- 1Defitech Chair in Brain Machine Interface, Center for Neuroprosthetics, Ecole Polytechnique Fédérale de Lausanne, Lausanne, Switzerland
- 2Helen Wills Neuroscience Institute, University of California, Berkeley, Berkeley, CA, United States
- 3Department of Psychology, University of California, Berkeley, Berkeley, CA, United States
Certain brain disorders resulting from brainstem infarcts, traumatic brain injury, cerebral palsy, stroke, and amyotrophic lateral sclerosis, limit verbal communication despite the patient being fully aware. People that cannot communicate due to neurological disorders would benefit from a system that can infer internal speech directly from brain signals. In this review article, we describe the state of the art in decoding inner speech, ranging from early acoustic sound features, to higher order speech units. We focused on intracranial recordings, as this technique allows monitoring brain activity with high spatial, temporal, and spectral resolution, and therefore is a good candidate to investigate inner speech. Despite intense efforts, investigating how the human cortex encodes inner speech remains an elusive challenge, due to the lack of behavioral and observable measures. We emphasize various challenges commonly encountered when investigating inner speech decoding, and propose potential solutions in order to get closer to a natural speech assistive device.
Introduction
Neural engineering research has made tremendous advances in decoding motor (Ajiboye et al., 2017) or visual neural signals (Lewis et al., 2015) for assisting and restoring lost functions in patients with disabling neurological conditions. An important extension of these approaches is the development of assistive devices that restore natural communication in patients with intact language systems but limited verbal communication due to neurological disorder. Several brain-computer interfaces have allowed relevant communication applications, such as moving a cursor on the screen (Wolpaw et al., 1991) and spelling letters (Farwell and Donchin, 1988; Gilja et al., 2015; Jarosiewicz et al., 2015; Vansteensel et al., 2016; Pandarinath et al., 2017). Although this type of interface has proven to be useful, patients had to learn to modulate their brain activity in an unnatural and unintuitive way—i.e., performing mental tasks like a rotating cube, mental calculus, movement attempts to operate an interface (Millán et al., 2009), or detecting rapidly presented letters on a screen, such as in the P300-speller (see Fazel-Rezai et al., 2012 for a review) and steady-state visual evoked potentials paradigm(Srinivasan et al., 2006; Nijboer et al., 2008).
As an alternative, people with speech deficits would benefit from a communication system that can directly infer inner speech from brain signals—allowing them to interact more naturally with the world. Inner speech (also called imagined speech, internal speech, covert speech, silent speech, speech imagery, or verbal thoughts) is defined here as the ability to generate internal speech representations, in the absence of any external speech stimulation or self-generated overt speech. While much has been learnt about actual speech perception and production (see Price, 2000; Démonet et al., 2005; Hickok and Poeppel, 2007, for reviews), investigating inner speech has remained a challenging task due to the lack of behavioral output. Indeed, it remains difficult to study this internal neural process due to the difficulty to time-lock precise events (acoustic features, phonemes, words) to neural activity during inner speech. Therefore, substantial efforts have aimed to develop new strategies for analyzing these brain signals.
Investigating the underlying neural representations associated with these different speech features during inner speech is central for engineering speech neuroprosthetic devices. For instance, speech processing includes various processing steps—such as acoustic processing in the early auditory cortex, phonetic, and categorical encoding in posterior areas of the temporal lobe and semantic and higher level of linguistic processes in later stages (Hickok and Poeppel, 2007). One can ask what are the appropriate speech stimulus-neural response mappings to target for efficient decoding and designing optimal communication technologies. For example, a decoding model can target continuous auditory spectrotemporal features predicted from the brain activity. Alternatively, decoding discrete phonemes allows building words and sentences directly.
In this review article, we describe recent research findings on understanding and decoding the neural correlates associated with inner speech, for targeting communication assistive technologies. We focused on studies that have used electrocorticographic (ECoG) recordings in the human cortex, as this promising technique allows monitoring brain activity with high spatial, temporal, and spectral resolution, as compared to electroencephalographic recordings, and the electrodes cover broader brain areas compared to intracortical recordings (Ritaccio et al., 2015). We discuss different decoding and experimental strategies to deal with common challenges that are encountered when tackling inner speech decoding. We consider new avenues and future directions to meet the key scientific and technical challenges in development of a realistic, natural speech decoding device.
In the next section, we first briefly present the properties of electrocorticography, together with its advantages for investigating the neural representation of human speech. We next describe several neuro-computational modeling approaches to neural decoding of speech features.
Electrocorticographic Recordings
Electrocorticography (ECoG), also called intracranial recording or intracranial electroencephalography (iEEG), is used in patients with intractable epilepsy to localize the seizure onset zone, prior to brain tissue ablation. In this procedure, electrode grids, strips or depth electrodes are temporarily implanted onto the cortical surface, either above (epidural) or below (subdural) the dura mater (Figure 1). Because of its invasiveness, only in rare cases, patients are implanted with such electrodes, and it remains exclusively for clinical purposes; nevertheless, the implantation time provides a unique opportunity to investigate human brain functions, with high spatial (millimeters), temporal (milliseconds), and spectral resolution (0–500 Hz). In addition, it covers broad brain areas (typically frontal, temporal, and parietal cortex), which is beneficial given the complex and widely distributed network associated with speech. Finally, electrocorticography is suitable for neuroprosthetic and brain-computer interface applications (Leuthardt et al., 2004, 2006; Felton et al., 2007; Schalk et al., 2007; Blakely et al., 2009; Wang et al., 2013; Kapeller et al., 2014; Moses et al., 2018). Therefore, this technique is an ideal recording candidate for investigating speech functions and for targeting speech neuroprosthetic devices.
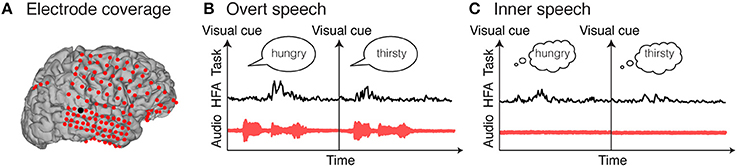
Figure 1. Electrocorticographic recordings. Example of electrocorticographic grid locations overlaid on cortical surface reconstructions of a subject's MRI scan (A). Examples of single trial high frequency activity (HFA) for an electrode highlighted in black in (A). Single trials represent examples of overt speech word repetition (B) and inner speech word repetition (C).
ECoG activity contains different signal components (Marshall et al., 2006; Miller et al., 2007; Buzsáki and Wang, 2012; Giraud and Poeppel, 2012) that may relate to different underlying physiological mechanisms, and therefore different mappings between speech stimulus and neural response. For example, the neural representation of speech has been mainly studied using both high frequency (~70–500 Hz) and low frequency (~4–8 Hz).
High frequency activity (HFA; ~70–500 Hz) has been correlated with multiunit spike rate and asynchronous post-synaptic current of the underlying neuronal population (Manning et al., 2009; Whittingstall and Logothetis, 2009; Buzsáki et al., 2012; Lachaux et al., 2012; Rich and Wallis, 2017). In particular, HFA has been shown to robustly encode various speech representations, such as early spectrotemporal acoustic features of speech in the superior temporal gyrus (Pasley et al., 2012; Kubanek et al., 2013)—a region commonly associated with speech perception. In addition, the superior temporal gyrus plays an important role in transforming these acoustic cues into categorical speech units (Chang et al., 2010; Pasley et al., 2011; Mesgarani et al., 2014). HFA in the ventral sensorimotor cortex has been shown to encode acoustic (Pasley and Knight, 2013; Martin et al., 2014; Cheung et al., 2016) and phonetic representations during speech perception, and somatotopically arranged articulator representations (lips, tongue, larynx, and jaw) during speech production (Bouchard et al., 2013; Cheung et al., 2016; Conant et al., 2018).
Low frequencies, such as theta band, have been shown to track the acoustic envelope of speech, to correlate with syllabic rate, and to discriminate spoken sentences (Luo and Poeppel, 2007; Ding and Simon, 2012; Giraud and Poeppel, 2012; Zion Golumbic et al., 2013). In addition, theta rhythms showed significant power changes in Broca's area and temporal language areas during a verb generation task, and showed interactions with high frequency band, through amplitude-amplitude and phase-amplitude coupling (Hermes et al., 2014).
The next section briefly introduces neural decoding models, which have been widely used in the field of speech.
Decoding Models—General Framework
Traditionally, cognitive functions have been investigated using a set of stimuli that typically vary along a single dimension of interest (e.g., attended versus not attended target). Brain activity evoked by different stimuli are then averaged and compared in order to provide new insights about the neural mechanisms under study. Conversely, decoding these cognitive functions in real-time for targeting brain-machine interfaces requires more sophisticated predictive modeling. Decoding models allow researchers to apply multivariate neural features to rich, complex and naturalistic stimuli or behavioral conditions (Kay et al., 2008; Kay and Gallant, 2009; Naselaris et al., 2011).
A commonly used modeling approach uses a regression framework to link brain activity and a stimulus or mental state representation. For instance, the stimulus features at a given time can be modeled as a weighted sum of the neural activity, as follows:
where Y(t) is the stimulus feature at time t, X(t, p) is the neural activity at time t and feature p, w(p) is the weight for a given feature p. Classification is a type of decoding model in which the neural activity is identified as belonging to a discrete event type from a finite set of choices. Both types of models can use various machine learning algorithms, ranging from simple regression techniques, to more complex non-linear approaches, such as hidden Markov models, support-vector algorithms and neural networks. Holdgraf et al. (2017) provide a review article that illustrates best-practices in conducting these analyses, and included a small sample dataset, along with several scripts in the form of jupyter notebooks. The general framework is common to all methods (Figure 2) and consists of the following steps:
1. Feature extraction: input and output features are extracted from the neural activity and from the stimulus features, respectively. Examples of speech representations typically used in decoding models are the auditory frequencies, the modulation rates, or phonemes for natural speech. For neural representations, firing rate from single unit spiking activity, or amplitudes in specific frequency bands are typically extracted from the recorded electrophysiological signal (for example, the high gamma band).
2. Model estimation: models are estimated by mapping input features to output features. The weights are calculated by minimizing a metric of error between the predicted and actual output on a training set. For example, in a linear regression model, the output is a weighted sum of input features.
3. Validation: Once a model is fit, it is then validated on new unseen data not used for training, in order to avoid overfitting and aid generalization to new data. To evaluate the accuracy, the predicted output is compared directly to the original representation.
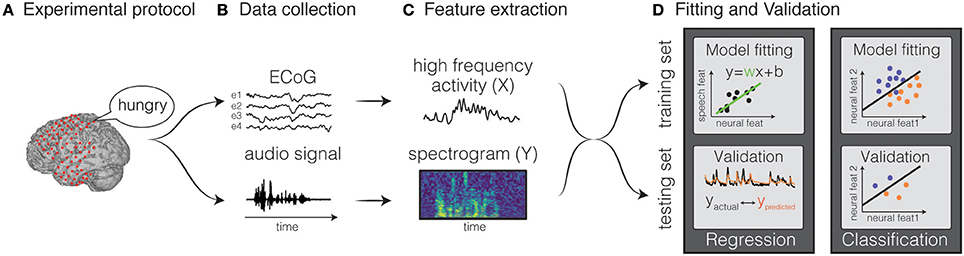
Figure 2. Decoding framework. The general framework for fitting a decoding model is depicted. The first step consists in designing a protocol (A) and recording the data (B). Then, input and output features are extracted (C), and the data are split in training and testing set. The training set is used to fit the weights of the model and the testing set is used to validated the model (D). Figures adapted from Holdgraf et al. (2017) with permissions.
In the next section, we review ECoG studies that have employed decoding models to understand and decode cognitive states associated with various inner speech representations.
Decoding Inner Speech Using Electrocorticography
A key challenge to understanding the neural representation of inner speech is to quantify the relationship between neural response and the imagined stimulus, from low-level auditory to higher-level speech representations. Several studies have exploited the advantageous properties of intracranial recordings to characterize inner speech representations. For instance, a recent study described the spatiotemporal evolution of high frequency activity during an overt and covert word repetition using trial averaging (Pei et al., 2011b; Leuthardt et al., 2012). In particular, they revealed high frequency changes in the superior temporal lobe and the supramarginal gyrus during covert speech repetition. During a covert verb generation task, high frequency activity (65–95 Hz) showed significant brain activity in Broca's area, in the middle temporal gyrus, and temporal parietal junction, and interacted with theta frequency activity (4–8 Hz) through cross-frequency coupling (Hermes et al., 2014). Finally, a recent study compared the electrocorticographic activity related to overt vs. covert conditions, and revealed a common network of brain regions (Brumberg et al., 2016).
To directly quantify the relationship between inner speech and neural response, the decoding model framework can be applied. Recently, we used a decoding model approach to reconstruct continuous auditory features from high gamma neural activity (70–150 Hz) recorded during inner speech (Martin et al., 2014). Due to the lack of any measurable behavioral output, standard decoding models (e.g., linear regression) that assume temporal alignment of input and output data are not immediately applicable. One simple approach is to take advantage of prior research demonstrating that speech perception and imagery, to some extent, share common neural mechanisms (Hinke et al., 1993; Yetkin et al., 1995; McGuire et al., 1996; Rosen et al., 2000; Palmer et al., 2001; Aleman, 2004; Aziz-Zadeh et al., 2005; Hubbard, 2010; Geva and Warburton, 2011; Perrone-Bertolotti et al., 2014). Under the assumption that perception and imagery share overlapping neural representations, we built a decoding model from an overt speech condition, and applied this decoder to neural data generated during inner speech. To evaluate performance, the reconstruction in the inner speech condition was compared to the representation of the corresponding original sound spoken out loud—using dynamic time warping (Ellis, 2003)—a temporal realignment algorithm. Results showed that spectrotemporal features of inner speech were decoded with significant predictive accuracy from models built from overt speech data in seven patients (Figure 3A). These findings provided further support that overt and inner speech share underlying neural mechanisms. However, this approach assumes that imagery neural data are generated from a similar neural process as perception. The predictive power of this “cross-condition” model is negatively impacted by discrepancies between perception and imagery neural mechanisms, and is therefore expected to be less optimal compared to directly modeling imagery data in train and test phases.
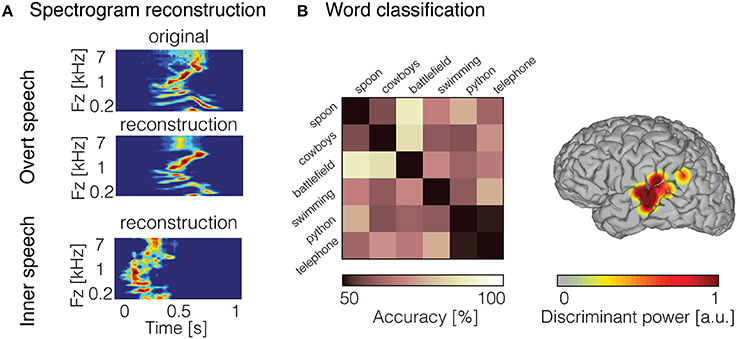
Figure 3. Decoded inner speech representation. (A) Examples of overt speech and inner speech spectrogram reconstruction using linear regression models. Original spectrogram of the recorded overt speech sound is displayed (top panel). Reconstruction of the spectrogram for the overt speech condition (middle panel) and inner speech condition (bottom panel). (B) Examples of word pair classification during inner speech (left panel). Chance level was 50% (diagonal elements), whereas pairwise classification accuracy (off-diagonal elements) reached 88% and was significantly above chancel level across the 15 pairs of word (mean = 69%). Discriminant information displayed on the surface reconstruction of the participant's brain (right panel) for the classification accuracy shown in the left panel. Figures adapted from Martin et al. (2014, 2016) with permissions.
Beyond relatively low-level acoustic representation, invariant phonetic information is extracted from a highly variable continuous acoustic signal at a mid-level neural representation (Chang et al., 2010). During inner speech, behavioral studies have provided evidence that phoneme substitution errors occurred between phonemes sharing similar features (phonemic similarity effect; Corley et al., 2011), and a similar behavior occurs during overt speech. In addition, brain imaging studies have revealed anatomical brain regions involved in silent articulation, such as the sensorimotor cortex, the inferior frontal gyrus, and temporo-parietal brain areas (Pulvermuller et al., 2006). Recently, electrophysiological studies have shown that the neural activity of a listener that perceives a specific phoneme that has been acoustically degraded, replaced or masked by noise is grounded into acoustic neural representations (Holdgraf et al., 2016; Leonard et al., 2016). This phenomenon, called the phonetic masking effect shows that even in the absence of a given speech sound, the neural patterns correlate with those that would have been elicited by the actual speech sound. These findings suggest that phonemes are represented during inner speech in the human cortex. From a decoding perspective, several studies have succeeded in classifying individual inner speech units into different categories, such as covertly articulated vowels (Ikeda et al., 2014), vowels and consonants during covert word production (Pei et al., 2011a), and intended phonemes (Brumberg et al., 2011). These studies represent a proof of concept for basic decoding of individual speech units, but further research is required to define the ability to decode phonemes during continuous, conversational speech.
While several studies have demonstrated phoneme classification during inner speech (Brumberg et al., 2011; Pei et al., 2011a; Tankus et al., 2012; Ikeda et al., 2014), fewer results are available for word-level classification. Words have been decoded during overt speech from neural signals in the inferior frontal gyrus, superior temporal gyrus, and motor areas (Kellis et al., 2010; Pasley et al., 2012; Martin et al., 2014). In recent work, we classified individual words from high frequency activity recorded during an inner speech word repetition task (Martin et al., 2016). To this end, we took advantage of the high temporal resolution offered by ECoG, and classified neural features in the time domain using a support-vector machine model. In order to account for temporal irregularities across trials, we introduced a non-linear time alignment into the classification framework. Pairwise classification results showed that the classification accuracy was significant across five patients. An example of classification accuracy is depicted in Figure 3B (left panel), where the classification accuracy across the 15 pairs of word were above chance level (average across all pairs = 69%; chance level = 50%). Most of the discriminant information came from the posterior temporal gyrus (Figure 3B; right panel). This study represents a proof of concept for basic decoding of speech imagery, and highlights the potential for targeting a speech prosthesis that allows to communicate a few words that are clinically relevant (e.g., hungry, pain, etc.).
Finally, an alternative study that shows further evidence of acoustic processing during imagery comes from a music imagery study. In this study, we investigated the neural encoding of auditory features during imagery using a novel experimental paradigm that allowed direct modeling of auditory imagery data (as opposed to cross-condition) (Martin et al., 2017). This study is not directly related to speech representations, yet it helps understanding the neural representation of inner subjective experiences, such as general auditory imagery. In addition, evidence has shown that music and speech share common brain networks (Callan et al., 2006; Schön et al., 2010). This study relied on a rare clinical case in which a patient undergoing neurosurgery for epilepsy treatment was also an adept piano player. While previous brain imaging studies have identified anatomical regions active during auditory imagery (Zatorre et al., 1996; Griffiths, 1999; Halpern and Zatorre, 1999; Rauschecker, 2001; Halpern et al., 2004; Kraemer et al., 2005), underlying neural tuning to auditory frequencies in imagined sounds was uncharacterized. ECoG activity was recorded during a task that allowed direct alignment of neural response and the spectrotemporal content of the intended music imagery. The patient played two piano pieces with and without auditory feedback of the sound produced by the electronic piano. The audio signal from the keyboard was recorded in synchrony with the ECoG signal, which allowed synchronizing the audio output with neural activity in both conditions. In this task design, it is assumed that the patient's auditory imagery closely matches the output of the keyboard in both timing and spectral content. This study therefore provided a unique opportunity to apply direct (as opposed to cross-condition) receptive field modeling techniques (Aertsen et al., 1981; Clopton and Backoff, 1991; Theunissen et al., 2000; Chi et al., 2005; Pasley et al., 2012), which describe neural response properties, for example auditory frequency tuning. We found robust similarities between perception and imagery neural representations in both frequency and temporal tuning properties in auditory areas. Furthermore, these findings also demonstrated that decoding models, typically applied in neuroprosthetics for motor and visual restoration, are applicable to auditory imagery, representing an important step toward development of algorithms that could be used in neural interfaces for communication based on auditory or speech imagery.
Challenges and Solutions
An important but challenging step in future research is to describe the extent to which speech representations, such as acoustic processing, phonetic encoding and higher level of linguistic functions apply to inner speech. The lack of behavioral output during imagery and inability to monitor the spectrotemporal structure of inner speech represent a major challenge. Critically, inner speech cannot be directly observed by an experimenter. As a consequence, it is complicated to time-lock brain activity to a measurable stimulus or behavioral state, which precludes the use of standard models that assume synchronized input-output data. In addition, natural speech expression is not just operated under conscious control, but is affected by various factors, including gender, emotional state, tempo, pronunciation, and dialect, resulting in temporal irregularities (stretching/compressing, onset/offset delays) across repetitions. As a result, this leads to problems in exploiting the temporal resolution of electrocorticography to investigate inner speech. In this section, we highlight several additional challenges that are encountered when investigating inner speech, as well as new avenues to improve the decoding outcome.
Improving Task Design
The lack of behavioral output and temporal irregularities may be alleviated by designing tasks that maximize the accuracy when labeling the content of inner speech, such as cueing the participants in a rhythmical manner. Despite this, results may still show inconsistencies between the actual cue and the intended speech onset/offset. Alternatively, a verb generation task (Hermes et al., 2014) or picture naming task (Riès et al., 2015) might improve the signal-to-noise ratio, as the cognitive load is more demanding than during a simple word repetition task.
Training Participants
In order to improve accuracy, patients should be familiarized with the tasks before entering in the epilepsy monitoring unit. Indeed, studies have shown that participants with musical training exhibited better pitch and temporal acuity in auditory imagery and enlarged tonotopic maps located in the STG than did participants with little or no musical training (Pantev et al., 1998; Janata and Paroo, 2006; Herholz et al., 2008). As such, we argue it would be beneficial to train subjects on speech imagery, in order to have an increased signal-to-noise ratio and for them to be more consistent in the way of performing the mental imagery. This will improve the performances of any pattern recognition algorithm.
Finding Behavioral Markers
Finding a behavioral or neural metric that allows marking more precisely the inner speech time course would reduce temporal variability during inner speech. This will be increasingly important when moving toward asynchronous protocols, i.e., when patients spontaneously produce inner speech, as opposed to experimental protocols that generally employ timing cues. For instance, behavioral and psychology studies rely on indirect measures to infer the existence and properties of the intended inner experience (Hubbard, 2010). For example, participants were instructed to image the pitch of a sine wave tone for a given instrument, and they had to subsequently judge if the timber of a second presented tone matched the timber of the first one (Crowder, 1989). Response times were faster, when the timbre of the second tone matched the timbre of the first one they had to imagine (see Hubbard, 2010 for a complete review). Therefore, objective monitoring of performance and vividness through external markers may allow certain sources of variability during inner speech to be estimated and accounted for in the modeling process.
Incorporating Speech Recognition Models
Recently, electrophysiological studies on speech decoding have shown promising results by integrating knowledge from the field of speech recognition (Herff et al., 2015; Moses et al., 2016, 2018). Speech recognition has been concerned with the statistical modeling of natural language for many decades, and has faced many problems that are similar to decoding neural pattern associated with speech. As such, we argue that integrating those tools into the field of neuroscience is a necessary element to succeed in the ultimate goal of a clinically reliable speech prosthesis. For instance, speech recognition has developed methodologies that enable the recognition and translation of spoken language into text. This was achieved by incorporating extensive knowledge about how speech is produced and perceived at various phonetic levels (acoustic, auditory, articulatory features), and from advances in computer resources and big data management to build now common applications, such as spellcheck tools, text-to-speech synthesizers, and machine translation programs. Similarly, advanced machine learning models might be more adapted in order to deal with problems associated with speech production temporal irregularities compared to approach like dynamic time warping, which is less robust for noisy data.
Increasing the Amount of Data
More complex models with increasing number of parameters can be used, but require more data to train and evaluate the models. When using electrocorticographic recordings, available data are limited. Experimental paradigms usually do not last long to avoid overloading the patients. As an alternative to traditional protocols, researchers are slowly moving toward continuous brain monitoring during the electrode implantation time. This allows increasing the amount of recorded data and is less constraining to the participant as he or she is recorded in the existing hospital environment, e.g., watching television, interacting with relatives and clinicians, reading, etc. Continuous monitoring of speech perception and production may provide sufficient data to develop more complex and robust decoding models.
Using Unsupervised Learning
The major problem with recording continuous data is how to label precisely the recordings. Indeed, while it is currently possible to monitor conversations with a microphone, the continuous labeling of categories or events during a movie or a dialogue is a tedious process, and often requires human intervention. In addition, as mentioned earlier, monitoring and labeling internal mental states, such as mood, emotions, internal speech, is problematical. We suggest that unsupervised learning methods might be adapted in this context, and alleviate issues associated with speech segmentation. Unsupervised learning is a type of machine learning algorithms that allows drawing inferences from unlabeled responses, i.e., the labels of the observations are not available. This approach has been used in the field of computer vision, such as to learn the features in order to recognize objects (e.g., a car or a motorcycle).
Improving the Electrode Design
Although electrocorticography provides the opportunity to investigate speech neural representation, the configuration, location and duration of implantation are not optimized for experiments, but rather solely for clinical purposes. The design of the intracranial recording electrodes has been shown to be an important factor in motor decoding performance. Namely, the spatial resolution of a cortical surface electrode array depends on the size and spacing of the electrodes, as well as the volume of tissue to which each electrode is sensitive (Wodlinger et al., 2011). Many researchers have attempted to define what the optimal electrode spacing and size could be (Slutzky et al., 2010), but this is still an open area of research. Emerging evidence showed that decoding performance was improved when neural activity was derived from very high-density grids (Blakely et al., 2008; Rouse et al., 2013). However, although a smaller inter-electrode spacing increases the spatial resolution, it poses additional technical issues related to the electrode grid design. Higher density grids placed at specific speech locations would provide higher spatial resolution and potentially enhance the signal's discriminability. Ongoing work in many labs is aimed at increasing the number of recording contacts (Khodagholy et al., 2014) and using biocompatible materials and wireless telemetry for transmission of recordings from multiple electrode implants (Brumberg et al., 2011; Khodagholy et al., 2014). Finally, long-term implantation capability in humans is lacking, as compared to non-human primate studies that showed stable neural decoding for extended periods of time (weeks to months; Ashmore et al., 2012). Reasons for these technical difficulties are the increased impedance leading to loss of signal and increase in the foreign body response to electrodes (Groothuis et al., 2014). Indeed, device material and electrode-architecture influences the tissue reaction. Softer neural implants with shape and elasticity of dura mater increase electrode conductivity and improve the implant-tissue integration (Minev et al., 2015).
Opportunities
Neural decoding models provide a promising research tool to derive data driven conclusions underlying complex speech representations, and for uncovering the link between inner speech representations and neural responses. Quantitative, model-based characterizations have showed that brain activity is tuned to various levels of speech representation.
The various types of language deficits exemplify the challenge in building a specific speech prosthesis that addresses individual needs. In this regard, the first step is to identify injured neural circuits and brain functions. Once damaged and healthy brain functions are identified, decoding models can be used for the design of effective speech prostheses. In particular, the feasibility to decode various speech representations during inner speech—i.e., acoustic features, phonetic representations, and individual words—suggests that various strategies and designs could be employed and combined for building a natural communication device depending on specific, residual speech functions. Every speech representation has pros and cons for targeting speech devices. For instance, decoding acoustic features opens the door to brain-based speech synthesis, in which audible speech is synthetized directly from decoded neural patterns. This approach has already been demonstrated, where predicted speech was synthesized, and acoustically fed back to the user (Guenther et al., 2009; Brumberg et al., 2010) from intracortical brain activity recorded from the motor cortex. Yet the understandability of the produced speech sounds and the best speech parameters to model remain to be demonstrated. Alternatively, decoding units of speech, such as phonemes or words provides greater naturalness, but the optimal speech unit size to be analyzed, is still a matter of debate—i.e., the longer the unit, the larger the database needed to cover the required domain, while smaller units offer more degrees of freedom, and can build a larger set of complex utterances, as shown in Herff et al. (2015) and Moses et al. (2016). A tradeoff is the decoding of a limited vocabulary of words (Martin et al., 2016), which carry specific semantic information, and would be relevant in a basic clinical setting (“hungry,” “thirsty,” “yes,” “no,” etc.).
An alternative to a speech-interface based solely on brain decoding is to build a system, which acquires sensor data from multiple elements of the human speech production system, and combine the different signals to optimize speech synthesis (see Brumberg et al., 2010, for a review). For instance, recording sensors allow characterizing the vocal tract by measuring its configuration directly or by sounding it acoustically using electromagnetic articulography, ultra-sound, or optical imaging of the tongue and lip. Alternatively, electrical measurements can infer articulation from actuator muscle signals [i.e., using surface electromyography (EMG)] or signals obtained directly from the brain (mainly EEG and ECoG). Using different sensors and different speech representations allow exploiting an individual's residual speech functions to operate the speech synthesis.
Unique opportunities for targeting communication assistive technologies are offered by combining different research fields. Neuroscience reveals which anatomical locations and brain signals should be modeled. Linguistic fields support development of decoding models that incorporate linguistic and contextual specifications—including segmental elements and supra-segmental elements. Combining insights from these research fields with machine learning and speech recognition algorithms is a key element to improve prediction accuracy. Finally, the success of speech neuroprostheses depends on the continuous technological improvements to enhance signal quality and resolution, and allow developing more portable and biocompatible invasive recording devices. Merging various fields together will allow tackling the challenges central to decoding inner speech.
Conclusion
To conclude, we described the potential of using decoding models to unravel neural mechanisms associated with complex speech functions. Speech representations during inner speech, such as acoustic features, phonetic features and individual words could be decoded from high frequency neural signals. Although, these results reveal a promising avenue for direct decoding of natural speech, they also emphasize that performance is currently insufficient to build a realistic brain-based device. Accordingly, we highlighted numerous challenges that likely precluded better performances, such as the low signal-to-noise-ratio, and the difficulty in monitoring precisely inner speech. As such challenges are solved, decoding speech directly from neural activity opens the door to new communication interfaces that may allow for more natural speech-like communication in patients with severe communication deficits.
Author Contributions
All authors listed have made a substantial, direct and intellectual contribution to the work, and approved it for publication.
Funding
This research was supported by NINDS Grant R3721135, DARPA D16PC00053.
Conflict of Interest Statement
The authors declare that the research was conducted in the absence of any commercial or financial relationships that could be construed as a potential conflict of interest.
Acknowledgments
This article is adapted from the following doctorate thesis: Understanding and decoding imagined speech using intracranial recordings in the human brain (Martin, 2017).
References
Aertsen, A. M. H. J., Olders, J. H. J., and Johannesma, P. I. M. (1981). Spectro-temporal receptive fields of auditory neurons in the grassfrog: III. analysis of the stimulus-event relation for natural stimuli. Biol. Cybern. 39, 195–209. doi: 10.1007/BF00342772
Ajiboye, A. B., Willett, F. R., Young, D. R., Memberg, W. D., Murphy, B. A., Miller, J. P., et al. (2017). Restoration of reaching and grasping movements through brain-controlled muscle stimulation in a person with tetraplegia: a proof-of-concept demonstration. Lancet 389, 1821–1830. doi: 10.1016/S0140-6736(17)30601-3
Aleman, A. (2004). The functional neuroanatomy of metrical stress evaluation of perceived and imagined spoken words. Cereb. Cortex 15, 221–228. doi: 10.1093/cercor/bhh124
Ashmore, R. C., Endler, B. M., Smalianchuk, I., Degenhart, A. D., Hatsopoulos, N. G., Tyler-Kabara, E. C., et al. (2012). Stable online control of an electrocorticographic brain-computer interface using a static decoder. Conf. Proc. IEEE Eng. Med. Biol. Soc. 2012, 1740–1744. doi: 10.1109/EMBC.2012.6346285
Aziz-Zadeh, L., Cattaneo, L., Rochat, M., and Rizzolatti, G. (2005). Covert speech arrest induced by rTMS over both motor and nonmotor left hemisphere frontal sites. J. Cogn. Neurosci. 17, 928–938. doi: 10.1162/0898929054021157
Blakely, T., Miller, K. J., Rao, R. P. N., Holmes, M. D., and Ojemann, J. G. (2008). Localization and classification of phonemes using high spatial resolution electrocorticography (ECoG) grids. IEEE Eng. Med. Biol. Soc. Conf. 2008, 4964–4967. doi: 10.1109/IEMBS.2008.4650328
Blakely, T., Miller, K. J., Zanos, S. P., Rao, R. P. N., and Ojemann, J. G. (2009). Robust, long-term control of an electrocorticographic brain-computer interface with fixed parameters. Neurosurg. Focus 27:E13. doi: 10.3171/2009.4.FOCUS0977
Bouchard, K. E., Mesgarani, N., Johnson, K., and Chang, E. F. (2013). Functional organization of human sensorimotor cortex for speech articulation. Nature 495, 327–332. doi: 10.1038/nature11911
Brumberg, J. S., Krusienski, D. J., Chakrabarti, S., Gunduz, A., Brunner, P., Ritaccio, A. L., et al. (2016). Spatio-temporal progression of cortical activity related to continuous overt and covert speech production in a reading task. PLoS ONE 11:e0166872. doi: 10.1371/journal.pone.0166872
Brumberg, J. S., Nieto-Castanon, A., Kennedy, P. R., and Guenther, F. H. (2010). Brain–computer interfaces for speech communication. Speech Commun. 52, 367–379. doi: 10.1016/j.specom.2010.01.001
Brumberg, J. S., Wright, E. J., Andreasen, D. S., Guenther, F. H., and Kennedy, P. R. (2011). Classification of intended phoneme production from chronic intracortical microelectrode recordings in speech-motor cortex. Front. Neurosci. 5:65. doi: 10.3389/fnins.2011.00065
Buzsáki, G., Anastassiou, C. A., and Koch, C. (2012). The origin of extracellular fields and currents — EEG, ECoG, LFP and spikes. Nat. Rev. Neurosci. 13, 407–420. doi: 10.1038/nrn3241
Buzsáki, G., and Wang, X.-J. (2012). Mechanisms of gamma oscillations. Annu. Rev. Neurosci. 35, 203–225. doi: 10.1146/annurev-neuro-062111-150444
Callan, D. E., Tsytsarev, V., Hanakawa, T., Callan, A. M., Katsuhara, M., Fukuyama, H., et al. (2006). Song and speech: brain regions involved with perception and covert production. Neuroimage 31, 1327–1342. doi: 10.1016/j.neuroimage.2006.01.036
Chang, R. J. W., Johnson, K., Berger, M. S., Barbaro, N. M., and Knight, R. T. (2010). Categorical speech representation in human superior temporal gyrus. Nat. Neurosci. 13, 1428–1432. doi: 10.1038/nn.2641
Cheung, C., Hamiton, L. S., Johnson, K., and Chang, E. F. (2016). The auditory representation of speech sounds in human motor cortex. eLife 5:12577. doi: 10.7554/eLife.12577
Chi, T., Ru, P., and Shamma, S. A. (2005). Multiresolution spectrotemporal analysis of complex sounds. J. Acoust. Soc. Am. 118:887. doi: 10.1121/1.1945807
Clopton, B. M., and Backoff, P. M. (1991). Spectrotemporal receptive fields of neurons in cochlear nucleus of guinea pig. Hear. Res. 52, 329–344.
Conant, D. F., Bouchard, K. E., Leonard, M. K., and Chang, E. F. (2018). Human sensorimotor cortex control of directly measured vocal tract movements during vowel production. J. Neurosci. 38, 2955–2966. doi: 10.1523/JNEUROSCI.2382-17.2018
Corley, M., Brocklehurst, P. H., and Moat, H. S. (2011). Error biases in inner and overt speech: evidence from tongue twisters. J. Exp. Psychol. Learn. Mem. Cogn. 37, 162–175. doi: 10.1037/a0021321
Crowder, R. G. (1989). Imagery for musical timbre. J. Exp. Psychol. Hum. Percept. Perform. 15, 472–478. doi: 10.1037/0096-1523.15.3.472
Démonet, J.-F., Thierry, G., and Cardebat, D. (2005). Renewal of the neurophysiology of language: functional neuroimaging. Physiol. Rev. 85, 49–95. doi: 10.1152/physrev.00049.2003
Ding, N., and Simon, J. Z. (2012). Emergence of neural encoding of auditory objects while listening to competing speakers. Proc. Natl. Acad. Sci. U.S.A. 109, 11854–11859. doi: 10.1073/pnas.1205381109
Ellis, D. (2003). Dynamic Time Warping (DTW) in Matlab. Available online at: http://www.ee.columbia.edu/~dpwe/resources/matlab/dtw/ (Accessed November 21, 2014).
Farwell, L. A., and Donchin, E. (1988). Talking off the top of your head: toward a mental prosthesis utilizing event-related brain potentials. Electroencephalogr. Clin. Neurophysiol. 70, 510–523.
Fazel-Rezai, R., Allison, B. Z., Guger, C., Sellers, E. W., Kleih, S. C., and Kübler, A. (2012). P300 brain computer interface: current challenges and emerging trends. Front. Neuroeng. 5:14. doi: 10.3389/fneng.2012.00014
Felton, E. A., Wilson, J. A., Williams, J. C., and Garell, P. C. (2007). Electrocorticographically controlled brain-computer interfaces using motor and sensory imagery in patients with temporary subdural electrode implants. Report of four cases. J. Neurosurg. 106, 495–500. doi: 10.3171/jns.2007.106.3.495
Geva, S., Correia, M., and Warburton, E. A. (2011). Diffusion tensor imaging in the study of language and aphasia. Aphasiology 25, 543–558. doi: 10.1080/02687038.2010.534803
Gilja, V., Pandarinath, C., Blabe, C. H., Nuyujukian, P., Simeral, J. D., Sarma, A. A., et al. (2015). Clinical translation of a high-performance neural prosthesis. Nat. Med. 21, 1142–1145. doi: 10.1038/nm.3953
Giraud, A.-L., and Poeppel, D. (2012). Cortical oscillations and speech processing: emerging computational principles and operations. Nat. Neurosci. 15, 511–517. doi: 10.1038/nn.3063
Groothuis, J., Ramsey, N. F., Ramakers, G. M. J., and van der Plasse, G. (2014). Physiological challenges for intracortical electrodes. Brain Stimulat. 7, 1–6. doi: 10.1016/j.brs.2013.07.001
Guenther, F. H., Brumberg, J. S., Wright, E. J., Nieto-Castanon, A., Tourville, J. A., Panko, M., et al. (2009). A wireless brain-machine interface for real-time speech synthesis. PLoS ONE 4:e8218. doi: 10.1371/journal.pone.0008218
Halpern, A. R., and Zatorre, R. J. (1999). When that tune runs through your head: a PET investigation of auditory imagery for familiar melodies. Cereb. Cortex 9, 697–704. doi: 10.1093/cercor/9.7.697
Halpern, A. R., Zatorre, R. J., Bouffard, M., and Johnson, J. A. (2004). Behavioral and neural correlates of perceived and imagined musical timbre. Neuropsychologia 42, 1281–1292. doi: 10.1016/j.neuropsychologia.2003.12.017
Herff, C., Heger, D., de Pesters, A., Telaar, D., Brunner, P., Schalk, G., et al. (2015). Brain-to-text: decoding spoken phrases from phone representations in the brain. Front. Neurosci. 9:217. doi: 10.3389/fnins.2015.00217
Herholz, S. C., Lappe, C., Knief, A., and Pantev, C. (2008). Neural basis of music imagery and the effect of musical expertise. Eur. J. Neurosci. 28, 2352–2360. doi: 10.1111/j.1460-9568.2008.06515.x
Hermes, D., Miller, K. J., Vansteensel, M. J., Edwards, E., Ferrier, C. H., Bleichner, M. G., et al. (2014). Cortical theta wanes for language. Neuroimage 85, 738–748. doi: 10.1016/j.neuroimage.2013.07.029
Hickok, G., and Poeppel, D. (2007). The cortical organization of speech processing. Nat. Rev. Neurosci. 8, 393–402. doi: 10.1038/nrn2113
Hinke, R. M., Hu, X., Stillman, A. E., Kim, S. G., Merkle, H., Salmi, R., et al. (1993). Functional magnetic resonance imaging of Broca's area during internal speech. Neuroreport 4, 675–678.
Holdgraf, C. R., de Heer, W., Pasley, B., Rieger, J., Crone, N., Lin, J. J., et al. (2016). Rapid tuning shifts in human auditory cortex enhance speech intelligibility. Nat. Commun. 7:13654. doi: 10.1038/ncomms13654
Holdgraf, C. R., Rieger, J. W., Micheli, C., Martin, S., Knight, R. T., and Theunissen, F. E. (2017). Encoding and decoding models in cognitive electrophysiology. Front. Syst. Neurosci. 11:61. doi: 10.3389/fnsys.2017.00061
Hubbard, T. L. (2010). Auditory imagery: Empirical findings. Psychol. Bull. 136, 302–329. doi: 10.1037/a0018436
Ikeda, S., Shibata, T., Nakano, N., Okada, R., Tsuyuguchi, N., Ikeda, K., et al. (2014). Neural decoding of single vowels during covert articulation using electrocorticography. Front. Hum. Neurosci. 8:125. doi: 10.3389/fnhum.2014.00125
Janata, P., and Paroo, K. (2006). Acuity of auditory images in pitch and time. Percept. Psychophys. 68, 829–844. doi: 10.3758/BF03193705
Jarosiewicz, B., Sarma, A. A., Bacher, D., Masse, N. Y., Simeral, J. D., Sorice, B., et al. (2015). Virtual typing by people with tetraplegia using a self-calibrating intracortical brain-computer interface. Sci. Transl. Med. 7:313ra179. doi: 10.1126/scitranslmed.aac7328
Kapeller, C., Kamada, K., Ogawa, H., Prueckl, R., Scharinger, J., and Guger, C. (2014). An electrocorticographic BCI using code-based VEP for control in video applications: a single-subject study. Front. Syst. Neurosci. 8:139. doi: 10.3389/fnsys.2014.00139
Kay, K. N., and Gallant, J. L. (2009). I can see what you see. Nat. Neurosci. 12:245. doi: 10.1038/nn0309-245
Kay, K. N., Naselaris, T., Prenger, R. J., and Gallant, J. L. (2008). Identifying natural images from human brain activity. Nature 452, 352–355. doi: 10.1038/nature06713
Kellis, S., Miller, K., Thomson, K., Brown, R., House, P., and Greger, B. (2010). Decoding spoken words using local field potentials recorded from the cortical surface. J. Neural Eng. 7:056007. doi: 10.1088/1741-2560/7/5/056007
Khodagholy, D., Gelinas, J. N., Thesen, T., Doyle, W., Devinsky, O., Malliaras, G. G., et al. (2014). NeuroGrid: recording action potentials from the surface of the brain. Nat. Neurosci. 18, 310–315. doi: 10.1038/nn.3905
Kraemer, D. J. M., Macrae, C. N., Green, A. E., and Kelley, W. M. (2005). Musical imagery: Sound of silence activates auditory cortex. Nature 434:158. doi: 10.1038/434158a
Kubanek, J., Brunner, P., Gunduz, A., Poeppel, D., and Schalk, G. (2013). The tracking of speech envelope in the human cortex. PLoS ONE 8:e53398. doi: 10.1371/journal.pone.0053398
Lachaux, J.-P., Axmacher, N., Mormann, F., Halgren, E., and Crone, N. E. (2012). High-frequency neural activity and human cognition: past, present and possible future of intracranial EEG research. Prog. Neurobiol. 98, 279–301. doi: 10.1016/j.pneurobio.2012.06.008
Leonard, M. K., Baud, M. O., Sjerps, M. J., and Chang, E. F. (2016). Perceptual restoration of masked speech in human cortex. Nat. Commun. 7:13619. doi: 10.1038/ncomms13619
Luo, H., and Poeppel, D. (2007). Phase patterns of neuronal responses reliably discriminate speech in human auditory cortex. Neuron 54, 1001–1010. doi: 10.1016/j.neuron.2007.06.004
Leuthardt, E. C., Miller, K. J., Schalk, G., Rao, R. P. N., and Ojemann, J. G. (2006). Electrocorticography-based brain computer interface–the Seattle experience. IEEE Eng. Med. Biol. Soc. 14, 194–198. doi: 10.1109/TNSRE.2006.875536
Leuthardt, E. C., Pei, X.-M., Breshears, J., Gaona, C., Sharma, M., Freudenberg, Z., et al. (2012). Temporal evolution of gamma activity in human cortex during an overt and covert word repetition task. Front. Hum. Neurosci. 6:99. doi: 10.3389/fnhum.2012.00099
Leuthardt, S. G., Wolpaw, J. R., Ojemann, J. G., and Moran, D. W. (2004). A brain–computer interface using electrocorticographic signals in humans. J. Neural Eng. 1, 63–71. doi: 10.1088/1741-2560/1/2/001
Lewis, P. M., Ackland, H. M., Lowery, A. J., and Rosenfeld, J. V. (2015). Restoration of vision in blind individuals using bionic devices: a review with a focus on cortical visual prostheses. Brain Res. 1595, 51–73. doi: 10.1016/j.brainres.2014.11.020
Manning, J. R., Jacobs, J., Fried, I., and Kahana, M. J. (2009). Broadband shifts in local field potential power spectra are correlated with single-neuron spiking in humans. J. Neurosci. 29, 13613–13620. doi: 10.1523/JNEUROSCI.2041-09.2009
Marshall, L., Helgadóttir, H., Mölle, M., and Born, J. (2006). Boosting slow oscillations during sleep potentiates memory. Nature 444, 610–613. doi: 10.1038/nature05278
Martin, S. (2017). Understanding and Decoding Imagined Speech using Electrocorticographic Recordings in Humans. Ecole Polytechnique Fédérale de Lausanne.
Martin, S., Brunner, P., Holdgraf, C., Heinze, H.-J., Crone, N. E., Rieger, J., et al. (2014). Decoding spectrotemporal features of overt and covert speech from the human cortex. Front. Neuroengineering 7:14. doi: 10.3389/fneng.2014.00014
Martin, S., Brunner, P., Iturrate, I., del Millán, J. R., Schalk, G., Knight, R. T., et al. (2016). Word pair classification during imagined speech using direct brain recordings. Sci. Rep. 6:25803. doi: 10.1038/srep25803
Martin, S., Mikutta, C., Leonard, M. K., Hungate, D., Koelsch, S., Shamma, S., et al. (2017). Neural encoding of auditory features during music perception and imagery. Cereb. Cortex 27, 1–12. doi: 10.1093/cercor/bhx277
McGuire, P. K., Silbersweig, D. A., Murray, R. M., David, A. S., Frackowiak, R. S., and Frith, C. D. (1996). Functional anatomy of inner speech and auditory verbal imagery. Psychol. Med. 26, 29–38.
Mesgarani, N., Cheung, C., Johnson, K., and Chang, E. F. (2014). Phonetic feature encoding in human superior temporal gyrus. Science 343, 1006–1010. doi: 10.1126/science.1245994
Millán, G. F., Vanhooydonck, D., Lew, E., Philips, J., and Nuttin, M. (2009). Asynchronous non-invasive brain-actuated control of an intelligent wheelchair. IEEE Eng. Med. Biol. Soc. Conf. 2009, 3361–3364. doi: 10.1109/IEMBS.2009.5332828
Miller, K. J., Leuthardt, E. C., Schalk, G., Rao, R. P. N., Anderson, N. R., Moran, D. W., et al. (2007). Spectral changes in cortical surface potentials during motor movement. J. Neurosci. 27, 2424–2432. doi: 10.1523/JNEUROSCI.3886-06.2007
Minev, I. R., Musienko, P., Hirsch, A., Barraud, Q., Wenger, N., Moraud, E. M., et al. (2015). Electronic dura mater for long-term multimodal neural interfaces. Science 347, 159–163. doi: 10.1126/science.1260318
Moses, D. A., Leonard, M. K., and Chang, E. F. (2018). Real-time classification of auditory sentences using evoked cortical activity in humans. J. Neural Eng. 15:036005. doi: 10.1088/1741-2552/aaab6f
Moses, D. A., Mesgarani, N., Leonard, M. K., and Chang, E. F. (2016). Neural speech recognition: continuous phoneme decoding using spatiotemporal representations of human cortical activity. J. Neural Eng. 13:056004. doi: 10.1088/1741-2560/13/5/056004
Naselaris, T., Kay, K. N., Nishimoto, S., and Gallant, J. L. (2011). Encoding and decoding in fMRI. NeuroImage 56, 400–410. doi: 10.1016/j.neuroimage.2010.07.073
Nijboer, F., Sellers, E. W., Mellinger, J., Jordan, M. A., Matuz, T., Furdea, A., et al. (2008). A P300-based brain-computer interface for people with amyotrophic lateral sclerosis. Clin. Neurophysiol. 119, 1909–1916. doi: 10.1016/j.clinph.2008.03.034
Palmer, E. D., Rosen, H. J., Ojemann, J. G., Buckner, R. L., Kelley, W. M., and Petersen, S. E. (2001). An event-related fMRI study of overt and covert word stem completion. Neuroimage 14, 182–193. doi: 10.1006/nimg.2001.0779
Pandarinath, C., Nuyujukian, P., Blabe, C. H., Sorice, B. L., Saab, J., Willett, F. R., et al. (2017). High performance communication by people with paralysis using an intracortical brain-computer interface. eLife 6:e18554. doi: 10.7554/eLife.18554
Pantev, C., Oostenveld, R., Engelien, A., Ross, B., Roberts, L. E., and Hoke, M. (1998). Increased auditory cortical representation in musicians. Nature 392, 811–814. doi: 10.1038/33918
Pasley, B., Crone, N., Knight, R., and Chang, E. (2011). Phonetic encoding by intracranial signals in human auditory cortex. Front. Hum. Neurosci. 5:287. doi: 10.3389/conf.fnhum.2011.207.00287
Pasley, B. N., David, S. V., Mesgarani, N., Flinker, A., Shamma, S. A., Crone, N. E., et al. (2012). Reconstructing speech from human auditory cortex. PLoS Biol. 10:e1001251. doi: 10.1371/journal.pbio.1001251
Pasley, B. N., and Knight, R. T. (2013). “Decoding speech for understanding and treating aphasia,” in Progress in Brain Research (Elsevier), 435–456. Available online at: http://linkinghub.elsevier.com/retrieve/pii/B9780444633279000187 (Accessed May 3, 2015).
Pei, B. D. L., Leuthardt, E. C., and Schalk, G. (2011a). Decoding vowels and consonants in spoken and imagined words using electrocorticographic signals in humans. J. Neural Eng. doi: 10.1088/1741-2560/8/4/046028
Pei, X., Leuthardt, E. C., Gaona, C. M., Brunner, P., Wolpaw, J. R., and Schalk, G. (2011b). Spatiotemporal dynamics of electrocorticographic high gamma activity during overt and covert word repetition. Neuroimage 54, 2960–2972. doi: 10.1016/j.neuroimage.2010.10.029
Perrone-Bertolotti, M., Rapin, L., Lachaux, J.-P., Baciu, M., and Lœvenbruck, H. (2014). What is that little voice inside my head? Inner speech phenomenology, its role in cognitive performance, and its relation to self-monitoring. Behav. Brain Res. 261, 220–239. doi: 10.1016/j.bbr.2013.12.034
Price, C. J. (2000). The anatomy of language: contributions from functional neuroimaging. J. Anat. 197, 335–359. doi: 10.1046/j.1469-7580.2000.19730335.x
Pulvermuller, F., Huss, M., Kherif, F., Moscoso del Prado Martin, F., Hauk, O., and Shtyrov, Y. (2006). Motor cortex maps articulatory features of speech sounds. Proc. Natl. Acad. Sci. U.S.A. 103, 7865–7870. doi: 10.1073/pnas.0509989103
Rauschecker, J. P. (2001). Cortical plasticity and music. Ann. N. Y. Acad. Sci. 930, 330–336. doi: 10.1111/j.1749-6632.2001.tb05742.x
Rich, E. L., and Wallis, J. D. (2017). Spatiotemporal dynamics of information encoding revealed in orbitofrontal high-gamma. Nat. Commun. 8:1139. doi: 10.1038/s41467-017-01253-5
Riès, S. K., Karzmark, C. R., Navarrete, E., Knight, R. T., and Dronkers, N. F. (2015). Specifying the role of the left prefrontal cortex in word selection. Brain Lang. 149, 135–147. doi: 10.1016/j.bandl.2015.07.007
Ritaccio, A., Matsumoto, R., Morrell, M., Kamada, K., Koubeissi, M., Poeppel, D., et al. (2015). Proceedings of the seventh international workshop on advances in electrocorticography. Epilepsy Behav. 51, 312–320. doi: 10.1016/j.yebeh.2015.08.002
Rosen, H. J., Ojemann, J. G., Ollinger, J. M., and Petersen, S. E. (2000). Comparison of brain activation during word retrieval done silently and aloud using fMRI. Brain Cogn. 42, 201–217. doi: 10.1006/brcg.1999.1100
Rouse, A. G., Williams, J. J., Wheeler, J. J., and Moran, D. W. (2013). Cortical adaptation to a chronic micro-electrocorticographic brain computer interface. J. Neurosci. 33, 1326–1330. doi: 10.1523/JNEUROSCI.0271-12.2013
Schalk, G., Kubánek, J., Miller, K. J., Anderson, N. R., Leuthardt, E. C., Ojemann, J. G., et al. (2007). Decoding two-dimensional movement trajectories using electrocorticographic signals in humans. J. Neural Eng. 4, 264–275. doi: 10.1088/1741-2560/4/3/012
Schön, D., Gordon, R., Campagne, A., Magne, C., Astésano, C., Anton, J.-L., et al. (2010). Similar cerebral networks in language, music and song perception. Neuroimage 51, 450–461. doi: 10.1016/j.neuroimage.2010.02.023
Slutzky, M. W., Jordan, L. R., Krieg, T., Chen, M., Mogul, D. J., and Miller, L. E. (2010). Optimal spacing of surface electrode arrays for brain–machine interface applications. J. Neural Eng. 7:026004. doi: 10.1088/1741-2560/7/2/026004
Srinivasan, R., Bibi, F. A., and Nunez, P. L. (2006). Steady-state visual evoked potentials: distributed local sources and wave-like dynamics are sensitive to flicker frequency. Brain Topogr. 18, 167–187. doi: 10.1007/s10548-006-0267-4
Tankus, A., Fried, I., and Shoham, S. (2012). Structured neuronal encoding and decoding of human speech features. Nat. Commun. 3:1015. doi: 10.1038/ncomms1995
Theunissen, F. E., Sen, K., and Doupe, A. J. (2000). Spectral-temporal receptive fields of nonlinear auditory neurons obtained using natural sounds. J. Neurosci. 20, 2315–2331. doi: 10.1523/JNEUROSCI.20-06-02315.2000
Vansteensel, M. J., Pels, E. G. M., Bleichner, M. G., Branco, M. P., Denison, T., Freudenburg, Z. V., et al. (2016). Fully implanted brain–computer interface in a locked-in patient with ALS. N. Engl. J. Med. 375, 2060–2066. doi: 10.1056/NEJMoa1608085
Wang, W., Collinger, J. L., Degenhart, A. D., Tyler-Kabara, E. C., Schwartz, A. B., Moran, D. W., et al. (2013). An electrocorticographic brain interface in an individual with tetraplegia. PLoS ONE 8:e55344. doi: 10.1371/journal.pone.0055344
Whittingstall, K., and Logothetis, N. K. (2009). Frequency-band coupling in surface EEG reflects spiking activity in monkey visual cortex. Neuron 64, 281–289. doi: 10.1016/j.neuron.2009.08.016
Wodlinger, B., Degenhart, A. D., Collinger, J. L., Tyler-Kabara, E. C., and Wei, W. (2011). The impact of electrode characteristics on electrocorticography (ECoG). Conf Proc IEEE Eng Med Biol Soc. 2011, 3083–3086. doi: 10.1109/IEMBS.2011.6090842
Wolpaw, J. R., McFarland, D. J., Neat, G. W., and Forneris, C. A. (1991). An EEG-based brain-computer interface for cursor control. Electroencephalogr. Clin. Neurophysiol. 78, 252–259.
Yetkin, F. Z., Hammeke, T. A., Swanson, S. J., Morris, G. L., Mueller, W. M., McAuliffe, T. L., et al. (1995). A comparison of functional MR activation patterns during silent and audible language tasks. AJNR Am. J. Neuroradiol. 16, 1087–1092.
Zatorre, R. J., Halpern, A. R., Perry, D. W., Meyer, E., and Evans, A. C. (1996). Hearing in the mind's ear: a PET investigation of musical imagery and perception. J. Cogn. Neurosci. 8, 29–46. doi: 10.1162/jocn.1996.8.1.29
Keywords: inner speech, electrocorticography, decoding, neuroprosthetics, brain-computer interface
Citation: Martin S, Iturrate I, Millán JdR, Knight RT and Pasley BN (2018) Decoding Inner Speech Using Electrocorticography: Progress and Challenges Toward a Speech Prosthesis. Front. Neurosci. 12:422. doi: 10.3389/fnins.2018.00422
Received: 28 February 2018; Accepted: 04 June 2018;
Published: 21 June 2018.
Edited by:
Christoph Guger, Guger Technologies, AustriaReviewed by:
Christian Herff, University of Bremen, GermanyJonas Obleser, Universität zu Lübeck, Germany
Copyright © 2018 Martin, Iturrate, Millán, Knight and Pasley. This is an open-access article distributed under the terms of the Creative Commons Attribution License (CC BY). The use, distribution or reproduction in other forums is permitted, provided the original author(s) and the copyright owner are credited and that the original publication in this journal is cited, in accordance with accepted academic practice. No use, distribution or reproduction is permitted which does not comply with these terms.
*Correspondence: Stephanie Martin, bWFydGluLnN0ZXBoYW5pZS5iQGdtYWlsLmNvbQ==