- 1Université Gustave Eiffel (IFSTTAR), COSYS Department, PICS-L, Versailles, France
- 2QUT, CARRS-Q Laboratory, Brisbane, QLD, Australia
- 3Université Gustave Eiffel (IFSTTAR), Département COSYS, ERENA, Bordeaux, France
- 4Université Gustave Eiffel (IFSTTAR), Département COSYS, Champs sur Marne, France
With the growing development of Cooperative, Connected, and Automated Mobility (CCAM), questions arise about the real impact of this innovative mobility on our daily life. CCAM originally promised to improve road safety. It is now a holistic solution for future mobility: the CCAM is there to optimize traffic, which can translate into strategies for reducing energy consumption or polluting emissions, without compromising road safety. The capability of CCAM is dependent on the reliability and robustness of its components, as it will be making life-impacting decisions. It is therefore necessary to be able to guarantee a high-level quality of sensors, communication, software, and hardware architecture. In this mobility ecosystem, the infrastructure and data that it will be able to produce is at the heart of current research issues. This paper addresses the following question: Are the Connected and automated Vehicles (CAVs) the silver bullet solution with which to answer the issues of the current mobility systems? This question is discussed by investigating the technologies used, the digital infrastructures, its robustness to cyber-attack, and their relationship with the claimed benefits on safety, energy and pollution management, traffic optimization, deployment strategy, and a link with the new generation of road infrastructures.
1. Introduction
Within the context of an increasingly marked international competition, economic growth is often based on technological innovation. That radical innovation leads to structural change and economic growth is a central hypothesis in many evolutionary perspectives on the relationship between technology and growth (Freeman and Soete, 1990; Verspagen, 2001). Transport and mobility are no exception to this observation, and the race for technological innovation in this field has, in the last 20 years, reached its climax with the digitalization of one of the most important players in mobility systems: the driver. This digitalization, commonly known as “driving automation,” does not occur in isolation but in a symbiotic way with progress in terms of communication systems whether based on infrastructure or vehicles. The latest advances made in communication systems are thus rapidly integrated into the development of automated transport systems and the requirements of these systems guide research on communicating systems.
To be useful to society, in addition to promising improvement of the economy, a technological innovation must also make it possible to improve safety (reduce the accident risks, Webb et al., 2020) and health and reduce the tensions that mankind has placed on the environment (rationalize the use of energy resources). Are automated transport systems offering these societal benefits on top of the economical ones?
As praise continues to accumulate on these promising technologies (Tesla, 2020; Waymo, 2020), concerns related to poor design or misuse have begun to emerge since 2015 (Schoettle and Sivak, 2015). Recent crash data analysis has highlighted misuse of driving assistance encompassing lateral and longitudinal control (Russell et al., 2018; Dunn et al., 2019). Despite the recommendations and warnings from car manufacturers, drivers continue to place too much trust in this technology. New ADAS are often sold with misleading names and capability description. On a recent Hype cycle carried out by the Gartner cabinet in 2019 (Gartner, 2019), the so-called level 4 Automated Vehicles (AV) has thus entered a phase of disillusionment, showing the generalization of fears on this subject. This topic of over-trust, according to Dixon (2020), is also influenced by marketing managers who overstate the AV capacities.
The impact of AV has already been assessed in different studies. In the works of Workshop) (2018), a list of potential issues was provided depending on the usage scenarios of AVs. However, the only figure provided deals with fuel economy in truck platooning, which is estimated about 10%. The works from Hörl et al. (2016) estimated the potential impact of AD on traffic, city planning, the car industry, work organization, user profiles, delivery, and price. A more user-oriented but speculative impact study has been carried out by Gruel and Stanford (2016), and it shows how the different usage can change with AVs. Another study for (Coelho and Guarnaccia, 2020) also proposed methodologies to evaluate the impact of Automated Driving (AD) on pollutants emissions, noise, and safety but relies on the estimation of driving pattern changes. A more recent work from Litman (2020) discussed the impact of ADs on transport planning, but it also provided an interesting timeline of AV requirement timeline that clearly shows what you should be proven before generalizing AV testing, usage, and eventually, finally, forbidding human from driving (in 2070).
The objective of this paper is to discuss potential impacts, benefits, but also the weaknesses of the new technologies on the mobility performances. The deployment of the connected and automated vehicles (CAV) enters in this domain. It is important to keep in mind that improving this domain will have a significant impact on the infrastructure, the mobility capacity and efficiency, the energy management, the pollution level, and the user's health. This study is therefore structured to highlight efficiency in terms of safety, energy use, and traffic congestion issues.
The remainder of the paper is organized as follows. In the first part, the concept of ADAS, its limitations, and future developments are addressed. The next section focuses on the communication technologies, management, and modeling dedicated to cooperative applications. Then, the following sections analyze the benefits, advantages, limitations, and weaknesses of the CAV in terms of safety, energy and pollution, and traffic. A last part addresses the societal aspects. Finally, the topics require further research, and improvements will be identified and discussed.
2. Driving Assistance, Connected, and Automated Driving
2.1. The Automated Transportation System
The core concept of automation is to delegate repetitive and elementary tasks to a machine rather than a human. The machine is expected to achieve these tasks faster in a reliable and repeatable manner within a well-defined environment. Automation has transformed several working environments and has deeply impacted the relationship between the humans and their workplaces. The application to the transportation field was envisioned several decades ago, but the first applications are more recent. Since the 80's, the development of automated transportation systems has spread worldwide, initially from the universities, the defense sector, and few car manufacturers or road operators. The race to develop this technology has strongly accelerated in the last 10 years with software and service companies.
2.1.1. Level of Automation
The Society of Automotive Engineers has provided a standard terminology of automation in transportation (SAE, 2016, 2018) from the initial works of BAsT (Gasser and Westhoff, 2012; Gasser, 2014, 2016). The level of Automation depends on the entity who oversees: the control of the actuators, the monitoring of the environment, the supervision of the system, the fallback solution, and the limitation of the operating domain. They range from 0 to 5, i.e., from no automation to full automation. The details, specific operating domains, and levels of human interactions of these different levels of automation are given in Figure 1.
2.1.2. Road Map for the AV in the Next 30 Years
The deployment Road Maps of AV technology are consistent worldwide. However, there is a distinction between the passenger car deployment path and the freight/urban mobility deployment path (Figure 2). Freight and passenger vehicles do not have similar constraints and objectives. Automated technologies related to passenger cars aim at improving mobility, road safety, and as passenger comfort. Given the broad variety of situations these vehicles will face, car manufacturers deployed their technology in a progressive manner, balancing advanced functionalities with the operating domain. The first, and simplest, application is to deploy Highly AD in a highway situation. The highway domain has many characteristics: limited interaction between users, such as only one-way traffic to monitor and limited lateral interaction. Highways also have well-maintained and high-quality infrastructure such as lane markings and traffic signs; vehicles are very unlikely to encounter pedestrian. Automation on highway provides comfort to the driver as it is often a long trip.
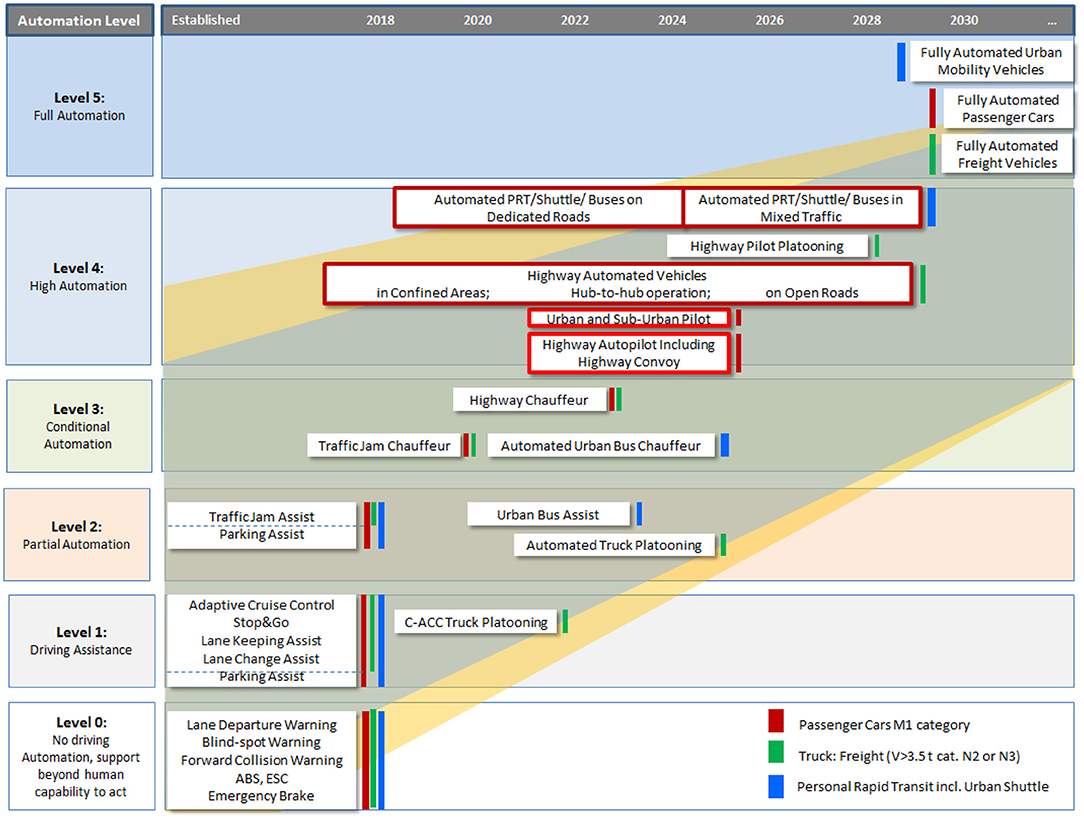
Figure 2. Deployment path for Automated driving technology (Connected and Automated Driving Roadmap – ERTRAC – 3/2019), vehicle freight, and public transport.
Automated technologies for freight and public transport have radically different design requirements. The ultimate objective is to remove the driver from the driving task, as the human salary is the largest operational cost in terms of fleet operation. Driver fatigue or drowsy driving is a safety risk for the road fleet transport industry. Legislation related to fatigue management introduces cost to fleet organizations. Heavy vehicle crashes receive tremendous media attention, which is another cost factor in addition to the societal cost of crashes.
Although long-distance freight transport share several automated features with passenger cars and follow the same progressive path, in a particular operational domain such as the highway, specific applications such as platooning are specifically design to reduce the truck's energy consumption. Short-distance transport, such as last mile delivery, public transport with shuttle, are drastically different, as they rely on a specific development of the AD system, noting that most new AVs are electric. These new forms of transports operate on a limited spatial domain with a predefined route and at low speed, and the technology deployment is not progressive. Shuttle manufacturers, for instance, aim at providing fully AV, without human intervention. In other words, they directly work on Level 5 SAE and ignore Level 3 and 4.
The availability of such technology is still discrete. Experiments of automated passenger vehicle or long-range transport (SAE level 4+) demonstrate that the technology is still not ready to manage all the situations, even in a specific domain, and is prone to failure (Dixit et al., 2016; Favarò et al., 2018). Automated shuttle's speed is very low and requires having an operator in the vehicle when it is moving on open road environment. A recent report from the Austroads (https://austroads.com.au/) forecasts the technology deployment and penetration in the automotive market in 2030. It concludes that despite a low adoption in the truck industry, the potential adoption and benefit may be promoted by the freight's operators; passenger cars may first benefit from Automated Emergency Braking and its derivatives and from connectivity, first with a cloud and then to provide C-ITS services. Highly AD functionalities will still be marginal in 2030.
2.2. Driving Assistance, Necessary but Not Sufficient
However, active applications up to the AV require very high reliability and robustness. A close and limited perception performance of the on-board sensors is no longer sufficient. Indeed, in SAE level 3 of automation, the human factor is still involved, and the driver can, at any time, be asked to resume the driving task. Unfortunately, to achieve this transition, it is necessary to guarantee a sufficient period of time for an inattentive driver to perceive the current environment as well as analyze and understand it in order to make the safest decision allowing to act on the vehicle actuators safely (pedals, steering wheel, and gearbox). Thus, to be informed of a disengagement from the AD system, it must have information distant enough spatially and temporally to allow it to analyze, interpret, and predict the future situation and estimate their level of risk. The mean take-over time (TOT) ranges from 0.69 to 19.79 s, most of the distribution being below 7 s (Zhang et al., 2019) with a strong time penalty in case of having a secondary task with a handheld device (Melcher et al., 2015; Zhang et al., 2019). With 7 s to take over control, on a highway, the vehicle will have driven more than 200 m, far more than any available automotive sensor. The way to have this distant knowledge is to use the knowledge available to other road users through connectivity (connection of a vehicle to the road infrastructure and vehicles between them).
2.3. Connectivity, an Automation Enabler
By its capacity to exchange information between vehicles, and to increase (spatially, temporally, and semantically) the perception of the AV, connectivity is the key element with which to ensure a high level of security. The connectivity will allow the AV to face critical situations that it would not otherwise be able to face (construction site, toll zone, etc.). Its implementation is based on a single system: the Cooperative Intelligent Transport System (C-ITS). One also talks about V2X communication. The operating principle is as follows: vehicles have on-board sensors that they use to automatically generate messages on the situations they encounter (Site alert, slippery road, and obstacle on the road). These messages are broadcasted in their immediate environment and can be received by other vehicles (V2V: vehicle to vehicle) and by road managers (V2I: vehicle to infrastructure). The infrastructure manager can also transmit information related to the operation of its road network (traffic, network viability, road information, etc.) to vehicles fitted with on-board sensors (I2V: infrastructure to the vehicle). From a technical point of view, today there are three modes of communication for C-ITS, and these can be mixed to enhance connectivity:
• Very short-range "direct" communication using RFID antenna (Mohsen et al., 2018) and Zigbee (Von Arnim et al., 2008).
• Short-range "direct" communications using the either 2.4 or 5.9 GHz frequency band (ITS G5 based on the IEEE Wifi 802.11p standard). This communication mean is currently used for VANET (Vehicular ad-hoc Network).
• Long-range communications, which use the cellular networks of mobile operators (3/4 G cellular).
A delay, interference, or modification of the information contained in the data frame will have a significant impact on the extended perception of the environment and on the quality of the data (imprecision, uncertainty, reliability, and belief), making them potentially unusable under penalty of producing a wrong decision and by extension an accident. The same goes for the management of automated platoons. The only way to ensure the stability of a platoon of more than five vehicles and to avoid the "accordion" effects is to use communications so that the "leader" of the convoy transmits his maneuvering intentions to the other "follower" vehicles. In this case, all the vehicles in the convoy will be able to react at the same time and not have any accumulation of delay due to the limited scope of the perception and the inability to be instantly informed of the driver's maneuvers. In the European project SARTRE, Volvo Car Corporation and its partners have developed an automated platoon with a truck as leader following by four cars driving in full automated mode at a middle speed (90 km/h max) with, in some situations, a very small vehicle inter-distance (up to 4 m). Recent research about the cooperative and automated platooning applications has been developed, studied, implemented, and tested in Latrech et al. (2018) and Schwab and Lunze (2019). Another interesting work (Gong and Du, 2018) proposes to manage CAVs in a platooning configuration but with mixed traffic involving human drivers. In Smith et al. (2019), the authors propose an approach to balance safety and traffic throughput issues in a cooperative vehicle platooning context.
2.4. Performance of Communication Technology for AD
In order to be able to anticipate risky situations and develop a co-driver for delegated and automated driving, it is first necessary to study and to implement the communication means to be able to quantify the quality, performance and limits of the communication means. This communication means will be essential in the development of alert applications and Extended Dynamic Perception Maps building. Demmel et al. (2014) performed work on the impact of the communication use to reduce collisions first in simulation and then in real conditions. This very important work has made it possible to demonstrate that with a rate of 25% of communication equipment, a very significant reduction of collisions is obtained. Then, a study of WiFi communications (802.11p) in real conditions revealed certain limitations of this medium in terms of range and transmission delay as a function of the relative speed between a transmitter and a receiver and the size of the transmitted frame. The study made it possible to calculate a global risk, thus allowing for prediction of the presence of a risky situation more than 7 s in advance (Demmel, 2012). This result is also important because, in the development of automated mobility means and in the detection of problems at medium range, it will potentially give the driver enough time to safely resume the driving task.
However, this work raises other questions. Indeed, the use of the number of collisions to assess the contribution of an inter-vehicle communication system for the safety of a line of vehicles was always more pessimistic than the use of a severity criterion using the Equivalent Energetic Speed (EES). The EES is the speed equivalent to the energy being dissipated in a collision. The study also shows that, while the number of collisions decreases significantly, the severity of the remaining accidents does not necessarily decrease. Additional studies based on a more optimistic criterion (i.e., the number of collisions) would be relevant in order to identify the exact causes of this behavior and show its repeatability in different use cases. This raises questions about the reaction and the decisions to be made by embedded applications. Is it better to allow collisions to minimize the rate of serious injuries, or is it imperative to minimize the number of collisions at the risk of fatalities?
Additional theoretical studies have been carried out (Giang et al., 2015) concerning the capacity and energy of message transmission for ad-hoc vehicle networks (VANET). Theoretical models have been developed in order to be able to approach spatial reuse in a precise manner, and to analytically calculate the optimal distance between the transmitters for good communication. Beyond this problem of theoretical network capacity, this study allows us to evaluate the limit capacity of a network to ensure a certain level of quality of communication for V2X applications by taking into account interference, the SINR (Signal / interference and noise ratio), and the error rate in the frames. All these models can be used as a first tool for sizing the communication means for safety applications (Giang et al., 2015). In fact, the more information is transmitted "practically" simultaneously in the same spatial area, the more message "collisions" will lead to loss of communication. It is therefore important to be able to propose dynamic VANET organization strategies to optimize the capacity and effectiveness of communications. The works of Zhang and Cassandras (2019) also showed how the use of communication systems in a traffic system could reduce energy consumption.
3. Impact of CAV and AD on safety
How could the mobility ecosystem be defined? From a high-level overview, this complex system can be summarized with the following definition: the road ecosystem is made up of humans/users who use/interact/cooperate with technologies and mobility means on dedicated and adapted infrastructures to move efficiently and optimally according to four main criteria (Safety, Energy and pollution, Mobility and traffic, Comfort and health) for personal or economic goals. For each level, domain, and key components (KC), research is currently ongoing to improve the efficiency of mobility means with new technologies.
Figure 3 gives an overview of this road ecosystem with a set of existing researches dedicated to the use, improvement, management, study, and modeling of the four key domains: the users (vulnerable and non-vulnerable), technologies (vehicles, sensors, hardware, and software architectures), infrastructure (new generation of road with new functionalities, communication means, smart road signs, etc.), and mobility's rules, regulations, and strategies. Of course, these four domains are inter-dependent and are an active part of cities, clusters of cities and their suburbs (agglomerations), and territories. The research works on each domain have for main task to optimize the mobility means with respect to three key issues (safety, energy, and mobility/traffic) and an additional one about comfort and health. Acting on one of these issues generates a positive or negative feedback on the other ones. OREED represents the 5 KCs (Obstacle, Road, Ego-vehicle, Environment, and Driver) needed to build the perception maps. It is important to keep in mind that the new generation of ADAS needs to find a relevant, efficient, and adequate balance between these issues. By reducing perception/decision/actions errors, CAVs could potentially reduce trauma and deaths from road crashes (Fagnant and Kockelman, 2015).
3.1. The Driver as the Main Contributing Factor to Crashes?
Recent statistics from the European Commission about road crashes (European Commission, 2019) show an important issue with 1 million road crashes per year, 1.4 million injuries per year, and 25,600 fatalities per year. Worldwide, road crashes cause 1.35 million fatalities and 20–50 million seriously injured or disabled each year [World Health Organization, Road Traffic Injuries, 2019 (https://www.who.int/health-topics/road-safety)]. From French statistics (Figure 4), it can be concluded that with the application of specific interventions, a plateau of fatalities has been reached for a couple of years with an average of 3,400 fatalities per year. But if injured people are taken into account, the figure is higher with 70,000 fatalities and injuries. It is a huge cost for the society.
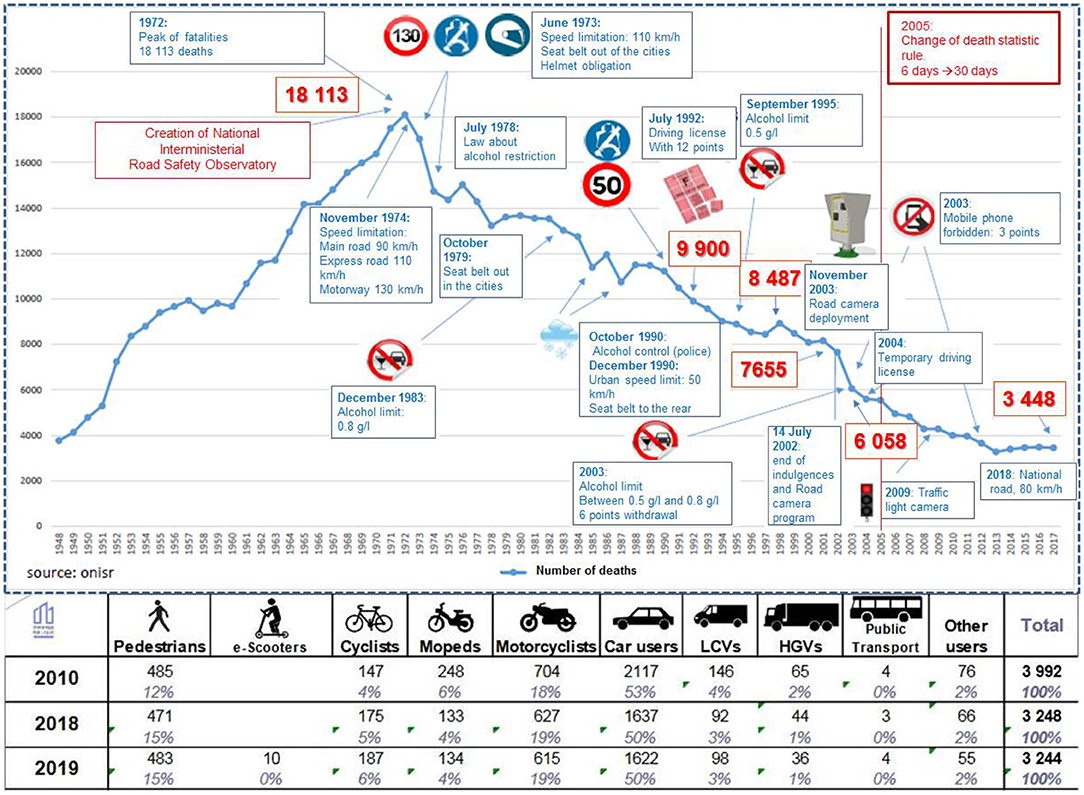
Figure 4. Road crashes fatalities and injuries trends in France (National Inter Ministerial Road Safety Observatory: ONISR).
What is the real responsibility of the human in these accidents? Based on the numerous statistical studies carried out over the past decade on the crash causation in North America, Europe, and Australia, it appears that the main contributing factor to road crashes is the driver. Human error is a contributing factor in 93% of crashes (NHTSA, 2008, 2015). Errors could occur at perception, interpretation, evaluation, decision, or action levels.
More precisely, the driver may lack visibility or may, due to the complexity of road situations, have a raw and/or hasty entry of information, leading to a misunderstanding and a wrong scene interpretation. This is of course adds to the problems of deliberate traffic violation, alcohol or narcotics consumption, driver inexperience, and problems of fatigue, hypo-vigilance, monotony, or even distraction. From NHTSA (2015) (US stats), crash reasons attributed to driver behavior mainly focus on Recognition errors (41%), Decision errors (33%), Performance errors (11%), Non-performance error like sleep, hypo-vigilance, monotony (7%), and other causes (8%).
The human-related errors demonstrate a huge potential in car fatalities and injury reduction. The initial idea was to propose embedded system in order to limit the impact of the human failure and to mitigate or avoid the collisions and/or ego-vehicle crashes. This is the topic of the L1 and L2 marketed functions. But it is also clear it is not enough. The second idea is to develop PADAS (Partially Automated Driving Assistance Systems) and automated systems to replace the driver in controlled road environments. But now, the question is about the real capability of the "copilot" to improve the road safety. In the European H2020 project MANTRA (Penttinen et al., 2019), the authors tackle this research question. In fact, the efficiency of automated systems will depend on how safely AD functions could carry out the driving task they are designed for. But in specific and critical situation, the automated system should have the ability to self-disengage to give back the driving task to the driver. Moreover, in L4 and L5, the system should guarantee it could both safely reduce human errors but also be tolerant and robust to system failures (sensors, hardware and software systems)(OECD/ITF, 2018).
Is it relevant to replace human driver by automated functions that copy human tasks and behavior? Under what conditions and configurations is it useful? According to Wickens et al. (2003) and ITF/OECD (2018), a first set of responses are given and are coherent with previous paragraphs:
• In dangerous situations or configurations that prevent the human driver from carrying out a driving task (alcohol or narcotics consumption, fatigue, hypo-vigilance, monotony, or even distraction).
• In specific situations where it is impossible for human driver to carry out the driving task [night-time sensing, strong adverse weather conditions (rain, snow, and fog)].
• In a specific situation where it is difficult for the human driver to carry out a driving task (scene misunderstanding and interpretations, very fast reaction times needed).
• Just for the pleasure to travel in an automated vehicle.
According to ITF/OECD (2018), the current deployment of AD functions seems to be mainly motivated by all these different measures improving road safety. The comparison of human and Hardware/Software performances in various aspects is summarized in the Figure 5. Safety considerations in automation levels L2 to L4 are the main issues in the safe handover from automated systems to the human driver when the system could not perceive/interpret/understand its environment with a high level of accuracy, confidence, robustness, and reliability. Another issue is how to efficiently manage the handover of the driving task when the vehicle is simply approaching the end of its Operational Design Domain (ODD). The following issues need to be addressed when assessing the potential safety effects of the use of AD systems (ITF/OECD, 2018; Noy et al., 2018).
• Task allocation: Which tasks are left to the humans, and which are handled by automation?
• De-skilling: Lack of practice or imperfect situational awareness leads to reduced skills and may hence cause delays for humans to carry out the driving tasks when required.
• Cognition: Lack of cognitive engagement in the driving task leads to lower levels of situational awareness, and hence longer reaction times if the AD function disengages.
• Control: Driving is a learned skill; less time spent driving can lead to worsening skills in handling the vehicle.
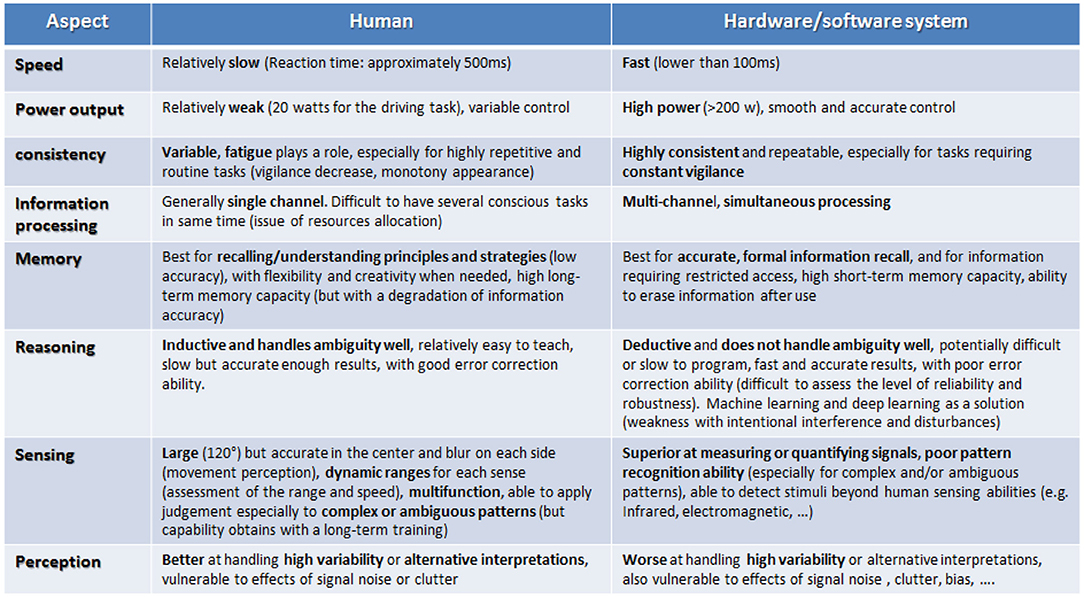
Figure 5. Fitts list of strengths and weaknesses of both Human and embedded system (sensors/computer/processing) across various criteria according to ITF/OECD (2018), Schoettle (2017), Cummings (2014), and de Winter and Dodou (2014).
However, these results are often questioned, in particular with regard to the methodology used, based on an analysis of the direct reports of accidents by police units which often judge drivers for insurance reasons. It would be entirely possible, by carrying out a detailed analysis, to find, in certain errors of perception or decision by drivers, an origin linked to infrastructure. However, there is still a fairly broad consensus that the driver is primarily responsible for accidents. Also, this is consistent with other areas of transport, such as aviation, where 85% of accidents are due to the pilot as shown by Li's work (Li et al., 2001) in 2001 on the basis of more than 27,000 accidents. Moreover, there is also the field related to the creation of new typologies of crashes because of the interaction between CAV and human. Less driver experiences reduce the control capacities of human drivers.
3.2. Driving Assistance as a Solution to Mitigate Accidents Risks
3.2.1. Advantages of CAV Deployment
It is therefore this observation that gave the idea that it is essential to reduce the number of accidents and to minimize the risk of a road situation. To reach this goal, it would be necessary to provide the driver with both informative (alerts, instructions, and expertise) and active (automation, delegation, and sharing of conduct) assistance. These assistances must then make it possible to remedy, at best, the limits of perception, action, and driver failures. These driving assistances necessarily involve a dynamic and adaptive perception of the environment and the construction of cooperative local and global dynamic perception maps integrating an assessment of the KC attributes. Having information on the state of all these KCs, then ensures a sufficiently comprehensive perception to be usable by a large number of on-board "short-term" security applications.
In Benson et al. (2018), the authors present results about potential reductions in crashes, injuries, and deaths from large-scale deployment of ADAS and by extension AV. In this paper, the technologies examined [forward collision warning (FCW), automatic emergency braking (AEB), lane departure warning (LDW), lane keeping assistance (LKA), and blind spot monitoring (BSM) systems] are estimated to have the potential to prevent a combined total of approximately 40% of all passenger-vehicle crashes, 37% of injuries that occur in crashes involving passenger vehicles, and 29% of all deaths in crashes that involve passenger vehicles. FCW/AEB and LDW/LKA systems were each estimated to have the potential to help prevent approximately 14% of all motor vehicle crash fatalities. However, FCW/AEB systems were estimated to be relevant to more than four times as many crashes and injuries as LDW/LKA.
The European project eIMPACT (eIMPACT project, 2008) (Figure 6) has shown the great safety potential of ADAS deployment. This project has studied and assessed the expected reduction in fatalities in Europe in the high penetration rate scenario in 2020, as well as the potential reduction if all vehicles and roads would be equipped. Results show that few systems have a real and strong impact on safety improvement. But some of them have a small impact. As a benchmark, each percentage reduction in fatalities represents approximately 230 fatalities. In the case of ESC in the 2020 high rate of equipment scenario (14%), 3,253 fatalities would be avoided at the penetration rate of about 75%.
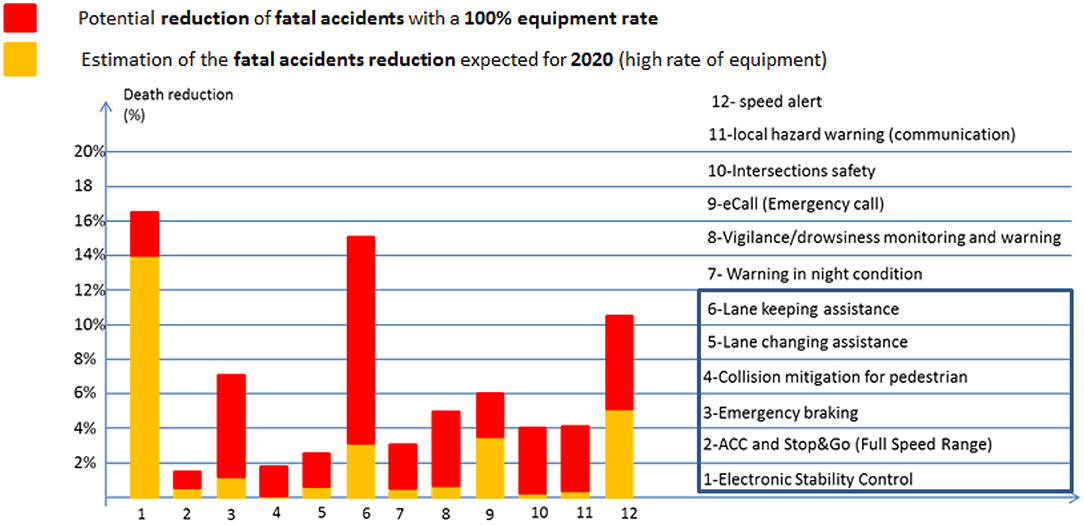
Figure 6. Benefit (in percentage reduction in fatalities) of 12 ADAS. Assessment made in eIMPACT project (eIMPACT project, 2008) for 2020 extrapolation scenario.
For the cooperative systems using infrastructure, eIMPACT also shown that the potential of eCall (implying 100% penetration for a fair distribution of infrastructure equipment costs) represented a significant reduction of fatalities and severe injuries. The difference in penetration rate explains the large differences between the 2020 high scenario and the potential scenario for some systems, such as the Lane Keeping System. But it is necessary to stay prudent with these data because this project made a 10-year projection.
From the existing applications 1 to 6 presented in Figure 6 and good monitoring/decision/path planning strategies, it is theoretically possible to design an efficient copilot function. For instance, in this study, which is based on simulation stages and in specific critical situations, a huge benefit can be remarked on in term of safety and fatality reduction if all the vehicles are equipped with the identified set of ADAS.
Fildes et al. (2015) provides some more recent results about the real impact of Low Speed AEB technology of the crash reduction. He highlights that this technology led to a 38% reduction in real-world rear-end crashes. Moreover, they affirm there was no significant difference between urban and rural crash benefits. Moreover, Low Speed AEB technology needs widespread fitment for maximum benefits.
Although these driver assistance systems are considered, the ideal solution for reducing the number of accidents, the first developments of AVs, representing the pinnacle of driver assistance systems, has faced many cases of accidents, some of them fatal. The works of Morando et al. (2018) using traffic simulation (Vissim software) showed that the number of potential safety conflicts will be reduced whatever the test scenario is. However, despite millions of kilometers traveled in automated mode, Waymo, Tesla, Delphi, Audi, and others have already faced serious accidents such as the fatal accident of Uber in 2017 which put its AV program on hold or even the Tesla accident in 2016. Noy et al. (2018) also claims that safety benefits of AD and human driver errors are often exaggerated and it should be more relevant to better analyze how the road infrastructure or the technology-induced human driver errors.
3.2.2. Is CAV Really Reducing Collisions and Improve Safety?
In 2015, according (Schoettle and Sivak, 2015), AVs had statistically five times more accidents than vehicles driven by human beings. It is worth mentioning that this study focused on prototype vehicles. Automated features are still under development, and the crash rate may therefore be higher. Also, according to Hsu (2017), an AV should drive more than 250 million miles without incident to be considered as safe as a standard vehicle. At the time of its fatal accident in 2016, the Tesla had only traveled 130 million miles. CAVs are still under development, therefor the access to real crash database involving SAE level 3 or more CAVs are limited. Trials, worldwide, depict the current state of the art in terms of automated driving systems. In Figure 7, Favarò et al. (2017) found that CAVs were over-represented in the front position during rear-end collisions. The detailed database from the California Department of Motor Vehicles (CaDMV) shows the collision often results from human driver misunderstanding of the CAV behavior. Events such as “emergency braking when nothing happens in the environment” or “stopping at an intersection when the roads are clear” are mentioned in the collision report. Such collisions occur mostly at low speed with no injuries reported.
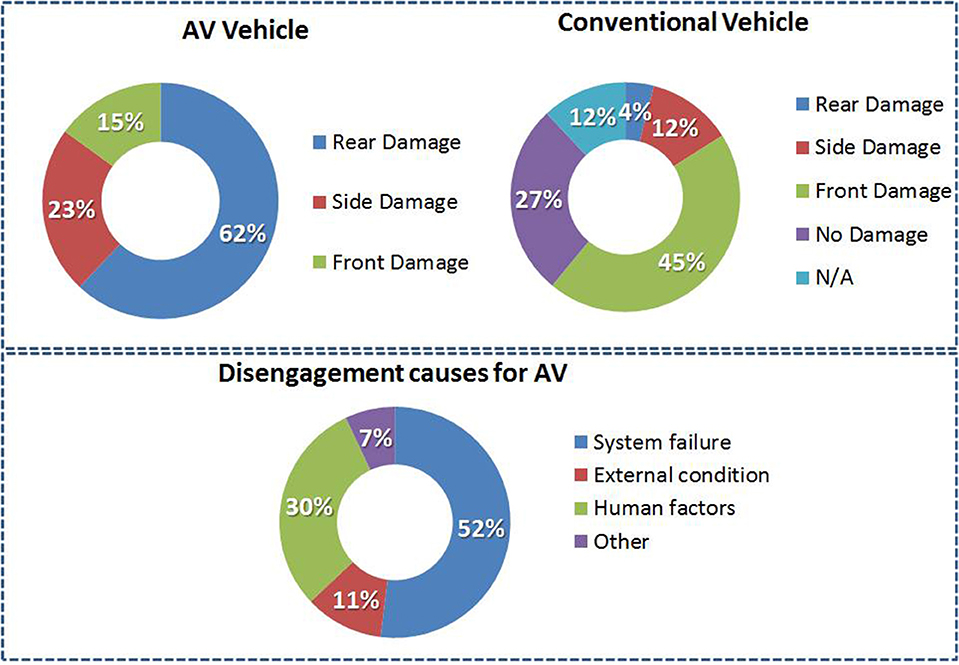
Figure 7. (Top) Automated vehicle crashes and damage locations (Favarò et al., 2017), and (Bottom) disengagement of embedded automated systems in reports from Dixit et al. (2016) and Favarò et al. (2018).
At the time of this study, the CaDMV (Californian Department of Motor Vehicles) published 26 reports. Moreover, several methods and approaches exist to estimate the impacts of AD and their limitations. In fact, three types of methods are used: the first approach employs historical crash data to investigate the potential impact of CAVs, the second uses integrated multi-level custom-built simulation framework to simulate CAVs, which generally incorporates traffic simulator, and the last one uses traffic micro-simulation software and its extensions. It is also mandatory for all experimenters to report disengagements in less concise reports. Dixit et al. (2016) and Favarò et al. (2018) (Figure 7) evaluate from a component of the dataset (n = 2,616, on nearly 6,000 now) events resulting in a disengagement. System failure (52%) and Human factors (30%) refer mostly to the understanding of the situation and the interaction between users. They are the most predominant reasons (reckless driver, cyclist, pedestrian, or take over from the expert driver because of safety and discomfort). The disengagement mostly occurs in an urban environment (street 49%) in good weather conditions (noted as clear 82%).
Of course, this result does not formally demonstrate that the Tesla vehicle is more dangerous in automated mode than in normal mode, but it demonstrates that one should not have blind faith in these systems.
Also, theoretical studies on the AV show the risks associated with changing the driving mode, which is very complex to implement in specific cases. It is indeed difficult to ask the driver to take over by hand at any time, even when the vehicle performs all of the driving tasks for him. This therefore poses a technical problem of interactions between humans and machines but also a legal problem of knowing whether one can really ask a passenger to become a driver during a journey while the vehicle is moving. Also, this transfer task requires at least 8 s to be completed (Lu et al., 2017), until the user is physically ready to regain control of his vehicle.
In Dixit et al. (2016), a study made to identify the type of accidents involving an AV (Figure 8) clearly showed that the main causes of the crashes involving an AV are due to system failure or driver inexperience.
Finally, from this point of view, V2X systems can provide a solution making it possible to partially or entirely overcome these problems as has been demonstrated in the work of Demmel (2012) and Gruyer et al. (2013).
To understand why the AV can, in some cases, be more dangerous than a conventional vehicle, it is necessary to give details on its constituents that can lead to a danger.
• Embedded Sensor technologies: Enough reliable new technologies are yet to be developed (laser depth camera, Event camera, multi-modal Radar, etc.), and there are issues about sensors operating in adverse weather conditions (heavy rain interferes with roof-mounted laser sensors, snow can interfere with cameras, etc.).
• Operating safety: Monitoring of embedded systems (computers / sensors / actuators / algorithms) is necessary to detect and to identify malfunctions and failures. Even just a minor glitch could cause worse crashes than anything that human error might bring about.
• Sensitivity to hacking: Getting into the vehicle's software and controlling or affecting its operation would be a major security issue.
In AD applications, the more critical part is the sensor part. Without an adequate perception level, it will be impossible to achieve an efficient, reliable, and safe automated driving task. But what about this sensor layer and about the capabilities of the existing technologies? Are they reliable enough in all conditions and road situations? The next section tries to give an answer.
3.2.3. Is Embedded Sensor Technologies Enough to Guarantee Data Quality?
From Figure 9, it is possible to have a clear overview of the different frequency/wavelength intervals used by the different information sources technologies with their potential overlapping. But now, what are the issues encounter by each technology in each frequencies bands? The Figure 10 gives a first overview of the advantages, disadvantages, limits, and weaknesses of the different technologies of embedded sensors. It is clear that only one type of sensor could not guarantee a level of quality enough high for the AV deployment. The development of new technologies, new data processing and filtering, and new multi-sensors data fusion architecture will be essential.
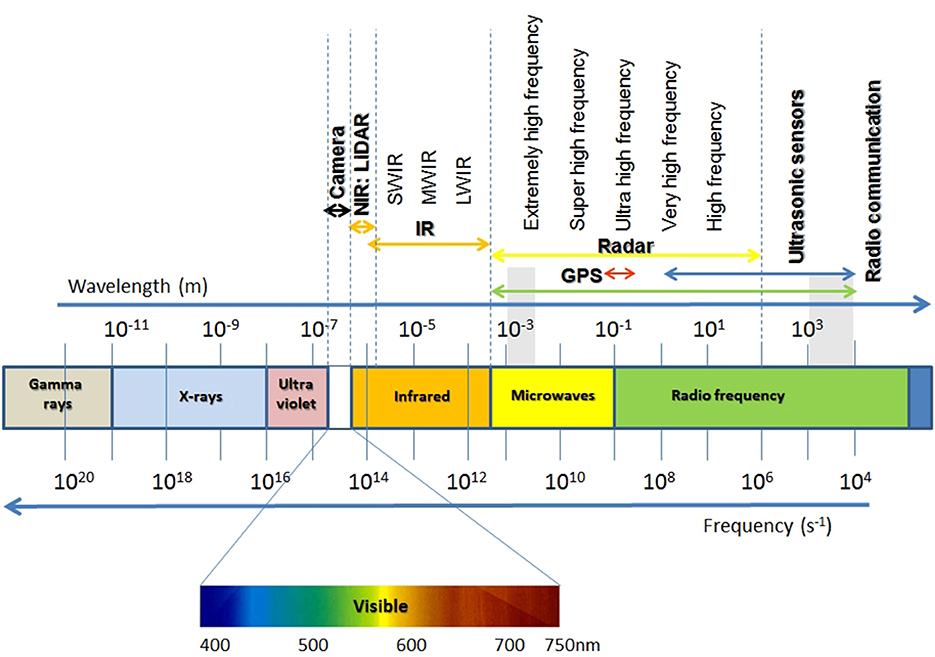
Figure 9. Electromagnetic spectrum: a better understanding of the operating range of each type of information sources used in CAV development.
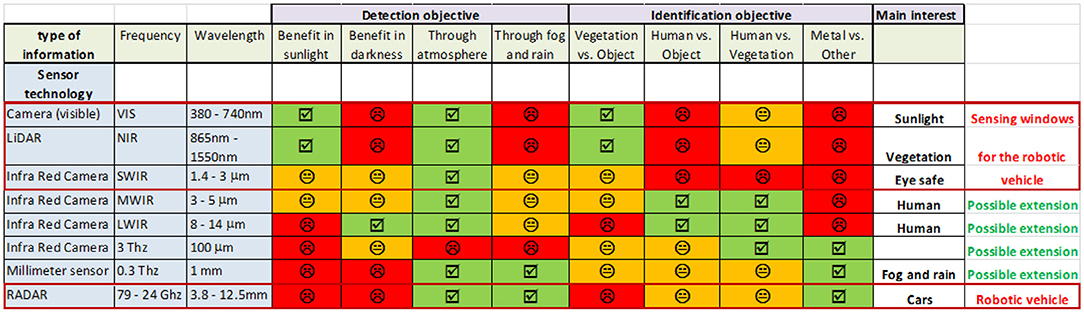
Figure 10. Trends of perception technologies for CAVs. Green color means Yes, Red color means No, and Orange represents Maybe (Tschudi and Girardin, 2018).
In Gruyer et al. (2017), a study is proposed about the embedded sensors technologies used in perception, information processing and modeling stages. In this paper it is clearly demonstrated that sensors and perception methods/approaches/modules are critical stages in the AD application development. In this study, the weakness and limitations of sensors and perception architecture are highlighted. In Brummelen et al. (2018), a complementary survey of current and future works and technologies of perception applied to the CAV is also proposed. These two review papers give a clear overview of the main issues and challenges to be addressed to guarantee a good enough perception quality for CAV.
Recently, new methods and applications have been proposed for two complementary objectives. The first objective is about accurate and certain objects and situation detection and identification. The methods which are now developed are mainly based on the use of IA and Deep Learning methods to be more efficient in the environment segmentation and semantic area recognition. Unfortunately, even if, in the majority of cases, the results are good enough, some specific configurations (intentional or unintentional disturbances) provide failures. Moreover, these methods are based on a training stage. This implies that applications learn only the features included in the training dataset, and it needs to use a very large and representative database. The second objective addresses the fault, drawbacks, and failures detection and identification.
3.2.4. Is Complexity of Data Processing Too High?
In this context, is there any hope that the AV will become sufficiently safe? If a comparison of this objective is made with what is achievable in a benchmark sector (aviation), it is clear and obvious that the AV already contains much more on-board technology than an airplane. A current premium vehicle can thus contain up to 100 computing units, which is more than an Airbus A380; a Boeing 787 Dream liner will contain 6.5 million lines of code, and an AV can reach 300 million showing that the complexity is well superior (McCandless, 2015). This difference in the number of lines of code, resulting from the differences in terms of the environment, interactions with other users and the types of objects to be detected, does not mean that as long as security is treated at the same level. For the moment, it is likely that the developers of AVs will focus on the feasibility of autonomy and that safety will come later. Many researchers are thus satisfied with 95 to 98% efficiency of their algorithms when the reliability should reach 99.9999% to be identical to that of a human being. From the estimate of ARM (2019), there are probably 1 billion lines of code that will have to be embedded to obtain a fully automated car.
4. Energy, Pollution, and Traffic: Complementary and Additional Challenges to Safety
Safety is not the only important issue, and for the last 20 years, a great effort has been set on the energy management and the traffic regulation. On one side, we are facing global climate change induced by human activities, especially due to greenhouse gas emissions and on the other hand, air pollution became a serious threat to health. Every year, according to the WHO (World Health Organization), 4.2 million of fatalities are related to outdoor air pollution and a share of this pollution is due to the transportation sector.
Concerning CO2 emissions, for instance, more than 60% of the CO2 emissions are due to the SEDAN (in France more than 50%) (Figure 11). So an international decision has been taken to reduce the CO2 emissions up to 130 grams per kilometers and this limit is going down year by year. To reach this goal, road KCs must be addressed. These KCs involved the ego-vehicle, the road, the obstacles, the environment, and the driver. The vehicle consumption is considering the three firsts. ADAS must consider conflicting parameters in the cost function to optimize as road safety, energy consumption, and time spent in the traffic. Moreover, in non-AD systems, the driver behavior could have a significant impact on the energy consumption, and the environment KC could provide an estimation of visibility distance and infrastructure singularities.
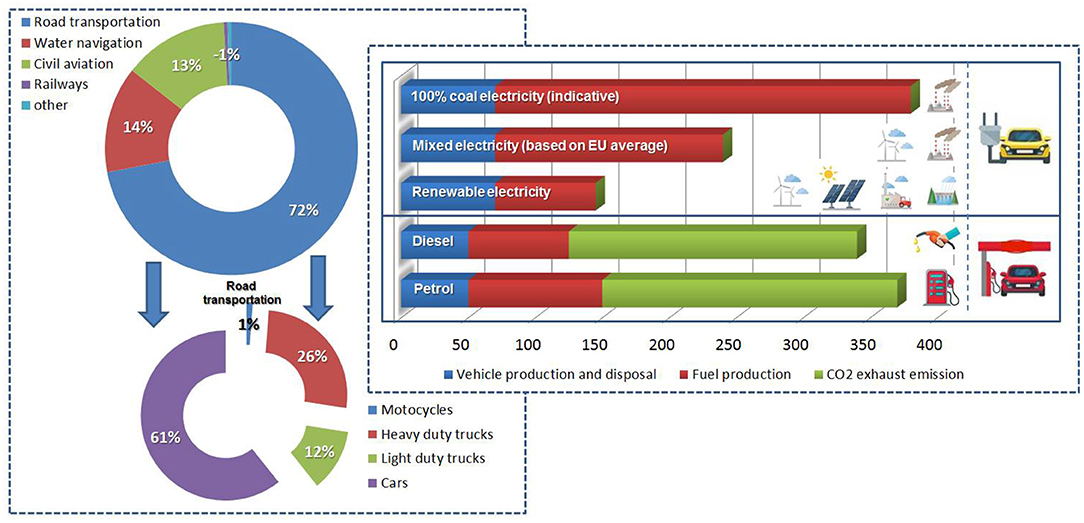
Figure 11. CO2 emission in the different mean of transport https://www.europarl.europa.eu/news/en/headlines/society/20190313STO31218/co2-emissions-from-cars-facts-and-figures-infographics. (Left) Emissions breakdown by transport mode (2016); (Right) Range of life-cycle CO2 emissions for different vehicle and fuel types.
More and more the road network suffers from a strong growth of the vehicle density and of course of the traffic jams. So now in the development of the new ADAS, it is necessary to solve a multiple criteria and constraint equation involving the safety, the energy consumption, and the traffic. Unfortunately, these three constraints are very dependent.
Moreover, in the development of these new services and solutions, we need to estimate and to use the five KCs of the mobility system.
Then, to summarize, a very interdependent and complex system has to be handled with not only one safety objective but three interdependent objectives. So, in the development of the new ADAS, it is necessary to find the best balance between these three constraints to minimize the energy consumption and maximize the traffic efficiency without compromising (and even improving) the road safety.
4.1. Eco-Driving for Fuel Consumption Optimization
Recent research and studies over the past decade have shown than eco-driving applications could have has a major impact on fuel consumption for automated vehicles. In these recent studies, the use of real-time “eco-coaching” for human driver has shown a significant reduction of consumption between 10 and 20%. In the virtual copilot system, it could be relevant to apply a layer in the decision process in order to follow eco-driving best practices. Nevertheless, in an automated vehicle, some automated driving functionalities could affect the fuel economy even with the using of eco-driving modules. These functions that impact the eco-driving capability include adaptive cruise control, V2I communication, and V2V communication (for instance for platooning, risk assessment, extended perception, and warning). Studies have proven that a direct result of these functions is a reduction of the braking. For thermal motor, braking is a direct waste of energy, so any reduction in braking increases energy efficiency. With electrical engines, it is a bit different because of the regenerative functionality which collects the braking energy in order to reload the batteries.
Nevertheless, fuel consumption reductions could plateau due to congestion caused by the slower driving speeds and smoother accelerations inherent to eco-driving practices (Zia et al., 2016). In her researche, Freitas (2016) obtained a solution that allowed her to save up to 20% of energy.
In Mersky and Samaras (2016) some results show that AV following algorithms designed without considering energy efficiency can degrade fuel economy by up to 3%, while the use of specific and well-tuned strategies focused on energy efficiency could provide equal or slightly better results (up to 10%) than the existing EPA (Environmental Protection Agency) fuel economy test results. This remark suggests the need to propose new approaches in fuel economy tests to better take into account the deployment of connected and automated vehicles. In addition, this tells us very clearly that the development of automated vehicles must not only be done from the safety point of view but must also take into account, very seriously, the energy management aspects.
4.2. Transportation Emissions on the Rise
Significantly reducing CO2 emissions from transport will not be easy, as the rate of emission reductions has slowed. Other sectors have cut emissions since 1990, but as more people become more mobile, CO2 emissions from transport are increasing. Efforts to improve the fuel efficiency of new cars are also slowing. After a steady decline, newly registered cars emitted on average 0.4 g of CO2 per kilometer more in 2017 than the year before.
To curb the trend, the EU is introducing new CO2 emission targets, which aim to cut harmful emissions from new cars and vans. MEPs adopted the new rules during the plenary session on 27 March. On 18 April, MEPs also approved a proposal to cut CO2 emissions from new trucks by 30% by 2030 compared to 2019 emission levels.
Makridis et al. (2020) has made a recent micro-simulation about the impact of AV and CAV on traffic flow and CO2 emission. The results show that with traditional car-following models AVs seem not facilitate Traffic Flow, and generate the highest CO2 emission values per kilometer. CAVs increase the capacity of the network and therefore during peak hours, they generate more emissions in absolute values. Moreover, the differences in emissions per kilometer driven between CVs (Conventional Vehicle), AVs, CAVs do not exceed 6%. Unfortunately, this work is questionable because it uses Gipps model (collision free) and the AIMSUN's micro-simulation, which is not really reliable for this type of study. Moreover, the communication is too simple (also perfect communication). About acceleration parameters, the authors use different values for the three types of vehicles.
4.3. Passenger Cars as the Main Polluters
CO2 emissions from passenger transport vary significantly depending on the transport mode. Passenger cars are a major polluter, accounting for 60.7% of total CO2 emissions from road transport in Europe (Figure 11). However, modern cars could be among the cleanest modes of transport if shared (as opposed to driving along), but with an average of 1.7 people per car in Europe, other modes of transport, such as buses, are currently a cleaner alternative.
In this context, the main levers for action by driver assistance systems to reduce the impact on the environment are: The number of kilometers traveled, the efficiency of the vehicle-driver couple and external factors (traffic, road geometry, weather, etc.). Several studies have shown, in this sense, that driving assistance can reduce energy consumption by 5–15% under real conditions of use (Freitas, 2016; Fontaras et al., 2017). Also, optimizing the speed profile of an automated vehicle to ensure that it practices eco-driving is also an important step in reducing its energy use. Then, down-sizing reduction works on vehicles make it possible to reduce the vehicle mass and thus the size of the engine without compromising the passive safety of the vehicle. Finally, innovative mobility services such as car sharing or ride splitting make it possible to quickly reduce the number of kilometers traveled while limiting congestion. While these services improve the impact of vehicles on energy consumption, there is no evidence to suggest that automating them would further improve the situation. Several studies have thus been initiated to understand the impact.
Among the interesting solutions at this point, platooning can be cited. But Platooning applications must be based on communication systems to be effective. This strategy, which consists of placing several vehicles in a line with low inter distances, minimizes aerodynamic efforts. Consumption of follower vehicles can thus be reduced by up to 16 and by 8% for the leading vehicle (Lammert et al., 2014; Muratori et al., 2017). In Tsugawa et al. (2016), the relationship between the fuel saving improvement and the gaps within the platoon is given and confirm the previous results. These gains can be further increased by introducing automated vehicle control with low latency communications.
The works of Zia et al. (2016) and the US energy administration shows that many factors implemented in AVs could indeed reduce the total consumption of vehicles, but certain factors can also affect this consumption, such as vehicles maintained higher on the highway, new features present in vehicles (large screens, connectivity, etc.), the increase in journeys due to the reduction in costs and therefore the increase in demand and the new uses calling for new customers in the form of a mode transfer transport. The works from (Vahidi and Sciarretta, 2018) also assessed how AD could influence energy efficiency on a theoretical and mechanical point of view.
4.4. Is Electric Mobility the Solution?
More and more, the solutions to reduce the carbon impact of transportation means seems to be the hybrid, hydrogen, or electrical technologies. In terms of electrical technology, recent research has provided relevant and future solutions, such as the additional batteries rental concept. This solution is proposed by the start-up EpTender (https://eptender.com/). Moreover, new battery technologies have appeared, such as batteries with a solid electrolyte (Pr. Goodenough). This solution seems to have several advantages: an energy density three times higher than the lithium-ion batteries, no problem of battery burning, more robustness, compatibility with fast load systems, and the ability to operate up to –20 degrees Celsius. This new generation of battery with solid electrolyte is made from glass with an alkali metal anode that can be composed of lithium, sodium, or potassium. It is clear that the capacity and efficiency of the new generations of battery and their ultra fast loading will be strongly improved in near future (Figure 12). Nevertheless, it is relevant to consider the method of energy generation; for instance, in Australia (Queensland), a small electric car (Renault Zoe) could pollute more than a four-wheel Drive diesel car (Figure 11). Also, it is interesting to remark that a human driver has an energy consumption of approximately 2 watts while the embedded functionalities necessary for CAV will be upper than 200 Watts. In Baxter et al. (2018), an estimation made with only one configuration taking into account embedded sensors and computer energy consumption is provided. This consumption is 200 watts for 3 LiDAR, 2 RADAR, 1 camera, 1 IMU with a GNSS, and 1 computer. For instance, the OUSTER's LiDAR 3D has a consumption between 14 and 20 watts. If we apply the sensor configuration (28 sensors) expected for L5 vehicle presented in Tschudi and Girardin (2018), the same energy consumption of at least 200 watts is obtained. So, from the energy point of view, the AV will be less efficient than human.
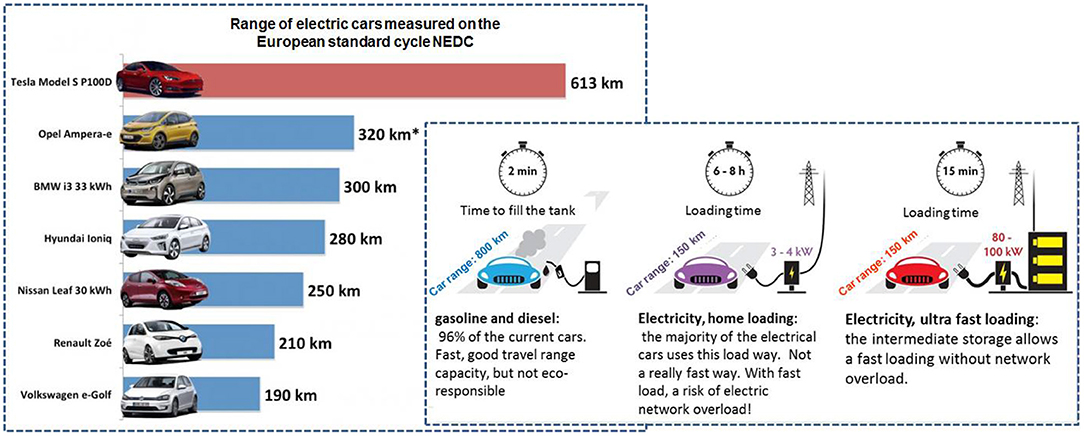
Figure 12. Is electric mobility good enough in term of autonomy and energy loading time? (Left) Current electrical car autonomy. (Right) Different ways to load car battery with associated waiting time.
4.5. And What's About the Impact of CAVs on Traffic?
The approach to optimally manage traffic using communication technologies is also presented to overcome a number of difficulties that can be caused by AVs in terms of congestion and traffic.
A position aspect of CAVs on traffic congestion has been evaluated by Condliffe (2017) and Stern et al. (2018). In their experiment, they use 20 vehicles moving in a loop with only one automated vehicle. The goal was to try to minimize the traffic congestion effect. The authors of this experiment have shown that with a simple control law and a well-chosen strategy, the traffic congestion fully disappeared. Moreover, some other studies have proven that, in specific situations, the CAVs could improve the capacity of a road network and reduce the congestion and the wave effect of congestion (cooperative and connected platooning). In terms of cooperative platooning, however, some questions still have to be solved. Which strategies have to be applied in order to manage platoon entrance/exit? Which strategy must be developed and applied in order to change the platoon leader? How do we efficiently manage the interaction and cooperation within and outside of the platoons? (Konstantinopoulou et al., 2019). Which strategy do we use to negotiate and manage compromises in singular and complex situations like intersections and roundabouts?
Nevertheless, traffic could be coordinated more easily in urban areas to prevent long tailbacks at busy times. Commute times could be reduced drastically.
Speed limits could be increased to reflect the safer driving, shortening journey times.
The theoretical study of Friedrich (2016) is dedicated to the impact of AV on traffic and more specifically traffic capacity and stability. Macro traffic models have been used to show that traffic capacity would be increased up to 36% if all vehicles were automated. A different study from Lu et al. (2019) has found similar figures (23% increase of capacity) but using traffic micro simulation.
On the one hand, we are facing new situations where vehicles can have no occupants. If people who commute by car then send their car home because parking is too expensive near their place of work, then the traffic demand could therefore almost double. Some jurists (Muoio, 2017) have consequently already proposed the introduction of a tax on the kilometers traveled by AVs (0.025$ / mile).
Also, it is well-known that drivers do not respect safety distances in large cities. However, the AVs will initially be required to comply with them, which again risks increasing the length of congestion queues. Second, AVs will have more difficulty interacting with vulnerable users, thereby slowing traffic in areas with high pedestrian density.
In terms of the human factor point of view, automation of the mobility will reduce the driving skills of human drivers who therefore risk bringing additional problems in the event of mixed traffic whether it be in terms of safety, energy consumption, or fluidity of the traffic. For instance, recent studies (Figure 13) shows a worse view of the expected traffic conditions for the next decades with partial CAVs deployment rate. With mixed traffic, the traffic congestion will be worse until a high level of CAV deployment. The same remark can be done for the average travel time which will not give a significant gain.
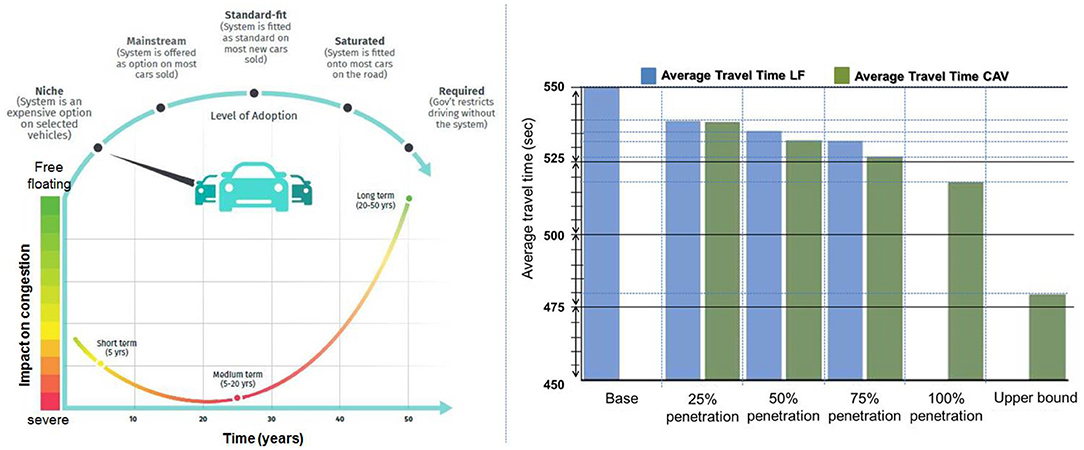
Figure 13. Is CAV deployment an interesting solution in order to optimize traffic? (Left) Impact of CAVs deployment on traffic congestion; (Right) Impact of CAV on travel time saving (ATKINS, 2016).
Finally, the deployment of automated vehicles will also allow many people with reduced mobility, and who could not drive and move easily, to have easier access to mobility. This will also be the case for teenagers and elders. Consequently, the risk of the large-scale deployment of the CCAM could be to strongly increase the number of vehicles on the roads network and to aggravate the traffic problems.
5. Discussions
5.1. Trusting the Virtual Copilot and Its Capabilities
Watching the impact of the AVs on various objectives such as the environment, safety, or even traffic conditions independently provides initial information to understand the role of this technology. However, these same objectives are strongly correlated. A change in traffic conditions will immediately have an impact on energy consumption and road safety.
For example, when the technology for driving autonomously improves, the safety requirements to use them will decrease accordingly, generating new safety-related risks, such as very low inter-vehicular distance. This will clearly increase the road traffic capacity and reduce the energy use but will generate accidents risks. An interesting anthropological approach has been undertaken by Pink et al. (2020) to propose new frameworks for trust in AD. About these CAV capabilities and impact on traffic rules, the question is how to tune the copilot system and how to trust in this system? (ATKINS, 2016). For instance, if a high assertive behavior is applied then it means that a great confidence is given to the copilot, in this condition, the safety distance can be strongly reduced. What happens in case of a car failure? On the other hand, if an extremely cautious behavior is applied, then the capacity of road traffic should collapse and serious congestion situation could occur. Another time, it will be necessary to find the good balance between safety, energy, and traffic criteria.
5.2. Development of a New Generation of Road Infrastructure
In the deployment of the future automated mobility means and HQoS communication for transport applications and services, it is important to address the digital and physical infrastructures and what their future configurations and features could be.
The importance of the physical infrastructure and its link with the digital infrastructure has been emphasized by Ehrlich et al. (2016). Indeed, to move safely, AVs need to access accurate knowledge in terms of which road they are traveling on. AVs are expecting from infrastructure that it fulfills some requirement in order to maximize safety. In fact, the infrastructure needed to be “readable” by the vehicle. Ehrlich et al. (2016) investigates the concept of High Quality of Service Highway (HQoSH), and the main infrastructure features (and their metrics) were identified. Among them, lane marking, traffic light status, and road sign readability can be quoted. Moreover, road attributes (curvature, cant, slope, and skid resistance), geometric and weather visibility, and singularities detection (ruts, potholes, ruptures, and cracks) also are relevant. All these features are useful for vehicle stability and guidance.
At first glance, the technical challenge is to develop the relevant indicators that link the state of these infrastructure elements with the sensing capacities of the automated vehicles, and to develop maintenance strategies to maintain these indicators at a suitable level. In addition, because of the dynamics of change of certain infrastructure components, in particular due to weather conditions, HQoS of communication is again the solution to obtain a dynamic map of these indicators. This digital map is thus an additional layer of the physical infrastructure, initially designed for the human driver. One might speak about a hybridization of the physical infrastructure.
This is where the problem becomes complex. Due to the constant evolution in sensor development (or the absence of technical standards of AVs technologies), it is therefore difficult to develop standards for the infrastructure that follow the same development as the strategic goals of road owners, namely, reducing the direct costs and the environmental footprint of existing road infrastructures and road transport. There is thus a permanent conflict between two policy objectives: road asset management and road innovation. This conflict can be solved by changing the regulation or by finding innovative solutions, which combine cost reduction and problem resolutions (Hautière et al., 2017).
This is the aim of the 5th Road Generation (R5G) research program (Hautière and Bourquin, 2017) (Figure 14) developed by University Gustave Eiffel (r5g.ifsttar.fr), linked with the Forever Open Road program (www.foreveropenroad.eu). These programs aims at designing and building demonstrators following a system approach of a new road generation that takes into account all challenges related to road infrastructures and gathers the best ideas all over the world (Lamb et al., 2012; Hautière, 2019). While aiming at easing the deployment of electric vehicles and automated vehicles, the goal of the program is to reduce the total cost of ownership of road infrastructures of 30% by 2030 while meeting the multiple goals of the different societal transitions.
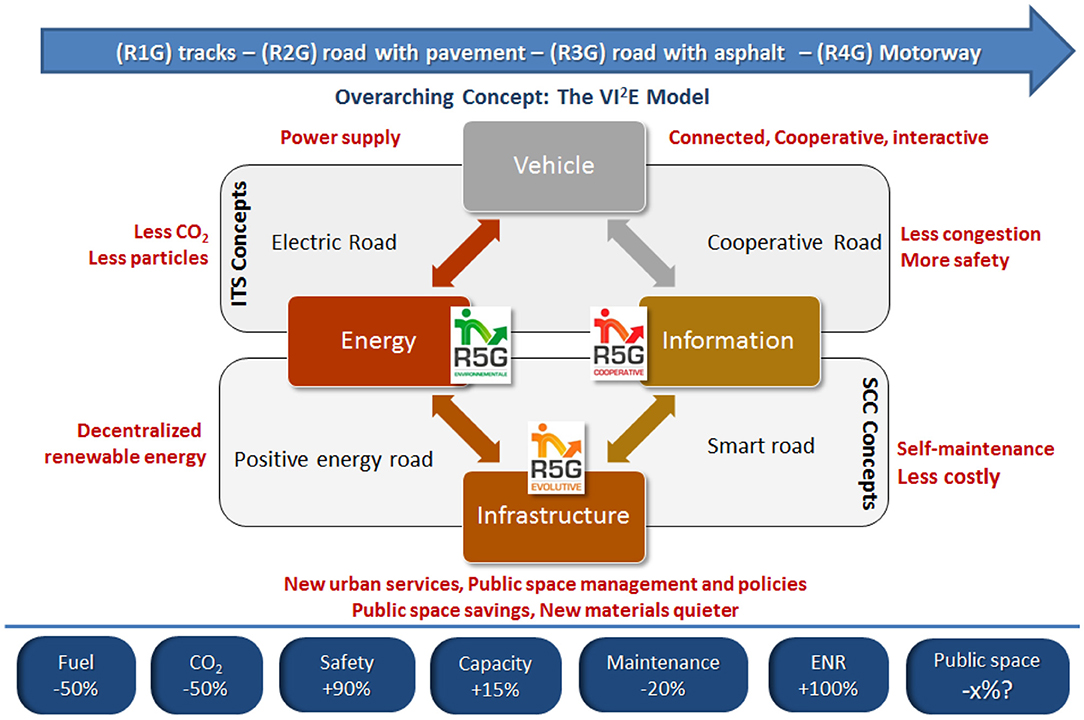
Figure 14. R5G, the 5th Road Generation for a smart, sustainable, and resilient infrastructure useful for CAVs deployment.
The strategy is to put into synergy three elements in a gradual manner: The Automated Road, the Adaptable Road, and the Resilient Road. The timing is as follows: Individual components testing from 2010 to 2015, subsystem experimentation from 2015 to 2020, and system integration after 2020.
5.2.1. The Automated Road
The challenge of the automated road is to make the most of technologies to manage road traffic, and to support the development of new forms of mobility like CAVs.
The first step is to put in place new traffic management strategies. These are based on the growing connectivity and automation of vehicles (Ehrlich et al., 2016; Ehrlich, 2017a,b) in order to improve the performance of the road system, increase its profitability, and make journeys safer and more reliable. They make it possible to optimize the use of infrastructures by taking advantage of cooperative ITS, vehicle convoys, tariff variations or even personalized traffic information. Among the current challenges, three can be quoted: The first one is the construction of collective optima combining collective measures of traffic management and individual nomadic services, the second one is about the construction of reliable data chains, and the last one address the coexistence of automated and conventional vehicles.
The next step is to deploy roadside systems merging data from sensors integrated in infrastructure and on-board vehicles. The goal is to be able to use existing sensor systems while integrating new generations to build more accurate and less expensive traffic data acquisition (Besnier et al., 2014). This requires open and standardized interfaces to put suppliers in competition and cooperate between actors independent of the supplier. The challenge is to avoid rapid obsolescence of investments.
Behind these objectives lie legal obstacles that must be removed by building a regulatory framework adapted to the deployment of cooperative ITS and traffic automation. Finally, it is a matter of supporting the players in their transformations through a harmonized deployment framework, involving managers and training the players in new skills.
In Carreras et al. (2018), the authors propose a simple classification scheme of the features, functionalities, and services coming from infrastructure and improving the quality of the CAV services. This proposal of classification is one of the topics developed in the H2020 European project INFRAMIX (https://www.inframix.eu/). This modeling starts from two observations: Firstly, the perception coming from embedded sensors and perception modules is limited by the capability of the sensors. Secondly, the road infrastructures have growing capacities. Effectively, road infrastructure operators have implemented a lot of sensors on the infrastructure in order to monitor both weather and traffic conditions. It is clear that this information could be useful in order to improve the perception of automated vehicles.
This classification proposes, like for SAE levels, to harmonize the capabilities of a road infrastructure in the CAV context. These levels are shared into two main categories. The first one provides the road infrastructure capabilities in their current state (conventional infrastructure) and the second one supplies the future digital capabilities. This classification, and its levels, is called ISAD for Infrastructure Support for Automated Driving (Figure 15). These levels have main objectives with which to provide the “readiness” state of the road network for the deployment of automated mobility (mainly in highway areas). With level A and in mixed traffic, the AVs can been seen as variables for traffic optimization.
5.2.2. The Adaptable Road
As not all new mobility offers are likely to be deployed, the challenge of the adaptable road is to provide a flexible and profitable road system, capable of adapting to future travel demands. The key solutions to make the road more scalable are of different kinds.
The first step is to develop new methods of road construction. These will be prefabricated in the factory with modular solutions (de Larrard et al., 2013). These techniques indeed offer rapid replacement solutions in the event of damaged sections while natively integrating quality control procedures. These processes will be based on low-carbon, recycled materials requiring little energy for their implementation and self-repairing to allow increased longevity of the heritage (Blanc et al., 2019).
The next step is to develop wearing courses offering a good compromise between rolling noise, water uplift, rolling resistance, and grip, which 3D printing technologies will allow. Robotics or automated methods will allow increased requirements in terms of maintenance and upkeep, including under traffic. Solutions include the inclusion in pavement structures of sensor mats to monitor road conditions (Barriera et al., 2020).
Finally, the road must be able to integrate, if necessary, supply systems and renewable energy capture to recharge electric vehicles (Laporte et al., 2019; Vizzari et al., 2020) and power equipment, such as roadside units, signage, or public lighting (Shahar et al., 2018).
5.2.3. The Resilient Road
The road must also be more resilient to the effects of climate change. Among the actions to be taken, the following actions might be highlighted:
• Identifying the needs of managers in terms of climate.
• The harmonization of climate data at European level.
• Assessment of the economic and social impacts in the event of disruption.
• The identification of possible network vulnerabilities.
• Establishing future service levels for road networks.
• Identification of key technologies contributing to mitigation objectives.
• Investigation of cost-effective adaptation processes.
In this busy agenda, a number of off-the-shelf solutions can already be listed, such as drainage systems capable of adapting to an increase in the frequency and severity of precipitation, thermo-regulated pavements for reduce surface freezing and the effects of extreme heat (Touz and Dumoulin, 2015; Asfour et al., 2016), including in the city of depolluting materials (Le Pivert et al., 2019).
5.3. Cyber-Security, Failure, and Fault Detection and Identification
Additionally, cyber-security issues are more prominent, such as those relating to interference generation and malicious deception. These attacks, which can have several objectives (data theft, intentional failure, takeover, etc.) are becoming more and more common and require the implementation of increasingly complex security strategies and protocols. Gupta et al. (2020), Alnassera et al. (2019), Will et al. (2017), Kaur and Kad (2015), and Mokhtar and Azab (2015) give an overview of the concept of security and the underlying issues for CAV using VANETs. The security constraints of V2X can be divided into five categories: availability, data integrity, confidentiality, authenticity, and non-repudiation. However, in V2X, the most dangerous attacks are those that target information availability. This is because they prevent authorized users from accessing information. It is obvious that this type of problem will be a challenge is term of CAVs security and will have a significant impact on the efficiency of the CAVs as well as on the safety and traffic aspects.
5.4. Societal Impact of CAD
CAD will not only impact technology-oriented aspects but also the everyday lives of users and people living with them. A first list of already identified and potential advantages of AD cars has been developed:
• Impact in terms of security, safety, accessibility, employment, emissions reduction, and management required time should be considered.
• Without the need for a driver, cars could become mini-leisure rooms. There would be more space and no need for everyone to face forwards. Entertainment technology, such as video screens, could be used without driver distraction.
• People who historically have difficulties with driving, such as disabled people, older citizens, as well as the very young, would be able to experience the freedom of car travel. A study from (Spence et al., 2020) generalized this question of mobility for all people. The AVs will change the way people are moving and their conclusion is that AVs might be the most used travel means for local travel in 2050s,
• AVs could bring about a massive reduction in insurance premiums for car owners.
• Possible reduction of the social health insurance cost (less fatalities and injuries).
• Self-aware vehicles would lead to a reduction in car theft, but it will be necessary and essential to take into account hacking issues, possible misuse of this technology for terrorism, and illegal activities. For instance, the works of Boloor et al. (2019) showed how it was possible to easily hack vision-based end-to-end AD technology.
At the same time, all those advantages could have their drawback counterparts:
• AD vehicles would likely be inaccessible to most people when introduced, likely costing over $100,000. A study from Kolarova et al. (2019) showed that the perceived value of travel-time savings using AVs is lower than conventional vehicles apart from shared AVs.
• Truck drivers and taxi drivers may lose their jobs, as AVs would take over even if new services and new work will be created: communications specialists, IT technicians, electrical engineers, mechatronics, analysts, AD engineers, modeling and simulation specialists, researchers, software programmers, mechanics and maintenance, cyber protection. Those issues are discussed in Pettigrew et al. (2018). Their conclusions are that there is a need for planning in advance required changes within the society and all companies should be prepared for the AV revolution (Alonso et al., 2020),
• If the vehicle crashes without a driver who would then be the responsible party: the software designer, the car maker, the OEM, the infrastructure (local authorities), or the owner of the vehicle?
• The cars would rely on the collection of location and user information, creating major privacy concerns. The principles of data avoidance and data economy need to be balanced between the requirements of data protection, cyber-security, road safety, and the competitiveness in global value-added chains. The implementation of different modes of CAD therefore requires reasonable solutions in the areas of processing and exploitation of data. The interoperability among C-ITS deployments around the world will also need to be ensured. In this context, the goals of data protection and promotion of innovations do not necessarily contradict each other but may jointly create an added value.
• Ethical problems could arise that a machine might struggle to deal with. Faced with a choice between plowing into a group of schoolchildren or going off a bridge and killing all its passengers, what does the vehicle do? Should the vehicle always serve to avoid animals on the road or always prioritize the safety and comfort of passengers? Here, a study using the moral machine of the MIT (Awad et al., 2018, https://www.moralmachine.net/) gave us some insight into what people think about this issue. However, this automated decision system issue is still debated.
• Human behavior such as hand signals are difficult for a computer to understand. What will then be the future interactions between humans, especially vulnerable road users, and AVs?
• How would the police interact with AD vehicles, especially in the case of accidents or crimes? Here, another dimension of the liability issues is highlighted and needs to be addressed.
All these remarks and questions have been taken into account in the first international proposal for CAV deployment rules (committee, 2020). This regulatory text has been adopted in June 2020. Nevertheless, these first rules from the United Nations are very cautious. The UN clearly wants to control with a high level of safety the deployment of these new mobility means. The UN confirms these first rules, and this first regulation document are just an introduction. In the future, this regulatory text will be adapted in order to respond properly to new needs. The main adopted rules are the following:
• A level 3 of AD can be activated as soon as a human driver takes place in the driver's seat with seat belt fastened.
• The UN prohibits the use of embedded automated systems on roads where pedestrians and cyclists are around AV. At this moment, the mixing of traffic with vulnerable users is forbidden.
• It is also forbidden to use AD system on a road that does not contain a physical separation between the two traffic lane directions. This rule will imply specific infrastructure adaptation.
• A restricted speed is adopted. The CAV maximum speed will be fixed to 60 km/h. It is too low for Highway use (in France, given a normal traffic density, the minimum speed is 80 km/h). This rule will imply adapted infrastructure for CAV use.
• The on-board multimedia screens must be disconnected when the driver regains vehicle control.
• It is necessary to install additional sensors in the AD system to monitor the driver's behavior and check his ability to get back the driving task (acting on actuators). This rule is critical and appropriate Human/Machine Interactions should be developed.
• It is necessary to equip the vehicle with a "black box," called "data storage system for AD," which is started automatically when AD is activated.
• AD systems must keep up to date on cyber-security aspects to avoid hacking. Same question about the drawback, failures, and fault detection, identification, and potential correction/mitigation.
6. Conclusion
The development and deployment of automated mobility are complex issues that cannot be done without using communication. However, there are still many questions and constraints to address in order to obtain an optimized, reliable, and robust medium for its use in active and automated mobility interacting with its environment. Various questions arise, such as the transmission range, the loss rate, the induced delays, the interference, and security issues. There are also questions about strategies to be implemented to manage and transmit information while guaranteeing a High Quality of Service. With the deployment of 5G (Brahmi et al., 2019), even if it will increase the level of security and speed, there is the problem of the effective transmission range.
In Lee et al. (2016), the authors define the following concept: Internet of Vehicles. The authors start from the notion of Intelligent Grid and apply this concept to the AV. In this case they define the Vehicular "Fogs" concept. By fogs, they mean an extension of cloud concept. The authors address the Internet of vehicles, the vehicular cloud, the fog computing, and the vehicular network for CAVs. This research and concepts proposal address the issue of the efficient management and Quality of Service of these distributed, cooperative, and connected moving and highly dynamic network of CAVs.
The development of the new active and connected mobility needs the management of a great quantity of information with various properties and constraints. Effectively, this information will be imperfect (uncertain, inaccurate, unreliable, and unconfident), incomplete, asynchronous, multi-scale, and multi-modal. Moreover, the processing stages will be distributed and cooperative. The information sources will be also different and will address different frequency domains. It will be relevant to detect and manage conflict and ambiguities. To avoid crashes, these systems of systems will have to guarantee the quality, the robustness and the reliability of information and sub systems. An additional level of processing will thus be necessary in order to monitor the reliability and the current operating state of embedded and distributed systems.
To understand the current and future complex and interactive environments, it will be essential and critical to build local and extended dynamic perception maps. These dynamic perception maps will have to assess the main KCs representing the road environment. It is obvious that, to guarantee a high level of information, perception, and service quality, the infrastructures will have to be reworked and redesigned to be smarter and more adapted to the CAVs.
In summary, the next generation of CCAM means will have to meet the challenge to handle, manage, filter, analyze, and interpret a huge quantity of data in order to understand and to anticipate both the KCs behaviors and the causes of singular events (collision, near accident, etc.).
Until the human stays in the driving loop, a Driver Monitoring System is necessary. The L3 and L4 systems can be seen as adaptive ADAS. These active ADAS must manage interdependent issues: safety, energy consumption and pollution, traffic management, comfort, and health.
The environment is the interaction and the cooperation of several independent worlds inside and outside the vehicle but with constraints. In this framework, the next generation of road networks will be essential for an efficient deployment of automated and connected mobility. It is now critical to take into account not only the user but also the surrounding with a real redesign of the road infrastructures and services. Increasing amounts of studies and concepts are proposed on these topics: the "readiness" (ISAD levels), "resilient," "smart," "automated," and "hybrid" road infrastructures. An interesting overview of this topic is given by Intel in an e-book (Sehra and Staff, 2020), which addresses the question of "how city and transportation leaders can develop Smart City strategies for improving road infrastructure." In this context, infrastructures will have to be adapted in order to be ready, readable, and cooperative in all context and weather conditions. The communication will play a crucial role.
But in all the possible solutions, information and data stay the main issue and the core of the new generation of transportation means. It is essential to address them and to know how to analyze, model, merge, use, and manage them. These different levels of processing will allow answers to be provided with more efficiency to tackle the challenges of tomorrow: an interactive, cooperative, active, optimal, reliable, and robust mobility for and between cities and for and with users, for a smart, responsible, and resilient world.
Author Contributions
All authors listed have made a substantial, direct and intellectual contribution to the work, and approved it for publication.
Funding
This paper is supported by European Union's Horizon 2020 research and innovation programme under grant agreement No. 815003, project Trustonomy (Building Acceptance and Trust in automated Mobility).
Conflict of Interest
The authors declare that the research was conducted in the absence of any commercial or financial relationships that could be construed as a potential conflict of interest.
References
Alnassera, A., Sun, H., and Jiang, J. (2019). Cyber security challenges and solutions for v2x communications: a survey. Comput. Netw. 151, 52–67. doi: 10.1016/j.comnet.2018.12.018
Alonso, E., Arpon, C., Gonzalez, M., Fernandez, R. Á., and Nieto, M. (2020). Economic impact of autonomous vehicles in Spain. Eur. Transp. Res. Rev. 12, 1–17. doi: 10.1186/s12544-020-00452-4
ARM (2019). “Accelerating autonomous vehicle technology. what challenges do we need to consider for the safe deployment of autonomous vehicles at scale?,” in IEEE Spectrum. Available online at: https://spectrum.ieee.org/transportation/self-driving/accelerating-autonomous-vehicle-technology
Asfour, S., Bernardin, F., Toussaint, E. T., and Piau, J.-M. (2016). Hydrothermal modeling of porous pavement for its surface de-freezing. applied thermal engineering. Appl. Therm. Eng. 107, 493–500. doi: 10.1016/j.applthermaleng.2016.06.138
ATKINS (2016). Research on the Impacts of Connected and Autonomous Vehicles (cavs) on Traffic Flow. Summary Report.
Awad, E., Dsouza, S., Kim, R., Schulz, J., Henrich, J., Shariff, A., et al. (2018). The moral machine experiment. Nature 563, 59–64. doi: 10.1038/s41586-018-0637-6
Barriera, M., Pouget, S., Lebental, B., and Van Rompu, J. (2020). In situ pavement monitoring: a review. Infrastructures 5:18. doi: 10.3390/infrastructures5020018
Baxter, J. A., Merced, D. A., Costinett, D. J., Tolbert, L. M., and Ozpineci, B. (2018). “Review of electrical architectures and power requirements for automated vehicles,” in 2018 IEEE Transportation Electrification Conference and Expo (ITEC)(Long Beach, CA: US Department of Energy (DOE)).
Benson, A. J., Tefft, B. C., Svancara, A. M., and Horrey, W. J. (2018). Potential Reductions in Crashes, Injuries, and Deaths from Large-Scale Deployment of Advanced Driver Assistance Systems (Research Brief). Washington, DC: AAA Foundation for Traffic Safety.
Besnier, J., Ta, V.-B., Ehrlich, J., and Simon, L. (2014). “Score@f project: a new usage of datex II in cooperative systems,” in Transport Research Arena (TRA) (Paris).
Blanc, J., Chailleux, E., Hornych, P., Williams, R. C., Presti, D. L., Carrion, A. J. D. B., et al. (2019). Bio materials with reclaimed asphalt: from lab mixes properties to non-damaged full scale monitoring and mechanical simulation. Road Mater. Pavement Design 20(Suppl. 1), S95–S111. doi: 10.1080/14680629.2019.1589557
Boloor, A., Garimella, K., He, X., Gill, C. M., Vorobeychik, Y., and Zhang, X. (2019). Attacking vision-based perception in end-to-end autonomous driving models. ArXiv abs/1910.01907. doi: 10.1016/j.sysarc.2020.101766
Brahmi, N., Do, H., Fodor, G., Sun, W., Sorrentino, S., Brännström, F., et al. (2019). Fifth Generation Communication Automotive Research and Innovation. Final 5g v2x Radio Design. Deliverable D3.3.
Brummelen, J. V., O'Brien, M., Gruyer, D., and Najjaran, H. (2018). Autonomous vehicle perception: the technology of today and tomorrow. Transp. Res. Part C Emerg. Technol. 89, 384–406. doi: 10.1016/j.trc.2018.02.012
Carreras, A., Daura, X., Erhart, J., and Ruehrup, S. (2018). “Road infrastructure support levels for automated driving,” in 25th ITS World Congress (Copenhagen).
Coelho, M. C., and Guarnaccia, C. (2020). Driving information in a transition to a connected and autonomous vehicle environment: impacts on pollutants, noise and safety. Transp. Res. Proc. 45, 740–746. doi: 10.1016/j.trpro.2020.02.103
Condliffe, J. (2017). A single autonomous car has a huge impact on alleviating traffic even intelligent cruise control systems could be used to clear up congestion. MIT Technology review. Available online at: https://www.technologyreview.com/s/607841/a-single-autonomous-car-has-a-huge-impact-on-alleviating-traffic/
Cummings, M. (2014). Man versus machine or man + machine? IEEE Intell. Syst. 29, 62–69. doi: 10.1109/MIS.2014.87
de Larrard, F., Sedran, T., and Balay, J.-M. (2013). Removable urban pavements: an innovative, sustainable technology. Int. J. Pavement Eng. 14, 1–11. doi: 10.1080/10298436.2011.634912
de Winter, J., and Dodou, D. (2014). Why the fitts list has persisted throughout the history of function allocation. Cogn. Technol. Work 16, 1–11. doi: 10.1007/s10111-011-0188-1
Demmel, S. (2012). Construction de cartes étendues des difficultés de la route fondée sur la fusion de cartes locales (Cotutelle Ph. D. thesis), QUT CARRS-Q, Brisbane, QLD, Australia; University Versailles Saint Quentin, Versailles, France.
Demmel, S., Lambert, A., Gruyer, D., Larue, G., and Rakotonirainy, A. (2014). Ieee 802.11p empirical performance model from evaluations on test tracks. J. Netw. 17, 1485–1495. doi: 10.4304/jnw.9.6.1485-1495
Dixit, V., Chand, S., and Nait, D. (2016). Autonomous vehicles: disengagements, accidents and reaction times. PLoS ONE 11:e0168054. doi: 10.1371/journal.pone.0168054
Dixon, L. (2020). Autonowashing: the greenwashing of vehicle automation. Transp. Res. Interdiscipl. Perspect. 5:100113. doi: 10.1016/j.trip.2020.100113
Dunn, N., Dingus, T., and Soccolich, S. (2019). Understanding the Impact of Technology: Do Advanced Driver Assistance and Semi-Automated Vehicle Systems Lead to Improper Driving Behavior? Washington, DC: AAA Foundation for Traffic Safety; Virginia Tech Transportation Institute.
Ehrlich, J. (2017a). Connected vehicles, floating car data and self-driving vehicles. Routes Roads Magaz. 373, 21–22.
Ehrlich, J. (2017b). Infrastructure requirements for the self-driving vehicle. Routes Roads Magaz. 373, 43–46.
Ehrlich, J., Gruyer, D., Orfila, O., and Hautière, N. (2016). “Autonomous vehicle: the concept of high quality of service highway,” in Conference FISITA 2016 (Busan).
eIMPACT project (2008). Final Report and Integration of Results and Perspectives for Market Introduction of Ivss. Deliverable D10 (incl. D9). eIMPACT (Assessing the Impacts of Intelligent Vehicle Safety Systems) Socio-economic Impact Assessment of Stand-alone and Co-operative Intelligent Vehicle Safety Systems (IVSS) in Europe.
European Commission (2019). “Road safety in the European Union. Trends, statistics and main challenges, April 2018,” in 26th International Technical Conference on the Enhanced Safety of Vehicles (Eindhoven).
Fagnant, D. J., and Kockelman, K. (2015). Preparing a nation for autonomous vehicles: opportunities, barriers and policy recommendations. Transp. Res. Part A 77, 167–181. doi: 10.1016/j.tra.2015.04.003
Favarò, F., Eurich, S. O., and Nader, N. (2018). Autonomous vehicles' disengagements: trends, triggers, and regulatory limitations. Accid. Anal. Prev. 110, 136–148. doi: 10.1016/j.aap.2017.11.001
Favarò, F., Nader, N., Eurich, S. O., and Varadaraju, N. (2017). Examining accident reports involving autonomous vehicles in California. PLoS ONE 12:e0184952. doi: 10.1371/journal.pone.0184952
Fildes, B., Keall, M., Bos, N., Lie, A., Page, Y., Pastor, C., et al. (2015). Effectiveness of low speed autonomous emergency braking in real-world rear-end crashes. Accid. Anal. Prev. 81, 24–29. doi: 10.1016/j.aap.2015.03.029
Fontaras, G., Zacharof, N.-G., and Ciuffo, B. (2017). Fuel consumption and Co2 emissions from passenger cars in Europe - laboratory versus real-world emissions. Prog. Energy Combust. Sci. 60, 97–131. doi: 10.1016/j.pecs.2016.12.004
Freeman, C., and Soete, L. (1990). Fast structural change and slow productivity change: some paradoxes in the economics of information technology. Struct. Change Econ. Dyn. 1, 225–242. doi: 10.1016/0954-349X(90)90003-Q
Freitas, C. (2016). Bio inspired decarbonized vehicle concepts using CK theory (Ph.D. thesis). University Paris Saclay, Evry, France.
Friedrich, B. (2016). The Effect of Autonomous Vehicles on Traffic. Berlin; Heidelberg: Springer, 317–334.
Gartner (2019). Gartner Hype Cycle for Emerging Technologies. Web site Gartner. Available online at: https://www.gartner.com/SmarterWithGartner/
Gasser, T. A. (2014). “Vehicle automation: definitions, legal aspects, research needs,” in UNECE-Workshop: Towards a New Transportation Culture: Technology Innovations for Safe, Efficient and Sustainable Mobility (Brussels).
Gasser, T. A., and Westhoff, D. (2012). “Bast-study: definitions of automation and legal issues in Germany,” in TRB (Transportation Research Board, Road Vehicle Automation Workshop(Washington, DC).
Gasser, T. M. (2016). “Fundamental and special legal questions for autonomous vehicles,” in Autonomous Driving, Technical, Legal and Social Aspects, M. Maurer, J. Christian Gerdes, B. Lenz, and H. Winner (SpringerOpen), 523–551.
Giang, A. T., Busson, A., Lambert, A., and Gruyer, D. (2015). “Spatial capacity of ieee 802.11p based vanet: models, simulations and experimentations,” in IEEE Transaction on Vehicular Technology, 1115–1126.
Gong, S., and Du, L. (2018). Cooperative platoon control for a mixed traffic flow including human drive vehicles and connected and autonomous vehicles. Transp. Res. Part B Methodol. 116, 25–61. doi: 10.1016/j.trb.2018.07.005
Gruel, W., and Stanford, J. M. (2016). Assessing the long-term effects of autonomous vehicles: a speculative approach. Transp. Res. Proc. 13, 18–29. doi: 10.1016/j.trpro.2016.05.003
Gruyer, D., Demmel, S., d'Andréa-Novel, B., Larue, G., and Rakotonirainy, A. (2013). Simulating cooperative systems applications: a new complete architecture. Int. J. Adv. Comp. Sci. Appl. 4, 171–180. doi: 10.14569/IJACSA.2013.041226
Gruyer, D., Magnier, V., Hamdi, K., Claussmann, L., Orfila, O., and Rakotonirainy, A. (2017). Perception, information processing and modeling: critical stages for autonomous driving applications. Annu. Rev. Control 44, 323–341. doi: 10.1016/j.arcontrol.2017.09.012
Gupta, B. B., Perez, G. M., Agrawal, D. P., and Gupta, D. (2020). Handbook of Computer Networks and Cyber Security: Principles and Paradigm. Edition Springer Nature Switzerland. doi: 10.1007/978-3-030-22277-2
Hautière, N., Aniss, H., Dumoulin, J., Chailleux, E., Hornych, P., and Laporte, S. (2017). Innovative road-building technologies dedicated to the innovative road. Routes Roads 374, 19–24.
Hautière, N., and Bourquin, F. (2017). “Instrumentation and monitoring of the next gen roadinfrastructure: some results and perspectives from the R5G project [abstract],” in Proceedings of the 19th European Geosciences Union General Assembly (Vienna), 18874.
Hörl, S., Ciari, F., and Axhausen, K. (2016). Recent perspectives on the impact of autonomous vehicles. Semantic Scholar. doi: 10.3929/ETHZ-B-000121359
Hsu, J. (2017). When it comes to safety, autonomous cars are still 'teen drivers'. Scientific American.
ITF/OECD (2018). Safer Roads With Automated Vehicles? Corporate Partnership Board Report. Report draws conclusions from the Workshop “Safety and Security on the Road to Automated Transport: The Good, the Uncertain and the Necessary” held in November 2017 in Paris, France.
Kaur, N., and Kad, S. (2015). A review on security related aspects in vehicular ad-hoc networks. Proc. Comput. Sci. 78, 387–394. doi: 10.1016/j.procs.2016.02.079
Kolarova, V., Steck, F., and Bahamonde-Birke, F. J. (2019). Assessing the effect of autonomous driving on value of travel time savings: a comparison between current and future preferences. Transp. Res. Part A Policy Pract. 129, 155–169. doi: 10.1016/j.tra.2019.08.011
Konstantinopoulou, L., Coda, A., and Schmidt, F. (2019). “Specifications for multi-brand truck platooning,” in ICWIM8, 8th International Conference on Weigh-In-Motion (Prague).
Lamb, M., Collis, R., Deix, S., Krieger, B., and Hautière, N. (2012). The forever open road: defining the next generation road. Routes Roads 352–353, 120–129.
Lammert, M., Duran, A., Diez, J., Burton, K., and Nicholson, A. (2014). Effect of platooning on fuel consumption of class 8 vehicles over a range of speeds, following distances, and mass. SAE Int. J. Commerc. Vehicles 7, 626–639. doi: 10.4271/2014-01-2438
Laporte, S., Coquery, G., Deniau, V., Bernardinis, A. D., and Hautière, N. (2019). Dynamic wireless power transfer charging infrastructure for future evs: from experimental track to real circulated roads demonstrations. World Electr. Vehicle J. 10:84. doi: 10.3390/wevj10040084
Latrech, C., Chaibet, A., Boukhnifer, M., and Glaser, S. (2018). Integrated longitudinal and lateral networked control system design for vehicle platooning. Sensors 18:3085. doi: 10.3390/s18093085
Le Pivert, M., Poupart, R., Capochichi-Gnambodoe, M., Martin, N., and Leprince-Wang, Y. (2019). Direct growth of zno nanowires on civil engineering materials: smart materials for supported photodegradation. Microsyst. Nanoeng. 5, 1–7. doi: 10.1038/s41378-019-0102-1
Lee, E.-K., Gerla, M., Pau, G., Lee, U., and Lim, J.-H. (2016). Internet of vehicles: from intelligent grid to autonomous cars and vehicular fogs. Int. J. Distrib. Sensor Netw. 12, 1–14. doi: 10.1177/1550147716665500
Li, G., Baker, S., Grabowski, J., and Rebok, G. (2001). Factors associated with pilot error in aviation crashes. Aviat. Space Environ. Med. 72, 52–58. Available online at: https://www.researchgate.net/publication/12136068_Factors_associated_with_pilot_error_in_aviation_crashes
Litman, T. A. (2020). Autonomous Vehicle Implementation Predictions: Implications for Transport Planning. Victoria, BC: Victoria Transport Policy Institute.
Lu, Q., Tettamanti, T., Hörcher, D., and Varga, I. (2019). The impact of autonomous vehicles on urban traffic network capacity: an experimental analysis by microscopic traffic simulation. Transp. Lett. 12, 540–549. doi: 10.1080/19427867.2019.1662561
Lu, Z., Coster, X., and de Winter, J. (2017). How much time do drivers need to obtain situation awareness? A laboratory-based study of automated driving. Appl. Ergon. 70, 293–304. doi: 10.1016/j.apergo.2016.12.003
Makridis, M., Mattas, K., Mogno, C., Ciuffo, B., and Fontaras, G. (2020). The impact of automation and connectivity on traffic flow and Co2 emissions. A detailed micro simulation study. Atmos. Environ. 226:117399. doi: 10.1016/j.atmosenv.2020.117399
Melcher, V., Rauh, S., Diederichs, F., Widlroither, H., and Bauer, W. (2015). “Takeover requests for automated driving,” in 6th International Conference on Applied Human Factors and Ergonomics (AHFE 2015) and the Affiliated Conferences, AHFE 2015, Procedia Manufacturing, Vol. 3 (Las Vegas, NV), 2867–2873.
Mersky, A., and Samaras, C. (2016). Fuel economy testing of autonomous vehicles. Transp. Res. Part C: Emerg. Technol. 65, 31–48. doi: 10.1016/j.trc.2016.01.001
Mohsen, I., Houdali, N., Ditchi, T., Holé, S., and Géron, E. (2018). V2i electromagnetic system for lateral position estimation of a vehicle. Sensors Actuat. A Phys. 274, 141–147. doi: 10.1016/j.sna.2018.01.020
Mokhtar, B., and Azab, M. (2015). Survey on security issues in vehicular ad-hoc networks. Alexandria Eng. J. 54, 1115–1126. doi: 10.1016/j.aej.2015.07.011
Morando, M. M., Tian, Q., Truong, L. T., and Vu, H. L. (2018). Studying the safety impact of autonomous vehicles using simulation-based surrogate safety measures. J. Adv. Transp. 2018, 1–11. doi: 10.1155/2018/6135183
Muoio, D. (2017). Self-Driving Cars Could Be Terrible for Traffic - Here's Why? Available online at: https://www.businessinsider.in/self-driving-cars-could-be-terrible-for-traffic-heres-why/articleshow/58988161.cms (accessed June, 2017)
Muratori, M., Holden, J., Lammert, M., Duran, A., Young, S., and Gonder, J. (2017). “Potentials for platooning in U.S. highway freight transport,” in SAE World Congress Experience Detroit (Detroit, MI).
NHTSA (2015). Critical Reasons for Crashes Investigated in the National Motor Vehicle Crash Causation Survey. Report, Traffic Safety Facts. Crash & Stats, DOT HS 812 115. US Department of Transportation.
Noy, I. Y., Shinar, D., and Horrey, W. J. (2018). Automated driving: safety blind spots. Saf. Sci. 102, 68–78. doi: 10.1016/j.ssci.2017.07.018
Penttinen, M., van der Tuin, M., Farah, H., de Almeida Correia, G. H., Carsten, Z. W. O., and Kulmala, R. (2019). Impacts of Connected and Automated Vehicles — State of the Art. Deliverable eliverable D3.1, H2020 project MANTRA.
Pettigrew, S., Fritschi, L., and Norman, R. (2018). The potential implications of autonomous vehicles in and around the workplace. Int. J. Environ. Res. Public Health 15:1876. doi: 10.3390/ijerph15091876
Pink, S., Osz, K., Raats, K., Lindgren, T., and Fors, V. (2020). Design anthropology for emerging technologies: trust and sharing in autonomous driving futures. Design Stud 69:100942. doi: 10.1016/j.destud.2020.04.002
Russell, S., Blanco, M., Atwood, J., Schaudt, W., Fitchett, V., and Tidwell, S. (2018). Naturalistic Study of Level 2 Driving Automation Functions (report no. dot hs 812 642). Washington, DC: National Highway Traffic Safety Administration.
SAE (2016). SAE International Releases Updated Visual Chart for Its “Levels of Driving Automation?” Standard for Self-Driving Vehicles.
SAE (2018). Taxonomy and Definitions for Terms Related to Driving Automation Systems for On-road Motor Vehicles. Web site SAE International, Technical Report. Available online at: https://www.sae.org/standards/content/j3016_201806/
Schoettle, B. (2017). Sensor Fusion: A Comparison of Sensing Capabilities of Human Drivers and Highly Automated Vehicles. Report No. SWT-2017-12.
Schoettle, B., and Sivak, M. (2015). A Preliminary Analysis of Real-World Crashes Involving Self-Driving Vehicles. UMTRI Report, 3.
Schwab, A., and Lunze, J. (2019). Vehicle platooning and cooperative merging. IFAC Pap. Online 52, 353–358. doi: 10.1016/j.ifacol.2019.09.057
Sehra, S. A., and Staff, I. (2020). Paving the way Forward: Intelligent Road Infrastructure. IoT Smart Cities and Transportation, Intel Corporation.
Shahar, A., Brémond, R., and Villa, C. (2018). Can led-based road studs improve vehicle control in curves at night? a driving simulator study. Light. Res. Technol. 50, 266–281. doi: 10.1177/1477153516660146
Smith, S. W., Kim, Y., Guanetti, J., Kurzhanskiy, A. A., Arcak, M., and Borrelli, F. (2019). “Balancing safety and traffic throughput in cooperative vehicle platooning,” in 18th European Control Conference (ECC 2019) (Napoli).
Spence, J. C., Kim, Y.-B., Lamboglia, C. G., Lindeman, C., Mangan, A. J., McCurdy, A. P., et al. (2020). Potential impact of autonomous vehicles on movement behavior: a scoping review. Am. J. Prev. Med. 58, e191–e199. doi: 10.1016/j.amepre.2020.01.010
Stern, R. E., Cui, S., Monache, M. L. D., Bhadani, R., Bunting, M., Churchill, M., et al. (2018). Dissipation of stop-and-go waves via control of autonomous vehicles: field experiments. Transp. Res. Part C Emerg. Technol. 89, 205–221. doi: 10.1016/j.trc.2018.02.005
Tesla (2020). Tesla Vehicle Safety Report, q3. Available online at: https://www.tesla.com/VehicleSafetyReport
Touz, N. L., and Dumoulin, J. (2015). “Numerical study of the thermal behavior of a new deicing road structure design with energy harvesting capabilities,” in EGU General Assembly 2015 (Vienna).
Tschudi, Y., and Girardin, G. (2018). “Road to robots sensors and computing for autonomous vehicle,” in Automotive vehicle sensors conference 2018 (San Jose, CA).
Tsugawa, S., Jeschke, S., and Shladover, S. (2016). A review of truck platooning projects for energy savings. IEEE Trans. Intell. Vehicles 1, 68–77. doi: 10.1109/TIV.2016.2577499
United Nations Committee (2020). “Consideration of proposals for new un regulations submitted by the working parties subsidiary to the world forum proposal for a new un regulation on uniform provisions concerning the approval of vehicles with regards to automated lane keeping system,” in World Forum for Harmonization of Vehicle Regulations (Geneva: Economic Commission for Europe).
Vahidi, A., and Sciarretta, A. (2018). Energy saving potentials of connected and automated vehicles. Transp. Res. Part C Emerg. Technol. 95, 822–843. doi: 10.1016/j.trc.2018.09.001
Verspagen, B. (2001). “Economic growth and technological change: an evolutionary interpretation,” in Working Papers from OECD on Science, Technology and Industry (Paris: OECD).
Vizzari, D., Chailleux, E., Lavaud, S., Gennesseaux, E., and Bouron, S. (2020). Fraction factorial design of a novel semi-transparent layer for applications on solar roads. Infrastructures 5:5. doi: 10.3390/infrastructures5010005
Von Arnim, A., Arief, B., and Fusee, A. (2008). “Cooperative road sign and traffic light using near infrared identification and zigbee smartdust,” in 15th World Congress on Intelligent Transport Systems and ITS America's 2008. (New York, NY).
Webb, N., Smith, D., Ludwick, C., Victor, T., Hommes, Q., Favaro, F., et al. (2020). Waymo's safety methodologies and safety readiness determinations. Waymo. Available online at: https://arxiv.org/abs/2011.00054
Wickens, C., Gordon-Becker, S., Liu, Y., and Lee, J. (2003). An Introduction to Human Factors Engineering, 2nd Edn. eds B. Gupta, G. M. Perez, D. P. Agrawal, and D. Gupta. (Pearson Prentice-Hall, Inc. Division of Simon and Schuster One Lake Street Upper Saddle River, NJ).
Will, D., Gronerth, P., Steven von Bargen, F. B. L., and Larini, G. (2017). Report on the State of the Art of Connected and Automated Driving in Europe (Final). European project SCOUT Deliverable D3.2.
Workshop), A. P. U. (2018). Impacts and Potential Benefits of Autonomous Vehicles: From an International Context to Grand Paris. APUR Report.
Zhang, B., de Winter, J., Varotto, S., Happee, R., and Martens, M. (2019). Determinants of take-over time from automated driving: a meta-analysis of 129 studies. Transp. Res. Part F Traffic Psychol. Behav. 64, 285–307. doi: 10.1016/j.trf.2019.04.020
Zhang, Y., and Cassandras, C. G. (2019). An impact study of integrating connected automated vehicles with conventional traffic. Annu. Rev. Control 48, 347–356. doi: 10.1016/j.arcontrol.2019.04.009
Keywords: connected and automated vehicles (CAV), road safety, environment perception, traffic, communication technology, smart infrastructure, sensors technologies, impact of CAV
Citation: Gruyer D, Orfila O, Glaser S, Hedhli A, Hautière N and Rakotonirainy A (2021) Are Connected and Automated Vehicles the Silver Bullet for Future Transportation Challenges? Benefits and Weaknesses on Safety, Consumption, and Traffic Congestion. Front. Sustain. Cities 2:607054. doi: 10.3389/frsc.2020.607054
Received: 16 September 2020; Accepted: 17 November 2020;
Published: 08 January 2021.
Edited by:
Krzysztof Goniewicz, Military University of Aviation, PolandReviewed by:
Witold Pawłowski, Medical University of Lublin, PolandFrancisco Alonso, University of Valencia, Spain
Copyright © 2021 Gruyer, Orfila, Glaser, Hedhli, Hautière and Rakotonirainy. This is an open-access article distributed under the terms of the Creative Commons Attribution License (CC BY). The use, distribution or reproduction in other forums is permitted, provided the original author(s) and the copyright owner(s) are credited and that the original publication in this journal is cited, in accordance with accepted academic practice. No use, distribution or reproduction is permitted which does not comply with these terms.
*Correspondence: Dominique Gruyer, dominique.gruyer@univ-eiffel.fr