- 1Department of Neurology, The First Affiliated Hospital of Anhui Medical University, Hefei, China
- 2Anhui Province Key Laboratory of Cognition and Neuropsychiatric Disorders, Hefei, China
- 3Collaborative Innovation Center of Neuropsychiatric Disorders and Mental Health, Hefei, China
- 4Department of Medical Psychology, The First Affiliated Hospital of Anhui Medical University, Hefei, China
- 5Department of Radiology, The First Affiliated Hospital of Anhui Medical University, Hefei, China
Alzheimer’s disease (AD) is a common memory-impairment disorder frequently accompanied by olfactory identification (OI) impairments. In fact, OI is a valuable marker for distinguishing AD from normal age-related cognitive impairment and may predict the risk of mild cognitive impairment (MCI)-to-AD transition. However, current olfactory tests were developed based on Western social and cultural conditions, and are not very suitable for Chinese patients. Moreover, the neural substrate of OI in AD is still unknown. The present study investigated the utility of a newly developed Chinese smell identification test (CSIT) for OI assessment in Chinese AD and MCI patients. We then performed a correlation analysis of gray matter volume (GMV) at the voxel and region-of-interest (ROI) levels to reveal the neural substrates of OI in AD. Thirty-seven AD, 27 MCI, and 30 normal controls (NCs) completed the CSIT and MRI scans. Patients (combined AD plus MCI) scored significantly lower on the CSIT compared to NCs [F(2,91) = 62.597, p < 0.001)]. Voxel-level GMV analysis revealed strong relationships between CSIT score and volumes of the left precentral gyrus and left inferior frontal gyrus (L-IFG). In addition, ROI-level GMV analysis revealed associations between CSIT score and left amygdala volumes. Our results suggest the following: (1) OI, as measured by the CSIT, is impaired in AD and MCI patients compared with healthy controls in the Chinese population; (2) the severity of OI dysfunction can distinguish patients with cognitive impairment from controls and AD from MCI patients; and (3) the left-precentral cortex and L-IFG may be involved in the processing of olfactory cues.
Introduction
Alzheimer’s disease (AD) is the most common age-related neurodegenerative disorder and is characterized by progressive impairment of cognitive function, ultimately leading to incapacitation and death (McKhann et al., 2011). Thus, AD places a considerable emotional burden on families and economic burden on society (McDade and Bateman, 2017). Multiple studies have shown that neurobiological changes associated with AD are present for many years before the appearance of clinical symptoms. Many studies have focused on identifying neurological and behavioral changes that can predict AD onset and thereby allow early intervention. Mild cognitive impairment (MCI) is an intermediate state between normal age-related cognitive decline and dementia (Petersen, 2007); about 3 – 15% of MCI patients progress to AD annually (Petersen et al., 2001). Although there is still no effective treatment for AD (Miller, 2012), a previous study suggested that timely intervention can alleviate early symptoms, and delay AD progression (Baazaoui and Iqbal, 2018). Therefore, early detection of MCI is important for the short-term prognosis of AD. To this end, it is critical to identify biomarkers that can distinguish among AD, MCI, and healthy aging.
Previous studies have indicated that olfactory perception is frequently disrupted in the early stages of AD (Djordjevic et al., 2008; Silva et al., 2018), especially when preceded by MCI (Devanand, 2000; Larsson et al., 2000; Roalf et al., 2017). Impairments of olfactory perception involve deficits in several domains, including odor detection threshold, olfactory identification (OI), olfactory discrimination, and olfactory memory (Mesholam, 1998; Silva et al., 2018). Many studies have shown that olfactory dysfunction can accurately detect cognitive impairment (Graves et al., 1999; Peters, 2003; Schubert et al., 2008), differentiate AD from normal aging with high sensitivity (0.88) and specificity (0.91) (Ewers et al., 2012; Ritchie et al., 2014), and predict the potential for the progression of MCI to AD (Devanand et al., 2000, 2014; Roberts et al., 2016; Tahmasebi et al., 2019). Mounting evidence suggests that OI deficits are the predominant factor contributing to olfactory dysfunction in AD, as OI deficits occur earlier, and are more strongly correlated with memory impairments than deficits in other olfactory domains (Djordjevic et al., 2008). Moreover, impaired OI has been linked to accelerated decline in cognitive function (Dintica et al., 2019). Therefore, OI may be a valuable biomarker for preclinical AD.
There are many OI tests, such as the University of Pennsylvania Smell Identification Test (UPSIT), Connecticut Chemosensory Clinical Research Center (CCCRC) olfactory test, and “Sniffin Sticks” olfactory test. Each includes odors common in the local culture; however, performance may be affected by various semantic and cultural factors (Schab, 1991; Ayabe-Kanamura et al., 1998; Kobayashi et al., 2006). For instance, some items familiar to Western patients may not be easily recognizable by the Chinese population (and vice versa). Based on this consideration, Zhou developed the Chinese smell identification test (CSIT) (Feng et al., 2019), which adopts odor items that are familiar to and identifiable by most Chinese people. As such, the CSIT provides an effective tool (test-retest reliability of 0.92) for the assessment of olfactory function in the Chinese population. To our knowledge, however, there are few studies investigating OI dysfunction among AD and MCI patients using Chinese olfactory tests. In the present study, we examined whether CSIT can distinguish between normal aging, MCI, and AD in the Chinese population.
The neural substrates of OI dysfunction in AD and MCI patients are still unclear. Several neuroimaging studies have found associations between OI dysfunction and structural abnormities in brain regions that contribute to olfactory processing, such as the olfactory cortex (OC). The OC is the center of olfactory processing and functional and structural anomalies are strongly implicated in OI dysfunction (Thomann et al., 2009b; Servello et al., 2015; Vasavada et al., 2017). Hippocampal atrophy is a well-known pathological feature of AD and MCI (Prestia et al., 2010), and so may also contribute to OI dysfunction in AD and MCI. Indeed, several studies have reported a positive relationship between OI performance and hippocampal volume in patients with MCI or AD (Kjelvik et al., 2014). The amygdala is a key node linking the olfactory and hippocampal cortices (Price, 2003; LeDoux, 2007). Amygdala nuclei receive inputs from and send outputs to multiple brain regions subserving olfactory-associated functions, including emotional salience (Hamann, 2001). Thus, it is possible that the amygdala plays a key role in OI dysfunction among AD patients. Indeed, an vivo MRI study found that the amygdala was closely related to the olfactory loop in AD (Cavedo et al., 2011). The amygdala also has abundant neural connections with the hippocampus, and modulates both the encoding and the storage of hippocampal-dependent memories (Phelps, 2004).
Although several neuroimaging studies have implicated OC, hippocampal, and (or) amygdala abnormalities in OI dysfunction among AD and MCI patients, multiple studies have also reported contrary results. For example, Servello and colleagues (Feng et al., 2019) found no correlation between OC volume and olfactory function in AD, MCI, and NC. In contrast, several groups have found a correlation between the decline in OI and structural degeneration of the OC among AD patients (Claire Murphy, 2003; Thomann et al., 2009a; Marigliano et al., 2014; Servello et al., 2015). These discrepancies may be attributed to factors such as sample heterogeneity and differences in OI tests. In addition, neuroimaging analysis based on regions of interest (ROIs) may contribute to variability as the coordinates of specific ROIs differ markedly across studies (Ji et al., 2017). Thus, voxel-based analysis may help mitigate such inconsistencies.
In this study, we first compared OI performance among AD, MCI, and normal aging in the Chinese population using the CSIT. Subsequently, we conducted correlational analysis of CSIT scores and regional GMV at both ROI and whole-brain voxel levels to explore the neural substrates of OI.
Materials and Methods
Study Subjects
A total of 94 right-handed participants (37 AD, 27 MCI, and 30 age-matched cognitively NCs were enrolled in this study. The AD and MCI patients were recruited from the Dysmnesia Outpatient Department at the First Affiliated Hospital of Anhui Medical University, Anhui Province, China. The NCs were recruited from the local community through advertisement or were the spouses of the study patients. The present study was approval by the Research Ethics Committee of the First Affiliated Hospital of Anhui Medical University. All subjects gave written informed consent in accordance with the Declaration of Helsinki.
Patients With AD
The AD subjects were clinically diagnosed by a specialist in accordance with NINCDS-ADRDA (McKhann et al., 1984) criteria: (a) Meeting criteria of possible or probable AD (McKhann et al., 2011), (b) mini-mental state examination (MMSE) score < 24, and (c) clinical dementia rating (CDR) score ranging from 0.5 to 2.
The exclusion criteria were as follows: (a) sudden onset, (b) early occurrence of gait disturbances, seizures, or behavioral changes, (c) focal neurological features such as hemiparesis, sensory loss, or visual field deficits, and (d) early extra-pyramidal signs or other severe disorders such as trauma, major depression, severe cerebrovascular disease, or metabolic abnormalities (Dubois et al., 2007).
Patients With MCI
Participants with MCI were clinically diagnosed by experts according to Peterson’s criteria (Petersen et al., 1999) and NINCDS-ADRDA criteria as follows: (a) complaints of memory loss/other cognitive decline (including from the patient’s family or doctor), (b) unexpectedly poor performance on one or more cognitive functions given the patient’s age and educational background, (c) ability to maintain independence of daily living (i.e., no dementia) (Albert et al., 2011), (d) MMSE score > 24, (e) CDR score of 0.5. The exclusion criteria were the same as defined for AD patients.
Normal Controls
The NCs fulfilled the following criteria: cognitively normal, no neurological or psychiatric disorders, no psychoactive medication use, MMSE score of 28 or higher, and CDR score of 0.
Common Participant Criteria
White mater hyper-intensities (WMHs) were graded according to the Fazekas scale (Fazekas et al., 1987) based on visual assessment of both periventricular and subcortical areas (Helenius et al., 2017). However, as the presence of mild to moderate WMH frequently accompanies normal aging as well as neurodegenerative diseases (Wardlaw et al., 2013), this was not considered a criterion (Liu et al., 2011).
In order to rule out any confounds that could adversely influence the study results, we excluded subjects who engaged in long-term smoking and drinking as these behaviors have been shown to affect cognition and olfaction (Frye, 1990). We also checked for complications specific to olfactory dysfunction (e.g., nasal polyps, nasal obstruction, respiratory distress, head trauma, active sinus/upper respiratory infection, and allergies) and for contraindications to MRI (e.g., not-MRI-safe metal implants, severe claustrophobia). However, no participants were excluded from the study based upon these criteria.
Finally, 37 AD and 27 MCI patients with initial diagnosis and currently not treated with a cholinesterase inhibitor (donepezil, galantamine, or rivastigmine) were enrolled. Detailed background information on all groups is summarized in Table 1.
Neuropsychological Assessment
All participates underwent a clinical evaluation and neuropsychological assessment. The following neuropsychological test battery was administered to each subject for the purpose of establishing a clinical diagnosis as described previously (Lezak et al., 2004; Woodward et al., 2017). (i) General cognitive function was assessed with the Mini Mental State Examination (MMSE) (Burns, 1998) and the (global) Clinical Dementia Rating Scale (CDR) (Morris, 1993) as a proxy for disease severity. (ii) The Chinese version of the auditory verbal learning test (AVLT) was used to evaluate memory. (iii) The Hamilton Depression Rating Scale (HAMD) was used to assess depressive symptoms. (iv) Daily function was assessed using the Lawton-Brody activities of daily living (ADL) scale (Salmon and Bondi, 2009). Testing was administered by board-certified neuropsychologists and research staff under the supervision of neuropsychologists.
Olfactory Identification Assessment
The Chinese smell identification test (CSIT) developed by the Institute of Psychology, Chinese Academy of Sciences, was applied to evaluate OI performance (Feng et al., 2019). The CSIT consists of two parts. The first part is a self-assessment questionnaire (CSIT-self) that surveys medical factors that may confound olfactory function (septal deviation, difficulty breathing through one side of the nose, history of radiation or chemotherapy, history of nasal surgery) (Tabert et al., 2005). Participants were asked to rate their sense of smell relative to others on a 5-point scale as follows: 1 = poor, 2 = low, 3 = normal, 4 = good, and 5 = superior (to others).
The second part (CSIT-OI) includes olfactory tests of 40 familiar and easy to recognize odors such as strawberry, jujube, haw, and sesame oil. Subjects were required to name each odor from a list of four alternatives. The CSIT-OI test method is similar to the 40-item UPSIT (Doty et al., 1984), but CSIT is more suitable for people with a Chinese cultural background. Odorants of the CSIT were presented in felt-tip pens (Hummel et al., 1997), each filled with 1 ml of liquid. The cap of the pen was removed and the pen tip was placed approximately 2 cm in front of the subject’s nostrils. Participants were requested to sniff the presented odor for 5 s then to pick the correct response. The tests were carried out in an environment with efficient air circulation and no other odors. The CSIT-IO score is the total of correct choices for the 40 odors.
Imaging Data Acquisition
Structural MRI images were acquired on a General Electric HD 750 w 3.0 T MRI scanner with an 8-channel head-coil (General Electric, Waukesha, WI, United States). Structural imaging included T1-weighted three-dimensional (3D-T1), axial T2-weighted, and fluid-attenuated inversion recovery images. According to axial T2-weighted and fluid-attenuated inversion recovery images, subjects with abnormalities other than atrophy, or leukoaraiosis were excluded. The 3D-T1 images were collected using a fast-spoiled gradient recalled echo sequence [TR = 8.5 ms, TE = 3.2 ms, Inversion time (TI) = 450 ms, Matrix 256 × 256, FOV 256 mm × 256 mm, flip angle = 12°, and slice thickness of 1.0 mm without intervals]. The total scan duration for 3D-T1 image acquisition was 4 min, 30 s.
Image Processing and VBM Analyses
The VBM8 toolbox1, a software package based on statistical parametric mapping software (SPM 82) was used for VBM analyses. VBM8 was used to calculate GMV corrected for total intracranial volume, age, sex, and education level. T1-weighted images were segmented into GM, white matter, and cerebrospinal fluid using a fully automated algorithm in SPM 8. Images were normalized by diffeomorphic anatomical registration through exponentiated Lie algebra normalization, and transformed to Montreal Neurological Institute space to preserve local differences in anatomy across subjects, thereby allowing quantification. Finally, the normalized GM images were smoothed for statistical analysis (Wang et al., 2017).
For ROI-level analysis, we used SPM 8 for automatic anatomic segmentation and volumetric measurement of brain structures. We adopted the anatomical automatic labeling (AAL)-based structural ROI method for ex vivo measurement of each individual ROI signal (Tzourio-Mazoyer et al., 2002), and used the extracted signal for subsequent analysis. In this study, the ROIs were the hippocampus, amygdala, and OC, brain regions strongly related to olfactory processing. A voxel-based analysis was then applied over the whole brain to explore regions associated with CSIT score in AD, MCI, and NC groups.
Statistics Analysis
Statistical analyses of behavioral data were conducted using SPSS for Windows (22.0, IBM). Sex ratios were compared across diagnostic groups (AD, MCI, and NC) using the chi-square test. Demographic variables such as age, education level, neuropsychological features, and CSIT scores were compared across groups by one-way analysis of covariance (ANCOVA) (Vasavada et al., 2015) followed by a post hoc Bonferroni test for multiple comparisons. For non-normally distributed data [denoted by Md (p25,p75)], we used Kruskal–Wallis analysis of variance (ANOVA) to evaluate differences among groups followed by a Dunn–Bonferroni test for post hoc comparisons. The effectiveness of CSIT for identifying AD, MCI, and NC was assessed by receiver operating characteristic curve (ROC) analysis. The sensitivity and false positive rate (1 – specificity) of CSIT-OI, CSIT-self, and CSIT-OI + self was calculated for ROC curves, and the area under the ROC curve was used to determine classification accuracy. A α < 0.05 (two-tailed) was considered significant for all tests.
Spearman correlation analysis was performed between CSIT scores and mean GMV of each ROI (OC, amygdala, and hippocampus). Significant correlations between CSIT and GMV of each ROI were corrected for false discovery rate. The mean GMV of each ROI was also compared between groups by ANCOVA with sex, age, and education as covariates. At the whole-brain voxel level, behavior – neuroimaging correlation analysis was conducted using SPM 8 with sex, age, and education as covariates. The statistical maps were thresholded using the Gaussian random field (GRF) correction with a voxel-level threshold of P < 0.001 and a cluster-level threshold of P < 0.05.
Results
Clinical Characteristics of the Study Cohort
The demographic and baseline clinical characteristics of the study subjects are summarized in Table 1. There were no significant differences in age, sex ratio, family history, WMH score, and vascular risk factors among the three groups. However, there were significant differences in years of education (AD = 6.78 ± 5.26, MCI = 8.48 ± 5.53, NC = 12.20 ± 4.44; p < 0.001).
Neuropsychological Deficits in MCI and AD
There were significant differences in several neuropsychological test outcomes among the three groups as revealed by one-way ANOVA (Table 2). As expected, MMSE (p < 0.001) and CDR (p < 0.001) differed markedly, with significantly lower scores in the AD group compared to MCI and NC groups. In addition, there were significant inter-group differences in AVLT (immediate, delay, or recognition; p < 0.001). Daily functions were significantly impaired only in AD patients; both MCI and AD groups showed a gradual decline, but a worse performance was observed in the latter patients (Table 2). Mean HAMD scores were higher in AD and MCI groups than in the NC group, and higher in MCI than AD (Table 2). The CSIT-OI score was positively correlated with AVLT [delay] and AVLT [recognize] and negatively correlated with CDR in AD patients but not in the other groups (Table 3).
CSIT Scores Distinguish MCI and AD From Age-Matched Controls
Group Differences in CSIT-OI and CSIT-Self Scores
There were significant differences in CSIT-OI score among groups, with significantly lower scores (indicating poorer OI) in the AD and MCI group compared to NCs (p < 0.001), and lower mean score in AD than MCI (Figure 1 and Table 2). The CSIT-self score was also lower in AD and MCI groups compared to NCs. The CSIT-self score was positively correlated with CSIT-OI for the entire cohort (R = 0.415; p < 0.001); however, the correlation did not reach significance within individual diagnostic groups due to lack of statistical power (p > 0.05).
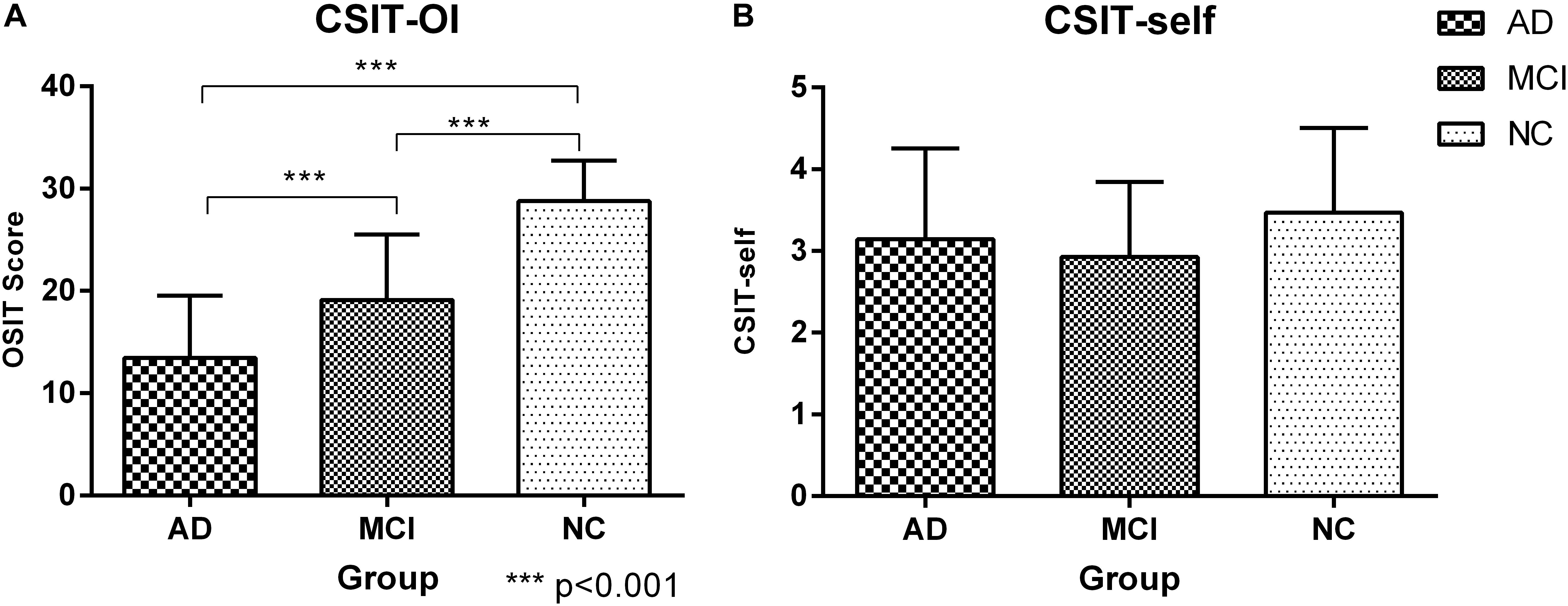
Figure 1. Histogram of CSIT scores for all participants in this study. (A) The CSIT-OI scores of patients with AD and MCI were lower than NC, and the scores of AD were the lowest and there were significant statistical differences (P < 0.001); ∗∗∗<0.001. (B) There was no difference in the CSIT-self scores of the three groups (P > 0.05).
Power of Discrimination
Patients vs. normal controls
In the ROC analysis, both CSIT-OI score and combined CSIT-OI plus CSIT-self score distinguished AD from NC and MCI from NC, while CSIT-self score alone did not (note that AUC = 0.5 indicates no discriminative power) (Figures 2A,B and Table 4). Using a cut-off value of 26, CSIT-OI score distinguished MCI from NC with 80.0% sensitivity and 89.0% specificity; using a cut-off value of 22.5 distinguished AD from NC with 93% sensitivity and 95% specificity.
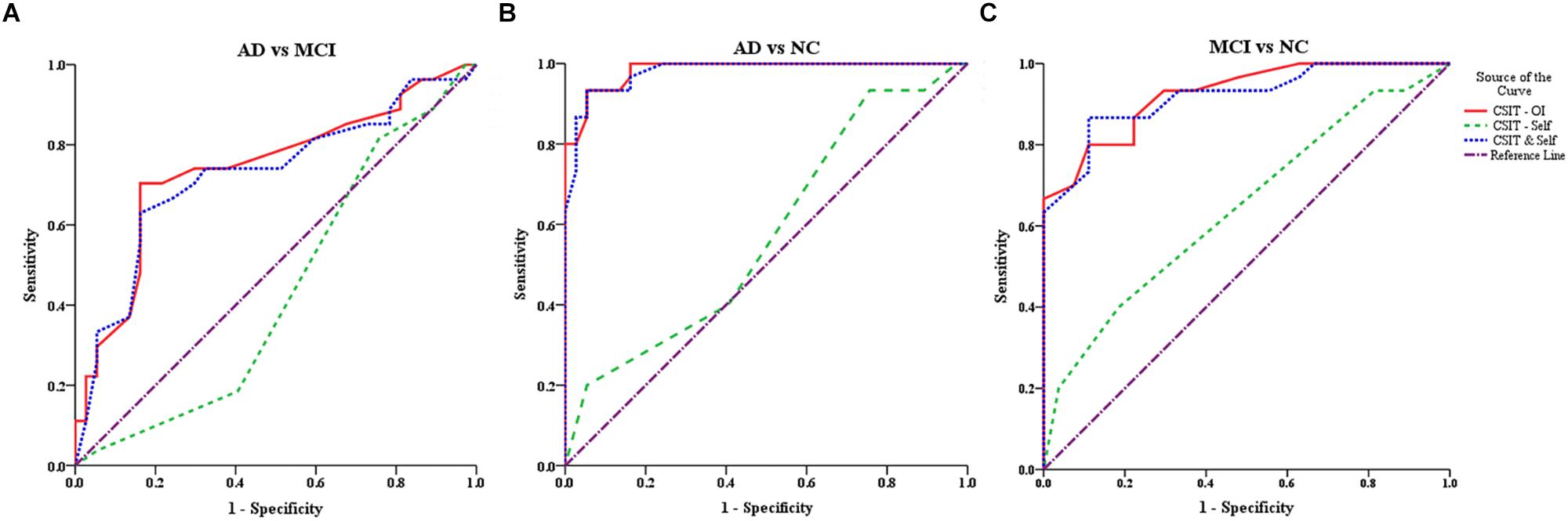
Figure 2. ROC curves for the CSIT-OI, CSIT-Self and CSIT-OI + Self (A–C). (A) ROC curves for the CSIT-OI, CSIT-self, and CSIT-OI + Self between AD and MCI. (B) ROC curves for the CSIT-OI, CSIT-self, and CSIT-OI + Self between AD and NC. (C) ROC curves for the CSIT-OI, CSIT-self, and CSIT-OI + Self between MCI and NC. The x-axis indicates the error of the second kind (100%-specificity). The y-axis indicates sensitivity. The area under the curve (AUC) shows the discriminative power between the two groups. The diagonal from (0,0) to (100,100) with AUC = 0.5 indicates a total lack of discriminative power.
AD vs. MCI
According to ROC analysis, CSIT-OI score and CSIT-OI plus CSIT-self score also distinguished AD from MCI, while again CSIT-self score did not (Figure 2C and Table 4). Using a cutoff value of 18.5, CSIT-OI score distinguished AD from MCI with 70.4% sensitivity and 83.8% specificity.
Correlations Between CSIT Scores and Regional GMV Values at the ROI and Voxel Levels
At the ROI level, CSIT-OI scores of AD patients were positively correlated with left amygdala volume (r = 0.38, p = 0.046), with a trend observed for the left hippocampus (r = 0.35, p = 0.051) (Table 5). At the voxel level, CSIT-OI score was associated with volumes of the left inferior frontal gyrus (IFG, peak location x = –33, y = 20, z = –18, peak intensity = 0.612) and left precentral gyrus (peak location: x = –42, y = –5, z = 33, peak intensity = 0.707) (p < 0.05; Figure 3 and Table 5). There was a significant relationship between CSIT-OI score and GMV in the all participants at the ROI or voxel level, but no significant relationships were found between CSIT-OI score and GMV in the MCI and NC groups at either the ROI or voxel level (Table 5).
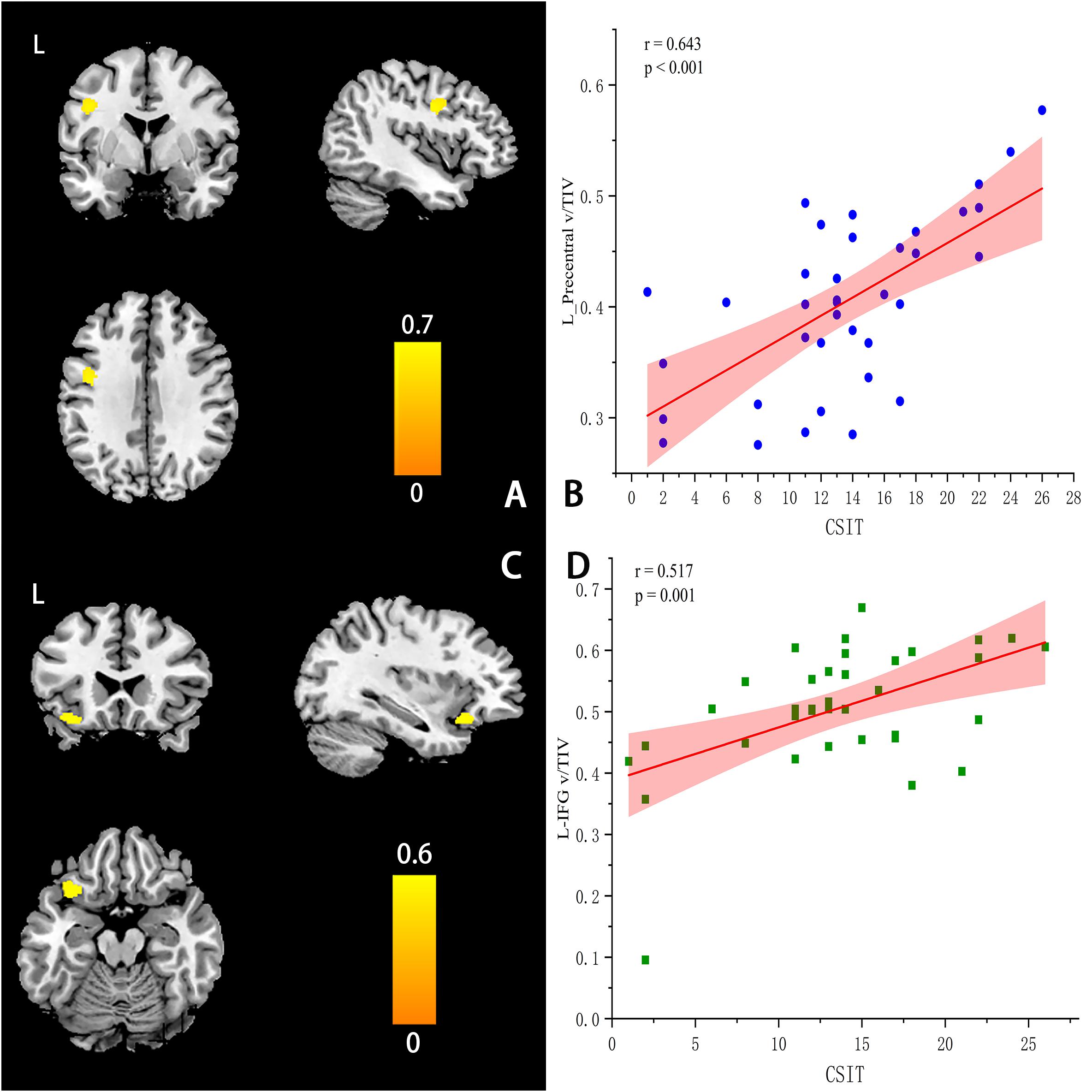
Figure 3. Correlations between CSIT scores and regional GMV values at the voxel levels (A–D). (A) The location of left-Precentral Gyrus (L-PG) (peak MINI: x = −42, y =–5, z = 33, Peak intensity: 0.707). (C) The location of left-Inferior Frontal Gyrus (L-IFG) (peak MINI x = –33, y = 20, z = –18, Peak intensity = 0.612). (B,D) The scatter plot and linear fit show the relationship between the GMV of L-PG & L-IFG and CSIT score in AD. The results suggested that the GMV of L-PG (r = 0.643, p < 0.001) & L – IFG (r = 0.517, p = 0.001) were significantly correlated with the CSIT scores in AD.
Discussion
We demonstrate that OI as measured by the CSIT is impaired in AD and MCI patients compared to age-matched healthy controls in the Chinese population, and that the severity of OI dysfunction can distinguish AD and MCI patients from controls and AD from MCI with high sensitivity and specificity. In addition, CSIT scores were significantly associated with specific memory assessment outcomes but not with measures of general cognitive function such as MMSE score. Based on ROI-level GMV analysis, the OI of AD patients was significantly correlated with left amygdala volume, with a similar trend observed for the left hippocampus (but not bilateral OC, right amygdala, or hippocampus); meanwhile, a voxel-level analysis revealed that the OI of AD patients was also associated with the volumes of precentral gyrus-L and IFG-L. In contrast, no such CSIT-GMV associations were found in the MCI and NC groups. Therefore, OI dysfunction as assessed by a culturally appropriate test (the CSIT) may be a convenient AD screening tool.
This study confirmed that OI is impaired in ethnic Chinese AD and MCI patients, consistent with previous studies in Western populations. In all three diagnostic groups (MCI, AD, and NC), OI decreased progressively with age (Larsson et al., 2000; Devanand et al., 2014; Roalf et al., 2017; Silva et al., 2018). There was also a disconnect between objective and subjective OI capacity, as AD patients did not rate their sense of smell as inferior despite lower CSIT-OI scores. In line with our findings (Doty et al., 1984; Burns, 1998) reported that only 6% of AD patients complained of olfactory dysfunction, while 90% had actual olfactory deficits as demonstrated by olfactory tests. Alternatively, MCI patients did provide relatively low self-assessments (Yu et al., 2018). This may result from impaired self-concept in AD but not in MCI. Further, average score among AD patients (13/40 = 32.5%) was near the chance level of 25%, indicating that sense of smell was substantially degraded. Therefore, it is necessary to measure olfactory function using sensitive and objective olfactory tests. Thus, OI may not be suitable for gauging AD progression but could be useful for measuring MCI progression and deficits during normal aging.
Although CSIT is based on UPSIT, group differences in scores were greater than in past studies using the UPSIT, suggesting better discriminative efficacy. Our ROC analysis suggests that the CSIT can distinguish MCI and AD patients from normal elderly individuals and accurately distinguish AD from MCI. This level of discrimination is similar to cerebral spinal fluid (CSF) biomarkers, only slightly inferior to amyloid imaging and structural MRI, and significantly greater than UPSIT (sensitivity: 0.88, specificity: 0.91) (Devanand, 2016; Hagemeier et al., 2016). The OI test classifies individuals showing cognitive decline correctly at a higher rate than a global cognitive test (Graves et al., 1999). Consistent with these findings, CSIT-OI score was positively correlated with memory function (AVLT-delay and -recognition), and negatively correlated with the severity of cognitive impairment (CDR score) in AD patients. This demonstrates that the OI deficit in AD is mainly related to impairment of semantic (cognitive) rather than perceptual processing (Lehrner et al., 1999). However, the CSIT-OI score was not associated with MMSE scores in AD patients, consistent with previous studies (Roalf et al., 2017; Silva et al., 2018), possibly because the MMSE is a general test of cognition that does not provide information on specific cognitive dysfunctions in AD. Therefore, we conclude that CSIT is more suitable for use in the Chinese Han population than the UPSIT, and is a useful biomarker for cognitive deficit.
Volumes of left IFG and precentral cortex were significantly associated with OI performance in AD patients, a finding that to the best of our knowledge has not been reported previously. While previous reports have indicated that OI is related to the olfactory bulb, OC, hippocampus, and parahippocampus, few have found associated changes in motor-related areas. The precentral cortex is an important component of the motor network (Yousry et al., 1997; Hopkins et al., 2017). In addition, however, recent reports have found that the precentral cortex participates in a variety of perceptual and integrative processes (Chen et al., 2008), including contributions to cognitive functions such as language and executive control (Ferreira et al., 2017; Mugler et al., 2018). Bi et al. (2018) found significant changes in the precentral cortex volume of AD patients compared to NCs. In addition, Rizzo et al. (2018) found that the fiber bundle connection between the amygdala and precentral gyrus is abnormal in AD. Furthermore, the strength of functional connections between posteromedial and precentral cortices was reduced, which is significantly correlated with cognitive function in AD (Wu et al., 2016). The IFG is part of Broca’s area, which is involved in semantic processing and phoneme production (Mugler et al., 2018), and some studies have also reported a relationship with memory function (Smith and Jonides, 1999). The IFG does not contribute to the processing of single syllables, but rather acts in combination with the precentral gyrus for responding to understood speech, including the ordering of multiple syllables. A number of functional imaging studies have demonstrated that L-IFG and L-precentral gyrus regions are active during speech perception and comprehension (Wilson et al., 2004; Pulvermuller et al., 2006), particularly when participants listen attentively to speech signals that are noisy or degraded (Hervais-Adelman et al., 2012; Wild et al., 2012). On the ROI level, the volume of the left amygdala was significantly associated with OI performance in AD patients, suggesting that structural changes in the left amygdala are due to involvement of the olfactory network. Based on previous reports and our findings, we speculate that functional anomalies in the precentral gyrus, IFG, amygdala, and hippocampus lead to defective OI in AD patients, and that the amygdala–precentral gyrus pathway plays a predominant role in OI. Unraveling the specific contributions of this precentral gyrus shrinkage to OI deficits requires further research.
This study has several limitations. First, our AD patient group included both early-onset and late-onset patients, which may introduce heterogeneity to morphometric changes. A previous study found that patients with early-onset AD showed bilateral reductions in the medial temporal lobes, inferior parietal lobules, precuneus and perisylvian cortices, and cingulate cortices, as well as in the right inferior frontal gyrus, whereas late-onset patients with AD showed atrophy only in bilateral medial temporal cortices. Second, we did not compare differences between AD patients with and without olfactory dysfunction, and such a comparison could also reveal mechanisms underlying OI disorders. Third, this is a cross-sectional rather than a longitudinal study, so we do not know if these differences were caused by the progression of AD. Fourth, we excluded subjects who engaged in long-term smoking and drinking, as these behaviors affect cognition and olfaction (Frye, 1990). This could result in selection bias but better reflects central mechanisms of olfactory recognition (Vasavada et al., 2017). Lastly, the sample was small; therefore, studies in a larger population are needed in order to confirm whether this measure can consistently distinguish controls from AD patients.
Conclusion
Our study confirms that the CSIT is a culturally appropriate olfactory recognition test for the Chinese Han population that can effectively distinguish among AD, MCI, and healthy aging. Gray matter voxel-based MRI analysis demonstrated that OI is more strongly related to the left cerebral hemisphere (left hippocampus, left amygdala, left-precentral gyrus, left-IFG, and bilateral olfactory cortex) than to the right hemisphere (right hippocampus and right amygdala). We also found that the left-precentral gyrus and left-IFG may be involved in OI. We therefore speculate that language processing may contribute to the expression of olfactory recognition, and that AD patients are impaired in this domain. However, further research is needed to elucidate the precise mechanisms for OI dysfunction and relationships to other aspects of MCI and AD pathology.
Data Availability
All datasets generated for this study are included in the manuscript and/or the supplementary files.
Ethics Statement
The AD and MCI patients were recruited from the Dysmnesia Outpatient Department at the First Affiliated Hospital of Anhui Medical University, Anhui Province, China. The NCs were recruited from the local community through advertisement or were the spouses of the study patients. This study was approved by the Research Ethics Committee of the First Affiliated Hospital of Anhui Medical University. All subjects gave written informed consent in accordance with the Declaration of Helsinki.
Author Contributions
XW performed the analysis and wrote the manuscript. ZG, SZ, LW, WZ, and YY helped to collect the behavioral and imaging data. TB and G-JJ helped in MRI data analysis. YT and KW designed and supervised the study.
Funding
This work was supported by the National Key R&D Program of China (Grant Nos. 2016YFC1306400, 2016YFC1305904, 2018YFC1314504, and 2018YFC1314200), the National Natural Science Foundation of China (Grant Nos. 91432301, 91732303, and 81771817), and the Provincial Natural Science Foundation Project of Anhui (Grant No. 1608085MH169).
Conflict of Interest Statement
The authors declare that the research was conducted in the absence of any commercial or financial relationships that could be construed as a potential conflict of interest.
Acknowledgments
We thank the participants for their cooperation during this study and WZ for providing the CSIT Kit.
Footnotes
References
Albert, M. S., DeKosky, S. T., Dickson, D., Dubois, B., Feldman, H. H., Fox, N. C., et al. (2011). The diagnosis of mild cognitive impairment due to Alzheimer’s disease: recommendations from the National Institute on Aging-Alzheimer’s association workgroups on diagnostic guidelines for Alzheimer’s disease. Alzheimers Dement 7, 270–279. doi: 10.1016/j.jalz.2011.03.008
Ayabe-Kanamura, S., Schicker, I., Laska, M., Hudson, R., Distel, H., Kobayakawa, T., et al. (1998). Differences in perception of everyday odors: a Japanese-German cross-cultural study. Chem. Senses 23, 31–38. doi: 10.1093/chemse/23.1.31
Baazaoui, N., and Iqbal, K. (2018). A novel therapeutic approach to treat Alzheimer’s disease by neurotrophic support during the period of synaptic compensation. J. Alzheimers Dis. 62, 1211–1218. doi: 10.3233/JAD-170839
Bi, X. A., Jiang, Q., Sun, Q., Shu, Q., and Liu, Y. (2018). Analysis of Alzheimer’s disease based on the random neural network cluster in fMRI. Front. Neuroinform. 12:60. doi: 10.3389/fninf.2018.00060
Burns, A. (1998). Key papers in geriatric psychiatry: mini-mental state: a practical method for grading the cognitive state of patients for the clinician. M. Folstein, S. Folstein and P. McHugh, journal of psychiatric research (1975) 12, 189-198. Int. J. Geriatr. Psych. 13, 285–285.
Cavedo, E., Boccardi, M., Ganzola, R., Canu, E., Beltramello, A., Caltagirone, C., et al. (2011). Local amygdala structural differences with 3T MRI in patients with Alzheimer disease. Neurology 2011:8.
Chen, J. L., Penhune, V. B., and Zatorre, R. J. (2008). Listening to musical rhythms recruits motor regions of the brain. Cereb. Cortex 18, 2844–2854. doi: 10.1093/cercor/bhn042
Claire Murphy, T. L. (2003). Jernigan,christine fennema, left hippocampal volume loss in Alzheimer’s disease is reflected in performance on odor identification: a structural MRI study. J. Int. Neuropsychol. Soc. 2003:13.
Devanand, D., Lee, S., Manly, J., Andrews, H., Schupf, N., Brickman, A. M., et al. (2014). Olfactory identification deficits predict the transition from MCI to Ad in a multi-ethnic community sample. Alzheimer Dement. 10:803.
Devanand, D. P. (2000). Olfactory deficits in patients with mild cognitive impairment predict Alzheimer’s disease at follow-up. Am. J. Psychiatry 2000:7.
Devanand, D. P. (2016). Olfactory identification deficits, cognitive decline, and dementia in older adults. Am. J. Geriatr. Psychiatry 24, 1151–1157. doi: 10.1016/j.jagp.2016.08.010
Devanand, D. P., Michaels-Marston, K. S., Liu, X. H., Pelton, G. H., Padilla, M., Marder, K., et al. (2000). Olfactory deficits in patients with mild cognitive impairment predict Alzheimer’s disease at follow-up. Am. J. Psychiat. 157, 1399–1405. doi: 10.1176/appi.ajp.157.9.1399
Dintica, C. S., Marseglia, A., Rizzuto, D., Wang, R., Seubert, J., Arfanakis, K., et al. (2019). Impaired olfaction is associated with cognitive decline and neurodegeneration in the brain. Neurology 92, e700–e709. doi: 10.1212/WNL.0000000000006919
Djordjevic, J., Jones-Gotman, M., De Sousa, K., and Chertkow, H. (2008). Olfaction in patients with mild cognitive impairment and Alzheimer’s disease. Neurobiol. Aging 29, 693–706.
Doty, R. L., Shaman, P., and Dann, M. (1984). Development of the university of pennsylvania smell identification smell identification test - a standardized microencapsulated test of olfactory function. Physiol. Behav. 32, 489–502. doi: 10.1016/0031-9384(84)90269-5
Dubois, B., Feldman, H. H., Jacova, C., DeKosky, S. T., Barberger-Gateau, P., Cummings, J., et al. (2007). Research criteria for the diagnosis of Alzheimer’s disease: revising the NINCDS–ADRDA criteria. Lancet Neurol. 6, 734–746. doi: 10.1016/s1474-4422(07)70178-3
Ewers, M., Walsh, C., Trojanowski, J. Q., Shaw, L. M., Petersen, R. C., Jack, C. R., et al. (2012). Prediction of conversion from mild cognitive impairment to Alzheimer’s disease dementia based upon biomarkers and neuropsychological test performance. Neurobiol. Aging 33, 1203–1214. doi: 10.1016/j.neurobiolaging.2010.10.019
Fazekas, F., Chawluk, J. B., Alavi, A., Hurtig, H. I., and Zimmerman, R. A. (1987). MR signal abnormalities at 1.5-T in Alzheimer dementia and normal aging. Am. J. Roentgenol. 149, 351–356. doi: 10.2214/ajr.149.2.351
Feng, G., Zhuang, Y., Yao, F., Ye, Y., Wan, Q., and Zhou, W. (2019). Development of the Chinese smell identification test. Chem. Senses 44, 189–195. doi: 10.1093/chemse/bjz006
Ferreira, D., Machado, A., Molina, Y., Nieto, A., Correia, R., Westman, E., et al. (2017). Cognitive variability during middle-age: possible association with neurodegeneration and cognitive reserve. Front. Aging Neurosci. 9:188. doi: 10.3389/fnagi.2017.00188
Graves, A. B., Bowen, J. D., Rajaram, L., McCormick, W. C., McCurry, S. M., Schellenberg, G. D., et al. (1999). Impaired olfaction as a marker for cognitive decline - interaction with apolipoprotein E epsilon 4 status. Neurology 53, 1480–1487.
Hagemeier, J., Woodward, M. R., Rafique, U. A., Amrutkar, C. V., Bergsland, N., Dwyer, M. G., et al. (2016). Odor identification deficit in mild cognitive impairment and Alzheimer’s disease is associated with hippocampal and deep gray matter atrophy. Psychiatry Res. Neuroimaging 255, 87–93. doi: 10.1016/j.pscychresns.2016.08.003
Hamann, S. (2001). Cognitive and neural mechanisms of emotional memory. Trends Cogn. Sci. 5, 394–400. doi: 10.1016/s1364-6613(00)01707-1
Helenius, J., Mayasi, Y., and Henninger, N. (2017). White matter hyperintensity lesion burden is associated with the infarct volume and 90-day outcome in small subcortical infarcts. Acta Neurol. Scand. 135, 585–592. doi: 10.1111/ane.12670
Hervais-Adelman, A. G., Carlyon, R. P., Johnsrude, I. S., and Davis, M. H. (2012). Brain regions recruited for the effortful comprehension of noise-vocoded words. Lang. Cogn. Process. 27, 1145–1166. doi: 10.1080/01690965.2012.662280
Hopkins, W. D., Meguerditchian, A., Coulon, O., Misiura, M., Pope, S., Mareno, M. C., et al. (2017). Motor skill for tool-use is associated with asymmetries in Broca’s area and the motor hand area of the precentral gyrus in chimpanzees (Pan troglodytes). Behav. Brain Res. 318, 71–81. doi: 10.1016/j.bbr.2016.10.048
Hummel, T., Sekinger, B., Wolf, S. R., Pauli, E., and Kobal, G. (1997). ‘Sniffin’ Sticks’: olfactory performance assessed by the combined testing of odor identification, odor discrimination and olfactory threshold. Chem. Senses 22, 39–52. doi: 10.1093/chemse/22.1.39
Ji, G.-J., Liao, W., Chen, F.-F., Zhang, L., and Wang, K. (2017). Low-frequency blood oxygen level-dependent fluctuations in the brain white matter: more than just noise. Sci. Bull. 62, 656–657. doi: 10.1016/j.scib.2017.03.021
Kjelvik, G., Saltvedt, I., White, L. R., Stenumgård, P., Sletvold, O., Engedal, K., et al. (2014). The brain structural and cognitive basis of odor identification deficits in mild cognitive impairment and Alzheimer’s disease. BMC Neurol. 2014:10.
Kobayashi, M., Saito, S., Kobayakawa, T., Deguchi, Y., and Costanzo, R. M. (2006). Cross-cultural comparison of data using the odor stick identification test for Japanese (OSIT-J). Chem. Senses 31, 335–342. doi: 10.1093/chemse/bjj037
Larsson, M., Finkel, D., and Pedersen, N. L. (2000). Odor identification: influences of age, gender, cognition, and personality. J. Gerontol. B.Psychol. 55, 304–310.
Lehrner, J. P., Walla, P., Laska, M., and Deecke, L. (1999). Different forms of human odor memory: a developmental study. Neurosci. Lett. 272, 17–20. doi: 10.1016/s0304-3940(99)00566-2
Lezak, M. D., Howieson, D. B., Loring, D. W., and Fischer, J. S. (2004). Neuropsychological Assessment. New York, NY: Oxford University Press.
Liu, Y. W., Spulber, G., Lehtimaki, K. K., Kononen, M., Hallikainen, I., Grohn, H., et al. (2011). Diffusion tensor imaging and tract-based spatial statistics in Alzheimer’s disease and mild cognitive impairment. Neurobiol. Aging 32, 1558–1571. doi: 10.1016/j.neurobiolaging.2009.10.006
Marigliano, V., Gualdi, G., Servello, A., Marigliano, B., Volpe, L. D., Fioretti, A., et al. (2014). Olfactory deficit and hippocampal volume loss for early diagnosis of Alzheimer Disease a pilot study. Alzheimer Dis. Assoc. Disord. 28:4. doi: 10.1097/WAD.0b013e31827bdb9f
McKhann, G., Drachman, D., Folstein, M., Katzman, R., Price, D., and Stadlan, E. M. (1984). Clinical-diagnosis of Alzheimers disease report of the NINCADS-ADRDA work group under the auspices of department of health and human services task foecr on Alzheimers disease. Neurology 34, 939–944.
McKhann, G. M., Knopman, D. S., Chertkow, H., Hyman, B. T., Jack, C. R., Kawas, C. H., et al. (2011). The diagnosis of dementia due to Alzheimer’s disease: recommendations from the national institute on Aging-Alzheimer’s association workgroups on diagnostic guidelines for Alzheimer’s disease. Alzheimers Dement. 7, 263–269.
Mesholam, R. I. (1998). Olfaction in neurodegenerative disease a meta-analysis of olfactory functioning in Alzheimer’s and Parkinson’s diseases. Arch. Neurol. 1998:7.
Miller, G. (2012). Stopping Alzheimer’s before it starts. Science 337, 790–792. doi: 10.1126/science.337.6096.790
Morris, J. C. (1993). The clinical dementia rating (CDR) - current version and scoring rules. Neurology 43, 2412–2414.
Mugler, E. M., Tate, M. C., Livescu, K., Templer, J. W., Goldrick, M. A., and Slutzky, M. W. (2018). Differential representation of articulatory gestures and phonemes in precentral and inferior frontal gyri. J. Neurosci. 38, 9803–9813. doi: 10.1523/JNEUROSCI.1206-18.2018
Peters, J. M. (2003). Olfactory function in mild cognitive impairment and Alzheimer’s disease: an investigation using psychophysical and electrophysiological techniques. Am. J. Psychiatry 2003:8.
Petersen, R. C. (2007). Mild cognitive impairment: current research and clinical implications. Semin. Neurol. 27, 22–31.
Petersen, R. C., Doody, R., Kurz, A., Mohs, R. C., Morris, J. C., Rabins, P. V., et al. (2001). Current concepts in mild cognitive impairment. Arch. Neurol. 58, 1985–1992.
Petersen, R. C., Smith, G. E., Waring, S. C., Ivnik, R. J., Tangalos, E. G., and Kokmen, E. (1999). Mild cognitive impairment: clinical characterization and outcome. Arch. Neurol. 56, 303–308.
Phelps, E. A. (2004). Human emotion and memory: interactions of the amygdala and hippocampal complex. Curr. Opin. Neurobiol. 14, 198–202. doi: 10.1016/j.conb.2004.03.015
Prestia, A., Drago, V., Rasser, P. E., Bonetti, M., Thompson, P. M., and Frisoni, G. B. (2010). Cortical changes in incipient Alzheimer’s disease. J. Alzheimers. Dis. 22, 1339–1349. doi: 10.3233/jad-2010-101191
Price, J. L. (2003). “Comparative aspects of amygdala connectivity,” in Amygdala in Brain Function: Bacic and Clinical Approaches, eds P. ShinnickGallagher, A. Pitkanen, A. Shekhar, and L. Cahill, (New York: New York Acadmic Sciences), 50–58. doi: 10.1111/j.1749-6632.2003.tb07070.x
Pulvermuller, F., Huss, M., Kherif, F., Martin, F., Hauk, O., and Shtyrov, Y. (2006). Motor cortex maps articulatory features of speech sounds. Proc. Natl. Acad. Sci. U.S.A. 103, 7865–7870. doi: 10.1073/pnas.0509989103
Ritchie, C., Smailagic, N., Noel-Storr, A. H., Takwoingi, Y., Flicker, L., Mason, S. E., et al. (2014). Plasma and cerebrospinal fluid amyloid beta for the diagnosis of Alzheimer’s disease dementia and other dementias in people with mild cognitive impairment (MCI)*. Cochrane Database Syst. Rev. CD008782. doi: 10.1002/14651858.CD008782.pub4
Rizzo, G., Milardi, D., Bertino, S., Basile, G. A., Di Mauro, D., Calamuneri, A., et al. (2018). The limbic and sensorimotor pathways of the human amygdala: a structural connectivity study. Neuroscience 385, 166–180. doi: 10.1016/j.neuroscience.2018.05.051
Roalf, D. R., Moberg, M. J., Turetsky, B. I., Brennan, L., Kabadi, S., Wolk, D. A., et al. (2017). A quantitative meta-analysis of olfactory dysfunction in mild cognitive impairment. J. Neurol. Neurosurg. Psychiatry 88, 226–232. doi: 10.1136/jnnp-2016-314638
Roberts, R. O., Christianson, T. J., Kremers, W. K., Mielke, M. M., Machulda, M. M., Vassilaki, M., et al. (2016). Association between olfactory dysfunction and amnestic mild cognitive impairment and Alzheimer Disease Dementia. JAMA Neurol. 73, 93–101. doi: 10.1001/jamaneurol.2015.2952
Salmon, D. P., and Bondi, M. W. (2009). Neuropsychological assessment of dementia. Annu. Rev. Psychol. 60, 257–282. doi: 10.1146/annurev.psych.57.102904.190024
Schab, F. R. (1991). Odor memory - taking stock. Psychol. Bull. 109, 242–251. doi: 10.1037//0033-2909.109.2.242
Schubert, C. R., Carmichael, L. L., Murphy, C., Klein, B. E. K., Klein, R., and Cruickshanks, K. J. (2008). Olfaction and the 5-year incidence of cognitive impairment in an epidemiological study of older adults. J. Am. Geriatr. Soc. 56, 1517–1521. doi: 10.1111/j.1532-5415.2008.01826.x
Servello, A., Fioretti, A., Gualdi, G., Di Biasi, C., Pittalis, A., Sollaku, S., et al. (2015). Olfactory dysfunction, olfactory bulb volume and Alzheimer’s disease: is there a correlation? a pilot study1. J. Alzheimers Dis. 48, 395–402. doi: 10.3233/JAD-150232
Silva, M. M. E., Mercer, P. B. S., Witt, M. C. Z., and Pessoa, R. R. (2018). Olfactory dysfunction in Alzheimer’s disease systematic review and meta-analysis. Dement Neuropsychol. 12, 123–132. doi: 10.1590/1980-57642018dn12-020004
Smith, E. E., and Jonides, J. (1999). Neuroscience - storage and executive processes in the frontal lobes. Science 283, 1657–1661. doi: 10.1126/science.283.5408.1657
Tabert, M. H., Liu, X., Doty, R. L., Serby, M., Zamora, D., Pelton, G. H., et al. (2005). A 10-item smell identification scale related to risk for Alzheimer’s disease. Ann. Neurol. 58, 155–160. doi: 10.1002/ana.20533
Tahmasebi, R., Zehetmayer, S., Pusswald, G., Kovacs, G., Stogmann, E., and Lehrner, J. (2019). Identification of odors, faces, cities and naming of objects in patients with subjective cognitive decline, mild cognitive impairment and Alzheimer s disease: a longitudinal study. Int. Psychogeriatr. 31, 537–549. doi: 10.1017/S1041610218001114
Thomann, P. A., Dos Santos, V., Seidl, U., Toro, P., Essig, M., and Schroder, J. (2009a). MRI-derived atrophy of the olfactory bulb and tract in mild cognitive impairment and Alzheimer’s disease. J. Alzheimers Dis. 17, 213–221. doi: 10.3233/JAD-2009-1036
Thomann, P. A., Dos Santos, V., Toro, P., Schonknecht, P., Essig, M., and Schroder, J. (2009b). Reduced olfactory bulb and tract volume in early Alzheimer’s disease–a MRI study. Neurobiol. Aging 30, 838–841. doi: 10.1016/j.neurobiolaging.2007.08.001
Tzourio-Mazoyer, N., Landeau, B., Papathanassiou, D., Crivello, F., Etard, O., Delcroix, N., et al. (2002). Automated anatomical labeling of activations in SPM using a macroscopic anatomical parcellation of the MNI MRI single-subject brain. Neuroimage 15, 273–289. doi: 10.1006/nimg.2001.0978
Vasavada, M. M., Martinez, B., Wang, J., Eslinger, P. J., Gill, D. J., Sun, X., et al. (2017). Central olfactory dysfunction in Alzheimer’s disease and mild cognitive impairment: a functional MRI study. J. Alzheimers Dis. 59, 359–368. doi: 10.3233/JAD-170310
Vasavada, M. M., Wang, J. L., Eslinger, P. J., Gill, D. J., Sun, X. Y., Karunanayaka, P., et al. (2015). Olfactory cortex degeneration in Alzheimer’s disease and mild cognitive impairment. J. Alzheimers Dis. 45, 947–958. doi: 10.3233/JAD-141947
Wang, J. J., Wei, Q., Bai, T. J., Zhou, X. Q., Sun, H., Becker, B., et al. (2017). Electroconvulsive therapy selectively enhanced feedforward connectivity from fusiform face area to amygdala in major depressive disorder. Soc. Cogn. Affect. Neur. 12, 1983–1992. doi: 10.1093/scan/nsx100
Wardlaw, J. M., Smith, E. E., Biessels, G. J., Cordonnier, C., Fazekas, F., Frayne, R., et al. (2013). Neuroimaging standards for research into small vessel disease and its contribution to ageing and neurodegeneration. Lancet Neurol. 12, 822–838. doi: 10.1016/S1474-4422(13)70124-8
Wild, C. J., Yusuf, A., Wilson, D. E., Peelle, J. E., Davis, M. H., and Johnsrude, I. S. (2012). Effortful listening: the processing of degraded speech depends critically on attention. J. Neurosci. 32, 14010–14021. doi: 10.1523/JNEUROSCI.1528-12.2012
Wilson, S. M., Saygin, A. P., Sereno, M. I., and Iacoboni, M. (2004). Listening to speech activates motor areas involved in speech production. Nat. Neurosci. 7, 701–702. doi: 10.1038/nn1263
Woodward, M. R., Amrutkar, C. V., Shah, H. C., Benedict, R. H. B., Rajakrishnan, S., Doody, R. S., et al. (2017). Validation of olfactory deficit as a biomarker of Alzheimer disease. Neurol.Clin. Pract. 7, 5–14. doi: 10.1212/CPJ.0000000000000293
Wu, Y., Zhang, Y., Liu, Y., Liu, J., Duan, Y., Wei, X., et al. (2016). Distinct changes in functional connectivity in posteromedial cortex subregions during the progress of Alzheimer’s disease. Front. Neuroanat. 10:41. doi: 10.3389/fnana.2016.00041
Yousry, T. A., Schmid, U. D., Alkadhi, H., Schmidt, D., Peraud, A., Buettner, A., et al. (1997). Localization of the motor hand area to a knob on the precentral gyrus - A new landmark. Brain 120, 141–157. doi: 10.1093/brain/120.1.141
Keywords: Alzheimer’s disease, mild cognitive impairment, olfactory disorder, Chinese smell identification test, magnetic resonance imaging, voxel-based morphometry
Citation: Wu X, Geng Z, Zhou S, Bai T, Wei L, Ji G-J, Zhu W, Yu Y, Tian Y and Wang K (2019) Brain Structural Correlates of Odor Identification in Mild Cognitive Impairment and Alzheimer’s Disease Revealed by Magnetic Resonance Imaging and a Chinese Olfactory Identification Test. Front. Neurosci. 13:842. doi: 10.3389/fnins.2019.00842
Received: 31 March 2019; Accepted: 26 July 2019;
Published: 14 August 2019.
Edited by:
Beatrice Arosio, University of Milan, ItalyReviewed by:
Johann Lehrner, Medical University of Vienna, AustriaJiaojian Wang, University of Pennsylvania, United States
Copyright © 2019 Wu, Geng, Zhou, Bai, Wei, Ji, Zhu, Yu, Tian and Wang. This is an open-access article distributed under the terms of the Creative Commons Attribution License (CC BY). The use, distribution or reproduction in other forums is permitted, provided the original author(s) and the copyright owner(s) are credited and that the original publication in this journal is cited, in accordance with accepted academic practice. No use, distribution or reproduction is permitted which does not comply with these terms.
*Correspondence: Kai Wang, d2FuZ2thaTE5NjRAMTI2LmNvbQ==; Yongqiang Yu, Y2pyLnl1eW9uZ3FpYW5nQHZpcC4xNjMuY29t; Yanghua Tian, YXlmeXR5aEAxMjYuY29t