- 1 Department of Physiology and Biophysics, Howard Hughes Medical Institute, University of Washington, Seattle, WA, USA
- 2 Department of Philosophy, Dartmouth College, Hanover, NH, USA
This essay reviews recent developments in neurobiology which are beginning to expose the mechanisms that underlie some elements of decision-making that bear on attributions of responsibility. These “elements” have been mainly studied in simple perceptual decision tasks, which are performed similarly by humans and non-human primates. Here we consider the role of neural noise, and suggest that thinking about the role of noise can shift the focus of discussions of randomness in decision-making away from its role in enabling alternate possibilities and toward a potential grounding role for responsibility.
Introduction
As neuroscience begins to expose the brain mechanisms that give rise to decisions, what do the assortment of facts tell us about such philosophical concepts as responsibility and free will? To many, these concepts seem threatened because of an inability to reconcile a truly free choice with either deterministic brain mechanisms on the one hand or stochastic effects on the other. The former seem to negate the notion of choice by rendering it predictable, at least in principle, or as being under the control of forces external to the agent. The latter reduces choice to caprice, a weak freedom that precludes any meaningful assignment of responsibility. In this essay, we offer an alternative perspective that is informed by the neural mechanisms that underlie decision-making.
Some of these mechanisms point to features that distinguish agents from each other and allow us to understand why one agent might make a better or worse choice than another agent. We suggest that more attention be paid to these aspects of decision-making, and that such attention may help bridge the neurobiology of decision-making (NBDM) and philosophical problems in ethics and metaphysics. Our idea is not that the neurobiology supports one particular philosophical position, but that certain principles of the NBDM are relevant to ethicists of many a philosophical persuasion.
Neuroscience and the Philosophy of Freedom and Responsibility
Figure 1 shows in broad brushstrokes the main philosophical positions regarding free will. In the philosophical literature, theorists can be classified according to the relation they see between the truth of determinism and the possibility of freedom.
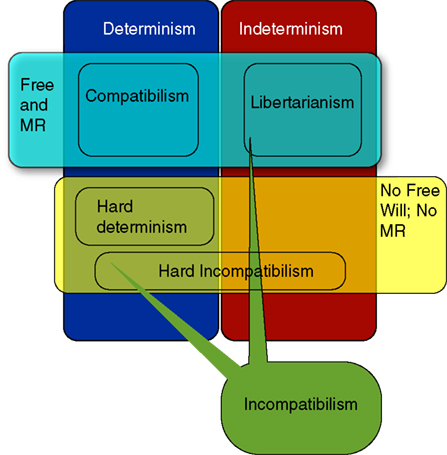
Figure 1. Basic layout of traditional positions in philosophy regarding the relation of free will (FW) and moral responsibility (MR) to determinism. Compatibilism is the view that FW and MR are compatible with a deterministic universe. Incompatibilism is the view that they are not. Incompatibilists come in a variety of flavors: Hard determinists think that determinism is true and we are not free; Libertarians believe that determinism is false and we are free in virtue of indeterminism. Hard incompatibilists believe that FW and MR are impossible, for they are not compatible with either determinism or indeterminism. There are a variety of compatibilist positions that provide different accounts of how FW and MR are not threatened by determinism. There are also accounts (not discussed here) that separate the conditions for FW and MR, and thus allow that MR is possible in a deterministic universe even if FW is not. These are sometimes referred to as versions of compatibilism, and sometimes called semi-compatibilism.
To some, the more knowledge we have about the workings of the brain, the less it seems possible that we exercise free will when we make choices, and the less it seems that we can be held responsible for our decisions (Crick, 1994; Schall, 2001; Greene and Cohen, 2004; Glimcher, 2005). It is not just the physicalist concept that the mind is the brain, but that as we come to understand more about how the brain gives rise to choices, mechanisms seem to displace freedom. At least some philosophers and many neuroscientists wonder whether moral responsibility is something that we would reject if we knew everything about the machinery of the human brain. They worry that the neuroscience of decision-making will render concepts like free will and responsibility “quaint fictions” – although perhaps essential ones that we rely upon as social agents. As the NBDM exposes the mechanisms that underlie choice behavior, our agency seems to be replaced by a machine that converts circumstances into an outcome without any real choice at all. NBDM is thus perceived by many as supporting “hard determinism”1: rendering the cause–effect chains with a modern brush.
Compatibilists argue that determinism does not strip the agent of choice, responsibility, or freedom (Frankfurt, 1971; Strawson, 1974; Hume, 1975/1748; Dennett, 1984; Bok, 1998; Blackburn, 1999; James, 2005/1884). Indeed, some compatibilists deny that the practice of ethics, and the concept of responsibility which it presupposes, depends upon any reconciliation of human action with fundamental physics for justification (Strawson, 1974; Williams, 1985). Even if one adopts a compatibilist view, however, there is no particular reason to exclude neuroscientific facts from ethical discussion. Although neuroscience is not foundational to ethics, it has the potential to illuminate capacities and limitations of a decision maker. Capacities like impulsivity and rationality are obvious examples.
Box 1. Some definitions.
Physicalism: The thesis that all that exists is physical or supervenes on the physical.
Reductionism: The thesis that all complex systems can be explained by explaining their component elements.
Emergent properties: New properties that arise in a complex system as a result of low-level interactions.
Eliminativism: The view that the terms we use to describe a domain are either redundant or in error and thus could be eliminated from our discourse.
Neural noise: Variability in neural signal not tied to the signaling function of the neuron.
Compatibilism: The thesis that free will is compatible with determinism.
Incompatibilism: The thesis that free will is incompatible with determinism.
Hard determinism: The view that free will is incompatible with determinism, determinism is true, and we therefore lack freedom.
Libertarian free will: Free will dependent on indeterminism; the main idea is that indeterminism allows agents to break free of the chain of causation.
In this essay, we explain why we think that neuroscience reveals aspects of decision-making with the potential to illuminate our conception of ethical responsibility.
Like nearly all neuroscientists, we accept physicalism. All matters mental are caused by brains. This leaves open the possibility that not every aspect of our thoughts and feelings can be adequately expressed in reductionist terms. We leave open the possibility of emergent phenomena: properties that arise from simpler causes but which are not explained away by them2.
Our goal is to demonstrate a correspondence between neural mechanisms and some elements that compatibilists have long suspected must be present. Rather than “explain away” free will, the neurobiology enhances our conception of ourselves as having will, agency, authorship, and real options. In the end, we hope to convince a certain kind of compatibilist that neuroscience matters in ways that he tends to miss because he is so focused on dismissing the entire body of physical knowledge wielded by the hard determinist to argue against freedom and responsibility. And we hope to convince the incompatibilist that neuroscience can be explanatory without rendering responsibility and free will quaint but illusory.
Free Will and Responsibility
Having free will minimally implies that when I choose A (i) I do so with some degree of autonomy, and (ii) in some sense, I could have made another choice3. The first condition implies ownership of the choice. My choice cannot be explained entirely by forces outside the ones I control as an agent. The second means that there is a real alternative and that I could choose that alternative. Our arguments here will focus on the former condition, although they have some impact on the second as well.
Most people take it that moral responsibility implies freedom: One can only be responsible if one is free. For someone to be held responsible for an action, they must be, in some sense, a cause of that action. Moreover, assignment of moral responsibility is relative to the properties of a decision maker or agent. This invites us to explain a relevant part of the decision as depending fundamentally on properties of the deciding agent. The relevant properties are, loosely, what we refer to as constitution, temperament, values, interests, passions, capacities, and so forth. In our discussion of the neurobiology, we will refer to such properties as policies that govern parameters of the decision-making process, such as the tradeoff between speed and accuracy.
Predictability and Determinism
Some scientists might conceptualize the problem a little differently than the organization depicted in Figure 1, but the same basic elements are present: causes and effects, randomness and predictability (Crick, 1994; Schall, 2001; Glimcher, 2005). Many neuroscientists, physicists, and mathematical theorists subscribe to the following position: they are (1) physicalists who (2) believe the mental is explained by a physical brain through chains of causation, but (3) they also embrace some elements of randomness. The randomness can be fundamental indeterminism, based on principles of quantum mechanics, or it can be uncertainty that arises from complexity in a deterministic system whose quantum effects are negligible. This randomness implies that an agent’s choices are not practically predictable from the history of events or the state of the brain beyond probabilistic expectations.
Libertarians deny that freedom is compatible with determinism, but believe that indeterminism is true and makes freedom possible (Kane, 2002). Many scientists likewise deny that freedom is compatible with determinism, and reject the notion that the universe (or brain) is determined, because of the likelihood of randomness. However, unlike Libertarians, they reject the idea that randomness confers freedom or responsibility. Let us call them “Scientific Hard Incompatibilists” or SHIs. SHIs think the sources of randomness provide the basis for a physical understanding of the unpredictable, and recognize that in the real world even deterministic processes are coupled with a randomness that muddies the deterministic machinery from the perspectives of both actor and the observer. Prediction is imperfect. Choices can be dissected into determined and random components (necessity and chance). However, and perhaps ironically, SHIs also believe that randomness cannot confer free will and responsibility. There is no “willing” and certainly no responsibility for a choice that is explained only by randomness. In this sense, “Chance is as relentless as necessity” (Blackburn, 1999). Therefore, the SHI concludes that free will and responsibility are illusory.
By focusing on the question only of whether low-level deterministic or indeterministic processes make room for free will and responsibility, we believe SHI’s dissection leaves out something essential. As explained in the next section, the neurobiology invites us to view uncertainty not so much as it bears on predictability but on the strategy that an agent adopts when making a choice in the face of uncertainty. The neurobiology sheds light on how these strategies are implemented and therefore why one decision maker may make one choice, whereas another individual may choose differently.
Neurobiology of Decision-Making
Here we provide a brief and highly selective review of some findings in neuroscience about the neural bases of decision-making. Neurobiology is beginning to illuminate the mechanisms that explain why one agent makes one choice, whereas another would choose differently. We discuss the role of randomness in explaining such choices. The role that this randomness plays in our argument is not to confer freedom but to necessitate high-level policies regarding decisions. Although these policies themselves do not immediately provide conceptual grounding for responsibility, they provide a potential locus for philosophical arguments linking the nature of the agent to his or her decisions.
Simple Decisions
A decision is a commitment to a proposition or plan, and a decision process encompasses the steps that lead to this commitment, what is often termed deliberation among options. These options may take the form of actions, plans, hypotheses, or propositions. Most decisions are based on a variety of factors: evidence bearing on prior knowledge about the options, prior knowledge concerning the relative merit of the options, expected costs and rewards associated with the matrix of possible decisions and their outcomes, and other costs associated with gathering evidence (e.g., the cost of elapsed time). This formulation is not exhaustive, but it covers many types of decisions, ranging from simple to complex. Because the elements listed in this paragraph play a role in simple decisions as well as complex ones, it is possible to study the NBDM in non-human animals, including our evolutionarily close relatives, monkeys. This research has begun to expose basic principles that are applicable to the more complex decisions we make in our lives, including those for which we can be held morally responsible.
The process of deciding generally has a beginning and an end. For perceptual decisions about the direction of motion, like the one depicted in Figure 2A,B, the onset of a random-dot visual stimulus marks the beginning of the decision process. Of course, other aspects of the decision process are already in play before this. They might be lumped together as establishing the rules of engagement from various contextual cues: something in the brain establishes that a decision is to be made in the first place, that the source of information resides in a region of the visual field, that the useful information is encoded by a set of neurons in the visual cortex, and that the mode of response will be an eye movement to a target. The stream of information from the stimulus is processed by specific regions in visual cortex, which supply a stream of evidence to downstream processes. This momentary evidence furnishes a fresh piece of information at each instant that bears on the decision process. These bits of evidence are accumulated until there is enough to render a decision. Mainly for convenience, we term this commitment point the end of the decision process. After that, either an action ensues to communicate or enact the decision, or there is some delay during which such an action is planned (the occasional change of mind is understood as a second decision process; Resulaj et al., 2009).
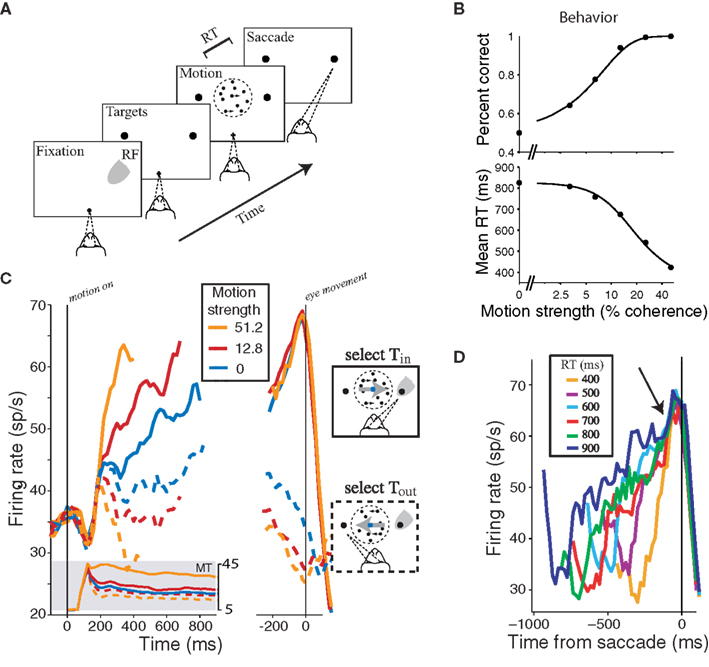
Figure 2. Neural mechanism of a decision about direction of motion. (A) Choice-reaction time (RT) version of the direction discrimination task. The subject views a patch of dynamic random dots and decides the net direction of motion. The decision is indicated by an eye movement to a peripheral target. The subject controls the viewing duration by terminating each trial with an eye movement whenever ready. The gray patch shows the location of the response field (RF) of an LIP neuron. (B) Effect of stimulus difficulty on choice accuracy and decision time. Solid curves are fits of a diffusion model, which accounts simultaneously for choice and decision time. (C) Response of LIP neurons during decision formation. Average firing rate from 54 LIP neurons is shown for three levels of difficulty. Responses are grouped by motion strength and direction of choice, as indicated. Left graph, The responses are aligned to onset of random-dot motion and truncated at the median RT. These responses accompany decision formation. Shaded insert shows average responses from direction selective neurons in area MT to motion in the preferred and anti-preferred directions (solid and dashed traces, respectively). After a transient, MT responds at a nearly constant rate. The LIP firing rates approximate the integral of a difference in firing rate between MT neurons with opposite direction preferences. Right graph, The responses are aligned to the eye movement. For Tin choices (solid curves), all trials reach a stereotyped firing rate before saccade initiation. We think this level represents a threshold or bound, which is sensed by other brain regions to terminate the decision. (D) Responses grouped by RT. Only Tin choices are shown. Arrow shows the stereotyped firing rate occurs ∼70 ms before saccade initiation. Adapted with permission from Gold and Shadlen (2007) insert from on line-data base used in Britten et al. (1992), www.neuralsignal.org data base nsa2004.1.
We know much about the neurobiology underlying this type of simple decision. The stream of momentary evidence comes from neurons in the visual cortex, concentrated in an area of the macaque brain called the middle temporal visual area (MT; also known as V5). These neurons respond better when motion through their receptive fields is in one direction and not in another. They have a background discharge, which is modulated by the random-dot motion stimulus. If the neuron prefers rightward motion, then it tends to produce action potentials at a faster rate when motion is to the right than when it is to the left. When the decision is difficult – that is, when only a small fraction of randomly appearing dots actually move to the right at any moment – the same neuron increases its discharge albeit less vigorously. When the motion is leftward but not strongly coherent, the neuron also increases its discharge, though now to an even lesser degree. For strong motion to the left the neuron would typically discharge at the background rate or possibly slightly below. The mechanisms for extracting this momentary evidence about direction are reasonably well understood (Born and Bradley, 2005). It is also clear from lesion and microstimulation experiments that these MT neurons supply this evidence to the decision process (for reviews, see Parker and Newsome, 1998; Gold and Shadlen, 2007).
The decision on this task benefits from an accumulation of evidence in time. The direction selective sensory neurons described in the previous paragraph do not accumulate evidence (Figure 2C, inset). Their responses represent the momentary information in the stimulus. Other neurons, which reside in association cortex, represent the accumulation of this momentary evidence. A key property of neurons in these areas – the vast majority of the cortical mantle in primates – is the capacity to maintain discharge for longish periods in the absence of an immediate sensory stimulus, or an immediate motor effect. The exact parameterization of “longish” is not known, but it is at least in the seconds range. This is in marked contrast to sensory neurons like the ones discussed above, which keep up with a changing environment (tens of milliseconds) or motor neurons, which cause changes in body musculature on a similar timescale. Indeed it is likely that this flexibility in timescale underlies many of the higher cognitive capacities that we cherish.
Some of these neurons in association cortex produce firing rates that reflect the accumulated evidence from the motion stimulus. For example, neurons in the lateral intraparietal area (LIP) in parietal cortex respond to visual stimuli in a restricted portion of space, termed the response field (RF), but they also respond when the RF has been cued as a potential target of an eye movement. These neurons “associate” information from vision with plans to look (Gnadt and Andersen, 1988; Andersen, 1995; Mazzoni et al., 1996; Colby and Goldberg, 1999; Lewis and Van Essen, 2000). During the decision process, these LIP neurons represent the accumulated evidence that one of the choice targets is a better choice (given the task) than the other. While MT neurons are producing spikes at a roughly constant rate, neurons in LIP gradually increase or decrease their rate of discharge as more evidence mounts for or against one of the choices. If the stimulus is turned off and a delay period ensues, MT neurons return to their baseline firing rates, but LIP neurons, whose response fields contain the chosen target, emit a sustained discharge that indicates the outcome of the decision-effectively, a plan to make an eye movement to that target.
When the decision maker is permitted to answer at will, the LIP neurons also lend insight into the mechanism whereby the decision terminates. As shown in Figures 2C,D, the decision ends when the firing rates of certain LIP neurons achieve a critical level. Whether the decision was based on strong or weak evidence and whether the process transpired quickly or not, the LIP responses achieve the same level of discharge at the moment of decision. This is an indication that there is a threshold for terminating the decision process. Since the LIP firing rate represents the accumulation of momentary evidence, the termination “rule” is to commit to a choice when the accumulated evidence reaches a critical level. For example, the rule might be: if the rightward preferring MT neurons have produced ∼6 spikes per neuron more than the leftward preferring neurons, choose right; else if the leftward preferring MT neurons have produced ∼6 spikes per neuron more that the rightward preferring neurons, choose left; else continue to accumulate evidence. This implies that LIP neurons are effectively computing the integral of the difference in firing rates between rightward and leftward preferring MT neurons (Ditterich et al., 2003; Huk and Shadlen, 2005; Hanks et al., 2006; Wong et al., 2007).
This mechanism of accumulation of evidence to a threshold level is called bounded accumulation (or bounded drift–diffusion, or random walk to bound; Figure 3). The idea was developed in the 1940s as a statistical process for deciding between alternatives (Wald, 1947), and it played a key role in British wartime code-breaking (Good, 1979). It has found application in areas of sensory psychology (Link, 1992) and cognitive psychology (Ratcliff and Rouder, 2000; Usher and McClelland, 2001; Bogacz et al., 2006). In all of these cases the threshold for terminating the decision process, what we will call the “bound,” controls both the speed and the accuracy of the decision process (e.g., Figure 2B). This tradeoff is an example of a policy that the brain implements to shape its decisions.
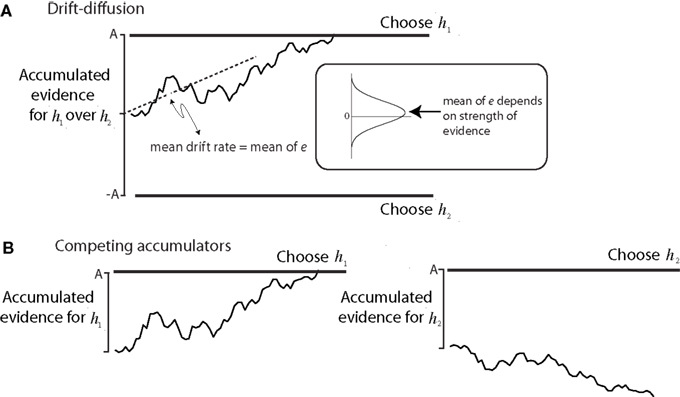
Figure 3. Models of bounded evidence accumulation. (A) Random walk or drift diffusion. Noisy momentary evidence for/against hypotheses h1 and h2 is accumulated (irregular trace) until it reaches an upper or lower termination bound, leading to a choice in favor of h1 or h2. In the motion task, h1 and h2 are opposite directions (e.g., right and left). The momentary evidence is the difference in firing rates between pools of direction selective neurons that prefer right and left. At each moment, this difference is a noisy draw from a Gaussian distribution with mean proportional to motion strength. The mean difference is the expected drift rate of the diffusion process. (B) Competing accumulators. The same mechanism is realized by two accumulators that race. If the evidence for h1 and h2 are opposite, then the race is mathematically identical to symmetric drift–diffusion. The race is a better approximation to the physiology, since there are neurons in LIP that accumulate evidence for each of the choices. This mechanism extends to account for choices and RT when there are more than two alternatives. Reprinted with permission from Gold and Shadlen (2007).
Typically, when a stream of evidence is available, a decision maker will tend to make fewer errors if she takes more time. In the motion task, it appears that this is achieved by raising the level of the bound for terminating the decision process. This simple adjustment to the mechanism leads to longer decision times and to more reliable evidence at the point of termination (Palmer et al., 2005). In the case of the motion experiment, the policy is establishing the tradeoff between speed and accuracy of the direction judgments. The resultant payoff is something like the rate over which reward is obtained and errors avoided (Gold and Shadlen, 2002; Bogacz et al., 2006).
The neurobiology underlying the setting of the bound (and detecting that the accumulation in LIP has reached the bound) is not currently known; it ought to be an area of intense study. The most promising candidate mechanisms involve the basal ganglia. These structures seem to possess the requisite circuitry to terminate the decision process based on a threshold crossing and to adjust the bound based on cues about how the current “policy” for making decisions is paying off (Bogacz et al., 2006; Lo and Wang, 2006).
Neurobiology supports the view that a decision process balances evidence gathering with other “policy” factors. Other factors that affect simple decisions also assert themselves in the negotiation between evidence and bound. These include valuation of – or relative weight assigned to – (i) potential rewards and punishments associated with success and failure (for reviews, see Sugrue et al., 2005; Padoa-Schioppa, 2011), (ii) prior knowledge in the absence of new evidence about which of the alternatives is likely to be correct (Hanks et al., 2011), (iii) social and emotional factors, and (iv) the passage of time itself. Elapsed time is associated with opportunity costs and alters the value of an expected reward. There may also be a deadline to complete a decision by a certain point in time. Interestingly, the neurons that encode accumulated evidence in the motion task also encode elapsed time (Leon and Shadlen, 2003; Janssen and Shadlen, 2005; Maimon and Assad, 2006) in a way that incorporates the sense of urgency in the decision process (Drugowitsch et al., 2012). We suggest that increased attention to these elements, and their role in decision-making will provide insight into the active role of the agent in shaping decision processes.
Noise
The picture we have painted thus far captures some of the important neurobiological determinants of decisions, but an important aspect has been left out. That is the issue of noise.
The mechanisms outlined so far are causal mechanisms, and as such one might think that these mechanisms will always evolve in the same way under the same circumstances. However, the neurons that represent evidence – whether from vision (Britten et al., 1992) or via associations of cues with their bearing on a proposition (Yang and Shadlen, 2007) – do so in a “noisy” way. These neurons do not convey the same number of action potentials per unit time even when they are exposed to the identical condition over and over (at least as identical as can be tested in the laboratory). There is nothing magical about this noise, although the source of noise remains unknown, as does whether it reflects fundamentally deterministic or indeterministic processes. As far as we understand, the existence of noise does not confer any special properties, like freedom, will, consciousness, etc. However, the noise does have very real effects. For example, errors in perceptual decisions can be traced to the variable discharge of cortical neurons (Parker and Newsome, 1998). There are two ways to think that noise might bear upon our understanding of freedom and responsibility. The first concerns the source of noise, and the second its effects.
What is the source of noise?
The origins of noise in the neocortex are probably in the complexity of synaptic integration with large numbers of excitatory and inhibitory inputs (Shadlen and Newsome, 1994, 1998; van Vreeswijk and Sompolinsky, 1996).
The representation of information by neurons is affected by noise. Moreover, this noise is an ineliminable aspect of brain function. Even in the parts of the brain that are reasonably well understood, such as the visual cortex, when the exact same stimulus is presented in a highly controlled setting, a neuron might emit 10 spikes on one exposure, 6 on the next, 17 on the next, and so on. In the neocortex, if the mean spike rate is 10 spikes in some epoch (say 1/4 s), then variance is typically about 15. The square root of this number, the SD, is just under 4. Roughly then, we might characterize the count as a random number that tends to be near 10 but falls between 2 and 18 (±2 SDs of 10) with 95% probability. That is a very large range of variability.
Of course, there are many neurons in any patch of cortex. So the brain can achieve an improvement in this variability by averaging the spikes from many neurons. However, there is a limit to this improvement because the neurons are weakly correlated, and thus share some variability. It has been shown that the improvement in signal to noise can only be reduced by a factor of about 3 (Zohary et al., 1994; Shadlen and Newsome, 1998; Mazurek and Shadlen, 2002). This is one of the reasons that neuroscientists can record activity from single neurons and find them so informative about what an entire neural population, and even an animal, senses, decides, and does.
Although it is commonly said that neurons compute with spikes, this truism obscures a deeper truth about the currency of information exchange the cortex. Cortical neurons compute with spike rates. They access information from other neurons even in the temporal gaps between the spikes of any one neuron that contributes information to a computation. In many subcortical structures and in many simpler nervous systems, a neuron emits a spike if only one (or a few) of its inputs are active. The inputs are simple or relatively sparse, and these neurons effectively pass on the action potentials from those inputs. In contrast, neurons in the cortex compute new information by combining quantities potentially representing many different things: position of a stimulus in the left eye’s view compared to the right or whether a quantity x is greater than another quantity y and if so, by how much. The numbers that are to be added, subtracted, and compared are not just all or none. They are intensities: contrast, level of evidence, etc. For the new computation to occur, it would be inefficient for a neuron to wait through the period between spikes arriving from the various inputs that represent, for example, x and y. Instead, the circuit establishes a representation of x and y that is present through the interspike interval of any one neuron.
To achieve this, many neurons represent x and y. That way, in a very narrow time window (e.g., ∼1/100 s) the neuron that is doing the comparison gets a sample of the intensities of x and y, as represented by many neurons. This calculating neuron gets to know x by averaging the spikes and silences across neurons instead of averaging the spikes (and silences) from one neuron across time. That makes for a fast cortex that can compute new things. But it poses a problem. We know that it takes only ∼10–20 excitatory inputs in a few ms epoch to make a neuron fire. If we think about the number of inputs that are needed to achieve the computations in question – that is to permit calculations with numbers ranging from 10 to 100 spikes per second, it turns out we need on order 100 neurons representing x and another 100 that represent y. That would lead to far too many resultant spikes – there would be a surfeit of excitation. Neurons would not be able to maintain a graded range of responses: they would quickly saturate their firing rates.
To counter this, the cortex balances excitation with inhibition (Shadlen and Newsome, 1994). In cortex, when a neuron is driven to discharge at a higher rate, both the rate of its excitatory input and inhibitory input increase. In fact, we think there is a delicate balance that allows this to work. It controls the dynamic range of firing. Now the spiking occurs when the neuron has accumulated an excess of excitation compared to inhibition. But since both are occurring, the effect is like a particle in Brownian motion. The neuron’s state (e.g., membrane voltage) wanders until it bumps into a positive threshold and produces a spike. The net effect is a preservation of dynamic range among inputs and outputs, but the cost is irregularity. The spikes occur when the random path (called a random walk) of voltage happens to bump into a threshold. That is an irregular process. In fact it explains the high irregularity that one typically observes when recording from cortical neurons. It explains the variance of the spikes counted in an epoch. That irregularity also results in asynchronous spiking. That means another neuron will not be fooled into “thinking” that spike rate has increased because spikes from several neurons arrive all at the same time.
There are a number of interesting implications of this mechanism, but the one we wish to emphasize concerns the relationship between inputs and outputs. There is an important intuition that one ought to have about diffusion and random walks. It is that the state variable that undergoes the walk – what we are thinking of here as membrane voltage – tends to meander from its starting position by a distance given roughly by the square root of the number of steps it has taken multiplied by the size of a unitary step. Suppose that the amount of depolarization required to generate a spike is equivalent to 20 excitatory steps. Then for the random walk, we would expect it to take 400 steps (half excitatory and half inhibitory) for the membrane voltage to meander this far from its starting point, on average. And, approximately half the time the displacement is in the wrong direction, away from spike threshold. This intuition allows us to appreciate why a balance of excitation and inhibition allows a cortical neuron to operate in a regime in which it is bombarded with many inputs from other neurons. The random walk achieves a kind of compression in number of input events to output events.
So why are neurons noisy? It is an inescapable price the neocortex pays for its ability to combine and manipulate information. To perform their computations, cortical neurons receive many excitatory inputs from other neurons, and they must balance this excitation with inhibition (balanced E/I). Balanced E/I leads to the variable discharge that is observed in electrode recordings.
The effects of noise
The fact that this variability exists is not controversial, although its implications are often debated (Glimcher, 2005; Faisal et al., 2008). One particularly relevant fact that is not disputed is that this variability is a source of errors in simple decisions. Noise limits perceptual sensitivity and motor precision. This fact makes one very suspicious of claims that the variability is just due to causes that the experimenter has not controlled for (or cannot control; e.g., variation in motivational state). That would be a valid concern were it not for the fact that the rest of the brain also does not seem to know that this variability is not part of the signals it uses for subsequent computation and behavior. Were the causes of variability in sensory evidence known to the rest of the brain, that variability would not induce errors. Downstream structures would know that the 17 spikes it received was anomalous and that the real signal had magnitude 10.
This leads to another important point. Consider the time that a spike occurs. In actuality, it was preceded by a particular path that the membrane voltage took before the voltage threshold for the spike was attained. This path reflects detailed information about when the input spikes (excitatory and inhibitory) occurred. But, because of the presence of noise and the random walk of the membrane voltage, there are many paths that could lead to the identical spike time and many more that could have led to a range of spike times that would be indistinguishable from the point of view of downstream neurons. The detailed information about the path that led to a particular spike is lost. Downstream neurons see only the outcome – the spike. They are not privy to the particular trajectory of membrane voltage that led to this spike. Thus, downstream neurons do not “know” the exact cause of the inputs impinging upon them, nor can they reconstruct this from the data available to them. They cannot differentiate signal from noise, and any computational characterization of the processes they support must incorporate ineliminable probabilistic features.
This observation has implications for neural coding. For example, it renders implausible a baroque code of information in spatial and temporal patterns of spikes. That is not to say that which neurons are active, and when, is not the code of information. Perhaps, the fine detail of the spike pattern across the population of neurons – like a constellation of flickering stars – conveys information. However, the details of the spike patterns in time – the time sequence of the flickering – are removed from the neural record. They are represented in the particular trajectories that the receiving neurons’ membrane voltages undergo between their spikes and are thus lost in transmission. Other neurons in the brain do not benefit from this information.
This observation also has important philosophical implications. It implies a fundamental epistemic break in the flow of information. From the effect, i.e. the spikes of some set of output neurons, the system cannot reconstruct its causes (the times of all the inputs). This means that the variability on the outputs cannot be predictively accommodated. If in some epoch a neuron emits five spikes instead of four, it is often impossible for the brain to trace this difference to an event in inputs that would lead another neuron to discount this extra spike as anomalous. Although the variability can emerge from deterministic processes (no quantum effects) it should be viewed as fundamental, because there is no way to trace it to its source or to negate it.
Noise is at least in part a result of complexity at the synaptic level, a manifestation of a chaotic mechanism that balances excitation and inhibition (Shadlen and Newsome, 1994, 1998; van Vreeswijk and Sompolinsky, 1996). Indeterministic or chaotic neural activity has been postulated by some philosophers to make possible free will (Kane, 2002). Some people (including one of the authors, Michael N. Shadlen) might be inclined to think that noise in the nervous system shows determinism to be false (Glimcher, 2005). However, without being able to identify the source of noise, we cannot attribute it, with certainty, either to indeterministic brain events, such as effects of quantum indeterminacy, or to complex but deterministic processes. And if determinism is true, the spikes produced at some level of neural organization are completely caused by prior physical events, and their precise timing can in principle be accounted for in its entirety. For example, the firing pattern of neurons that represent momentary evidence are completely caused by the impulses from other neurons. However, as argued above, this precise timing does not convey information, nor can it be exploited for prediction. So we are wise to look at spike rates as a random value with an expectation (or central tendency) and uncertainty.
We have already noted that this variability has an effect on behavior, namely on the accuracy and speed of decisions. So it is a quantity that we ought to care about. Yet, it is useless to try to account for it by tracing it to more elementary causes. The variability might as well have arisen de novo at the level we measure it. Thus, despite the fact that the system may be deterministic in the physical sense, it cannot be understood properly in terms of only its prior causes. This is arguably an example of emergence, a principle that applies to many macroscopic properties in biology (Anderson, 1972; Mayr, 2004; Gazzaniga, 2011).
The presence of noise implies that there is some uncertainty involved in every calculation the brain makes. Thus, even if we know conditions in the world, we cannot be sure about what outcome they will cause via the workings of a brain that must make a decision, because it is not clear, even to the brain, exactly what state it is in. Because the brain operates on noisy data with noisy mechanisms, it must enact strategies or policies to control accuracy. For example it must balance the speed of its decisions against a targeted accuracy. Such policies underlie distinctions that separate one decision maker from another, and we will argue that they are relevant to assessments of free will and responsibility. We explicitly deny that the brain or the agent can (always) identify noise as distinct from signal. However, through experience the agent can tell that he does not always track the world correctly, or that his decisions are not the right ones. He thus must learn to modulate his decisions in order to compensate for uncertainty, where that uncertainty is generated (at least in part) by noise. For example, a high error rate might induce the agent to change policy by slowing down. Neither the agent nor the brain need know about the noise, but by changing the bound height, the brain (and agent) would reduce the error rate.
Responsibility, Policy, and Where the Buck Stops
On most moral views, capacities, attitudes, and policies are relevant to assessments of ethical responsibility (e.g., Strawson, 1974; Wallace, 1998; Smith, 2003). Capacities set broad outlines for domains of possibility for the engagement of certain functions important for social agency. Some, such as basic abilities to comprehend facts, make valuations, and control impulses may be necessary conditions for responsible agency, whereas consideration of others, such as perceptual acuity, memory, attentional control, and mentalizing abilities may modulate responsibility judgments. Attitudes or policies such as explicit beliefs about moral obligations, risk-aversiveness, and in/out-group attitudes may affect decision-making in ways that we consider subject to moral assessment4. The neuroscience of motivation and social behavior is beginning to shed some light on the neuroscience of social attitudes, but at this point only in broad-brush ways that do not yet illuminate mechanism. Policies are high-level heuristics that affect the parameters of decision-making and can be modulated in a context-dependent way. These include the relative weighting of speed versus accuracy, the relative weighting given to different types of information, and the cost assigned to different degree of expected error. Some of these elements are formalized mathematically in decision theory (Jaynes, 2003). Our focus here will be on policies.
In Section “Neurobiology of Decision-Making,” we suggested that neuroscience is beginning to expose the brain mechanisms that establish at least some such policies. The speed–accuracy tradeoff is a paradigmatic example. We focus here on the tradeoff between speed and accuracy because it is something we are beginning to understand (Palmer et al., 2005; Hanks et al., 2009). Neural mechanisms responsible for other decision policies are probably not far behind. In principle, the same kinds of mechanisms that operate on perceptual decisions are probably at play in social decisions (Deaner et al., 2005), economic decisions involving relative value (Glimcher, 2003; Sugrue et al., 2005; Lee, 2006), and decisions about what (and whether) to engage – deciding what to decide about (Shadlen and Kiani, 2007, 2011).
That policies can have a role in the assessment of responsibility is plain. Policies are malleable, context-dependent, and pervasive. Consider the following outcomes due to decisions made by two doctors. Doctor A made a hasty, inaccurate diagnosis of her patient because she valued speed over accuracy. Doctor B, valuing accuracy more than speed, made a correct diagnosis, and saved the patient. Doctor C, also valuing accuracy over speed, failed to act in time to stanch the bleeding of his patient. Decisions cannot be explained in the absence of considerations of policy, and the suitability of policies must be tuned to circumstances. These policies are center stage in our consideration of the qualities of these three doctors’ decisions. It is not the policy itself, but the application of the policy in particular circumstances that is important: That is why our moral assessments of Doctor’s B and C differ, even though they have the same policy. On the other hand, even if Doctor B had not saved the patient due to chance factors she could not control, we would have no grounds for moral sanction. Thus, it is the policy, not just the outcome, that is relevant to moral assessment.
Recent work indicates that policy elements of decision-making are beginning to be explicable in neural terms. Importantly, the elucidation of the neural mechanism that gives rise to policy does not explain the policy away, nor does it make it less relevant to ethical assessment. Policies may be chosen poorly, but they are also revisable. So over time, policies should better track what they must accomplish. Agents can be morally assessed for failing to revise. Indeed setting a policy often requires decisions (as well as learning and other factors). The process might also be subject to noise and uncertainty, but again, the noise neither confers freedom nor lack of responsibility; it invites consideration of policy affecting decisions about policy. These policies are also targets for moral assessment. For example, Doctor A in the story, who made a hasty, inaccurate diagnosis of her patient because she valued speed over accuracy, might ask us to excuse her action on the grounds that her policy, favoring speed, was merely the outcome of noise. The argument concerns policy and thus has bearing on our evaluation, but it is not compelling, because one would counter (in effect) that training in medicine should lead to non-volatile policies, which are resilient to noise, emotional factors, distraction, and sleep deprivation. However, were Doctor A poisoned by a drug (or disease process) that affected the bound-setting mechanism, we might be inclined to accept this fact as mitigating.
The important insight is that the neurobiology is relevant in the sense that it points us toward the consideration of policy in our moral assessments. We may not have direct access to the internal policy in the way we can observe an act, but we can infer settings like bound height from behavioral observations, just as we can infer accuracy. We also have direct access to the agent’s communications about these policies. Although indirect and possibly non-veridical, they are expressions of metacognitive states – analogous to confidence – concerning a decision. Thus, when we engage in ethical evaluation of a decision or act, policy is one natural place to focus our inquiry.
To recap, we have argued that ineliminable noise in neural systems requires the agent to make certain kinds of commitments in order to make decisions, and these commitments can be thought of as the establishment of policies. Noise puts a limit on an agent’s capacities and control, but invites the agent to compensate for these limitations by high-level decisions or policies that may be (a) consciously accessible; (b) voluntarily malleable; and (c) indicative of character. Any or all these elements may play a role in moral assessment. It remains to be seen how such information about policy might bear upon our view of free will and responsibility. The answer will depend in part on what one’s basic views on free will and responsibility are. It will also depend on whether the arguments about noise are taken to illustrate a purely epistemic limitation about what we know about the causes of our behavior, or whether one can muster arguments to the effect that a fundamental epistemic limitation brings with it metaphysical consequences.
One of us (Adina L. Roskies), thinks that policy decisions are a higher-level form of decision that establishes parameters for first-order decisions, and that to the extent that policies are set consciously or deliberately, or are subject to feedback from learning, policy decisions should be considered significant in attributions of responsibility, and the ability of the agent to manipulate them as important in attributions of free will. It is possible that policy decisions should be considered significant in attributions of responsibility even when they are set without conscious deliberation. Another of us (Michael N. Shadlen), agrees with the above, and in addition holds that the special status of policies is also a consequence of their emergence as entities orphaned from the chain of cause and effect that led to their implementation in neural machinery. This will be explained further in the next section.
Does the fact that we cannot know the precise neural causes of some effect mean that we can conclude that they are in some relevant sense undetermined? If one rejects the notion that the unpredictability of noise entitles one to take the noise as fundamentally equivalent to indeterminacy – because the limitations are only epistemic in character – the noise argument cannot be used to argue for the falsity of determinism and the consequent falsity of positions tied to the truth of determinism. Thus, the focus on policies does little to address the worries of the hard incompatibilist.
However, those with compatibilist leanings might think like this: As a compatibilist, your concerns are not with the truth of determinism or indeterminism, or even with predictability. Instead, you think that capacities and other properties of agents are the criteria upon which to establish responsibility. For example, if you think that responsibility judgments are relativized to the information available to an agent, then noise, whether deterministic or indeterministic, puts limits on perfect information and forces the agent to make policy decisions based on prior experience. This is just an augmentation of the imperfect information or uncertainty that we already take to exist in decision-making. If one accepts that mechanism need not undermine mindedness, then we can examine whether policies are based on conscious decisions/intentions, and whether agents can be held accountable for how policies are set. The plasticity of this system will undoubtedly be an important aspect of responsibility. Notice that this same reasoning can be applied to decisions themselves (or, perhaps only decisions for reasons that the agent is aware of), so it is not clear we get anywhere with traditional philosophical problems, but it does point to an aspect relevant to moral assessment that is often overlooked, and for which we have some insight from neuroscience. The important point the science gives us is that the policies are necessitated not by indeterminism but by noise, an established physical fact that all sides can agree on. This makes the traditional debate about determinism/indeterminism moot, and instead puts emphasis on the importance of capacities and how they ground responsibility. Moreover, if one thinks that the information available to the agent is an important factor to weigh in assessments of responsibility, the recognition of noise puts important limits on even ideal measures of that quantity.
Suppose, on the other hand, you argue that the ineliminability of noise, and the information loss it results in, provides a basis for a belief in indeterminism5. You may try to leverage an argument that will be persuasive to the scientist who is tempted by incompatibilism, but one who is worried about scientific reductionism rather than determinism, and thus worried that neuroscience will explain away agency.
Here would be a sketch of such an argument: Brain states including those that underlie the establishment and implementation of high-level policies in decision-making possess low-level explanations and causes. However, due to the information loss that noise engenders, there is a fundamental limitation to the kinds of reductionist accounts that will be available. The inability to offer a reductionist explanation is an epistemic limitation, but one could argue that the due to noise, high-level brain states or processes, including the policies that are developed to deal with noise, represent a form of emergence.
The argument for emergence is analogous to one that the evolutionary biologist, Ernst Mayr, made regarding species. In principle, we can trace the sequence of events leading to the evolution of zebras, for example, from early vertebrates, but we do not recognize this causal accounting as being fully explanatory. The chain of cause and effect in evolution – here the path of evolution from early vertebrates – could have diverged vastly differently from the one we can piece together in retrospect. This vastness of possibility follows from the mechanism of evolution, and in brief, this degree of divergence necessitates that we cannot explain the zebra’s status as an entity equivalent to “early vertebrates plus the mechanism of evolution,” since there are multiple ways evolution might have gone. Thus we postulate the ontological independence of the zebra in our biological theories. A similar argument can be made with respect to causal processes in the brain that lead to the establishment of policy. Emergence does not contradict the fact that a chain of cause and effect led to a brain state. But it does imply that we cannot explain a behaviorally relevant neural state solely in terms of its causal history; too much of the entire causal history would be needed to account for the final state. And because in human interaction we need to explain behaviors, and explaining behavior is important for assessments of moral responsibility, we have to stop trying to trace back causal chains beyond the noise, and focus on higher-level regularities such as policy decisions. Thus, if you object to freedom and responsibility, not because of the presence of causal chains but because of eliminativist worries that mechanism precludes responsibility, we urge you to consider the following: First, recognize that policies are real and ineliminable aspects of decision-making, necessitated by the limited information available to neural systems. Second, take these high-level policy decisions as a basis for responsibility assessments.
In other words, if noise in the brain arises from a mechanism that is analogous to the emergence phenomenon in evolution it might imbue brain states with the same type of status that a species has in evolution – an ontologically real entity. If this were to hold for policy, then an incompatibilist might be nudged toward explanations of decisions that recognize irreducible elements in the brain of the decision maker, elements that cannot be explained away on the basis of prior causes. These elements can provide a basis for accountability and responsibility that focuses on the agent, rather than on prior causes.
That said, if you are a hard incompatibilist, and reject freedom and responsibility on the grounds that neither determinism nor indeterminism can support freedom, the foregoing argument for freedom and responsibility may not move you at all, for policies themselves have a causal basis, and the same arguments that block responsibility in the first-order case will also block it in the case of higher order policies.
Final Remarks
We have attempted to account for the role of noise in decision-making, based on an understanding of the underlying science. Our philosophical conclusions are modest. For example, we do not say much here explicitly about freedom, although we think the points made here will prove relevant to considerations of freedom in the neural context. We have, however, argued that:
(1) Neural noise is relevant to the understanding of agency, free will, and moral responsibility.
(2) Noise is relevant to these questions for its effects on decision-making, not because it addresses the question of determinism/indeterminism.
(3) Policies, and related ways of managing noise and uncertainty, are appropriate objects of moral consideration. This is true whether you think of them as resulting from or exemplifying relevant capacities of an agent, or whether you argue that policies are emergent properties of agency.
This argument might have further implications for understanding and investigating conditions for moral responsibility, such as:
(1) Investigations of policy mechanisms and their relation to higher-level control should be a matter of priority for decision researchers. How policies are set, assessed, and revised are important elements for a neuroscientific theory of agency and a compatibilist theory of responsibility.
(2) The importance of policies suggests that diseases that affect policy mechanisms should be thought of as having particular bearing upon moral responsibility.
(3) Although we have not explicitly argued for this here, it is plausible to think that agents might be held morally responsible even for decisions that are not conscious, if those decisions are due to policy settings which are expressions of the agent.
(4) If this is true, it puts pressure on the argument that agents act for automatic and not conscious reasons (Sie and Wouters, 2008, 2010), and thus cannot be held responsible for their actions. If agents can be held responsible for policies that in some sense determine decisions, they can be held responsible for those decisions, even if they do not have access to the reasons for those decisions.
Summary
Recent advances in neurobiology have exposed brain mechanisms that underlie simple forms of decisions. Up until now, the role played by noise in decision-systems has not been considered in detail. We have argued that the science suggests that noise does not bear on the formulation of the problem of free will in terms of determinism as traditionally thought, but rather that it shifts the focus of the debate to higher-level processes we call “policies”. Our argument is compatibilist in spirit. It implies that NBDM does not threaten belief in freedom because it discloses the causes of action. Rather, NBDM sheds light on the mechanisms that might lead an agent to make one choice in circumstances that might lead another, even very similar agent to choose differently. We have not appealed to randomness or noise as a source of freedom, but rather recognize that such randomness establishes the background against which policies have to be adopted, for example, for trading speed against accuracy. We thus offer a glimpse of an aspect of compatibilism that does not address the compatibility of freedom with determinism per se, but instead addresses the compatibilism of responsibility with neurobiology and mechanism. By showing how choices are made, the neurobiology does not dismiss choice as illusory, but highlight’s the agent’s capacity to choose.
Conflict of Interest Statement
The authors declare that the research was conducted in the absence of any commercial or financial relationships that could be construed as a potential conflict of interest.
Acknowledgments
Michael N. Shadlen thanks Larry Abbott, Simon Blackburn, Dan Braun, Helen Brew, Patricia and Anne Churchland, Roozbeh Kiani, Josh Gold, Tim Hanks, Gerald Shadlen, S. Shushruth, Xiao-Jing Wang, and Daniel Wolpert for helpful discussions and comments on an earlier draft of this manuscript, and acknowledges support from HHMI, NEI, NIDA and a Visiting Fellow Commoner Fellowship from Trinity College, University of Cambridge, UK. Adina L. Roskies thanks the Princeton University Center for Human Values and the Big Questions in Free Will Project, funded by the John Templeton Foundation for support. The opinions expressed in this article are our own and do not necessarily reflect the views of the John Templeton Foundation.
Footnotes
- ^Hard determinism is the position that (a) freedom is incompatible with determinism, and (b) determinism is true. Many hard determinist arguments are based on the inexorability of causal chains and the resultant lack of ability to do otherwise. For more definitions of technical terms, see Box 1.
- ^A highly intuitive discussion of emergence can be found in Gazzaniga’s (2011) recent book. The concept of emergence is a matter of ongoing debate in philosophy (e.g. see Kim, 2010 for discussion). Our arguments do not depend on a metaphysically demanding notion of emergence, but rather on a weak notion of emergence that prevents radical eliminativism of high-level properties.
- ^There is considerable philosophical dispute about in what sense (ii) must be true, and some have argued that it is not essential, or that it can be true even if there is only one way the world can evolve (Frankfurt, 1971; Dennett, 1984; Vihvelin, 2012).
- ^It is not clear how to distinguish attitudes and policies. We refer to them as if they are different, but it could just be that we have the beginning of an understanding of the neural basis of policies and how they affect decision-making, but so far no real handle on the neural realization of things we consider attitudes.
- ^One of us (Michael N. Shadlen) believes this is the case: the limitation imposed by noise is not merely epistemic; it represents indeterminacy that is fundamental. This is because the complexity of the brain magnifies exponentially the finite variation in initial state. This finite variation is a property of nature, not a consequence of measurement imprecision. It is the notion of infinite precision that is fictional (invented for the calculus). This variation leads to exponential divergence of state in chaotic (deterministic) processes. Because this variation cannot be traced back in time to its original causes, chaos supports metaphysical (as well as epistemic) indeterminacy.
References
Andersen, R. A. (1995). Encoding of intention and spatial location in the posterior parietal cortex. Cereb. Cortex 5, 457–469.
Bogacz, R., Brown, E., Moehlis, J., Holmes, P., and Cohen, J. D. (2006). The physics of optimal decision making: a formal analysis of models of performance in two-alternative forced-choice tasks. Psychol. Rev. 113, 700–765.
Born, R. T., and Bradley, D. C. (2005). Structure and function of visual area MT. Annu. Rev. Neurosci. 28, 157–189.
Britten, K. H., Shadlen, M. N., Newsome, W. T., and Movshon, J. A. (1992). The analysis of visual motion: a comparison of neuronal and psychophysical performance. J. Neurosci. 12, 4745–4765.
Colby, C. L., and Goldberg, M. E. (1999). Space and attention in parietal cortex. Annu. Rev. Neurosci. 22, 319–349.
Crick, F. (1994). The Astonishing Hypothesis: The Scientific Search for the Soul. New York: Charles Scribner’s Sons.
Deaner, R. O., Khera, A. V., and Platt, M. L. (2005). Monkeys pay per view: adaptive valuation of social images by rhesus macaques. Curr. Biol. 15, 543–548.
Ditterich, J., Mazurek, M., and Shadlen, M. N. (2003). Microstimulation of visual cortex affects the speed of perceptual decisions. Nat. Neurosci. 6, 891–898.
Drugowitsch, J., Moreno-Bote, R., Churchland, A. K., Shadlen, M. N., and Pouget, A. (2012). The cost of accumulating evidence in perceptual decision making. J. Neurosci. 32, 3612–3628.
Faisal, A. A., Selen, L. P., and Wolpert, D. M. (2008). Noise in the nervous system. Nat. Rev. Neurosci. 9, 292–303.
Glimcher, P. (2003). Decisions, Uncertainty, and the Brain: The Science of Neuroeconomics. Cambridge, MA: MIT Press.
Gnadt, J. W., and Andersen, R. A. (1988). Memory related motor planning activity in posterior parietal cortex of monkey. Exp. Brain Res. 70, 216–220.
Gold, J. I., and Shadlen, M. N. (2002). Banburismus and the brain: decoding the relationship between sensory stimuli, decisions, and reward. Neuron 36, 299–308.
Gold, J. I., and Shadlen, M. N. (2007). The neural basis of decision making. Annu. Rev. Neurosci. 30, 535–574.
Good, I. J. (1979). Studies in the history of probability and statistics. XXXVII A.M. Turing’s statistical work in World War II. Biometrika 66, 393–396.
Greene, J., and Cohen, J. (2004). For the law, neuroscience changes nothing and everything. Philos. Trans. R. Soc. Lond. B Biol. Sci. 359, 1775–1785.
Hanks, T. D., Ditterich, J., and Shadlen, M. N. (2006). Microstimulation of macaque area LIP affects decision-making in a motion discrimination task. Nat. Neurosci. 9, 682–689.
Hanks, T. D., Kiani, R., and Shadlen, M. N. (2009) “A neural correlate of the tradeoff between the speed and accuracy of a decision,” in Neuroscience Meeting Planner (Chicago, IL: Society for Neuroscience).
Hanks, T. D., Mazurek, M. E., Kiani, R., Hopp, E., and Shadlen, M. N. (2011). Elapsed decision time affects the weighting of prior probability in a perceptual decision task. J. Neurosci. 31, 6339–6352.
Huk, A. C., and Shadlen, M. N. (2005). Neural activity in macaque parietal cortex reflects temporal integration of visual motion signals during perceptual decision making. J. Neurosci. 25, 10420–10436.
Janssen, P., and Shadlen, M. N. (2005). A representation of the hazard rate of elapsed time in macaque area LIP. Nat. Neurosci. 8, 234–241.
Jaynes, E. T. (2003). Probability Theory: The Logic of Science. Cambridge: Cambridge University Press.
Kane, R. (2002). “Free will: new directions for an ancient problem,” in Free Will, ed. R. Kane (Oxford: Blackwell), 222–246.
Leon, M. I., and Shadlen, M. N. (2003). Representation of time by neurons in the posterior parietal cortex of the macaque. Neuron 38, 317–327.
Lewis, J. W., and Van Essen, D. C. (2000). Corticocortical connections of visual, sensorimotor, and multimodal processing areas in the parietal lobe of the macaque monkey. J. Comp. Neurol. 428, 112–137.
Link, S. W. (1992). The Wave Theory of Difference and Similarity. Hillsdale, NJ: Lawrence Erlbaum Associates.
Lo, C. C., and Wang, X. J. (2006). Cortico-basal ganglia circuit mechanism for a decision threshold in reaction time tasks. Nat. Neurosci. 9, 956–963.
Maimon, G., and Assad, J. A. (2006). A cognitive signal for the proactive timing of action in macaque LIP. Nat. Neurosci. 9, 948–955.
Mayr, E. (2004). What Makes Biology Unique? Considerations on the Autonomy of a Scientific Discipline. Cambridge: Cambridge University Press.
Mazurek, M. E., and Shadlen, M. N. (2002). Limits to the temporal fidelity of cortical spike rate signals. Nat. Neurosci. 5, 463–471.
Mazzoni, P., Bracewell, R. M., Barash, S., and Andersen, R. A. (1996). Motor intention activity in the macaque’s lateral intraparietal area. I. Dissociation of motor plan from sensory mechanisms and behavioral modulations. J. Neurophysiol. 76, 1439–1456.
Padoa-Schioppa, C. (2011). Neurobiology of economic choice: a good-based model. Annu. Rev. Neurosci. 34, 333–359.
Palmer, J., Huk, A. C., and Shadlen, M. N. (2005). The effect of stimulus strength on the speed and accuracy of a perceptual decision. J. Vis. 5, 376–404.
Parker, A. J., and Newsome, W. T. (1998). Sense and the single neuron: probing the physiology of perception. Annu. Rev. Neurosci. 21, 227–277.
Ratcliff, R., and Rouder, J. N. (2000). A diffusion model account of masking in two-choice letter identification. J. Exp. Psychol. Hum. Percept. Perform. 26, 127–140.
Resulaj, A., Kiani, R., Wolpert, D. M., and Shadlen, M. N. (2009). Changes of mind in decision-making. Nature 461, 263–266.
Shadlen, M. N., and Kiani, R. (2011). “Consciousness as a decision to engage,” in Characterizing Consciousness: From Cognition to the Clinic? Research and Perspectives in Neurosciences, eds S. Dehaene, and Y. Christen (Berlin: Springer-Verlag), 27–46.
Shadlen, M. N., and Newsome, W. T. (1994). Noise, neural codes and cortical organization. Curr. Opin. Neurobiol. 4, 569–579.
Shadlen, M. N., and Newsome, W. T. (1998). The variable discharge of cortical neurons: implications for connectivity, computation and information coding. J. Neurosci. 18, 3870–3896.
Sie, M., and Wouters, A. (2008). The real challenge to free will and responsibility. Trends Cogn. Sci. (Regul. Ed.) 12, 3–4; author reply 4.
Sie, M., and Wouters, A. (2010). The BCN challenge to compatibilist free will and personal responsibility. Neuroethics 3, 121–133.
Smith, M. (2003). “Rational capacities, or: how to distinguish recklessness, weakness, and compulsion,” in Weakness of Will and Practical Irrationality, eds S. Stroud, and C. Tappolet (New York: Clarendon Press), 17–38.
Sugrue, L. P., Corrado, G. S., and Newsome, W. T. (2005). Choosing the greater of two goods: neural currencies for valuation and decision making. Nat. Rev. Neurosci. 6, 363–375.
Usher, M., and McClelland, J. L. (2001). The time course of perceptual choice: the leaky, competing accumulator model. Psychol. Rev. 108, 550–592.
van Vreeswijk, C., and Sompolinsky, H. (1996). Chaos in neuronal networks with balanced excitatory and inhibitory activity. Science 274, 1724–1726.
Vihvelin, K. (2012). Causes, Laws, and FreeWill: An Essay on the Determinism Problem. Oxford: Oxford University Press.
Williams, B. A. O. (1985). Ethics and the Limits of Philosophy. Cambridge, MA: Harvard University Press.
Wong, K. F., Huk, A. C., Shadlen, M. N., and Wang, X. J. (2007). Neural circuit dynamics underlying accumulation of time-varying evidence during decision-making. Front. Comput. Neurosci. 1:6. doi: 10.3389/neuro.10.006.2007
Keywords: free will, responsibility, lateral intraparietal area, motion perception, compatibilism, determinism, noise, policy
Citation: Shadlen MN and Roskies AL (2012) The neurobiology of decision-making and responsibility: Reconciling mechanism and mindedness. Front. Neurosci. 6:56. doi: 10.3389/fnins.2012.00056
Received: 07 February 2012; Accepted: 30 March 2012;
Published online: 23 April 2012.
Edited by:
Carlos Eduardo De Sousa, Northern Rio de Janeiro State University, BrazilReviewed by:
Dario L. Ringach, University of California Los Angeles, USACarlos Eduardo De Sousa, Northern Rio de Janeiro State University, Brazil
Copyright: © 2012 Shadlen and Roskies. This is an open-access article distributed under the terms of the Creative Commons Attribution Non Commercial License, which permits non-commercial use, distribution, and reproduction in other forums, provided the original authors and source are credited.
*Correspondence: Michael N. Shadlen, Department of Physiology and Biophysics, Howard Hughes Medical Institute, University of Washington, Seattle, WA 98195-7290, USA. e-mail:c2hhZGxlbkB1dy5lZHU=