- 1School of Biomedical Engineering, Health Science Center, Shenzhen University, Shenzhen, China
- 2Clinical Research Center for Neurological Diseases, Shenzhen University, Shenzhen, China
- 3Department of Pain Medicine and Shenzhen Municipal Key Laboratory for Pain Medicine, Shenzhen Sixth Hospital of Guangdong Medical University, Shenzhen, China
- 4Department of Neurosurgery, Shenzhen University General Hospital, Shenzhen, China
Herpes zoster (HZ) can cause a blistering skin rash with severe neuropathic pain. Pharmacotherapy is the most common treatment for HZ patients. However, most patients are usually the elderly or those that are immunocompromised, and thus often suffer from side effects or easily get intractable post-herpetic neuralgia (PHN) if medication fails. It is challenging for clinicians to tailor treatment to patients, due to the lack of prognosis information on the neurological pathogenesis that underlies HZ. In the current study, we aimed at characterizing the brain structural pattern of HZ before treatment with medication that could help predict medication responses. High-resolution structural magnetic resonance imaging (MRI) scans of 14 right-handed HZ patients (aged 61.0 ± 7.0, 8 males) with poor response and 15 (aged 62.6 ± 8.3, 5 males) age- (p = 0.58), gender-matched (p = 0.20) patients responding well, were acquired and analyzed. Multivoxel pattern analysis (MVPA) with a searchlight algorithm and support vector machine (SVM), was applied to identify the spatial pattern of the gray matter (GM) volume, with high predicting accuracy. The predictive regions, with an accuracy higher than 79%, were located within the cerebellum, posterior insular cortex (pIC), middle and orbital frontal lobes (mFC and OFC), anterior and middle cingulum (ACC and MCC), precuneus (PCu) and cuneus. Among these regions, mFC, pIC and MCC displayed significant increases of GM volumes in patients with poor response, compared to those with a good response. The combination of sMRI and MVPA might be a useful tool to explore the neuroanatomical imaging biomarkers of HZ-related pain associated with medication responses.
Introduction
Resulting from the reactivation of a latent varicella-zoster virus, herpes zoster (HZ) is characterized by a unilateral, localized painful blistering skin rash with the complication of pain varying from burning, tingling, allodynia to hyperalgesia (Johnson and Rice, 2014; Hadley et al., 2016). The primary objectives of treating HZ are to accelerate the healing of skin lesions, and more importantly, to reduce the duration of zoster-associated pain, in order to lower the risk of progression to post-herpetic neuralgia (PHN) (Johnson and Rice, 2014). Medication treatment with central nervous system drugs is commonly used to ease the pain of HZ patients (Harden et al., 2013). Most HZ patients are middle-aged or elderly people with severe pain due to their lower immunity (Jung et al., 2004). They are more likely to suffer from multiple side effects of medication and have poor treatment responses (Jung et al., 2004). However, a great challenge remains for clinicians to evaluate the medication responses, before planning precise treatment protocols, which could further delay the proper treatment exposing patients to the high risk of getting intractable PHN (Hadley et al., 2016). In order to promote the efficiency of medication treatment, as well as to ease the economic and mental burden of HZ patients, it is necessary to explore the neural biomarkers with prognostic value in medication treatment.
Over the last decade, functional MRI (fMRI) has been increasingly applied in studying abnormal brain activity of HZ and PHN patients (Geha et al., 2007, 2008; Liu et al., 2013; Zhang et al., 2014; Jiang et al., 2016; Cao et al., 2017a, 2018; Yu and Yu, 2017; Hong et al., 2018). By contrast, few structural changes of these patients, which potentially underlie the functional abnormalities, have been explored. Recently, a study applying the voxel-based morphometry (VBM) method in structural magnetic resonance imaging (sMRI) (Cao et al., 2018), reported that the development from HZ to PHN was associated with decreased gray matter (GM) volumes in the hippocampus, superior and medial frontal gyrus, thalamus, occipital lobe, and the parietal lobe, as well as increased GM volumes in the bilateral cerebellum, inferior and the middle temporal gyrus. We supposed that the dynamic changes in brain structure not only manifests in the development of HZ to PHN but also occurs at the early initiation of HZ, which may mediate the responses of patients to medication.
Voxel-based morphometry is the most widely used technique to study regional cerebral volume or tissue concentration difference in sMRI analysis (Ashburner and Friston, 2000; Good et al., 2001). As a univariate method, it performs statistical analysis at each voxel separately, either in a ROI-wise or whole brain data-driven manner. There are drawbacks in both methods of analysis. The ROI approach often requires a prior hypothesis of potential pathophysiological brain regions, while whole brain voxel-wise analysis with the restriction of multiple comparisons would cause a loss of sensitivity (Hendler et al., 2014). They are also subjected to a large sample size to attain statistical power. Further, VBM overlooks the dependency of the focal set of voxels in localizing informative regions relevant to anatomical differences of brains (Haynes and Rees, 2006). To overcome these shortcomings, multivoxel pattern analysis (MVPA) has been proposed to take advantage of multiple voxels’ information in depicting the pattern of the human brain (Ecker et al., 2010; Bendfeldt et al., 2012; Haxby, 2012). MVPA has become increasingly popular in neuroimaging research, because it has great benefits, including its efficiency in detecting subtle anatomical differences (Ashburner, 2009; Uddin et al., 2011; Liu et al., 2012; Zhang et al., 2018), and a greater sensitivity and specificity than mass-univariate analyses, with generally complementary results (Haynes and Rees, 2005; Kamitani and Tong, 2005; Jimura and Poldrack, 2012).
Machine learning (ML) based MVPA has been used in previous studies to classify patients from healthy controls (Klöppel et al., 2015; Wolfers et al., 2015; Zhang et al., 2018), or to predict which patients might have different medication responses (Liu et al., 2012; Qin et al., 2015) with high sensitivity and specificity. Of note, the high dimensionality of neuroimaging data is a big challenge when applying ML methods, because voxel-wise features greatly exceed the sample size. In order to achieve a fully data-driven, whole-brain classification based on MVPA, we chose to use the searchlight method combined with the commonly used ML classifier, support vector machine (SVM), in the current study. With each voxel as the center, a searchlight is defined with a spherical set of voxels and then used to train and test SVM. Accuracy of this classifier is assigned to the central voxel of the sphere. Finally, a parametric accuracy map is created and used to identify significant brain regions in classification (Kriegeskorte et al., 2006). The searchlight method is appealing in the following aspects: (a) minimizing the effect of curse of dimensionality (each searchlight includes a few voxels); (b) takes advantage of information from multiple adjacent voxels in pattern detection; (c) produces a whole-brain result map that is superficially similar in appearance to the whole-brain significance maps produced by more familiar mass-univariate analyses (based on the general linear model) (Etzel et al., 2013).
In the present study, we aimed to explore the relationship between potential structural changes and medication responses in HZ patients. We hypothesized that the combination of the sMRI and MVPA technique could detect brain structural differences between HZ patients with different medication responses.
Materials and Methods
Subjects
This study was approved by the Ethics Committee of the Shenzhen Sixth Hospital of Guangdong Medical University. In total, 36 subjects were recruited from patients at their first initiation of HZ in this hospital from 1 January 2017 to 30 August 2018. Diagnosis of central neuralgia was performed by experienced clinicians in the Department of Pain Medicine of the Shenzhen Sixth Hospital of Guangdong Medical University, according to general practice guidelines (Jeon, 2015). Pain severity in these patients was evaluated via a 10-point visual analog scale (VAS) every day after hospitalization. Specifically, pain intensity assessed right before MRI scanning and after treatment were termed as pre-scanning VAS and post-treatment VAS, respectively. Since the MRI scan could not be arranged while the patients were hospitalized, MRI data were generally acquired within 3 days after patients were hospitalized, at which time they could already have been medicated or not. For those who were already medicated, two experienced clinicians measured VAS and made sure that effective changes in pain intensity had not occurred before MRI acquisition, which meant that pain intensity in these patients was approximately the same as that before medication. Inclusion criteria were: age between 55 and 79 years old; right-handed; no history of psychiatric or neurological disorders; a primary symptom of HZ-related acute pain (duration of less than 1 month); and a pre-scanning VAS higher than five. Each patient provided written informed consent according to the Declaration of Helsinki.
All patients were administrated according to the standardized medication protocol by experienced physicians for a week. This protocol was normally an individually calibrated with prescriptions of the following systemic central acting agents: the anticonvulsants gabapentin and pregabalin; and/or the tricyclic antidepressants amitriptyline. After a one-week treatment, the HZ patients with a reduction of VAS less than three were defined as having medication-resistant pain (MRP), while others were defined as having medication-sensitive pain (MSP).
Demographic and clinical data were compared between the two groups using two-sample t-tests or a Chi-square test in the Statistical Package for Social Science, version 19 (SPSS Inc., United States). The threshold level of significance was set at p < 0.05.
MRI Acquisition
Magnetic resonance imaging scanning was performed on a Siemens Skyra 3.0T scanner with an 8-channel head coil in the Shenzhen Sixth Hospital of Guangdong Medical University. All subjects were instructed to remain still and awake with their eyes closed during scanning. High-resolution T1-weighted structural images were obtained using a Siemens 3D MPRAGE sequence with the following parameters: 320 slices, slice thickness = 0.6 mm, TR/TE = 1900/2.12 ms, field of view = 256 × 256 mm2, data matrix = 448 × 448, spatial resolution = 0.57 × 0.57 × 0.60 mm3, inversion time = 900 ms, flipped angle = 9°.
MRI Data Analysis
All sMRI images were visually inspected and those with severe motion artifacts and/or visible anatomical deformation were excluded. We calculated the GM maps using the VBM toolbox1 in SPM8 (Version 6313, Wellcome Department of Imaging Neuroscience, London, United Kingdom2) imbedded in MATLAB R2013a. The VBM procedure is described briefly as follows: (1) registration to Montreal Neurological Institute (MNI) stereotactic space; (2) segmentation into three types of tissues, namely GM, white matter and cerebrospinal fluid; (3) creation of a study-specific template via the high-dimensional Diffeomorphic Anatomical Registration Through Exponentiated Lie Algebra (DARTEL) algorithm; (4) non-linear registration to the DARTEL existing template; (5) modulation to preserve the total volume of each brain tissue; (6) smoothing using a Gaussian kernel with full-width-half-maximum (FWHM) of 8 mm. Smoothing is a standard step in the VBM analysis pipeline, to render the data more normally distributed and to compensate for the inexact nature of the spatial normalization (Mechelli et al., 2005).
The MVPA technique implemented in this study a combined searchlight algorithm and support vector machine (SVM) (Uddin et al., 2011). Generally, a searchlight algorithm, which considers the information of multiple voxels, can be more sensitive to group differences over traditional univariate measures (Ecker et al., 2010; Bendfeldt et al., 2012). In our study, MVPA was performed on the smoothed GM maps obtained in the VBM procedure. The flowchart of MVPA based classification is shown in Figure 1 and the details of the MVPA procedure are as follows. First, at each voxel (Vi), a sphere with 5-mm radius was defined centering at Vi. Notice that previous studies defined the radius by experience (Uddin et al., 2011; Liu et al., 2012; Zhang et al., 2018). In our study, we tested different radii (e.g., 8, 10, and 12 mm) and found no significant difference among the ultimate results (Supplementary Figure S1). Besides, a large sphere radius would result in the omission of some subtle spatial pattern information. Thus, we chose a small radius (5 mm) to present the results. First, a high-dimensional feature vector was acquired by extracting the values of all 171 voxels in the sphere. Second, with such a feature vector, a non-linear support vector machine (SVM) with radial basis function (RBF) was applied to predict medication responses using the LIBSVM software3. The parameters were set to default to construct the SVM model for each Vi. Then leave-one-out cross-validation (LOOCV) was adopted to yield the classification accuracy of Vi. We split all subjects into a training set (N-1 subjects, N denotes the number of all subjects) and a testing set (the remaining subject). Then two feature matrices M(N1)∗v and MN2∗v (N1, N2, and V denote the numbers of subjects in the training and testing sets, and the number of voxels in the sphere) representative of the spatial patterns of the two data sets were obtained using the aforementioned high-dimensional feature vectors. Input features of training data were normalized and used to construct an SVM model. After repeating this procedure for all voxels in gray matter, a three-dimensional accuracy map was finally gained to represent the structural pattern of the discriminating ability of classifying MRP from MSP.
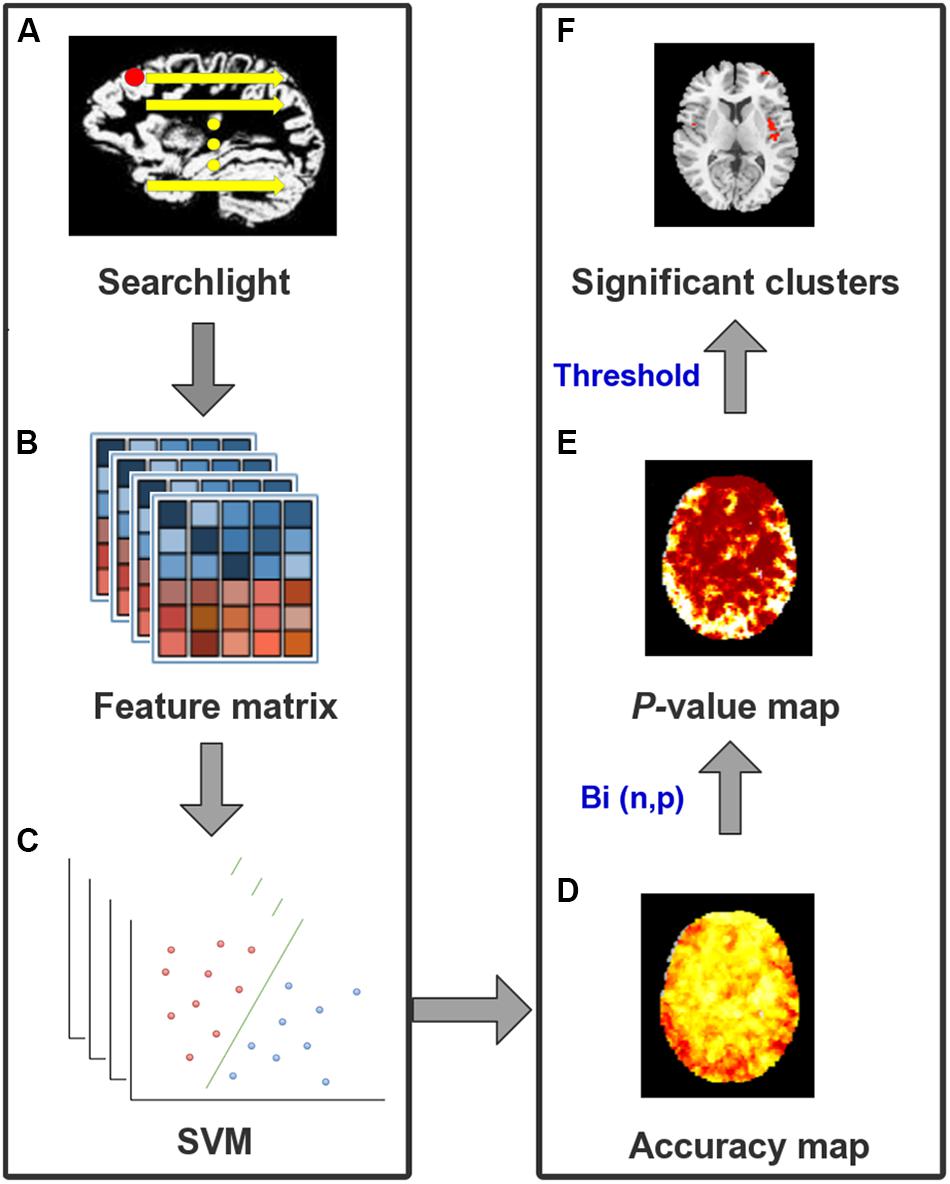
Figure 1. The flowchart for MVPA procedure. (A) For each voxel in GM as a center, a 5-mm sphere was defined as a searchlight. (B) GM volumes of all voxels in the same sphere were extracted from all subjects to construct a feature matrix. (C) SVM classifier with LOOCV was built to produce an accuracy value for the central voxel. (D) This procedure was repeated after the whole brain accuracy map was created. (E) Binominal distribution, with a null hypothesis that there were no differences between two groups, was tested to convert the accuracy map into a p-value map. (F) With a threshold of p < 0.0001 and cluster size >50 voxels, significant clusters to classify different groups were identified finally.
To identify clusters with statistical significance, we first converted the accuracy map into a p-value map, then conducted a connected algorithm on the p-value map to find the clusters that survived the threshold. To be specific, with a null hypothesis that there is no difference between this two groups, we assumed that the accuracy map followed a binomial distribution Bi(n, p) (Pereira et al., 2009). Herein, n denoted the number of all patients and the probability of p was equal to 0.5. When k subjects were successfully classified out of n, we defined the probability of a random variable m exceeding k as a p-value. This procedure converts the accuracy map into a p-value map. After that, a connected algorithm was applied to the p-value map. Compared with previous studies (Uddin et al., 2011; Liu et al., 2012), a stricter threshold for a significant cluster was set as voxel-wise p < 0.0001 (corresponding to a voxel-wise accuracy of 79%) with at least 50 adjacent voxels. Therefore, for each voxel with p < 0.0001, its 18 neighboring voxels would be examined if their p-values below 0.0001. Those satisfied with the threshold would be labeled as a component belonging to the same cluster as the center voxel. After all GM voxels finished labeling, clusters with more than 50 contiguous voxels were extracted as the MVPA clusters.
In order to further evaluate the statistical significance of the detected clusters that survived our threshold, permutation testing with 1000 iterations was implemented (Ojala and Garriga, 2010). The labels (MRP or MSP) were randomly assigned to the input data. LOOCV was used to generate accuracy values for each permutation test. A total of 1000 values were acquired under the null-hypothesis distribution, with which we could calculate the proportion of accuracy values equal to or greater than the real accuracy. When actual accuracy exceeded 95% (namely, one-tailed p < 0.05) of resulting values from permutations, it was considered statistically significant.
Post hoc Analyses
To further study the difference of GM volumes between MRP and MSP, post hoc analyses in those clusters detected with MVPA were performed. This analysis may also help interpret the potential mechanism underlying drug actions and treatment outcomes. With age and gender as covariates, voxel-wise two-sample t-test was performed in these regions to determine the significant differences between MRP patients and MSP patients. AlphaSim correction was performed on the statistical map in the REST software4 for multiple comparisons. Specifically, the AlphaSim procedure generates an estimate of the overall significance level achieved from various combinations of the probability threshold and cluster size threshold. Herein, the threshold was estimated to be p < 0.01 with a minimum cluster size of 40 contiguous voxels, which was corresponding to p < 0.05 before correction.
Additionally, for each subject in the MSP group, the mean GM volume of each identified cluster was calculated. Spearman’s correlation was used to examine the relationship between the mean GM volume and the change of pain intensity after treatment, which was defined as ΔVAS (pre-scanning VAS minus post-treatment VAS).
Results
Patient Demographics
Three patients were excluded, due to unsatisfactory image quality, and four patients dropped out for alternative therapies. Finally, 29 patients were included for the following data analyses, including 14 MRP and 15 MSP. Demographic and clinical characteristics of the included patients are shown in Table 1. The MRP group (n = 14) was comprised of six females and eight males (mean age ± std: 61.0 ± 7.0 years), while the MSP group (n = 15) consisted of 10 females and five males (mean age ± std: 62.6 ± 8.3 years). There was no significant difference in age (p = 0.58), gender ratio (p = 0.20) and pain duration (p = 0.07) between the two groups. The Pittsburgh sleep quality index between MSP and MRP showed no significant difference before treatment (Pre_PSQI) and was significantly lower in MSP than in MRP after treatment (Post_PSQI). Between-group comparison of pre-scanning VAS showed an insignificant difference (p = 0.09), while post-treatment VAS was significantly higher in MRP patients than in the MSP group (p < 0.0001).
Spatial Patterns Characterized by MVPA
The accuracy map and p-value map at the intermediate procedure of MVPA are displayed in Figures 2, 3, respectively. And the spatial patterns of GM maps characterized by MVPA without covariates regression before classifier training are shown in Figure 4. For comparisons between significant clusters detected by MVPA with and without covariates regression, please see Supplementary Figure S2. The clusters included in the spatial patterns have a voxel-wise accuracy of at least 79% for the classification between MRP and MSP. The peak accuracy values of these clusters are reported in Table 2. The prominently discriminative cortical and subcortical areas included bilateral posterior cerebellum, bilateral superior temporal lobe mostly extending to the posterior insular cortex (pIC), inferior orbital frontal cortex (OFC, right), middle frontal cortex (mFC, right), inferior frontal lobe (IFC, bilateral), anterior and middle cingulum (ACC and MCC), inferior parietal lobe (IPL), precuneus (PCu) and the cuneus. All clusters detected by MVPA exhibited a statistical significance in the permutation test (p < 0.05, Table 2).
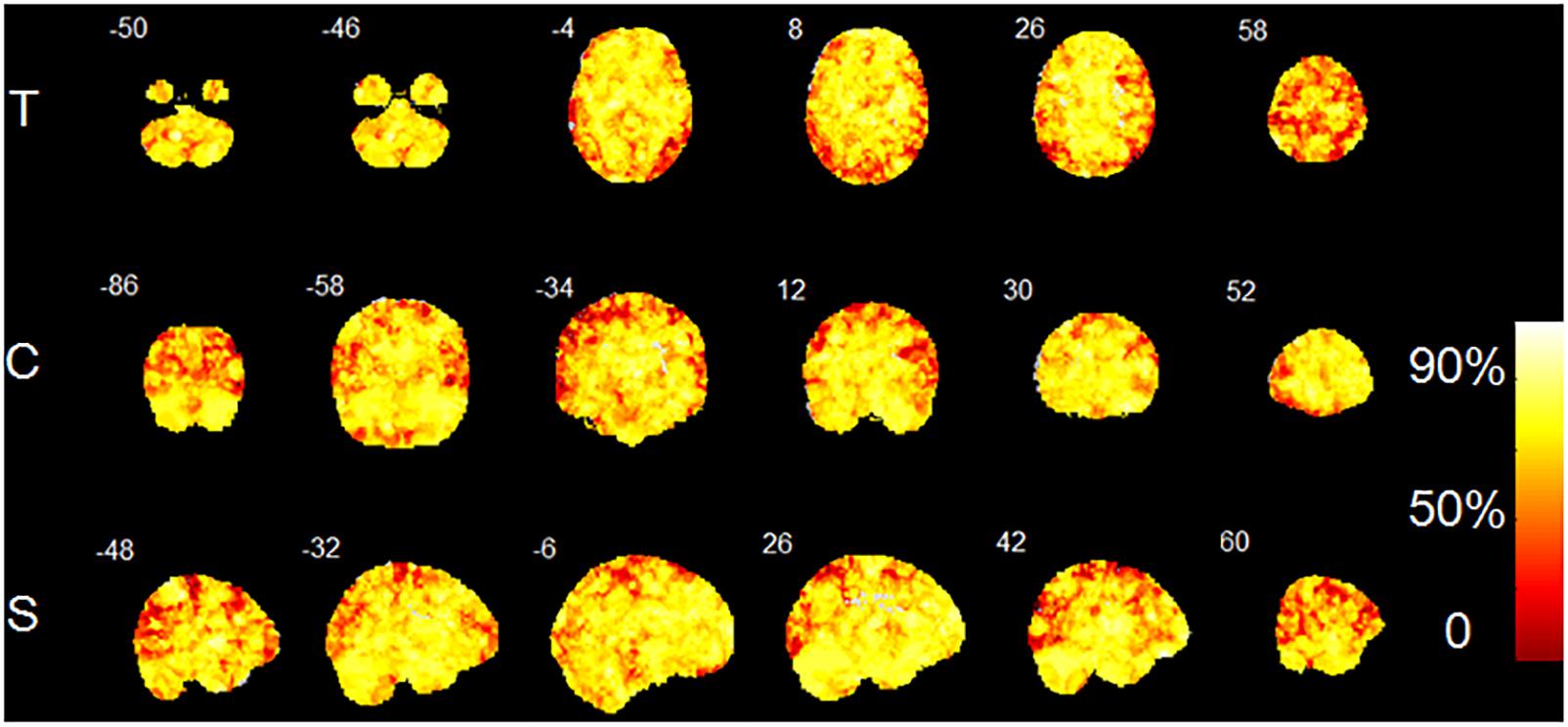
Figure 2. Accuracy map created by MVPA procedure. T, transverse direction; C, coronal direction; S, sagittal direction. The color-bar indicates the classification accuracy values of the whole brain GM voxels. The image is displayed in the neurologic convention, with the left side corresponding to the left-brain hemisphere.
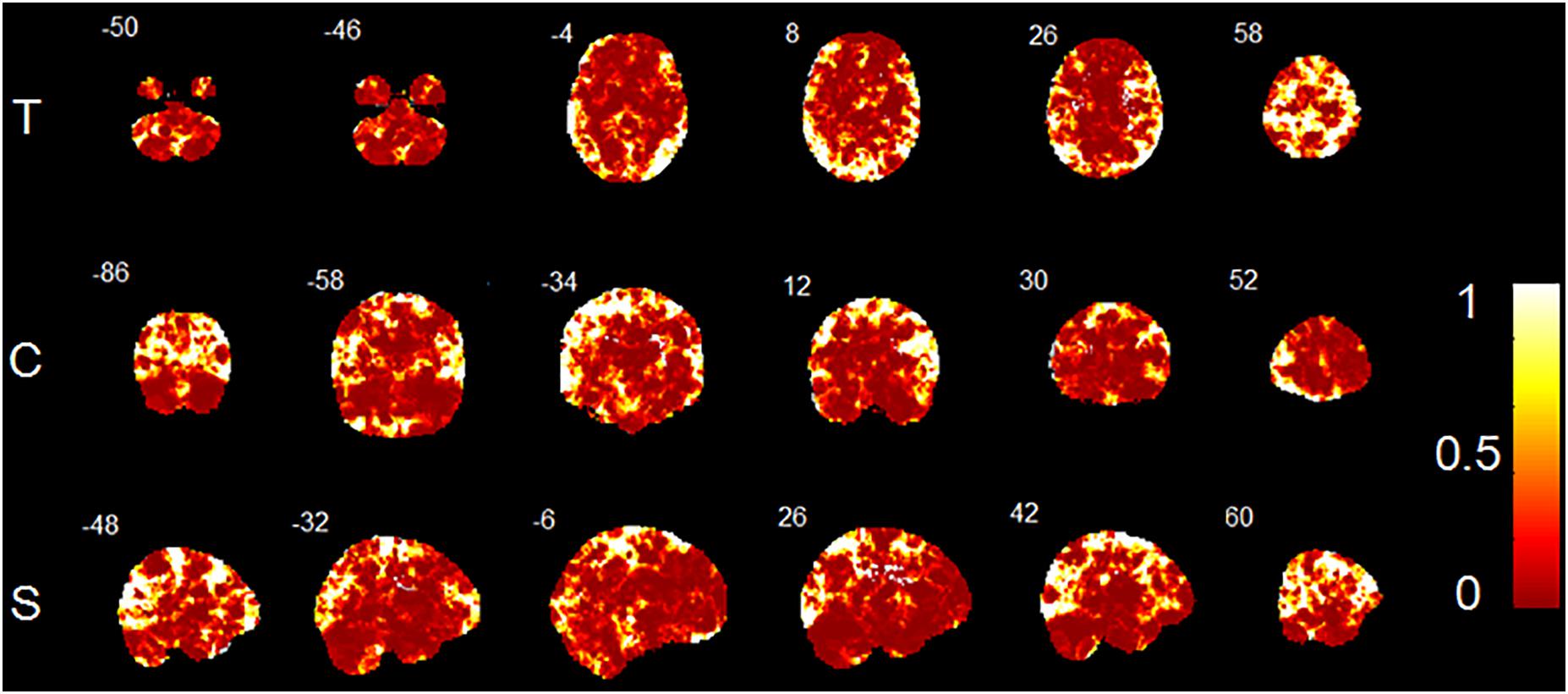
Figure 3. P-value map converted from accuracy map. T, transverse direction; C, coronal direction; S, sagittal direction. The color-bar indicates the p-values of the whole brain GM voxels. The image is displayed in the neurologic convention, with the left side corresponding to the left-brain hemisphere.
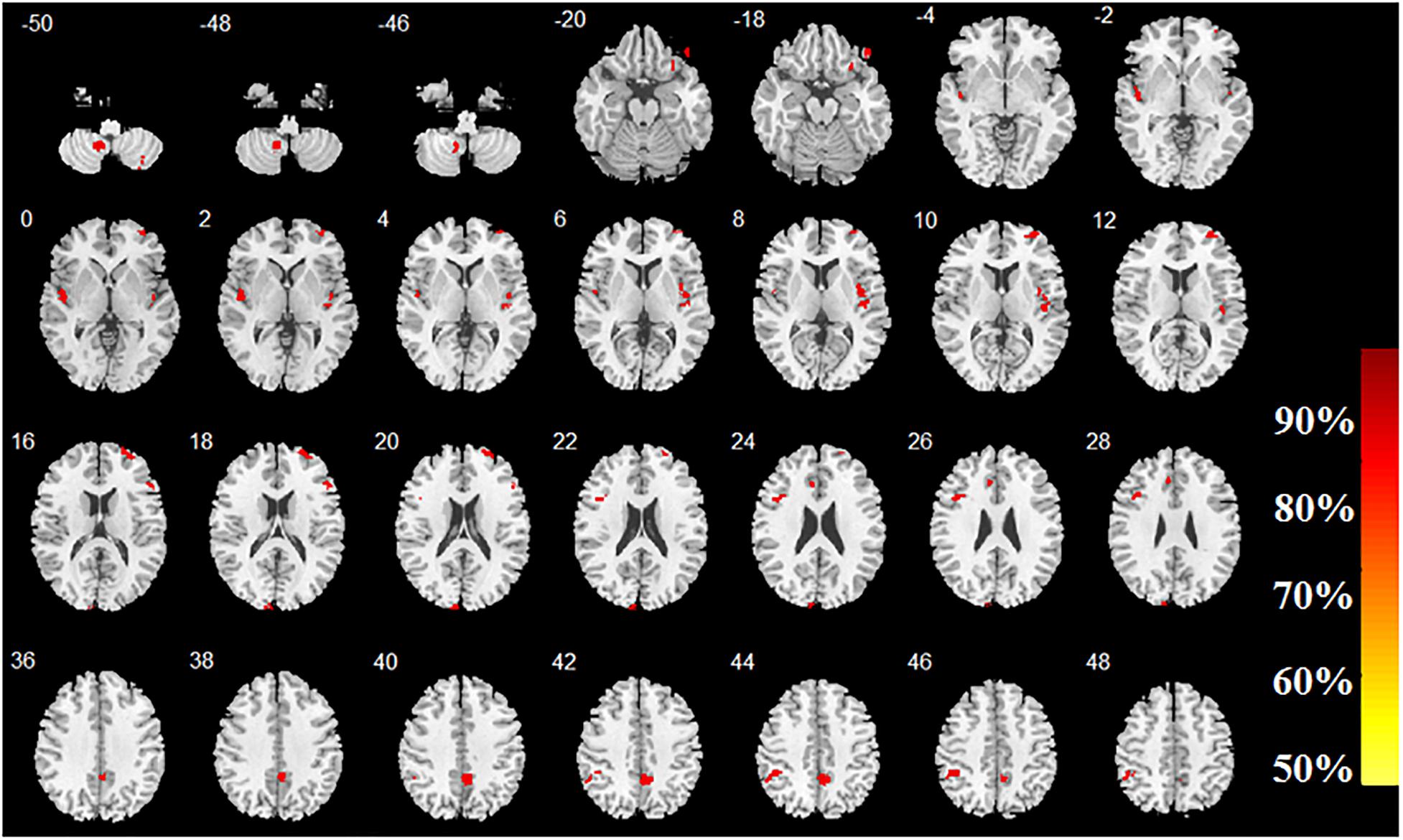
Figure 4. Brain regions with high classification accuracy identified by MVPA. The color bar indicates the classification accuracy of these brain regions. The image is displayed in the neurologic convention, with the left side corresponding to the left-brain hemisphere.
Post hoc Analyses
Two-sample t-tests with an AlphaSim correction (p < 0.05, cluster size >40) showed that five out of the 14 clusters identified by MVPA, exhibited significant GM volume decreases in MRP patients, compared to those with MSP (Table 3 and Figure 5). These brain regions consisted of the frontal lobes (mFC and OFC, right), superior temporal lobes (mainly pIC, bilateral), and MCC (extending to PCu). No regions showed significantly higher GM volumes in MRP patients compared to the MSP patients.
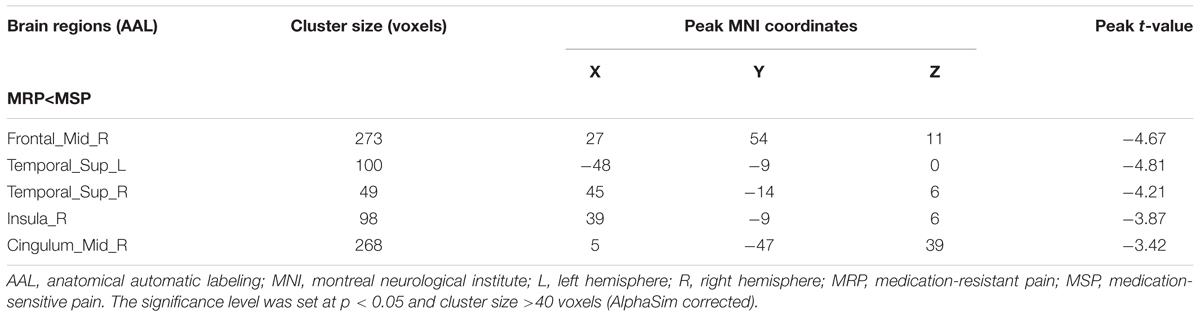
Table 3. Brain regions with GM volume differences between MRP patients and MSP patients by post hoc VBM analysis.
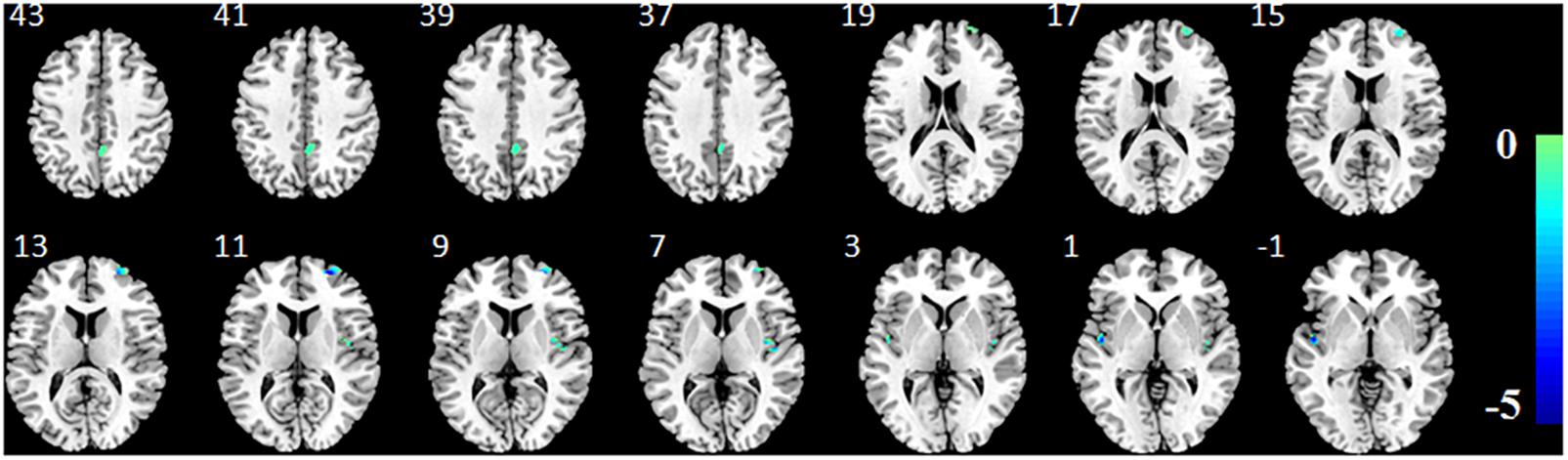
Figure 5. Brain regions with significant GM volume differences between MRP patients and MSP patients by post hoc VBM analysis. The color bar shows the corresponding peak t-values of the clusters and the negative values imply smaller GM volumes in patients with MRP than those with MSP. The image is displayed in the neurologic convention, with the left side corresponding to the left-brain hemisphere.
In the MSP group, no significantly positive or negative correlations were detected between GM volumes in MVPA-identified regions and ΔVAS.
Discussion
In this study, we applied MVPA to characterize the potential neurological biomarkers in sMRI to predict the medication responses of HZ patients. The cerebellum, insula, frontal lobe, ACC and PCu showed a pattern of high classification accuracy using the MVPA method. These regions implied a deficiency in both the sensory-discriminative and affective/cognitive aspects of pain processing in HZ patients, as shown in previous studies based on brain MRI (Yu and Yu, 2017; Cao et al., 2018; Hong et al., 2018).
Antidepressants and anticonvulsants are uniformly recommended as first-line medications for neuropathic pain caused by herpetic infections (Gore et al., 2007). In the current study, a combination of these two kinds of drugs were prescribed to HZ patients for pain management. The pharmacological mechanisms of anticonvulsants and antidepressants are different. Anticonvulsants, such as gabapentin and pregabalin, bind to the alpha-2-delta protein to reduce the release of excitatory neurotransmitters like glutamine and noradrenaline (Gore et al., 2007). And tricyclic antidepressants, amitriptyline, inhibits the reuptake of serotonin and noradrenaline and increases their concentration in intrasynaptic space (Coluzzi and Mattia, 2005), which could modulate activity in endogenous descending pain inhibitory pathways. Distinct actions of antidepressants and anticonvulsants on the neurotransmitter system could both produce antinociception, analgesic and anxiolytic activity.
However, the central mechanisms of these drugs for pain relief are largely unclear. Though limited, there are some studies based on MRI techniques which reveals that activity or morphology changes in frontal lobe, insular cortex, ACC and cerebellum of neuropathic pain were affected by these central nervous system drugs (Marchand, 2008). Structural/functional deficiency in these regions was not only detected in our study, but also in other previous studies on neuropathic pain, such as chronic lower back pain (Buckalew et al., 2008), headache (Dettmers et al., 2001; Naegel et al., 2014) and fibromyalgia (Shi et al., 2016). Therefore, antidepressants and anticonvulsants may have potential effects on these brain regions of HZ patients.
Posterior insular cortex receives nociceptive signals from the thalamus-spinal ascending system (Davis and Moayedi, 2013) because it has direct anatomical connections to spinothalamic tracts. Gabapentin could reduce the activations in the bilateral operculoinsular cortex to modulate nociceptive transmission in humans (Iannetti et al., 2005). Additionally, for individuals with evoked or clinical pain, the concentration of glutamine in pIC changed after non-pharmacological treatment (Harris et al., 2008). A study on fibromyalgia patients (Harris et al., 2013) also found decreased glutamine in this region after pregabalin treatment rather than a placebo, suggesting that the insular cortex could be a potential target for pregabalin. Our findings showed significant decrease of GM in bilateral pIC of MRP patients compared to MSP patients. Since inhibition of neural activity and neurotransmitter (e.g., glutamine) release in pIC are closely related to pain remission, structural deficiency in this region may have a negative impact on its activity modulated by drugs, thus resulting in the poor response of MRP patients.
The cingulate cortex and prefrontal lobe are critical regions for emotional and cognitive regulation of perceived pain. Accumulating evidence from fMRI studies showed an abnormal cingulum and frontal lobe response in HZ and PHN patients compared to healthy controls (Peyron et al., 2000; Geha et al., 2007, 2008; Liu et al., 2013; Dong et al., 2014, 2015; Jiang et al., 2016; Cao et al., 2017b; Hong et al., 2018), suggesting that dysfunction in controlling emotion, anticipation and evaluation could modify pain perception. In line with these studies, in our study ACC and PFC structural impairments were observed in HZ patients, supported by a significantly increased GM volume of the cingulate cortex in MRP rather than MSP. It may indicate that ACC and PFC could be important regions that influence pain regulation in patients with HZ-related pain or PHN. These two regions could even be potential targets of neuropathic pain drugs, since accumulating evidence has proven the regulation of activity by antidepressants and anticonvulsants in ACC and PFC. In mice with neuropathic pain induced by partial sciatic nerve ligation (Wang et al., 2015), pregabalin treatment decreased the expression of c-Fos, an indicator of transient and rapid neuronal activity, in neurons of the ACC. Another study (Lin et al., 2014) reported that synaptic transmission of ACC in adult mice could be inhibited by gabapentin. For healthy volunteers with capsaicin-induced central sensitization (Iannetti et al., 2005), gabapentin also suppressed stimulus evoked deactivation in PFC. When irritable bowel syndrome patients were stressful (Morgan et al., 2005), their pain related activation of ACC and left posterior parietal cortex were reduced by amitriptyline compared with baseline state. Altogether, we speculate that compared to MSP, MRP patients with more negative anatomy changes in the cingulate cortex and PFC, may respond insufficiently to medication, which may result in lessened remission of pain after treatment.
Patients with neuropathic pain are often characterized by symptoms of mood disorders (Inoue et al., 2017). This comorbidity supports that neuropathic pain and affective disturbances may share some common pathogenetic mechanisms (Aloisi et al., 2016). HZ patients in our study also manifested psychological distress and loss of sleep due to severe pain, supported by relative higher PSQI scores compared to healthy people before treatment. After treatment with antidepressants and anticonvulsants, MSP patients received a mood boost and their sleep recovered. Medial PFC is one of the most common brain regions involved with pain in affective disturbances (Descalzi et al., 2017). Not surprisingly, we also found a PFC morphologic difference between MSP and MRP patients, which could predict pharmacological responses accurately. Experiments from preclinical models demonstrated that neuroinflammation in affective forebrain regions would be evoked by nerve injury, and disrupts the normal physiological process which deals with affective disturbances (Fiore and Austin, 2016). It turns out that neurogenesis in adults can be modulated by psychoactive agents, including antidepressants and anticonvulsants (Fiore and Austin, 2016). Therefore, these drugs may help to eliminate inflammation, promote neuronal growth in affective brain regions, and result in an analgesic effect in HZ patients. Since MRP patients suffered prolonged structural changes in PFC compared to MSP patients, they may not benefit from drugs for such a short duration of treatment, which needs to be confirmed by a longitudinal follow-up study.
PFC, IPL, and PCu are hub regions included in the default mode network (DMN), which is often deactivated during painful stimuli and other tasks (Iannetti et al., 2005). We found that structural differences in these DMN components were able to predict medication response in HZ patients. An fMRI study demonstrated that gabapentin had an antihyperalgesic effect by reducing the magnitude of deactivation in DMN regions in central sensitization, but not in a normal state (Iannetti et al., 2005). Evidence from an EEG study stated that PCu activation was correlated with pain sensitivity (Goffaux et al., 2014). It is possible that antidepressants could modulate DMN activity of HZ patients to restore their function from central sensitization, such as hyperalgesia and allodynia which commonly occurs in neuropathic pain patients. Additionally, genetic and environmental factors that contribute to pre-existing structural differences in DMN regions, would be partly related to the medication response of HZ patients. This could be further supported by a sMRI study, which found that the higher the pain sensitivity in healthy individuals, the less GM volume was present in their PCu (Emerson et al., 2014). This finding may suggest that a poor response to medication for MRP patients, could possibly be due a significantly lower GM volume in DMN regions compared to that in MSP patients. Though investigations regarding the effects of antidepressants and anticonvulsants on neural plasticity remains lacking, such kind of structural deficiency might not be easily changed by medication.
The posterior lobes of the cerebellum in our study also yielded high classification accuracy. Recently, a multimodality MRI study described GM volume increase in posterior cerebellum during the transition from HZ to PHN (Cao et al., 2018). Thus, the cerebellum may play an important role in the development of chronic pain. So far, no studies have reported any direct response of cerebellum to antidepressants or anticonvulsants in neuropathic pain. But noradrenergic and serotoninergic systems, originating in the brain stem and projecting to the spinal cord dorsal horn, could be influenced by antidepressants, leading to the modulation of pain perception (Valverderk et al., 1994). Since the brain stem has a direct connection to the cerebellum, it cannot be excluded that the activity of the cerebellum would be indirectly affected by antidepressants through the changes of neurotransmitter levels in the brain stem. This indicates that a difference in the cerebellum between MSP and MRP patients, may potentially mediate the different responses to medication.
Several brain regions including the OFC, ACC, PCu and the cuneus showed a significant predictive power to classify MRP from MSP patients. However, GM volumes of these areas did not show significant differences between MSP and MRP in our post hoc VBM analysis. This observation may be mainly attributed to the methodological difference between the two methods. Specifically, VBM applies voxel-wise two-sample t-test between groups, while MVPA extracts values of all voxels in the sphere as informative features and takes full advantage of the machine learning algorithm to eventually learn good feature representations for classification. Therefore, MVPA would be more sensitive in detecting subtle differences in the aforementioned brain regions between the two groups, as compared to VBM. In this view, the performance of MVPA may be better than the traditional univariate VBM method.
Some limitations of the present study need to be considered. First, the sample size is relatively small, even though it seems moderate compared to previous studies that had sample sizes varying from 11 to 22 to explore HZ- or PHN-related brain abnormality (Geha et al., 2007, 2008; Liu et al., 2013; Zhang et al., 2014, 2016; Jiang et al., 2016; Cao et al., 2017a,b, 2018). We performed a post hoc estimation of the sample size required for detecting the difference of GM volumes in the brain regions characterized by MVPA. Average GM volumes of MVPA-detected clusters were used to calculate the possible effect sizes, because GM volume was the variable required to distinguish MSP from MRP. With such effect sizes we calculated the sample size to be around 8∼25 patients per group. This may partly justify that the sample size in the current study could be appropriate in yielding a reliable result. It should, however, be noted that future work, with a larger sample, especially from a multicenter, would be necessary to further verify these preliminary findings. Second, an Alphasim correction using the Monte Carlo simulation was applied to control type I error in post hoc VBM analysis, which was insufficient compared to the standard FWE or FDR correction. We have conducted FWE and FDR correction on VBM statistical maps but found no surviving clusters. This is partly due to a small sample size in our study. Previous studies have justified that a sample size smaller than 40 in each group, would reduce the reproducibility of results variously, no matter which multiple comparisons strategy was adopted (Button et al., 2013; Chen et al., 2018). Though less strict than FDR and FWE corrections, Alphasim correction is commonly used in the neuroimaging field with a reasonable underlying principle that “true regions of abnormality will tend to occur over contiguous voxels, whereas noise has much less of a tendency to form clusters of distinguish between signal and noise” (Ward, 2000). As an exploratory study, the present work adopted the Alphasim correction to provide some illuminating results to deepen our understanding of potential drug actions on the central system of HZ patients. Therefore, further studies with a strict multiple comparisons approach are needed to confirm these preliminary results. Third, when using MVPA, it is still challenging to interpret the inherent nature of the structural pattern that leads to an accurate prediction. One possible scheme would be longitudinal studies, which could assist in monitoring the dynamic changes of the brain structure. Third, the treatment effect of HZ patients with polypharmacy is often superior to that of monotherapy. However, medication actions are far more complex in polypharmacy treatment. Thus, findings from the current study may not be sufficient enough to elucidate whether synergy or single actions of these drugs mediate the response of brain regions of HZ patients. To explore a specific drug effect on the central nervous system, monotherapy studies are warranted in the future. Finally, a collective dataset of different modalities, such as functional MRI and DTI, would be helpful to depict explicit neural intersections of spatially distributed brain areas.
Conclusion
In this study, MVPA was applied to a structural MRI to characterize the spatial patterns in predicting the medication responses of HZ patients. The anatomical deficiency in MRP and MSP patients was mainly identified in the insula, ACC, MCC, PFC, IPL, PCu, cuneus and the cerebellum, which are all highly involved in the sensory, emotional and cognitive aspects of pain. These findings may provide new insights into the neural biomarkers that could serve as medication targets for HZ-related pain. Such insights could also assist in providing precise clinical interventions to expedite patient recovery and to prevent the progression to intractable PHN.
Ethics Statements
The protocol was approved by the ethics committee of the Shenzhen Sixth Hospital of Guangdong Medical University. All subjects provided written informed consent in accordance with the Declaration of Helsinki.
Author Contributions
JH, CQ, and YL collected the data. PZ and SW analyzed the data. FC, WS, WT, JZ, ZY, SZ, ZZ, LX, and BH discussed the results.
Funding
The study was funded by Seed Funding from the Scientific and Technical Innovation Council of Shenzhen Government (No. 000048) and the Shenzhen Municipal Scheme for Basic Research (No. JCYJ20160429181451546).
Conflict of Interest Statement
The authors declare that the research was conducted in the absence of any commercial or financial relationships that could be construed as a potential conflict of interest.
Supplementary Material
The Supplementary Material for this article can be found online at: https://www.frontiersin.org/articles/10.3389/fnins.2019.00534/full#supplementary-material
Footnotes
- ^http://dbm.neuro.uni-jena.de/vbm8/
- ^http://www.fil.ion.ucl.ac.uk/spm
- ^https://www.csie.ntu.edu.tw/∼cjlin/libsvm/
- ^http://sourceforge.net/projects/resting-fmri
References
Aloisi, A. M., Berlincioni, V., Torta, R., Nappi, R. E., Tassorelli, C., Barale, F., et al. (2016). The role of gender, psycho-social factors and anthropological-cultural dimensions on pain in neurorehabilitation. evidence and recommendations from the italian consensus conference on pain in neurorehabilitation. Eur. J. Phys. Rehab. Med. 52, 730–740.
Ashburner, J. (2009). Computational anatomy with the SPM software. Magn. Reson. Imaging 27, 1163–1174. doi: 10.1016/j.mri.2009.01.006
Ashburner, J., and Friston, K. J. (2000). Voxel-based morphometry - the methods. Neuroimage 11, 805–821. doi: 10.1006/nimg.2000.0582
Bendfeldt, K., Kloppel, S., Nichols, T. E., Smieskova, R., Kuster, P., Traud, S., et al. (2012). Multivariate pattern classification of gray matter pathology in multiple sclerosis. Neuroimage 60, 400–408. doi: 10.1016/j.neuroimage.2011.12.070
Buckalew, N., Haut, M. W., Morrow, L., and Weiner, D. (2008). Chronic pain is associated with brain volume loss in older adults: preliminary evidence. Pain Med. 9, 240–248. doi: 10.1111/j.1526-4637.2008.00412.x
Button, K. S., Ioannidis, J. P. A., Mokrysz, C., Nosek, B. A., Flint, J., Robinson, E. S. J., et al. (2013). Power failure: why small sample size undermines the reliability of neuroscience. Nat. Rev. Neurosci. 14, 365–376. doi: 10.1038/nrn3475
Cao, S., Li, Y., Deng, W., Qin, B., Zhang, Y., Xie, P., et al. (2017a). Local brain activity differences between herpes zoster and postherpetic neuralgia patients: a resting-state functional MRI study. Pain Phys. 20, E687–E699.
Cao, S., Song, G., Zhang, Y., Xie, P., Tu, Y., Li, Y., et al. (2017b). abnormal local brain activity beyond the pain matrix in postherpetic neuralgia patients: a resting-state functional MRI study. Pain Phys. 20, E303–E314.
Cao, S., Qin, B., Zhang, Y., Yuan, J., Fu, B., Xie, P., et al. (2018). Herpes zoster chronification to postherpetic neuralgia induces brain activity and grey matter volume change. Am. J. Transl. Res. 10, 184–199.
Chen, X., Lu, B., and Yan, C.-G. (2018). Reproducibility of R-fMRI metrics on the impact of different strategies for multiple comparison correction and sample sizes. Hum. Brain Mapp. 39, 300–318. doi: 10.1002/hbm.23843
Coluzzi, F., and Mattia, C. (2005). Mechanism-based treatment in chronic neuropathic pain: the role of antidepressants. Curr. Pharm. Des. 11, 2945–2960. doi: 10.2174/1381612054864993
Davis, K. D., and Moayedi, M. (2013). Central mechanisms of pain revealed through functional and structural MRI. J. Neuroimmune Pharmacol. 8, 518–534. doi: 10.1007/s11481-012-9386-9388
Descalzi, G., Mitsi, V., Purushothaman, I., Gaspari, S., Avrampou, K., Loh, Y. E., et al. (2017). Neuropathic pain promotes adaptive changes in gene expression in brain networks involved in stress and depression. Sci. Signal. 10:eaaj1549. doi: 10.1126/scisignal.aaj1549
Dettmers, C., Adler, T., Rzanny, R., van Schayck, R., Gaser, C., Weiss, T., et al. (2001). Increased excitability in the primary motor cortex and supplementary motor area in patients with phantom limb pain after upper limb amputation. Neurosci. Lett. 307, 109–112. doi: 10.1016/s0304-3940(01)01953-x
Dong, M., Li, J., Shi, X., Gao, S., Fu, S., Liu, Z., et al. (2015). Altered baseline brain activity in experts measured by amplitude of low frequency fluctuations (ALFF): a resting state fMRI study using expertise model of acupuncturists. Front. Hum. Neurosci. 9:99. doi: 10.3389/fnhum.2015.00099
Dong, M., Qin, W., Zhao, L., Yang, X., Yuan, K., Zeng, F., et al. (2014). Expertise modulates local regional homogeneity of spontaneous brain activity in the resting brain: an fMRI study using the model of skilled acupuncturists. Hum. Brain Mapp. 35, 1074–1084. doi: 10.1002/hbm.22235
Ecker, C., Rocha-Rego, V., Johnston, P., Mourao-Miranda, J., Marquand, A., Daly, E. M., et al. (2010). Investigating the predictive value of whole-brain structural MR scans in autism: a pattern classification approach. Neuroimage 49, 44–56. doi: 10.1016/j.neuroimage.2009.08.024
Emerson, N. M., Zeidan, F., Lobanov, O. V., Hadsel, M. S., Martucci, K. T., Quevedo, A. S., et al. (2014). Pain sensitivity is inversely related to regional grey matter density in the brain. Pain 155, 566–573. doi: 10.1016/j.pain.2013.12.004
Etzel, J. A., Zacks, J. M., and Braver, T. S. (2013). Searchlight analysis: promise, pitfalls, and potential. Neuroimage 78, 261–269. doi: 10.1016/j.neuroimage.2013.03.041
Fiore, N. T., and Austin, P. J. (2016). Are the emergence of affective disturbances in neuropathic pain states contingent on supraspinal neuroinflammation? Brain Behav. Immun. 56, 397–411. doi: 10.1016/j.bbi.2016.04.012
Geha, P. Y., Baliki, M. N., Chialvo, D. R., Harden, R. N., Paice, J. A., and Apkarian, A. V. (2007). Brain activity for spontaneous pain of postherpetic neuralgia and its modulation by lidocaine patch therapy. Pain 128, 88–100. doi: 10.1016/j.pain.2006.09.014
Geha, P. Y., Baliki, M. N., Wang, X., Harden, R. N., Paice, J. A., and Apkarian, A. V. (2008). Brain dynamics for perception of tactile allodynia (touch-induced pain) in postherpetic neuralgia. Pain 138, 641–656. doi: 10.1016/j.pain.2008.02.021
Goffaux, P., Girard-Tremblay, L., Marchand, S., Daigle, K., and Whittingstall, K. (2014). Individual differences in pain sensitivity vary as a function of precuneus reactivity. Brain Topogr. 27, 366–374. doi: 10.1007/s10548-013-0291-290
Good, C. D., Johnsrude, I. S., Ashburner, J., Henson, R. N., Friston, K. J., and Frackowiak, R. S. (2001). A voxel-based morphometric study of ageing in 465 normal adult human brains. Neuroimage 14, 21–36. doi: 10.1006/nimg.2001.0786
Gore, M., Sadosky, A., Tai, K.-S., and Stacey, B. (2007). A retrospective evaluation of the use of gabapentin and pregabalin in patients with postherpetic neuralgia in usual-care settings. Clin. Ther. 29, 1655–1670. doi: 10.1016/j.clinthera.2007.08.019
Hadley, G. R., Gayle, J. A., Ripoll, J., Jones, M. R., Argoff, C. E., Kaye, R. J., et al. (2016). Post-herpetic neuralgia: a review. Curr. Pain Headache Rep. 20:17. doi: 10.1007/s11916-016-0548-x
Harden, R. N., Kaye, A. D., Kintanar, T., and Argoff, C. E. (2013). Evidence-based guidance for the management of postherpetic neuralgia in primary care. Postgrad. Med. 125, 191–202. doi: 10.3810/pgm.2013.07.2690
Harris, R. E., Napadow, V., Huggins, J. P., Pauer, L., Kim, J., Hampson, J., et al. (2013). Pregabalin rectifies aberrant brain chemistry, connectivity, and functional response in chronic pain patients. anesthesiology. J. Am. Soc. Anesthesiol. 119, 1453–1464. doi: 10.1097/ALN.0000000000000017
Harris, R. E., Sundgren, P. C., Pang, Y., Hsu, M., Petrou, M., Kim, S. H., et al. (2008). Dynamic levels of glutamate within the insula are associated with improvements in multiple pain domains in fibromyalgia. Arthritis Rheum. 58, 903–907. doi: 10.1002/art.23223
Haxby, J. V. (2012). Multivariate pattern analysis of fMRI: the early beginnings. Neuroimage 62, 852–855. doi: 10.1016/j.neuroimage.2012.03.016
Haynes, J. D., and Rees, G. (2005). Predicting the orientation of invisible stimuli from activity in human primary visual cortex. Nat. Neurosci. 8, 686–691. doi: 10.1038/nn1445
Haynes, J. D., and Rees, G. (2006). Decoding mental states from brain activity in humans. Nat. Rev. Neurosci. 7, 523–534. doi: 10.1038/nrn1931
Hendler, T., Gonen, T., Harel, E. V., and Sharon, H. (2014). From circuit activity to network connectivity and back: the case of obsessive-compulsive disorder. Biol. Psychiatry 75, 590–592. doi: 10.1016/j.biopsych.2014.02.017
Hong, S., Gu, L., Zhou, F., Liu, J., Huang, M., Jiang, J., et al. (2018). Altered functional connectivity density in patients with herpes zoster and postherpetic neuralgia. J. Pain Res. 11, 881–888. doi: 10.2147/JPR.S154314
Iannetti, G. D., Zambreanu, L., Wise, R. G., Buchanan, T. J., Huggins, J. P., Smart, T. S., et al. (2005). Pharmacological modulation of pain-related brain activity during normal and central sensitization states in humans. Proc. Natl. Acad. Sci. U.S.A. 102, 18195–18200. doi: 10.1073/pnas.0506624102
Inoue, S., Taguchi, T., Yamashita, T., Nakamura, M., and Ushida, T. (2017). The prevalence and impact of chronic neuropathic pain on daily and social life: a nationwide study in a Japanese population. Eur. J. Pain 21, 727–737. doi: 10.1002/ejp.977
Jeon, Y. H. (2015). Herpes zoster and postherpetic neuralgia: practical consideration for prevention and treatment. Korean J. Pain 28, 177–184. doi: 10.3344/kjp.2015.28.3.177
Jiang, J., Gu, L., Bao, D., Hong, S., He, W., Tan, Y., et al. (2016). Altered homotopic connectivity in postherpetic neuralgia: a resting state fMRI study. J. Pain Res. 9, 877–886. doi: 10.2147/JPR.S117787
Jimura, K., and Poldrack, R. A. (2012). Analyses of regional-average activation and multivoxel pattern information tell complementary stories. Neuropsychologia 50, 544–552. doi: 10.1016/j.neuropsychologia.2011.11.007
Johnson, R. W., and Rice, A. S. (2014). Clinical practice. postherpetic neuralgia. N. Engl. J. Med. 371, 1526–1533. doi: 10.1056/NEJMcp1403062
Jung, B. F., Johnson, R. W., Griffin, D. R., and Dworkin, R. H. (2004). Risk factors for postherpetic neuralgia in patients with herpes zoster. Neurology 62, 1545–1551. doi: 10.1212/01.wnl.0000123261.00004.29
Kamitani, Y., and Tong, F. (2005). Decoding the visual and subjective contents of the human brain. Nat. Neurosci. 8, 679–685. doi: 10.1038/nn1444
Klöppel, S., Peter, J., Ludl, A., Pilatus, A., Maier, S., Mader, I., et al. (2015). Applying automated MR-based diagnostic methods to the memory clinic: a prospective study. J. Alzheimers Dis. 47, 939–954. doi: 10.3233/jad-150334
Kriegeskorte, N., Goebel, R., and Bandettini, P. (2006). Information-based functional brain mapping. Proc. Natl. Acad. Sci. U.S.A. 103, 3863–3868. doi: 10.1073/pnas.0600244103
Lin, H.-C., Huang, Y.-H., Chao, T.-H. H., Lin, W.-Y., Sun, W.-Z., and Yen, C.-T. (2014). Gabapentin reverses central hypersensitivity and suppresses medial prefrontal cortical glucose metabolism in rats with neuropathic pain. Mol. Pain 10:63. doi: 10.1186/1744-8069-10-63
Liu, F., Guo, W., Yu, D., Gao, Q., Gao, K., Xue, Z., et al. (2012). Classification of different therapeutic responses of major depressive disorder with multivariate pattern analysis method based on structural MR scans. PLoS One 7:e40968. doi: 10.1371/journal.pone.0040968
Liu, J., Hao, Y., Du, M., Wang, X., Zhang, J., Manor, B., et al. (2013). Quantitative cerebral blood flow mapping and functional connectivity of postherpetic neuralgia pain: a perfusion fMRI study. Pain 154, 110–118. doi: 10.1016/j.pain.2012.09.016
Marchand, S. (2008). The physiology of pain mechanisms: from the periphery to the brain. Rheum. Dis. Clin. North Am. 34, 285–309. doi: 10.1016/j.rdc.2008.04.003
Mechelli, A., Price, C. J., Friston, K. J., and Ashburner, J. (2005). Voxel-based morphometry of the human brain: methods and applications. Curr. Med. Imaging Rev. 1, 105–113. doi: 10.2174/1573405054038726
Morgan, V., Pickens, D., Gautam, S., Kessler, R., and Mertz, H. (2005). Amitriptyline reduces rectal pain related activation of the anterior cingulate cortex in patients with irritable bowel syndrome. Gut 54, 601–607.
Naegel, S., Holle, D., Desmarattes, N., Theysohn, N., Diener, H. C., Katsarava, Z., et al. (2014). Cortical plasticity in episodic and chronic cluster headache. Neuroimage Clin. 6, 415–423. doi: 10.1016/j.nicl.2014.10.003
Ojala, M., and Garriga, G. C. (2010). Permutation tests for studying classifier performance. J. Mach. Learn. Res. 11, 1833–1863.
Pereira, F., Mitchell, T., and Botvinick, M. (2009). Machine learning classifiers and fMRI: a tutorial overview. Neuroimage 45, S199–S209. doi: 10.1016/j.neuroimage.2008.11.007
Peyron, R., Laurent, B., and Garcia-Larrea, L. (2000). Functional imaging of brain responses to pain. a review and meta-analysis (2000). Neurophysiol. Clin. 30, 263–288. doi: 10.1016/s0987-7053(00)00227-226
Qin, J., Shen, H., Zeng, L.-L., Jiang, W., Liu, L., and Hu, D. (2015). Predicting clinical responses in major depression using intrinsic functional connectivity. Neuroreport 26, 675–680. doi: 10.1097/wnr.0000000000000407
Shi, H., Yuan, C., Dai, Z., Ma, H., and Sheng, L. (2016). Gray matter abnormalities associated with fibromyalgia: a meta-analysis of voxel-based morphometric studies. Semin. Arthritis Rheum. 46, 330–337. doi: 10.1016/j.semarthrit.2016.06.002
Uddin, L. Q., Menon, V., Young, C. B., Ryali, S., Chen, T., Khouzam, A., et al. (2011). Multivariate searchlight classification of structural magnetic resonance imaging in children and adolescents with autism. Biol. Psychiatry 70, 833–841. doi: 10.1016/j.biopsych.2011.07.014
Valverderk, O., Mico, J. A., Maldonado, R., Mellado, M., and Gibert-Rahola, J. (1994). Participation of opioid and monoaminergic mechanisms on the antinociceptive effect induced by tricyclic antidepressants in two behavioural pain tests in mice. Prog. Neuropsychopharmacol. Biol. Psychiatry 18, 1073–1092.
Wang, T.-X., Yin, D., Guo, W., Liu, Y.-Y., Li, Y.-D., Qu, W.-M., et al. (2015). Antinociceptive and hypnotic activities of pregabalin in a neuropathic pain-like model in mice. Pharmacol. Biochem. Behav. 135, 31–39. doi: 10.1016/j.pbb.2015.05.007
Wolfers, T., Buitelaar, J. K., Beckmann, C. F., Franke, B., and Marquan, A. F. (2015). From estimating activation locality to predicting disorder: a review of pattern recognition for neuroimaging-based psychiatric diagnostics. Neurosci. Biobehav. Rev. 57, 328–349. doi: 10.1016/j.neubiorev.2015.08.001
Yu, T., and Yu, B. (2017). Local brain activity differences between herpes zoster and postherpetic neuralgia patients: a resting-state functional MRI study. Pain Phys. 20, E687–E699.
Zhang, J., Cao, W., Wang, M., Wang, N., Yao, S., and Huang, B. (2018). Multivoxel pattern analysis of structural MRI in children and adolescents with conduct disorder. Brain Imaging Behav. doi: 10.1007/s11682-018-9953-9956 [Epub ahead of print]
Zhang, Y., Liu, J., Li, L., Du, M., Fang, W., Wang, D., et al. (2014). A study on small-world brain functional networks altered by postherpetic neuralgia. Magn. Reson. Imaging 32, 359–365. doi: 10.1016/j.mri.2013.12.016
Keywords: herpes zoster, medication response, structural MRI, multivoxel pattern analysis, prediction
Citation: Zeng P, Huang J, Wu S, Qian C, Chen F, Sun W, Tao W, Liao Y, Zhang J, Yang Z, Zhong S, Zhang Z, Xiao L and Huang B (2019) Characterizing the Structural Pattern Predicting Medication Response in Herpes Zoster Patients Using Multivoxel Pattern Analysis. Front. Neurosci. 13:534. doi: 10.3389/fnins.2019.00534
Received: 16 January 2019; Accepted: 08 May 2019;
Published: 28 May 2019.
Edited by:
Yuhui Du, Mind Research Network (MRN), United StatesReviewed by:
Minghao Dong, Xidian University, ChinaDelin Sun, Duke University, United States
Anees Abrol, Mind Research Network (MRN), United States
Copyright © 2019 Zeng, Huang, Wu, Qian, Chen, Sun, Tao, Liao, Zhang, Yang, Zhong, Zhang, Xiao and Huang. This is an open-access article distributed under the terms of the Creative Commons Attribution License (CC BY). The use, distribution or reproduction in other forums is permitted, provided the original author(s) and the copyright owner(s) are credited and that the original publication in this journal is cited, in accordance with accepted academic practice. No use, distribution or reproduction is permitted which does not comply with these terms.
*Correspondence: Lizu Xiao, MTQxNzM0MzQzMkBxcS5jb20=; Bingsheng Huang, aHVhbmdiQHN6dS5lZHUuY24=
†Co-authors and have contributed equally to this work