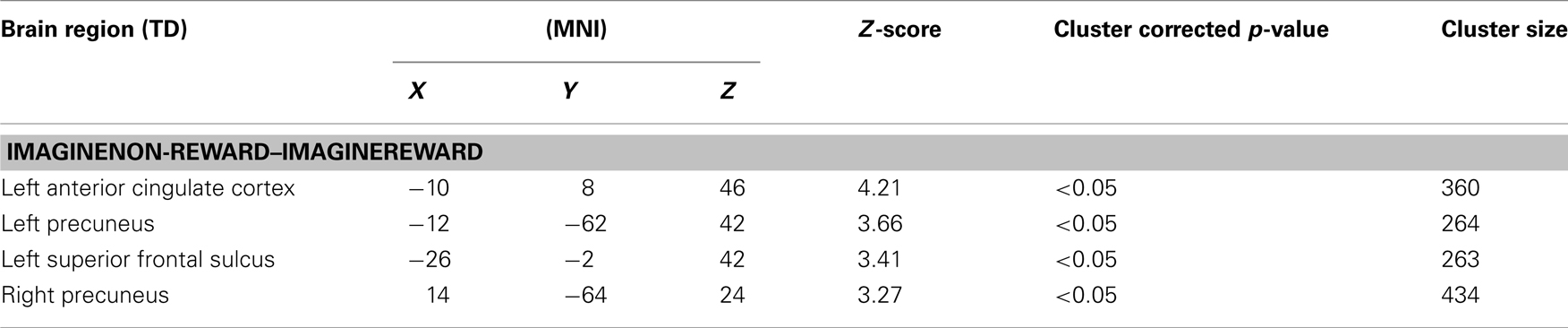
Table 1. Summary of activations for whole-brain analysis. Coordinates are reported in MNI space, and cluster size is given in number of contiguous voxels. All reported activations pass a cluster-corrected threshold of p < 0.05.
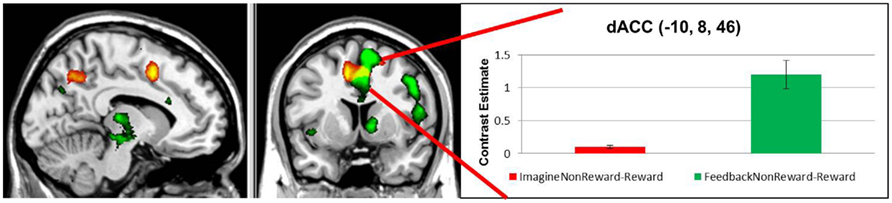
Figure 2. Red: regions showing increased activation in response to imagining a non-rewarded action contrasted with imagining a rewarded action response, depicted at p < 0.01, uncorrected. Green: areas showing greater activation for non-rewarding feedback contrasted with rewarding feedback, depicted at p < 0.001, uncorrected. Yellow: regions common to both contrasts.
Another possibility is that activation in the ACC represents response conflict (Botvinick et al., 2001). By this account, even when subjects imagine a non-rewarded action, they also maintain an active representation of the rewarded action as they subsequently intend to execute it. Thus, even though subjects were informed of the button response which would likely be rewarded – and therefore presumably were prepared for execution of this response – the presentation of an arrow cue for imagining a non-rewarded action would lead to preparation of the rewarded response. This would lead to greater summed motor cortex activity when imagining non-rewarded actions relative to imagining rewarded actions. This idea is consistent with previous research demonstrating that conflict activation in the ACC can precede actual response execution as forthcoming actions are anticipated (Sohn et al., 2007).
To address this possibility, we examined whether greater summed motor activation accompanied ImagineNon-Reward trials relative to ImagineReward trials as predicted by response conflict accounts. To do so, we identified regions in motor cortex (Areas 4 and 6) that showed effects of executing particular responses, i.e., RespondLeft > RespondRight (right motor cortex, MNI 46, −28, 54, k = 2161 voxels; Extent: 14 < x < 63, −39 < y < −6, 29 < z < 74) and RespondRight > RespondLeft (left motor cortex, MNI −34, −32, 46, k = 3437 voxels; Extent: −58 < x < −3, −48 < y < 9, 28 < z < 74) at a cluster corrected threshold of P < 0.001. We then extracted parameter estimates from these two regions for imagining rewarded and non-rewarded outcomes associated with either left or right button presses. This yielded 8 parameter estimates for each subject divided in a 2 (left/right motor cortex) × 2 (imagine left/right response) × 2 (imagine reward/non-reward) factorial design. These parameter estimates were analyzed using a three-way ANOVA, with subjects treated as a random factor. If ACC activation is driven by response conflict, we would expect a main effect of imagined outcome.
In contrast to the conflict monitoring predictions, there was no main effect of imagined outcome [F(1,18) = 0; p = 0.97]. Instead, there was a significant three-way interaction between motor cortex, imagined response, and imagined outcome; [F(1,18) = 45.23; p < 0.0001]. As depicted in Figure 3, subjects only exhibited motor cortex activity associated with the rewarded button press even when presented with a cue instructing them to imagine making a non-rewarded button press. This interaction suggests that the observed activity in both motor cortices was not due to summed motor activity for imagining non-rewarded outcomes associated with both left and right button presses. Thus, it appears that the present data cannot be explained by a conflict effect.
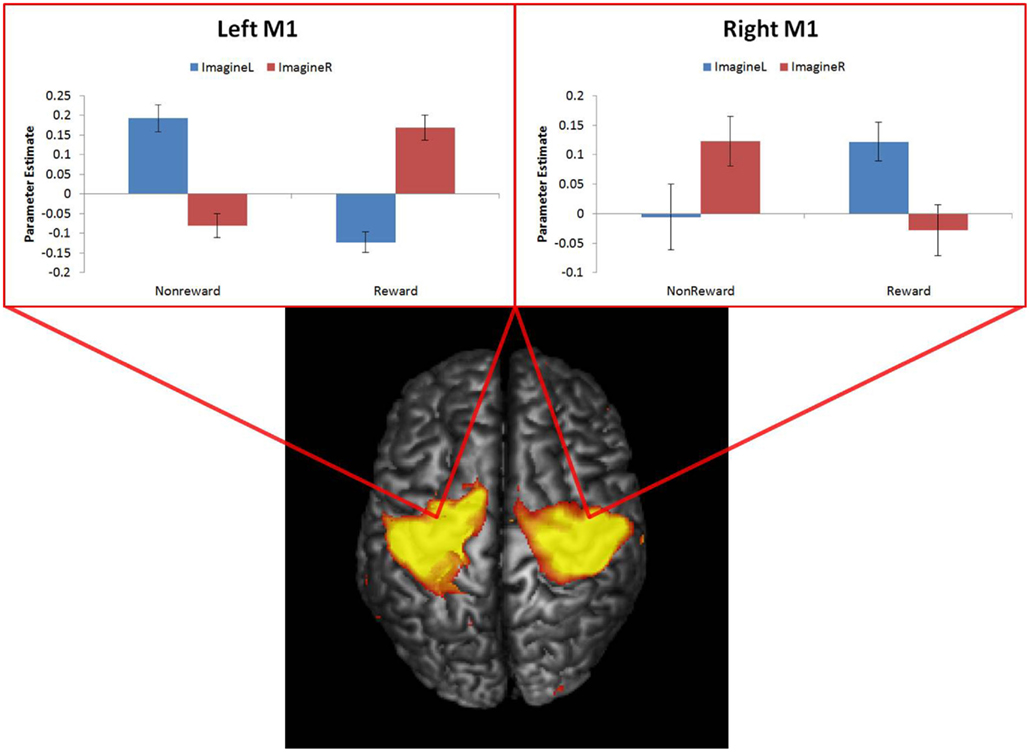
Figure 3. Parameter estimates extracted from left and right motor cortex for Imagining Rewarded and Imagining Non-Rewarded outcomes associated with left and right button presses. ImagineL, ImagineLeft; ImagineR, ImagineRight.
Discussion
The present study sought to explore the neural mechanisms involved in imagining possible actions and predicting their potential consequences, a concept variously referred as mentation (Goldman-Rakic, 1996) or “dynamic evaluation lookahead” (Van Der Meer and Redish, 2010) based on learned R–O predictions (Colwill and Rescorla, 1990). We identified the mPFC as playing a potential role in action outcome prediction. In prior studies, the mPFC has been implicated in predicting action outcomes (Brown and Braver, 2005; Valentin et al., 2007; Glascher et al., 2009; Krawitz et al., 2011) or similarly learning the value of actions (Kennerley et al., 2006; Rushworth et al., 2007), although previous studies have not isolated R–O prediction from the actual execution of the corresponding responses.
Because of the absence of explicit feedback and motor response during the Imagine condition, our findings in mPFC are unlikely to be accounted for by models assigning a role for error detection (Gehring et al., 1993). Our findings of greater ACC activity for imagining non-rewarded actions, combined with greater motor cortex activity representing the rewarded action response while subjects imagined non-rewarded actions, might initially seem consistent with the response conflict model (Botvinick et al., 2001) as extended to anticipation (Sohn et al., 2007). Nevertheless, multiple responses can lead to ACC activity even without response conflict (Brown, 2009), which suggests that ACC may reflect the anticipated responses and outcomes rather than conflict per se. Furthermore, anticipatory effects in ACC likewise do not necessarily entail response conflict (Aarts et al., 2008). Another possible alternative account of ACC activity is that it correlates with time on task (Grinband et al., 2010). We attempted to control for this by equalizing the duration period for imagining both rewarded action and non-rewarded action outcomes. However, we cannot entirely rule out the possibility that participants spent unequal amounts of time imagining the rewarded action vs. non-rewarded action options.
Imagining non-rewarded outcomes also produced activation in the precuneus and superior frontal sulcus. Activations in these regions might reflect increased imagery/working memory demands when imagining non-rewarded outcomes while simultaneously keeping the rewarded outcome in mind. The precuneus is a region that has been shown to be involved in various forms of imagery, such as visuo-spatial imagery (Selemon and Goldman-Rakic, 1988), episodic memory retrieval (Henson et al., 1999), and self-processing (Kircher et al., 2000). More relevant to our current study, experiments have revealed that the precuneus shows greater activation to imagined motor actions as opposed to actual motor executions, specifically in the case of imagined finger movements (Gerardin et al., 2000; Hanakawa et al., 2003). Additionally, the superior frontal sulcus is strongly related to working memory, especially in the spatial domain (Courtney et al., 1998). Taken together, the observed effect in the ACC may be part of a larger network of brain regions involved in the predicting the outcomes of imagined actions more generally.
Given the above, our results are consistent with a comprehensive computational model of mPFC as anticipating and then evaluating the outcome of planned actions. We have recently developed a new model of mPFC, the predicted response–outcome (PRO) model, according to which R–O predictions are generated and subsequently evaluated against actual outcomes in the mPFC (Alexander and Brown, 2011). A key prediction of the model is that mPFC (and especially ACC) signals a prediction of the anticipated outcome of an action, which may be subsequently compared against the actual outcome. In the model, discrepancies between actual and predicted action outcomes form the basis of the error effect in mPFC. These discrepancy signals are not limited to errors; they also signal surprisingly good outcomes (Jessup et al., 2010). There is ample evidence that surprising action outcomes are detected in part by ACC in monkeys (Ito et al., 2003; Hayden et al., 2011) and humans (Nee et al., 2011). Nevertheless, two theoretical questions remained open. First, it was unclear where the R–O predictions might originate from in humans, though at least stimulus if not action value may be represented in the OFC of humans (Valentin et al., 2007; Glascher et al., 2009), and actions may be simulated in the precuneus and superior frontal sulcus (Courtney et al., 1998; Gerardin et al., 2000). Our results are consistent with the outcome predictions originating from within the mPFC, although these are likely derived from component information in other regions such as the precuneus and superior frontal sulcus. Second, it was unclear whether the R–O predictions would be represented in the mPFC even when action execution was not imminent. Our results are consistent with the PRO model predictions and indicate that mPFC activity may reflect a subjective prediction of action outcomes. The present results suggest that these action outcome predictions are present even when action execution is merely imagined and not imminent. The region that responds to imagined errors overlaps with the region that responds to actual errors, which is consistent with a partial overlap between regions that predict outcomes and regions that evaluate actual outcomes. These findings, combined with prior evidence that mPFC activity is key to risk avoidance (Brown and Braver, 2005, 2007; Magno et al., 2006), are consistent with proposals that mPFC is a region crucial to the ability to anticipate and avoid adverse consequences even when a risky action is not planned to be executed immediately. Indeed, over-activity of the mPFC and especially ACC appears to be a key ingredient in obsessive–compulsive disorder (Machlin et al., 1991), in which the excessive urge to avoid potential dangers may be experienced even when no action is otherwise imminent.
As a whole, the results are consistent with the PRO model account of the mPFC as involved in predicting the potential outcomes of an action. The results suggest that them PFC evaluates potential outcomes with a view toward guiding decisions among possible actions even when action is not imminent. Our results provide a view of the networks involved in guiding decisions about actions and especially how those networks function when dissociated from action execution. These networks are central to a number of clinical disorders, and a better understanding of their role is urgent given that the impaired ability to think about and take into account the outcomes or consequences of actions is a hallmark of various clinical disorders such as obsessive–compulsive compulsive disorder, schizophrenia, and drug abuse (Petry and Casarella, 1999; Bechara et al., 2002). The identification of the neural mechanisms involved in prospective decision-making has the potential to inform more effective pharmacological and cognitive treatments in patient populations.
Conflict of Interest Statement
The authors declare that the research was conducted in the absence of any commercial or financial relationships that could be construed as a potential conflict of interest.
Acknowledgments
Supported by R01 DA026457 (Joshua W. Brown) and the Indiana METACyt Initiative of Indiana University, funded in part through a major grant from the Lilly Endowment, Inc. We thank B. Pruce and C. Chung for assistance with data collection.
References
Aarts, E., and Roelofs, A. (2011). Attentional control in anterior cingulate cortex based on probabilistic cueing. J. Cogn. Neurosci. 23, 716–727.
Aarts, E., Roelofs, A., and Van Turennout, M. (2008). Anticipatory activity in anterior cingulate cortex can be independent of conflict and error likelihood. J. Neurosci. 28, 4671–4678.
Alexander, W., and Brown, J. (2010). Computational models of performance monitoring and cognitive control. Top. Cogn. Sci. 2, 658–677.
Alexander, W. H., and Brown, J. W. (2011). Medial prefrontal cortex as an action-outcome predictor. Nat. Neurosci. 14, 1338–1344.
Bechara, A., Dolan, S., and Hindes, A. (2002). Decision-making and addiction (part II): myopia for the future or hypersensitivity to reward? Neuropsychologia 40, 1690–1705.
Botvinick, M. M., Braver, T. S., Barch, D. M., Carter, C. S., and Cohen, J. D. (2001). Conflict monitoring and cognitive control. Psychol. Rev. 108, 624–652.
Brown, J., and Braver, T. S. (2007). Risk prediction and aversion by anterior cingulate cortex. Cogn. Affect. Behav. Neurosci. 7, 266–277.
Brown, J. W. (2009). Conflict effects without conflict in anterior cingulate cortex: multiple response effects and context specific representations. Neuroimage 47, 334–341.
Brown, J. W., and Braver, T. S. (2005). Learned predictions of error likelihood in the anterior cingulate cortex. Science 307, 1118–1121.
Colwill, R., and Rescorla, R. (1990). Evidence for the hierarchical structure of instrumental learning. Anim. Learn. Behav. 18, 71–82.
Courtney, S. M., Petit, L., Maisog, J. M., Ungerleider, L. G., and Haxby, J. V. (1998). An area specialized for spatial working memory in human frontal cortex. Science 279, 1347–1351.
Dale, A. M. (1999). Optimal experimental design for event-related fMRI. Hum. Brain Mapp. 8, 109–114.
Daw, N. D., Niv, Y., and Dayan, P. (2005). Uncertainty-based competition between prefrontal and dorsolateral striatal systems for behavioral control. Nat. Neurosci. 8, 1704–1711.
Dayan, P., and Niv, Y. (2008). Reinforcement learning: the good, the bad and the ugly. Curr. Opin. Neurobiol. 18, 185–196.
Deichmann, R., Gottfried, J. A., Hutton, C., and Turner, R. (2003). Optimized EPI for fMRI studies of the orbitofrontal cortex. Neuroimage 19, 430–441.
Gehring, W. J., Coles, M. G. H., Meyer, D. E., and Donchin, E. (1990). The error-related negativity: an event-related potential accompanying errors. Psychophysiology 27, S34.
Gehring, W. J., Goss, B., Coles, M. G. H., Meyer, D. E., and Donchin, E. (1993). A neural system for error-detection and compensation. Psychol. Sci. 4, 385–390.
Gerardin, E., Sirigu, A., Lehericy, S., Poline, J. B., Gaymard, B., Marsault, C., Agid, Y., and Le Bihan, D. (2000). Partially overlapping neural networks for real and imagined hand movements. Cereb. Cortex 10, 1093–1104.
Glascher, J., Daw, N., Dayan, P., and O’Doherty, J. P. (2010). States versus rewards: dissociable neural prediction error signals underlying model-based and model-free reinforcement learning. Neuron 66, 585–595.
Glascher, J., Hampton, A. N., and O’Doherty, J. P. (2009). Determining a role for ventromedial prefrontal cortex in encoding action-based value signals during reward-related decision making. Cereb. Cortex 19, 483–495.
Goldman-Rakic, P. S. (1996). “The prefrontal landscape: implications of functional architecture for understanding human mentation and the central executive,” in The Prefrontal Cortex: Executive and Cognitive Functions, eds A. C. Roberts, T. W. Robbins, and L. Weiskrantz (Oxford: Oxford University Press), 87–103.
Grinband, J., Savitskaya, J., Wager, T. D., Teichert, T., Ferrera, V. P., and Hirsch, J. (2010). The dorsal medial frontal cortex is sensitive to time on task, not response conflict or error likelihood. Neuroimage 57, 303–311.
Hanakawa, T., Immisch, I., Toma, K., Dimyan, M. A., Van Gelderen, P., and Hallett, M. (2003). Functional properties of brain areas associated with motor execution and imagery. J. Neurophysiol. 89, 989–1002.
Hassabis, D., Kumaran, D., Vann, S. D., and Maguire, E. A. (2007). Patients with hippocampal amnesia cannot imagine new experiences. Proc. Natl. Acad. Sci. U.S.A. 104, 1726–1731.
Hayden, B. Y., Heilbronner, S. R., Pearson, J. M., and Platt, M. L. (2011). Surprise signals in anterior cingulate cortex: neuronal encoding of unsigned reward prediction errors driving adjustment in behavior. J. Neurosci. 31, 4178–4187.
Henson, R. N., Shallice, T., and Dolan, R. J. (1999). Right prefrontal cortex and episodic memory retrieval: a functional MRI test of the monitoring hypothesis. Brain J. Neurol. 122(Pt 7), 1367–1381.
Hohnsbein, J., Falkenstein, M., and Hoorman, J. (1989). Error processing in visual and auditory choice reaction tasks. J. Psychophysiol. 3, 32.
Ito, S., Stuphorn, V., Brown, J., and Schall, J. D. (2003). Performance monitoring by anterior cingulate cortex during saccade countermanding. Science 302, 120–122.
Jessup, R. K., Busemeyer, J. R., and Brown, J. W. (2010). Error effects in anterior cingulate cortex reverse when error likelihood is high. J. Neurosci. 30, 3467–3472.
Johnson, S. H. (2000). Thinking ahead: the case for motor imagery in prospective judgements of prehension. Cognition 74, 33–70.
Kaelbling, L. P., Littman, M. L., and Moore, A. W. (1996). Reinforcement learning: a survey. J. Artif. Intell. Res. 4, 237–285.
Kennerley, S. W., Walton, M. E., Behrens, T. E., Buckley, M. J., and Rushworth, M. F. (2006). Optimal decision making and the anterior cingulate cortex. Nat. Neurosci. 9, 940–947.
Kircher, T. T., Senior, C., Phillips, M. L., Benson, P. J., Bullmore, E. T., Brammer, M., Simmons, A., Williams, S. C., Bartels, M., and David, A. S. (2000). Towards a functional neuroanatomy of self processing: effects of faces and words. Brain Res. Cogn. Brain Res. 10, 133–144.
Krawitz, A., Braver, T. S., Barch, D. M., and Brown, J. W. (2011). Impaired error-likelihood prediction in medial prefrontal cortex in schizophrenia. Neuroimage 54, 1506–1517.
Machlin, S. R., Harris, G. J., Pearlson, G. D., Hoehn-Saric, R., Jeffery, P., and Camargo, E. E. (1991). Elevated medial-frontal cerebral blood flow in obsessive-compulsive patients: a SPECT study. Am. J. Psychiatry 148, 1240–1242.
Magno, E., Foxe, J. J., Molholm, S., Robertson, I. H., and Garavan, H. (2006). The anterior cingulate and error avoidance. J. Neurosci. 26, 4769–4773.
Nee, D. E., Kastner, S., and Brown, J. W. (2011). Functional heterogeneity of conflict, error, task-switching, and unexpectedness effects within medial prefrontal cortex. Neuroimage 54, 528–540.
Newman, S. D., Greco, J. A., and Lee, D. (2009). An fMRI study of the Tower of London: a look at problem structure differences. Brain Res. 1286, 123–132.
Petry, N. M., and Casarella, T. (1999). Excessive discounting of delayed rewards in substance abusers with gambling problems. Drug Alcohol Depend. 56, 25–32.
Rushworth, M. F., Buckley, M. J., Behrens, T. E., Walton, M. E., and Bannerman, D. M. (2007). Functional organization of the medial frontal cortex. Curr. Opin. Neurobiol. 17, 220–227.
Schoenbaum, G., Setlow, B., Saddoris, M. P., and Gallagher, M. (2003). Encoding predicted outcome and acquired value in orbitofrontal cortex during cue sampling depends upon input from basolateral amygdala. Neuron 39, 855–867.
Selemon, L. D., and Goldman-Rakic, P. S. (1988). Common cortical and subcortical targets of the dorsolateral prefrontal and posterior parietal cortices in the rhesus monkey: evidence for a distributed neural network subserving spatially guided behavior. J. Neurosci. 8, 4049–4068.
Shadmehr, R., and Wise, S. P. (2004). “Motor learning and memory for reaching and pointing,” in The Cognitive Neurosciences III, 3rd Edn, ed. M. Gazzaniga (Cambridge: MIT Press), 511–524.
Sohn, M. H., Albert, M. V., Jung, K., Carter, C. S., and Anderson, J. R. (2007). Anticipation of conflict monitoring in the anterior cingulate cortex and the prefrontal cortex. Proc. Natl. Acad. Sci. U.S.A. 104, 10330–10334.
Tremblay, L., and Schultz, W. (1999). Relative reward preference in primate orbitofrontal cortex. Nature 398, 704–708.
Valentin, V. V., Dickinson, A., and O’Doherty, J. P. (2007). Determining the neural substrates of goal-directed learning in the human brain. J. Neurosci. 27, 4019–4026.
Keywords: action values, anterior cingulate cortex, cognitive control, prospection
Citation: Jahn A, Nee DE and Brown JW (2011) The neural basis of predicting the outcomes of imagined actions. Front. Neurosci. 5:128. doi: 10.3389/fnins.2011.00128
Received: 17 August 2011; Accepted: 01 November 2011;
Published online: 23 November 2011.
Edited by:
Hauke R. Heekeren, Freie Universität Berlin, GermanyReviewed by:
Floris P. De Lange, Radboud University Nijmegen, NetherlandsPaul Sajda, Columbia University, USA
Copyright: © 2011 Jahn, Nee and Brown. This is an open-access article subject to a non-exclusive license between the authors and Frontiers Media SA, which permits use, distribution and reproduction in other forums, provided the original authors and source are credited and other Frontiers conditions are complied with.
*Correspondence: Joshua W. Brown, Department of Psychological and Brain Sciences, Indiana University, 1101 East Tenth Street, Bloomington, IN 47405, USA. e-mail:andtYnJvd25AaW5kaWFuYS5lZHU=