- Department for Psychology I, Institute for Psychology, University of Würzburg, Würzburg, Germany
Brain–computer interfaces (BCI) based on event-related potentials (ERP) allow for selection of characters from a visually presented character-matrix and thus provide a communication channel for users with neurodegenerative disease. Although they have been topic of research for more than 20 years and were multiply proven to be a reliable communication method, BCIs are almost exclusively used in experimental settings, handled by qualified experts. This study investigates if ERP–BCIs can be handled independently by laymen without expert support, which is inevitable for establishing BCIs in end-user’s daily life situations. Furthermore we compared the classic character-by-character text entry against a predictive text entry (PTE) that directly incorporates predictive text into the character-matrix. N = 19 BCI novices handled a user-centered ERP–BCI application on their own without expert support. The software individually adjusted classifier weights and control parameters in the background, invisible to the user (auto-calibration). All participants were able to operate the software on their own and to twice correctly spell a sentence with the auto-calibrated classifier (once with PTE, once without). Our PTE increased spelling speed and, importantly, did not reduce accuracy. In sum, this study demonstrates feasibility of auto-calibrating ERP–BCI use, independently by laymen and the strong benefit of integrating predictive text directly into the character-matrix.
Introduction
Event-related potentials (ERP) are brain signals in the human electroencephalogram (EEG) that occur in response to stimuli, e.g., visual stimuli (for review, see Polich, 2007). Farwell and Donchin (1988) suggested to utilize these potentials for brain–computer interface (BCI) based communication, particularly dedicated to patients with impaired motor control due to neurodegenerative disease (e.g., patients suffering from amyotrophic lateral sclerosis, ALS). Such ERP–BCI is often referred to as P300-BCI due to its usual dependence on the P300, a prominent positive ERP approximately 200–400 ms post-stimulus (Picton, 1992; Polich et al., 1997). It is elicited in an oddball-paradigm, in which participants focus their attention on a rare stimulus (the odd stimulus) among many frequent but irrelevant stimuli. In the visual ERP–BCI this oddball is typically realized by presenting letters and numbers of the alphabet on a computer screen and flashing these characters randomly. Users are then asked to focus their attention on flashings of the character they intend to spell while ignoring all other characters (e.g., counting the target flashes). After a certain number of character flashings, a classification algorithm detects ERPs in the recorded signal and thereof determines the intended character. Hence, healthy as well as severely impaired users can communicate words on a character-by-character basis (e.g., Nijboer et al., 2008).
Today, visual ERP–BCIs are most commonly used systems for BCI-based communication (for a review, see Kleih et al., 2011). It has been shown that they are reliably accurate (in healthy participants, e.g., Guger et al., 2009; Kleih et al., 2010; in patients, e.g., Sellers et al., 2006, 2010; Townsend et al., 2010) and robust for long-term use (Nijboer et al., 2008; Sellers et al., 2010). However, when considering daily use of a visual ERP–BCI system at current status of technology, some main barriers in terms of practicability and usability can be identified:
First, preparation effort for EEG acquisition with commonly used wet electrodes and herewith originating inconvenience (e.g., electrode gel, washing hair) were reported as being highly discomforting for patients using BCIs (Zickler et al., 2011). This issue is currently addressed by several manufacturers, e.g., by investigating dry electrodes. First prototypes have been tested for use in BCI (e.g., Grozea et al., 2011; Zander et al., 2011, for a review see Mak et al., 2011).
Second, since its first description in 1988, numerous modifications to the ERP–BCI have been well studied and led to many improvements e.g., in signal processing and classification (e.g., Krusienski et al., 2006, 2008; Blankertz et al., 2011, for reviews see Lotte et al., 2007; Kleih et al., 2011) and on the stimulus presentation side (e.g., Sellers et al., 2006; Guger et al., 2009; Salvaris and Sepulveda, 2009; Takano et al., 2009; Jin et al., 2010b; Townsend et al., 2010; Frye et al., 2011; Kaufmann et al., 2011; McFarland et al., 2011). Many of these modifications have been incorporated into today’s BCI software systems and led to implementation of a plethora of parameters that allow for individual adjustment. However, being comprehensive and flexible for research purpose inevitably entails complexity and effort in user handling. Therefore, this constitutes a major problem when bringing BCI technology toward end-users (Zickler et al., 2009), as they and their care-givers may not be educated in handling of complex software as well as analysis and interpretation of acquired EEG data for calibrating the BCI system.
Third, communication speed and accuracy are low compared to existing solutions from the field of assistive technology (AT; Zickler et al., 2011). In parts this issue may be improved with further investigation of new signal processing methods and/or modifications of the paradigm. For example Kaufmann et al. (2011) recently investigated new stimuli, i.e., flashing characters with famous faces, which clearly outperformed classic character flashing in terms of communication speed and accuracy. Consequently, choice of appropriate stimuli may enhance bit rate. However, independent of all possible improvements, the commonly used ERP–BCI paradigm is necessarily limited to spelling on a character-by-character basis. Therefore, another attempt to foster bit rate is to integrate a predictive text entry (PTE) system into the BCI paradigm (Wolpaw et al., 2002). Besides the classic character-by-character text entry, Ryan et al. (2011) presented users with a separate window of numbered text suggestions, determined by a predictive text system. Users were provided with the possibility to select these numbered text predictions by focusing on their associated number in the matrix. For example, after spelling an “y,” a first suggestion “your” would appear with number “1” among other suggestions in the separate window and by focusing on “1” in the character-matrix, users would in fact spell “your.” We therefore further refer to this paradigm as “indirect paradigm.” The authors reported that integration of such PTE system into the BCI effectively decreased the time needed to spell a sentence. A likewise indirect approach was proposed by Jin et al. (2010a) who adapted a Chinese cell-phone input system for BCI use to enable communication for languages that comprise too many characters for classic character-by-character text entry (e.g., Chinese, Japanese). In their system, seven word suggestions were presented that could be chosen indirectly by focusing on the numbers 1–7 in the matrix. Unfortunately, Ryan et al. (2011) reported that overall spelling accuracy was significantly decreased when using such indirect PTE paradigms, which was explained with higher workload and task demands (dual task interference). However, it remains unclear if this negative side effect is generalizable for all paradigms using predictive text system or if the higher workload is evoked by the indirect paradigm used in this study, i.e., selecting predictive text by focusing on its related number in the main matrix.
We addressed the latter two barriers by developing and evaluating a software solution, further referred to as optimized communication interface (OCI). It was specifically designed for use by laymen and thus reduces handling of the whole BCI application to a minimum of several button presses, thereby automatically controlling all other necessary steps in the background. Its core feature lies in an auto-calibration of the classifier that – with one button press – individually adjusts classifier weights to the user’s brain signals. The system assesses quality of the signal recorded during a calibration session and decides if further calibration data is needed. Furthermore a PTE system was integrated as optional choice. Instead of numbering text suggestions and requiring the user to indirectly focus on the appropriate numbers (Ryan et al., 2011), we integrated predictive text directly into the matrix. This could decrease workload and task demand as users directly focus on the suggested word and its selection would be similar to selections of any other character in the matrix.
The herein presented study evaluated use of OCI by investigating (1) if auto-calibrating BCI systems can be used independently by laymen and (2) if predictive text incorporated into the speller matrix may better serve the goal of fast but highly accurate BCI-based communication than the classic character-by-character selection.
Materials and Methods
Optimized Communication Interface (OCI) Software
Implementation of OCI was based on the core modules of BCI2000 (Schalk et al., 2004, Version 3, http://bci2000.org) and compiled with Microsoft Visual Studio 2008 compiler. It can be grouped into three work stages: (1) modification of the graphical user interface, (2) implementation of an auto-calibration and (3) implementation of a stimulus presentation paradigm including a PTE system.
Graphical user interface
BCI2000’s graphical user interface was modified using Qt Designer 4.7. Handling of the whole BCI application was reduced to one button and an optional check mark for switching on the PTE system (see Figure 1). When starting OCI, the button acts as a start button for the calibration session. With one button press, all parameters are automatically set in the background and the classic character-matrix appears. After a predefined calibration word was spelled, the matrix disappears and auto-calibration is performed in the background without user intervention. In case of successful calibration, the button automatically turns into a start button for the online, free-spelling mode. Otherwise it would act as a start button for recalibration of the system.
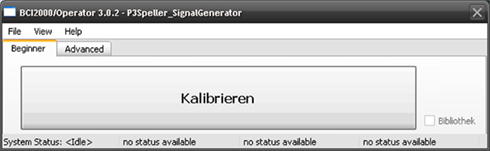
Figure 1. Graphical user interface of OCI. The application is controlled with one button only. When starting OCI, the button displays “Kalibrieren” (German for “calibrate”). During the calibration phase it acts as a stop button, whereas after successful calibration it turns into a start button for the online mode. Optionally, a predictive text entry system is switched on by selecting the “Bibliothek” check mark (German for “library”).
Auto-calibration
Auto-calibration was performed by automatically transferring the acquired data to MATLAB (The Mathworks, USA; version 2010b) using the MATLAB Component Runtime 4.14 to bridge between C++ and MATLAB code. A stepwise linear discriminant analysis (intervals of 800 ms post-stimulus, no spatial filtering) was performed based on data from all 12 EEG electrodes and thereof classifier weights were computed for the online sessions. Also, the number of sequences (NoS; a sequence consists of all rows and columns flashed once) used for flashing the characters in the online sessions was computed. Accuracy and stability of offline classification determined the outcome of the calibration session: (1) If accuracy was below 75% with 15 sequences, calibration session had to be performed again. (2) NoS used for online sessions was adjusted based on the stability of the classifier output, i.e., maximum accuracy remained stable after a given amount of data segments. In this case, NoS was defined as the minimum number of sequences to reach a stable offline accuracy maximum plus two sequences. E.g., if stable 100% accuracy were reached offline with seven sequences, NoS would have been nine during online sessions. (3) If no plateau was reached but classification accuracy was above 75%, NoS was set to 15. (4) The minimum NoS was restricted to eight to prevent from high error rates in long spelling sessions.
Note that auto-calibration was performed invisible to the user and all parameters for the online sessions were automatically adjusted without expert or user intervention.
Predictive text and stimulus presentation paradigm
First, we wrote an application that screened several prominent German internet pages (e.g., news pages) for words and counted their amount of occurrence. The word list was then sorted with decreasing occurrence and manually checked for non-sense words or words with internet specific content, e.g., name of the webpage holder. The final list comprised 82.616 words. This number includes various grammatical variations of the same word, e.g., plural forms.
Suggestion of predictive text and stimulus presentation matrix was implemented in Python 2.5 and connected to BCI2000 via user datagram protocol. After each letter selection, the application screened the list downwards to update the six most likely words that were than presented in the first column of the speller matrix. If less than six words matched the previously entered letters, fewer up to none text suggestions were presented. At the beginning of new words, i.e., after selection of space, word deletion or after selection of a predictive word, the six most frequent words were presented (first six words of the list).
For the control condition, i.e., a matrix without PTE, an identical Python based stimulus presentation paradigm was used. Only it did not contain the PTE. Figure 2 provides examples of both stimulus presentation matrices. All matrices were of size 6 × 6 and displayed on a computer screen approximately 70 cm distant from the participant (size: 22′′; refresh rate: 60 Hz; resolution: 1680 × 1050; size of matrix: 40 × 25 cm2).
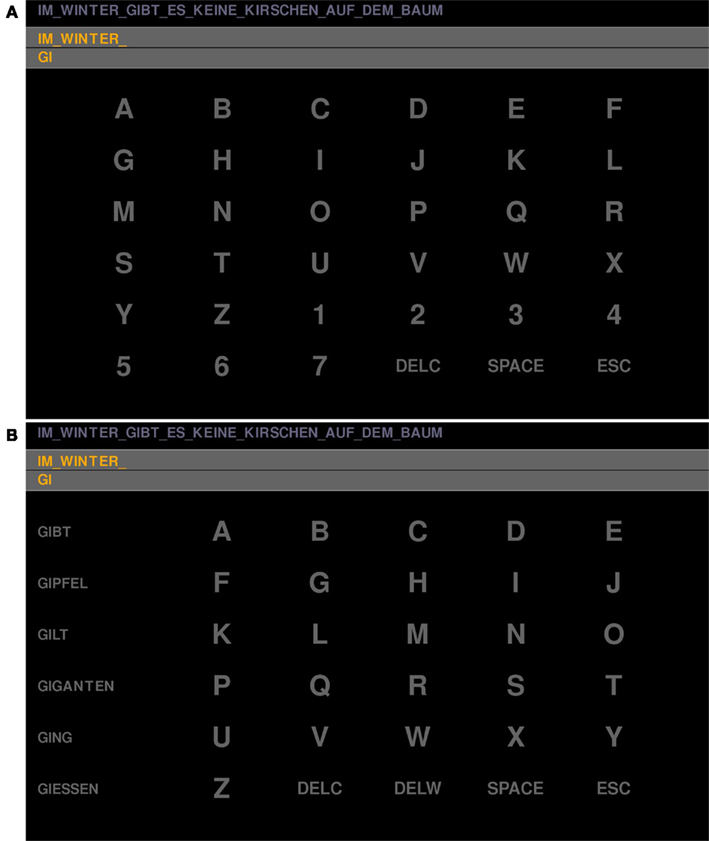
Figure 2. Two paradigms were tested. (A) The classic character matrix consisting of single characters. Furthermore the matrix contains functions for deletion (delete character), space, and escape (finish writing). (B) Our new predictive text entry paradigm with predictive text included into the first column of the matrix to allow for direct selection of words. Also, the matrix contains functions for deletion (delete character, delete word), space, and escape.
As predictive text was directly integrated into the matrix it could be flashed in a similar manner than usually done in ERP-BCIs, i.e., in both conditions (PTE and character-by-character entry) the full 6 × 6 matrix was flashed row/column wise. Thus, selection of predictive text was also performed by counting each highlighting of the intended word to spell. The BCI system always chose the cell in the matrix with highest probability, which could be a selection of predictive text, single characters or commands (delete, space, escape) depending on the content of the chosen matrix cell (see Figure 2).
Experimental Schedule
Electrodes were mounted by the experimenter. Thereafter, all participants handled the software fully on their own without expert support. Handling of OCI was explained in a condensed user manual (paper hardcopy) and with two short videos.
First, participants were required to start OCI and to start a calibration session where they had to spell the word “BRAINPOWER.” Each character was highlighted 30 times per letter to spell [NoS = 15; stimulus duration of 31.25 ms; inter-stimulus interval (ISI) of 125 ms]. This resulted in a final data set of 30 × 10 target and 150 × 10 non-target stimuli. From this data set, classifier weights were automatically computed in the background, invisible to the user.
Thereafter, participants twice spelled a German sentence comprising of 45 characters (nine words) using the automatically adjusted classifier weights and automatically determined NoS. Errors had to be corrected immediately such that the finalized sentence was 100% accurate. Then, spelling had to be terminated by selecting twice the “escape” function from the matrix, which brought them back to the main application window (necessary double selection constituted a safety mechanism of the “escape” function, to prevent unintended program termination due to selection error, i.e., selecting “escape” only once had no consequence). The inter-trial interval was 5 s to provide participants with enough time to perceive feedback on the spelled character/word and to screen all predictive text suggestions before the next stimulus sequence began. The same sentence was spelled once with PTE and once without, but both times with equal classifier weights and equal NoS to ensure comparability (within-subject design; calibration had to be performed only once). The order was randomized across participants.
After finalization of the spelling sessions, participants were asked to fill out questionnaires on handling of OCI and the PTE.
Participants
N = 20 healthy university students with normal or corrected to normal vision participated in the study. All participants were native German speakers and BCI novices. Due to equipment failure, data acquisition for N = 1 participant was aborted. The final sample comprised N = 19 participants (10 male, mean age 23.9 years, SD = 3.7, range 20–35). The experiment was conducted in accordance with standard ethical guidelines as defined by the Declaration of Helsinki (World Medical Association) and the European Council’s Convention for the Protection of Human Rights and Dignity of the Human Being with regard to the Application of Biology and Medicine (Convention on Human Rights and Biomedicine). All participants gave signed informed consent prior to the experiment and received 8 € per hour for taking part in the study.
Data Acquisition
Electroencephalogram was acquired from 12 passive Ag/AgCl electrodes that were placed according to the guidelines of the American Electroencephalographic Society at positions Fz, FC1, FC2, C3, Cz, C4, P3, Pz, P4, O1, Oz, and O2. Furthermore an electrooculogram (EOG) was obtained from two horizontal (hEOG) and two vertical (vEOG) sintered Ag/AgCl electrodes. Both, EEG and EOG were recorded at a sampling rate of 256 Hz and amplified with a 16-channel g.USBamp amplifier (g.tec Medical Engineering GmbH, Austria).
Analysis
The study aimed at investigating two independent aspects, i.e., feasibility of auto-calibrated BCI use and benefit of a new PTE paradigm.
Suitability of the auto-calibration was assessed by evaluating offline classifier accuracy as well as online spelling performance with the classic character-by-character-matrix condition. As auto-calibrated classifiers may build upon ocular artifacts due to missing control by the experimenter, we compared the auto-calibrated classifier accuracy to classifier accuracy after offline ocular artifact correction (Gratton et al., 1983). Benefit of our new PTE paradigm was assessed by comparing to the classic character-by-character-matrix the overall time needed to finish the sentence correctly, the amount of errors per selected item as well as achieved bit rate. Also, we evaluated results from questionnaires in terms of user satisfaction.
Besides computation of bit rate (as described e.g., in Wolpaw et al., 2000; Wolpaw et al., 2002; Serby et al., 2005) we computed a bit rate measure incorporating the information transferred with selections of whole words. PTE systems enhance bit rate in that with one selection many characters are communicated at once. Thus true bit rate is not only depending on the number of accurate selections made but also on the information transferred with each selection. Certainly such measure heavily depends on the length of the selected words. For example true bit rate would be five times higher when selecting a 10-character word instead of a two-character word, although bit rate in terms of selections/minute would be identical. Therefore true bit rate has to be interpreted with caution and is only provided to display the potential of PTE systems.
For offline analysis of ERPs, EEG data were filtered with 0.1 Hz high and 30 Hz low pass and corrected for ocular artifacts (Gratton et al., 1983). To avoid influence of overlapping ERPs due to the short ISI used in this study (ISI = 125 ms), only target stimuli with a minimum time distance of 469 ms (i.e., at least two non-target stimuli in between) were taken into account for ERP analysis. For statistical comparison, P300 peak amplitudes were obtained as maximum potentials between 200 and 300 ms at electrode Cz for both conditions.
Apart from ocular artifact correction with Brain Vision Analyser (Brain Products GmbH, Germany), all data analysis was performed using Matlab (The Mathworks, USA; version 2010b), and SPSS statistics (IBM, USA). Statistical comparison was performed using analysis of variance (ANOVA), except for not normally distributed data for which Wilcoxon-Test was used.
Results
All participants were able to handle OCI on their own without expert support and to twice spell the predefined sentence correctly using the auto-calibrated classifier. In a forced-choice questionnaire all participants reported, that handling of the software was straightforward (average score: 3.84, with 1 = “not at all,” 2 = “not really,” 3 = “mostly,” 4 = “completely”), that they would be able to manage the BCI application on their own in the future (3.84) and to explain it to others (3.74).
Auto-Calibration
For all participants, classifier weights were adjusted automatically after one calibration session and no repetitions were needed. In the consecutively scheduled online spelling sessions participants achieved overall good performance levels with regards to the length of the spelled sentence (average performance with classic character-by-character paradigm: 91.2%, SD = 7.8, range: 76–100% accuracy).
As auto-calibration may lead to classification of ocular artifacts, classifier accuracy of the auto-calibration as well as achieved stable NoS (see Auto-Calibration) were compared to accuracy and NoS after ocular artifact detection. No significant difference was found for the automatically selected NoS [F(1, 18) = 0.156, p = .697, η2partial = 0.009]. Also, offline classification accuracy did not differ before and after correction [With minimal NoS possible (NoS = 1): F(1, 18) = 0.518, p = .481, η2partial=0.028; with minimal NoS used online (NoS = 8): Z = −1.0, p = .317]. Furthermore, we checked how many NoS were necessary to achieve a stable classifier accuracy of 100%. Difference was not significant before and after ocular artifact correction [F(1, 18) = 0.010, p = .920, η2partial = 0.001].
Predictive Text Entry
Figure 3 summarizes the overall time needed to finish each sentence correctly. In line with Ryan et al. (2011), PTE significantly decreased spelling time [F(1, 18) = 95.74, p < .001, η2partial = 0.84]. Average time needed to finish the task was reduced by factor 1.8 (12.4 min as compared to 22.3 min without PTE).
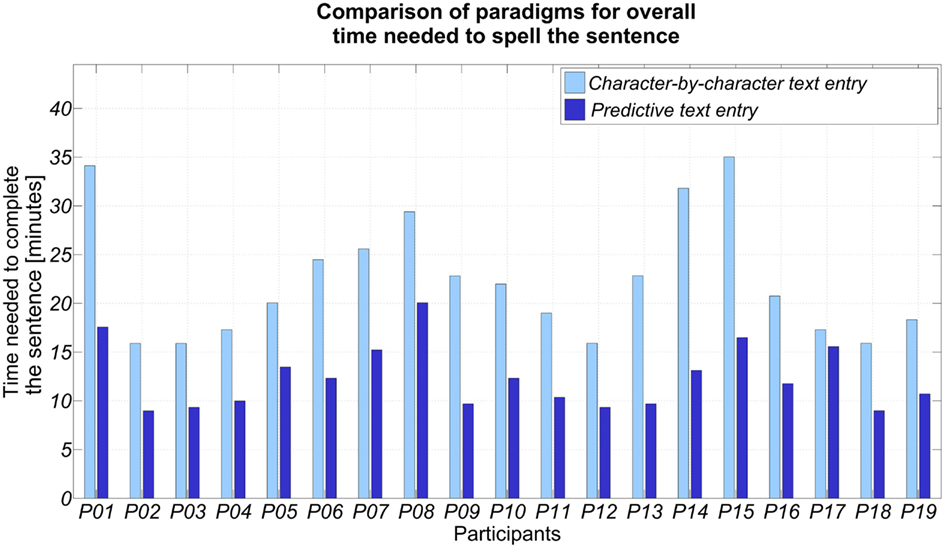
Figure 3. Overall time needed to copy spell the sentence (including error correction and escape of spelling mode after finalization of 100% accurate sentence): within-subject comparison between classic character-by-character text entry and predictive text entry paradigm.
However, in contrast to the indirect paradigm by Ryan et al. (2011), accuracy did not decrease with our PTE paradigm [F(1, 18) = 1.64, p = .216, η2partial = 0.084]. Performance ranged from 100% accuracy to 76% (classic character-by-character condition) and to 74% respectively (PTE condition), see Figure 4. Bit rate (in terms of selections per minute) was comparably high for both conditions (character-by-character: M = 15.1 characters/min, SD = 5.6; PTE: M = 15.7 characters/min, SD = 5.7). Figure 5 displays bit rate as well as true bit rate. True bit rate was higher for all participants, when using the PTE paradigm. For those participants that made many errors with this paradigm (P08, P17) benefit in terms of true bit rate was small. On the other hand, participants with high accuracy also show a high benefit. Average true bit rate for the sentence was M = 12.0 characters/min (SD = 2.7) with character-by-character text entry and M = 20.6 characters/min (SD = 5.3) with PTE. Please note that true bit rate heavily depends on the length of the selected words (see Analysis) and thus needs to be interpreted with caution. In this experiment, word length of the selected words was M = 4.1 characters on average (SD = 1.9; range: 2–8 characters).
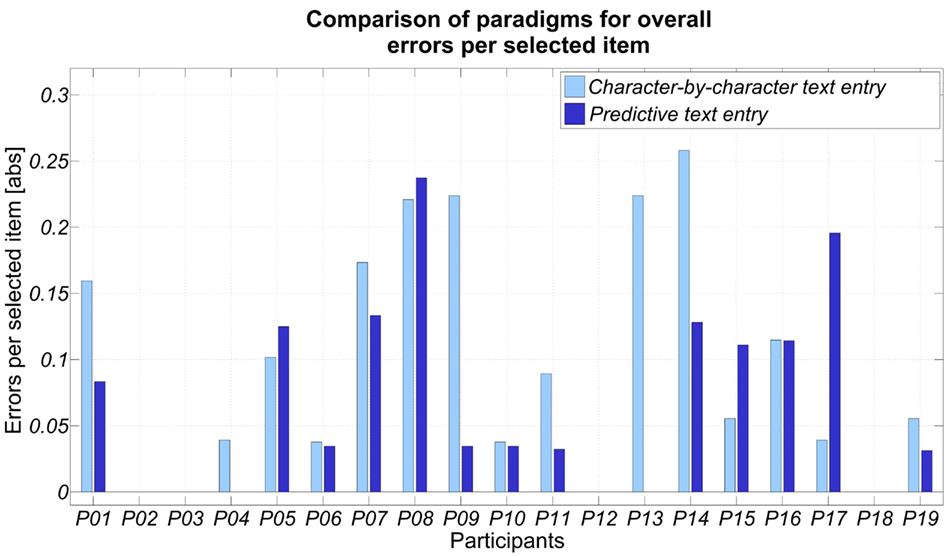
Figure 4. Average number of errors per selected item: within-subject comparison between classic character-by-character text entry and predictive text entry paradigm. As the number of items selected to finish the sentence varied between participants and conditions (e.g., due to error correction or selection of predictive text) no absolute amount of errors is presented but instead the amount of errors per selected item.
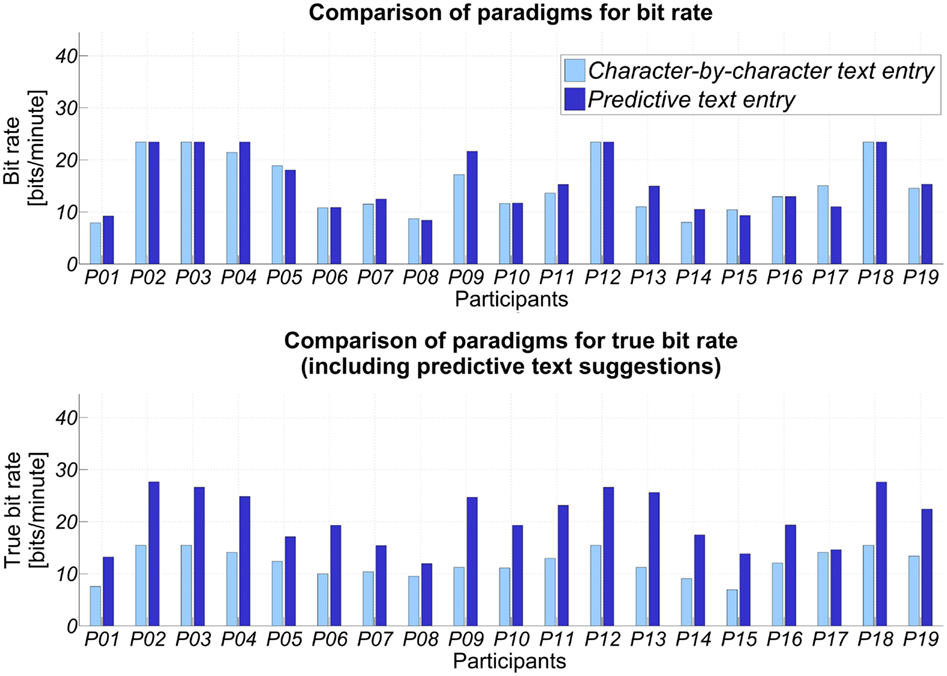
Figure 5. Bit rate (in terms of selections per time unit) and true bit rate (in terms of communicated characters per time unit): within-subject comparison between classic character-by-character text entry and predictive text entry paradigm.
Figure 6 displays the grand average ERPs for both conditions with most prominent P300 amplitude at electrode Cz around 246.5 ms (SD = 23.2; character-by-character text entry) and 250.0 ms (SD = 24.3; PTE). Grand average P300 amplitude was 3.55 μV (SD = 1.19) for character-by-character text entry and 3.44 μV (SD = 1.40) for PTE. No within-subject difference was found for P300 amplitudes between the conditions [F(1, 18) = 0.72, p = .410, η2partial = 0.038].
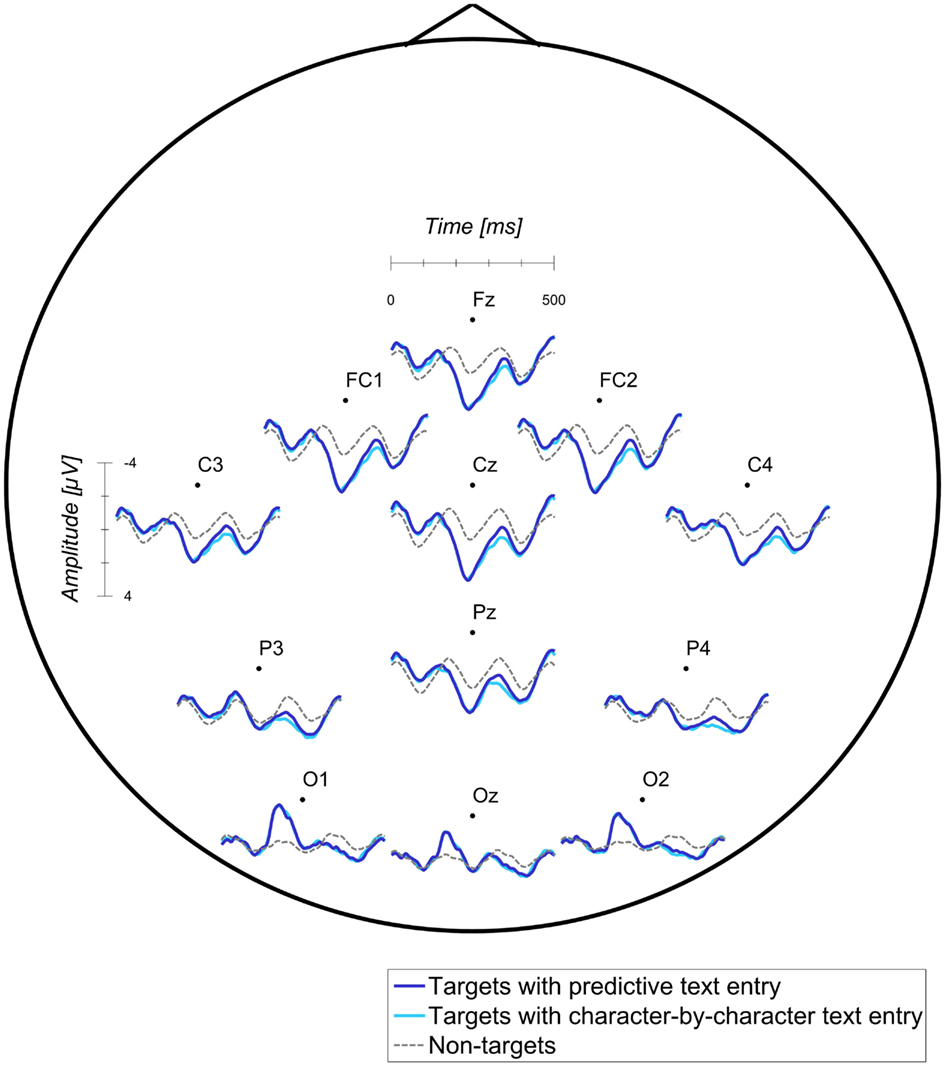
Figure 6. Grand average event-related potentials: within-subject comparison between classic character-by-character text entry and predictive text entry paradigm.
We further compared the amount of errors made when selecting a character against the amount of errors made when selecting a word or command. When using the classic character-by-character text entry, participants made M = 2.2 errors (SD = 2.2) on average for selecting a single character and M = 4.2 errors (SD = 4.8) for selecting a command. Error rates in the PTE condition were slightly lower (single character selection: M = 1.3, SD = 1.6; selection of command or word: M = 1.6, SD = 2.5). To write the sentence without any error, 10 commands (8× space, 2× escape) and 37 characters were necessary in the character-by-character text entry condition, whereas 11 commands and words and 16 characters were necessary in the PTE condition.
Evaluation of questionnaires revealed that all participants would prefer to use the PTE paradigm instead of character-by-character spelling, when exposed to BCI-based spelling in the future. Only one participant (participant 9) reported higher effort in selecting complete words as compared to single characters, which however was not reflected in his performance.
Discussion
This study provides evidence, that current BCI technology can be automatized and operated by BCI novices through a user-centered, easy-to-use graphical user interface. This is inevitable when bringing BCIs out of the laboratory environment to end-user’s homes. Zickler et al. (2009) assessed user needs and requirements from N = 77 AT users and reported “functionality,” “possibility of independent use,” and “easiness of use” as most important requirements. The latter was one of the main reasons for dissatisfaction with current AT solutions. Importantly, our results from handling of OCI indicate high satisfaction with both, “easiness of use” and “possibility of independent use.” Furthermore, “functionality” was high as none of the auto-calibration sessions had to be performed twice and all participants were able to correctly spell the sentences. Overall spelling accuracy was high and none of the participants performed with lower than 70% accuracy – an accuracy level previously described as minimum level for communication (Kübler et al., 2001).
When considering adoption of a new AT solution, “functionality/effectiveness” was identified as the major critical factor for potential end-users (Zickler et al., 2009). This study suggested integration of predictive text directly into the matrix and proofed high benefit in terms of spelling speed (effectiveness) without loss of accuracy (functionality). As can be particularly seen from the true bit rate of participants with lower accuracy in the PTE paradigm, a high performance level is inevitable to clearly benefit from a PTE system. The unaffected P300 amplitudes in our study show that when integrating predictive text directly into the matrix workload may remain low, as predictive text is selected in a similar manner than single characters. The drop in performance reported by Ryan et al. (2011) may thus indeed be attributed to higher workload and dual task interference in the indirect PTE paradigm. The remaining performance level in our study, as well as the heavily decreased time needed to spell a sentence may well explain why none of the participants reported to prefer the character-by-character over the PTE paradigm. Advantageously, daily life application with such PTE system could easily be equipped with algorithms for grammar recognition and learning of new words.
Limitations and Future Experimentation
To reduce the amount of errors during spelling of long sentences, we restricted the automatically adjusted NoS to a minimum of eight sequences. This limit was set on basis of previous experience. Apparently, there is a trade-off, as low NoS increases the probability of errors due to a low number of trials to average, whereas high NoS may entail errors due to increased duration of spelling time. We speculate that for some participants NoS could be further reduced. This could also be realized with recently introduced dynamic stopping methods. (e.g., Lenhardt et al., 2008; Höhne et al., 2010; Liu et al., 2010; Schreuder et al., 2011b; for comparison of methods see Schreuder et al., 2011a). Instead of flashing characters with a fixed NoS, dynamic stopping algorithms adjust the NoS in every trial, i.e., stimulus presentation stops as soon as probability of correct target classification reaches a certain level.
This study aimed at investigating if spelling at high accuracy level is possible using an auto-calibrated classifier. Comparison to manually calibrated classifier performance needs separate investigation. Manual calibration may still be valuable for some cases (e.g., for exclusion of bad channels or detection of equipment failure). Auto-calibration may also be further equipped with mechanisms for detecting such cases. Our results are promising in that high accuracy could be achieved in all participants using auto-calibration and none of the participants needed recalibration of the system.
We assume that our validation results from healthy users may well transfer to end-users as we cautiously addressed their major concerns (Zickler et al., 2009). However, Zickler et al. (2010) found differences between end-users and care-givers with regards to their expectations of new AT solutions – differences which may certainly also exist in our healthy sample. Thus, it is inevitable to develop and validate new BCI technology in close discussion with them. Future experimentation therefore needs to validate our results in an end-user sample.
Conclusion
In sum, this study specifically addressed critical aspects of ERP–BCI-based communication reported by end-users, i.e., “functionality/effectiveness,” “easiness of use,” and “possibility of independent use.” Our results proofed feasibility of expert independent BCI-based communication using auto-calibration and underlined the strong benefit of predictive text directly integrated into the spelling matrix.
Conflict of Interest Statement
The authors declare that the research was conducted in the absence of any commercial or financial relationships that could be construed as a potential conflict of interest.
Acknowledgments
We thank Ruben Real for his support. This work is supported by the European ICT Programme Project FP7-224631. This paper only reflects the authors’ views and funding agencies are not liable for any use that may be made of the information contained herein. Open access publication was funded by the German Research Foundation (DFG) and the University of Würzburg in the funding programme Open Access Publishing.”
References
Blankertz, B., Lemm, S., Treder, M., Haufe, S., and Müller, K.-R. (2011). Single-trial analysis and classification of ERP components – a tutorial. Neuroimage 56, 814–825.
Farwell, L., and Donchin, E. (1988). Talking off the top of your head: toward a mental prosthesis utilizing event-related brain potentials. Electroencephalogr. Clin. Neurophysiol. 70, 510–523.
Frye, G. E., Hauser, C. K., Townsend, G., and Sellers, E. W. (2011). Suppressing flashes of items surrounding targets during calibration of a P300-based brain-computer interface improves performance. J. Neural Eng. 8, 025024.
Gratton, G., Coles, M. G., and Donchin, E. (1983). A new method for off-line removal of ocular artifact. Electroencephalogr. Clin. Neurophysiol. 55, 468–484.
Grozea, C., Voinescu, C. D., and Fazli, S. (2011). Bristle-sensors – low-cost flexible passive dry EEG electrodes for neurofeedback and BCI applications. J. Neural Eng. 8, 025008.
Guger, C., Daban, S., Sellers, E., Holzner, C., Krausz, G., Carabalona, R., Gramatica, F., and Edlinger, G. (2009). How many people are able to control a P300-based brain-computer interface (BCI)? Neurosci. Lett. 462, 94–98.
Höhne, J., Schreuder, M., Blankertz, B., and Tangermann, M. (2010). Two-dimensional auditory p300 speller with predictive text system. Conf. Proc. IEEE Eng. Med. Biol. Soc. 2010, 4185–4188.
Jin, J., Allison, B. Z., Brunner, C., Wang, B., Wang, X., Zhang, J., Neuper, C., and Pfurtscheller, G. (2010a). P300 Chinese input system based on Bayesian LDA. Biomed. Tech. (Berl.) 55, 5–18. doi:10.1515/BMT.2010.003
Jin, J., Horki, P., Brunner, C., Wang, X., Neuper, C., and Pfurtscheller, G. (2010b). A new P300 stimulus presentation pattern for EEG-based spelling systems. Biomed. Tech. (Berl.) 55, 203–210. doi:10.1515/BMT.2010.029
Kaufmann, T., Schulz, S. M., Grünzinger, C., and Kübler, A. (2011). Flashing characters with famous faces improves ERP-based brain-computer interface performance. J. Neural Eng. 8, 056016.
Kleih, S. C., Kaufmann, T., Zickler, C., Halder, S., Leotta, F., Cincotti, F., Aloise, F., Riccio, A., Herbert, C., Mattia, D., and Kübler, A. (2011). Out of the frying pan into the fire – the P300-based BCI faces real-world challenges. Prog. Brain Res. 194, 27–46.
Kleih, S. C., Nijboer, F., Halder, S., and Kübler, A. (2010). Motivation modulates the P300 amplitude during brain-computer interface use. Clin. Neurophysiol. 121, 1023–1031.
Krusienski, D. J., Sellers, E. W., McFarland, D. J., Vaughan, T. M., and Wolpaw, J. R. (2008). Toward enhanced P300 speller performance. J. Neurosci. Methods 167, 15–21.
Krusienski, D. J., Sellers, E. W., Cabestaing, F., Bayoudh, S., McFarland, D. J., Vaughan, T. M., and Wolpaw, J. R. (2006). A comparison of classification techniques for the P300 Speller. J. Neural Eng. 3, 299–305.
Kübler, A., Neumann, N., Kaiser, J., Kotchoubey, B., Hinterberger, T., and Birbaumer, N. P. (2001). Brain-computer communication: self-regulation of slow cortical potentials for verbal communication. Arch. Phys. Med. Rehabil. 82, 1533–1539.
Lenhardt, A., Kaper, M., and Ritter, H. J. (2008). An adaptive P300-based online brain-computer interface. IEEE Trans. Neural Syst. Rehabil. Eng. 16, 121–130.
Liu, T., Goldberg, L., Gao, S., and Hong, B. (2010). An online brain-computer interface using non-flashing visual evoked potentials. J. Neural Eng. 7, 036003.
Lotte, F., Congedo, M., Lécuyer, A., Lamarche, F., and Arnaldi, B. (2007). A review of classification algorithms for EEG-based brain-computer interfaces. J. Neural Eng. 4, R1–R13.
Mak, J. N., Arbel, Y., Minett, J. W., McCane, L. M., Yuksel, B., Ryan, D., Thompson, D., Bianchi, L., and Erdogmus, D. (2011). Optimizing the P300-based brain-computer interface: current status, limitations and future directions. J. Neural Eng. 8, 025003.
McFarland, D. J., Sarnacki, W. A., Townsend, G., Vaughan, T., and Wolpaw, J. R. (2011). The P300-based brain-computer interface (BCI): effects of stimulus rate. Clin. Neurophysiol. 122, 731–737.
Nijboer, F., Sellers, E. W., Mellinger, J., Jordan, M. A., Matuz, T., Furdea, A., Halder, S., Mochty, U., Krusienski, D. J., Vaughan, T. M., Wolpaw, J. R., Birbaumer, N., and Kübler, A. (2008). A P300-based brain – computer interface for people with amyotrophic lateral sclerosis. Clin. Neurophysiol. 119, 1909-1916.
Picton, T. W. (1992). The P300 wave of the human event-related potential. J. Clin. Neurophysiol. 9, 456–479.
Polich, J. (2007). Updating P300: an integrative theory of P3a and P3b. Clin. Neurophysiol. 118, 2128–2148.
Polich, J., Alexander, J. E., Bauer, L. O., Kuperman, S., Morzorati, S., O’Connor, S. J., Porjesz, B., Rohrbaugh, J., and Begleiter, H. (1997). P300 topography of amplitude/latency correlations. Brain Topogr. 9, 275–282.
Ryan, D. B., Frye, G. E., Townsend, G., Berry, D. R., Mesa-G, S., Gates, N. A., and Sellers, E. W. (2011). Predictive spelling with a P300-based brain-computer interface: increasing the rate of communication. Int. J. Hum. Comput. Interact. 27, 69–84.
Salvaris, M., and Sepulveda, F. (2009). Visual modifications on the P300 speller BCI paradigm. J. Neural Eng. 6, 046011.
Schalk, G., McFarland, D. J., Hinterberger, T., Birbaumer, N., and Wolpaw, J. R. (2004). BCI2000: a general-purpose brain-computer interface (BCI) system. IEEE Trans. Biomed. Eng. 51, 1034–1043.
Schreuder, M., Höhne, J., Treder, M., Blankertz, B., and Tangermann, M. (2011a). Performance optimization of ERP-based BCIs using dynamic stopping. Conf. Proc. IEEE Eng. Med. Biol. Soc. 2011, 4580–4583.
Schreuder, M., Rost, T., and Tangermann, M. (2011b). Listen, you are writing! speeding up online spelling with a dynamic auditory BCI. Front. Neurosci. 5:112. doi:10.3389/fnins.2011.00112
Sellers, E. W., Kübler, A., and Donchin, E. (2006). Brain-computer interface research at the University of South Florida Cognitive Psychophysiology Laboratory: the P300 Speller. IEEE Trans. Neural Syst. Rehabil. Eng. 14, 221–224.
Sellers, E. W., Vaughan, T. M., and Wolpaw, J. R. (2010). A brain-computer interface for long-term independent home use. Amyotroph. Lateral Scler. 11, 449–455.
Serby, H., Yom-Tov, E., and Inbar, G. F. (2005). An improved P300-based brain-computer interface. IEEE Trans. Rehabil. Eng. 13, 89–98.
Takano, K., Komatsu, T., Hata, N., Nakajima, Y., and Kansaku, K. (2009). Visual stimuli for the P300 brain–computer interface: a comparison of white/gray and green/blue flicker matrices. Clin. Neurophysiol. 120, 1562–1566.
Townsend, G., LaPallo, B. K., Boulay, C. B., Krusienski, D. J., Frye, G. E., Hauser, C. K., Schwartz, N. E., Vaughan, T. M., Wolpaw, J. R., and Sellers, E. W. (2010). A novel P300-based brain-computer interface stimulus presentation paradigm: moving beyond rows and columns. Clin. Neurophysiol. 121, 1109–1120.
Wolpaw, J. R., Birbaumer, N., Heetderks, W. J., McFarland, D. J., Peckham, P. H., Schalk, G., Donchin, E., Quatrano, L. A., Robinson, C. J., and Vaughan, T. M. (2000). Brain-computer interface technology: a review of the first international meeting. IEEE Trans. Rehabil. Eng. 8, 164–173.
Wolpaw, J. R., Birbaumer, N., McFarland, D. J., Pfurtscheller, G., and Vaughan, T. M. (2002). Brain-computer interfaces for communication and control. Clin. Neurophysiol. 113, 767–791.
Zander, T. O., Lehne, M., Ihme, K., Jatzev, S., Correia, J., Kothe, C., Picht, B., and Nijboer, F. (2011). A dry EEG-system for scientific research and brain-computer interfaces. Front. Neurosci. 5:53. doi:10.3389/fnins.2011.00053
Zickler, C., Di Donna, V., Kaiser, V., Al-Khodairy, A., Kleih, S. C., and Kübler, A. (2009). “BCI applications for people with disabilities: defining user needs and user requirements,” in Assistive Technology from Adapted Equipment to Inclusive Environments, AAATE. 25 Assistive Technology Research Series. eds P. L. Emiliani, L. Burzagli, A. Como, F. Gabbanini, and A.-L. Salminen (Amsterdam: IOS Press), 185–189.
Zickler, C., Kaiser, V., Al-Khodairy, A., Kleih, S. C., Kübler, A., Mattia, D., Mongardi, S., Rohm, M., Rupp, R., Staiger-Sälzer, P., and Hoogerwerf, E. J. (2010). “BCI-applications: requirements of disabled end-users and professional users,” in Poster presented at the 1st TOBI Workshop 2010, Graz.
Keywords: brain–computer interface, user-centered, auto-calibration, predictive text entry, event-related potentials, assisitve technology, P300-Speller, ERP-BCI
Citation: Kaufmann T, Völker S, Gunesch L and Kübler A (2012) Spelling is Just a Click Away – A User-Centered Brain–Computer Interface Including Auto-Calibration and Predictive Text Entry. Front. Neurosci. 6:72. doi: 10.3389/fnins.2012.00072
Received: 14 February 2012; Accepted: 30 April 2012;
Published online: 23 May 2012.
Edited by:
Emanuel Donchin, University of South Florida, USACopyright: © 2012 Kaufmann, Völker, Gunesch and Kübler. This is an open-access article distributed under the terms of the Creative Commons Attribution Non Commercial License, which permits non-commercial use, distribution, and reproduction in other forums, provided the original authors and source are credited.
*Correspondence: Tobias Kaufmann, Department for Psychology I, University of Würzburg, Marcusstr. 9-11, 97070 Würzburg, Germany. e-mail:dG9iaWFzLmthdWZtYW5uQHVuaS13dWVyemJ1cmcuZGU=