- 1Neuroimaging Research Branch, National Institute on Drug Abuse (NIDA) Intramural Research Program, National Institutes of Health, Bethesda, MD, United States
- 2Division of Pharmacology & Toxicology, College of Pharmacy, The University of Texas at Austin, Austin, TX, United States
- 3Waggoner Center for Alcohol & Addiction Research, The University of Texas at Austin, Austin, TX, United States
Resting-state functional connectivity (rsFC) is emerging as a research tool for systems and clinical neuroscience. The mechanism underlying resting-state functional MRI (rsfMRI) signal, however, remains incompletely understood. A widely held assumption is that the spontaneous fluctuations in blood oxygenation level-dependent (BOLD) signal reflect ongoing neuronal processes (herein called “neurocentric” model). In support of this model, evidence from human and animal studies collectively reveals that the spatial synchrony of spontaneously occurring electrophysiological signal recapitulates BOLD rsFC networks. Two recent experiments from independent labs designed to specifically examine neuronal origins of rsFC, however, suggest that spontaneously occurring neuronal events, as assessed by multiunit activity or local field potential (LFP), although statistically significant, explain only a small portion (∼10%) of variance in resting-state BOLD fluctuations. These two studies, although each with its own limitations, suggest that the spontaneous fluctuations in rsfMRI, may have complex cellular origins, and the “neurocentric” model may not apply to all brain regions.
Introduction
Noise exists in all measurements. The significance of spatially coherent “noise” in blood oxygenation level-dependent (BOLD) signal was appreciated in a seminal paper by Biswal et al. (1995), who revealed temporal synchrony in human sensorimotor system. As similar results accumulated for other brain systems (Greicius et al., 2003; Beckmann et al., 2005; Fox et al., 2005; Seeley et al., 2007; Habas et al., 2009; Raichle, 2011), in particular the discovery of the “default mode network (DMN) (Raichle et al., 2001; Greicius et al., 2003),” a concept emerged suggesting that the persistent, correlated spontaneous activity between brain regions [functional connectivity (FC)], initially thought to be noise (i.e., random error) in BOLD measurements, is in fact a meaningful source of information, reflecting a fundamental feature of brain functional organization (Raichle, 2009). Further supporting this view, a recent study defined depression subtypes based on FC patterns; the resulting biotypes predicted individuals’ responsiveness to transcranial magnetic stimulation (TMS) therapy, pointing to clinical potentials of resting-state functional connectivity (rsFC) (Greicius, 2008).
A cornerstone assumption in rsFC is that the ongoing, spontaneously occurring synchrony among brain areas reflects the inherent functional organization of the neural network. The definition of “neural network” in the context of resting-state functional MRI (rsfMRI), however, remains vague. Data accumulated over the past decade, more or less, lend support to the hypothesis that synchronized neuronal activity underlies the BOLD FC. Recent animal studies from two independent labs designed to directly test this hypothesis, however, suggest cautious interpretation of the data (see below). As rsFC becomes increasingly used as a research tool for basic and clinical neuroscience, a thorough understanding of its physiological basis and origin becomes essential and critical.
In the spirit of stimulating scientific debate, in this opinionated mini-review, we will start with methodological considerations that we deem important in elucidating the physiological basis of BOLD fluctuations, followed by critical review of recent evidence that supports neuronal origin of the rsfMRI signal, herein we will call it “neurocentric” model; we will then present several lines of recent evidence that appear divergent from this concept. Finally, we will bring forward testable hypotheses from a perspective of BOLD signal transduction. Extensive review of this subject can be found elsewhere (Leopold and Maier, 2012; Lu and Stein, 2013). We will focus on progress in the past 5 years.
Methodological Considerations
Brain’s electrical signals can be measured at different scales, from intra- and extra-cellular recording, local field potential (LFP) to electroencephalogram and magnetoencephalography (Buzsáki et al., 2012). The LFP signal has been shown to be correlated with BOLD response to a task (e.g., visual stimulation) (Lauritzen, 2001; Logothetis et al., 2001). By extension, LFP is often used to investigate the electrophysiological correlate of the rsfMRI signal (Lu et al., 2007; Mantini et al., 2007; He et al., 2008; Shmuel and Leopold, 2008; de Pasquale et al., 2010; Schölvinck et al., 2010; Pan et al., 2011; Wang et al., 2012; Hutchison et al., 2015; Shi et al., 2019). The underlying assumption is that BOLD fluctuation in the resting-state and the evoked BOLD response to a task manipulation share the same signal transduction mechanism. As will be discussed later in this article, this assumption may require a careful assessment.
On the other hand, BOLD signal results from the mismatch between blood flow and oxygen metabolism (Fox and Raichle, 1986; Kim and Ogawa, 2012), indirectly reflecting neuronal activity. Thus, LFP and BOLD signals are two fundamentally different readouts of brain activity. We have previously argued that (Lu and Stein, 2013), in order for the fMRI signal to be considered as a surrogate of a specific neuronal physiological measure, at a minimum, the following criteria should be met: (i) the temporal fluctuations of the electrical and the BOLD signals should remain correlated, and such correlation should be persistent across brain states; (ii) the spatially correlated patterns from electrical signal should recapitulate that from BOLD signal across brain states; and (iii) each pattern should be unique to each network. To meet the above three criteria, it would appear necessary that both types of signals should be recorded simultaneously on the same subject. Due to substantial technical difficulties and for practical reasons, to the best of our knowledge, most published studies employed experimental designs that partially meet these criteria, and thus should be considered critically.
Spatially Correlated Patterns of the Electrical Signal Recapitulate Bold rsFC
Perhaps the most intuitive evidence to support the “neurocentric” model is the distinct spatial patterns of the electrical signal, which bear remarkable similarity to BOLD FC (Fukushima et al., 2012; Liu et al., 2015; Hacker et al., 2017; Kucyi et al., 2018; Shi et al., 2019). Data from voltage sensitive dye (VSD) fluorescent imaging appear particularly compelling (Mohajerani et al., 2010). Conventional electrophysiological recording necessitates reference and ground electrodes; and there is more or less “volume conduction effect” (Kajikawa and Schroeder, 2011). Confounds from these technical aspects lead to certain degrees of ambiguity in terms of spatial localization of the electrical signal. This could potentially introduce artifactual inter-regional correlation. VSD imaging measures membrane potential changes, avoiding these confounds entirely.
In a mouse model with a large craniotomy preparation, Mohajerani et al. (2010) simultaneously recorded VSD signals in both hemispheres. Strong oscillations exist in spontaneous ongoing VSD signals, which mirror LFP signal in the low frequency band (3–6 Hz). The oscillations in homotopic cortical regions were correlated; discrete peaks characterized each region. Awake and urethane anesthetized mice showed similar inter-hemispheric synchrony. Furthermore, they found that, in genetically acallosal mice, the interhemispheric synchrony was significantly reduced, a finding similar to rsFC studies in humans (Lowe et al., 1997; Quigley et al., 2003; Johnston et al., 2008). The localized synchrony patterns in homotopic cortical regions recorded using VSD are strikingly similar to rsFC reported in rodent fMRI literature (Lu et al., 2007, 2012; Pawela et al., 2008; Zhao et al., 2008; Hutchison et al., 2010; Liang et al., 2011; Magnuson et al., 2014; Gozzi and Schwarz, 2016), including sensory networks in the forelimb region, whisker cortex, motor cortex etc. Notably, the retrosplenial cortex, a major component of the DMN (Lu et al., 2012), was also depicted in the VSD data.
Spatially correlated patterns between electrophysiological signals recorded in areas of classic FC networks were also observed in humans (He et al., 2008; Hacker et al., 2017; Kucyi et al., 2018). In these studies, patients underwent neurosurgical electrocorticography (ECoG) electrode implantation. Based on clinical needs, each study had a unique cohort of patients with electrodes covering specific brain regions. Taken together, the electrodes covered sensory motor network (SMN), dorsal attention network (DAN), DMN, and frontoparietal control system (FPC). In general, these studies found a higher within network correlation than between network correlation, and there is a spatial correspondence between ECoG and BOLD FC patterns. Less consistent was which frequency band contributes the most to the within and between network correlations. For example, Hacker et al. (2017) showed that the correspondence appeared to be frequency band-specific: theta (4–8 Hz) band-limited power (BLP) correspondence appeared stronger in the DMN and FPC, while theta (8–12 Hz) BLP correspondence was stronger in the SMN and DAN. They also found that gamma BLP correspondence was commonly observed throughout the brain. Kucyi et al. (2018) reported that correlation patterns in high frequency broadband (70–170 Hz) power were consistent during wakeful rest and sleep; although similar correlation pattern exist in lower-frequency (1–70 Hz) power, but the spatial specificity and temporal consistency were inferior to higher frequency broadband power.
A recent study by Shi et al. (2019) recorded ECoG signal from the primary somatosensory cortex (areas 3b and 1) of anesthetized monkeys, and found that spontaneous fluctuations in low frequency LFP signal was the major contributor to resting-state LFP coherence. Furthermore, they reported that the temporal dynamics in BOLD FC behaved most similarly to the low frequency LFP coherence. These results are generally in line with the conclusions from studies in anesthetized rats (Lu et al., 2007, 2014; Pan et al., 2010; Magnuson et al., 2014). It is not clear whether the use of anesthesia in rats and monkeys played a role in the discrepancy mentioned above.
In summary, evidence from animals and humans collectively suggests a high degree of correspondence in spatial correlation patterns derived from electrophysiological recording and BOLD rsFC, it would appear tempting to conclude that the electrophysiological signal underlies BOLD rsFC. However, making this leap requires correspondence in temporal behavior of these two types of brain readouts, which necessitates simultaneous measurement of both types of signals. Unfortunately, two lines of evidence from simultaneously recorded electrophysiological and hemodynamic signals seem difficult to reconcile with the “neurocentric” model.
Lfp–Bold Correlation is Weak
We developed a simultaneous fMRI–electrophysiological recording technique (Jaime et al., 2018), and performed chronic repetitive recordings in rat striatum. The electrophysiological recording and BOLD data acquisition were coupled with pharmacological modulation of the well-defined dopaminergic pathway (Figure 1A). We found three distinct BOLD rsFC networks using the independent component analysis. These three networks are consistent with well-known three functional domains in rat striatum (Voorn et al., 2004). We thus implanted silicon-based microelectrode array (16 contacts) that covered the dorsolateral to ventral medial striatum (Figures 1B,C). With microinjection of alpha-amino-3-hydroxy-5-methyl-4-isoxazole propionic acid (AMPA) receptor agonist into the ventral tegmental area (VTA), we systematically modulated dopamine release and neuronal activity in the striatum, to which VTA dopamine neurons project most densely. As shown in Figure 1D, both the amplitude and the frequency of the striatal LFP signal were modulated. VTA AMPA microinjection significantly modulated FC only in the ventral striatum (nucleus accumbens) (Figures 1E,F), consistent with known neuroanatomy.
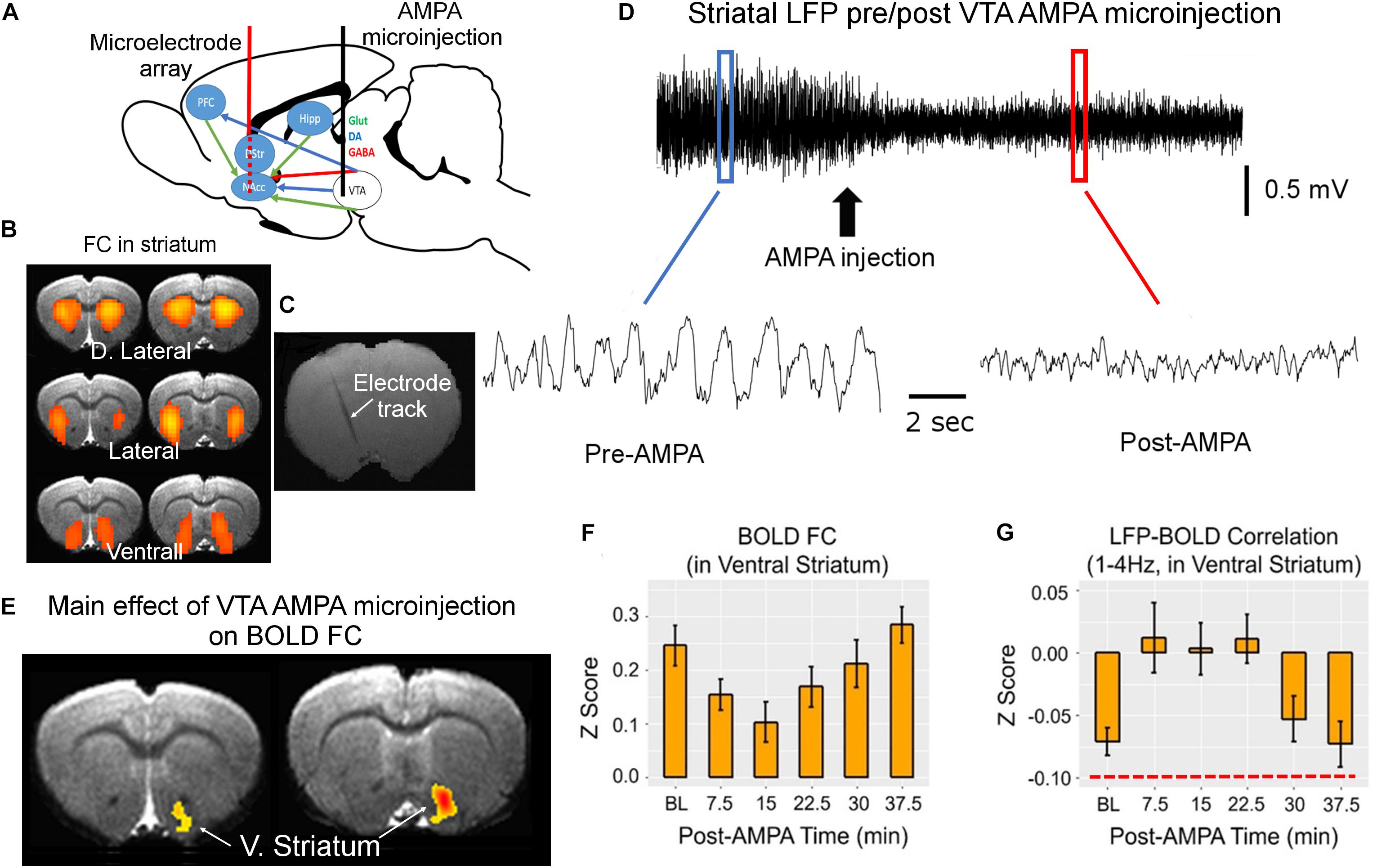
Figure 1. MRI-compatible linear electrode array was implanted into the rat striatum covering the dorsolateral and ventrolateral domains (A–C). These domains were identified based on the three bilateral rsFC network in rat striatum that correspond to the three functional domains of the rat striatum (B). Alpha-amino-3-hydroxy-5-methyl-4-isoxazole propionic acid (AMPA) was injected into the ventral tegmental area (VTA) via a guide cannula to enhance the activity of VTA dopaminergic neurons, which project primarily to the ventral striatum, modulating striatal local field potential (LFP) signal (D). The waveforms before and after AMPA injection were derived from the blue and red boxes, respectively. (E,F) The main effect of AMPA on BOLD functional connectivity (FC). (G) The effects of VTA AMPA microinjections LFP–BOLD correlation (corr.). Note the low correlation value in LFP–BOLD correlation, indicated by red dashed line in panel (G). All maps are thresholded at p < 0.05 after correction for multiple comparisons. DA, dopamine; D. Striatum, dorsal striatum; V. Striatum, ventral striatum (nucleus accumbens); GABA, gamma-aminobutyric acid; Glut, glutamate; Hipp, hippocampus; NAcc, nucleus accumbens core; and PFC, prefrontal cortex (adapted with permission from the authors).
Concurrent LFP and BOLD signal recording allowed us to directly interrogate the temporal relationship of these two types of brain readouts. Perhaps the most unexpected finding is the low correlation between LFP and BOLD signal: although the LFP-BOLD time courses were statistically correlated, the average LFP-BOLD correlation was below 0.1 (Figure 1G). The correlation between gamma LFP and the fMRI signal was similar but opposite in sign (Jaime et al., 2017). These data suggest that spontaneous LFP fluctuations explain only a small portion of variance in BOLD fluctuations (Sumiyoshi et al., 2019).
The above observation by Jaime et al. (2017) is corroborated by a recent optical imaging study (Winder et al., 2017). In this study, Winder et al. (2017) applied intrinsic optical imaging to measure hemodynamic signal [total hemoglobin signal, reflecting cerebral blood volume (CBV)] while at the same time recording neuronal activity in the whisker barrel cortex of the awake, head-fixed mice. What made this study especially unique in the context of spontaneous brain activity was that they carefully monitored whisker and body movements. They found that spontaneous CBV changes in the absence of experimenter-delivered sensory input were largely driven by volitional whisker and body movements. During periods of “rest” when there was no experimenter-delivered sensory stimulation, volitional whisker and body movements, CBV signal was only weakly correlated with neural activity assessed with either gamma band LFP or multiunit activity (with an R2 of about 0.1, Figure 2). They performed pharmacological manipulations to block local neural spiking, glutamatergic input and noradrenergic receptors, and found that spontaneous fluctuations in CBV and vessel diameter persisted, indicating that spontaneous CBV fluctuations may have a non-neuronal origin.
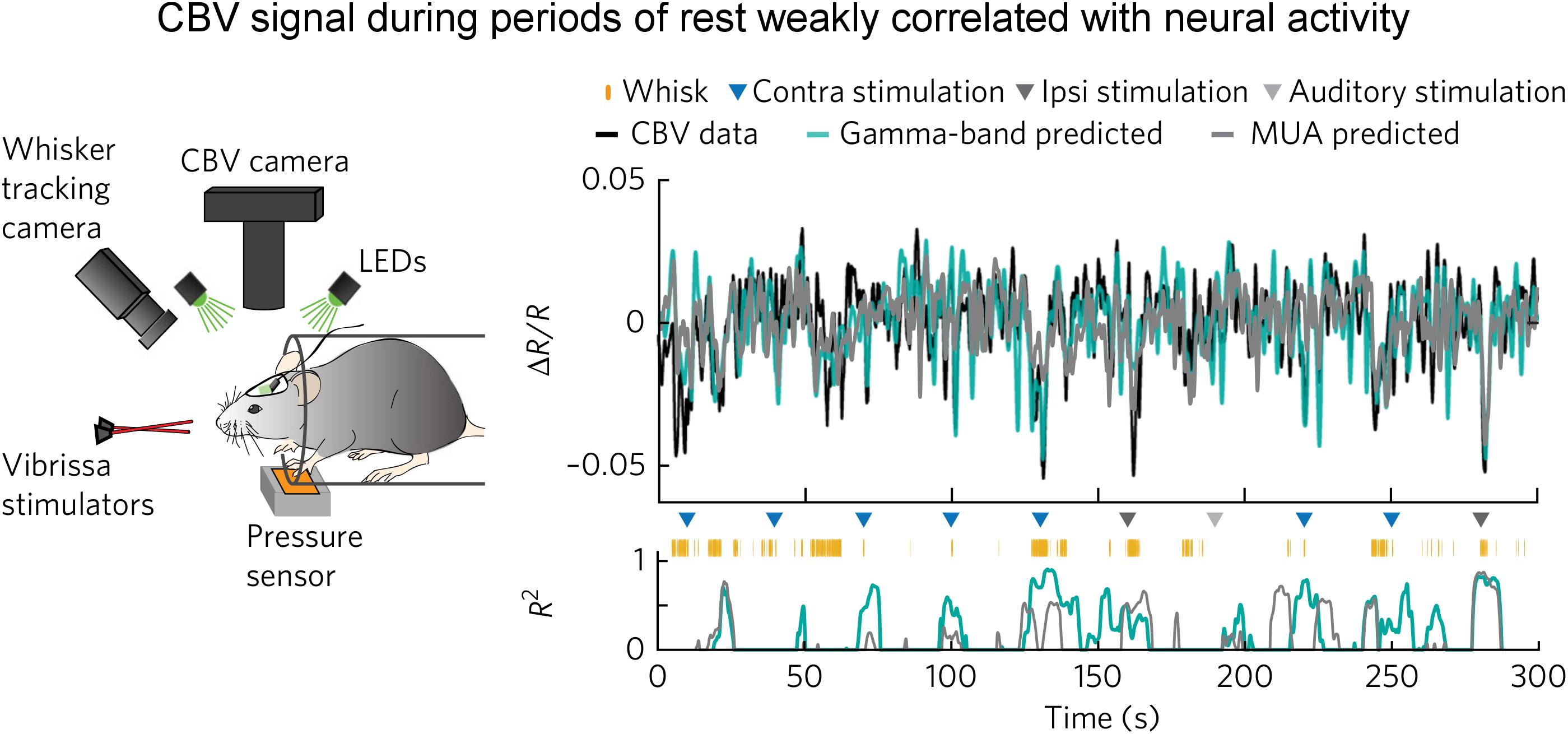
Figure 2. Weak correlation between electrophysiological and hemodynamic signal during periods of “rest.” (Left) Schematic of the experimental setup for optical imaging of intrinsic signal (the isosbestic point green light measures total hemoglobin signal, reflecting CBV). Animal’s behavior and movement were dynamically monitored. (Right) Predictions of ongoing CBV for a single trial. (Top) Predictions by gamma-band (teal blue, R2 = 0.29) and MUA-derived (gray, R2 = 0.20) HRFs. (Bottom) Goodness-of-fit (R2) for 8-s sliding windows. Colored triangles indicate sensory stimuli. Orange tick marks indicate volitional whisking events. Note that R2 was low during the “rest.” Here, the “rest” was defined as the periods absent of experimenter-delivered stimulation, fidgeting, and volitional whisking events (adapted with permission from the authors).
The results reported by Winder et al. (2017) are divergent from several recent optical studies (Matsui et al., 2011; Ma et al., 2016; Mateo et al., 2017). For example, Ma et al. (2016) reported that spontaneous CBV fluctuations highly correlated with ongoing neuronal activity as measured with GCaMP optical imaging. The reasons for this discrepancy are unknown, but one might speculate that the differential definition of “resting-state” may have played a role, an issue particularly relevant in studies employing awake animals. Ma et al. (2016) stated in their paper: “Awake mice were imaged head-fixed but positioned on a saucer wheel and were free to run during imaging. The motion of the wheel was monitored throughout imaging using a webcam synchronized with image acquisition. All periods of running were removed, and ‘resting-state’ epochs were defined as periods of at least 30 s of continuous rest.” Over a duration of 30 s or more, a head-fixed awake mouse likely whisks volitionally (Winder et al., 2017). Ma et al. (2016) did not specifically state whether they had taken whisking behavior or fidgeting into account in their definition of the “resting-state.”
Whisking is an important, albeit subtle, behavior in rodents. As pointed out by Sofroniew and Svoboda (2015): “rodents use their mechanosensitive whiskers for a diverse range of tactile behaviors such as navigation, object recognition, and social interactions. These animals move their whiskers in a purposive manner to locations of interest.” Furthermore, whisking triggers cortical dynamics in many brain regions including S1, S2, M1, M2, PPC, thalamus, etc. (O’Connor et al., 2010; Sofroniew et al., 2015; Helmchen et al., 2018). Thus, the individual moments when a mouse volitionally whisks and fidgets should be treated as individual task events; both GCaMP and CBV recordings during these periods should be excluded for analyzing neuronal correlates of resting-state hemodynamic signal. Winder et al. (2017) showed that auditory, whisker stimulation and some volitional whisking events induced distinct hemodynamic responses – an observation appears compelling. Furthermore, Winder et al. (2017) showed that the high correlation between electrophysiological signal (MUA and LFP) was largely driven by these events, a point that warrants further exploration (see section “Technical Limitations”). Additionally, certain transgenic mouse lines are known to have aberrant firing patterns (Steinmetz et al., 2017); it is unknown whether the mouse line used by Ma et al. (2016) had normal basal cortical activity.
The neurophysiological basis of rsFC has been under debate for more than a decade. The debate has been framed in the context of “neurocentric” model. In support of this view, in the spatial domain, converging evidence from animals and human suggests that the interregional correlation patterns derived from electrophysiological recording recapitulate BOLD rsFC (Hacker et al., 2017; Kucyi et al., 2018).
Experiments designed to specifically test the “neurocentric” model only appeared in recent years (Matsui et al., 2011; Ma et al., 2016; Jaime et al., 2017; Mateo et al., 2017; Winder et al., 2017). The two independent studies (Jaime et al., 2017; Winder et al., 2017) employed concurrent electrophysiological recording and hemodynamic measurement, and found weak LFP–BOLD correlation. In particular, the study by Winder et al. (2017) suggests that hemodynamic signal may reflect a combination of potential sources, including behavior, local neural activity, and putatively non-neural processes, calling for careful definition of the “resting-state.” This may be especially relevant in studies using animal models.
Technical Limitations
The weak correlation between ongoing neuronal activity and hemodynamic signals during the resting-state is somewhat unexpected, and is not in line with the “neurocentric” doctrine of rsfMRI. Several technical issues must be critically reviewed when interpreting this finding. Jaime et al. (2017) performed LFP and rsfMRI recordings in the striatum of anesthetized rats. The anesthetic regime, a combination of low dose isoflurane and dexmedetomdine, has been shown to preserve brain networks (Lu et al., 2012; Brynildsen et al., 2016). But LFP signals recorded with this regime are dominated by low frequency (delta) activity. It is unknown how the use of anesthetics affects neurovascular coupling, particularly in the resting-state. Additionally, the longitudinally implanted microelectrode array unavoidably degrades the quality of rsfMRI signal in voxels around the electrodes. Cytoarchitectonically, inhibitory medium spiny neurons represent 95% of neuronal cells in rat striatum, as opposed to in the neocortex where a majority of neurons are excitatory glutamatergic neurons (Kreitzer and Malenka, 2008). Several studies reported differential neurovascular coupling in the striatum than in the neocortex (Shih et al., 2009, 2012). Specifically, Shih et al. documented bilateral CBV decreases associated with enhanced neuronal activity in the caudate–putamen induced by unilateral noxious electrical stimulation, and that the activation of dopamine D2 receptor played a role in this process (Chen et al., 2005). It is thus possible that finding in the striatum may not be generalizable to other brain regions.
The study by Winder et al. (2017) was performed in the neocortex of awake mice, and thus avoided the confounding effects from anesthesia. Nevertheless, they inserted Teflon-coated tungsten-wire stereotrode into the barrel cortex, which necessitated an invasive cranial window preparation, and in some experiments, a cannula was chronically implanted for pharmacological manipulations. Since an acute cranial window preparation likely causes certain degree of tissue insult, it is unknown whether the tissue insult arising from this procedure might have affected neurovascular coupling in the resting-state, although they were able to record robust hemodynamic response to whisker stimulation. In support of this argument, Hudetz et al. (1998) pointed out that spontaneous fluctuation in cerebral blood flow requires the preservation of the flow control system. The fluctuations are absent in focally ischemic cortical territories when the ischemia is severe.
Potential Roles of Astrocyte in rsfMRI Signal
The potential roles of astrocytes in rsfMRI have been largely ignored. Neurons and associated astrocytes are organized in large-scale synaptic and astrocytic networks. Complex signaling within and between these networks causes fluctuations in cerebral metabolic rate of oxygen (CMRO2) and hemodynamic response (Attwell and Iadecola, 2002). The BOLD signal is vascular in origin, and ultimately relies on vasoactive substance release. Neuronal activity is known to cause the release of many vasoactive agents, including H+, K+, adenosine, arachidonic acid metabolites, nitric oxide (NO) etc. (Iadecola and Nedergaard, 2007). On the other hand, astrocytes are strategically positioned between neuronal and vascular systems: astrocytes forms end-feet on capillaries and arterioles while having contacts with synapses (Mishra, 2017). Importantly, astrocytes are known to express many ion channels, receptors, transporters, and vesicles (Verkhratsky and Nedergaard, 2018). Indeed, astrocytic Ca2+ signaling has been shown to play an role in regulating neurovascular coupling (Zonta et al., 2003) and in controlling vessel tone (Rosenegger et al., 2015). Furthermore, several lines of evidence suggest that alterations in parenchymal vascular tone influence astrocytic Ca2+ activity and potentially neuronal activity as well, such that the “hemo-neural” hypothesis has been proposed (Moore and Cao, 2008; Kim et al., 2016). Modeling studies suggest that cyclic neuron-astrocyte cross talk could produce slow oscillations in BOLD signal (DiNuzzo et al., 2011; DiNuzzo, 2015). Thus, in addition to the “neurocentral” model, an alternative testable hypothesis could be that the spontaneous BOLD fluctuation is at least partially caused by spontaneous astrocyte activity. Additionally, the role of spontaneous vasomotion in spontaneous BOLD fluctuation may warrant further investigation.
Imaging of astrocytic activity has traditionally relied on fluorescent dyes (Mulligan and MacVicar, 2004; Scemes and Giaume, 2006; Schulz et al., 2012; Otsu et al., 2015). The development of transgenic mouse lines, viral vector targeting strategies and genetically encode Ca2+ indicators has made astrocyte-specific recording and manipulation more readily available (Biesecker et al., 2016; Poskanzer and Yuste, 2016; Takata et al., 2018). For example, using transgenic approaches, Takata et al. (2018) generated mice expressing channelrhodopsin specifically in neurons or in astrocytes, and measured BOLD response to astrocyte-specific optogenetic stimulation. They reported that optogenetic activation of astrocytes, in the absence of apparent neuronal modulation, evoked BOLD response. It is thus conceivable that spontaneous activity in astrocyte may cause spontaneous vasodilation and vasoconstriction, leading to variations in BOLD signal that are decoupled from spontaneous neural activity. Future experiments that combine optical readouts of neuronal and astrocytic calcium activities with hemodynamic measurement may shed light on the origins of rsfMRI signal.
Author Contributions
All authors listed have made a substantial, direct and intellectual contribution to the work, and approved it for publication.
Funding
This work was supported by the NIDA Intramural Research Program, NIH.
Conflict of Interest
The authors declare that the research was conducted in the absence of any commercial or financial relationships that could be construed as a potential conflict of interest.
References
Attwell, D., and Iadecola, C. (2002). The neural basis of functional brain imaging signals. Trends Neurosci. 25, 621–625. doi: 10.1016/s0166-2236(02)02264-6
Beckmann, C., DeLuca, M., Devlin, J., and Smith, S. (2005). Investigations into resting-state connectivity using independent component analysis. Philos. Trans. R. Soc. Lond. B Biol. Sci. 360, 1001–1013. doi: 10.1098/rstb.2005.1634
Biesecker, K. R., Srienc, A. I., Shimoda, A. M., Agarwal, A., Bergles, D. E., Kofuji, P., et al. (2016). Glial cell calcium signaling mediates capillary regulation of blood flow in the retina. J. Neurosci. 36, 9435–9445. doi: 10.1523/JNEUROSCI.1782-16.2016
Biswal, B., Yetkin, F. Z., Haughton, V. M., and Hyde, J. S. (1995). Functional connectivity in the motor cortex of resting human brain using echo-planar MRI. Magn. Reson. Med. 34, 537–541. doi: 10.1002/mrm.1910340409
Brynildsen, J. K., Hsu, L. M., Ross, T. J., Stein, E. A., Yang, Y., and Lu, H. (2016). Physiological characterization of a robust survival rodent fMRI method. Magn. Reson. Imaging 35, 54–60. doi: 10.1016/j.mri.2016.08.010
Buzsáki, G. F., Anastassiou, C. A., and Koch, C. (2012). The origin of extracellular fields and currents–EEG, ECoG, LFP and spikes. Nat. Rev. Neurosci. 13, 407–420. doi: 10.1038/nrn3241
Chen, Y. C., Choi, J. K., Andersen, S. L., Rosen, B. R., and Jenkins, B. G. (2005). Mapping dopamine D2/D3 receptor function using pharmacological magnetic resonance imaging. Psychopharmacology 180, 705–715. doi: 10.1007/s00213-004-2034-0
de Pasquale, F., Della Penna, S., Snyder, A. Z., Lewis, C., Mantini, D., Marzetti, L., et al. (2010). Temporal dynamics of spontaneous MEG activity in brain networks. Proc. Natl. Acad. Sci. U.S.A. 107, 6040–6045. doi: 10.1073/pnas.0913863107
DiNuzzo, M. (2015). Isn’t functional neuroimaging all about Ca2+ signaling in astrocytes? J. Neurophysiol. 114, 1353–1356. doi: 10.1152/jn.00826.2014
DiNuzzo, M., Gili, T., Maraviglia, B., and Giove, F. (2011). Modeling the contribution of neuron-astrocyte cross talk to slow blood oxygenation level-dependent signal oscillations. J. Neurophysiol. 106, 3010–3018. doi: 10.1152/jn.00416.2011
Fox, M., Snyder, A., Vincent, J., Corbetta, M., Van Essen, D., and Raichle, M. (2005). The human brain is intrinsically organized into dynamic, anticorrelated functional networks. Proc. Natl. Acad. Sci. U.S.A. 102, 9673–9678. doi: 10.1073/pnas.0504136102
Fox, P. T., and Raichle, M. E. (1986). Focal physiological uncoupling of cerebral blood flow and oxidative metabolism during somatosensory stimulation in human subjects. Proc. Natl. Acad. Sci. U.S.A. 83, 1140–1144. doi: 10.1073/pnas.83.4.1140
Fukushima, M., Saunders, R. C., Leopold, D. A., Mishkin, M., and Averbeck, B. B. (2012). Spontaneous high-gamma band activity reflects functional organization of auditory cortex in the awake macaque. Neuron 74, 899–910. doi: 10.1016/j.neuron.2012.04.014
Gozzi, A., and Schwarz, A. J. (2016). Large-scale functional connectivity networks in the rodent brain. Neuroimage 127, 496–509. doi: 10.1016/j.neuroimage.2015.12.017
Greicius, M. (2008). Resting-state functional connectivity in neuropsychiatric disorders. Curr. Opin. Neurol 21, 424–430. doi: 10.1097/WCO.0b013e328306f2c5
Greicius, M., Krasnow, B., Reiss, A., and Menon, V. (2003). Functional connectivity in the resting brain: a network analysis of the default mode hypothesis. Proc. Natl. Acad. Sci. U.S.A. 100, 253–258. doi: 10.1073/pnas.0135058100
Habas, C., Kamdar, N., Nguyen, D., Prater, K., Beckmann, C. F., Menon, V., et al. (2009). Distinct cerebellar contributions to intrinsic connectivity networks. J. Neurosci. 29, 8586–8594. doi: 10.1523/jneurosci.1868-09.2009
Hacker, C. D., Snyder, A. Z., Pahwa, M., Corbetta, M., and Leuthardt, E. C. (2017). Frequency-specific electrophysiologic correlates of resting state fMRI networks. Neuroimage 149, 446–457. doi: 10.1016/j.neuroimage.2017.01.054
He, B. J., Snyder, A. Z., Zempel, J. M., Smyth, M. D., and Raichle, M. E. (2008). Electrophysiological correlates of the brain’s intrinsic large-scale functional architecture. Proc. Natl. Acad. Sci. U.S.A. 105, 16039–16044. doi: 10.1073/pnas.0807010105
Helmchen, F., Gilad, A., and Chen, J. L. (2018). Neocortical dynamics during whisker-based sensory discrimination in head-restrained mice. Neuroscience 368, 57–69. doi: 10.1016/j.neuroscience.2017.09.003
Hudetz, A. G., Biswal, B. B., Shen, H., Lauer, K. K., and Kampine, J. P. (1998). Spontaneous fluctuations in cerebral oxygen supply. An introduction. Adv. Exp. Med. Biol. 454, 551–559. doi: 10.1007/978-1-4615-4863-8_66
Hutchison, R. M., Hashemi, N., Gati, J. S., Menon, R. S., and Everling, S. (2015). Electrophysiological signatures of spontaneous BOLD fluctuations in macaque prefrontal cortex. Neuroimage 113, 257–267. doi: 10.1016/j.neuroimage.2015.03.062
Hutchison, R. M., Mirsattari, S. M., Jones, C. K., Gati, J. S., and Leung, L. S. (2010). Functional networks in the anesthetized rat brain revealed by independent component analysis of resting-state FMRI. J. Neurophysiol. 103, 3398–3406. doi: 10.1152/jn.00141.2010
Iadecola, C., and Nedergaard, M. (2007). Glial regulation of the cerebral microvasculature. Nat. Neurosci. 10, 1369–1376. doi: 10.1038/nn2003
Jaime, S., Cavazos, J. E., Yang, Y., and Lu, H. (2018). Longitudinal observations using simultaneous fMRI, multiple channel electrophysiology recording, and chemical microiontophoresis in the rat brain. J. Neurosci. Methods 306, 68–76. doi: 10.1016/j.jneumeth.2018.05.010
Jaime, S., Gu, H., Sadacca, B. F., Stein, E. A., Cavazos, J. E., Yang, Y., et al. (2017). Delta rhythm orchestrates the neural activity underlying the resting state BOLD signal via phase-amplitude coupling. Cereb. Cortex 29, 119–133. doi: 10.1093/cercor/bhx310
Johnston, J. M., Vaishnavi, S. N., Smyth, M. D., Zhang, D., He, B. J., Zempel, J. M., et al. (2008). Loss of resting interhemispheric functional connectivity after complete section of the corpus callosum. J. Neurosci. 28, 6453–6458. doi: 10.1523/JNEUROSCI.0573-08.2008
Kajikawa, Y., and Schroeder, C. E. (2011). How local is the local field potential? Neuron 72, 847–858. doi: 10.1016/j.neuron.2011.09.029
Kim, K. J., Ramiro Diaz, J. J., Iddings, A., and Filosa, J. A. (2016). Vasculo-neuronal coupling: retrograde vascular communication to brain neurons. J. Neurosci. 36, 12624–12639. doi: 10.1523/jneurosci.1300-16.2016
Kim, S. G., and Ogawa, S. (2012). Biophysical and physiological origins of blood oxygenation level-dependent fMRI signals. J. Cereb. Blood Flow Metab. 32, 1188–1206. doi: 10.1038/jcbfm.2012.23
Kreitzer, A. C., and Malenka, R. C. (2008). Striatal plasticity and basal ganglia circuit function. Neuron 60, 543–554. doi: 10.1016/j.neuron.2008.11.005
Kucyi, A., Schrouff, J., Bickel, S., Foster, B. L., Shine, J. M., and Parvizi, J. (2018). Intracranial electrophysiology reveals reproducible intrinsic functional connectivity within human brain networks. J. Neurosci. 38, 4230–4242. doi: 10.1523/JNEUROSCI.0217-18.2018
Lauritzen, M. (2001). Relationship of spikes, synaptic activity, and local changes of cerebral blood flow. J. Cereb. Blood Flow Metab. 21, 1367–1383. doi: 10.1097/00004647-200112000-00001
Leopold, D. A., and Maier, A. (2012). Ongoing physiological processes in the cerebral cortex. Neuroimage 62, 2190–2200. doi: 10.1016/j.neuroimage.2011.10.059
Liang, Z., King, J., and Zhang, N. (2011). Uncovering intrinsic connectional architecture of functional networks in awake rat brain. J. Neurosci. 31, 3776–3783. doi: 10.1523/JNEUROSCI.4557-10.2011
Liu, X., Yanagawa, T., Leopold, D. A., Fujii, N., and Duyn, J. H. (2015). Robust long-range coordination of spontaneous neural activity in waking, sleep and anesthesia. Cereb. Cortex 25, 2929–2938. doi: 10.1093/cercor/bhu089
Logothetis, N. K., Pauls, J., Augath, M., Trinath, T., and Oeltermann, A. (2001). Neurophysiological investigation of the basis of the fMRI signal. Nature 412, 150–157. doi: 10.1038/35084005
Lowe, M., Rutecki, P., Woodard, A., Turski, P., and Sorenson, J. (1997). Auditory cortex fMRI noise correlations in callosal agenesis. Hum. Brain Mapp. 5, S194.
Lu, H., and Stein, E. A. (2013). Resting state functional connectivity: its physiological basis and application in neuropharmacology. Neuropharmacology 84, 79–89. doi: 10.1016/j.neuropharm.2013.08.023
Lu, H., Wang, L., Rea, W. W., Brynildsen, J. K., Jaime, S., Zuo, Y., et al. (2014). Low- but not high-frequency LFP correlates with spontaneous BOLD fluctuations in rat whisker barrel cortex. Cereb. Cortex 26, 683–694. doi: 10.1093/cercor/bhu248
Lu, H., Zou, Q., Gu, H., Raichle, M. E., Stein, E. A., and Yang, Y. (2012). Rat brains also have a default mode network. Proc. Natl. Acad. Sci. U.S.A. 109, 3979–3984. doi: 10.1073/pnas.1200506109
Lu, H., Zuo, Y., Gu, H., Waltz, J. A., Zhan, W., Scholl, C. A., et al. (2007). Synchronized delta oscillations correlate with the resting-state functional MRI signal. Proc. Natl. Acad. Sci. U.S.A. 104, 18265–18269. doi: 10.1073/pnas.0705791104
Ma, Y., Shaik, M. A., Kozberg, M. G., Kim, S. H., Portes, J. P., Timerman, D., et al. (2016). Resting-state hemodynamics are spatiotemporally coupled to synchronized and symmetric neural activity in excitatory neurons. Proc. Natl. Acad. Sci. U.S.A. 113, E8463–E8471. doi: 10.1073/pnas.1525369113
Magnuson, M. E., Thompson, G. J., Pan, W. J., and Keilholz, S. D. (2014). Effects of severing the corpus callosum on electrical and BOLD functional connectivity and spontaneous dynamic activity in the rat brain. Brain Connect. 4, 15–29. doi: 10.1089/brain.2013.0167
Mantini, D., Perrucci, M. G., Del Gratta, C., Romani, G. L., and Corbetta, M. (2007). Electrophysiological signatures of resting state networks in the human brain. Proc. Natl. Acad. Sci. U.S.A. 104, 13170–13175. doi: 10.1073/pnas.0700668104
Mateo, C., Knutsen, P. M., Tsai, P. S., Shih, A. Y., and Kleinfeld, D. (2017). Entrainment of arteriole vasomotor fluctuations by neural activity is a basis of blood-oxygenation-level-dependent “resting-state” connectivity. Neuron 96, 936.e3–948.e3. doi: 10.1016/j.neuron.2017.10.012
Matsui, T., Tamura, K., Koyano, K. W., Takeuchi, D., Adachi, Y., Osada, T., et al. (2011). Direct comparison of spontaneous functional connectivity and effective connectivity measured by intracortical microstimulation: an fMRI study in macaque monkeys. Cereb. Cortex 21, 2348–2356. doi: 10.1093/cercor/bhr019
Mishra, A. (2017). Binaural blood flow control by astrocytes: listening to synapses and the vasculature. J. Physiol. 595, 1885–1902. doi: 10.1113/JP270979
Mohajerani, M. H., McVea, D. A., Fingas, M., and Murphy, T. H. (2010). Mirrored bilateral slow-wave cortical activity within local circuits revealed by fast bihemispheric voltage-sensitive dye imaging in anesthetized and awake mice. J. Neurosci. 30, 3745–3751. doi: 10.1523/JNEUROSCI.6437-09.2010
Moore, C. I., and Cao, R. (2008). The hemo-neural hypothesis: on the role of blood flow in information processing. J. Neurophysiol. 99, 2035–2047. doi: 10.1152/jn.01366.2006
Mulligan, S. J., and MacVicar, B. A. (2004). Calcium transients in astrocyte endfeet cause cerebrovascular constrictions. Nature 431, 195–199. doi: 10.1038/nature02827
O’Connor, D. H., Clack, N. G., Huber, D., Komiyama, T., Myers, E. W., and Svoboda, K. (2010). Vibrissa-based object localization in head-fixed mice. J. Neurosci. 30, 1947–1967. doi: 10.1523/JNEUROSCI.3762-09.2010
Otsu, Y., Couchman, K., Lyons, D. G., Collot, M., Agarwal, A., Mallet, J. M., et al. (2015). Calcium dynamics in astrocyte processes during neurovascular coupling. Nat. Neurosci. 18, 210–218. doi: 10.1038/nn.3906
Pan, W., Thompson, G., Magnuson, M., Majeed, W., Jaeger, D., and Keilholz, S. (2010). Simultaneous FMRI and electrophysiology in the rodent brain. J. Vis. Exp. 42:1901.
Pan, W. J., Thompson, G., Magnuson, M., Majeed, W., Jaeger, D., and Keilholz, S. (2011). Broadband local field potentials correlate with spontaneous fluctuations in functional magnetic resonance imaging signals in the rat somatosensory cortex under isoflurane anesthesia. Brain Connect. 1, 119–131. doi: 10.1089/brain.2011.0014
Pawela, C. P., Biswal, B. B., Cho, Y. R., Kao, D. S., Li, R., Jones, S. R., et al. (2008). Resting-state functional connectivity of the rat brain. Magn. Reson. Med. 59, 1021–1029. doi: 10.1002/mrm.21524
Poskanzer, K. E., and Yuste, R. (2016). Astrocytes regulate cortical state switching in vivo. Proc. Natl. Acad. Sci. U.S.A. 113, E2675–E2684. doi: 10.1073/pnas.1520759113
Quigley, M., Cordes, D., Turski, P., Moritz, C., Haughton, V., Seth, R., et al. (2003). Role of the corpus callosum in functional connectivity. Am. J. Neuroradiol. 24, 208–212.
Raichle, M., MacLeod, A., Snyder, A., Powers, W., Gusnard, D., and Shulman, G. (2001). A default mode of brain function. Proc. Natl. Acad. Sci. U.S.A. 98, 676–682.
Raichle, M. E. (2009). A paradigm shift in functional brain imaging. J. Neurosci. 29, 12729–12734. doi: 10.1523/jneurosci.4366-09.2009
Rosenegger, D. G., Tran, C. H., Wamsteeker Cusulin, I. J., and Gordon, G. R. (2015). Tonic local brain blood flow control by astrocytes independent of phasic neurovascular coupling. J. Neurosci. 35, 13463–13474. doi: 10.1523/JNEUROSCI.1780-15.2015
Scemes, E., and Giaume, C. (2006). Astrocyte calcium waves: what they are and what they do. Glia 54, 716–725. doi: 10.1002/glia.20374
Schölvinck, M. L., Maier, A., Ye, F. Q., Duyn, J. H., and Leopold, D. A. (2010). Neural basis of global resting-state fMRI activity. Proc. Natl. Acad. Sci. U.S.A. 107, 10238–10243. doi: 10.1073/pnas.0913110107
Schulz, K., Sydekum, E., Krueppel, R., Engelbrecht, C. J., Schlegel, F., Schröter, A., et al. (2012). Simultaneous BOLD fMRI and fiber-optic calcium recording in rat neocortex. Nat. Methods 9, 597–602. doi: 10.1038/nmeth.2013
Seeley, W. W., Menon, V., Schatzberg, A. F., Keller, J., Glover, G. H., Kenna, H., et al. (2007). Dissociable intrinsic connectivity networks for salience processing and executive control. J. Neurosci. 27, 2349–2356. doi: 10.1523/jneurosci.5587-06.2007
Shi, Z., Wilkes, D. M., Yang, P. F., Wang, F., Wu, R., Wu, T. L., et al. (2019). On the relationship between MRI and local field potential measurements of spatial and temporal variations in functional connectivity. Sci. Rep. 9:8871. doi: 10.1038/s41598-019-45404-8
Shih, Y. Y., Chen, C. C., Shyu, B. C., Lin, Z. J., Chiang, Y. C., Jaw, F. S., et al. (2009). A new scenario for negative functional magnetic resonance imaging signals: endogenous neurotransmission. J. Neurosci. 29, 3036–3044. doi: 10.1523/JNEUROSCI.3447-08.2009
Shih, Y. Y., Chiang, Y. C., Shyu, B. C., Jaw, F. S., Duong, T. Q., and Chang, C. (2012). Endogenous opioid-dopamine neurotransmission underlie negative CBV fMRI signals. Exp. Neurol. 234, 382–388. doi: 10.1016/j.expneurol.2011.12.042
Shmuel, A., and Leopold, D. A. (2008). Neuronal correlates of spontaneous fluctuations in fMRI signals in monkey visual cortex: implications for functional connectivity at rest. Hum. Brain Mapp. 29, 751–761. doi: 10.1002/hbm.20580
Sofroniew, N. J., and Svoboda, K. (2015). Whisking. Curr. Biol. 25, R137–R140. doi: 10.1016/j.cub.2015.01.008
Sofroniew, N. J., Vlasov, Y. A., Hires, S. A., Freeman, J., and Svoboda, K. (2015). Neural coding in barrel cortex during whisker-guided locomotion. eLife 4:e12559. doi: 10.7554/eLife.12559
Steinmetz, N. A., Buetfering, C., Lecoq, J., Lee, C. R., Peters, A. J., Jacobs, E. A. K., et al. (2017). Aberrant cortical activity in multiple gcamp6-expressing transgenic mouse lines. eNeuro 4:ENEURO.0207-17.2017. doi: 10.1523/ENEURO.0207-17.2017
Sumiyoshi, A., Keeley, R. J., and Lu, H. (2019). Physiological considerations of functional magnetic resonance imaging in animal models. Biol. Psychiatry Cogn. Neurosci. Neuroimaging 4, 522–532. doi: 10.1016/j.bpsc.2018.08.002
Takata, N., Sugiura, Y., Yoshida, K., Koizumi, M., Hiroshi, N., Honda, K., et al. (2018). Optogenetic astrocyte activation evokes BOLD fMRI response with oxygen consumption without neuronal activity modulation. Glia 66, 2013–2023. doi: 10.1002/glia.23454
Verkhratsky, A., and Nedergaard, M. (2018). Physiology of astroglia. Physiol. Rev. 98, 239–389. doi: 10.1152/physrev.00042.2016
Voorn, P., Vanderschuren, L. J., Groenewegen, H. J., Robbins, T. W., and Pennartz, C. M. (2004). Putting a spin on the dorsal-ventral divide of the striatum. Trends Neurosci. 27, 468–474. doi: 10.1016/j.tins.2004.06.006
Wang, L., Saalmann, Y. B., Pinsk, M. A., Arcaro, M. J., and Kastner, S. (2012). Electrophysiological low-frequency coherence and cross-frequency coupling contribute to BOLD connectivity. Neuron 76, 1010–1020. doi: 10.1016/j.neuron.2012.09.033
Winder, A. T., Echagarruga, C., Zhang, Q., and Drew, P. J. (2017). Weak correlations between hemodynamic signals and ongoing neural activity during the resting state. Nat. Neurosci. 20, 1761–1769. doi: 10.1038/s41593-017-0007-y
Zhao, F., Zhao, T., Zhou, L., Wu, Q., and Hu, X. (2008). BOLD study of stimulation-induced neural activity and resting-state connectivity in medetomidine-sedated rat. Neuroimage 39, 248–260. doi: 10.1016/j.neuroimage.2007.07.063
Keywords: resting-state MRI, functional connectivity, MUA, LFP, BOLD
Citation: Lu H, Jaime S and Yang Y (2019) Origins of the Resting-State Functional MRI Signal: Potential Limitations of the “Neurocentric” Model. Front. Neurosci. 13:1136. doi: 10.3389/fnins.2019.01136
Received: 06 July 2019; Accepted: 08 October 2019;
Published: 23 October 2019.
Edited by:
Garth John Thompson, ShanghaiTech University, ChinaReviewed by:
Wen-Ju Pan, Emory University, United StatesMauro DiNuzzo, Independent Researcher, Rome, Italy
Copyright © 2019 Lu, Jaime and Yang. This is an open-access article distributed under the terms of the Creative Commons Attribution License (CC BY). The use, distribution or reproduction in other forums is permitted, provided the original author(s) and the copyright owner(s) are credited and that the original publication in this journal is cited, in accordance with accepted academic practice. No use, distribution or reproduction is permitted which does not comply with these terms.
*Correspondence: Hanbing Lu, bHVoYUBtYWlsLm5paC5nb3Y=