- 1Microbes in the Food Chain, Quadram Institute Bioscience, Norwich, United Kingdom
- 2Norwich Medical School, University of East Anglia, Norwich, United Kingdom
We report here the identification of four gene functions of principal importance for the tolerance of meropenem stress in Escherichia coli: cell division, cell envelope synthesis and maintenance, ATP metabolism, and transcription regulation. The primary mechanism of β-lactam antibiotics such as meropenem is inhibition of penicillin binding proteins, thus interfering with peptidoglycan crosslinking, weakening the cell envelope, and promoting cell lysis. However, recent systems biology approaches have revealed numerous downstream effects that are triggered by cell envelope damage and involve diverse cell processes. Subpopulations of persister cells can also arise, which can survive elevated concentrations of meropenem despite the absence of a specific resistance factor. We used Transposon-Directed Insertion Sequencing with inducible gene expression to simultaneously assay the effects of upregulation, downregulation, and disruption of every gene in a model E. coli strain on survival of exposure to four concentrations of meropenem. Automated Gene Functional Classification and manual categorization highlighted the importance at all meropenem concentrations of genes involved in peptidoglycan remodeling during cell division, suggesting that cell division is the primary function affected by meropenem. Genes involved in cell envelope synthesis and maintenance, ATP metabolism, and transcriptional regulation were generally important at higher meropenem concentrations, suggesting that these three functions are therefore secondary or downstream targets. Our analysis revealed the importance of multiple two-component signal transduction mechanisms, suggesting an as-yet unexplored coordinated transcriptional response to meropenem stress. The inclusion of an inducible, transposon-encoded promoter allowed sensitive detection of genes involved in proton transport, ATP production and tRNA synthesis, for which modulation of expression affects survival in the presence of meropenem: a finding that would not be possible with other technologies. We were also able to suggest new targets for future antibiotic development or for synergistic effects between gene or protein inhibitors and existing antibiotics. Overall, in a single massively parallel assay we were able to recapitulate many of the findings from decades of research into β-lactam antibiotics, add to the list of genes known to be important for meropenem tolerance, and categorize the four principal gene functions involved.
Introduction
Meropenem is a bactericidal β-lactam antibiotic of the carbapenem class. Like other β-lactams, its primary mode of action is inhibition of penicillin binding proteins that mediate the crosslinking of peptidoglycan chains in the biogenesis of the cell wall (Drusano, 1997). This weakens the structure of the cell envelope and leads to cell lysis. In Escherichia coli, meropenem binds with greatest affinity to penicillin binding protein 2 (mrdA) and penicillin binding protein 4 (dacB), but also binds to PBP1a (mrcA), PBP1b (mrcB) and PBP3 (ftsI) (Davies et al., 2008; Kocaoglu and Carlson, 2015). Meropenem is highly resistant to degradation by many β-lactamases and cephalosporinases, has a broad spectrum of antibacterial activity and displays enhanced activity against Gram-negative bacteria compared to many other β-lactams (Yang et al., 1995). As such it is considered by the World Health Organization to be critical for human medicine.
Resistance to an antibiotic is the ability of a population of cells to grow at concentrations of antibiotic above a designated breakpoint minimum inhibitory concentration (MIC) (Balaban et al., 2019). However, cells also experience stress when exposed to concentrations below the MIC, and the study of their response to such conditions can provide insights into the biological mechanisms of antibiotic action and recalcitrance. Even above the MIC, some cells may display tolerance to antibiotics: surviving when other cells would not, despite the lack of a specific resistance factor; for example, due to slower growth (Dörr, 2021). Antibiotic tolerance can facilitate the evolution of resistance (Levin-Reisman et al., 2017; Windels et al., 2019) and is a contributary factor to the antibiotic resistance crisis (Church and McKillip, 2021). Subpopulations of tolerant cells that can survive treatment above the MIC of a bactericidal antibiotic and resume growth when exposure ends are known as persister cells (Balaban et al., 2019). An understanding of the separate but related mechanisms giving rise to antibiotic resistance, tolerance and persistence remains elusive, but a significant advance could be made by defining the sets of genes involved in each phenomenon: the “resistome”, “tolerome” and “persistome”. This would facilitate our ability to develop treatments to combat acute and recurrent bacterial infections (Fisher et al., 2017) and help to identify drug targets for use in combination therapies to potentiate the effects of existing antibiotics.
Transposon-Directed Insertion Sequencing with inducible gene expression (TraDIS-Xpress) provides a rapid and sensitive means to assess the contribution to survival in a defined growth condition of every gene within a genome by growing a pooled library of transposon-insertion mutants and quantifying the relative competitive success of every mutant (Langridge et al., 2009; Yasir et al., 2020). The mutant library used here was constructed by high frequency transposition of a mini-Tn5 derivative transposon containing a kanamycin resistance marker to allow selection for transformants, and an inducible promoter (Ptac). Typically, hundreds of thousands of mutants are generated and, because of the heterogeneity of Tn5 insertion site recognition, multiple transposon-insertion mutants will be created for every region of the genome. Transposon insertions within a gene will lead to inactivation of that gene by truncation or frame shift (termed “knockout insertions” here). Therefore, when the library is cultured, cells containing knockout insertions in genes that are important for growth in the selected conditions will exhibit differential growth and survival rates. Insertions at each location can be enumerated by sequencing, with changes in insertion frequency between conditions providing an indication of the relative importance of each genomic locus.
Additionally, when Ptac is induced, transposons located upstream of a gene will drive increased transcription, while transposons downstream of a gene will down-regulate transcription through RNA-interference effects. Therefore, TraDIS-Xpress can simultaneously assay the growth effects of inactivation, up-regulation, and down-regulation of every gene in the genome. We have found this approach to be powerful for assaying the roles of essential genes in stress responses based upon changes to expression (Yasir et al., 2020). Essential genes are often blind spots in traditional transposon mutagenesis studies as they cannot be inactivated.
Despite the long history of studying β-lactam antibiotics and their effects on cell physiology, we still have not arrived at a complete understanding of the mechanisms involved in killing and antagonism. In particular, the knock-on effects in multiple cell processes caused by acute damage to the peptidoglycan layer and the response to various concentrations of antibiotic have not been fully explored. We therefore applied TraDIS-Xpress to study the response of E. coli strain K-12 substrain BW25113 cells to a range of meropenem concentrations equivalent to 0.25×, 0.5×, 1× and 2× the MIC.
Materials and methods
Bacterial strains and growth conditions
The TraDIS-Xpress transposon mutant library was constructed in E. coli BW25113 and stored at −80°C in 15% glycerol; and has been described previously (Yasir et al., 2020). Briefly, a mini-Tn5 transposon encoding a kanamycin resistance cassette and an outward-transcribing, isopropyl-β-D-1-thiogalactopyranoside (IPTG)-inducible promoter (Ptac) was mixed with an EZ-Tn5 Transposase (Lucigen) and electroporated into competent cells. Transformants were selected on lysogeny agar plates containing kanamycin then collected and stored as a single pool. The library contains >700,000 unique transposon-insertion mutants.
Selection of transposon-insertion mutants in the presence of meropenem was performed in duplicate. Approximately 107 c.f.u. from the library were grown overnight at 37°C in 1 mL of lysogeny broth containing meropenem at 2×, 1×, 0.5×, and 0.25× the MIC of 0.032 mg.mL−1. Control cultures without meropenem were grown in parallel. For each concentration of meropenem, cultures were grown in which 0 (uninduced), 0.2 or 1.0 mM IPTG was added.
TraDIS-Xpress sequencing
DNA extraction and sequencing were carried out as described previously (Yasir et al., 2020). Briefly, after growing the cultures as described above, genomic DNA was extracted using a Quick-DNA Fungal/Bacterial 96 kit (Zymo Research) and fragmented using a Nextera tagmentation kit (Illumina). The fragmented DNA was amplified by PCR using oligonucleotide primers that hybridized at the transposon ends and contained an Illumina adapter sequence at their 5′ end. Sequencing of the resulting DNA libraries was done on a NextSeq 500 sequencing machine (Illumina) using a NextSeq 500/550 high output v2 kit for 75 cycles (Illumina).
Insertion sequence data processing and analysis
Nucleotide read data were demultiplexed and converted to fastq format by bcl2fastq v. 2.20 (Illumina). Reads were then mapped to the E. coli BW25113 genome (CP009273) to identify the sites of transposon insertion using the Burrows-Wheeler Aligner (BWA) program (Li and Durbin, 2009) within Bio::TraDIS v. 1.4.1 (Barquist et al., 2016). The resulting transposon insertion site plots were manually inspected in the Artemis genome browser (Carver et al., 2012). AlbaTraDIS v. 0.0.5 (Page et al., 2020) was used to compare transposon insertion data between conditions. For each gene, insertions were counted within the coding sequence and within the 198 bp before and after the gene, with separate counts for the “forward”, “reverse” and “combined” orientations. This information was used to identify genes and adjacent regions with significantly changed transposon insertion patterns between condition and control. Data for the three IPTG induction concentrations were pooled and analyzed together for each meropenem concentration. This simplified downstream data analysis but allowed inspection of individual induction conditions if required.
AlbaTraDIS identified genes with significant changes in knockout insertions, insertions upstream of a gene (5′) and insertions downstream of a gene (3′) and provided values for the log2 fold change between control and condition (log2FC); number of reads located at each locus (counts) per million total reads for the sample, converted to log2 (log2CPM); a p-value of statistical significance; a q-value (the p-value adjusted for the false discovery rate); and a gene report suggesting the most likely interpretation of the data such as “knockout” (insertion within the coding region), “upregulated” (transcribed by Ptac) or “downregulated” (RNAi effect of Ptac). These data were further processed using Excel (Microsoft) and R v. 4.1.2 (R Core Team, 2021).
Gene functional classification
Gene Functional Classification was performed using the Database for Annotation, Visualization and Integrated Discovery (DAVID) knowledgebase (Huang et al., 2009a; Huang et al., 2009b). The AlbaTraDIS output was filtered for knockout insertions for which at least one meropenem concentration met the criteria of q<0.0001 and log2CPM>3. This identified 296 gene names, which were uploaded to the DAVID website. The Gene Functional Classification tool was used to compare functional annotation terms for each gene in the list. A kappa distance score was calculated for every pair of genes, which governed the partitioning of genes into functional clusters using a fuzzy heuristic partitioning approach that allows genes to participate in more than one cluster to reflect the complexity of protein function. Kappa score calculation used a similarity term overlap = 4 and similarity threshold = 0.40. Classification into groups used an initial group membership = 5, final group membership = 5 and multiple linkage threshold = 0.60. E. coli strain K-12 substrain MG1655 was used as a reference genome to calculate an enrichment score for clustered genes.
Relative antibiotic minimum inhibitory concentration and synergy measurement
These were performed using 96-well microplates with 200 µL lysogeny broth per well. Two-fold serial dilutions were used. Approximately 105 c.f.u. of bacteria were added and incubated at 37°C overnight. The concentration of antibiotic at which the growth was inhibited >90% based on optical density was taken as the MIC. Antibacterial synergy between cefsulodin and meropenem was assessed similarly, employing combinations of concentrations of both and these were performed twice.
Results
More than 500 genes (q < 0.01) were identified as playing a role in the response of E. coli to meropenem based on phenotypes generated through knockout insertions, and upregulation or RNA interference from the transposon-encoded Ptac (Supplementary Table 1). However, to focus on the most significant hits, stricter cut-off parameters of q < 0.0001 and log2CPM > 3 for at least one meropenem concentration were used, ensuring all loci meeting these criteria had a highly significant change in transposon abundance between conditions and were represented by many mutants. This identified 296 genes with a very significant change in the number of knockout insertions. Hierarchical clustering by Euclidean distance of log2FC revealed robust clustering by meropenem concentration, suggesting an overall correlation between dose and effect of knockout mutations (Figure 1). Approximately two-thirds of the significant genes had a positive log2FC, indicating that knockouts of those genes tend to increase survival with meropenem. The other one-third had a negative log2FC in knockout insertions, indicating that loss of function of those genes lowers fitness for meropenem exposure.
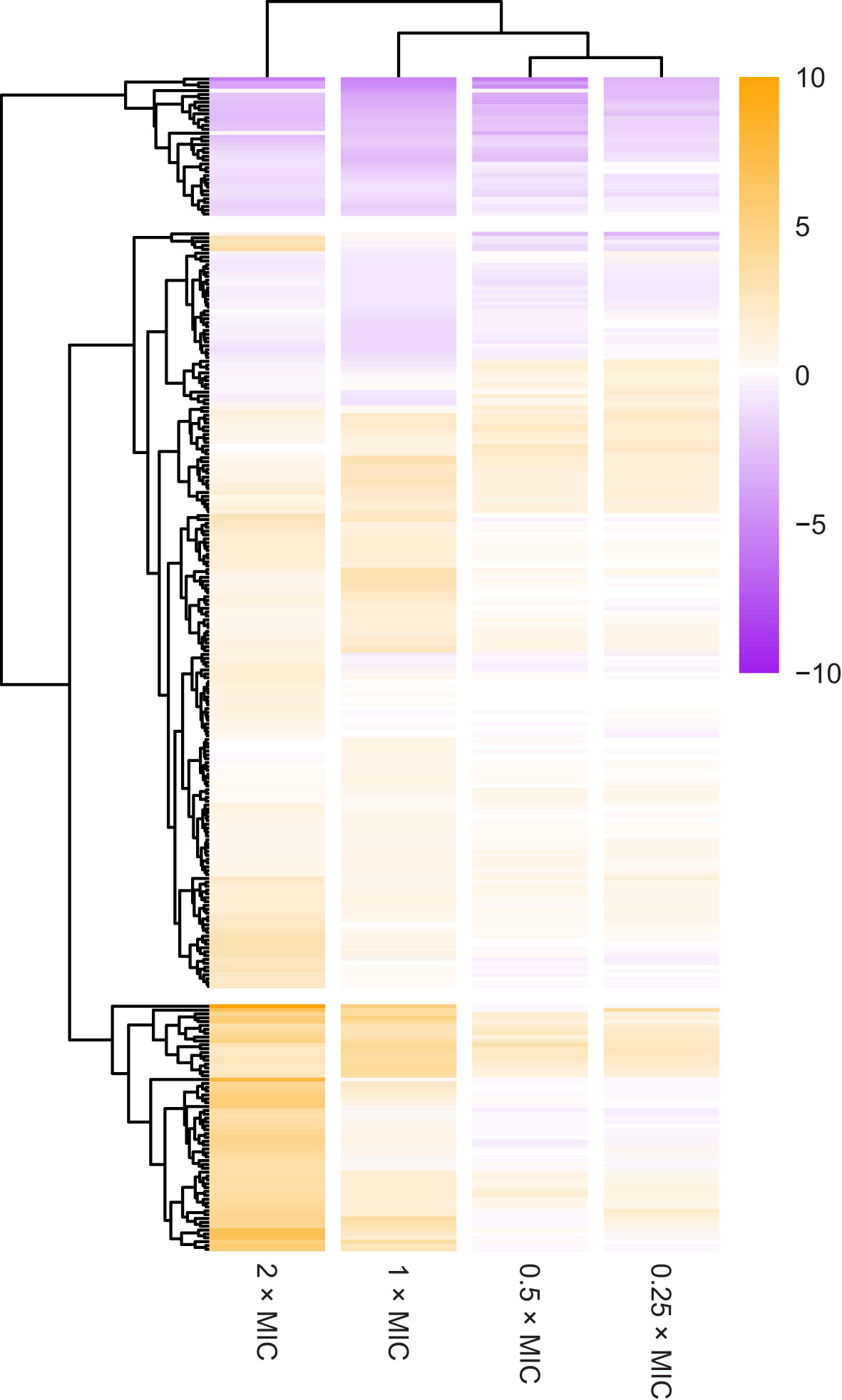
Figure 1 Overview of selection for transposon insertion mutants in the 296 most significant genes. The heatmap shows changes in number of transposon insertions identified within the coding sequence of each gene at each meropenem concentration tested, compared to control cultures without meropenem. Genes are ordered by hierarchical clustering by Euclidian distance of log2FC. Positive log2FC values indicate that transposon insertion mutants are selected for, suggesting that disruption of the gene is beneficial to survival under those conditions. Negative log2FC values indicate that transposon insertion mutants are selected against, suggesting that the gene is important for survival under those conditions.
Individual genes clustered into three main groups based on survival of knockout insertion mutants at different meropenem concentrations (Figure 1): 36 genes had negative log2FC values at all meropenem concentrations; 64 genes primarily had a positive log2FC and knockout insertions tended to increase tolerance more at 2× and 1×MIC than below the MIC; and the remaining 196 genes form a cluster containing the genes that had more moderate log2FC values, with a mixture of dose response patterns.
Four principal gene functions are involved in meropenem tolerance
Gene Functional Classification using the 296 most significant genes classified 108 genes into nine functional groups whose functions were over-represented compared to the E. coli MG1655 genome (Figure 2 and Supplementary Table 2). Eight of the nine groups could be assigned to one of three high-level functions that play the most significant roles in E. coli exposure to meropenem: cell envelope biogenesis, ATP metabolism, and regulation of transcription (Figure 3). Gene Functional Classification provides an overview of the most important groups of genes in an experiment. However, because it depends on annotation to cluster genes with similar functions it can overlook or falsely partition similar genes that have been annotated with different terms. For example, nuo and atp genes that all encode components of large enzyme complexes were placed in different groups based on their biochemical function within the enzyme complex (Figure 2).
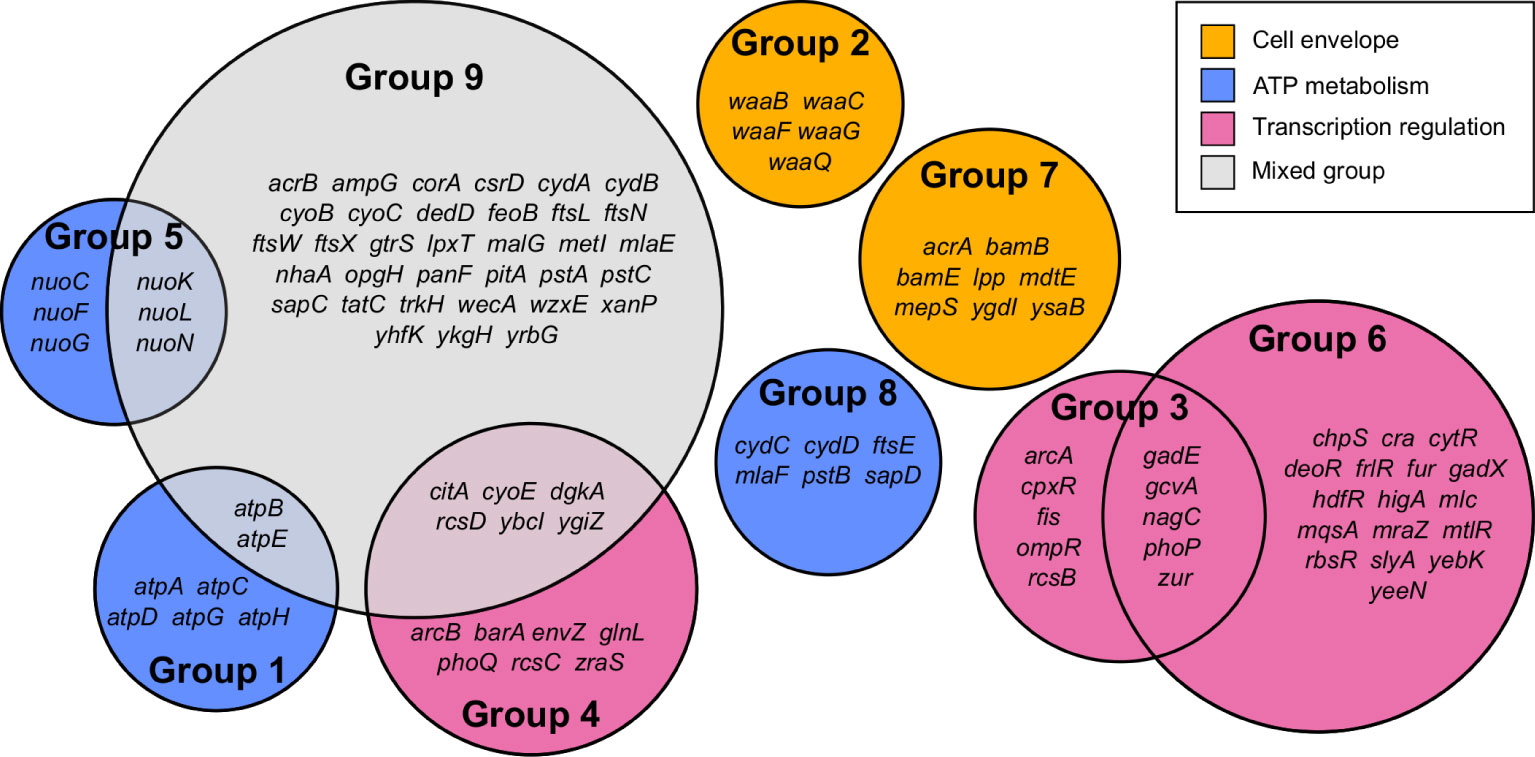
Figure 2 Summary of 108 genes comprising nine functional groups identified by Gene Functional Classification. The list of 296 most significant genes was compared to the E. coli str. K-12 substr. MG1655 genome to identify over-represented gene functions, which were then clustered by their categories in multiple databases.
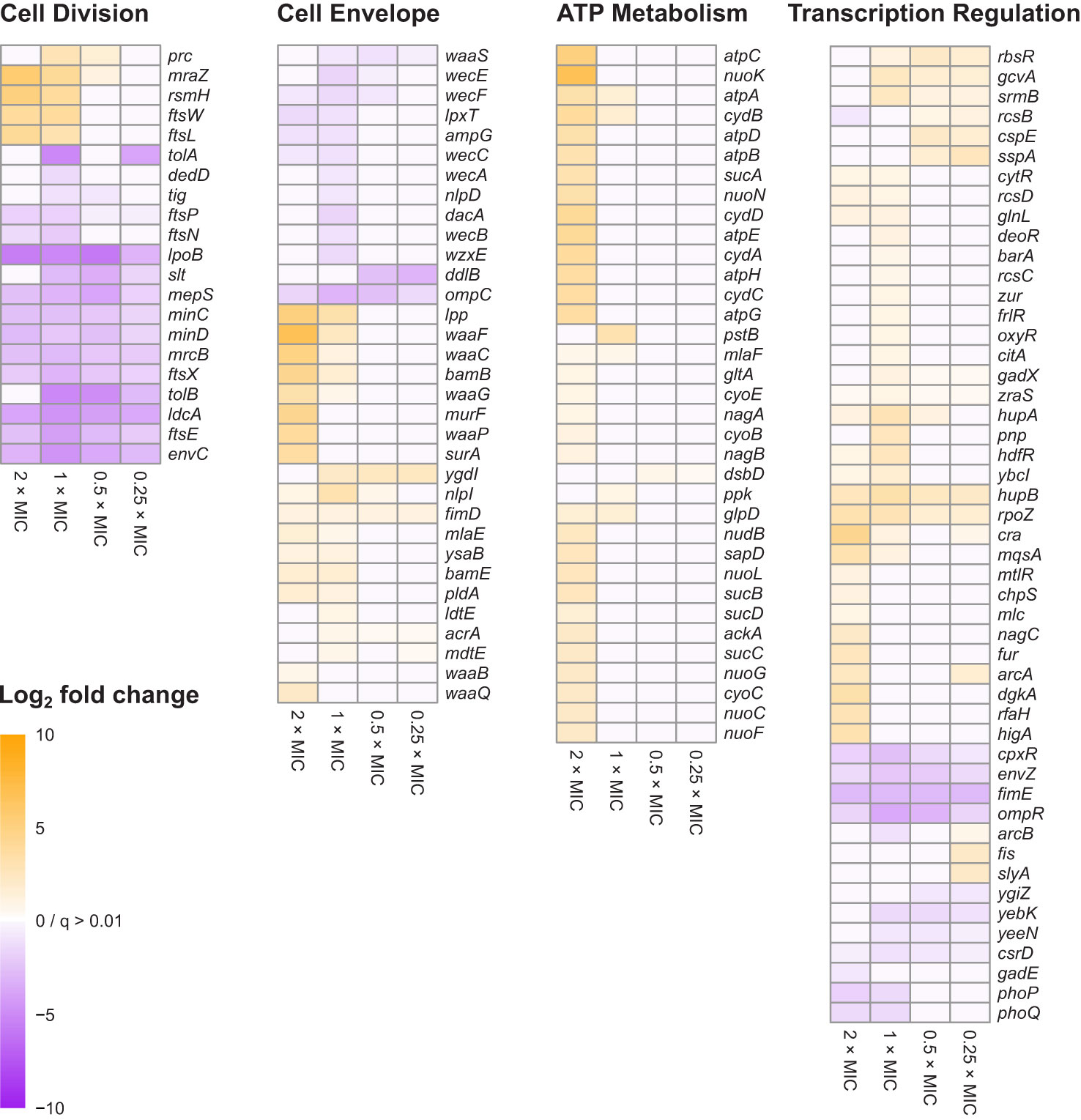
Figure 3 Heat map representations of log2FC for 138 genes belonging to the four principal gene functions involved in E. coli tolerance of meropenem stress. Principal gene functions were identified by Gene Functional Classification, as shown in Figure 2. Manual categorization of the 296 most significant genes (q<0.0001; log2CPM>3) then both expanded and refined the lists of genes belonging to each function. Cells are only colored when q<0.01 for the corresponding log2FC value. Genes in each heat map are arranged according to hierarchical clustering by Euclidian distance of log2FC, although for simplicity trees are not shown.
Cell envelope biogenesis is represented by group 2, containing genes responsible for synthesis of the oligosaccharide core region of the lipopolysaccharide layer (waaQGB and waaFC, from two operons) and group 7, containing genes for lipoproteins with functions involved in the synthesis and structure of the outer membrane and peptidoglycan (murein) sacculus. ATP metabolism-related genes fell into three groups containing the atpBEHAGDC genes (group 1) for components of ATP synthase, the nuoCFGKLN genes (group 5) encoding components of respiratory complex I (NADH:ubiquinone oxidoreductase), and a cluster of 6 genes that all encode ATP-binding proteins (group 8). Meanwhile, three groups include genes that are involved in regulation of gene expression. Groups 3 and 4 may be considered as a pair because they predominantly include members of two-component regulatory systems, with one containing the transcriptional regulators arcA, ompR, phoP and rcsB, and the other containing their respective cognate signal transducers arcB, envZ, phoQ and rcsCD. Group 6 contains a broad range of transcription regulators; primarily transcriptional repressors, for which knockouts were beneficial to survival with meropenem, but also gadE, phoP, yebK and yeeN, for which knockouts were detrimental. The remaining cluster (group 9) contains 45 over-represented genes, mainly belonging to a mixture of the gene functions described above.
Manual inspection of the 187 unclustered genes identified other examples of genes belonging to these functional groups. Also apparent upon close inspection is that many of the most significant genes encode proteins that are involved in cell division. Gene Functional Classification did not identify a separate cluster for cell division because it categorizes genes according to the structural or enzymatic roles of their products, rather than the overall cell process. For example, ftsE, whose product powers the constriction of the mitotic septal ring (Arends et al., 2009), was clustered with other ATP-binding proteins, while the ftsX gene encoding the FtsE binding partner, is in the heterogeneous cluster of 45 over-represented genes together with ftsL, ftsN and ftsW. Genes for important peptidoglycan-modifying enzymes with key functions in cell division also fall into this category, including the cell division-specific penicillin binding protein 1b (mrcB) and prc, whose product cleaves the progenitor of penicillin binding protein 3 (FtsI) to form a mature protein that interacts with MrcB, FtsW and FtsZ. Therefore, cell division is a fourth important gene function in the response of E. coli to meropenem. Figure 3 shows the log2FC for 138 genes belonging to the four principal gene functions following manual categorization of the 296 most significant genes.
Cell division machinery is critical to meropenem tolerance
The AlbaTraDIS workflow identified multiple members of the dcw gene cluster as important for growth during meropenem exposure. The dcw cluster is a transcriptional unit of 16 genes involved in cell division, which contains multiple transcriptional promoters but no terminator and thus may function as an operon (Vicente et al., 1998). The AlbaTraDIS results suggested that knockout insertions in some of these genes are beneficial under meropenem stress (Figure 3). However, inspection of the transposon insertion pattern within the dcw cluster revealed that the genes are essential (Goodall et al., 2018) and that transposon insertions were confined to specific regions towards the beginning and end of some genes and in intergenic spaces. Crucially, Ptac was always oriented in the same direction as the genes (Figure 4) showing that rather than knockout insertions, it is increased transcription of the dcw gene cluster which aids survival in the presence of meropenem. Furthermore, insertions in the same orientation before and within the dispensable genes mraZ and rsmH were highly beneficial because they are located at the start of the operon and therefore drive transcription of the whole operon.
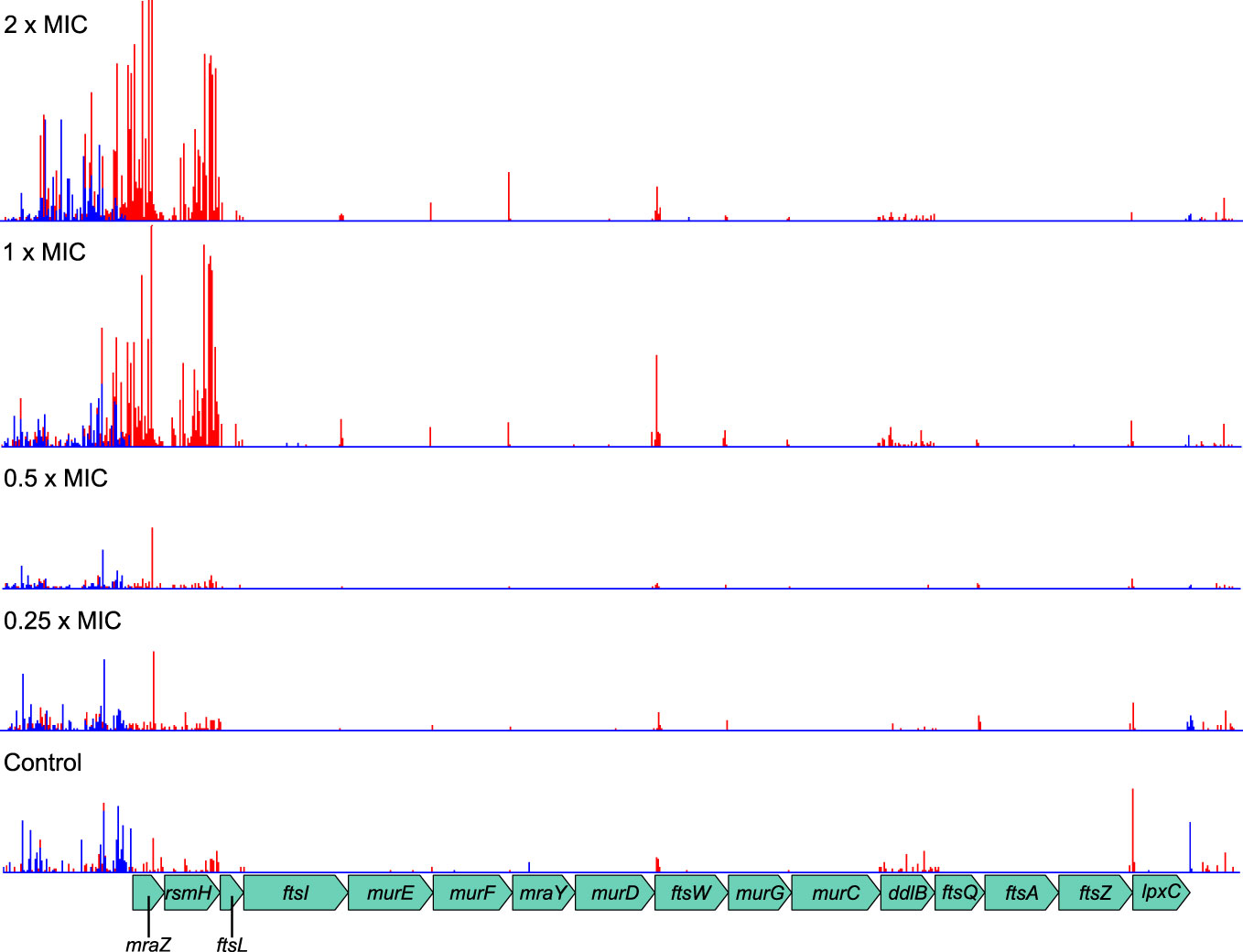
Figure 4 Transposon insertion pattern for the dcw gene cluster. Most genes in the cluster are essential and so transposon insertions within their coding sequences are not tolerated. However, insertions resulting in promotion of transcription from Ptac are beneficial to survival of meropenem treatment. Top to bottom: 2×MIC, 1×MIC, 0.5×MIC, 0.25×MIC, control. The red histogram indicates transposon insertions in which Ptac is oriented to transcribe from left to right, while the blue histogram indicates transposon insertions oriented to transcribe from right to left. For clarity, only one repeat of each concentration is displayed. Insertion patterns between repeats were highly similar.
The benefits of increased transcription were dependent on meropenem concentration, becoming particularly significant above the MIC. This is probably because the native levels of gene expression can cope with meropenem concentrations below the MIC, while increased levels are only required at or above the MIC. This result also illustrates the power of TraDIS-Xpress to reveal such detailed gene regulation effects as it is the only procedure able to assay transcription in this manner.
In contrast to the dcw cluster, knockout insertions in other genes central to cell division, such as ftsE/X and minC/D were detrimental to survival at all meropenem concentrations. Cell division is a highly dynamic process involving considerable re-modelling of the cell wall, and enzymes that are key to the recycling of peptidoglycan during this process, such as ldcA and slt were also important at all concentrations, as was envC, which encodes a factor that interacts with FtsEX and activates the peptidoglycan amidases AmiA and AmiB to enable septal splitting (Priyadarshini et al., 2007; Uehara et al., 2010; Cook et al., 2020). It is also notable that a larger proportion of the significant genes for cell division were important below the MIC of meropenem than for any of the other principal gene functions (Figure 3). Overall, this points to a critical role for the cell division machinery in the response to meropenem-induced stress.
Dynamic remodeling of cell envelope is involved in meropenem tolerance
For cell envelope synthesis and remodeling genes that are not directly involved in cell division, there was an almost even split between genes for which knockout insertions reduced susceptibility to meropenem (positive log2FC), and those for which susceptibility was increased (negative log2FC). Overall, inactivation of these genes had a smaller effect than for cell division-related genes and tended to be important only at or above the MIC (Figure 3). This suggests that peptidoglycan remodeling proteins of the divisome may play a more important role in E. coli tolerance of meropenem than functionally similar proteins involved in other processes such as elongation.
Penicillin-binding proteins were not prominent within the data. Considering their central role in β-lactam tolerance, this is surprising. However, very small decreases in survival could be observed by manual inspection of knockout insertion sites for mrcA (PBP1a), pbpC (PBP1c) and dacB (PBP4), while mrdA (PBP2) and ftsI (PBP3) are essential genes and are therefore difficult to assay. dacA (PBP5) showed a significant negative log2FC at 1×MIC only. It is possible that the ability of meropenem to bind multiple PBPs means that inactivation of individual PBP genes have relatively small effects. The only PBP gene to show a significant log2FC at all meropenem concentrations was mrcB (PBP1b). As PBP1b provides transglycosylase and transpeptidase activity for the core enzyme complex of the divisome (Leclercq et al., 2017), this lends some support to the assertion that cell division-related genes have particular importance in meropenem tolerance.
In addition to peptidoglycan metabolism, the lipopolysaccharide layer—particularly the waa and wec gene clusters—was also important for meropenem tolerance (Figure 3). Products of waa genes catalyze the synthesis of the lipopolysaccharide core region, while wec gene products synthesize the enterobacterial common antigen, which attaches to the lipopolysaccharide (Rinno et al., 1980). The insertion site plots for the waa genes show that knockout insertions in hldD/waaFC in one operon, and in waaQGP in a second operon, are positively selected under high meropenem concentrations (Figure 5). Transposon insertions, many of which are expected to disrupt gene function, were tolerated throughout these two operons in our data, indicating that these genes are not essential (Figure 5). On the other hand, transposon insertions in waaA (in a third operon) were not detected because waaA is essential (Gerdes et al., 2003; Goodall et al., 2018) as it encodes 3-deoxy-D-manno-octulosonic acid transferase, which is fundamental to the production of lipid A (Belunis and Raetz, 1992; Klein et al., 2009). Therefore, it might be the case that interrupting certain genes leads to specific truncations of the lipopolysaccharide that facilitate survival at high meropenem concentrations (Joloba et al., 2004; Nakao et al., 2012), while complete loss of either the LPS or ECA is detrimental.
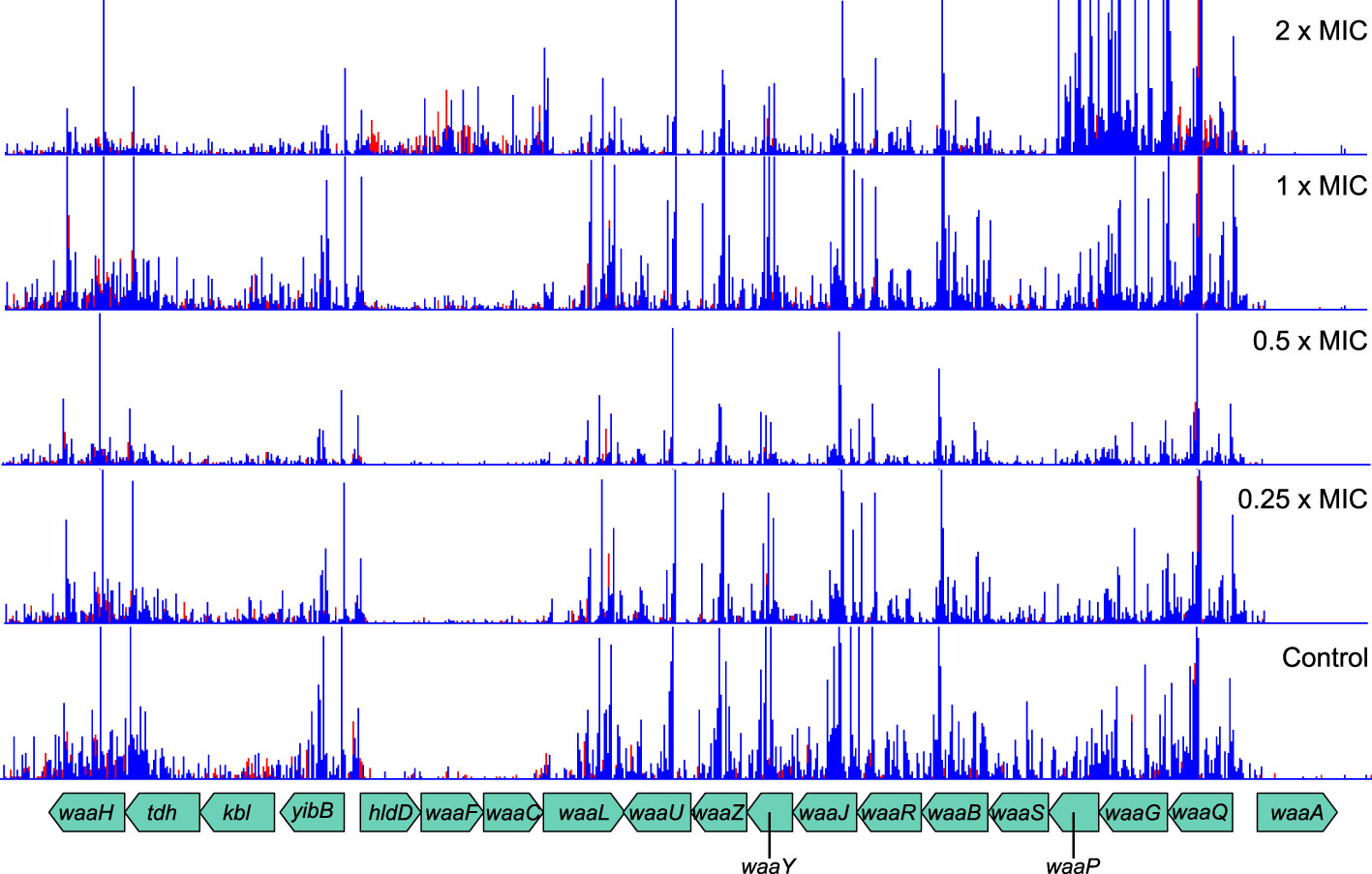
Figure 5 Transposon insertion site plots for the genomic region encoding the waa genes. While insertions in the essential waaA are not tolerated, insertions in other genes are selected for at high meropenem concentrations. Top to bottom: 2×MIC, 1×MIC, 0.5×MIC, 0.25×MIC, control. The red histogram indicates transposon insertions in which Ptac is oriented to transcribe from left to right, while the blue histogram indicates transposon insertions oriented to transcribe from right to left. For clarity, only one repeat of each concentration is displayed. Insertion patterns between repeats were highly similar.
One gene for which knockout insertions were surprisingly beneficial was lpp, which encodes a major peptidoglycan lipoprotein. Lpp forms a covalent link between the outer membrane and the peptidoglycan layer and has been estimated to account for up to 40% of peptidoglycan by mass (Braun and Rehn, 1969). Knockout insertions appeared to favor locations towards the start or center of the gene, suggesting that truncation rather than complete inactivation may be important for this effect (Figure 6). Loss of the cell wall and spheroplast formation is a strategy for β-lactam tolerance employed by Pseudomonas aeruginosa (Monahan et al., 2014) and Lpp regulates the mechanical properties of the cell envelope (Mathelié-Guinlet et al., 2020) so this observation might indicate a similar mechanism in E. coli.
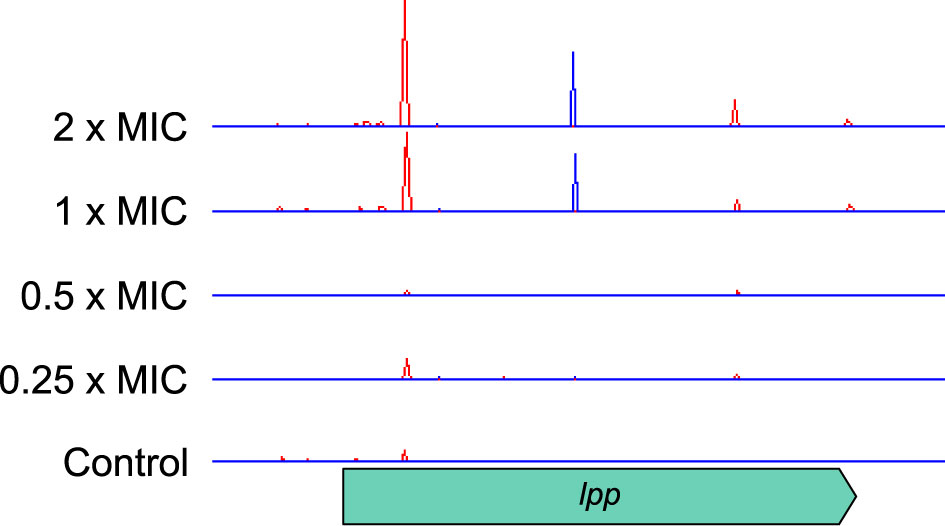
Figure 6 Transposon insertion site plots for the lpp gene and its flanking genes. Insertions are positively selected at high meropenem concentrations, with two locations, towards the start and centre of the gene, particularly favoured. Top to bottom: 2×MIC, 1×MIC, 0.5×MIC, 0.25×MIC, control. The red histogram indicates transposon insertions in which Ptac is oriented to transcribe from left to right, while the blue histogram indicates transposon insertions oriented to transcribe from right to left. For clarity, only one repeat of each concentration is displayed. Insertion patterns between repeats were highly similar.
The dose response of cell envelope genes was important, as they showed significant log2FC at the highest concentrations of meropenem (2×MIC and 1×MIC) but were not selected by exposures below the MIC. For example, lpp had log2FC values of 5.02 and 3.34 at 2×MIC and 1×MIC, respectively, but no significant change below the MIC. This might indicate a reduction in cell growth and division, and thus a reduced requirement for peptidoglycan and outer membrane synthesis at high meropenem concentrations. Alternatively, disruption of genes for preferred pathways might force cells to utilize alternative cell wall and membrane synthesis pathways that are less susceptible to meropenem.
Maintenance of proton motive force aids survival with meropenem
The atpBEHAGDC and nuoCFGKLN genes identified by Gene Functional Classification operate on opposite sides of the ATP generation cycle. NADH:ubiquinone oxidoreductase, encoded by the nuo genes is the first complex in the electron transfer chain of respiration and uses the free energy derived from oxidation of NADH to expel protons from the cell. The F0F1 proton-translocating ATPase, encoded by the atp genes later harnesses the proton gradient thus accumulated to drive the production of ATP while allowing protons back into the cell. Although both complexes had significantly increased transposon insertions, again the ability to assay transcriptional effects on the genes is illuminating.
The atp operon tolerates insertions within genes in both directions and shows no effects of transcription from Ptac (Figure 7A). The log2FC is proportionally large, especially at higher meropenem concentrations, but this is partly because even the control sample has low absolute numbers of insertions. This probably arises as a balance is struck between maintaining the activity of ATP synthase for efficient energy generation and the beneficial effect of reducing that activity in the presence of meropenem.
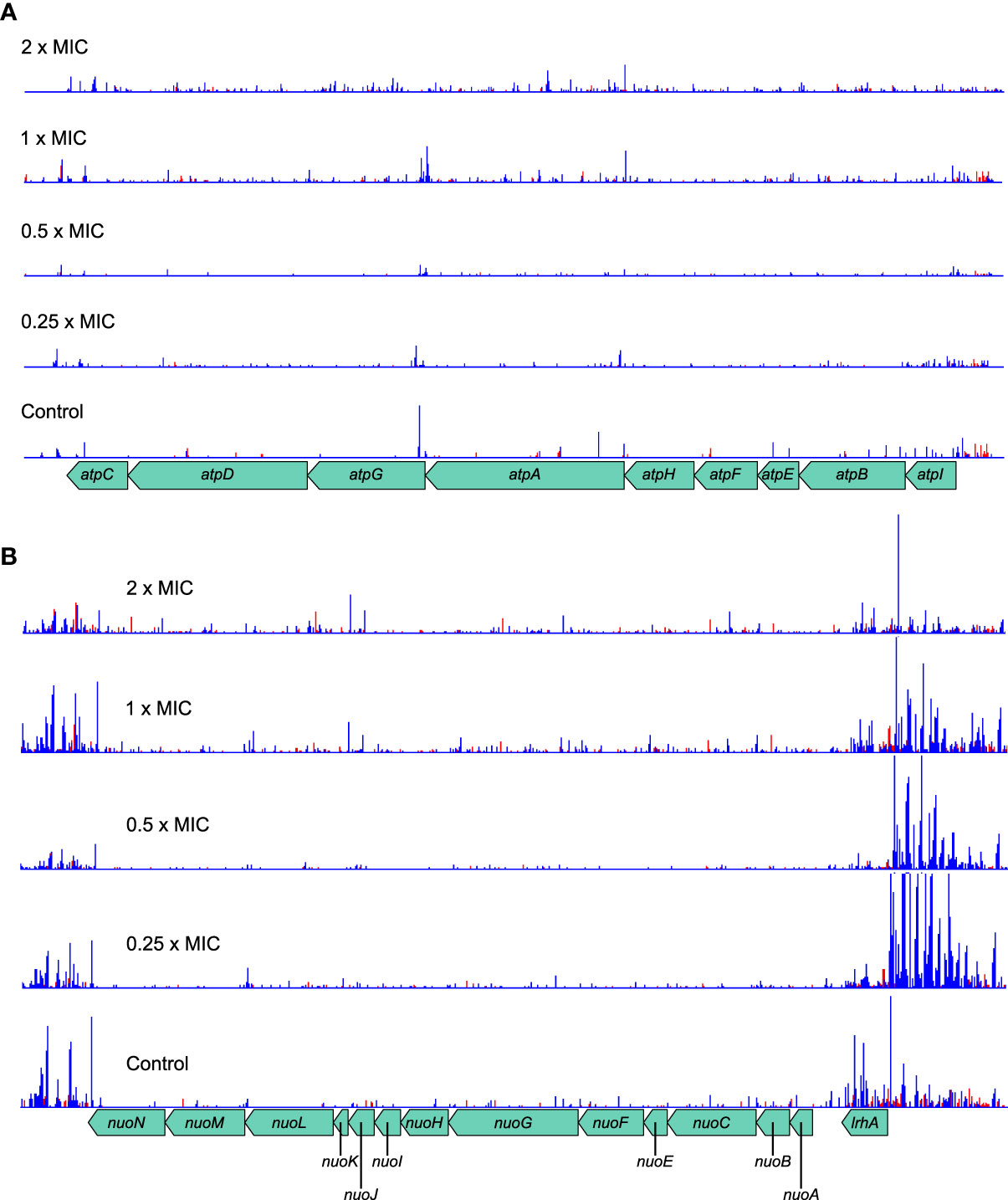
Figure 7 Transposon insertion patterns for the atp and nuo operons. (A) The atp operon tolerates transposon insertions in both orientations, which confer a selective advantage at high meropenem concentrations. Although the absolute number of insertions is low, the relative change at high concentrations is large. The overall effect is expected to decrease ATP synthesis activity of the F0F1 ATP synthase. (B) The nuo operon also tolerates insertions within the genes. However, insertions upstream of the operon, with Ptac oriented so as to transcribe the nuo genes, are highly favored in the presence of meropenem. The overall effect is expected to be increased activity of NADH:ubiquinone oxidoreductase, thus promoting the maintenance of the proton motive force. Top to bottom: 2×MIC, 1×MIC, 0.5×MIC, 0.25×MIC, control. The red histogram indicates transposon insertions in which Ptac is oriented to transcribe from left to right, while the blue histogram indicates transposon insertions oriented to transcribe from right to left. For clarity, only one repeat of each concentration is displayed. Insertion patterns between repeats were highly similar.
The nuo operon shows a similar pattern of knockout insertions, with a slight preference for insertions at higher concentrations (Figure 7B). However, the region immediately upstream of the operon, containing lrhA and alaA is highly enriched in insertions oriented towards the nuo operon and therefore favoring its increased transcription. These insertions are particularly numerous at low concentrations, suggesting that NADH:ubiquinone oxidoreductase activity is more beneficial below the MIC for meropenem. Taken together, this suggests the greatest net benefit for meropenem tolerance is obtained by increasing nuo gene expression and minimizing atp gene expression. This seems most likely to have arisen as the result of a benefit gained through maintenance of the proton motive force as it would maintain proton export but minimize their import, thereby shifting the balance of metabolic flux away from ATP metabolism.
This hypothesis is supported by the identification of Gene Functional Classification group 3 containing ATP-binding proteins, for which knockout insertions confer increased tolerance of meropenem (Figure 2). Cells that minimize non-essential energy consumption through these proteins might reduce demand for ATP, thus preserving the proton motive force. This may also facilitate slowing cell growth to reduce the dependence on peptidoglycan synthesis and remodeling, and prevent extra stress being imposed upon the cell by reactive oxygen species that are generated during oxidative phosphorylation.
Global changes in gene regulation
AlbaTraDIS identified a wide range of transcriptional regulators and related genes, which exhibited varying transposon insertion patterns. As identified by Gene Functional Classification, an important class of transcription-regulating genes were those belonging to two-component signal transduction systems (Figure 2 and Supplementary Table 2). Four transducer-regulator pairs were identified, while the transcriptional regulator cpxR and the signal transducers barA, citA, glnL and zraS were identified without their partners. Interestingly, knockout insertions in the arcA/B and rcsB/CD genes were beneficial to survival with meropenem (positive log2FC) while knockout insertions in the ompR/envZ and phoP/Q systems were detrimental (negative log2FC). The ompR/envZ and cpxR genes had significant log2FC values at all concentrations, suggesting that they might play more fundamental roles in meropenem tolerance than the other systems. Both systems are involved in the response to cell envelope damage.
Global transcriptional regulators were also identified, including fis [regulation of up to 21% of genes (Cho et al., 2008)], zur (Zn-binding transcriptional repressor) and cra (repressor-activator for carbon metabolism). However, the effects of these knockout insertions were only significant at specific meropenem concentrations. Therefore, large-scale transcriptional regulation of multiple systems may provide some benefit by, for example, reducing cell growth rate, but is unlikely to be a key factor in meropenem tolerance. Instead, more targeted responses such as those mediated by the two-component systems identified above are more likely to be important.
Influence of the stringent response on meropenem tolerance
Separate from transcriptional control of gene expression, the E. coli stringent response increases survival under a range of starvation conditions. Increased intracellular concentrations of (p)ppGpp inhibit rRNA and tRNA production, thus attenuating the rates of transcription, translation, and cell growth (Srivatsan and Wang, 2008). The key genes involved in regulating (p)ppGpp levels are relA, whose product produces ppGpp from GDP and ATP, and spoT, whose product can produce (p)ppGpp but also recycles it back to GTP or GDP. In this experiment, spoT knockout mutants showed increased tolerance to high concentrations of meropenem, suggesting a role for the stringent response in antibiotic tolerance. relA knockout insertions had no significant effect; however, 5′ transcription-enhancing insertions in the upstream rlmD gene also increased tolerance to high concentrations of meropenem. Therefore, increased levels of relA and decreased levels of spoT are beneficial to survival with meropenem. Furthermore, knockout insertions in rpoS, which mediates the general stress response and is enhanced by high (p)ppGpp levels (Lelong et al., 2007; Battesti et al., 2011), were also detrimental at high meropenem concentrations.
Transcriptional interference of tRNA genes
In an expedient demonstration of the unique capabilities of TraDIS-Xpress both to assay essential genes and to determine transcriptional effects, we identified that RNA interference of six aminoacyl-tRNA synthetase genes was beneficial to survival at high concentrations of meropenem (Table 1). Although knockout insertions were not tolerated, transposon insertions downstream of the genes and oriented such that Ptac transcribed the gene in reverse were selected for (Figure 8). This indicates that RNA interference caused by transcription from the lac promoter decreased expression of these genes and led to a fitness benefit. The effect of such insertions was large (1.9 – 11.0 log2FC), but only at or above the MIC. Interestingly, the stringent response acts partly through suppressing tRNA synthesis, so decreasing tRNA-related gene transcription in this way might have similar effects in a non-(p)ppGpp-dependent manner. Disruption of the C-terminus of MetG by insertion of a transposon increases persister cell formation by 10,000× compared to the wild-type (Girgis et al., 2012).
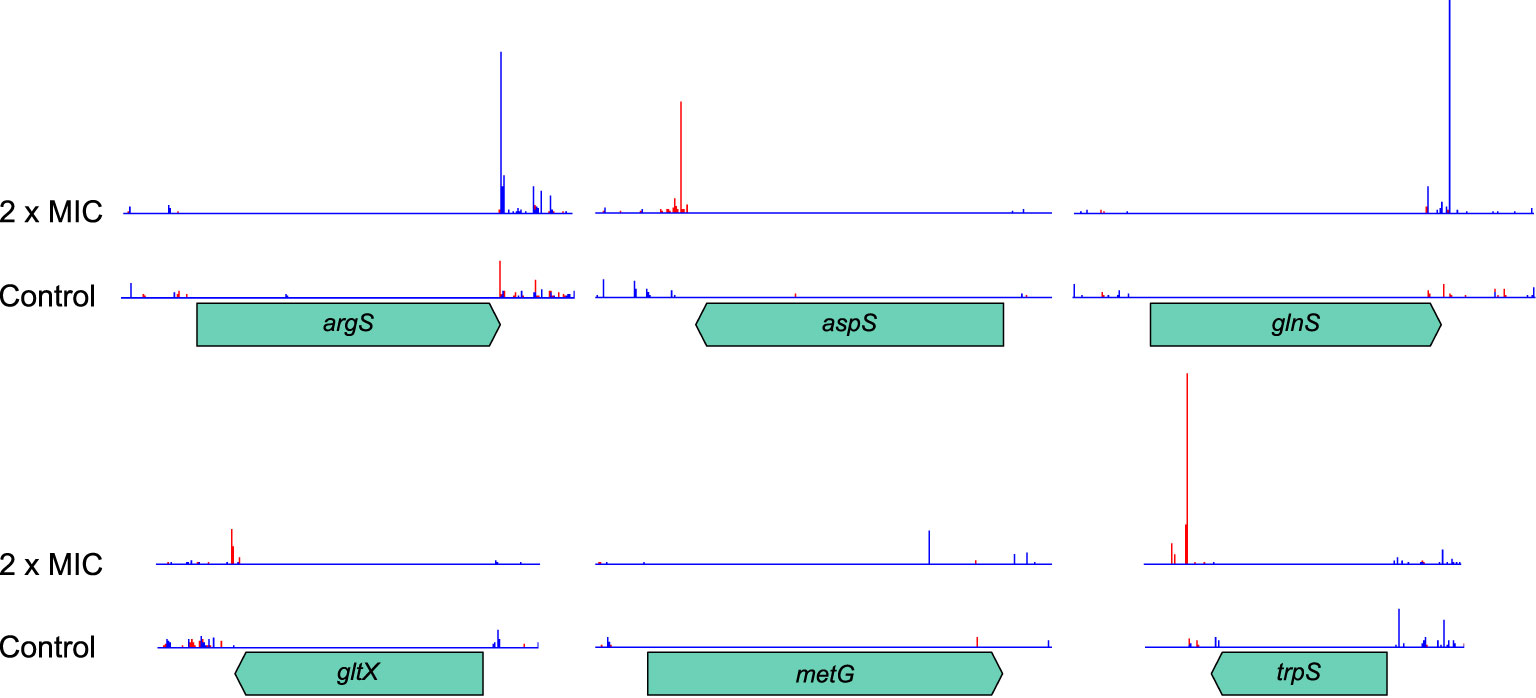
Figure 8 Transposon insertion site plots for the six tRNA synthetase genes with significant transcriptional interference. Insertions are not tolerated within the coding region of any of the genes. However, downstream insertions oriented such that transcription from Ptac proceeds in the opposite direction to the synthetase gene are selected for at high meropenem concentrations. The red histogram indicates transposon insertions in which Ptac is oriented to transcribe from left to right, while the blue histogram indicates transposon insertions oriented to transcribe from right to left. For clarity, histograms are only shown for the control and 2 × MIC samples.
Identification of potential gene targets for meropenem-synergizing drugs
Identifying the full range of genes contributing to meropenem susceptibility allows a rational approach to predicting antibiotic combinations which act additively or synergistically. Genes for which knockout insertions cause the most significant decreases in log2FC should be the best targets for drugs that will provide a synergistic effect when administered in combination with meropenem (Table 2). The gene with the largest such effect was lpoB, encoding an outer membrane lipoprotein that binds to and stimulates penicillin binding protein 1b (mrcB; also identified by this experiment as a potential drug target). The next largest effects were seen for tolA and tolB, both of which encode members of the Tol-Pal system, with roles in outer membrane invagination and peptidoglycan processing during cell division. However, the effects were not significant at all meropenem concentrations, raising doubts about their suitability as drug targets. Knockout insertions in genes for other peptidoglycan-processing proteins such as ldcA, envC, mepS and slt did show significantly increased susceptibility at all concentrations and so are more promising targets (together with the amidases amiA and amiB that EnvC activates, although these genes were not identified as significant in this experiment).
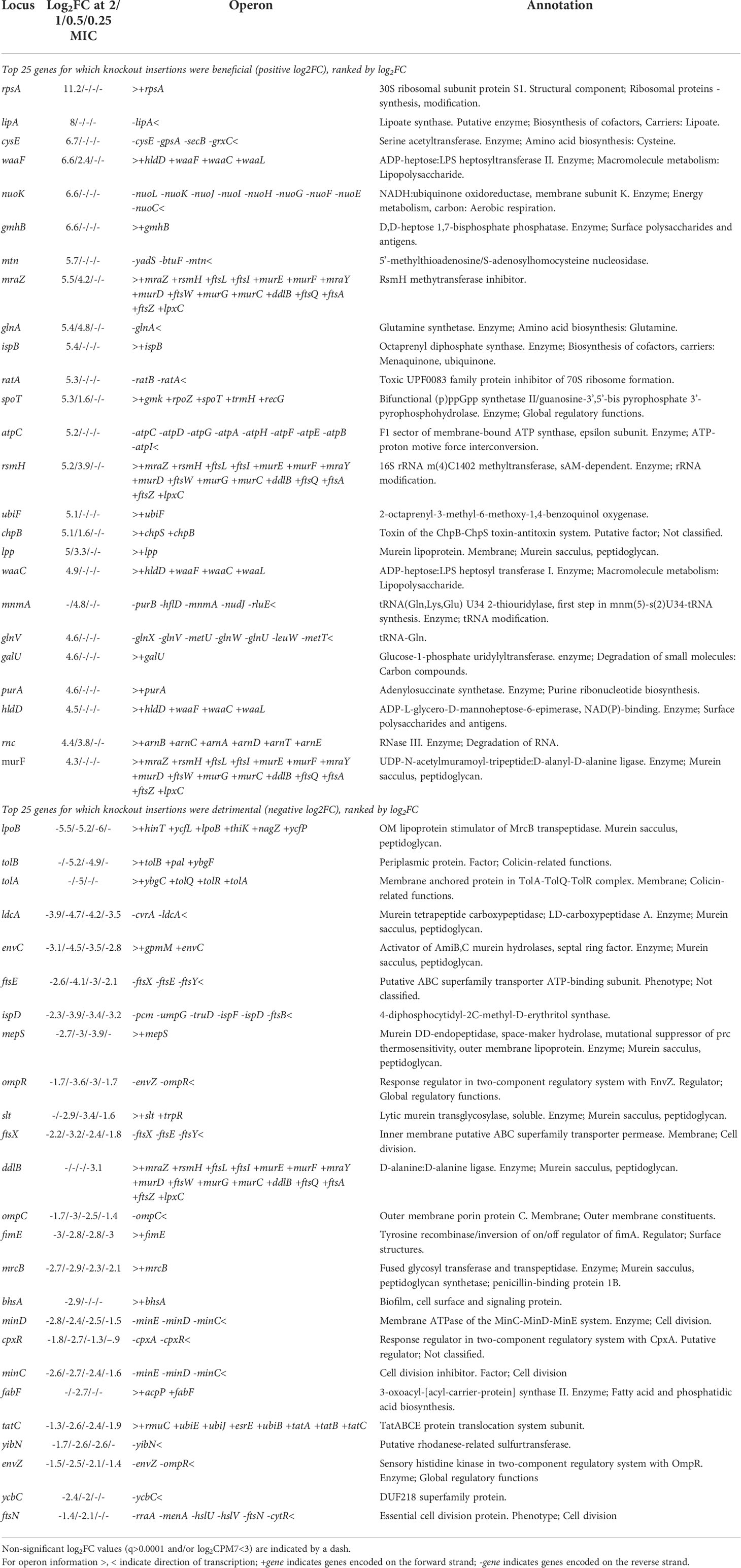
Table 2 Genes with the largest log2FC in knockout insertions (positive and negative presented separately) selected by meropenem treatment.
The cephalosporin β-lactam cefsulodin specifically inhibits PBP1a and PBP1b (Joseleau-Petit et al., 2007; Sarkar et al., 2012), so we tested for a synergistic relationship between cefsulodin and meropenem. The fractional inhibitory concentration index (FICI) is a measure of whether antibiotic combinations act synergistically (FICI<0.5), additively (FICI = 0.5 – 1), or do not interact (FICI>1) (Hall et al., 1983). The relative MICs for meropenem and cefsulodin were determined as 3.2 and 16 mg/L respectively. However, with cefsulodin at 8 mg/L, in one additive/synergy measurement the MIC for meropenem was 0.2 mg/L (FICI = 0.56) and in the other 0.1 mg/L (FICI = 0.53), indicating an additive effect. This result supports the identification of lpoB and mrcB as important for tolerance of meropenem and exemplifies the sensitivity of TraDIS-Xpress to predict relatively weak additive activity.
Knockout insertions in genes for both members of the FtsEX complex also increased susceptibility at all meropenem concentrations, as did insertions in minC and minD of the MinCDE complex. This experiment has highlighted the importance of cell division to meropenem tolerance, so targeting the machinery involved is a rational strategy for increasing the efficacy of β-lactams. Therefore, these two complexes are attractive targets that if inactivated will have detrimental effects at all downstream stages of septum formation and cell division.
This experiment has also highlighted the possibility of two-component signal transduction systems being important to β-lactam tolerance. Based on log2FC, the EnvZ/OmpR (osmotic stress response) and CpxRA (generalized membrane stress response) systems are the most promising targets. Intriguingly, these two systems are thought to be linked by the protein MzrA (Gerken et al., 2009). Another novel target that was revealed by the measurement of RNA interference effects is RNA gene expression, whether through directly inhibiting transcription of rRNA and tRNA genes or through inhibiting the stringent response by deactivating relA.
Discussion
By employing TraDIS-Xpress to study the mechanisms involved in E. coli tolerance of meropenem, we have identified an extraordinary number of genes involved in susceptibility. Even by concentrating only on the most significant hits, we still identified 296 knockout insertions, 52 insertions with 5′ transcription-promoting activity and 48 insertions with 3′ transcription-inhibiting activity. This strongly suggests that the mechanism of action of meropenem reaches far beyond the canonical inhibition of peptidoglycan crosslinking by targeting penicillin binding proteins. It also indicates that there are multiple mechanisms by which resistance to meropenem could develop and raises the possibility that multiple subpopulations of cells arise upon meropenem exposure, in which different tolerance strategies are employed.
We identified four gene function categories of principal importance for meropenem tolerance. Unsurprisingly, cell envelope (particularly peptidoglycan) biogenesis was among these functions. However, the high degree of intersection with components of the divisome emphasizes the importance of cell division for meropenem efficacy. Furthermore, the fact that cell division genes showed highly significant log2FC at all meropenem concentrations indicates that peptidoglycan remodeling during cell division is the primary target of meropenem. Some transcription regulators were also important at all meropenem concentrations, reflecting a broad, generalized response to cell envelope perturbations caused by meropenem. However, ATP metabolism was only a significant function at higher meropenem concentrations.
A β-lactam-induced futile cycle of peptidoglycan synthesis and degradation has been proposed by Cho et al. (Cho et al., 2014). For the mecillinam-induced toxicity that they described, Slt (a lytic transglycosylase encoded by slt) was responsible for the turnover of nascent peptidoglycan. However, they did not identify the non-Slt protein(s) responsible for turnover upon cefsulodin treatment. In the present study, an alternative lytic transglycosylase, LdcA, and the amidase-activating EnvC were identified together with Slt. Mecillinam specifically targets PBP2, while meropenem has multiple targets (Kocaoglu and Carlson, 2015). Therefore, it is likely that a greater variety of uncrosslinked peptidoglycans are generated by meropenem and other β-lactams with multiple targets, necessitating the action of Slt, LdcA and one or both of AmiA and AmiB for their breakdown.
A recent report found that the mecillinam-induced peptidoglycan futile cycle leads to wide-scale downstream effects: futile cycling increases energy demand, causing an imbalance in central carbon metabolism, which generates reactive oxygen species and leads to disruption of the redox environment of the cell. This damages macromolecules such as DNA and proteins, leading to increased protein synthesis, which further increases energy demand. The eventual depletion of ATP and accumulated cell damage leads to death (Lobritz et al., 2022). Although so far only demonstrated for mecillinam, this runaway process of cell damage intriguingly implicates our other two principal gene functions of ATP metabolism and transcription regulation in a network of far-reaching downstream effects from β-lactam-induced cell envelope stress. Our results point to NADH:ubiquinone oxidoreductase and ATP synthase as the primary regulators of the metabolic response to peptidoglycan futile cycling. We observed that 5′ transcription-promoting insertions that increase expression of nuo genes increase survival, while for atp genes, knockout insertions are beneficial. A combination of these effects may be conducive to maintenance of the redox environment. However, our data point towards the importance of maintaining the membrane potential for meropenem tolerance; a consideration that was not directly addressed in (Lobritz et al., 2022).
Previous reports have also discussed the transcriptional response to β-lactams (Srivatsan and Wang, 2008; Battesti et al., 2011; Møller et al., 2016; Gray and Wenzel, 2020), including the roles played by the cpxRA (Guest et al., 2017) and envZ/ompR (Adler et al., 2016) two-factor systems. Clearly the intersecting relationships between β-lactam stress and all the mechanisms discussed here are extremely complex and will also be influenced by environmental and experimental conditions. For example, Guest et al. (Guest et al., 2017) found that CpxR inhibits nuo gene expression in response to envelope stress (although they did not test β-lactam antibiotic stress), while our results suggested that although the CpxRA system is involved in meropenem tolerance, increased expression can be beneficial under some conditions.
Recent work has demonstrated inter-linked effects on β-lactam tolerance of the membrane potential (Kaneti et al., 2016; Wang et al., 2021), ATP production (Shetty and Dick, 2018; Lindman and Dick, 2019), sugar metabolism (Thorsing et al., 2015), and the stringent response (Rodionov et al., 1995; Lai et al., 2017) in E. coli and other species. The membrane potential is also required for cell division (Strahl and Hamoen, 2010; Chimerel et al., 2012) and persister cell viability (Radzikowski et al., 2016; Manuse et al., 2021; Wang et al., 2021). We identified genetic components of all these processes as important contributors towards the response to meropenem stress. Often, we only observed significant selective pressures on these processes at or above the MIC, which provides compelling evidence that tolerant subpopulations of cells arose during the experiment.
Based on their significance at low meropenem concentrations, we identified proteins involved in peptidoglycan remodeling during cell division as potential targets for meropenem-synergizing drugs. We tested one such prediction, which revealed an additive effect of cefsulodin and meropenem due to inhibition of mrcB. Other previously reported inhibitors of proteins involved in cell division include bulgecin A, which inhibits slt (Templin et al., 1992) and a dithiazoline inhibitor of ldcA (Baum et al., 2005). As deletion of slt renders E. coli hypersensitive to mecillinam (Templin et al., 1992; Bendezú and De Boer, 2008), in agreement with the data presented here for meropenem, our data suggest a similar effect for knockouts of ldcA, mepS and amidases, which have similar functions.
Our data also suggested some new potential drug targets. For example, knockouts in FtsZ-associated divisome machinery such as ftsEX and minCD potentiate the effects of meropenem, even when peptidoglycan-recycling proteins are expressed at wild-type levels. We are not aware of specific inhibitors for these proteins, but they appear to be attractive targets for antibacterial drug discovery. The F0F1 ATP synthase and NADH:ubiquinone oxidoreductase might also be useful targets for drugs to modulate their expression or activity in such a way as to synergize with the effects of β-lactam antibiotics.
Limitations of the study
The uniquely high-throughput approach of TraDIS-Xpress simultaneously assays upregulation, downregulation, and knockouts of every gene in the genome and is an extremely powerful way to identify multiple important mechanisms. However, the parallel nature of the assay does lead to convolution of results from different mechanisms and different populations of cells. This experiment provided evidence of tolerance arising through loss of the cell wall (waa and wec operons; lpp), modulation of metabolism and the membrane potential (atp and nuo operons) reduced growth rate (cell division machinery) and the stringent response (spoT/relA; tRNA genes). It has long been established that non-growing cells are intrinsically more resistant to β-lactams than when actively growing (Tuomanen, 1986; Lee et al., 2018), although growth reduction is not necessary for tolerance (Urbaniec et al., 2022). However, we cannot deduce how many separate subpopulations of tolerant cells emerged or which mechanisms gave rise to each. We also cannot determine the proportion of tolerant but (slowly) growing cells compared to persisters. Furthermore, TraDIS-Xpress measures a change in relative abundance of transposon insertion mutants. Therefore, it is challenging to distinguish between insertions that result in cells that can outgrow others in the population (an absolute increase in cell numbers) and those that simply “out-survive” others (e.g., by growing more slowly or becoming persisters: a relative increase in cell numbers).
Conclusions
We have used TraDIS-Xpress to identify many genes that are important for E. coli survival of meropenem stress and found that four principal gene functions of cell envelope biogenesis, cell division, ATP metabolism, and transcription regulation encompass the most significant and important responses. Some identified genes are involved in well-established mechanisms, validating the use of TraDIS-Xpress, while others are involved in downstream damage-limitation responses and support recent advances in our understanding of the systemic effects of β-lactams. We were also able to add to the list of genes known to be involved in meropenem susceptibility and tolerance, and to predict drug targets to potentiate the effects of meropenem. Similar experiments using different β-lactam antibiotics will enable comparisons to be made, to better understand the mode of action of each drug and to identify common and/or unique targets between drugs. Our data strongly suggest that multiple routes to meropenem tolerance are important. Therefore, identification and further characterization of the individual pathways leading to meropenem tolerance would be illuminating.
Data availability statement
The nucleotide sequencing data for this study can be found in the Array Express repository under accession number E-MTAB-11802.
Author contributions
NT performed data analysis, produced figures, and wrote the manuscript; AT and MY designed and performed the experiments and curated the data; SB and ML helped to curate the data and performed computational analyses; MW and IC helped design experiments and provided managerial oversight. All authors contributed to the article and approved the submitted version.
Funding
This work was funded by the Biotechnology and Biological Sciences Research Council Institute Strategic Programme Microbes in the Food Chain BB/R012504/1 and its constituent project BBS/E/F/000PR10349.
Conflict of interest
The authors declare that the research was conducted in the absence of any commercial or financial relationships that could be construed as a potential conflict of interest.
Publisher’s note
All claims expressed in this article are solely those of the authors and do not necessarily represent those of their affiliated organizations, or those of the publisher, the editors and the reviewers. Any product that may be evaluated in this article, or claim that may be made by its manufacturer, is not guaranteed or endorsed by the publisher.
Supplementary material
The Supplementary Material for this article can be found online at: https://www.frontiersin.org/articles/10.3389/frabi.2022.957942/full#supplementary-material
References
Adler M., Anjum M., Andersson D. I., Sandegren L. (2016). Combinations of mutations in envZ, ftsI, mrdA, acrB and acrR can cause high-level carbapenem resistance in escherichia coli. J. Antimicrob. Chemother. 71, 1188–1198. doi: 10.1093/jac/dkv475
Arends S. J. R., Kustusch R. J., Weiss D. S. (2009). ATP-binding site lesions in FtsE impair cell division. J. Bacteriol. 191, 3772–3784. doi: 10.1128/JB.00179-09
Balaban N. Q., Helaine S., Lewis K., Ackermann M., Aldridge B., Andersson D. I., et al. (2019). Definitions and guidelines for research on antibiotic persistence. Nat. Rev. Microbiol. 17, 441–448. doi: 10.1038/s41579-019-0196-3
Barquist L., Mayho M., Cummins C., Cain A. K., Boinett C. J., Page A. J., et al. (2016). The TraDIS toolkit: Sequencing and analysis for dense transposon mutant libraries. Bioinformatics 32, 1109–1111. doi: 10.1093/bioinformatics/btw022
Battesti A., Majdalani N., Gottesman S. (2011). The RpoS-mediated general stress response in escherichia coli. Annu. Rev. Microbiol. 65, 189–213. doi: 10.1146/annurev-micro-090110-102946
Baum E. Z., Crespo-Carbone S. M., Foleno B., Peng S., Hilliard J. J., Abbanat D., et al. (2005). Identification of a dithiazoline inhibitor of escherichia coli L,D-carboxypeptidase a. Antimicrob. Agents Chemother. 49, 4500–4507. doi: 10.1128/AAC.49.11.4500-4507.2005
Belunis C. J., Raetz C. R. H. (1992). Biosynthesis of endotoxins. purification and catalytic properties of 3- deoxy-d-manno-octulosonic acid transferase from escherichia coli. J. Biol. Chem. 267, 9988–9997. doi: 10.1016/s0021-9258(19)50189-2
Bendezú F. O., De Boer P. A. J. (2008). Conditional lethality, division defects, membrane involution, and endocytosis in mre and mrd shape mutants of escherichia coli. J. Bacteriol. 190, 1792–1811. doi: 10.1128/JB.01322-07
Braun V., Rehn K. (1969). Chemical characterization, spatial distribution and function of a lipoprotein (Murein-lipoprotein) of the e. coli cell wall: The specific effect of trypsin on the membrane structure. Eur. J. Biochem. 10, 426–438. doi: 10.1111/j.1432-1033.1969.tb00707.x
Carver T., Harris S. R., Berriman M., Parkhill J., McQuillan J. A. (2012). Artemis: An integrated platform for visualization and analysis of high-throughput sequence-based experimental data. Bioinformatics 28, 464–469. doi: 10.1093/bioinformatics/btr703
Chimerel C., Field C. M., Piñero-Fernandez S., Keyser U. F., Summers D. K. (2012). Indole prevents escherichia coli cell division by modulating membrane potential. Biochim. Biophys. Acta 1818, 1590–1594. doi: 10.1016/j.bbamem.2012.02.022
Cho B. K., Knight E. M., Barrett C. L., Palsson B. (2008). Genome-wide analysis of Fis binding in escherichia coli indicates a causative role for a-/AT-tracts. Genome Res. 18, 900–910. doi: 10.1101/gr.070276.107
Cho H., Uehara T., Bernhardt T. G. (2014). Beta-lactam antibiotics induce a lethal malfunctioning of the bacterial cell wall synthesis machinery. Cell 159, 1300–1311. doi: 10.1016/j.cell.2014.11.017
Church N. A., McKillip J. L. (2021). Antibiotic resistance crisis: challenges and imperatives. Biol. (Bratisl) 76, 1535–1550. doi: 10.1007/s11756-021-00697-x
Cook J., Baverstock T. C., McAndrew M. B. L., Stansfeld P. J., Roper D. I., Crow A. (2020). Insights into bacterial cell division from a structure of EnvC bound to the FtsX periplasmic domain. Proc. Natl. Acad. Sci. U. S. A. 117, 28355–28365. doi: 10.1073/pnas.2017134117
Davies T. A., Shang W., Bush K., Flamm R. K. (2008). Affinity of doripenem and comparators to penicillin-binding proteins in escherichia coli and pseudomonas aeruginosa. Antimicrob. Agents Chemother. 52, 1510–1512. doi: 10.1128/AAC.01529-07
Dörr T. (2021). Understanding tolerance to cell wall–active antibiotics. Ann. N. Y. Acad. Sci. 1496, 35–58. doi: 10.1111/nyas.14541
Drusano G. (1997). Meropenem: Laboratory and clinical data. Clin. Microbiol. Infect. 3, 4S51–4S59. doi: 10.1016/s1198-743x(14)65034-5
Fisher R. A., Gollan B., Helaine S. (2017). Persistent bacterial infections and persister cells. Nat. Rev. Microbiol. 15, 453–464. doi: 10.1038/nrmicro.2017.42
Gerdes S. Y., Scholle M. D., Campbell J. W., Balázsi G., Ravasz E., Daugherty M. D., et al. (2003). Experimental determination and system level analysis of essential genes in escherichia coli MG1655. J. Bacteriol. 185, 5673–5684. doi: 10.1128/JB.185.19.5673-5684.2003
Gerken H., Charlson E. S., Cicirelli E. M., Kenney L. J., Misra R. (2009). MzrA: A novel modulator of the EnvZ/OmpR two-component regulon. Mol. Microbiol. 72, 1408–1422. doi: 10.1111/j.1365-2958.2009.06728.x
Girgis H. S., Harris K., Tavazoie S. (2012). Large Mutational target size for rapid emergence of bacterial persistence. Proc. Natl. Acad. Sci. U. S. A. 109, 12740–12745. doi: 10.1073/pnas.1205124109
Goodall E. C. A., Robinson A., Johnston I. G., Jabbari S., Turner K. A., Cunningham A. F., et al. (2018). The essential genome of escherichia coli K-12. MBio 9, e02096–17. doi: 10.1128/mBio.02096-17
Gray D. A., Wenzel M. (2020). Multitarget approaches against multiresistant superbugs. ACS Infect. Dis. 6, 1346–1365. doi: 10.1021/acsinfecdis.0c00001
Guest R. L., Wang J., Wong J. L., Raivio T. L. (2017). A bacterial stress response regulates respiratory protein complexes to control envelope stress adaptation. J. Bacteriol. 199, 152–162. doi: 10.1128/JB.00153-17
Hall M. J., Middleton R. F., Westmacott D. (1983). The fractional inhibitory concentration (FIC) index as a measure of synergy. J. Antimicrob. Chemother. 11, 427–433. doi: 10.1093/jac/11.5.427
Huang D. W., Sherman B. T., Lempicki R. A. (2009a). Bioinformatics enrichment tools: Paths toward the comprehensive functional analysis of large gene lists. Nucleic Acids Res. 37, 1–13. doi: 10.1093/nar/gkn923
Huang D. W., Sherman B. T., Lempicki R. A. (2009b). Systematic and integrative analysis of large gene lists using DAVID bioinformatics resources. Nat. Protoc. 4, 44–57. doi: 10.1038/nprot.2008.211
Joloba M. L., Clemmer K. M., Sledjeski D. D., Rather P. N. (2004). Activation of the gab operon in an RpoS-dependent manner by mutations that truncate the inner core of lipopolysaccharide in escherichia coli. J. Bacteriol. 186, 8542–8546. doi: 10.1128/JB.186.24.8542-8546.2004
Joseleau-Petit D., Liébart J. C., Ayala J. A., D’Ari R. (2007). Unstable escherichia coli l forms revisited: Growth requires peptidoglycan synthesis. J. Bacteriol. 189, 6512–6520. doi: 10.1128/JB.00273-07
Kaneti G., Meir O., Mor A. (2016). Controlling bacterial infections by inhibiting proton-dependent processes. Biochim. Biophys. Acta - Biomembr. 1858, 995–1003. doi: 10.1016/j.bbamem.2015.10.022
Klein G., Lindner B., Brabetz W., Brade H., Raina S. (2009). Escherichia coli K-12 suppressor-free mutants lacking early glycosyltransferases and late acyltransferases. minimal lipopolysaccharide structure and induction of envelope stress response. J. Biol. Chem. 284, 15369–15389. doi: 10.1074/jbc.M900490200
Kocaoglu O., Carlson E. E. (2015). Profiling of β-lactam selectivity for penicillin-binding proteins in escherichia coli strain DC2. Antimicrob. Agents Chemother. 59, 2785–2790. doi: 10.1128/AAC.04552-14
Lai G. C., Cho H., Bernhardt T. G. (2017). The mecillinam resistome reveals a role for peptidoglycan endopeptidases in stimulating cell wall synthesis in escherichia coli. PloS Genet. 13, 1–22. doi: 10.1371/journal.pgen.1006934
Langridge G. C., Phan M. D., Turner D. J., Perkins T. T., Parts L., Haase J., et al. (2009). Simultaneous assay of every salmonella typhi gene using one million transposon mutants. Genome Res. 19, 2308–2316. doi: 10.1101/gr.097097.109
Leclercq S., Derouaux A., Olatunji S., Fraipont C., Egan A. J. F., Vollmer W., et al. (2017). Interplay between penicillin-binding proteins and SEDS proteins promotes bacterial cell wall synthesis. Sci. Rep. 7, 1–13. doi: 10.1038/srep43306
Lee A. J., Wang S., Meredith H. R., Zhuang B., Dai Z., You L. (2018). Robust, linear correlations between growth rates and β-lactam-mediated lysis rates. Proc. Natl. Acad. Sci. U. S. A. 115, 4069–4074. doi: 10.1073/pnas.1719504115
Lelong C., Aguiluz K., Luche S., Kuhn L., Garin J., Rabilloud T., et al. (2007). The crl-RpoS regulon of escherichia coli. Mol. Cell. Proteomics 6, 648–459. doi: 10.1074/mcp.M600191-MCP200
Levin-Reisman I., Ronin I., Gefen O., Braniss I., Shoresh N., Balaban N. Q. (2017). Antibiotic tolerance facilitates the evolution of resistance. Science 355, 826–830. doi: 10.1126/science.aaj2191
Li H., Durbin R. (2009). Fast and accurate short read alignment with burrows-wheeler transform. Bioinformatics 25, 1754–1760. doi: 10.1093/bioinformatics/btp324
Lindman M., Dick T. (2019). Bedaquiline eliminates bactericidal activity of β-lactams against mycobacterium abscessus. Antimicrob. Agents Chemother. 63, 1–5. doi: 10.1128/AAC.00827-19
Lobritz M. A., Andrews I. W., Braff D., Porter C. B. M., Gutierrez A., Furuta Y., et al. (2022). Increased energy demand from anabolic-catabolic processes drives β-lactam antibiotic lethality. Cell Chem. Biol., 29, 276–286. doi: 10.1016/j.chembiol.2021.12.010
Møller T. S. B., Rau M. H., Bonde C. S., Sommer M. O. A., Guardabassi L., Olsen J. E. (2016). Adaptive responses to cefotaxime treatment in ESBL-producing escherichia coli and the possible use of significantly regulated pathways as novel secondary targets. J. Antimicrob. Chemother. 71, 2449–2459. doi: 10.1093/jac/dkw198
Manuse S., Shan Y., Canas-Duarte S. J., Bakshi S., Sun W. S., Mori H., et al. (2021). Bacterial persisters are a stochastically formed subpopulation of low-energy cells. PloS Biol. 19, e3001194. doi: 10.1371/journal.pbio.3001194
Mathelié-Guinlet M., Asmar A. T., Collet J. F., Dufrêne Y. F. (2020). Lipoprotein lpp regulates the mechanical properties of the e. coli cell envelope. Nat. Commun. 11, 1789. doi: 10.1038/s41467-020-15489-1
Monahan L. G., Turnbull L., Osvath S. R., Birch D., Charles I. G., Whitchurch C. B. (2014). Rapid conversion of pseudomonas aeruginosa to a spherical cell morphotype facilitates tolerance to carbapenems and penicillins but increases susceptibility to antimicrobial peptides. Antimicrob. Agents Chemother. 58, 1956–1962. doi: 10.1128/AAC.01901-13
Nakao R., Ramstedt M., Wai S. N., Uhlin B. E. (2012). Enhanced biofilm formation by escherichia coli LPS mutants defective in hep biosynthesis. PloS One 7, e51241. doi: 10.1371/journal.pone.0051241
Page A. J., Bastkowski S., Yasir M., Turner A. K., Le Viet T., Savva G. M., et al. (2020). AlbaTraDIS: Comparative analysis of large datasets from parallel transposon mutagenesis experiments. PloS Comput. Biol. 16, 1–13. doi: 10.1371/journal.pcbi.1007980
Priyadarshini R., De Pedro M. A., Young K. D. (2007). Role of peptidoglycan amidases in the development and morphology of the division septum in escherichia coli. J. Bacteriol. 189, 5334–5347. doi: 10.1128/JB.00415-07
Radzikowski J. L., Vedelaar S., Siegel D., Ortega Á.D., Schmidt A., Heinemann M. (2016). Bacterial persistence is an active σ s stress response to metabolic flux limitation. Mol. Syst. Biol. 1–18, 882. doi: 10.15252/MSB.20166998
R Core Team (2021) R: A language and environment for statistical computing. Available at: https://www.r-project.org/.
Rinno J., Gmeiner J., Golecki J. R., Mayer H. (1980). Localization of enterobacterial common antigen: Proteus mirabilis and its various l-forms. J. Bacteriol. 141, 822–827. doi: 10.1128/jb.141.2.822-827.1980
Rodionov D. G., Pisabarro A. G., De Pedro M. A., Kusser W., Ishiguro E. E. (1995). β-lactam-induced bacteriolysis of amino acid-deprived escherichia coli is dependent on phospholipid synthesis. J. Bacteriol. 177, 992–997. doi: 10.1128/jb.177.4.992-997.1995
Sarkar S. K., Dutta M., Kumar A., Mallik D., Ghosh A. S. (2012). Sub-Inhibitory cefsulodin sensitization of e. coli to β-lactams is mediated by PBP1b inhibition. PloS One 7, 1–5. doi: 10.1371/journal.pone.0048598
Shetty A., Dick T. (2018). Mycobacterial cell wall synthesis inhibitors cause lethal ATP burst. Front. Microbiol. 9. doi: 10.3389/fmicb.2018.01898
Srivatsan A., Wang J. D. (2008). Control of bacterial transcription, translation and replication by (p)ppGpp. Curr. Opin. Microbiol. 11, 100–105. doi: 10.1016/j.mib.2008.02.001
Strahl H., Hamoen L. W. (2010). Membrane potential is important for bacterial cell division. Proc. Natl. Acad. Sci. U.S.A. 107, 12281–12286. doi: 10.1073/pnas.1005485107
Templin M. F., Edwards D. H., Holtje J. V. (1992). A murein hydrolase is the specific target of bulgecin in escherichia coli. J. Biol. Chem. 267, 20039–20043. doi: 10.1016/s0021-9258(19)88662-3
Thorsing M., Bentin T., Givskov M., Tolker-Nielsen T., Goltermann L. (2015). The bactericidal activity of β-lactam antibiotics is increased by metabolizable sugar species. Microbiol. (United Kingdom) 161, 1999–2007. doi: 10.1099/mic.0.000152
Tuomanen E. (1986). Phenotypic tolerance: the search for 3-lactam antibiotics that kill nongrowing bacteria. Rev. Infect. Dis. 8, 279–291. doi: 10.1093/clinids/8.Supplement_3.S279
Uehara T., Parzych K. R., Dinh T., Bernhardt T. G. (2010). Daughter cell separation is controlled by cytokinetic ring-activated cell wall hydrolysis. EMBO J. 29, 1412–1422. doi: 10.1038/emboj.2010.36
Urbaniec J., Xu Y., Hu Y., Hingley-Wilson S., McFadden J. (2022). Phenotypic heterogeneity in persisters: A novel “hunker” theory of persistence. FEMS Microbiol. Rev. 46, 1–16. doi: 10.1093/femsre/fuab042
Vicente M., Gomez M. J., Ayala J. A. (1998). Regulation of transcription of cell division genes in the eschericia coli dcw cluster. Cell. Mol. Life Sci. 54, 317–324. doi: 10.1007/s000180050158
Wang M., Chan E. W. C., Wan Y., Wong M.H.y., Chen S. (2021). Active maintenance of proton motive force mediates starvation-induced bacterial antibiotic tolerance in escherichia coli. Commun. Biol. 4, 1–11. doi: 10.1038/s42003-021-02612-1
Windels E. M., Michiels J. E., van den Bergh B., Fauvart M., Michiels J. (2019). Antibiotics: Combatting tolerance to stop resistance. MBio 10, e02095–19. doi: 10.1128/mBio.02095-19
Yang Y., Bhachech N., Bush K. (1995). Biochemical comparison of imipenem, meropenem and biapenem: Permeability, binding to penicillin-binding proteins, and stability to hydrolysis by β-lactamases. J. Antimicrob. Chemother. 35, 75–84. doi: 10.1093/jac/35.1.75
Keywords: TraDIS-Xpress, Escherichia coli, meropenem, cell division, cell envelope, ATP, transcription, antibiotic tolerance
Citation: Thomson NM, Turner AK, Yasir M, Bastkowski S, Lott M, Webber MA and Charles IG (2022) A whole-genome assay identifies four principal gene functions that confer tolerance of meropenem stress upon Escherichia coli. Front. Antibiot. 1:957942. doi: 10.3389/frabi.2022.957942
Received: 31 May 2022; Accepted: 23 August 2022;
Published: 16 September 2022.
Edited by:
Jianping Xie, Southwest University, ChinaReviewed by:
Absar Talat, Aligarh Muslim University, IndiaYasir Bashir, Aligarh Muslim University, India
Hasan Ejaz, Al Jouf University, Saudi Arabia
Rehab Mahmoud Abd El-Baky, Minia University, Egypt
Amitabha Bhattacharjee, Assam University, India
Copyright © 2022 Thomson, Turner, Yasir, Bastkowski, Lott, Webber and Charles. This is an open-access article distributed under the terms of the Creative Commons Attribution License (CC BY). The use, distribution or reproduction in other forums is permitted, provided the original author(s) and the copyright owner(s) are credited and that the original publication in this journal is cited, in accordance with accepted academic practice. No use, distribution or reproduction is permitted which does not comply with these terms.
*Correspondence: Mark A. Webber, bWFyay53ZWJiZXJAcXVhZHJhbS5hYy51aw==