- 1Department of Media and Communication, LMU Munich, Munich, Germany
- 2Institute of Media and Communication, TU Dresden, Dresden, Germany
With social media now being ubiquitously used by citizens and political actors, concerns over the incivility of interactions on these platforms have grown. While research has already started to investigate some of the factors that lead users to leave incivil comments on political social media posts, we are lacking a comprehensive understanding of the influence of platform, post, and person characteristics. Using automated text analysis methods on a large body of U.S. Congress Members' social media posts (n = 253,884) and the associated user comments (n = 49,508,863), we investigate how different social media platforms (Facebook, Twitter), characteristics of the original post (e.g., incivility, reach), and personal characteristics of the politicians (e.g., gender, ethnicity) affect the occurrence of incivil user comments. Our results show that ~23% of all comments can be classified as incivil but that there are important temporal and contextual dynamics. Having incivil comments on one's social media page seems more likely on Twitter than on Facebook and more likely when politicians use incivil language themselves, while the influence of personal characteristics is less clear-cut. Our findings add to the literature on political incivility by providing important insights regarding the dynamics of uncivil discourse, thus helping platforms, political actors, and educators to address associated problems.
Introduction
Social media have become an important part of political communication: Especially during election campaigns, but also during more routine political times, politicians have widely adopted social media for broadcasting information, interacting with relevant publics, or mobilizing voters (Larsson and Kalsnes, 2014; Stier et al., 2018). Likewise, citizens now routinely use various social media platforms to follow political information and actors (Marquart et al., 2020; Newman et al., 2021). However, this increased engagement is accompanied by concerns over the incivility of interactions on social media platforms, which we broadly define here as “features of discussion that convey an unnecessarily disrespectful tone toward the discussion forum, its participants, or its topics” (Coe et al., 2014, p. 660). Due to their distinct digital architectures and communication norms, social media are believed to be “an ideal place” (Theocharis et al., 2020, p. 5) for—at least sporadically—engaging in incivil behavior or speech. Although incivility is relative and not per se a bad thing (Chen et al., 2019), previous research has identified considerable negative effects on perceptions, attitudes, and behaviors of those exposed to (political) incivility—both for “ordinary” users (Gervais, 2015; Chen and Lu, 2017) and not least for the targets of incivility (Sobieraj, 2018; Searles et al., 2020). Knowing what triggers incivility in political social media discussions is thus crucial to understand the dynamics of uncivil discourse and develop strategies for dealing with potential negative effects.
While research has already explored characteristics of platform (Oz et al., 2018; Jaidka et al., 2019), post (Stroud et al., 2015; Theocharis et al., 2020), and person characteristics (Rheault et al., 2019; Gorrell et al., 2020) that lead users to leave incivil comments on political social media posts, we are lacking a comprehensive understanding of the (combined) influence of these factors. This research project builds on and extends prior research by using automated text analysis methods on a large body of politicians' social media posts (n = 253,884) and the comments left under these posts by users (n = 49,508,863) during the period of one full year. More specifically, our empirical analysis is focused on Members of the 117th United States Congress, as almost all of them use social media, there is sufficient variation regarding personal characteristics, and their accounts constitute an important space for citizens to address and interact with their political representatives. Being interested in what influences incivility in the user comments, we test the role different social media platforms (Facebook, Twitter), features of the original post (incivility, reach, time), and personal characteristics of the posting politician (party, gender, ethnicity, sexual orientation) exert. Although recent research suggests that the politicians themselves will be the main target of incivil comments to their social media posts (Rossini, 2021), our research deliberately focuses on various utterances of incivility, as reflected in the broad definition offered above. This decision is not only informed by methodological considerations related to our approach of automated incivility detection, but most importantly by the fact that incivility has been shown to be “contagious” (e.g., Kim et al., 2021; Rega and Marchetti, 2021). As such, incivility directed toward other users or even the issue addressed in a post might deteriorate the entire discussion, lead to more (targeted) incivility, and is thus likely to have the abovementioned negative consequences for both bystanders and the posting politicians.
We start our article by looking at the role of social media for political communication in general and Members of Congress specifically, with a specific focus on the factors that make the social media pages of politicians a breeding ground for incivil communication. Building on that, we discuss three sets of predictors—platform, post, and person characteristics—that are likely to influence the amount of incivility in user comments on Congresspeople's social media pages.
Literature Review
Social Media and (Congressional) Political Communication
Social media platforms such as Facebook, Twitter, and Instagram are now a routine way for politicians around the globe to communicate with citizens and other target audiences (e.g., Jungherr, 2016; Bossetta, 2018; Stier et al., 2018). Whether during election campaigns or in everyday political business, social media mainly fulfill three functions in that regard: (1) a push function (i.e., directly providing information without relying on gatekeepers), (2) a pull function (i.e., seeking input from citizens—either by asking them or observing their behavior), and (3) a networking function (DePaula et al., 2018; see also Kelm et al., 2019). However, while some politicians regularly make use of the interactive features of social media platforms, the majority tends to focus on broadcasting information, thus seeming less interested in engaging with the public (Graham et al., 2016; Tromble, 2018). Stromer-Galley (2019) has established the concept of “controlled interactivity” to describe the tendency to deploy interactive features only to an extent that helps a candidate to get (re-)elected (see also Freelon, 2017). Yet, even if politicians do not have an interest in actual interaction, being on social media makes them approachable by citizens, who can easily post comments on the content politicians provide (Rossini et al., 2021a). Being directly associated with politicians' posts, the public comments on social media platforms may shape other users' perceptions of the candidate, the public's opinion, or of the addressed issues (for an overview see Ksiazek and Springer, 2018; Ziegele et al., 2018). Thus, it seems of high importance to not only consider the content that is provided by political actors, but also the responses and comments to said content.
Social media has also changed the way that Members of the United States Congress communicate, equipping “both Congressional representatives and constituents with new opportunities to reach their goals” (Barbish et al., 2019, p. 8; see also Golbeck et al., 2010; O'Connell, 2018). Current research shows that Members of the U.S. Congress seem to be avid users of social media and that this engagement has increased in recent years (van Kessel et al., 2020): In 2020, a typical Member of Congress has produced 81% more tweets and 48% more Facebook posts than in a comparable period in 2016. Moreover, follower numbers and the average number of retweets/shares have grown as well. According to van Kessel et al.'s (2020) analyses, Democratic Congresspeople tend to post more often and have more followers on Twitter than Republican ones, while this gap is considerably smaller on Facebook. This points to differences between the platforms, for example, in terms of affordances, the typical (political) audience, or politicians' differing perceptions of the expected communication behavior. Following a characteristic long tail distribution, only a small group of Congresspeople accounts for the majority of social media engagement, with the 10% most-followed politicians receiving more than 75% of the engagement metrics (e.g., favorites, reactions). As the study by van Kessel et al. (2020) does not have data on either the amount or the content of the user comments left on the social media posts, differences in users' commenting behavior resulting from the platform's or politicians' characteristics cannot be accounted for. However, building on existing research, there is reason to believe that the comments posted to the social media pages of Congresspeople might not always be friendly and constructive (Theocharis et al., 2020; Ward and McLoughlin, 2020; Rossini, 2021; Rossini et al., 2021a; Southern and Harmer, 2021).
Indeed, political actors seem to be more likely to become targets of (verbal) abuse due to two main reasons: First, politicians are public figures and perceived as people with power who thus “deserve” to be judged and criticized (Ward and McLoughlin, 2020). Of course, in democratic societies, political actors should be open to citizens' criticism, but the evidence suggests that criticism often goes hand in hand with the use of profanities or personal attacks (Rossini, 2021). Second, politicians regularly engage in incivil behavior themselves, which has been shown to be a trigger for “bottom-up incivility” (Rega and Marchetti, 2021, p. 125; see also Gervais, 2017; Kim et al., 2021). In addition, the specific characteristics of social media platforms are likely to amplify the problem of incivility. First, social media—as “more personally oriented networks” (Metz et al., 2020, p. 206)—have accelerated overarching tendencies for political personalization, thus putting the focus on the (private) individual rather than their party or professional stances (Metz et al., 2020, see also Enli and Skogerbø, 2013; Barbish et al., 2019). As Ward and McLoughlin (2020, p. 54) argue, such strategies are likely to “invite more personalized (negative) comments in return.” Second, the actual or perceived anonymity on social media platforms can encourage people to be more aggressive in their communication behavior because they feel disinhibited and less likely to face sanctions for their behavior (Suler, 2004; Brown, 2018; Ward and McLoughlin, 2020). Relatedly, not only are the commenters lulled into a sense of anonymity, they also do not see the politicians' reactions or facial expressions, which makes incivil behavior appear less hurtful (Lapidot-Lefler and Barak, 2012). Third, social media communication tends to be low-cost and more immediate, thus stimulating a more emotional and less considerate type of speech (Theocharis et al., 2020; Ward and McLoughlin, 2020). Fourth, engaging in incivil behavior online can also have community-building properties by creating a sentiment of “us (ordinary people)” vs. “them (powerful politicians);” in that regard, name-calling or verbal attacks may serve the strengthening of bonds among social media users (Rieger et al., 2021; Rossini, 2021).
Taken together, previous research clearly indicates the central role of social media for (Congressional) political communication and further suggests that Congresspeople will at least sporadically be faced with disrespectful, rude, or outright aggressive comments on their social media pages. While we have already established that politicians as a generalized group might be especially susceptible to receive such comments, we will now focus more closely on the factors that might be responsible for users resorting to incivility in their comments.
Predictors of Incivility in Political Social Media Comments
Most of the research to date has focused on how politicians use social media, but not on how the public engages with their content and the factors that drive engagement as well as specific forms of (incivil) communication (see also Rossini et al., 2021a). As Xenos et al. (2017) have outlined, how users respond to politicians' social media activity is determined by factors that the political actors can actively control (i.e., how they themselves are communicating), but also by factors beyond their control (i.e., their [attributed] sociodemographic identity; general characteristics of the social media platform). To account for both of these factors, we focus on the role of platform characteristics, post characteristics, and personal characteristics of the posting Congress Member.
Platform Characteristics
While all social media platforms share certain characteristics (Bayer et al., 2020), there are also important differences in terms of their affordances, features, and user populations that might influence the (degree of) incivility in users' comments. Theorizing that the greater identifiability would lead people to be more civil on Facebook than on the more anonymous YouTube, Halpern and Gibbs (2013) have analyzed user comments posted to the official White House social media accounts on both platforms. Their analysis of about 7,000 comments left in the summer of 2010 showed that discussions on Facebook were indeed more polite and symmetrical than those on YouTube. However, in a more recent study conducted in the context of the Eurovision Song Contest win of drag queen Conchita Wurst, Yun et al. (2020) found that Facebook comments were more negative than YouTube comments. Using Twitter's doubling of the tweet character limit in November 2017 as the setting for a natural experiment, Jaidka et al. (2019) have investigated how changing the design within a platform might affect the character of political discussions. Relying on supervised and unsupervised natural language processing methods, the researchers have analyzed about 358,000 user comments to U.S. politicians' tweets left between January 2017 and March 2018. The results suggest that doubling the permissible length of a tweet led to less incivil, more polite, and more constructive discussions. That this “constraint affordance”—as the authors call it—might indeed be associated with the incivility of comments is also supported by a study from Oz et al. (2018). Comparing user comments posted in response to the White House Twitter account to those on the Facebook account, the authors found that people were more uncivil and impolite on Twitter than on Facebook. However, again, it is hard to determine whether the differences and similarities between the studies can actually be attributed to the hypothesized affordances (e.g., anonymity, constraint) or rather to changes in who is using the social media platforms, how people are using them, or imperceptible changes in the platforms' algorithms. Nevertheless, the existing research shows that differences between social media platforms are to be expected when it comes to the incivility of user comments and while it might be impossible to disentangle the reasons for these differences, knowing about them certainly will be helpful for political actors to deploy adequate social media strategies. Considering the centrality of Facebook and Twitter for political communication both among citizens and politicians in the U.S. context (van Kessel et al., 2020), the compatibility with previous research, and issues related to data access and analysis, we confine our analyses to these two social media platforms and raise the following research question:
RQ1: Does the probability of receiving incivil user comments differ between Facebook and Twitter?
Post Characteristics
Research into the characteristics of the original post has often focused on variations in topics, suggesting that divisive issues (e.g., abortion) tend to lead to more heated and incivil discussions (Stroud et al., 2015; Rega and Marchetti, 2021). Relatedly, temporal variations in the (in)civility of user comments are to be expected: When a politician posts—for example, in the immediate run-up to an election or after a personal scandal—could thus have implications for the amount of incivil comments (time). Indeed, previous studies have found “significant spikes of abuse on particular days” (Ward and McLoughlin, 2020, p. 61; see also Su et al., 2018 for social media pages of news outlets), but also that incivility always seems to be prevalent on social media to a certain degree (Theocharis et al., 2020). As indicated above, research has also shown that incivility in the original post influences the amount of incivility in user comments (Gervais, 2017; Kim et al., 2021; Rega and Marchetti, 2021; Shmargad et al., 2021). This kind of “contagious incivility” has been associated with different mechanisms, with explanations ranging from behavioral mimicry to incivility increasing feelings of anger or changing social media users' perceived social norms (for an overview, see Kim et al., 2021). Interestingly, more incivil and emotionally charged comments also seem to attract more engagement on social media (Stieglitz and Dang-Xuan, 2013; Kim et al., 2021). This might create a vicious circle in which a combination of social media algorithms and “natural” usage behavior highlights incivil comments, which then leads to even more incivility. Accordingly, it seems crucial to consider the reach of the original post, for which we—in the absence of more valid viewing metrics—consult the number of favorites or likes a post has received. Research indicates that social media attention focuses disproportionately on a handful of most prominent politicians, who also tend to receive the most engagement for their posts (Gorrell et al., 2020; van Kessel et al., 2020). Gorrell et al. (2020) show that the distribution of incivil comments is even more disproportionate, with the most prominent political actors receiving about 6% abuse in their replies, compared with around 1% for the average politician. Based on these considerations, we ask and hypothesize:
RQ2: Does the probability of receiving incivil user comments differ depending on time?
H1: Incivility in the original social media post is positively associated with the probability of receiving incivil user comments.
H2: The reach of the original social media post is positively associated with the probability of receiving incivil user comments.
Person Characteristics
Some politicians are at greater risk of becoming victims of incivility than others—especially certain sociodemographic and personal characteristics have been associated with incivil behavior by citizens. To begin with, research shows that gender plays a role, with female politicians generally being more likely to receive incivil comments than male politicians (Rheault et al., 2019; Gorrell et al., 2020; but see: Theocharis et al., 2020; Rossini et al., 2021a; Southern and Harmer, 2021). Moreover, digital abuse seems to be even more common for sexuality diverse persons who identify as lesbian, gay, or bisexual (Powell et al., 2020; Ştefsniţă and Buf, 2021). Likewise, ethnicity seems to be a trigger for incivility: For example, an analysis by Gorrell et al. (2019, p. 1) shows that UK Parliament politicians from ethnic minority groups were repeatedly confronted with “disturbing racial and religious abuse,” especially when they started conversations about race or religion. In the context of political discussions, it could also be relevant with which party a Member of Congress is affiliated. An analysis by Rossini et al. (2021b) shows that Democratic candidates seem to receive more incivil user comments than Republican candidates and Theocharis et al. (2020) have found that politicians who adopt a more extreme ideological position are more regularly confronted with incivility on social media. Overall, the evidence thus far suggests that personal characteristics might be a crucial driving force for incivility, presumably resulting both from (user-sided) prejudices against certain social groups as well as (communicator-sided) differences in posting behavior. If certain politicians indeed receive more incivil comments, this is potentially alarming, as research shows that this could also contribute to them retreating from online discussions (Sobieraj, 2018). Based on the previous findings regarding person characteristics, we hypothesize:
H3: Being female is positively associated with the probability of receiving incivil user comments.
H4: Being homosexual or bisexual is positively associated with the probability of receiving incivil user comments.
H5: Being a Person of Color (PoC) is positively associated with the probability of receiving incivil user comments.
H6: Being a member of the Democratic Party is positively associated with the probability of receiving incivil user comments.
Materials and Methods
U.S. Congress Social Media Posts and Comments Sample
The 117th U.S. Congress consists of 100 senators and 435 representatives. We first compiled a list of names, setting a cutoff date of March 1, 2021 (and thus excluding officials elected after said date), and identified official Facebook and Twitter accounts of all Members of Congress, with a limit of one account per platform and person (as several members use multiple accounts). Personal characteristics—including age, gender, sexual orientation, ethnicity, and party affiliation1—were scraped from Wikipedia profiles and manually cross-validated. We then obtained all posts made by these accounts from July 1, 2020, to June 30, 2021, thus covering about a half year each before and after the Congress convened, through the Twitter Academic API and CrowdTangle, respectively. Finally, we obtained user comments (or, in Twitter terminology, replies) made on these posts (tweets), again relying on the Twitter Academic API for tweets and the open-source application Facepager (Jünger and Keyling, 2021) to access Facebook's Graph API. Due to computational resources and API rate limits, we opted for a random sample of 60% of all original posts and up to 5,000 comments per post. We furthermore excluded accounts of Congress Members with <30 posts made during the period of investigation and all original posts and comments consisting of less than three characters. This led to a final sample of 49,508,863 comments left on 253,884 posts by 525 Members of Congress (see Table 1 for a breakdown by platform).
Incivility Classifier
To classify these millions of comments regarding their incivility, we fine-tuned the base, uncased BERT (Bidirectional Encoder Representations from Transformers; Devlin et al., 2019) model with a binary classification output layer using the Python module Transformers (Wolf et al., 2020). We used three datasets of manually labeled comments as training data, namely 6,277 online news website comments from Coe et al. (2014)2, 4,000 Twitter comments replying to or mentioning U.S. politicians from Theocharis et al. (2020), and 4,982 Reddit comments from Davidson et al. (2020). All three studies employed similar definitions of incivility, focusing on name-calling, aspersion and accusations of lying, pejorative speech, and vulgarity. While the latter two studies ultimately treat incivility as a binary construct (civil vs. incivil) in their empirical analysis, Coe et al. (2014) distinguish between five different forms of incivility (name-calling, aspersion, lying, vulgarity, and pejorative speech). To train a binary classifier, we thus re-labeled comments in this dataset as “incivil” if at least one of those forms of incivility was coded for a particular comment and as “civil” if this was not the case. We set 20% of comments per dataset aside for testing our model and used the remaining comments in one combined dataset for training the model for four epochs, with another 10% of the remaining comments used for in-training validation.
To gauge the performance of the model, we tested it on the held back testing samples of the three datasets individually and used the model trained by Davidson et al. (2020), explicitly labeled as an incivility classifier to be used “across social media platforms” (p. 95), as a benchmark. We furthermore manually labeled 2,000 randomly drawn posts and comments from the U.S. Congress Social Media Posts and Comments sample described in the previous section to test the performance of the model for this project specifically3. The classification results can be found in Table 2. Overall, results for the “civil” category suggest a reliable classification, with little differences between the two models and all relevant metrics of our classifier above 0.8. For the “incivil” category, results are less convincing but still on acceptable levels considering the heterogeneity of the concept. The one exception here is the Coe et al. (2014) dataset, where our classifier performs significantly better than the Davidson et al. (2020) model but still fails to reach reliable performance scores. However, it should be noted that other classifiers have struggled with this dataset before (Sadeque et al., 2019; Ozler et al., 2020) and that both the age and the domain of the comments (news website comments as opposed to social media comments) bear less resemblance to the project at hand than the other datasets. Comparing the two classifiers tested here, our model achieves higher scores on all datasets for both recall and the F1 (harmonic mean of recall and precision) measure, and as such is deemed the most viable option. For the project at hand, specifically, precision (74.9%), recall (60.0%), and F1 (66.7%) scores are in line with earlier investigations (Theocharis et al., 2020). However, the lower precision scores as compared to the benchmark—which are to be expected, considering the general trade-off between precision and recall—suggest that our results likely misjudge the actual prevalence of incivility to some degree. We will thus address this shortcoming both empirically and in our discussion.
Incivility Prediction Model
To test our hypotheses and research questions, we estimated a multilevel binomial regression model predicting whether a comment is incivil or not (reference category), with comments nested in posts nested in persons (i.e., Congress Members). As outlined above, we investigate platform (Facebook or Twitter), post (reach, approximated by the number of likes/favorites a post has received; the date the post was created; and whether the original post was classified as incivil or not), and person characteristics [party: Democrat or Republican; ethnicity: Person of Color (PoC) or White; gender: female or male; and sexual orientation: heterosexual or homo-/bisexual]4. To account for potential non-linear trends for date and reach, we used three-degree b-splines for both variables, which should be flexible enough to model overarching trends (e.g., incivility increasing around the run-up and aftermath of the presidential election) while at the same time avoiding unnecessary computational complexity. Furthermore, we used the common logarithm instead of the absolute value of likes/favorites as the predictor for reach, because as it is typical with social media posts, these metrics are highly skewed. All other person and post level predictors were modeled as binary predictors. Additionally, we included four more person-level variables as controls: chamber (Senator or Representative) to account for differences in term length (and, consequently, campaigning incentives); age (in years) and freshman status (first term or not) to account for differences in experience as well as the likelihood of professional social media account use; and frequency of posting per platform (common logarithm of amount of posts/tweets posted during the timespan of investigation) to account for the intensity of use of the respective social media platform. Data and scripts to reproduce the model are provided online (see Data Availability statement).
Results
Prevalence of Incivility
Before we investigate the specific research questions and hypotheses proposed for this project, we contextualize these results with the prevalence of incivility in the dataset. Overall, almost one-fourth (23.0%) of all user comments on Congress Members' social media posts in the investigated timespan are classified as containing incivil language. This is a bit higher than previous analyses of earlier timeframes (e.g., Theocharis et al., 2020 report a share of 15–20% between October 2016 and December 2017), but could indeed reflect further deterioration in discursive quality on social media since then. Crucially, Congress Members' own posts and tweets are estimated to contain much less incivility, with only about 4.1% of all original posts in the sample classified as incivil.
To address the potential distortion of these numbers by misclassification, we employ matrix back-calculation of misclassification matrix approximations based on our classifier testing data (see Bachl and Scharkow, 2017). Using the preferred standard method, treating our 2,000 manually labeled results—potentially biased themselves—as the “truth,” corrected estimations result in an even higher share of 31.3% incivil comments. Contrariwise, using the maximum possible accuracy method to approximate the misclassification matrix, the estimated share of incivil comments drops to 18.6%. In any case, these results both suggest a high share of incivility in user comments, but also a considerable amount of uncertainty in the automatic classification, which we will address in the discussion.
The share of incivility also fluctuates greatly between individual posts, Congress Members, and dates. On average, 17.6% of user comments on a single post are classified as incivil (the higher share of incivility across all posts suggesting that more popular posts receive more incivility), with the IQR ranging from 8.0 to 25.0% and both posts with no and only incivil comments present in the data. The share of incivil comments for individual Congress Members ranges from 0.6 to 38.7%, with three Congress Members estimated having to deal with more than one-third of their user comments being incivil. Finally, the daily share of incivility ranges from 13.7 to 31.2%, suggesting that the prevalence of incivility is also influenced by temporal factors such as political events.
Predictors of Incivility
Table 3 displays the results of the multilevel binomial regression model predicting incivility (described in Section Incivility Prediction Model), with estimated probabilities by predictor displayed in Figure 1. On the post level, platform (RQ1) emerged as the strongest predictor, with an average user comment incivility share of 19.3% for posts made on Twitter as opposed to 14.3% for posts made on Facebook5. Likewise, using incivil language in the original post increases the probability of incivil comments, confirming H1, with estimated probabilities of 18.9% for incivil original posts and 14.6% for civil original posts. No clearly discernible effect for the post's date emerges (RQ2)—while peak probabilities for incivility indeed center around the presidential election date (November 3rd, 2020), the estimated probabilities remain rather stable afterward. However, the slight increase in the first few months of the investigated timeframe suggests that some accounts may have only entered the public spotlight during the height of the election campaign and have been confronted with a higher share of incivility ever since. A more curvilinear relationship is apparent for post reach, with both little and very much “liked” posts receiving higher shares of incivil comments. The steep increase for very high values suggests that posts “going viral” may have unintended consequences with discussions getting more incivil. However, the comparably high share of incivility for low-reaching posts contradicts the linear relationship proposed in H2.
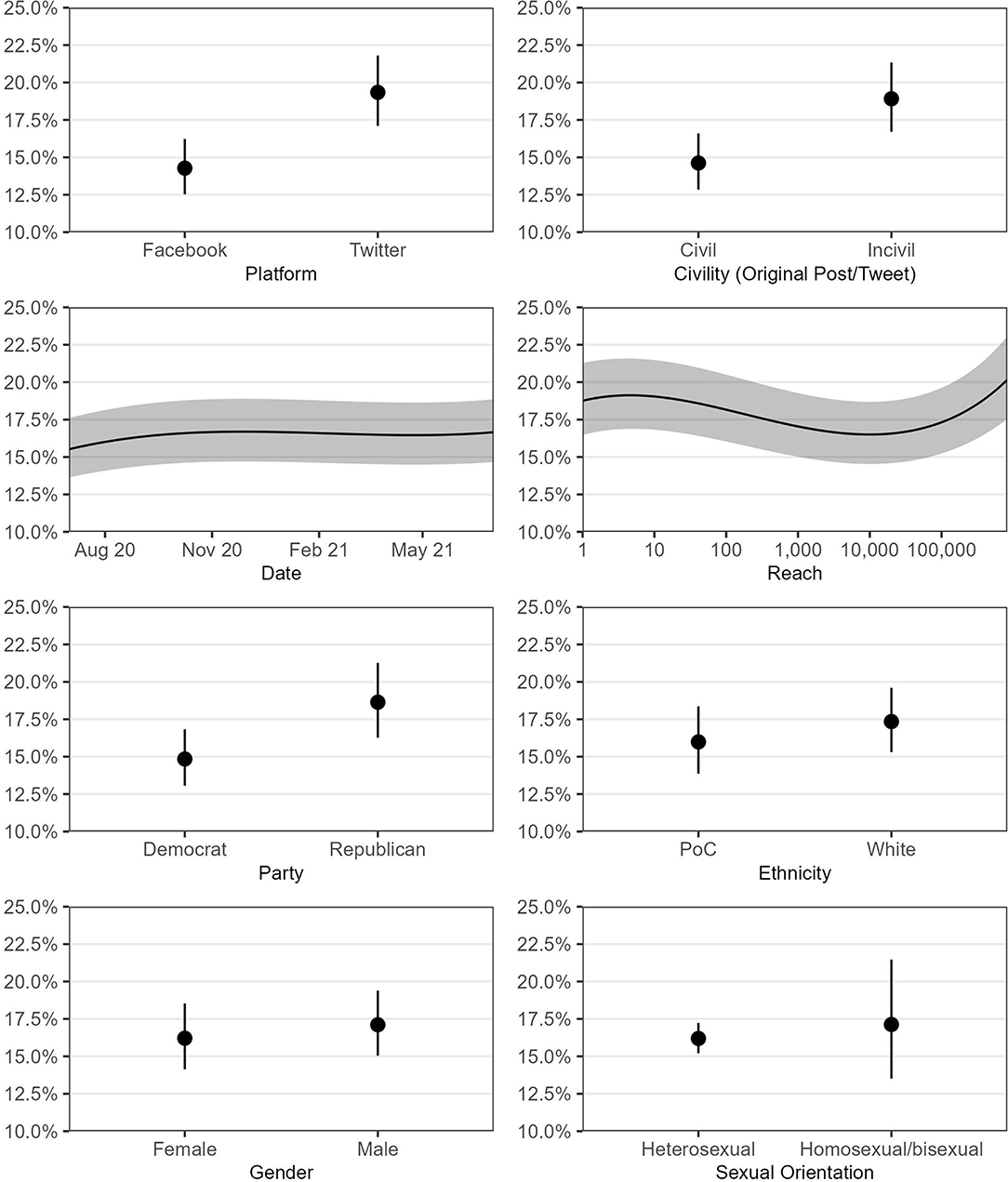
Figure 1. Estimated probabilities of incivility in comments by post and person characteristics. Vertical bars and shaded areas represent 99% confidence intervals. Probabilities estimated by calculating marginal means over both categories for other binary predictors and median values for other numerical predictors.
Effects on the person level appear to be weaker across the board. Differences for gender, sexual orientation, and ethnicity are small and in the opposite direction as hypothesized, with male members (17.1%) having slightly more incivil comments on their social media pages than female members (16.2%), contradicting H3, and White Members of Congress having more (17.3%) than PoC members (16.0%), contradicting H5. The interpretation of the very small difference for sexual orientation (H4) is further hindered by a large amount of uncertainty for the homo/-bisexual category due to the small number of openly lesbian, gay, or bisexual representatives and senators. Finally, but again contrary to expectations, posts by Republican Members of Congress receive a higher share of incivility (18.6%) than those by Democrats (14.8%). In total, these results confirm H1 (incivility of the original post), while we reject H2–6; in regard to our research questions, platform emerged as the strongest predictor overall, with posts on Twitter receiving on average five percentage points more incivil comments than posts on Facebook, while the influence of the post's date indicated a continuing growth of incivility in user comments.
Discussion
With the increasing use of social media platforms by political representatives, concerns over the incivility of political interactions have grown. Building on and extending prior research, the goal of this study was to provide a large-scale analysis of the factors that drive the use of incivil language in user comments left on social media posts by politicians. Using a fine-tuned BERT classifier, we analyzed Facebook and Twitter posts made by Members of the 117th U.S. Congress between July 1, 2020 and June 30, 2021 (n = 253,884), as well as the comments left under these posts (n = 49,508,863) regarding their incivility. Building on this dataset, we then tested the role different social media platforms (Facebook, Twitter), features of the original post (incivility, reach, time), and personal characteristics of the posting politician (party, gender, ethnicity, sexual orientation) play for the incivility of user comments.
This study confirms that incivility is highly prevalent in political discussions on social media: According to our estimations, around 23% of all user comments contained incivility, suggesting that the problem has further increased since similar investigations from a couple of years ago (Theocharis et al., 2020). However, the analysis also shows that the amount of incivility in user comments seems to be influenced by various temporal and contextual dynamics. Looking first at the differences between the two studied social media platforms, we find that it is more likely to encounter incivil user comments on Twitter than on Facebook, which mirrors previous findings in the political domain (Halpern and Gibbs, 2013; Oz et al., 2018; Jaidka et al., 2019). While the causal factors leading to this difference remain speculative—ranging from different affordances, user populations, or algorithmic curation processes—the results nevertheless suggest that politicians need to be receptive to communicative styles on different social media platforms and adjust their posting behavior accordingly. In fact, the findings also show that politicians can somewhat control users' use of incivility through leading by example. Consistent with prior studies (Gervais, 2017; Kim et al., 2021; Rega and Marchetti, 2021; Shmargad et al., 2021), our estimations provide evidence that using incivil language in the original post increases the probability of said post receiving incivil comments. Effects resulting from personal characteristics of the posting politicians are overall weaker and opposite to the direction we expected based on previous research. In contrast to findings by Rossini et al. (2021b), Republican Members of Congress in our sample were estimated to receive a higher share of incivility than Democrats. This, however, echoes findings by Su et al. (2018) who found that Facebook user comments on conservative news sites are more likely to feature extreme incivility than those on liberal news sites. Likewise, while it has previously been shown that women, sexuality diverse persons, and PoC politicians are more likely to become the targets of incivil communication online, our analysis in the context of the U.S. Congress does not support these findings. However, this should not be interpreted as there being no problem for these historically marginalized groups, as our findings are limited by the investigated timeframe (and, thus, the political climate), the composition of both the current Congress and user populations on the two studied platforms6, as well as our binary approach to measuring incivility.
This last point in particular should be kept in mind when interpreting the results of this study. As it was highlighted in similar studies on political incivility (e.g., Rossini, 2021), the classification of comments as being either civil or incivil does not allow to differentiate between “milder” and more extreme forms of incivility. For example, it might be that female politicians nominally receiver fewer incivil comments, but that the incivil comments they do receive are all the more blatant for it. In future investigations, more emphasis should thus be placed on different levels of “incivility escalation” as well as the specific content of the user comments. Moreover, it will be important to consider the different rhetorical functions incivility can have: Is it used to amplify or highlight one's opinion, to establish a sense of community, or simply to offend others? Furthermore, as our large-scale analysis demanded a focus on textual content, we are unable to account for incivility that is transmitted via animated images (e.g., GIFs), memes, or emojis (). Considering that such visual forms of communication are used more and more “for strategically masking bigoted and problematic arguments and messages” (Lobinger et al., 2020, p. 347), further research needs to focus on the problem of (audio-)visual incivility. Additional uncertainty arises from the used BERT classifier, which, while trained on three high-quality manually labeled training datasets and benchmarked against a published social media incivility classifier, showed varying results across the test datasets. As our results are generally in line with earlier investigations of the prevalence and predictors of incivility in the U.S. political context, we do not expect a systematic error in potentially misclassified posts and comments. However, this degree of uncertainty in the classifications adds a further constraint to the reliable detection of the heterogeneous concept of incivility through textual features alone. Last, our investigation is blind to influences resulting from characteristics of the posting users, that is, we are unable to tell how certain sociodemographic profiles or personality traits influence the use of incivility. To account for these user characteristics and still be able to analyze large datasets, it could be promising to use available digital trace data from users' social media accounts. In addition to inferring demographic information from social media profiles, this could also allow gathering information about users' network structure, (political) preferences, or even their personality traits (for an overview see Bleidorn and Hopwood, 2019). By tackling these issues, future research could advance our understanding of online incivility both theoretically and methodologically.
Notwithstanding these limitations, our analysis helps researchers to better understand what triggers incivility in political social media discussions, thus also assisting platforms and educators in addressing potential negative effects of incivil communication and political actors in making reasoned decisions about their social media engagement. Moreover, as research shows that merely being exposed to incivil comments can negatively affect people's perception about the trustworthiness of information and the deliberative potential of online discussions (Hwang et al., 2014; Graf et al., 2017), it is crucial to continuously monitor how the public engages with (political) content on social media.
Data Availability Statement
Data and scripts to reproduce the analysis can be found on the first author's GitHub: https://github.com/joon-e/incivility_congress.
Author Contributions
Both authors listed have made a substantial, direct, and intellectual contribution to the work and approved it for publication.
Funding
Open Access Funding by the Publication Fund of the TU Dresden.
Conflict of Interest
The authors declare that the research was conducted in the absence of any commercial or financial relationships that could be construed as a potential conflict of interest.
Publisher's Note
All claims expressed in this article are solely those of the authors and do not necessarily represent those of their affiliated organizations, or those of the publisher, the editors and the reviewers. Any product that may be evaluated in this article, or claim that may be made by its manufacturer, is not guaranteed or endorsed by the publisher.
Footnotes
1. ^Senators King and Sanders, both nominally independent, were both labeled as Democrat, as both caucus (and usually vote) with the Democratic Party.
2. ^The number of comments diverges from the number reported by Coe et al. (2014) somewhat, as the dataset provided several challenges, with comment texts spread across several hundred PDF files with different formatting, corrupted glyphs, inconsistent naming of files and folders, and no unique identifiers linking comment texts and coding table. Through a combination of automated text extraction based on text box positioning and manual revisions (e.g., file repair, renaming of files and folders), we were ultimately able to confidently match 6,277 out of 6,444 comments with their coded labels.
3. ^An intercoder reliability test based on 200 posts/replies labeled by both authors resulted in Krippendorff's α = 0.82, indicating high reliability.
4. ^Mathematically, platform and post characteristics are both on the post level in this model, with person characteristics on the higher-order person level.
5. ^All probabilities estimated by calculating marginal means over both categories for other binary predictors and median values for other numerical predictors.
6. ^A study by the Pew Research Center (Wojcik and Hughes, 2019) shows that U.S. Twitter users differ considerably from the overall U.S. adult population: They are younger, more likely than the general public to have a college degree, and more likely to identify with the Democratic Party. Moreover, they tend to have attitudes that are more liberal when it comes to issues of race, immigration, and gender.
References
Bachl, M., and Scharkow, M. (2017). Correcting measurement error in content analysis. Commun. Methods Meas. 11, 87–104. doi: 10.1080/19312458.2017.1305103
Barbish, V., Vaughn, K., Chikhladze, M., Nielsen, M., Corley, K., and Palacios, J. (2019). Congress, Constituents, and Social Media: Understanding Member Communications in the Age of Instantaneous Communication. Available online at: https://hdl.handle.net/1969.1/187026 (accessed February 9, 2022).
Bayer, J. B., Triêu, P., and Ellison, N. B. (2020). Social media elements, ecologies, and effects. Annu. Rev. Psychol. 71, 471–497. doi: 10.1146/annurev-psych-010419-050944
Bleidorn, W., and Hopwood, C. J. (2019). Using machine learning to advance personality assessment and theory. Pers. Soc. Psychol. Rev. 23, 190–203. doi: 10.1177/1088868318772990
Bossetta, M. (2018). The digital architectures of social media: comparing political campaigning on Facebook, Twitter, Instagram, and Snapchat in the 2016 U.S. election. J. Mass Commun. Q. 95, 471–496. doi: 10.1177/1077699018763307
Brown, A. (2018). What is so special about online (as compared to offline) hate speech? Ethnicities 18, 297–326. doi: 10.1177/1468796817709846
Chen, G. M., and Lu, S. (2017). Online political discourse: exploring differences in effects of civil and uncivil disagreement in news website comments. J. Broadcast. Electron. Media 61, 108–125. doi: 10.1080/08838151.2016.1273922
Chen, G. M., Muddiman, A., Wilner, T., Pariser, E., and Stroud, N. J. (2019). We should not get rid of incivility online. Soc. Media Soc. 5, 2056305119862641. doi: 10.1177/2056305119862641
Coe, K., Kenski, K., and Rains, S. A. (2014). Online and uncivil? Patterns and determinants of incivility in newspaper website comments. J. Commun. 64, 658–679. doi: 10.1111/jcom.12104
Davidson, S., Sun, Q., and Wojcieszak, M. (2020). Developing a new classifier for automated identification of incivility in social media, in Proceedings of the Fourth Workshop on Online Abuse and Harms, 95–101.
DePaula, N., Dincelli, E., and Harrison, T. M. (2018). Toward a typology of government social media communication: democratic goals, symbolic acts and self-presentation. Gov. Inf. Q. 35, 98–108. doi: 10.1016/j.giq.2017.10.003
Devlin, J., Chang, M.-W., Lee, K., and Toutanova, K. (2019). BERT: pre-training of deep bidirectional transformers for language understanding. ArXiv.
Enli, G. S., and Skogerbø, E. (2013). Personalized campaigns in party-centred politics. Twitter and Facebook as arenas for political communication. Inform. Commun. Soc. 16, 757–774. doi: 10.1080/1369118X.2013.782330
Freelon, D. (2017). Campaigns in control: Analyzing controlled interactivity and message discipline on Facebook. J. Inform. Technol. Politics 14, 168–181. doi: 10.1080/19331681.2017.1309309
Gervais, B. T. (2015). Incivility online: affective and behavioral reactions to uncivil political posts in a web-based experiment. J. Inform. Technol. Politics 12, 167–185. doi: 10.1080/19331681.2014.997416
Gervais, B. T. (2017). More than mimicry? The role of anger in uncivil reactions to elite political incivility. Int. J. Public Opin. Res. 29, 384–405. doi: 10.1093/ijpor/edw010
Golbeck, J., Grimes, J. M., and Rogers, A. (2010). Twitter use by the U.S. Congress. J. Am. Soc. Inform. Sci. Technol. 61, 1612–1621. doi: 10.1002/asi.21344
Gorrell, G., Bakir, M. E., Greenwood, M. A., Roberts, I., and Bontcheva, K. (2019). Race and Religion in Online Abuse Towards UK Politicians. Available online at: http://arxiv.org/abs/1910.00920 (accessed February 9, 2022).
Gorrell, G., Bakir, M. E., Roberts, I., Greenwood, M. A., and Bontcheva, K. (2020). Which politicians receive abuse? Four factors illuminated in the UK general election 2019. EPJ Data Sci. 9, 18. doi: 10.1140/epjds/s13688-020-00236-9
Graf, J., Erba, J., and Harn, R.-W. (2017). The role of civility and anonymity on perceptions of online comments. Mass Commun. Soc. 20, 526–549. doi: 10.1080/15205436.2016.1274763
Graham, T., Jackson, D., and Broersma, M. (2016). New platform, old habits? Candidates' use of Twitter during the 2010 British and Dutch general election campaigns. New Media Soc. 18, 765–783. doi: 10.1177/1461444814546728
Halpern, D., and Gibbs, J. (2013). Social media as a catalyst for online deliberation? Exploring the affordances of Facebook and YouTube for political expression. Comput. Hum. Behav. 29, 1159–1168. doi: 10.1016/j.chb.2012.10.008
Hwang, H., Kim, Y., and Huh, C. U. (2014). Seeing is believing: effects of uncivil online debate on political polarization and expectations of deliberation. J. Broadcast. Electron. Media 58, 621–633. doi: 10.1080/08838151.2014.966365
Jaidka, K., Zhou, A., and Lelkes, Y. (2019). Brevity is the soul of Twitter: the constraint affordance and political discussion. J. Commun. 69, 345–372. doi: 10.1093/joc/jqz023
Jünger, J., and Keyling, T. (2021). Facepager. An Application for Automated Data Retrieval on the Web [Python]. Available online at: https://github.com/strohne/Facepager (accessed February 9, 2022). (Original work published 2012).
Jungherr, A. (2016). Twitter use in election campaigns: a systematic literature review. J. Inform. Technol. Politics 13, 72–91. doi: 10.1080/19331681.2015.1132401
Kelm, O., Dohle, M., and Bernhard, U. (2019). Politicians' self-reported social media activities and perceptions: Results from four surveys among German parliamentarians. Soc. Media Soc. 5, 2056305119837679. doi: 10.1177/2056305119837679
Kim, J. W., Guess, A., Nyhan, B., and Reifler, J. (2021). The distorting prism of social media: How self-selection and exposure to incivility fuel online comment toxicity. J. Commun. 71, 922–946. doi: 10.1093/joc/jqab034
Ksiazek, T. B., and Springer, N. (2018). User comments in digital journalism: current research and future directions, in The Routledge Handbook of Developments in Digital Journalism Studies, editors Eldridge, S. A., and Franklin, B., (London: Routledge), 475–486.
Lapidot-Lefler, N., and Barak, A. (2012). Effects of anonymity, invisibility, and lack of eye-contact on toxic online disinhibition. Comput. Hum. Behav. 28, 434–443. doi: 10.1016/j.chb.2011.10.014
Larsson, A. O., and Kalsnes, B. (2014). “Of course we are on Facebook”: use and non-use of social media among Swedish and Norwegian politicians. Eur. J. Commun. 29, 653–667. doi: 10.1177/0267323114531383
Lobinger, K., Krämer, B., Venema, R., and Benecchi, E. (2020). Pepe – just a funny frog? A visual meme caught between innocent humor, far-right ideology, and fandom,; in Perspectives on Populism and the Media, editors Krämer, B., and Holtz-Bacha, C., (Baden-Baden: Nomos), 333–352.
Marquart, F., Ohme, J., and Möller, J. (2020). Following politicians on social media: effects for political information, peer communication, and youth engagement. Media Commun. 8, 197–207. doi: 10.17645/mac.v8i2.2764
Metz, M., Kruikemeier, S., and Lecheler, S. (2020). Personalization of politics on Facebook: examining the content and effects of professional, emotional and private self-personalization. Inform. Commun. Soc. 23, 1481–1498. doi: 10.1080/1369118X.2019.1581244
Newman, N., Fletcher, R., Schulz, A., Andi, S., Robertson, C. T., and Nielsen, R. K. (2021). Reuters Institute Digital News Report 2021. Reuters Institute for the Study of Journalism.
O'Connell, D. (2018). #Selfie: Instagram and the United States Congress. Soc. Media Soc. 4. doi: 10.1177/2056305118813373
Oz, M., Zheng, P., and Chen, G. M. (2018). Twitter versus Facebook: comparing incivility, impoliteness, and deliberative attributes. New Media Soc. 20, 3400–3419. doi: 10.1177/1461444817749516
Ozler, K. B., Kenski, K., Rains, S., Shmargad, Y., Coe, K., and Bethard, S. (2020). Fine-tuning for multi-domain and multi-label uncivil language detection, Proceedings of the Fourth Workshop on Online Abuse and Harms, 28–33.
Powell, A., Scott, A. J., and Henry, N. (2020). Digital harassment and abuse: experiences of sexuality and gender minority adults. Eur. J. Criminol. 17, 199–223. doi: 10.1177/1477370818788006
Rega, R., and Marchetti, R. (2021). The strategic use of incivility in contemporary politics. The case of the 2018 Italian general election on Facebook. Commun. Rev. 24, 107–132. doi: 10.1080/10714421.2021.1938464
Rheault, L., Rayment, E., and Musulan, A. (2019). Politicians in the line of fire: incivility and the treatment of women on social media. Res. Politics 6, 2053168018816228. doi: 10.1177/2053168018816228
Rieger, D., Kümpel, A. S., Wich, M., Kiening, T., and Groh, G. (2021). Assessing the extent and types of hate speech in fringe communities: A case study of alt-right communities on 8chan, 4chan, and Reddit. Soc. Media Soc. doi: 10.1177/20563051211052906. [Epub ahead of print].
Rossini, P. (2021). More than just shouting? Distinguishing interpersonal-directed and elite-directed incivility in online political talk. Soc. Media Soc. doi: 10.1177/20563051211008827. [Epub ahead of print].
Rossini, P., Stromer-Galley, J., and Zhang, F. (2021a). Exploring the relationship between campaign discourse on Facebook and the public's comments: a case study of incivility during the 2016 US presidential election. Polit. Stud. 69, 89–107. doi: 10.1177/0032321719890818
Rossini, P., Sturm-Wikerson, H., and Johnson, T. J. (2021b). A wall of incivility? Public discourse and immigration in the 2016 U.S. Primaries. J. Inform. Technol. Politics 18, 243–257. doi: 10.1080/19331681.2020.1858218
Sadeque, F., Rains, S., Shmargad, Y., Kenski, K., Coe, K., and Bethard, S. (2019). Incivility detection in online comments, in Proceedings of the Eighth Joint Conference on Lexical and Computational Semantics (*SEM 2019), 283–291.
Searles, K., Spencer, S., and Duru, A. (2020). Don't read the comments: the effects of abusive comments on perceptions of women authors' credibility. Inform. Commun. Soc. 23, 947–962. doi: 10.1080/1369118X.2018.1534985
Shmargad, Y., Coe, K., Kenski, K., and Rains, S. A. (2021). Social norms and the dynamics of online incivility. Soc. Sci. Comput. Rev. doi: 10.1177/0894439320985527. [Epub ahead of print].
Sobieraj, S. (2018). Bitch, slut, skank, cunt: patterned resistance to women's visibility in digital publics. Inform. Commun. Soc. 21, 1700–1714. doi: 10.1080/1369118X.2017.1348535
Southern, R., and Harmer, E. (2021). Twitter, incivility and “everyday” gendered othering: an analysis of tweets sent to UK members of parliament. Soc. Sci. Comput. Rev. 39, 259–275. doi: 10.1177/0894439319865519
Ştefsniţă, O., and Buf, D.-M. (2021). Hate speech in social media and its effects on the LGBT community: a review of the current research. Romanian J. Commun. Public Relat. 23, 47–55. doi: 10.21018/rjcpr.2021.1.322
Stieglitz, S., and Dang-Xuan, L. (2013). Emotions and information diffusion in social media—Sentiment of microblogs and sharing behavior. J. Manage. Inform. Syst. 29, 217–248. doi: 10.2753/MIS0742-1222290408
Stier, S., Bleier, A., Lietz, H., and Strohmaier, M. (2018). Election campaigning on social media: politicians, audiences, and the mediation of political communication on Facebook and Twitter. Polit. Commun. 35, 50–74. doi: 10.1080/10584609.2017.1334728
Stromer-Galley, J. (2019). Presidential Campaigning in the Internet Age. 2nd Edn. New York, NY: Oxford University Press.
Stroud, N. J., Scacco, J. M., Muddiman, A., and Curry, A. L. (2015). Changing deliberative norms on news organizations' Facebook sites. J. Computer Mediat. Commun. 20, 188–203. doi: 10.1111/jcc4.12104
Su, L. Y.-F., Xenos, M. A., Rose, K. M., Wirz, C., Scheufele, D. A., and Brossard, D. (2018). Uncivil and personal? Comparing patterns of incivility in comments on the Facebook pages of news outlets. New Media Soc. 20, 3678–3699. doi: 10.1177/1461444818757205
Suler, J. (2004). The online disinhibition effect. Cyber Psychol. Behav. 7, 321–326. doi: 10.1089/1094931041291295
Theocharis, Y., Barberá, P., Fazekas, Z., and Popa, S. A. (2020). The dynamics of political incivility on Twitter. SAGE Open 10, 1–15. doi: 10.1177/2158244020919447
Tromble, R. (2018). Thanks for (actually) responding! How citizen demand shapes politicians' interactive practices on Twitter. New Media Soc. 20, 676–697. doi: 10.1177/1461444816669158
van Kessel, P., Widjaya, R., Shah, S., Smith, A., and Hughes, A. (2020). Congress Soars to New Heights on Social Media. Pew Research Center. Available online at: https://www.pewresearch.org/internet/2020/07/16/congress-soars-to-new-heights-on-social-media/ (accessed February 9, 2022).
Ward, S., and McLoughlin, L. (2020). Turds, traitors and tossers: the abuse of UK MPs via Twitter. J. Legislative Stud. 26, 47–73. doi: 10.1080/13572334.2020.1730502
Wojcik, S., and Hughes, A. (2019). Sizing Up Twitter users. Pew Research Center. Available online at: https://www.pewresearch.org/internet/2019/04/24/sizing-up-twitter-users/ (accessed February 9, 2022).
Wolf, T., Debut, L., Sanh, V., Chaumond, J., Delangue, C., Moi, A., et al. (2020). Transformers: state-of-the-art natural language processing, in Proceedings of the 2020 Conference on Empirical Methods in Natural Language Processing: System Demonstrations, 38–45.
Xenos, M. A., Macafee, T., and Pole, A. (2017). Understanding variations in user response to social media campaigns: a study of Facebook posts in the 2010 US elections. New Media Soc. 19, 826–842. doi: 10.1177/1461444815616617
Yun, G. W., Allgayer, S., and Park, S.-Y. (2020). Mind your social media manners: pseudonymity, imaginary audience, and incivility on Facebook vs. YouTube. Int. J. Commun. 14, 21.
Keywords: incivility, social media, political discussions, content analysis, computational methods
Citation: Unkel J and Kümpel AS (2022) Patterns of Incivility on U.S. Congress Members' Social Media Accounts: A Comprehensive Analysis of the Influence of Platform, Post, and Person Characteristics. Front. Polit. Sci. 4:809805. doi: 10.3389/fpos.2022.809805
Received: 05 November 2021; Accepted: 28 January 2022;
Published: 23 February 2022.
Edited by:
Alessandro Nai, University of Amsterdam, NetherlandsReviewed by:
Rodrigo Sandoval, Universidad Autónoma del Estado de México, MexicoBumsoo Kim, Joongbu University, South Korea
Copyright © 2022 Unkel and Kümpel. This is an open-access article distributed under the terms of the Creative Commons Attribution License (CC BY). The use, distribution or reproduction in other forums is permitted, provided the original author(s) and the copyright owner(s) are credited and that the original publication in this journal is cited, in accordance with accepted academic practice. No use, distribution or reproduction is permitted which does not comply with these terms.
*Correspondence: Julian Unkel, anVsaWFuLnVua2VsQGlma3cubG11LmRl; Anna Sophie Kümpel, YW5uYS5rdWVtcGVsQHR1LWRyZXNkZW4uZGU=