- 1 Department of Psychology, Stanford University, Stanford, CA, USA
- 2 Haas School of Business, University of California, Berkeley, CA, USA
Decision neuroscience offers the potential for decomposing differences in behavior across individuals into components of valuation intimately tied to brain function. One application of this approach lies in novel conceptualizations of behavioral attributes that are aberrant in psychiatric disorders. We investigated the relationship between social anxiety and behavior in a novel socially determined risk task. Behaviorally, higher scores on a social phobia inventory (SPIN) among healthy participants were associated with an increase in risky responses. Furthermore, activity in a region of the dorsal anterior insula (dAI) scaled in proportion to SPIN score in risky versus non-risky choices. This region of the insula was functionally connected to areas in the intraparietal sulcus and anterior cingulate cortex that were related to decision-making across all participants. Overall, social anxiety was associated with decreased risk aversion in our task, consistent with previous results investigating risk taking in many everyday behaviors. Moreover, this difference was linked to the anterior insula, a region commonly implicated in risk attitudes and socio-emotional processes.
Introduction
Among anxiety disorders, social anxiety has the highest lifetime prevalence in the U.S. population (Kessler et al., 1994). It is defined by fear and avoidance of a range of social situations, and negative physiological reactions during these encounters (Connor et al., 2000). Socially anxious individuals are typically stereotyped as withdrawn in social contexts, leading to overall less trusting, and more risk-averse behaviors (Erwin et al., 2003; Kashdan and McKnight, 2010). There is some evidence indicating that there is an overall difference in risk preferences with social anxiety; specifically, high social anxiety predicts greater risk aversion in the Balloon Analog Risk Task (BART; Maner et al., 2007). However, recent findings and theoretical arguments have argued that social anxiety may promote risk seeking in some circumstances. It has been shown that when expecting a positive outcome, social phobics have higher risk preferences than less socially anxious controls (Kashdan et al., 2006). Subsets of social phobics have also been found to engage in risky behaviors such as alcohol and drug use as emotion regulation strategies to protect against anxiety responses in social scenarios (Kashdan and McKnight, 2010). Similar compensatory behaviors may arise more generally, with increased aggression (or anger) expressed to protect against the consequences of anticipated withdrawal in social interactions (e.g., bargaining). Indeed, increased expression of anger has been found in individuals with high social anxiety (Erwin et al., 2003). Anger, in turn, has been associated in other work with increased risk-seeking choices (Lerner and Keltner, 2001). Overall, prior work indicates that risk taking may either increase or decrease as a function of social anxiety, particularly in anticipation of social interactions.
Given the complexity of the phenomenon, we aimed to study how social anxiety correlates with risk attitudes in a simplified social context. To do so, we employed an adapted version of a two-player two-stage response game (Figure 1, cf. Charness and Rabin, 2002; Charness and Rabin, 2005; Kosfeld et al., 2005; Krueger et al., 2007). The task was structured such that the target player decided between a risky or safe option in which the likelihood of greater reward depends on the anticipated beneficence of other players. We aimed to have the probability of different outcomes depend only on social factors while otherwise minimizing the impact of other player’s attitudes toward monetary gains. We expected that this feature of the task would maximize behavioral differences that depend on social anxiety, allowing for a direct assessment of the relationship between social anxiety and social risk taking in a simplified task. To enhance the emotional effects, we furthermore added subliminal social primes in the form of backward-masked fearful and happy faces. As a secondary aim, we examined the effect of fear versus happy social primes.
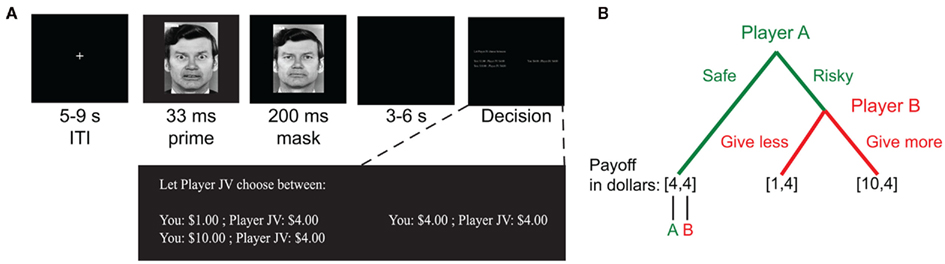
Figure 1. Task. (A) Trials began with a backward-masked emotion face prime (fearful or happy face). This was followed by a decision period in which participants were assigned the role of Player A in a two-person two-stage response game. No time limit was imposed, and options were displayed until the participant made a choice. (B) Participants selected between safe and risky choice options. For the safe option, both Player A and Player B received the same amount of money, V0 (e.g., $4). If the risky option was selected, Player A’s outcome depended on the choice made by Player B. Player B choices resulted in either smaller, Vmin (e.g., $1) or a larger, Vmax (e.g., $10), payments to Player A. In all possible outcomes, Player B always received the same amount of money, V0.
As indicated above, two hypotheses about how risk aversion may differ with social anxiety are suggested by the literature. We hoped to differentiate between these using measures of behavior and brain activity. With regard to brain activity, decision neuroscience has linked numerous brain areas to specific aspects of valuation in studies of risk and ambiguity. If social anxiety is associated with overall differences in risk aversion, then neuroimaging may reveal differences in neural structures associated with assessment and integration of the incentives in a risky choice. Numerous brain areas have been associated with these processes, most prominently areas in the prefrontal and posterior parietal cortex involved in cognitive and executive functions (Hsu et al., 2005; Huettel et al., 2005, 2006; Brand et al., 2007; Rangel et al., 2008). Conversely, if differences in risk aversion depend on emotional responses triggered by social context (cf. Kashdan et al., 2006; Kashdan and McKnight, 2010), then a different pattern of brain responses may emerge. Regions in the anterior insula have been associated with the emotional responses triggered by the anxiety associated with potential losses (Kuhnen and Knutson, 2005; Mohr et al., 2010). Intriguingly, social anxiety has been linked to hyperactivity in the anterior insula in other work (Etkin and Wager, 2007). Building from this, we therefore aimed to use neuroimaging to differentiate between the conflicting hypotheses about how risk attitudes are associated with social anxiety and to explore the patterns of neural activation that may give rise to these behavioral differences.
Materials and Methods
Participants
Twenty healthy males (ages 19–46 years, M = 25.0, SD = 6.8, one declined to specify) were recruited from the community surrounding Stanford University to participate in the study. To prevent knowledge of the purpose of the study, no mention of social anxiety was made during recruitment. We restricted recruitment only to males to reduce variability in emotional responses to emotional face primes used in the study (cf. Whalen et al., 1998). Two participants who exclusively chose only safe or only risky options were excluded from analysis, leaving a total of 18 participants included in the final data set (ages 19–46 years, M = 25.2, SD = 7.0). The study was approved by Stanford University’s Institutional Review Board, and all participants gave informed consent. Participants received $30 for participation in the 90-min experiment. Additionally, they were paid the outcome of one trial chosen at random from all choices made during the experiment.
Upon arrival, participants signed a consent form and completed a magnetic resonance screening form. They were presented with task instructions and two practice trials of the decision-making task on a laptop computer prior to entering the scanner.
Social Risk Task
The scanner session consisted of four task blocks of 16 trials each. The overall structure of the task is depicted in Figure 1A. Each trial contained a backward-masked face prime followed by a risky choice. The inter-trial interval was random, ranging from 5 to 9 s, during which a white fixation cross was shown. The fixation cross turned green 1 s before the onset of the face prime to signal that a trial was about to begin. A random inter stimulus interval of 3–6 s separated the face prime and the decision period.
Participants were instructed that they would be performing two tasks. The first task was a foil used to ensure that participants paid attention to the presentation of face stimuli that were otherwise instructed to be irrelevant. For this first task, participants were simply told to attend to the faces displayed in order to perform a recognition task at the end of the experiment. Face primes were fearful or happy expressions of eight individuals (eight fearful and eight happy primes per block; Ekman and Friesen, 1976). Backward-masked emotional faces were presented with a display time of 33 ms, mimicking the subliminal presentation of the same stimuli by others (Whalen et al., 1998). Each face presentation was immediately masked by a neutral face (of the same individual as the emotional face image) image for 200 ms. All images were in grayscale. We used this procedure to better elicit socially relevant emotions without consciously prompting participants to alter their decision-making strategy.
The second task was a two-player (Player A and B) decision task schematized in Figure 1B. Participants were instructed that they were to complete multiple one-shot iterations of a task with other anonymous players, whose responses were collected beforehand. They were further instructed that the other players in this task were unrelated to the faces shown for the memory task. Each round of the task proceeded as follows: for each choice, the amount the other player (Player B) received was fixed. To determine how much the participant (Player A) would receive, a choice was made between two options. One option guaranteed that both players each received the same amount of money (“safe” option; payment of V0 to both players). The other option allowed Player B to determine whether the participant received more (amount Vmax > V0) or less (Vmin < V0) money than the second player (“risky” option). For example, consider the choice depicted in Figure 1. For the safe option, the participant was guaranteed a payoff of $4. For the risky option, the participant may earn $1 or $10 depending on the action of Player B. Participants were told that since the other players changed on every trial, and only one random trial would be selected for payment, they should treat each trial as independent, and as if it were the only trial presented to them. The side of the screen on which the safe and risky options appeared was randomized across trials.
Player B choices were collected before the experiment by polling an independent random sample from the Stanford community. Participants (Players A) were informed that Player B responses were collected beforehand from real respondents, but were not instructed about how to assess the likelihood of Player B selecting either the higher or lower payment. They were also not provided feedback about the other players’ choices during the session. We did this to allow socially relevant emotions from the face presentation to better carry over to risky decision-making.
The range of values for the safe option (V0) was $3 to $8, the smaller value for the risky option (Vmin) was $1 to $7, and the larger values (Vmax) ranged from $5 to $14. The task included a total of 64 trials. In every trial the safe value was always intermediate between the large and small risky values (Vmin < V0<Vmax). A ratio term was computed for every trial with the following formula:

The ratio term is a measure of the relative potential gain over the potential loss of allowing the opponent to choose the outcome of the trial, and was the best predictor of choice outcomes in our experiment. Eight sets of eight trials were created, such that each set contained the same distributions of ratios ranging 1–3. The same sets of values were used for all participants. Two of these sets were randomly assigned to each block, one set for the fearful trials, and the other for the happy trials.
FMRI Acquisition and Analysis
Functional images were acquired with a 3-T General Electric Discovery scanner (Waukesha, WI, USA). T2*-sensitive gradient echo spiral in/out pulse sequences (Glover and Lai, 1998; Glover and Law, 2001) were used for functional imaging (33 oblique axial slices parallel to the AC–PC line, slice thickness = 4 mm, no gap, TR = 2000 ms, TE = 30, TE2 = 30.5, flip angle = 77, FOV = 20 cm, 64 × 64, ascending sequential). Spiral in/out methods have been shown to reduce signal loss in regions compromised by susceptibility-induced field gradients generated near air-tissue interfaces such as ventral PFC and striatum (Glover and Law, 2001; Preston et al., 2004). High-resolution T2-weighted fast spin-echo structural images (BRAVO) were acquired for anatomical reference (TR = 8.2 ms, TE = 3.2 ms, flip angle = 12 slice thickness = 1.0 mm, FOV = 24 cm, 256 × 256).
The imaging data were preprocessed and analyzed with SPM8 (Wellcome Department of Imaging Neuroscience, University of London). Preprocessing of the data used SPM8 for slice-timing correction, realignment to the first image for motion correction, coregistration, normalization to an Montreal Neurological Institute (MNI) template image, and spatial smoothing with an 8-mm full-width half-maximum Gaussian kernel.
Our main analyses were performed using whole-brain general linear model (GLM) analyses. Events of interest are described for individual analyses in Section “Results.” In all analyses we included a set of regressors to account for potentially confounding effects. Specifically, to account for variability in response times, we modeled the decision period using a boxcar with duration from the onset of the decision to the time of choice submission. We also included regressors for head movement during the experiment (estimated from realignment). Regressors of interest were convolved with a canonical hemodynamic response function.
AlphaSim (Ward, 2000) was used to calculate the appropriate cluster size for a corrected significance threshold of p < 0.05 (1000 Monte Carlo simulations). A minimum cluster size of 45 was required with a voxel-wise threshold of p < 0.005 given the smoothness of our preprocessed data.
Post-Scan Questionnaires
After completing the scanner session, participants completed the 17-item Social Phobia Inventory (SPIN; Connor et al., 2000) and the 20-item trait version of the Spielberger State-Trait Anxiety Inventory (STAI-trait; Spielberger, 1983). These questionnaires were administered at the end of the session to reduce subject knowledge of our hypotheses. We included the STAI-trait as a control measure for overall anxiety (non-social). STAI-trait scores did not correlate with any of the behavioral or neural indices discussed below. For succinctness, we therefore omit further discussion of this variable.
Results
Behavioral Results
On average, participants chose the risky option 57.8% of the time, but there were large individual differences in the proportion of risky choices made (SD = 17.6%, range = 25–84.4%). Mean reaction time was 5.0 s (mean reaction times across subjects: SD = 1.6 s, range = 2.7–7.4 s). There was no significant difference in reaction time for risky versus safe choices (p > 0.9). Furthermore, there was no significant relationship between average reaction time per subject and proportion of risky choices made (r = −0.0136, p > 0.9).
There was no significant effect of the emotion of the face prime on choice (fearful versus happy; p > 0.8 on β coefficient in logistic regression, see below). Because the specific emotion of the face prime had no significant effect on our observed results, for all the analyses that follow we averaged over fear and happy face primes, as has been done by others (Casey et al., 2011), to produce an aggregate measure of the effect of social emotions.
On the SPIN, participants scored an average of 19.0 out of a maximum of 68 (SD = 10.6, range = 1–46). Scores of 20 and above are considered clinically relevant. Our population therefore spanned a range of no significant social anxiety, to (in one case) severe. In subsequent analyses we analyzed SPIN scores as a continuous variable. However, for illustration purposes we split participants into low and high SPIN (e.g., Figure 2) around the median SPIN value of 18. Serendipitously, this median split approximately corresponds to clinically relevant and irrelevant SPIN scores.
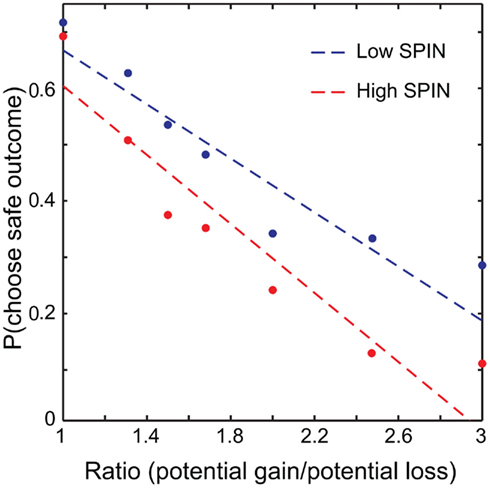
Figure 2. Probability of choosing the safe option plotted against ratio. A median split was performed, with data from low social phobia (SPIN) individuals plotted in blue and high SPIN in red. Linear fits were determined across all trials with ratio modeled as a continuous variable. For illustration, choices were grouped into seven ratio bins (1, 1.25/1.3333, 1.5, 1.6667/1.75, 2, 2.3333/2.5, 3) and plotted against mean probability of choosing the safe option for high and low SPIN participants.
To investigate the effects of social anxiety and ratio (see Eq. 1) on risky decisions, a linear mixed model was used with choice as the dependent variable, ratio, emotion prime, and SPIN as fixed effects and subject as a random effects variable. Variables were mean centered. As expected, the effect of ratio was highly significant: the higher the ratio, the more likely the risky choice was selected (p < 0.001). A main effect of social anxiety was also found: the probability that a risky option was chosen correlated positively with SPIN scores (p < 0.04). There was a significant ratio × SPIN interaction as well (p < 0.002). Higher SPIN scores were associated with higher differential sensitivity to ratio (Figure 2).
Neuroimaging Results
Correlations between behavior and neural activation
We performed two analyses to relate brain activity to choices. We began by constructing a GLM with five regressors of interest, one regressor each for fearful and happy emotion primes, and one for the decision period, with ratio and choice (1 for risky, −1 for safe) included as parametric modulators of decision-related activity. Using a fearful–happy contrast, no significant effects of emotion of face prime were found at our significance criterion anywhere in the brain. The ratio and choice regressors were separately used to identify candidate brain areas that govern evaluation of risk in our task. No main effects of choice were found at our significant threshold. However, brain areas that correlated with ratio include a number of areas that have been associated with risk assessment and decision-making in other work (Rushworth et al., 2004; Huettel et al., 2005, 2006; Rangel et al., 2008; Hare et al., 2009). We found significant effects in the supplementary motor area (SMA), anterior cingulate cortex (ACC), bilateral intraparietal sulcus (IPS), bilateral inferior frontal gyrus (IFG), and bilaterally in the ventral anterior insula (vAI; Figure 3; Table 1).
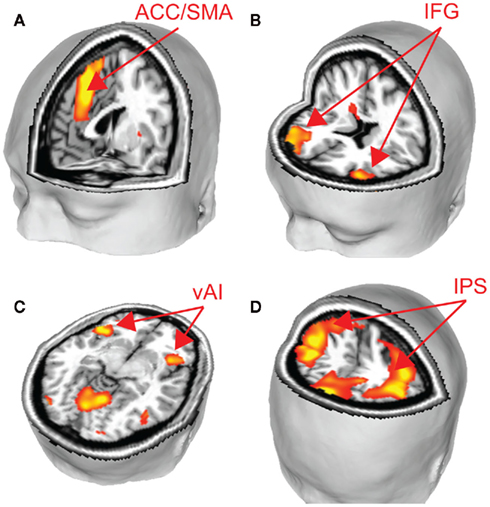
Figure 3. To identify brain areas that govern choice, we identified regions that correlated significantly with the ratio of potential gains and losses. Several key brain areas associated with risky decision-making were identified, including (A) SMA and ACC, (B) IFG, (C) vAI, and (D) IPS (p < 0.05, corrected). See Table 1.
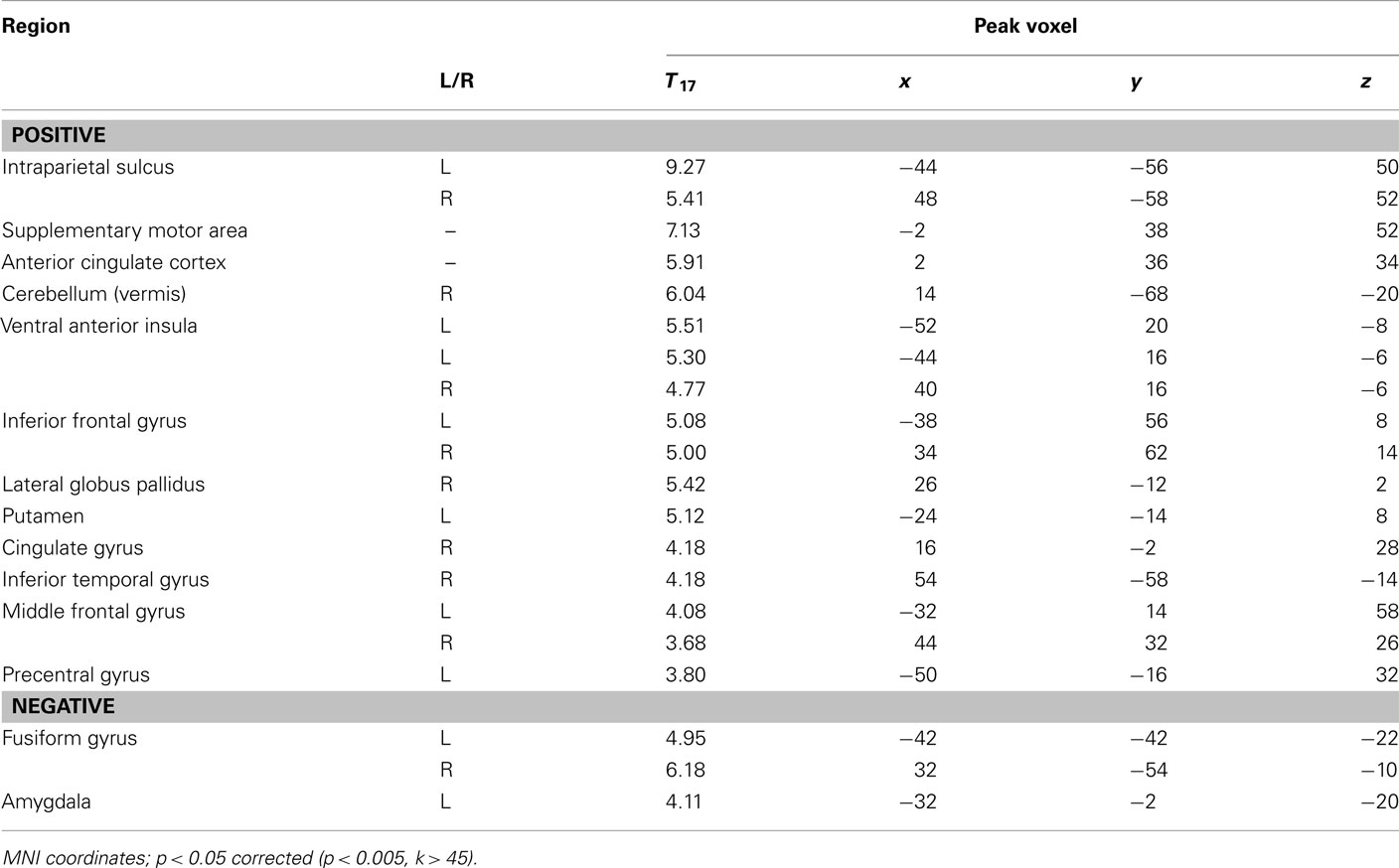
Table 1. Correlation between activity during the choice period and the ratio of potential gains to losses.
Because potential gain/loss ratio was found to be a strong predictor of choice in our behavioral analyses, we conducted an ROI analysis to further explore how the areas associated with ratio related to choice. ROIs were created using 6 mm radius spherical masks around the peak voxels from the following areas (coordinates are reported in MNI space): SMA (−2 38 52), ACC (2, 36, 34), bilateral IPS (46, −56, 50 and −44, −56, 50), bilateral vAI (40, 16, −6 and −44, 16, −6), bilateral IFG (31, 59, 14 and −38, 56, 8).
For each of these ROIs, the correlation between mean activity as a function of choice and the percentage of safe choices made was calculated across participants. Specifically, we hypothesized that if these brain areas are associated with decision-making, then differences in activity as a function of choice should predict individual propensities for selecting risky/safe alternatives. Results from this analysis are shown in Table 2. Using a threshold α of 0.0063, based on Bonferroni correction for multiple comparisons (p = 0.05/8 ROIs, SMA, ACC, and bilateral IFG, IPS, and vAI), significant negative correlations were found between activity in the left and right IPS and the proportion of safe choices made. Marginally significant results were found in each of the other ROIs except for the left vAI, ACC, and IFG. Based on these findings, we conclude that a number of areas are associated with evaluation of risky options in our task. However, the IPS (bilaterally) appears to play a particularly important role in governing individual differences in behavior (cf. Mohr et al., 2010).
Neural responses associated with effects of social anxiety on decision-making
To examine the effects of social anxiety, analyses were repeated with individual SPIN scores as a covariate. This allowed us to determine the correlation between social anxiety and BOLD signal change. A negative correlation was observed between SPIN score and BOLD activity for the choice (risky–safe) regressor in the left dorsal anterior insula (dAI; Figure 4A; Table 3). This region of the insula was distinct from that found in the analysis above, occupying a more dorsal/medial position (peak voxel at −28, 22, 2).
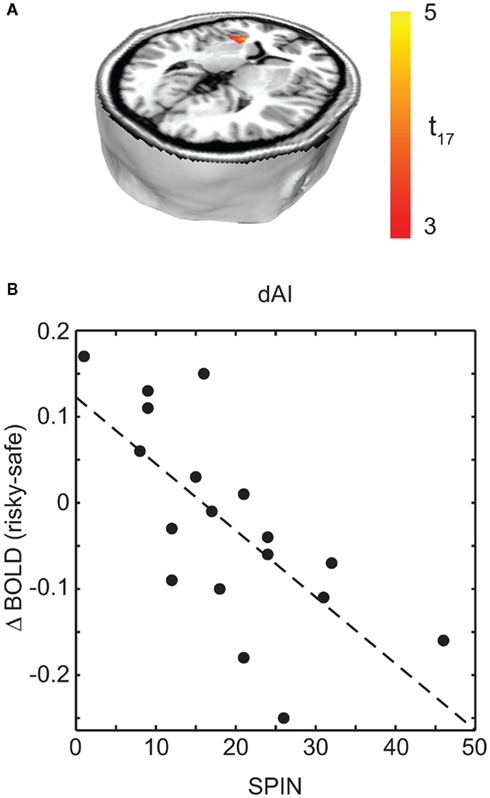
Figure 4. Regions associated with social anxiety were identified as those that show a difference based on choice (risky–safe) as a function of SPIN score. (A) This analysis identified a single region of interest in left dAI (p < 0.05, corrected). (B) Within this region of dAI, SPIN scores were negatively correlated with BOLD contrasts for risky–safe choices. Low SPIN individuals had higher activation in the dAI for risky versus safe choices, while the opposite was true in high social phobic individuals. See Table 3.
Interestingly, choice-dependent differences in dAI activity were of opposite sign for those participants with above and below clinically relevant SPIN scores. To illustrate this, Figure 4B shows mean dAI activity for each subject in a 6-mm sphere surrounding the peak dAI voxel identified in Figure 4A. Those participants with low social anxiety showed greater dAI activity in risky versus safe choices, consistent with previous reports (e.g., Kuhnen and Knutson, 2005; Mohr et al., 2010). The opposite finding held in participants with high SPIN scores. Specifically, choices for safe options were associated with greater dAI activity than choices made for risky options. A priori, we would have expected emotion regulation in high SPIN individuals to give reduced dAI activity, not that it would change the sign of the effect. Nonetheless, our critical hypothesis was confirmed: those participants with high SPIN scores show less activity in brain areas associated with emotions that lead to choice of safe outcomes.
Interaction between dAI region associated with social anxiety and brain areas responsible for evaluation of risk
We have identified the dAI as mediating the effects of social anxiety on behavior in our task. We have also identified a number of other areas as governing the effect of risk on choice. In this final analysis we determine how the dAI interacts with the regions associated with decision-making using functional connectivity analyses.
A psychophysiological interaction (PPI) analysis was conducted to find areas that show a stronger functional connectivity with the dAI during the decision periods of the task for risky versus safe choices. Using the peak voxel within the dAI as a seed region, raw time courses were extracted, z-normalized, corrected for linear drift, and used as regressors in a separate GLM analysis. In order to examine the task contrast of interest (risky versus safe choices), the time course values for six TRs after each onset of the decision period were multiplied by 1 for risky choices and −1 for safe. Six TRs (12 s) was the maximum time period possible for this design (to prevent interference from subsequent trials), and the full 12 s was included in our model so that an adequate signal could be obtained for PPI analysis (cf. Cohen et al., 2005; Park et al., 2010; van den Bos et al., 2011). Six motion regressors were included as regressors of no interest. Significant correlations were found in the ACC and the IPS for this model (Figure 5A; Table 4). These areas overlap with the regions that were found to be significantly correlated with ratio (the conjunction of results from the two analyses are shown in Figure 5B). This analysis was also carried out with the vAI as seed region. No significant connectivity was found with any other regions of the brain. Based on these findings, it seems that social anxiety influences risk preferences through interactions between the dAI and valuation processes in the ACC and IPS.
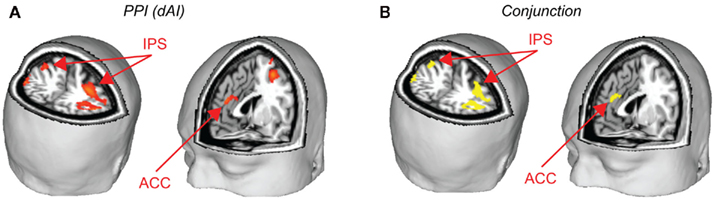
Figure 5. Psychophysical interaction analyses were performed to determine how the dAI interacts with brain areas related to decision-making. (A) Using the peak voxel of dAI as the seed, the IPS and ACC were identified as regions with higher functional connectivity to the dAI during risky versus safe choices. (B) These regions of the IPS and ACC overlapped with those identified as related to decision-making across all subjects. Yellow areas are those that were significant for the PPI analysis and which were also found to correlate with ratio (conjunction at p < 0.05, corrected, for both tests). See Table 4.
Discussion
Contrary to the common portrayal of social phobics as risk-averse and distrusting, the current study showed that the preference for risk on a social task correlated positively with social anxiety scores. As mentioned in the introduction, people with social anxiety have been found to employ risky behaviors as an emotion regulation strategy, especially when one expects positive outcomes to arise from these risks (Kashdan et al., 2006; Kashdan and McKnight, 2010). It is possible that in the modified version of the response game, in which the second players’ choices do not affect their own payoffs, participants might have expected that the other player would grant the larger sum. This expectation of a positive outcome may underlie the increased propensity of those with higher social anxiety scores to utilize risk as a compensatory strategy.
This rationale may also explain the discrepancies between the findings in this paper and those obtained using the BART as a measure of risk sensitivity. Maner et al. (2007) showed that social phobics were more risk-averse than controls using BART. One hypothesized cause for risky behavior in socially anxious individuals is that it compensates for anticipated anxiety in social situations. BART is strictly a single player game and, therefore, the need for compensatory emotion regulation does not arise. However, our task involves a second player and mimics a two-person interaction. These attributes may trigger socially anxious individuals to anticipate anxiety and compensate by increasing risk seeking. More generally, this social feature may highlight domains in which social anxiety is associated with greater or lesser risky behavior in daily life.
This is the first study using the task we employed. It is therefore relevant to note that the brain areas we identified as important for governing choices correspond well with areas associated with risky decision-making in other work. The IPS correlates with individual differences in risk attitudes in simple gambles (Huettel et al., 2006). Similarly, the ACC and SMA are involved in a number of decision-making tasks, including those that involve socially determined uncertainty (e.g., Sanfey et al., 2003; Baumgartner et al., 2008; van den Bos et al., 2009). Similarly, ventral regions of the AI correlate with perceptions of risk and drive choice outcomes accordingly (Sanfey et al., 2003; Kuhnen and Knutson, 2005; Bossaerts, 2010).
The anterior insula is increasingly being appreciated for its importance in numerous cognitive functions (Kurth et al., 2010; Deen et al., 2011). Ventral parts of the anterior insula have commonly been associated with perception of risk, as noted above (Bossaerts, 2010). The region of dAI that we find to be related to social anxiety lies at the intersection of regions associated with socio-emotional processes and cognitive processing in a recent large meta-analysis (Kurth et al., 2010). Our experiment was motivated by the hypothesis that assessment of socially determined risk would differentially trigger compensatory risk-seeking behavior as a function of social phobia. Relating this region of the dAI to social anxiety in our task therefore makes conceptual sense. Moreover, a recent study related hyperactivity of the same region of dAI to clinical presentation of social anxiety (Etkin and Wager, 2007).
Our analyses showed that the dAI is functionally connected to the IPS and ACC in a manner consistent with a role in biasing choice. At least in social contexts, even as simply approximated by our task, we conclude that the anterior insula is a region tied to clinically relevant behavior (risk seeking). This provides a new conceptual framework, rooted in cognitive neuroscience, for understanding aspects of the behavioral differences that manifest clinically as social anxiety.
Due to the small sample size of this study, we recruited only male participants to reduce sample variance. Furthermore, male participants were recruited because social phobia symptoms and risk taking behavior have been found to vary over the menstrual cycle (Chavanne and Gallup, 1998; Voelker, 1998; Bröder and Hohmann, 2003). Future studies including women are necessary in order to generalize the findings to both genders.
Advances in understanding the computational and brain bases of behavior have enabled a recent spurt of neurobiological accounts of various psychiatric disorders. For example, Maia and Frank (2011) link aspects of Parkinson’s disease, Tourette’s syndrome, ADHD, addiction and schizophrenia to specific functional deficits in cortico-basal ganglia circuitry. Some mood disorders have also been addressed. Differences in anterior insula activity associated with borderline personality disorder predict behavioral outcomes in a two-person trust game (King-Casas et al., 2008). Likewise, depression has been associated with specific computational deficits tied to serotonin function (Dayan and Huys, 2008). In non-clinical populations, behavioral preferences and associated brain activity also appear to depend on individual differences in personality traits; for example, in decisions involving risk, insula activity was found to correlate with harm avoidance and neuroticism, while activity in the temporal parietal junction, anterior insula, and ACC correlated with social value orientation (Paulus et al., 2003; van den Bos et al., 2009). Our results contribute to this growing literature in the domain of social anxiety.
Conflict of Interest Statement
The authors declare that the research was conducted in the absence of any commercial or financial relationships that could be construed as a potential conflict of interest.
Acknowledgments
This work was supported by a NeuroVentures pilot grant through Stanford University. We thank Bob Dougherty and Atsushi Takahashi for invaluable help at practically every stage of data collection and analysis. Chan Jean Lee made important contributions during the development of the task. Finally, we thank Kent Blake for help with data collection.
References
Baumgartner, T., Heinrichs, M., Vonlanthen, A., Fischbacher, U., and Fehr, E. (2008). Oxytocin shapes the neural circuitry of trust and trust adaptation in humans. Neuron 58, 639–650.
Bossaerts, P. (2010). Risk and risk prediction error signals in anterior insula. Brain Struct. Funct. 214, 645–653.
Brand, M., Grabenhorst, F., Starcke, K., Vandekerckhove, M. M. P., and Markowitsch, H. J. (2007). Role of the amygdala in decisions under ambiguity and decisions under risk: evidence from patients with Urbach-Wiethe disease. Neuropsychologia 45, 1305–1317.
Bröder, A., and Hohmann, N. (2003). Variations in risk taking behavior over the menstrual cycle. Evol. Hum. Behav. 24, 391–398.
Casey, B. J., Somerville, L. H., Gotlib, I. H., Ayduk, O., Franklin, N. T., Askren, M. K., Jonides, J., Berman, M. G., Wilson, N. L., Teslovich, T., Glover, G., Zayas, V., Mischel, W., and Shoda, Y. (2011). Behavioral and neural correlates of delay of gratification 40 years later. Proc. Natl. Acad. Sci. U.S.A. 108, 14998–15003.
Charness, G., and Rabin, M. (2002). Understanding social preferences with simple tests. Q. J. Econ. 117, 817–869.
Charness, G., and Rabin, M. (2005). Expressed preferences and behavior in experimental games. Games Econ. Behav. 53, 151–169.
Chavanne, T. J., and Gallup, G. G. (1998). Variation in risk taking behavior among female college students as a function of the menstrual cycle. Evol. Hum. Behav. 19, 27–32.
Cohen, M. X., Heller, A. S., and Ranganath, C. (2005). Functional connectivity with anterior cingulate and orbitofrontal cortices during decision-making. Cogn. Brain Res. 23, 61–70.
Connor, K. M., Davidson, J. R. T., Churchill, L. E., Sherwood, A., Foa, E., and Weisler, R. H. (2000). Psychometric properties of the Social Phobia Inventory (SPIN): new self-rating scale. Br. J. Psychiatry 176, 379–386.
Dayan, P., and Huys, Q. J. M. (2008). Serotonin, inhibition, and negative mood. PLoS Comput. Biol. 4, e4. doi:10.1371/journal.pcbi.0040004
Deen, B., Pitskel, N. B., and Pelphrey, K. A. (2011). Three systems of insular functional connectivity identified with cluster analysis. Cereb. Cortex 21, 1498–1506.
Ekman, P., and Friesen, W. V. (1976). Pictures of Facial Affect. Palo Alto, CA: Consulting Psychologists.
Erwin, B., Heimberg, R., Schneier, F., and Liebowitz, M. (2003). Anger experience and expression in social anxiety disorder: pretreatment profile and predictors of attrition and response to cognitive-behavioral treatment. Behav. Ther. 34, 331–350.
Etkin, A., and Wager, T. D. (2007). Functional neuroimaging of anxiety: a meta-analysis of emotional processing in PTSD, social anxiety disorder, and specific phobia. Am. J. Psychiatry 164, 1476–1488.
Glover, G. H., and Lai, S. (1998). Self-navigated spiral fMRI: interleaved versus single-shot. Magn. Reson. Med. 39, 361–368.
Glover, G. H., and Law, C. S. (2001). Spiral-in/out BOLD fMRI for increased SNR and reduced susceptibility artifacts. Magn. Reson. Med. 46, 515–522.
Hare, T. A., Camerer, C. F., and Rangel, A. (2009). Self-control in decision-making involves modulation of the vmPFC valuation system. Science 324, 646–648.
Hsu, M., Bhatt, M., Adolphs, R., Tranel, D., and Camerer, C. F. (2005). Neural systems responding to degrees of uncertainty in human decision-making. Science 310, 1680–1683.
Huettel, S. A., Song, A. W., and McCarthy, G. (2005). Decisions under uncertainty: probabilistic context influences activation of prefrontal and parietal cortices. Laterality 25, 3304–3311.
Huettel, S. A., Stowe, C. J., Gordon, E. M., Warner, B. T., and Platt, M. L. (2006). Neural signatures of economic preferences for risk and ambiguity. Neuron 49, 765–775.
Kashdan, T. B., Collins, R. L., and Elhai, J. D. (2006). Social anxiety and positive outcome expectancies on risk-taking behaviors. Cognit. Ther. Res. 30, 749–761.
Kashdan, T. B., and McKnight, P. E. (2010). The darker side of social anxiety: when aggressive impulsivity prevails over shy inhibition. Curr. Dir. Psychol. Sci. 19, 47–50.
Kessler, R. C., McGonagle, K. A., Zhao, S., Nelson, C. B., Hughes, M., Eshleman, S., Wittchen, H. U., and Kendler, K. S. (1994). Lifetime and 12-month prevalence of DSM-III-R psychiatric disorders in the United States. Results from the National Comorbidity Survey. Arch. Gen. Psychiatry 51, 8–19.
King-Casas, B., Sharp, C., Lomax-Bream, L., Lohrenz, T., Fonagy, P., and Montague, P. R. (2008). The rupture and repair of cooperation in borderline personality disorder. Science 321, 806–810.
Kosfeld, M., Heinrichs, M., Zak, P. J., Fischbacher, U., and Fehr, E. (2005). Oxytocin increases trust in humans. Nature 435, 673–676.
Krueger, F., McCabe, K., Moll, J., Kriegeskorte, N., Zahn, R., Strenziok, M., Heinecke, A., and Grafman, J. (2007). Neural correlates of trust. Proc. Natl. Acad. Sci. U.S.A. 104, 20084–20089.
Kuhnen, C. M., and Knutson, B. (2005). The neural basis of financial risk taking. Neuron 47, 763–770.
Kurth, F., Zilles, K., Fox, P. T., Laird, A. R., and Eickhoff, S. B. (2010). A link between the systems: functional differentiation and integration within the human insula revealed by meta-analysis. Brain Struct. Funct. 214, 519–534.
Maia, T. V., and Frank, M. J. (2011). From reinforcement learning models to psychiatric and neurological disorders. Nat. Neurosci. 14, 154–162.
Maner, J. K., Richey, J. A., Cromer, K., Mallott, M., Lejuez, C. W., Joiner, T. E., and Schmidt, N. B. (2007). Dispositional anxiety and risk-avoidant decision-making. Pers. Individ. Dif. 42, 665–675.
Mohr, P. N. C., Biele, G., and Heekeren, H. R. (2010). Neural processing of risk. J. Neurosci. 30, 6613–6619.
Park, S. Q., Kahnt, T., Beck, A., Cohen, M. X., Dolan, R. J., Wrase, J., and Heinz, A. (2010). Prefrontal cortex fails to learn from reward prediction errors in alcohol dependence. J. Neurosci. 30, 7749–7753.
Paulus, M. P., Rogalsky, C., Simmons, A., Feinstein, J. S., and Stein, M. B. (2003). Increased activation in the right insula during risk-taking decision making is related to harm avoidance and neuroticism. Neuroimage 19, 1439–1448.
Preston, A. R., Thomason, M. E., Ochsner, K. N., Cooper, J. C., and Glover, G. H. (2004). Comparison of spiral-in/out and spiral-out BOLD fMRI at 1.5 and 3 T. NeuroImage 21, 291–301.
Rangel, A., Camerer, C., and Montague, P. R. (2008). A framework for studying the neurobiology of value-based decision making. Nat. Rev. Neurosci. 9, 545–556.
Rushworth, M. F. S., Walton, M. E., Kennerley, S. W., and Bannerman, D. M. (2004). Action sets and decisions in the medial frontal cortex. Trends Cogn. Sci. (Regul. Ed.) 8, 410–417.
Sanfey, A. G., Rilling, J. K., Aronson, J. A., Nystrom, L. E., and Cohen, J. D. (2003). The neural basis of economic decision-making in the Ultimatum Game. Science 300, 1755–1758.
van den Bos, W., Cohen, M. X., Kahnt, T., and Crone, E. A. (2011). Striatum-medial prefrontal cortex connectivity predicts developmental changes in reinforcement learning. Cereb. Cortex. doi: 10.1093/cercor/bhr198
van den Bos, W., van Dijk, E., Westenberg, M., Rombouts, S. A. R. B., and Crone, E. A. (2009). What motivates repayment? Neural correlates of reciprocity in the Trust Game. Soc. Cogn. Affect. Neurosci. 4, 294–304.
Voelker, R. (1998). France and United Kingdom channel efforts to improve health services. JAMA 280, 681–683.
Ward, B. D. (2000). Simultaneous Inference for fMRI Data. Available at: http://afni.nimh.nih.gov/afni/docpdf/AlphaSim.pdf
Keywords: social anxiety, risk, SPIN, anterior insula, intraparietal sulcus, anterior cingulate cortex
Citation: Tang GS, van den Bos W, Andrade EB and McClure SM (2012) Social anxiety modulates risk sensitivity through activity in the anterior insula. Front. Neurosci. 5:142. doi: 10.3389/fnins.2011.00142
Received: 01 October 2011;
Accepted: 09 December 2011;
Published online: 03 January 2012.
Edited by:
Kerstin Preuschoff, University of Zurich, SwitzerlandReviewed by:
Martin P. Paulus, University of California San Diego, USALuke Clark, University of Cambridge, UK
Copyright: © 2012 Tang, van den Bos, Andrade and McClure. This is an open-access article distributed under the terms of the Creative Commons Attribution Non Commercial License, which permits non-commercial use, distribution, and reproduction in other forums, provided the original authors and source are credited.
*Correspondence: Grace S. Tang, Department of Psychology, Stanford University, 450 Serra Mall, Building 420, Stanford, CA 94305, USA. e-mail:Z3N0YW5nQHN0YW5mb3JkLmVkdQ==