Use of Climate Change Projections for Resilience Planning in Rio de Janeiro, Brazil
- 1Oswaldo Cruz Foundation (Fiocruz), Rio de Janeiro, Brazil
- 2Center for Climate Systems Research, Earth Institute, Columbia University, New York, NY, United States
- 3Department of Meteorology, Federal University of Rio de Janeiro, Rio de Janeiro, Brazil
- 4NASA Goddard Institute for Space Studies (NASA), New York, NY, United States
In this study we use three different methodologies to document and compare temperature and precipitation projections for the city of Rio de Janeiro (RJ) over the 21st century. It aims to explore in what way the differences and similarities of those methodologies and their outcomes support the incorporation of climate risks in urban planning and improve effective urban climate change governance. We compared the projections for RJ from the Eta Regional Climate Model from the Brazilian National Institute for Space Research nested in two Hadley Center Global Climate Models (GCMs) (Eta-HadCM3 method and Eta-HadGEM2-ES method) and 33 GCMs from the Coupled Model Intercomparison Project Phase 5 multi-model dataset (Urban Climate Change Research Network - UCCRN method). The three methods showed increasing temperatures for RJ at the end of the century. Precipitation projections span a 13% decrease to a 12% increase when using the UCCRN method or are reduced between 0.4 and 0.5%, when using the Eta-HadGEM2-ES method. However, the middle range of the projections from UCCRN and Eta-HadGEM2-ES is similar. The three methods project an increase of warm days and nights and a decrease of cold days and nights. Nevertheless, although the directions of change are the same applying the three methods, the magnitude differs when considering warm and cold nights. Hence, city stakeholders are better informed when we apply different projection methods as it gives them the opportunity to consider the level of risk they are willing to bear in the future. We observed that defining climate change projections on the city scale based on clear communication and an interactive process between scientists and stakeholders can be used to inform citywide adaptation strategies and sector-specific uses, as well as promote urban climate risk governance.
Introduction
There is a recognition that the occurrence probability of extreme events of high magnitudes is increasing, owing to anthropogenic climate change (IPCC, 2007, 2014; Seneviratne et al., 2012) and that climate change poses a range of hazards to urban systems and populations (Rosenzweig et al., 2011). There are studies from all around the world showing that densely populated areas in large cities may be vulnerable to disasters induced by extreme events at present, and this vulnerability may increase in the future (CEPED/UFSC, 2013; Gencer et al., 2018). These risks suggest a need for cities to rethink their methods of planning and operations in response to an evolving environment. For that, it is necessary to improve the knowledge of the city's hazards, vulnerability, and adaptive capacity to climate change (Rosenzweig et al., 2011). As a result, there is growing research and policy interest in urban climate risk assessment to inform adaptation strategies that lead to resilient and sustainable cities.
City-specific climate data and downscaled projections from global climate models (GCMs) can provide city decision-makers and other stakeholder groups with the scientific foundation for urban climate policies (Bader et al., 2018). For the Fifth Assessment Report (AR5) of the Intergovernmental Panel on Climate Change (IPCC) (IPCC, 2013), future climate changes were projected by over 30 GCMs (Taylor et al., 2012) for four representative concentration pathways (RCPs); (Moss et al., 2010) and their associated greenhouse gas (GHG) emission scenarios, expressed in terms of radiative forcing toward the end of the 21st century. Bearing in mind city-specific climate hazards, scientists are challenged to provide high-resolution projections that better characterize local and regional changes in climate patterns and extremes. This can help support city managers so that they can develop effective response strategies (Romero-Lankao et al., 2018; Rosenzweig et al., 2018).
In view of this challenge, the Urban Climate Change Research Network (UCCRN) downscaled city projections for the 2050s and 2080s, considering both mean and extreme climate variables, utilizing simulation results from 33 GCMs and two RCPs (RCP4.5 and RCP8.5) (Bader et al., 2018; Rosenzweig et al., 2018). These, and similar methods, have been applied to inform citywide adaptation plans in New York City (Rosenzweig and Solecki, 2010; Horton et al., 2011, 2015), and London (London Climate Change Partnership, 2002).
Since 1997, the Center for Weather Forecast and Climate Studies (CPTEC) from the Brazilian National Institute for Space Research (CPTEC/INPE) has been developing simulations with Eta, a regional climate model (RCM), to support the Brazilian National Communication to the United Nations Framework of Climate Change Convention (Chou et al., 2012, 2014; Lyra et al., 2018). Recently, CPTEC/INPE used the 5-km Eta RCM to simulate the future climate of Southeast Brazil with an emphasis on three metropolitan areas: Rio de Janeiro, São Paulo, and Santos. The historical period was simulated from 1960 to 2005, and the future climate was simulated from 2006 to 2100 (Lyra et al., 2018). This effort supports studies on the impacts, vulnerability, and adaptation to climate change in these major urban agglomerations, with the caveat that even finer resolution is needed to more completely capture the highly variable spatial configurations present in these cities.
Considering the recent efforts in local climate change projections, such as the ones developed by UCCRN and CPTEC/INPE, and the political interest of cities to reduce climate change risks through adaptation strategies, some questions are posed: Are these methodologies suitable to support managers of climate change risk in cities by reducing uncertainties? Is the information robust enough to be used when planning adaptation strategies to climate change in cities? Are the projections useful for incorporating climate risk into urban planning and improving urban climate change policies?
To answer these questions, we present and evaluate different climate change projections based on three methods developed for the city of Rio de Janeiro (RJ), Brazil. We analyze how the projections could influence the design of adaptation strategies to climate change in Rio de Janeiro and if they could be helpful in improving its climate change policymaking.
Geography and Meteorology
Rio de Janeiro is the largest coastal city and the second largest economic center of Brazil, estimated to have had 6.5 million inhabitants in 2016 (IBGE, 2016). More than 1 million people (~20% of its population) live in precarious settlements, especially vulnerable slums that are located throughout the city (IBGE, 2016). The economy of RJ is based on services (86%), followed by the industry sector of which oil and mining contribute 11% of the city's Gross Domestic Product (GDP) (Junqueira and Nery, 2015).
Population growth in RJ is projected to remain stable until 2030, when it is projected to decrease at a rate of ~0.5% per year (La Rovere and da Silva de Sousa, 2016). In 2010, the Gini index of per capita household income was 0.64 (IBGE/Censo Demográfico, 2010) and the municipality's human development index was 0.799, varying between 0.97 in neighborhoods with the highest income and 0.70 in those with the lowest income (Junqueira and Nery, 2015). Rio de Janeiro is divided into five Planning Areas (PA), comprising 33 Administrative Regions (AR) and 161 neighborhoods (La Rovere and da Silva de Sousa, 2016).
The city is located in Southeastern Brazil, between the 22.8° and 23.1°S parallels and the meridians of 43.1° and 43.8°W (Figure 1), and features a warm, rainy climate in the summer and dry weather in the winter (Dereczynski et al., 2009). The urban area is characterized by a high degree of temporal and spatial variations in meteorological elements. The city area receives influences from the Atlantic Ocean to its south, the Guanabara Bay to its east, and the Sepetiba Bay to its west. Its topography is marked by the formation of three massifs: Gericinó-Mendanha, at the north; the Tijuca massif at the east, where the Alto da Boa Vista weather station is located; and the Pedra Branca massif, at the west. The other areas of the city are lowlands with an average altitude of about 20 m. The Santa Cruz weather station is in the West Zone lowland. Both weather stations belong to the Brazilian Meteorology National Institute (INMET).
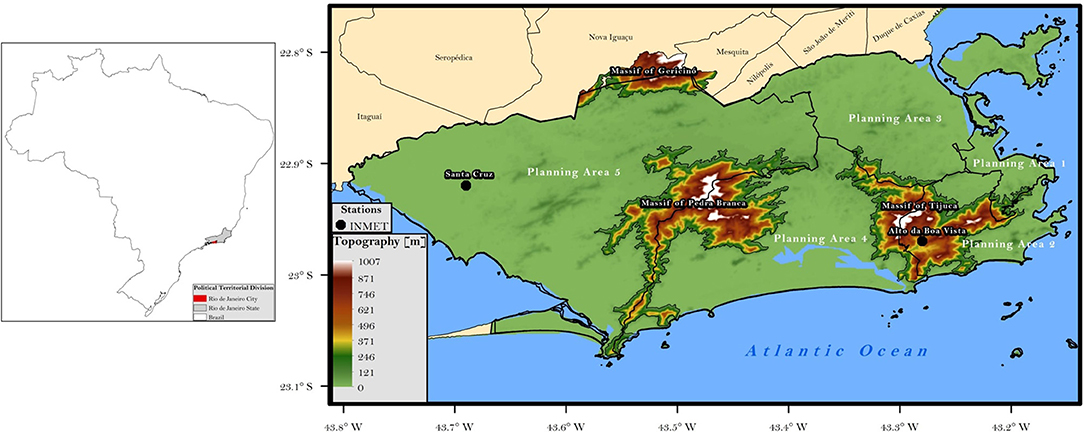
Figure 1. The city of Rio de Janeiro showing its topography, massifs, the five Planning Areas, and the location of INMET's Alto da Boa Vista and Santa Cruz meteorological stations.
The forest coverage of the massifs influences the observed patterns of temperature, winds, evaporation, cloud formation, and rainfall in the region (La Rovere and da Silva de Sousa, 2016). The massifs reach heights exceeding 1,000 m and shape the penetration of sea breezes into the interior. Therefore, the North and West Zones of the city are usually the driest and warmest areas, in contrast to the South Zone where the sea breeze cools the air. The West Zone is the most threatened by flooding and infectious disease (La Rovere and da Silva de Sousa, 2016).
Disaster Risks and Climate Change
Rio de Janeiro has developed over steep hills, wetlands, mangroves, and floodplains. The city has expanded its medium and high-density areas with a major presence of slums that lack natural green areas. Forest suppression and irregular occupation has greatly increased the city's vulnerabilities to flooding, landslides, and the urban heat island. These risks are exacerbated by climate change, with intense vulnerability for residents of precarious settlements that are especially threatened. Social housing programs have sometimes been located in areas prone to climate change impacts.
Disaster Risks
The city has a significant history of disasters caused by intense rainfall events, with associated fatalities and financial losses. Heavy rains recorded in 1966, 1967, and 1988 resulted in more than 280 deaths and 1,700 landslide occurrence reports. In April 2010, more than 60 deaths were recorded in the city after strong rains (La Rovere and da Silva de Sousa, 2016).
Since 1967, municipal agencies and programs were created in order to reduce the risks of socio-economic disasters associated with extreme precipitation events. In 1967, the municipal department GEO-RIO was established, responsible for the containment of slopes. It was followed in 1978 by the Civil Defense, responsible for preventing the occurrence of disasters or minimizing their effects; and in 1996 by the Rio Alert System. In 1998, the Rio-Águas Foundation became responsible for maintaining the city's rivers and canals for flood control. An Operations Center was created in 2010 to monitor and optimize the daily functioning of the city, foreseeing and managing weather conditions, landslides, floods, and accidents, among other functions. In 2011, the Community Alarm System program was linked to the Secretariat for Civil Defense and Protection to trigger sirens at times of high landslide risk, so that the local population can follow previously disseminated guidelines in order to prevent economic and financial loss, as well as the loss of life. Nevertheless, despite all these efforts, the current high rate of urbanization is still threatening uninhabited areas in Rio de Janeiro that are naturally sensitive to climate variability, applying pressure to green areas, and reducing soil infiltration capacity1.
Climate Change
Climate change assessment and action in Rio de Janeiro is more recent than its record of disaster and risk management. In 2007, the first climate risk assessment was conducted by the city agency responsible for providing information to city planners – the Pereira Passos Institute (IPP). This assessment was carried out by local scientists and was entitled “Rio Próximos 100 Anos” (Instituto Municipal de Urbanismo Pereira Passos–IPP/SMU, 2008). In 2010, a second assessment was conducted by a federal agency, the Center for Earth System Science at the National Institute for Space Research (CCST/INPE), funded by the British Embassy and the Foreign Commonwealth Office. Scientists from the 2008 study along with the IPP team were involved. Results from the earlier assessment were included in the report (Gusmão, 2011). In 2014, RJ embarked on a resilience program jointly developed under the auspices of the Mayor's Office and the Rockefeller Foundation's 100 Resilient Cities Program (100RC) – a program dedicated to helping cities around the world become more resilient to the physical, social, and economic challenges that are a growing part of the 21st century. The results were published in Junqueira and Nery (2015).
In 2015, the Centre for Integrated Studies on Climate Change and the Environment from the Federal University of Rio de Janeiro (Centro Clima/ COPPE/UFRJ), within the partnership established with the Climate Change and Sustainable Development Office of the Municipal Secretariat for the Environment (SMAC), launched the “Technical Assessment in Support of the Climate Change Adaptation Strategy for the City of Rio de Janeiro”. This assessment presents a roadmap to further develop climate risk management along with the generation of new opportunities that promote sustainable urban development along with protection of natural ecosystems. The study aimed to advance current knowledge, promote capacity-building of decision-makers, and foster the incorporation of climate hazards into government planning and management. The results of the study are summarized in “Climate Change Adaptation Strategy for the City of Rio de Janeiro” (La Rovere and da Silva de Sousa, 2016), which comprises a number of initiatives targeted at reducing the present and potential exposure and sensitivity of the city to climate hazards, while implementing actions that strengthen the adaptive capacity of its institutions and population.
These assessments are complemented by legal frameworks that contribute to climate change policymaking in Rio de Janeiro. In 2011, Law No. 5,248 was instituted by the Policy Council Climate Change and Sustainable Development and established targets for the reduction of anthropogenic emissions of greenhouse gases, as well as proposed other actions that contribute to the promotion of the UN Sustainable Development Goals (United Nations, 2015). It aims to ensure the sustainable development of the municipality through compatible economic and social development along with protection of the environment and the climate system, among others. Actions to meet this goal include stimulating research and development related to the climate system, integrating scientific research into government actions, identifying vulnerabilities, promoting effective actions to adapt to the potential negative impacts of climate change, and encouraging city leaders to plan for adaptation and resilience to climate change.
In 2019, Decree n° 46.079/19 instituted the Climate Program for the city. It presents a new climate governance structure, with the objective of proposing, planning, and integrating the execution of sustainable actions in conjunction with a path to low carbon development. The Program includes a Climate Action Plan, Climate Monitoring System, and Executive Committee on Climate Change. It is being led by the Municipal Secretariat of the Executive Office (CVL), through the Sub-Secretariat for Planning and Monitoring of Results (SPMR) and its Planning Office (IPP). The Executive Committee on Climate Change (ECCC) is composed of the SPRM, IPP, and the municipal secretariats of transport, urbanism, infrastructure and housing, public order, geotechnics, civil defense, energy and lighting, parks and gardens, and water, among others2.
The Climate Action Plan, named the Plan of Sustainable Development and Climate Action (CAP), is being developed jointly with the C40 Cities Climate Leadership Group network. It includes an adaptation plan for Rio de Janeiro that incorporates results from the historical assessments of the city, as well as new technical data provided by its staff and researchers. The technical staff of the ECCC, scientists, and stakeholders with expertise in this field are collaborating on this effort. The CAP will be integrated into RJ's 2030 Agenda and Sustainable Development Goals.
Table 1 shows some of the key climate change vulnerabilities of Rio de Janeiro identified by La Rovere and da Silva de Sousa (2016) for the Planning Areas identified in Figure 1. These key risks are useful when designing the Climate Change Adaptation Strategy and will be used in developing the Climate Action Plan (CAP).
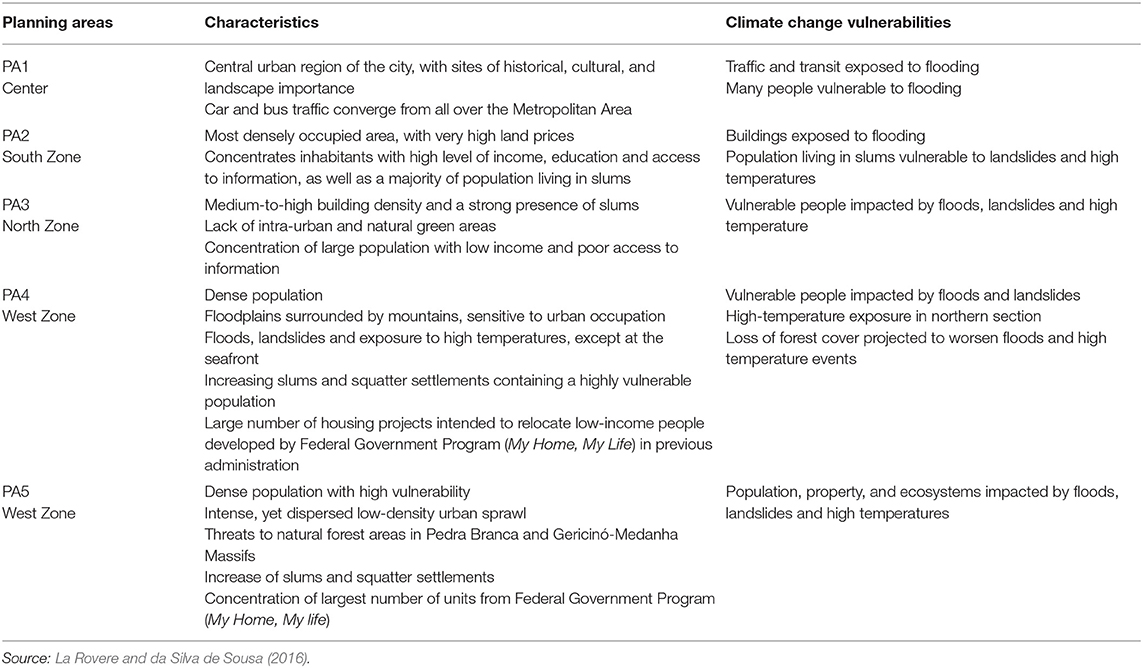
Table 1. Socio-ecological and biophysical aspects of climate change vulnerabilities for Planning Areas in Rio de Janeiro.
Materials and Methods
Three different climate projection methods developed for Rio de Janeiro are presented: Eta-HadCM3, UCCRN, and Eta-HadGEM2-ES. All the methods use global climate model simulations as a basis for the downscaled projections. GCMs are physics-based mathematical representations of the Earth's climate system over time that can be used to estimate how sensitive the climate system is to changes in atmospheric concentrations of greenhouse gas emissions and aerosols. Although it is not possible to predict the temperature or precipitation for a particular day, month or year, GCMs are valuable tools for projecting the likely range of changes over multi-decadal time periods.
Table 2 compares the Eta-HadCM3, UCCRN, and Eta-HadGEM2-ES methods, identifying differences in the type, number, and horizontal resolution of climate models, emissions scenarios, reference, and future time-slice periods.
Eta-HadCM3 Method
The first method, Eta-HadCM3, is based on the outcomes of Dereczynski et al. (2013), which provide projections of the RCM Eta from CPTEC/INPE, with 40-km horizontal resolution, nested within the GCM UK Met Office Hadley Centre (HadCM3), for Rio de Janeiro. These simulations, conducted by Chou et al. (2012), used the A1B scenario from the Special Report on Emissions Scenarios (SRES, 2000), considering 1961–1990 as the historical reference period. Future projections considered the means of precipitation and air temperature and climate extreme indices in three future periods (2011–2040, 2041–2070, and 2071–2100), known as time slices. In Chou et al. (2012), simulations were generated for a set of four members: the control member plus three integrations with physical disturbances, and different climate sensitivities, referred to as Ctrl, Low, Mid, and High. Each member's temperature projections are characteristic of low, medium, and high emissions from the IPCC SRES. The projections are made for one Eta-model grid point at RJ, located at 23°S/43.4°W.
UCCRN Method
Downscaled projections were made describing how two climate variables—temperature and precipitation—will likely change in RJ over the 21st century for three time slices 2010–2039; 2040–2069; and 2070–2099. The projections are expressed relative to the reference period 1971–2000. A combination of 33 GCMs simulations and two RCPs (RCP4.5 and RCP8.5) greenhouse gas emissions scenarios were used, totaling 66 possible outcomes for each climate variable in each time slice.
The GCMs run for the RJ projections were those that were used to create global projections for the AR5 (IPCC, 2013). They are downscaled from the bias-corrected and spatially disaggregated (BCSD)3 dataset, with 0.5-degree resolution (~50 km) that is derived from the Coupled Model Intercomparison Project Phase 5 (CMIP5)4 multi-model data set. RCPs represent the amount of radiative forcing caused by GHGs and other important agents such as aerosols. Each RCP is consistent with a trajectory of GHGs, aerosols, and land use changes developed for the climate modeling community as a basis for long-term and near-term climate modeling experiments (Moss et al., 2010). The scenarios used were RCP 4.5 and RCP 8.5, which, respectively, represent low and high future emissions trajectories and serve as inputs to the GCMs that guide the projected intensity of future climate changes.
For UCCRN, future climate extremes are calculated using the delta method, a long-established technique for developing local climate change projections (Gleick, 1986; Arnell, 1996; Wilby et al., 2004; Horton et al., 2011). This technique is a type of bias correction where the difference between each model's future simulation and that model's baseline simulation is used, rather than obtaining the “raw” outputs from the models. Similarly, mean precipitation change is based on the ratio of a given model's future precipitation to that model's baseline precipitation (expressed as a percentage change).
Monthly output from climate models is considered more reliable than daily output (Grotch and MacCracken, 1991), therefore a hybrid projection technique is used. Modeled changes in monthly temperature and precipitation are found by the same methods described for the annual data. Monthly changes through time in each of the GCM-RCP combinations are then applied (added in the case of degrees of temperature change and multiplied in the case of percentage change in precipitation) to the observed daily baseline temperature and precipitation data from the weather stations in RJ.
Extreme event projections for RJ were based on observational data of a series of maximum and minimum air temperatures and total daily rainfall of two weather stations from the INMET, which are Alto da Boa Vista (22°57'57.50”S/43°16′46.20″W) and Santa Cruz (22°55′19.59″S/43°41'12.90″W). These locations represent two extreme climate (and land cover) conditions, where the first is located in a tropical forest environment (Tijuca Forest National Park) at an altitude of 347.1 m, and the latter is positioned in the western zone of RJ at an altitude of 63.0 m, in a region where significant urbanization, densification, and expansion has taken place.
Eta-HadGEM2-ES Method
This method consists of projections for Rio de Janeiro, based on the outputs of the 5-km horizontal resolution RCM Eta of CPTEC/INPE, nested within the global model HadGEM2-ES, using two representative concentration pathways RCP 4.5 and RCP 8.5 (Lyra et al., 2018). The future projections of this method have the same methodology as Eta-HadCM3, except that two grid points are considered: one nearest to the Alto da Boa Vista meteorological station, located at 23.0°S/43.2°W and the second one nearest to the Santa Cruz meteorological station, located at 23.0°S/43.7°W.
Comparison of Climate Projection Methods
The mean and extreme climate variables for which the projections from the three different methods (Eta-HadCM3, UCCRN and Eta-HadGEM2-ES) are provided allow for a comparison among the types of climate science information available for cities (Bader et al., 2018), as well as the results of using different methodologies and how that may influence stakeholder decision-making.
The primary differences in the methods among the three analyses lie in the type of models (i.e., GCMs and RCMs), their horizontal resolutions, and the emissions scenarios used. UCCRN uses a suite of 33 GCMs, while Eta-HadCM3 and Eta-HadGEM2-ES each use only one RCM (Eta model) and one GCM (HadCM3 or HadGEM2-ES). The number of possible combinations, and therefore climate realizations, is greater using the UCCRN method. This enables the presentation of the results across a broader range of uncertainties than the other methods.
Results from all three methods are downscaled locally. The Eta-HadCM3 and UCCRN methods provide projections for a single grid box over the city, while the Eta-HadGEM2-ES method concentrates on two smaller geographic areas. The UCCRN method uses monthly bias-corrected and spatially disaggregated climate projections at 1/2° resolution derived from the World Climate Research Program's CMIP5 multi-model data set. The downscaling techniques differ in that the UCCRN method is statistically based, while Eta-HadCM3 and Eta-HadGEM2-ES are from a dynamically-based regional climate model. Statistical downscaling produces finer-scale features than GCMs, using historical relationships between the large and small scales. Dynamical downscaling is achieved by running a regional climate model at high resolution over a small spatial domain with forcing variables from a GCM.
There are also slight differences in the baselines and time slices. All three methods present projections as averages across similar 30-year future time periods and are expressed relative to the reference period– 1971–2000 for UCCRN and 1961–1990 for Eta-HadCM3 and Eta-HadGEM2-ES. These different baselines do have an effect on results, since warming in RJ is more prominent in the later period, thus dampening results of projected future changes.
Results
Temperature and Precipitation Projections for the 21st Century
Mean annual temperature results for Rio de Janeiro projected by UCCRN and Eta-HadGEM2-ES are presented, respectively, in Tables 3, 4. As the Eta-HadCM3 method uses a different emissions scenario (not RCPs 4.5 and 8.5), it was not included in the comparison here. However, all three methods show that temperatures are projected to increase in Rio throughout the 21st century.
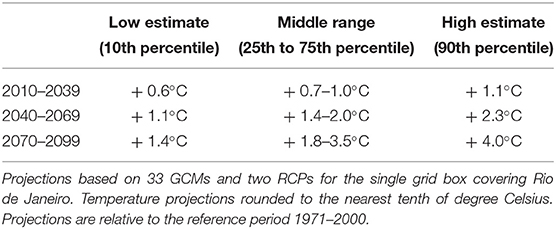
Table 3. Temperature projections using the UCCRN method for Rio de Janeiro, Brazil, over the 21st century.
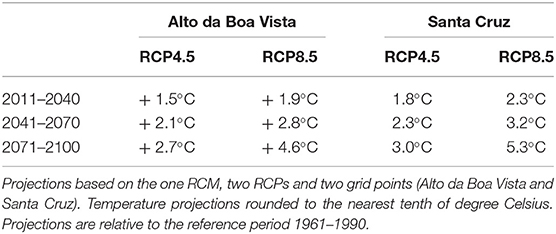
Table 4. Temperature projections for the Alto da Boa Vista and Santa Cruz meteorological stations, over the 21st century, based on the Eta-HadGEM2-ES method.
Tables 3, 4 show the temperature projections for the Rio de Janeiro stations location based on the UCCRN and Eta-HadGEM2-ES methods, from 2010 to 2100, using two RCPs (RCP4.5 and RCP8.5). Overall, both the ensemble of GCMs and the Eta-5 km model present a signal toward a warmer climate throughout the 21st century. It is evident that for the first time slice (i.e., 2011–2040 or 2010–2039), the positive change in relation to the reference period (1971–2000 for method UCCRN, and 1961–1990 for method Eta-HadGEM2-ES) is lower than for the other time slices, and that for the end of the 21st century (2071–2100 or 2070–2099), the projections of mean temperature increase are higher. These features are explained by the increase in greenhouse gas concentrations over time, which raise mean temperatures.
In addition, the two different representative concentration pathways (RCP4.5 and RCP8.5) both point to a warmer climate in RJ, with the RCP8.5 being the warmer one (due to higher radiative forcing). For both of these methods, those differences are smaller in the early 21st century and only increase toward the end of the 21st century. This result is similar to global projections that show similar rates of warming across RCP scenarios until about the 2050s due to the forcing mechanisms “built in” to the climate system. Furthermore, the UCCRN method projects lower warming (i.e., +4.0°C), than the Eta-HadGEM2-ES method (+4.9°C). This may have occurred because the Eta-HadGEM2-ES uses a regional climate model with a 5-km horizontal resolution, so it is capable of producing more extreme values than the GCMs with coarser resolution.
Given the large number of GCMs utilized in the UCCRN method, there is a wide range of precipitation projections, compared to only one GCM-RCM combination in the ETA method. However, the middle range of the projections from UCCRN presented in Table 5 and the Eta-HadGEM2-ES projections presented in Table 6 are similar.
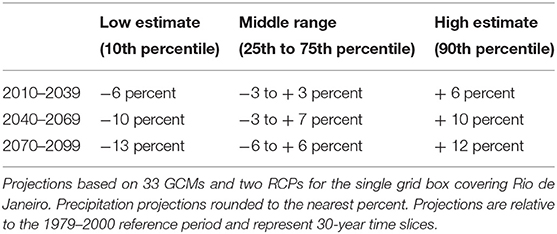
Table 5. Precipitation projections using the UCCRN method for Rio de Janeiro, Brazil, over the 21st century.
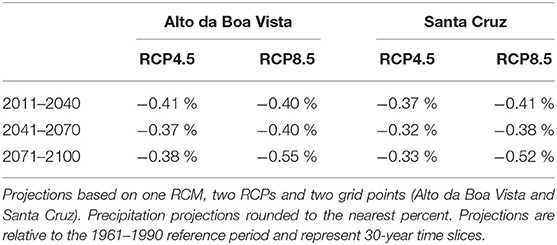
Table 6. Precipitation projections for Rio de Janeiro, Brazil, over the 21st century, based on Eta-HadGEM2-ES.
Projections of Temperature Extremes for the 21st Century
Looking at changes in warm days and cold days, and warm nights and cold nights presented in Table 7, the three methods all project increases in warm extremes. In the later part of the 21st century, the projections show an increase in the number of warm days and warm nights and a decrease in the number of cold days and cold nights. This was found for both the Alto da Boa Vista and Santa Cruz station locations. However, the magnitude of the increase in the warm nights differs across the three methods.
Discussion
Challenges for urban sustainability include climate risk management. Effective weather and climate forecasts of daily, weekly, and seasonal patterns and extreme events are already widely used at international, national, and regional scales. The wide use of these short-term forecasts demonstrates the value of clear communication of climate science for decision-makers (Bader et al., 2018). Climate change presents a similar opportunity to convey climate information on longer time scales so that decision-makers can develop resilience actions for urban infrastructure, ecosystems, and human services (Rosenzweig et al., 2018). In this context, climate change projections on the city scale, based on clear communication and an interactive process between scientists and stakeholders, can be used to inform citywide adaptation strategies and for sector-specific uses.
Bader et al. (2018) demonstrated that future climate projections from both GCMs and RCMs are being used by cities across the globe in a variety of applications. These include vulnerability assessments of urban populations (Barata et al., 2015; Quintão et al., 2017; Menezes et al., 2018; Vommaro et al., 2020); adaptation planning (London Climate Change Partnership, 2002; Rosenzweig and Solecki, 2010; La Rovere and da Silva de Sousa, 2016), and in sector-specific uses, for example city water resources (Chung et al., 2011) or transportation (Gidhagen et al., 2012). Each one of these studies applied local downscaled GCM or RCM projections.
Since 2007, efforts have been applied in Rio de Janeiro, aiming to provide climate risk information to city planners and to support them in managing those risks. Those efforts contributed to strengthening the partnership between city stakeholders and climate scientists, as well as improving city practitioner knowledge on the topic of climate change risk. Through this process, climate scientists developed temperature and precipitation projections up to the end of this century for the city, applying a range of methods.
Effective climate change policy requires leadership, legal frameworks, public participation mechanisms, information sharing, and financial resources (Romero-Lankao et al., 2018). Policies to reduce socio-ecological and economic impacts from climate change have recently been implemented in RJ. These policies were enabled by the improvement of research on climate change projections, the establishment of legal frameworks, and stakeholder-scientist interactions undertaken since 2007. The Climate Action Plan of Rio de Janeiro, entitled the Plan for Sustainable Development and Climate Action (CAP) is being established by municipal decree, with leadership by the Municipal Secretariat of the Executive Office (CVL), engagement of international NGOs, city stakeholders, and experts. The Plan aims to reduce climate impacts, both now and in the future. Through this Plan, new interventions in the city must consider both present and future climate hazards, its economic and environmental resources, as well as social vulnerabilities. A key element is the banning of construction of houses and other infrastructure in city areas exposed to present and future climate hazards, including landslides and sea level rise.
In this study, we compared the projections for RJ from RCMs (Eta-HadCM3 and Eta-HadGEM2-ES) and GCMs (UCCRN). Through the application of the UCCRN method (applied in 2015) and the HadGEM2-ES method (undertaken in 2018), it was possible to project average temperature increases towards the end of the century. All methods showed increasing temperatures for the city in the coming century.
Given that projections of precipitation are more uncertain than those for temperatures, the range of potential outcomes from the UCCRN method is suggested for policymakers. While this level of uncertainty may pose challenges for decision-makers, scientifically it is the more accurate approach to use at this time. The UCCRN method uses multiple climate model realizations, reflecting a broader set of plausible future climate outcomes. The finer-resolution ETA projections are more limited because they use only a single GCM-RCM pairing. However, the ETA projections do fall in the range of the UCCRN projections and are close to the midpoint. In any case, planners should avoid relocating low-income people to flood-prone areas, as designated by the Federal Government Program, My Home, My Life. A cost-benefit assessment of interventions to reduce flooding areas and to enhance resilience before building new houses should be carried out.
However, considering landslides and floods caused by precipitation, new studies of heavy rainfall, such as projections of maximum cumulative precipitation in 5 days, (Rx5-day) would also be useful. Such studies could provide further risk information for the development of climate change resilience policies in the city.
Two of the three methods provided projections of temperature extremes over the 21st century. Both of them showed an increasing number of warm days and nights. Although the magnitudes of the projected changes are not the same in the two methods, they demonstrate that the demand for cooling, especially in the northern region of the city is very likely to increase. This information is useful for planning housing, restoring and extending green areas, and other strategies for achieving a healthier city (Barata et al., 2011).
In this context, CAP should consider how to implement intra-urban and natural green areas, especially in PA3, as well as in part of PA4. Additionally, the interventions for improving the quality of life for people who live in slums should consider results of future temperature scenarios, as well as work to prevent landslides and flooding in vulnerable areas.
We observe that applying the different climate projection methods improves the city climate change policymaking process. It provides the opportunity for policymakers to consider the level of risk they are willing to plan for in the future. It also encourages them to foster interactions with scientists over time, as global and regional models will continue to support studies on impacts, vulnerabilities, and adaptation to climate change at urban scales.
The governance process in Rio de Janeiro aims to better manage current vulnerability as well as climate change risk. Cost-benefit analyses of possible strategies to reduce future impacts jointly with present vulnerability reduction should contribute to the improvement of urban resilience. The foundation for effective adaptation planning is the co-development of plans with policy-makers and scientists who can provide urban-scale information about climate risks—both current risks and projections of future changes in extreme events (Rosenzweig et al., 2018).
Final Remarks
Considering the recent efforts in development of local climate change resolution projections, such as the ones developed by UCCRN and CPTEC/INPE, there is growing political interest in reducing urban climate change risk through adaptation strategies. We can answer our initial questions by observing that urban climate projections using GCMs or RCMs are suitable tools to support managers of climate change risk in cities, as they explicitly bound climate uncertainties. The projections are robust so that they can be used as a scientific evidence base when planning adaptation strategies to climate change in cities. Further, they are highly relevant because they contribute to participatory processes among scientists, government, non-governmental organizations, and civil society actors. Nevertheless, research on this issue should be continued as climate models improve to better incorporate climate risks in urban planning and to sustain effective urban climate policy-making.
Climate change and extreme events present a significant risk to cities. Designing climate adaptation strategies to achieve sustainable and resilient cities represents a challenge for the future. Integrating the roles of city practitioners, stakeholders, and scientists to provide sustainable solutions for cities is essential. Urban climate projections using GCMs or RCMs are useful and relevant tools for addressing this challenge.
Considering the case study of Rio de Janeiro, we observe that the process of developing and improving methodologies for climate change scenarios as inputs to implementation of adaptation strategies in the city was valuable. The city is a key actor since it develops its own policies to reduce the socio-ecological and economic impacts of climate change. In Brazil, scientific capacity is being developed by INPE in order to create downscaled regional scenarios for use in cities. The UCCRN method can be useful for other cities in Latin America for informing climate change policies where downscaled scenarios are not available.
The use of climate science for cities is improving. Providing downscaled information about climate risks to urban decision makers is a key mechanism to address the significant challenges that climate change presents to cities, both now and in future.
Data Availability Statement
The datasets generated for this study are available on request to the corresponding author.
Author Contributions
MB: Lead the study's conception and design, data collection and analysis, and wrote the manuscript's first draft. DB: Participated in the study's conception and design, provided projections for both mean and extreme climate variables (Method 2), and helped write the paper. CD: Provided information regarding observed and projected extremes, applied Method 1, recommended bibliography, and assisted in writing the paper. PR: Provided information regarding observed and projected extremes (Method 3). CR: Participated in the study's conception, recommended bibliography, and assisted in writing the paper. All authors revised and approved the version for publishing.
Funding
This research was partially supported by the Brazilian Higher Education Personnel Improvement Coordination - CAPES: process 11724/13-7.
Conflict of Interest
The authors declare that the research was conducted in the absence of any commercial or financial relationships that could be construed as a potential conflict of interest.
Acknowledgments
We would like to thank CAPES for financial support, and the Columbia University Center for Climate Systems Research for making its facilities available for post-doctoral research. Many thanks to Dr. Wilson Savino, the director of Oswaldo Cruz Institute at the Oswaldo Cruz Foundation (Fiocruz) at the Brazilian Ministry of Health, for approval of the lead author's post-doctoral research in Columbia University.
Footnotes
1. ^http://www.rio.rj.gov.br/web/smo/geo-rio (accessed March 23, 2020); https://www.rio.rj.gov.br/web/defesacivil (accessed March 23, 2020); http://www.rio.rj.gov.br/web/rio-aguas;cor.rio/institucional (accessed March 23, 2020); http://www.rio.rj.gov.br/web/ipp/ (accessed March 23, 2020).
2. ^http://www.rio.rj.gov.br/web/planejamento/exibeconteudo?id=9857163 (accessed March 23, 2020).
3. ^https://gdo-dcp.ucllnl.org/downscaled_cmip_projections/dcpInterface.html (accessed March 23, 2020).
4. ^CMIP5 is the Coupled Model Intercomparison Project Phase 5. CMIP provides a framework for standard protocols and comparison in global climate modeling, and the outputs are used in IPCC assessments (see Taylor et al., 2012).
References
Arnell, N. W. (1996). Global Warming, River Flows and Water Resources. Chichester; West Sussex: Wiley.
Bader, D. A., Blake, R., Grimm, A., Hamdi, R., Kim, Y., Horton, R., et al. (2018). “Urban climate science,” in Climate Change and Cities: Second Assessment Report of the Urban Climate Change Research Network, eds C. Rosenzweig, W. Solecki, P. Romero-Lankao, S. Mehrota, S. Dhakal, and S. Ali Ibrahim (New York, NY: Cambridge University Press), 27–60.
Barata, M., Confalonieri, U., Marinho, D., Costa Neto, C., Giovanini, L., Oliveira, F., et al. (2015). “Mapa de vulnerabilidade da população do estado do rio de janeiro aos impactos das mudanças climáticas,” in: Metodologias de Estudos de Vulnerabilidade à Mudança do Clima, eds M. Chang, K. Goés, L. Fernandes, M.A. Freitas, L. P. Rosa. (Rio de Janeiro: Interciência, 63–88.
Barata, M., Ligeti, E., de Simone, G., Dickinson, T., Jack, D., Penney, J. et al. (2011). “Climate change and human health in cities,” in Climate Change and Cities: First Assessment Report of the Urban Climate Change Research Network, eds C. Rosenzweig, W. D. Solecki, S. A. Hammer, and S. Mehrotra (New York, NY: Cambridge University Press), 183–217.
CEPED/UFSC (2013). Atlas Brasileiro de Desastres Naturais 1991 a 2012. Florianópolis: Universidade Federal de Santa Catarina. Centro Universitário de Estudos e Pesquisas sobre desastres.
Chou, S. C., Lyra, A., Mourão, C., Dereczynski, C., Pilotto, I., Gomes, J. et al. (2014). Assessment of climate change over South America under RCP 4.5 and 8.5 downscaling scenarios. Am. J. Clim. Change 3, 512–526. doi: 10.4236/ajcc.2014.35043
Chou, S. C., Marengo, J. A., Lyra, A., Sueiro, G., Pesquero, J. F., Alves, L. M. et al. (2012). Downscaling of South America present climate driven by 4-member HadCM3. Clim. Dyn. 38, 635–653. doi: 10.1007/s00382-011-1002-8
Chung, E. -S., Park, K., and Lee, K. S. (2011). The relative impacts of climate change and urbanization on the hydrological response of a Korean urban watershed. Hydrol. Proces. 25, 544–560. doi: 10.1002/hyp.7781
Dereczynski, C. P., Luiz Silva, W., and Marengo, J. (2013). Detection and Projections of Climate Change in Rio de Janeiro, Brazil. Am. J. Clim. Change 2, 25–33. doi: 10.4236/ajcc.2013.21003
Dereczynski, C. P., Oliveira, J. S., and Machado, C. O. (2009). Climatologia da precipitação no município do Rio de Janeiro. Rev. Br. Meteorol. 24, 24–38. doi: 10.1590/S0102-77862009000100003
Gencer, E., Folorunsho, R., Linkin, M., Wang, X., Natenzon, C. E., Wajih, S., et al. (2018). “Disasters and risk in cities,” in Climate Change and Cities: Second Assessment Report of the Urban Climate Change Research Network, eds C. Rosenzweig, W. Solecki, P. Romero-Lankao, S. Mehrotra, S. Dhakal, and S. Ali Ibrahim (New York, NY: Cambridge University Press), 61–98.
Gidhagen, L., Engardt, M., Lövenheim, B., and Johansson, C. (2012). Modeling effects of climate change on air quality population exposure in urban planning scenarios. Adv. Meteorol. 2012:12. doi: 10.1155/2012/240894
Gleick, P. H. (1986). Methods for evaluating the regional hydrologic impacts of global climatic changes. J. Hydrol. 88, 97–116. doi: 10.1016/0022-1694(86)90199-X
Grotch, S. L., and MacCracken, M. C. (1991). The use of general circulation models to predict regional climatic change. J. Clim. 4, 286–303. doi: 10.1175/1520-0442(1991)004<0286:TUOGCM>2.0.CO;2
Gusmão, P. (2011). Megacidades, Vulnerabilidades e Mudanças Climáticas: Região Metropolitana do Rio de Janeiro. Available online at: http://www.data.rio/datasets/dc00f0608dc5449abe03271c4a66a804
Horton, R., Bader, D. A., Kushner, Y., Little, C., Blake, R., and Rosenzweig, C. (2015). New York City panel on climate change 2015 report: climate observations and projections. Ann. N. Y. Acad. Sci. 1336, 18–35. doi: 10.1111/nyas.12586
Horton, R. M., Gornitz, V., Bader, D. A., Ruane, A. C., Goldberg, R., and Rosenzweig, C. (2011). Climate hazard assessment for stakeholder adaptation planning in New York City. J. Appl. Meteorol. Climatol. 50, 2247–2266. doi: 10.1175/2011JAMC2521.1
IBGE (2016). IBGE Cidades, Rio de Janeiro. Conheça Cidades e Estados dO Brasil. Available online at: http://cidades.ibge.gov.br/xtras/perfil.ph?cod-mun=330455
IBGE/Censo Demográfico (2010). Índice de Gini da Renda Domiciliar per Capita Segundo Município. Available online at: http://tabnet.datasus.gov.br/cgi/ibge/censo/cnv/ginirj.def
Instituto Municipal de Urbanismo Pereira Passos–IPP/SMU (2008). Rio Próximo 100 Anos. Rio de Janeiro: IPP/SMU.
IPCC (2007). Climate Change 2007: Impacts, Adaptation and Vulnerability. Contribution of Working Group II to the Fourth Assessment Report of the Intergovernmental Panel on Climate Change, eds M. L. Parry, O. F. Canziani, J. P. Palutikof, P. J. van der Linden and C. E. Hanson. Cambridge University Press
IPCC (2013). Climate Change 2013: The Physical Science Basis. Contribution of Working Group I to the Fifth Assessment Report of the Intergovernmental Panel on Climate Change, eds T.F., Stocker, D. Qin, G.-K. Plattner, M. Tignor, S.K. Allen, J. Boschung, et al. Cambridge; New York, NY: Cambridge University Press.
IPCC (2014). Climate Change 2014: Synthesis Report. Contribution of Working Groups I, II, and III to the Fifth Assessment Report of the Intergovernmental Panel on Climate Change, eds R. K. Pachauri and L. A. Meyer. Geneva: IPCC, Core Writing Team.
Junqueira, P., and Nery, L. A. (2015). Rio Resiliente. Estratégia de Resiliência da Cidade do Rio de Janeiro. Rio de Janeiro: Prefeitura do Rio de Janeiro. Available online at: https://www.100resilientcities.org/wp-content/uploads/2017/07/estra_res_rio_port-1.pdf
La Rovere, E., and da Silva de Sousa, D. (2016). Estratégia de Adaptação às Mudanças Climáticas da Cidade do Rio de Janeiro. Available online at: http://www.rio.rj.gov.br/dlstatic/10112/6631312/4179912/ESTRATEGIA_PORT.pdf
London Climate Change Partnership (LCCP) (2002). London's Warming. The Impacts of Climate Change on London. Technical report (311). LCCP.
Lyra, A., Tavares, P., Chou, S. C., Sueiro, G., Dereczynski, C., Sondermann, M., et al. (2018). Climate change projections over three metropolitan regions in Southeast Brazil using the non-hydrostatic Eta regional climate model at 5-km resolution. Theor. Appl. Climatol. 132, 663–682. doi: 10.1007/s00704-017-2067-z
Menezes, J. A., Confalonieri, U., Madureira, A. P., Duval, I. B., Santos, R. B., and Margonari, C. (2018). Mapping human vulnerability to climate change in the Brazilian Amazon: the construction of a municipal vulnerability Index. PLoS ONE. 13:e0190808. doi: 10.1371/journal.pone.0190808
Moss, R. H., Edmonds, J. A., Hibbard, K. A., Manning, M. R., Rose, S. K., van Vuuren, D. P. et al. (2010). The next generation of scenarios for climate change research and assessment. Nature 463, 747–756. doi: 10.1038/nature08823
Quintão, A. F., Brito, I., Oliveira, F., Madureira, A. P., and Confalonieri, U. (2017). Social, environmental, and health vulnerability to climate change: the case of the municipalities of Minas Gerais, Brazil. J. Environ. Pub. Health 2017:2821343. doi: 10.1155/2017/2821343
Romero-Lankao, P., Burch, S., Auty, K., Aylett, A., Krellenberg, K., Nakano, R., et al. (2018). “Governance and Policy,” in Climate Change and Cities: Second Assessment Report of the Urban Climate Change Research Network, eds Rosenzweig C, Solecki W, Romero-Lankao P, Mehrota S, Dhakal S, and Ibrahim SA (New York, NY: Cambridge University Press), 585–606.
Rosenzweig, C., and Solecki, W. (eds.). (2010). Climate change adaptation in New York city: Building a risk management response. New York City Panel on Climate Change. Report. Annals of the New York Academy of Sciences V. 1244. 460p.
Rosenzweig, C., Solecki, W., Hammer, S., and Mehrotra, S. (2011). Climate Change and Cities: First Assessment Report of the Urban Climate Change Research Network. New York, NY: Cambridge University Press.
Rosenzweig, C., Solecki, W., Romero-Lankao, P., Mehrotra, S., Dhakal, S., and Ibrahim, S. A. (2018). Climate Change and Cities: Second Assessment Report of the Urban Climate Change Research Network. New York, NY: Cambridge University Press.
Seneviratne, S. I., Nicholls, N., Easterling, D., Goodess, C. M., Kanae, S., Kossin, J., et al. (2012). “Changes in Climate Extremes and their Impacts on the Natural Physical Environment,” in Managing the Risks of Extreme Events and Disasters to Advance Climate Change Adaptation. A Special Report of Working Groups I and II of the Intergovernmental Panel on Climate Change (IPCC), eds C. B. Field, V. Barros, T. F. Stocker, D. Qin, D. J. Dokken, K. L. Ebi, et al. (New York, NY: Cambridge University Press).
SRES (2000). Special Report on Emissions Scenarios (SRES) for the Intergovernmental Panel on Climate Change. Nakićenović et al., Working Group III, Intergovernmental Panel on Climate Change (IPCC), Cambridge University Press, Cambridge. ISBN:0-521-80493-0.
Taylor, K. E., Stouffer, R. J., and Meehl, G. A. (2012). An overview of CMIP5 and the experiment design. Bull. Amer. Meteor. Soc. (2012) 93, 485–498. doi: 10.1175/BAMS-D-11-00094.1
United Nations (2015). Transforming our World: The 2030 Agenda for Sustainable Development. Available online at: https://sustainabledevelopment.un.org/content/documents/21252030%20Agenda%20for%20Sustainable%20Development%20web.pdf
Vommaro, F., Menezes, J. A., and Barata, M. M. L. (2020). Contributions of municipal vulnerability map of the population of the state of Maranhão (Brazil) to the sustainable development goals. Sci. Total Environ. 706:134629. doi: 10.1016/j.scitotenv.2019.134629
Wilby, R. L., Charles, S., Zorita, E., Timbal, B., Whetton, P., and Mearns, L. (2004). Guidelines for Use of Climate Scenarios Developed From Statistical Downscaling Methods. Available online at: https://www.ipcc-data.org/guidelines/dgm_no2_v1_09_2004.pdf
Keywords: urban governance and management, urban climate science, climate change risk management, temperature, precipitation, city, tools for urban resilience
Citation: Barata MML, Bader DA, Dereczynski C, Regoto P and Rosenzweig C (2020) Use of Climate Change Projections for Resilience Planning in Rio de Janeiro, Brazil. Front. Sustain. Cities 2:28. doi: 10.3389/frsc.2020.00028
Received: 30 December 2019; Accepted: 14 May 2020;
Published: 03 July 2020.
Edited by:
Tathagata Chatterji, Xavier University, IndiaReviewed by:
Eduarda Marques Da Costa, University of Lisbon, PortugalYonn Dierwechter, University of Washington Tacoma, United States
Copyright © 2020 Barata, Bader, Dereczynski, Regoto and Rosenzweig. This is an open-access article distributed under the terms of the Creative Commons Attribution License (CC BY). The use, distribution or reproduction in other forums is permitted, provided the original author(s) and the copyright owner(s) are credited and that the original publication in this journal is cited, in accordance with accepted academic practice. No use, distribution or reproduction is permitted which does not comply with these terms.
*Correspondence: Martha M. L. Barata, baratamml@gmail.com